- 1Key Laboratory of Genetics and Germplasm Innovation of Tropical Special Forest Trees and Ornamental Plants, Ministry of Education, College of Forestry, Hainan University, Haikou, China
- 2Sanya Nanfan Research Institute, Hainan University, Sanya, China
- 3Intelligent Forestry Key Laboratory of Haikou City, College of Forestry, Hainan University, Haikou, China
Tropical forests, although covering only 7% of the world’s land area, have great forest carbon sequestration capacity, accounting for 20% of the world’s forest carbon sink. However, the growth dynamics and forest carbon sink potential of tropical forests remain unclear. Hainan Island is going to be China’s forest carbon trading center. Therefore, accurately assessing the future forest carbon sink potential of Hainan Island’s tropical forest is crucial. In this study, 393 forest permanent sample plots in Hainan Island in 2003, 2008, 2013, and 2018 were selected as the research objects. The dynamic model of tropical forest growth with the geospatial environmental indicators was established based on the measured and most accurate annual diameter at breast height (DBH) growth factors. The DBH growth prediction’s bias ranged from 0.46 to 0.07 cm, RMSE ranged from 1.50 to 5.29 cm, bias% ranged from -2.96 to 0.55%, and RRMSE ranged from 12.18 to 34.30%. In addition, the geospatial environmental indicators of forest growth provide scientific guidance for future ecological protection and land evolution of Hainan Island. Based on DBH–tree height–volume, volume–biomass, and biomass–forest carbon storage relationships, forest carbon sequestration potential could be accurately evaluated by DBH growth. The results show that within the next 30 years, the forest carbon sequestration in Hainan Island will account for 1.8% of the total forest carbon sequestration in China, while the forest area will only account for 0.88% of the total forest area in China. It is roughly estimated that in the next 30 years, the total carbon sink of the tropical forest in Hainan Island will be 83.59 TgC. This study further proves that the annual increase in DBH can accurately assess the forest carbon sink potential of the forest. The forest carbon sink prediction based on the annual increase in DBH can provide data support and theoretical basis for forest carbon sink trading between forest farms and enterprises.
1 Introduction
In recent years, the excessive burning of fossil fuels and increasing carbon emissions have not only increased surface temperatures but also aggravated the occurrence of natural disasters (Dong et al., 2018; Hao et al., 2021). The forest carbon sequestration capacity of forests has become a topic of scientific interest due to the role of carbon dioxide in climate change (Wen and He, 2016; He et al., 2018; Rajashekar et al., 2018; Rawat et al., 2019; Sheikh et al., 2020). Tropical forests are hot spots in the study of forest carbon sequestration capacity. They only cover 7%–10% of the earth’s land surface but store 25% of the world’s forest carbon above and below the ground (Bonan, 2008) and account for 34% of the primary productivity of the land (Beer et al., 2010). There are approximately 45,000 tree species globally, 96% of which are found in tropical forests (Poorter et al., 2015). Consequently, tropical forests have a rich diversity of tree species (Navarrete-Segueda et al., 2017), providing various economic and ecological benefits for human well-being (Mitchard, 2018). Forests primarily absorb carbon dioxide from the atmosphere via photosynthesis and respiration fixing in the trees and soil, which can be observed in their growth changes. Consequently, tropical forest growth change determines the forest carbon sequestration potential. Therefore, accurately describing the tropical forest carbon sequestration potential is of great significance for understanding the global carbon cycle (Aguilos et al., 2018; Fang et al., 2018).
