- 1Institute for Atmospheric and Climate Science, ETH Zurich, Zurich, Switzerland
- 2Wyss Academy for Nature, Climate and Environmental Physics, Oeschger Centre for Climate Change Research, University of Bern, Bern, Switzerland
- 3CENSAM, Singapore-MIT Alliance for Research and Technology (SMART), Singapore, Singapore
- 4TUMCREATES, Singapore, Singapore
- 5Geography Department, Humboldt University of Berlin, Berlin, Germany
On top of their well known positive impact on air quality and CO2 emissions, electric vehicles generate less exhaust heat compared to traditional vehicles thanks to their high engine efficiency. As such, electric vehicles have the potential to mitigate the excessive heat in urban areas—a problem which has been exacerbated due to urbanisation and climate change. Still, the heat mitigation potential of electric vehicles has not been fully understood. Here, we combine high-resolution traffic heat emission inventories with an urban climate model to simulate the impact of the fleet electrification to the near-surface air temperature in the tropical city of Singapore. We show that a full replacement of traditional internal combustion engine vehicles with electric vehicles reduces the near-surface air temperature by up to 0.6°C. The heat mitigation potential is highest during the morning traffic peak and over areas with the largest traffic density. Interestingly, the reduction in exhaust heat emissions due to the fleet electrification during the evening traffic peak hardly leads to a reduction of near-surface air-temperatures, which is attributed to the different atmospheric conditions during morning and evening. This study presents a new quantification of the city-wide impact of electric vehicles on the air temperature in a tropical urban area. The results may support policy-makers toward designing holistic solutions to address the challenge of climate change adaptation and mitigation in cities.
1 Introduction
The combined effect of climate change and the urban heat island challenges the living conditions in cites, with consequences on human health, the economy and ecosystems (Revi et al., 2014). Cities are asked to take an active role in mitigating their contribution to global warming and associated climate change by reducing their CO2 emissions (Hsu et al., 2020). At the same time, they need to implement local measures to adapt to warmer conditions (hereinafter heat mitigation), which are inevitable even with the most optimistic CO2 emission pathways (IPCC, 2014).
Heat mitigation in cities involves interventions in one of the following aspects, which are the main drivers of the urban heat island: urban materials, urban form, vegetation and anthropogenic heat (Oke et al., 2017). Anthropogenic heat emissions are produced by building heating, ventilation and cooling (HVAC), vehicular traffic and human metabolism (Sailor, 2011; Sun et al., 2018). Several studies have been performed on the impact of measures on HVAC on the air temperature in urban areas (Salamanca et al., 2014; Takane et al., 2019; Jin et al., 2020). By contrast, the urban climate impact of measures on vehicular traffic is yet mostly unexplored (Zhu et al., 2017).
The vehicular market is experiencing a radical change, with a growing number of electric vehicles (EVs). The quickly increasing share of EVs can be attributed to technological advances and supportive policies that include financial incentives to buy them (IEA, 2020). Such policies are motivated by the long-recognised environmental benefits of EVs (Hardman et al., 2017) which include their positive impact on air quality (Liang et al., 2019) and CO2 emissions (Wolfram and Lutsey, 2016). Importantly, EVs are also expected to reduce the amount of exhaust heat released in the urban environment. The reduced exhaust heat in EVs compared to internal combustion engine (ICE) vehicles is due to the higher energy efficiency of EVs, i.e., the amount of energy which is transferred to the drive system. The energy efficiency of EVs is about 80–90%, while those of ICE vehicles is of about 13–20% (Muneer and García, 2017). Accordingly, a substantial fleet electrification (i.e., increasing share of EVs) is expected to reduce the amount of exhaust heat released by vehicles into the atmosphere. However, it is still unclear to what extent a reduced amount of exhaust heat from vehicles would translate into spatio-temporal changes in near-surface air temperature. Such knowledge is essential when assessing how an increased share of EVs could reduce the urban heat and its adverse impacts.
A first estimation of the heat mitigation potential of EVs has been derived by simply scaling the literature data on the contribution of traffic to the urban heat island intensity (Li et al., 2015). Using this approach, that study found that a full conversion to EVs could reduce the average urban heat island intensity by approx. 1°C (Li et al., 2015). Although the approach is interesting, the main problem with this estimation is that surface and canopy-layer urban heat island intensity data have been mixed. Given that the surface temperature shows much larger variability than the canopy-layer air temperature (Oke et al., 2017), these results are likely overestimated. Moreover, the authors did not investigate on spatial and temporal patterns of the impact.
Currently, the Cooling Singapore project (Cooling Singapore, 2021) is performing an in-depth investigation on the urban climate impact of vehicular traffic in the city-state of Singapore. A preliminary modelling study shows that vehicular traffic has a small but considerable warming effect in the urban area of Singapore (Singh et al., 2022). Employing the Weather Research and Forecasting (WRF) model (Skamarock et al., 2005; Chen et al., 2011), previous studies found that traffic contributes up to 1.1°C to near-surface air temperature and a full transition to EVs could produce a reduction up to 0.9°C. Further studies are needed to corroborate the results and to understand the mechanisms which drive the spatial and temporal variability of these effects.