The most typical tropical forest in China is on Hainan Island. Due to the dense and complex canopy structure of the tropical forest on Hainan Island, it is difficult for human eyes to distinguish the height of a single tree. There are often large deviations in tree height measurement using traditional methods. Therefore, the traditional method of investigating the forest biomass carbon storage of tropical forest is not accurate. In addition, a traditional large-scale permanent sample plot survey is difficult to conduct because the traditional large-scale sample plot survey needs a lot of human and material resources. For example, China’s National Forest Continuous Inventory is carried out every 5 years, with a large time span, which is generally organized by the government. There are relatively few studies on the forest carbon dynamic model and the forest carbon sequestration potential. The forest carbon sequestration potential of forest ecosystems is one of the greatest uncertainties in the global carbon budget (Zapfack et al., 2020). The main reason for this is that the environmental determinants affecting the changes in forest carbon storage in tropical forests are still unclear and not well quantified (Malhi et al., 2006). The quality, quantity, and decomposition rate of organic matter in forest vegetation are affected by environmental conditions (Jaenicke et al., 2008), and this helps in determining the carbon balance of forest vegetation and the storage of CH4 and CO2 (Boothroyd et al., 2015). On 22 September 2020, China proposed to be “carbon neutral,” which implies offsetting the carbon dioxide emissions produced by enterprises or individuals through afforestation, energy conservation, and emission reduction to achieve “zero carbon dioxide emissions” (Tang et al., 2021). In August 2016, Saihanba, Hebei Province, reached China’s first forestry afforestation forest carbon sequestration transaction. However, there are still differences in the methods of accurately calculating carbon emissions. Due to the different data sources, assumptions, and methods of each province, the estimated values vary greatly, which is difficult to compare. In addition, the dynamic change of land cover after deforestation in each province has different effects on the energy flux of newly built forests in the future. Previously, only a few studies have described the relative importance of forest growth attributes and environmental drivers on the forest carbon sequestration capacity, particularly in tropical areas (Poorter et al., 2015; Johnson et al., 2017). Therefore, it is critical to accurately quantify the complex environmental driving factors and clearly reveal the scientific and quantitative relationship between tropical forest growth and geospatial environmental indicators to achieve an accurate prediction of tropical forest carbon storage.
The tropical forest canopy is more complex, and the canopy occlusion is serious. It is difficult to accurately measure the tree height with traditional methods. However, the measurement of the diameter at breast height (DBH) is not affected by this problem. Therefore, measuring DBH is easier than measuring the tree height, making it more suitable for further modeling to estimate the forest carbon sink. In this study, according to the data of China’s National Forest Continuous Inventory (2003, 2008, 2013, and 2018), the tropical forest in Hainan Island is divided into 13 main tree species groups. We used the data of surface meteorological observation stations and the forest ecosystem biomass in the last 20 years. This study discussed the quantitative scientific relationship between the DBH growth of main tree species and the local climate environment, such as terrain, soil thickness, annual average rainfall, annual average minimum temperature, and annual average maximum temperature. Therefore, we propose a geospatial environmental index for tropical forest growth, which can accurately predict future DBH changes. Moreover, the relationships among the DBH, tree height, volume, above ground biomass, and forest carbon storage were established. Furthermore, a forest carbon storage measurement system for the tropical forest in Hainan Island was constructed. The forest carbon storage from 2003 to 2050 was then estimated for the island’s forests. This study accurately predicted tropical forest carbon storage in Hainan Island from 2003 to 2050 and explored the influence mechanism of tropical forest carbon sequestration potential (Figure 1). The results will help in improving the scientific and technological level of decision-making and management of tropical forest resources, serving China’s goal of achieving carbon neutrality by 2060, and providing a theoretical basis for forest carbon storage calculations.
2 Materials and Methods
2.1 Data Sources
The Hainan Province has a total land area (primarily including Hainan Island, Xisha Islands, Zhongsha Islands, and Nansha Islands) of 35,400 km2, with a sea area of approximately 2,000,000 km2. Hainan Island is China’s special economic zone and pilot free trade zone, covering approximately 34,000 km2. It is the southernmost provincial administrative region in China. In Hainan Island, the terrain around the edge is low and flat, but in the middle, it rises high to form dome mountains. Wuzhishan and Yinggeling are located in the center of the uplift. Hainan Island has a tropical oceanic monsoon climate, indicating that it is warm and hot throughout the year with abundant rainfall. From 2003 to 2018, the forestland of Hainan Island was primarily concentrated in the south, and the eastern and northern areas, such as Haikou City, Chengmai County, and Danzhou City. The coastal areas of Hainan Island were mostly non-forest land and construction land (Figure 2).