In order to bridge this knowledge gap, this study investigates the impact of vehicular traffic on near-surface temperature using high-resolution urban climate modelling and high-resolution data on heat emissions from vehicular traffic. The heat mitigation induced by a full fleet electrification is quantified and compared to that of replacing individual air conditioning (AC) units with centralised AC units—another technological measures to reduce anthropogenic heat emissions in cities. The study focuses on the city-state island of Singapore. Located one degree north of the equator, the city-state island of Singapore experiences a tropical wet climate with abundant rainfall, high and uniform temperatures, and high humidity all year round. Singapore has the largest population density in the world and an abundant share of its territory (45%) is built-up (Yee et al., 2011; Gaw et al., 2019). It comes as no surprise that the anthropogenic heat is one of the largest contributor in the surface energy balance in Singapore. While buildings are responsible for the largest share (49–83%) of the total anthropogenic heat emission, exhaust heat from traffic makes up to one third in residential areas (Quah and Roth, 2012).
2 Materials and Methods
2.1 Model Set-Up
The simulations are performed using the regional climate model COSMO-CLM (Rockel et al., 2008), coupled with a multi-layer urban canopy model (Schubert et al., 2012). The model also includes a building energy model (Jin et al., 2021), which computes the anthropogenic heat generation due to indoor space cooling. A summary of the model setup is provided in the Supplementary Table S1. The simulations were performed for the period 01–30 April 2016 and the first 5 days were discarded as spin-up time. The model is applied using three nested domains with horizontal resolution of 0.1° (∼10 km), 0.02° (∼2 km) and 0.004° (∼400 m) (Supplementary Figure S1). Initial and boundary conditions are provided by the ERA5 reanalysis (Copernicus Climate Change Service, 2017), and used to drive the coarsest model domain. Land use information for the year 2016 are derived from ESA CCI land cover (ESA, 2017). The information regarding the urban area is derived using a combination of high-resolution datasets and parameters that were derived from literature. Specifically, the urban canopy parameters were generated using a 3D city model including all buildings in Singapore (courtesy of A*STAR, Singapore) and a high-resolution urban land cover map (Gaw et al., 2019). The datasets were transformed into model-ready urban canopy parameters using the python tool UCPgenerator (Mussetti, 2019; Mussetti et al., 2020). An overview of the most important urban canopy parameters is given in Supplementary Figure S2. Thermo-physical properties of urban materials follow the values proposed by previous studies (Demuzere et al., 2017), which are representative of a low-rise residential area in Singapore (Supplementary Table S2). It should be noted that this is a simplification, since material properties can vary between districts with different building functions and types.
The building energy model used a target indoor temperature of 25°C and a constant operation throughout the day. As a default, it was assumed that the exhaust heat due to indoor space cooling is released at the height of the corresponding floor (Jin et al., 2020). This model option corresponds to the case of individual (split) AC units for each floor. Further information on the AC set-up is given in Supplementary Table S3. An additional simulation has been performed (AC scenario) were it is assumed that 25% of the indoor space cooling uses centralised units with exhaust heat at roof level instead of default split units. The AC scenario is used in the study to represent an additional measure to reduce the (near-surface) exhaust heat emissions.
2.2 Traffic Heat Emissions
The sensible heat flux produced by vehicles (Qv) is computed using the agent-based traffic simulator CityMoS (TUM CREATE, 2020). CityMoS has been calibrated with trip duration data and validated against speed-band information (Ivanchev and Fonseca, 2020). The travel demand for a typical day is extracted from travel surveys and scaled up for the whole vehicle population using person expansion factors. The demand is used to perform traffic assignment which computes routes for all commuter trips. Based on those routes, congestion levels on every road are estimated and used together with road and vehicle parameters to determine the amount of fuel used by every vehicle on every road segment for different parts of the day (Ivanchev et al., 2020). Agent-based traffic simulations have the advantage to estimate congestion levels, which are hardly captured by traffic counts (Ivanchev et al., 2020). Congestion levels can significantly influence the fuel consumption and thus the amount of heat produced. The amount of heat produced is assumed to be equal to the amount of fuel used and is aggregated for each grid cell (approx. 400 m × 400 m) on an hourly basis. Further information regarding the assumptions on fuel consumption and how it varies with type of vehicle, type of engine and speed are provided in Ivanchev et al. (2020). In summary, CityMoS generates spatial and diurnal temporal distribution of Qv for a typical day (Figure 1). However, it should be noted that variations in traffic density between days in the week and between weekdays and weekend-days are not accounted for.
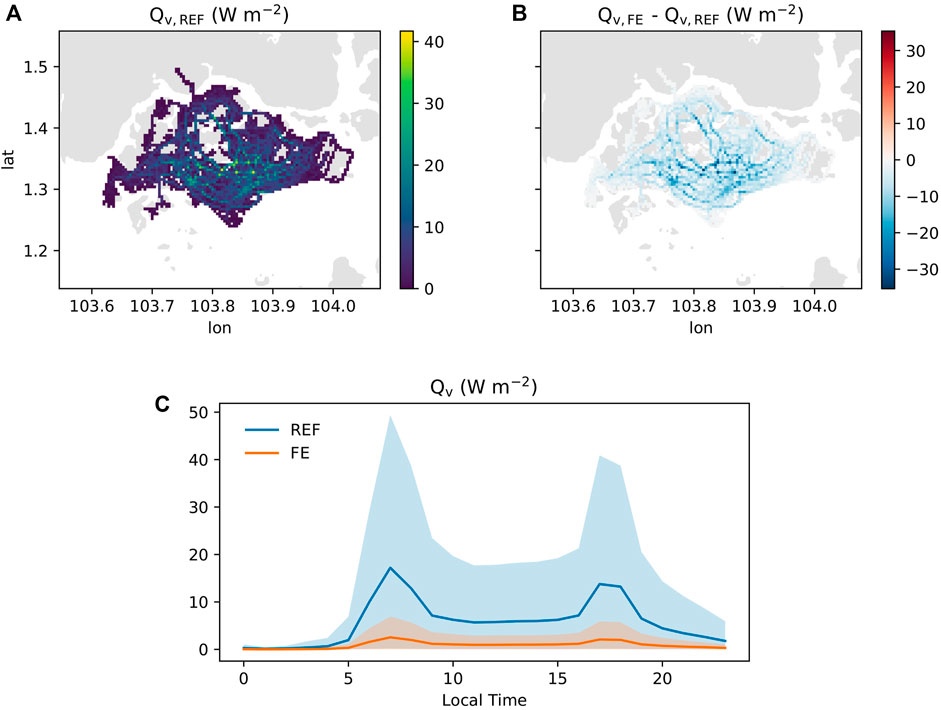
FIGURE 1. Sensible heat emissions from vehicles (Qv). (A) shows the spatial distribution of the daily-averaged Qv in the scenarios REF (current vehicles composition); (B) shows the difference between FE (full fleet electrification) and REF; (C) shows the diurnal evolution of Qv in the two scenarios, where solid lines and shaded areas show the mean value and the variability between 5th and 95th percentiles, respectively. The grey area in (A) and (B) represents land surfaces.
2.3 Implementation of Traffic Emissions Into Model
Sensible heat flux emission from traffic (Qv) is released at the lowest model level and generates an increment to the air temperature according to Eq. 1:
where
2.4 Model Evaluation
The model results are evaluated against observations of near-surface (2 m) air temperature (T) and 10-m wind speed (u). The evaluation is designed to support a specific model application, which quantifies the impact of heat emissions from traffic on air temperature. As such, this study does not aim to perform a comprehensive model evaluation, which may require using a larger variety of observations and atmospheric variables.
Hourly observations of T and u are provided by the Meteorological Service of Singapore (MSS). From the available sites, only those placed at ground level are used for the evaluation. Observations at roof level have been discarded as recommended by the WMO (Jarraud, 2008). The location of the selected sites is shown in Supplementary Figure S3. The model performance is evaluated against the observations using the following metrics: root-mean-square error (RMSE), mean bias error (MBE) and coefficient of determination (r2). When comparing model results with observations, it is important to keep in mind that the individual sites can be exposed to micro-climatic features. Thus, a specific site is not always representative of the model grid cell and caution is required when interpreting the results of the model evaluation.
The comparison between observed and modelled near-surface air temperature is shown in Supplementary Figure S4. The statistical scores are listed in Supplementary Table S4. The model is able to reproduce the day-to-day and diurnal variability of T in the majority of the sites. The difference in the diurnal profile of T between sites close to the coast (S102 and S108) and those slightly inland is well modelled. Quantitatively, the comparison indicates a very small MBE, which is found at most sites. Values of RMSE are moderately larger but still within the range of what has been reported in other urban climate modelling studies over Singapore (Mughal et al., 2019; Simón-Moral et al., 2020; Singh et al., 2022). The moderately large RMSE may be due to the strong weather variability associated with the tropical climate (e.g., cloud cover change and precipitation).
The comparison between observed and modelled near-surface wind speed is shown in Supplementary Figure S5. The statistical scores are listed in Supplementary Table S4. The model is able to reproduce very well both the day-to-day and diurnal variability of u. Importantly, the model simulates well the evolution of the land-sea breeze, which peaks in the afternoon. Quantitatively, the comparison indicates a very small mean bias error (average MBE = −0.16 ± 0.3 m s−1). Strong wind speed events are not always captured by the model, which results in a moderate RMSE (average RMSE = 1.19 ± 0.1 m s−1). Still, the agreement with observations is generally better than what has been reported in other urban climate modelling studies (Mughal et al., 2019).
3 Results
A full electrification of the fleet (FE) produces a reduction in near-surface air temperature compared to current fleet composition (REF) (ΔTFE = TFE − TREF, Figure 2). The daily-average ΔTFE is moderate and concentrated over the area with the largest traffic and urban density (max ΔTFE ∼−0.2°C). The reduction in the sensible heat emission from traffic (Qv, Figure 1) results in slight warming over areas with low traffic density. Secondary warming effects can be due to slight changes in thermally-driven circulation (e.g., sea-breeze) as well as changes in the local-scale urban heat advection (Bassett et al., 2016; Oke et al., 2017).
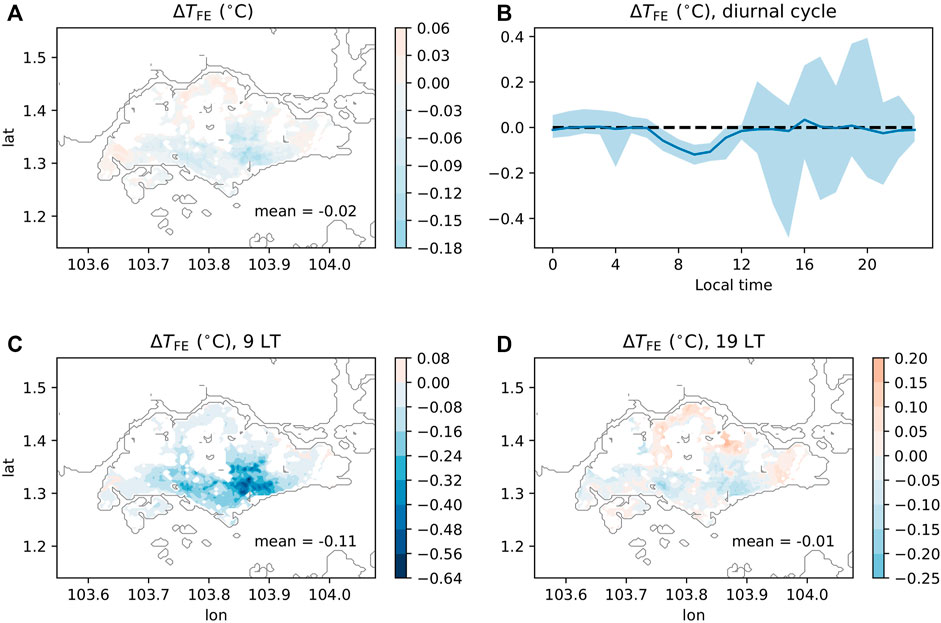
FIGURE 2. Full electrification of the fleet (FE) mitigates heat, mostly after the morning traffic peak in the central urban area. (A) shows the period-average near-surface air temperature difference (ΔTFE, °C) between FE and REF, (B) shows the period-average diurnal cycle of ΔTFE, (C) shows the period-average TFE at 9 local time (LT) and (D) shows the period-average ΔTFE at 19 LT. Only values over the urban area (defined as furb > 0.1) are shown.