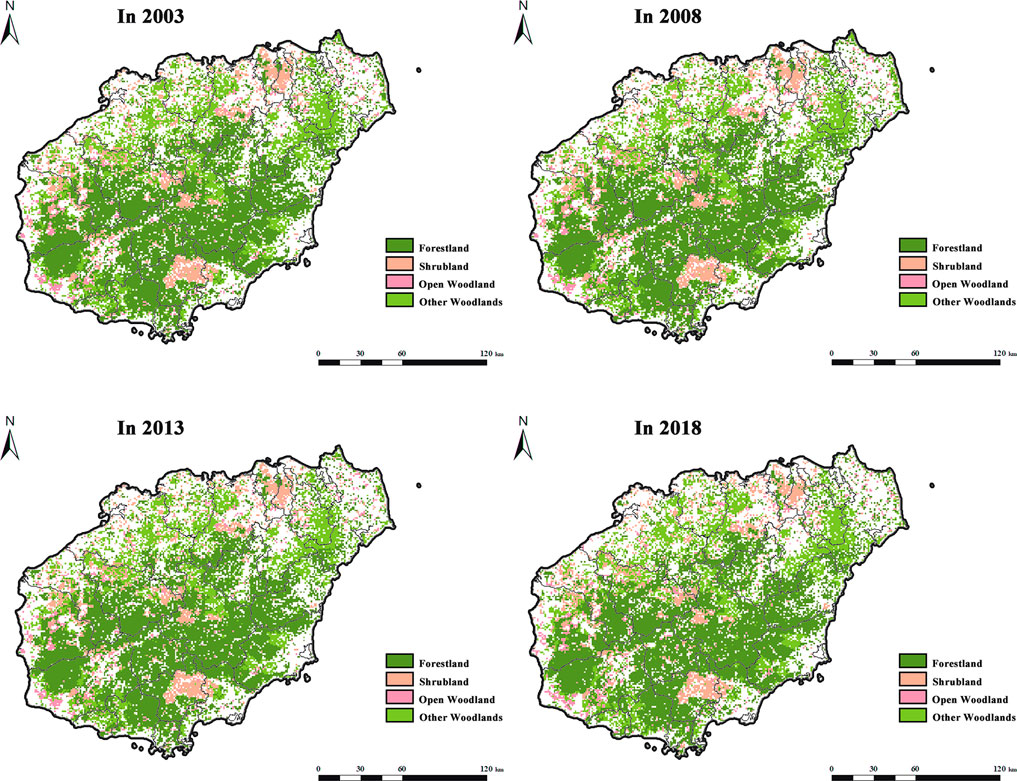
FIGURE 2. Distribution of forest types in Hainan Island in 2003, 2008, 2013, and 2018 (Forest land refers to natural forests and plantations with a canopy density >30%, including timber forests, economic forests, shelter forests, and other woodlands. Shrub land refers to the low woodland and shrub woodland with a canopy density >40% and height below 2 m. Open woodland refers to the woodland with a canopy density of 10–30%. Other woodlands refer to unforested woodlands, slashes, nurseries, and all kinds of gardens, such as orchards, mulberry gardens, tea gardens, and hot forest gardens).