The impact of FE is strongly dependent on the time of day (Figure 2B). FE has a large impact mostly during and shortly after the morning traffic peak. At 9 LT, FE reduces the near-surface temperature by up to ∼0.6°C (Figure 2C). Remarkably, FE has no significant impact during and shortly after the evening traffic peak (Figure 2C), even though the reduction in Qv during that time is comparable to that of the morning traffic peak (Figure 1).
As shown in Figures 2B,D a relatively heterogeneous model response was found in the afternoon, which highlights the model sensitivity to changes in sensible heat flux at the surface. In order to isolate the impact of FE from the internal model variability, statistical significance tests have been applied to the results (Supplementary Figure S6). ΔTFE is statistically-significant (p ≤ 0.05) over the area with highest traffic density during the morning. It is important to remark that the slight afternoon warming over areas with low traffic density is not statistically significant.
It is interesting to explore the conditions that determine the different response to the traffic heat emissions at the morning and evening traffic peaks. The contrasting response between morning and evening traffic peak may be attributed to changes in the atmospheric conditions (Figure 3). The heat mitigation (ΔTFE) due to a reduction in traffic heat emissions (ΔQv) is threefold in the morning (9 LT) compared to the evening (19 LT). ΔTFE was found to be correlated to the local wind speed (u) and the boundary layer height (HPBL), while urban morphology (fbld) appears to play a minor role. In other words, the reduction in traffic heat emissions has a stronger impact during low wind speed and shallow boundary layer conditions.
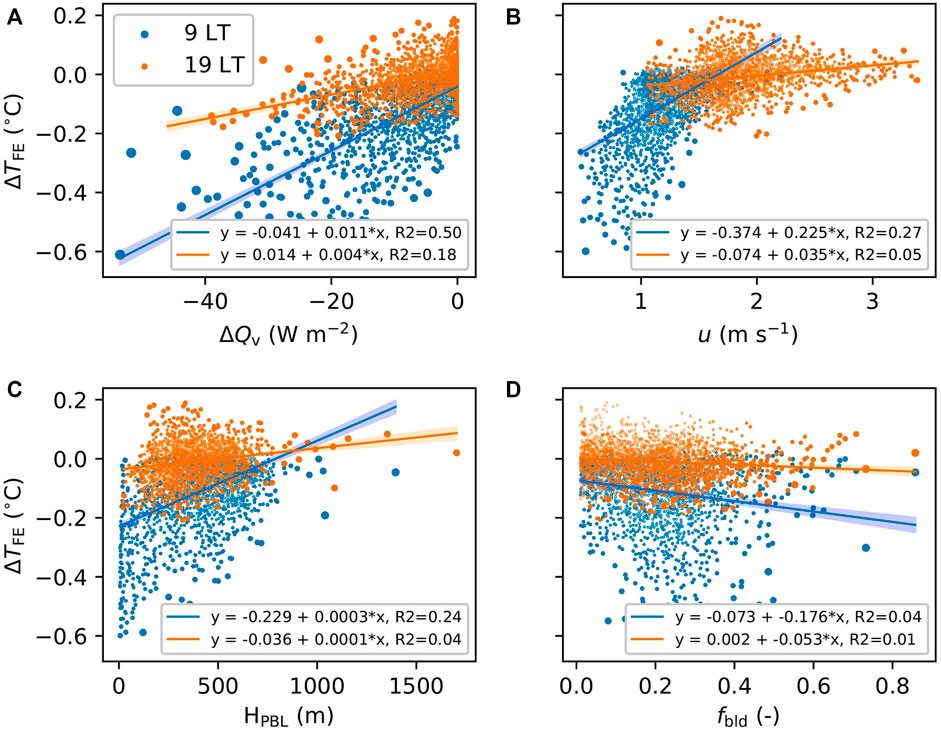
FIGURE 3. The contrasting response between morning and evening traffic peak may be attributed to changes in atmospheric conditions. Scatter plots of the heat mitigation (ΔTFE) of FE at 9 and 19 LT against the (A) reduction in traffic heat emissions (ΔQv), (B) near-surface wind speed (u), (C) boundary layer height (HPBL) and (D) fraction of building surfaces (fbld). The plot uses period-average hourly values. The solid lines and shaded areas represent the linear regression and the corresponding confidence intervals (95%), respectively.
The effect of a full fleet electrification (FE) has been compared with that of converting 25% of individual air conditioning (split) units to centralised units with exhaust release at the roof level (AC scenario, Figure 4). The heat mitigation (ΔTFE) from the AC scenario is much more uniform over the urban area than that of FE. Both FE and AC result in similar maximum impacts (∼0.2°C as daily mean ΔTFE), but the average impact of AC is 2.5 times larger. The most remarkable difference between FE and AC scenarios is their different temporal impact. While FE is only very effective during and shortly after the morning traffic peak, AC has a more homogeneous impact, with an almost constant heat mitigation during the night. The near-constant heat mitigation of AC during night-time may be partially due to the specific set-up with target temperature and constant schedule (see Section 2.1 and Supplementary Table S3). Nevertheless, FE and AC are both unable to reduce the near-surface air temperature during the central hours of the day (12–16 LT).
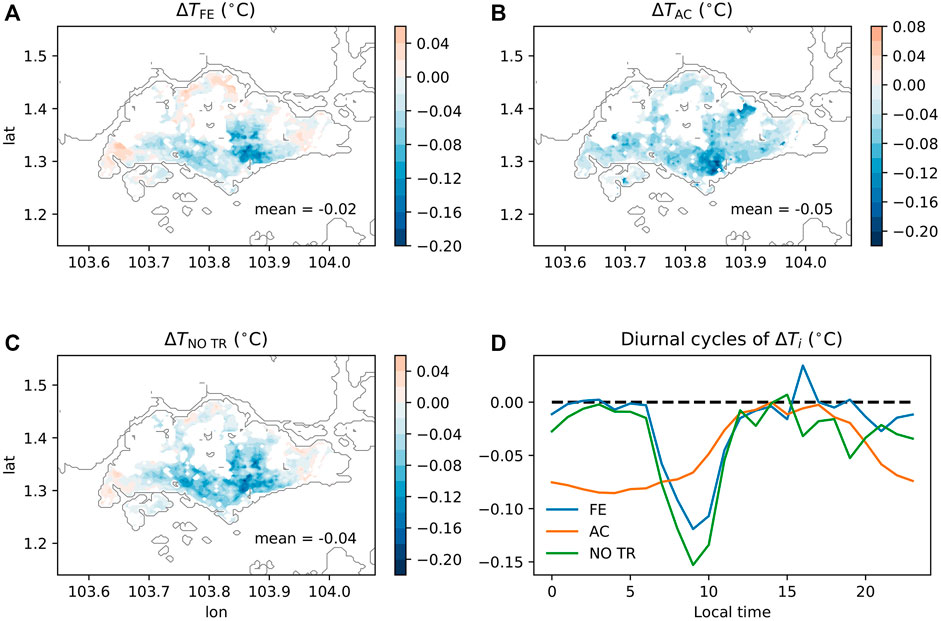
FIGURE 4. The heat mitigation of FE is more localised than measures on AC, both temporally and spatially. (A) shows the period-average near-surface air temperature difference (ΔTFE, °C) due to a full fleet electrification, (B) shows the period-average ΔTFE due to an increase in 25% in centralised AC (AC), (C) shows the period-average ΔTFE in the case of no traffic (NO TR) and (D) shows the period-average diurnal cycle of ΔTFE for the different scenarios.
4 Discussion
4.1 Heat Mitigation Potential of Electric Vehicles
The results of this study indicate that the full fleet electrification (FE) produces a reduction in near-surface air temperature, which is moderate at the daily scale but substantial during the morning traffic peak (up to 0.6°C). In this work, the estimated heat mitigation potential of EVs is lower than what found by Li et al. (2015) but on the same order of what found by Singh et al. (2022). Beside differences in climatic and traffic conditions, we argue that the estimation provided by Li et al. (2015) may overestimate the heat mitigation potential of EVs due to oversimplification of the complex interactions between exhaust heat emissions and atmospheric dynamics.
Our study highlights that the fleet electrification, and in general other measures which reduce the anthropogenic sensible heat emissions (such as the use of centralised AC units), are inefficient in reducing the air temperature during mid-day. The mechanism behind this reduced impact during daytime can be attributed to the specific atmospheric and surface energy balance (SEB) conditions. On the one hand, the boundary layer height (HPBL) determines the vertical column over which the (reduction in) sensible heat (Q) is distributed. Daytime is generally associated with large values of HPBL (Stull, 2012), which makes even substantial reductions in Q (e.g., anthropogenic heat reductions) mostly ineffective. Additionally, daytime SEB is dominated by the net incoming radiation, which reaches values that are up to 1 order of magnitude larger than those obtained by a FE [500 W m−2 at noon, according to Roth et al. (2017)]. As such, reducing the air temperature during daytime remains challenging. Interventions on other drivers of the urban heat island are necessary to efficiently reduce the daytime air temperature in tropical climates. Of the possible solutions, increasing the fraction of urban vegetation and applying highly reflective roofs (cool roofs) were found to perform best on daytime heat (Li and Norford, 2016; Schwaab et al., 2021; Wong et al., 2021).
4.2 Limitations and Future Studies
We acknowledge that there are a number of limitations of the study. The impact of local radiative forcing from gaseous and aerosols emissions from vehicular traffic has not been considered here. Previous studies found either small negative (Massoli et al., 2009) and positive (Balkanski et al., 2010) values of radiative forcing due to vehicular traffic emissions. It is therefore still unclear what role traffic-related aerosols play in local thermal conditions.
The model simulations have been performed in the so-called turbulence grey zone, a spatial resolution where the assumptions of some of the physical parameterisation are no longer valid (Honnert et al., 2020). Nevertheless, the use of meso-scale numerical models in the grey-zone is a common practice and may still provide useful results.
Only traffic conditions related to a “typical day” have been used in this study. This assumption is arguably reasonable for calculating monthly averaged impact. However, the impact of variations in traffic density between weekday and weekend, and between different days in the week, was not estimated.