In this study, 393 forest permanent sample plots were selected from China’s National Forest Continuous Inventory data in 2003, 2008, 2013, and 2018 (Figure 3). Forest permanent sample plots are square, with an area of 666.67 m2 and a length and width of 25.82 m. China’s National Forest Continuous Inventory database comprises sub-populations, sample plots number, dominant tree species groups code, average age, latitude, longitude, altitude, slope direction, slope position, slope gradient, the thickness of the overburden soil layer, tree species groups name, DBH of each period, and tree volume of each period (Zeng et al., 2015). The monthly climate datasets of Hainan Island from 2003 to 2018 are provided by the basic ground, and automatic weather stations of 19 national weather stations and meteorological networks in Hainan Island. The daily observational data of 19 national meteorological stations in Hainan Island from 2003 to 2013 comprised of average atmospheric pressure, average maximum temperature, average minimum temperature, sunshine hours, monthly sunshine percentage, average water pressure, average relative humidity, maximum daily precipitation, maximum wind speed, and wind direction of maximum wind speed.
2.2 Tropical Forest Growth Model Based on Geospatial Environmental Influence Indicators
There are approximately 4,800–5,800 species of vascular plants on Hainan Island, among which 397 are endemic. Endemic species are primarily distributed in the southwest region of Hainan Island, followed by the southeast (Zhu et al., 2021). The 393 forest permanent sample plots of China’s National Forest Continuous Inventory in Hainan Island were primarily distributed in the southwest and southeast of Hainan Island. In combination with “Hainan Flora,” 11 major tree species groups were screened out. The remaining trees were divided into other hard broadleaf trees and other soft broadleaf trees (Table 1). Moreover, combined with the meteorological data of the past 20 years, complex information such as the site environment and geographical position, is divided into growth geospatial environmental indicators (e.g., longitude, latitude, altitude, and temperature) and local and regional environmental indicators (e.g., slope gradient, slope direction, slope position, and soil thickness). For the cluster analysis, the DBH growth model of the tropical forest in Hainan Island tree species groups is as follows:
In Model 1,
When different feature vectors come together, data with small absolute values, such as the annual average minimum temperature, are vulnerable to data with large absolute values, such as rainfall. We then need to normalize the extracted feature vectors so that the normalized value of the feature vector is between 0–1 to ensure that each feature vector is treated equally by the classifier. The normalization of a feature vector is shown in Table 2: 1) from north to south, Hainan Island stretches from Mulan Bay (northern latitude: 20°9 “32”) to Jinmu Corner (northern latitude: 18°9 “21”); 2) from west to east, it stretches from Beibu Gulf (eastern latitude: 108°37 “15”) to Tonggu Corner (eastern latitude: 111°3 “6”); 3) Wuzhishan is the highest mountain on Hainan Island, with a peak altitude of 1,867.1 m, and the lowest altitude of Hainan Island is 0 m; 4) over approximately 20 years, the lowest annual average rainfall was in Southwest Hainan Island (Changjiang Li Autonomous County, Dongfang City, Ledong Li Autonomous County, Sanya City) from 2009 to 2013 and was 1,045.34 mm. The maximum annual average rainfall was in Central Hainan Island (Baoting Li Miao Autonomous County, Qiongzhong Li Miao Autonomous County, Tunchang County, Wuzhishan City) from 2009 to 2013 and was 2,552.08 mm; 5) the lowest annual average minimum temperature was in Central Hainan Island from 2004 to 2008 and was 19.873°C. The highest annual average minimum temperature was in Southwest Hainan Island from 2014 to 2018 and was 23.360°C; 6) the lowest annual average maximum temperature was in Northeast Hainan Island (Chengmai County, Dingan County, Haikou City, Wenchang City) from 2009 to 2013 and was 28.027°C. The highest annual average maximum temperature was in Northwest Hainan Island (Baisha Li Autonomous County, Danzhou City, Lingao County) from 2014 to 2018 and was 29.432°C; 7) the slope gradient was between 0° and 60°; 8) the slope direction was divided into 0°, 45°, 90°,..., 345°; 9) the slope position was divided into the upper slope position (1), middle slope position (0.625), and lower slope position (0); and 10) according to the forest permanent sample plot data used in this study, the thickness of the overburden soil layer was between 30 and 300 cm (Qiu et al., 2020).