The heat mitigation potential of a full fleet electrification (FE) has been investigated here for a specific city (Singapore)—characterised by its own traffic intensity, (spatio-temporal) traffic pattern and background climate—during an individual month within the dry season. The values of heat mitigation potential of a FE found in this study are directly representative for cities with similar characteristics (e.g., cities in South-East Asia). Cities in different climates, even with similar traffic conditions, may experience slightly different heat mitigation potential from FE due to different atmospheric conditions (e.g., local circulation and boundary layer dynamics) (Stull, 2012). Therefore, further studies are required to consolidate the understanding of the heat mitigation potential of a FE in different traffic and background-climate conditions.
Finally, futures studies would be required to explore how the heat mitigation dynamics of reductions in exhaust heat from traffic (such as FE) correlates with population exposure and, ultimately, how that would contributes to reducing heat-related health risks. It is known that population dynamics have a substantial impact on residents’ exposure to urban heat (Yang et al., 2019). Given that vehicular traffic peaks arguably correlate (both spatially and temporally) with commuting patterns, it is therefore possible that the heat mitigation potential of a FE is just delivered at the time and places where most needed.
Data Availability Statement
The data produced with this study is stored in a publicly available repository (Mussetti, 2021). COSMO-CLM is freely available for scientific use to the members of the CLM community. The code of COSMO-DCEP-BEM (Jin et al., 2021) is made freely available to any user with a valid CLM community membership. Traffic heat emission data are available under request to Ivanchev et al. (2020).
Author Contributions
GM conceived the work; JI, VS, and JA provided input data and observations; LJ provided the COSMO-DCEP-BEM model; GM conducted the experiments, GM and JS analysed the results. ED, JA, and SS supervised the work. GM wrote the manuscript with contribution from all authors.
Funding
The research was funded by the Singapore’s National Research Foundation (NRF).
Conflict of Interest
The authors declare that the research was conducted in the absence of any commercial or financial relationships that could be construed as a potential conflict of interest.
Publisher’s Note
All claims expressed in this article are solely those of the authors and do not necessarily represent those of their affiliated organizations, or those of the publisher, the editors and the reviewers. Any product that may be evaluated in this article, or claim that may be made by its manufacturer, is not guaranteed or endorsed by the publisher.
Acknowledgments
The research was conducted under the Cooling Singapore project. Cooling Singapore is a collaborative project led by the Singapore-ETH Centre (SEC), with the Singapore-MIT Alliance for Research and Technology (SMART), TUMCREATE (established by the Technical University of Munich), the National University of Singapore (NUS), and the Agency for Science, Technology and Research (A*STAR). The authors would like to thank Hee Joo Poh from A*STAR for providing the 3-D city model of Singapore. Lea A. Ruefenacht is greatly acknowledged for managing the project. We thank Muhammad O. Mughal and Gerhard Schmitt for their feedback on the work. The Swiss National Supercomputing Centre (CSCS) is greatly acknowledged for providing computational resources. We thank the two reviewers for their valuable comments.
Supplementary Material
The Supplementary Material for this article can be found online at: https://www.frontiersin.org/articles/10.3389/fenvs.2022.810342/full#supplementary-material
References
Balkanski, Y., Myhre, G., Gauss, M., Rädel, G., Highwood, E. J., and Shine, K. P. (2010). Direct Radiative Effect of Aerosols Emitted by Transport: from Road, Shipping and Aviation. Atmos. Chem. Phys. 10, 4477–4489. doi:10.5194/acp-10-4477-2010
Bassett, R., Cai, X., Chapman, L., Heaviside, C., Thornes, J. E., Muller, C. L., et al. (2016). Observations of Urban Heat Island Advection from a High-Density Monitoring Network. Q.J.R. Meteorol. Soc. 142, 2434–2441. doi:10.1002/qj.2836
Chen, F., Kusaka, H., Bornstein, R., Ching, J., Grimmond, C. S. B., Grossman-Clarke, S., et al. (2011). The Integrated Wrf/urban Modelling System: Development, Evaluation, and Applications to Urban Environmental Problems. Int. J. Climatol. 31, 273–288. doi:10.1002/joc.2158
Copernicus Climate Change Service (C3S) (2017). Era5: Fifth Generation of Ecmwf Atmospheric Reanalyses of the Global Climate. Available at: https://cds.climate.copernicus.eu/cdsapp#!/home (Accessed 08 13.2020
Demuzere, M., Harshan, S., Järvi, L., Roth, M., Grimmond, C. S. B., Masson, V., et al. (2017). Impact of Urban Canopy Models and External Parameters on the Modelled Urban Energy Balance in a Tropical City. Q.J.R. Meteorol. Soc. 143, 1581–1596. doi:10.1002/qj.3028
ESA (2017). “Land Cover CCI Product User Guide Version 2,” in Tech. rep., ESA. Available at: https://maps.elie.ucl.ac.be/CCI/viewer/download/ESACCI-LC-Ph2-PUGv2_2.0.pdf.
Gaw, L. Y.-F., Yee, A. T. K., and Richards, D. R. (2019). A High-Resolution Map of Singapore's Terrestrial Ecosystems. Data 4, 116. doi:10.3390/data4030116
Hardman, S., Chandan, A., Tal, G., and Turrentine, T. (2017). The Effectiveness of Financial purchase Incentives for Battery Electric Vehicles - A Review of the Evidence. Renew. Sustainable Energ. Rev. 80, 1100–1111. doi:10.1016/j.rser.2017.05.255
Honnert, R., Efstathiou, G. A., Beare, R. J., Ito, J., Lock, A., Neggers, R., et al. (2020). The Atmospheric Boundary Layer and the “gray Zone” of Turbulence: A Critical Review. J. Geophys. Res. Atmospheres 125, e2019JD030317. doi:10.1029/2019jd030317
Hsu, A., Tan, J., Ng, Y. M., Toh, W., Vanda, R., and Goyal, N. (2020). Performance Determinants Show European Cities Are Delivering on Climate Mitigation. Nat. Clim. Chang. 10, 1015–1022. doi:10.1038/s41558-020-0879-9
IPCC (2014). “Climate Change 2014: Impacts, Adaptation, and Vulnerability,” in Climate Change 2014: Impacts, Adaptation, and Vulnerability. Part A: Global and Sectoral Aspects. Contribution of Working Group II to the Fifth Assessment Report of the Intergovernmental Panel on Climate Change. Editors C. B. Field, V. R. Barros, D. J. Dokken, K. J. Mach, M. D. Mastrandrea, T. E. Biliret al. (Cambridge, United Kingdom and New York, NY, USA: Cambridge University Press), 535–612.
Ivanchev, J., and Fonseca, J. A. (2020). Anthropogenic Heat Due to Road Transport: A Mesoscopic Assessment and Mitigation Potential of Electric Vehicles and Autonomous Vehicles in Singapore. Tech. rep., ETH Zurich. doi:10.3929/ethz-b-000401288
Ivanchev, J., Fonseca, J., and Knoll, A. (2020). “Electrification and Automation of Road Transport: Impact Analysis of Heat and Carbon Emissions for singapore,” in Proceeding of the 2020 IEEE 23rd International Conference on Intelligent Transportation Systems (ITSC), Rhodes, Greece, 20-23 Sept. 2020 (IEEE), 1–8. doi:10.1109/ITSC45102.2020.9294274
Jarraud, M. (2008). Guide to Meteorological Instruments and Methods of Observation (Wmo-no. 8). Geneva, Switzerland: World Meteorological Organisation. 29.
Jin, L., Schubert, S., Fenner, D., Meier, F., and Schneider, C. (2021). Integration of a Building Energy Model in an Urban Climate Model and its Application. Boundary-layer Meteorol. 178, 249–281. doi:10.1007/s10546-020-00569-y
Jin, L., Schubert, S., Hefny Salim, M., and Schneider, C. (2020). Impact of Air Conditioning Systems on the Outdoor thermal Environment during Summer in berlin, germany. Ijerph 17, 4645. doi:10.3390/ijerph17134645
Li, C., Cao, Y., Zhang, M., Wang, J., Liu, J., Shi, H., et al. (2015). Hidden Benefits of Electric Vehicles for Addressing Climate Change. Sci. Rep. 5, 1–4. doi:10.1038/srep09213
Li, X.-X., and Norford, L. K. (2016). Evaluation of Cool Roof and Vegetations in Mitigating Urban Heat Island in a Tropical City, singapore. Urban Clim. 16, 59–74. doi:10.1016/j.uclim.2015.12.002
Liang, X., Zhang, S., Wu, Y., Xing, J., He, X., Zhang, K. M., et al. (2019). Air Quality and Health Benefits from Fleet Electrification in china. Nat. Sustain. 2, 962–971. doi:10.1038/s41893-019-0398-8
Massoli, P., Bates, T. S., Quinn, P. K., Lack, D. A., Baynard, T., Lerner, B. M., et al. (2009). Aerosol Optical and Hygroscopic Properties during TexAQS‐GoMACCS 2006 and Their Impact on Aerosol Direct Radiative Forcing. J. Geophys. Res. 114. doi:10.1029/2008JD011604
Mughal, M. O., Li, X. X., Yin, T., Martilli, A., Brousse, O., Dissegna, M. A., et al. (2019). High‐Resolution, Multilayer Modeling of Singapore's Urban Climate Incorporating Local Climate Zones. J. Geophys. Res. Atmos. 124, 7764–7785. doi:10.1029/2018JD029796
Muneer, T., and García, I. I. (2017). “The Automobile,” in Electric Vehicles: Prospects and Challenges. Editors T. Muneer, M. L. Kolhe, and A. Doyle (Elsevier), 1–91. doi:10.1016/B978-0-12-803021-9.00001-X
Mussetti, G., Brunner, D., Henne, S., Allegrini, J., Krayenhoff, E. S., Schubert, S., et al. (2020). COSMO-BEP-Tree v1.0: a Coupled Urban Climate Model with Explicit Representation of Street Trees. Geosci. Model. Dev. 13, 1685–1710. doi:10.5194/gmd-13-1685-2020
Mussetti, G. (2021). Data for ”Do Electric Vehicles Mitigate Urban Heat? The case a Trop. city”. doi:10.5281/zenodo.5788965
Oke, T. R., Mills, G., Christen, A., and Voogt, J. A. (2017). Urban Climates. Cambridge University Press. doi:10.1017/9781139016476
Quah, A. K. L., and Roth, M. (2012). Diurnal and Weekly Variation of Anthropogenic Heat Emissions in a Tropical City, singapore. Atmos. Environ. 46, 92–103. doi:10.1016/j.atmosenv.2011.10.015
Revi, A., Satterthwaite, D. E., Aragón-Durand, F., Corfee-Morlot, J., Kiunsi, R. B., Pelling, M., et al. (2014). “Urban Areas,” in Climate Change 2014: Impacts, Adaptation, and Vulnerability. Part A : Global and Sectoral Aspects. Contribution of Working Group II to the Fifth Assessment Report of th e Intergovernmental Panel on Climate Change. Editors C. B. Field, V. R. Barros, D. J. Dokken, K. J. Mach, M. D. Mastrandrea, T. E. Biliret al. (Cambridge, United Kingdom and Ne w York, NY, USA: Cambridge University Press), 535–612.
Rockel, B., Will, A., and Hense, A. (2008). The Regional Climate Model Cosmo-Clm (Cclm). metz 17, 347–348. doi:10.1127/0941-2948/2008/0309
Roth, M., Jansson, C., and Velasco, E. (2017). Multi-year Energy Balance and Carbon Dioxide Fluxes over a Residential Neighbourhood in a Tropical City. Int. J. Climatol. 37, 2679–2698. doi:10.1002/joc.4873
Sailor, D. J. (2011). A Review of Methods for Estimating Anthropogenic Heat and Moisture Emissions in the Urban Environment. Int. J. Climatol. 31, 189–199. doi:10.1002/joc.2106
Salamanca, F., Georgescu, M., Mahalov, A., Moustaoui, M., and Wang, M. (2014). Anthropogenic Heating of the Urban Environment Due to Air Conditioning. J. Geophys. Res. Atmos. 119, 5949–5965. doi:10.1002/2013jd021225
Schubert, S., Grossman-Clarke, S., and Martilli, A. (2012). A Double-canyon Radiation Scheme for Multi-Layer Urban Canopy Models. Boundary-layer Meteorol. 145, 439–468. doi:10.1007/s10546-012-9728-3
Schwaab, J., Meier, R., Mussetti, G., Seneviratne, S., Bürgi, C., and Davin, E. L. (2021). The Role of Urban Trees in Reducing Land Surface Temperatures in European Cities. Nat. Commun. 12, 1–11. doi:10.1038/s41467-021-26768-w
Simón‐Moral, A., Dipankar, A., Roth, M., Sánchez, C., Velasco, E., and Huang, X. Y. (2020). Application of MORUSES Single‐layer Urban Canopy Model in a Tropical City: Results from Singapore. QJR Meteorol. Soc. 146, 576–597. doi:10.1002/qj.3694
Singh, V. K., Mughal, M. O., Martilli, A., Acero, J. A., Ivanchev, J., and Norford, L. K. (2022). Numerical Analysis of the Impact of Anthropogenic Emissions on the Urban Environment of singapore. Sci. Total Environ. 806, 150534. doi:10.1016/j.scitotenv.2021.150534
Skamarock, W. C., Klemp, J. B., Dudhia, J., Gill, D. O., Barker, D. M., Wang, W., et al. (2005). A Description of the Advanced Research WRF Version 2. Tech. Rep. Boulder, CO: National Center For Atmospheric Research Boulder Co Mesoscale and Microscale.
Stull, R. B. (2012). An Introduction to Boundary Layer Meteorology, Vol. 13. Springer Science & Business Media. doi:10.1007/978-94-009-3027-8
Sun, R., Wang, Y., and Chen, L. (2018). A Distributed Model for Quantifying Temporal-Spatial Patterns of Anthropogenic Heat Based on Energy Consumption. J. Clean. Prod. 170, 601–609. doi:10.1016/j.jclepro.2017.09.153
Takane, Y., Kikegawa, Y., Hara, M., and Grimmond, C. S. B. (2019). Urban Warming and Future Air-Conditioning Use in an Asian Megacity: Importance of Positive Feedback. Npj Clim. Atmos. Sci. 2, 1–11. doi:10.1038/s41612-019-0096-2
TUM CREATE (2020). CityMoS. Available at: http://citymos.net.
Wolfram, P., and Lutsey, N. (2016). “Electric Vehicles: Literature Review of Technology Costs and Carbon Emissions,” in Tech. Rep. (Washington, DC, USA: ICCT).
Wong, N. H., Tan, C. L., Kolokotsa, D. D., and Takebayashi, H. (2021). Greenery as a Mitigation and Adaptation Strategy to Urban Heat. Nat. Rev. Earth Environ. 2, 166–181. doi:10.1038/s43017-020-00129-5
Yang, J., Hu, L., and Wang, C. (2019). Population Dynamics Modify Urban Residents' Exposure to Extreme Temperatures across the United States. Sci. Adv. 5, eaay3452. doi:10.1126/sciadv.aay3452
Yee, A. T. K., Corlett, R. T., Liew, S., and Tan, H. T. (2011). The Vegetation of singapore—an Updated Map. Gardens’ Bull. Singapore 63, 205–212.
Keywords: urban climate, electric vehicles, heat mitigation, urban heat island, Singapore
Citation: Mussetti G, Davin EL, Schwaab J, Acero JA, Ivanchev J, Singh VK, Jin L and Seneviratne SI (2022) Do Electric Vehicles Mitigate Urban Heat? The Case of a Tropical City. Front. Environ. Sci. 10:810342. doi: 10.3389/fenvs.2022.810342
Received: 06 November 2021; Accepted: 17 January 2022;
Published: 18 February 2022.
Edited by:
Chenghao Wang, Stanford University, United StatesReviewed by:
Isabella Capel-Timms, University College London, United KingdomPaul Osmond, University of New South Wales, Australia
Copyright © 2022 Mussetti, Davin, Schwaab, Acero, Ivanchev, Singh, Jin and Seneviratne. This is an open-access article distributed under the terms of the Creative Commons Attribution License (CC BY). The use, distribution or reproduction in other forums is permitted, provided the original author(s) and the copyright owner(s) are credited and that the original publication in this journal is cited, in accordance with accepted academic practice. No use, distribution or reproduction is permitted which does not comply with these terms.
*Correspondence: Gianluca Mussetti, bXVzc2V0dGkuZ2lhbmx1Y2FAZ21haWwuY29t