2.3 Accuracy Validation of DBH Growth Models for Major Tree Species Groups in Tropical Forest
The data of more than 45,000 trees from 393 forest permanent sample plots were randomly divided into five groups to ensure that each group contained all of the tree species information. Four groups of data were fitted with a DBH growth model for the major tree species groups in Hainan Island’s tropical forest. The other group was used for accuracy verification. To verify the accuracy of the model in predicting the DBH growth of the major tree species groups over 5 years, a set of reserved data was used. Bias, relative bias (bias%), root mean square error (RMSE), and relative root mean square error (RRMSE) were calculated (Qiu et al., 2018a; Qiu et al., 2018b), and the prediction of DBH growth was evaluated comprehensively.
In these models,
2.4 Tropical Forest Carbon Storage Measurement
Tropical forest carbon storage measurement primarily includes height curve model, forest volume model, forest biomass conversion model, and forest carbon content. The parameters of the models are summarized according to our previous results on the forest ecosystem carbon storage in China (Qiu et al., 2020).
DBH measurements are typically fast, convenient, and accurate; however, tree height measurements are time-consuming and laborious. Therefore, in the forest survey, tree height was only measured for some dominant trees. It is typically predicted using the tree height curve model (Sharma and Parton, 2007) for different tree species groups, as follows:
In Model 6,
The estimates of increasing forest volume can also serve as a basis for the estimates of aboveground forest biomass and carbon storage. This forest biomass and carbon storage data have gradually become the basis of international treaties as forest volume can be considered as the accumulation of tree volume (McRoberts et al., 2013). Therefore, volume calculation for tree species groups is the key to this investigation. Currently, most countries in the world use two variable volume equation tables as the basic tree volume tables. In recent years, the two variable volume equation table is widely used in China as a tree volume model for 56 major tree species (35 needles and 21 broad leaves), as compiled by the Chinese Agriculture and Forestry Department. The details are summed into tropical forest tree volume model parameters, as shown in Supplementary Appendix Table SA2. The forest volume
In Model 7 and Model 8,
Biomass expansion factor (BEF) can be defined as a fixed ratio of forest biomass and forest volume, and multiple reaction monitoring (MRM) is used to estimate the forest carbon storage in different areas. If forest biomass is calculated based on forest inventory data, BEF between forest biomass and volume must be established (Fang et al., 2001). Therefore, the forest biomass (Mg/ha) and forest biomass growth (Mg/ha·5 years) of the tropical forests are shown as follows:
In Model 9 and Model 10,
3 Results
3.1 Fitting Results of the Major Tropical Tree Species Groups’ Growth
The model-fitting R2 values of Other Pine (1), Casuarina, and Eucalyptus were 0.482, 0.431, and 0.603; the model-fitting R2 of other tree species groups was between 0.844 and 0.957 (Figure 4). The model-fitting results are shown in Table 3. The growth rate parameters of the major tree species groups ranged from 0.003 to 0.054, and the growth acceleration parameters ranged from 0.010 to 0.035. The growth rate and acceleration parameters for the different tree species groups had significant differences. The influence parameter of the latitude was 0.098, which indicates that from Jinmu Corner in the southernmost part of Hainan Island to Mulan Bay in the northernmost part, the higher the latitude, the better the trees grow. The influence coefficient of the longitude was 0.790, which indicates that the longitude can promote tree growth from Western Beibu Gulf to Eastern Tonggu Corner. The annual average minimum temperature was −0.276, which can inhibit tree growth. The annual average maximum temperature was 0.373, which can promote tree growth. The influence parameter of the annual average rainfall was 0.103, which indicates that the annual average rainfall can promote tree growth. The influence parameter of the slope gradient was −0.043, indicating that the slope has an inhibitory effect on tree growth. A parameter value of 0.057 for slope direction indicated that trees on the north slope grew better than those on the south slope. The influence parameter of the slope position was 0.013, which indicates that tree growth on the upper slope is better than that on the lower slope. The influence parameter of soil thickness is −0.083, which indicates that the soil thickness in the range of 15–100 mm can promote tree growth.
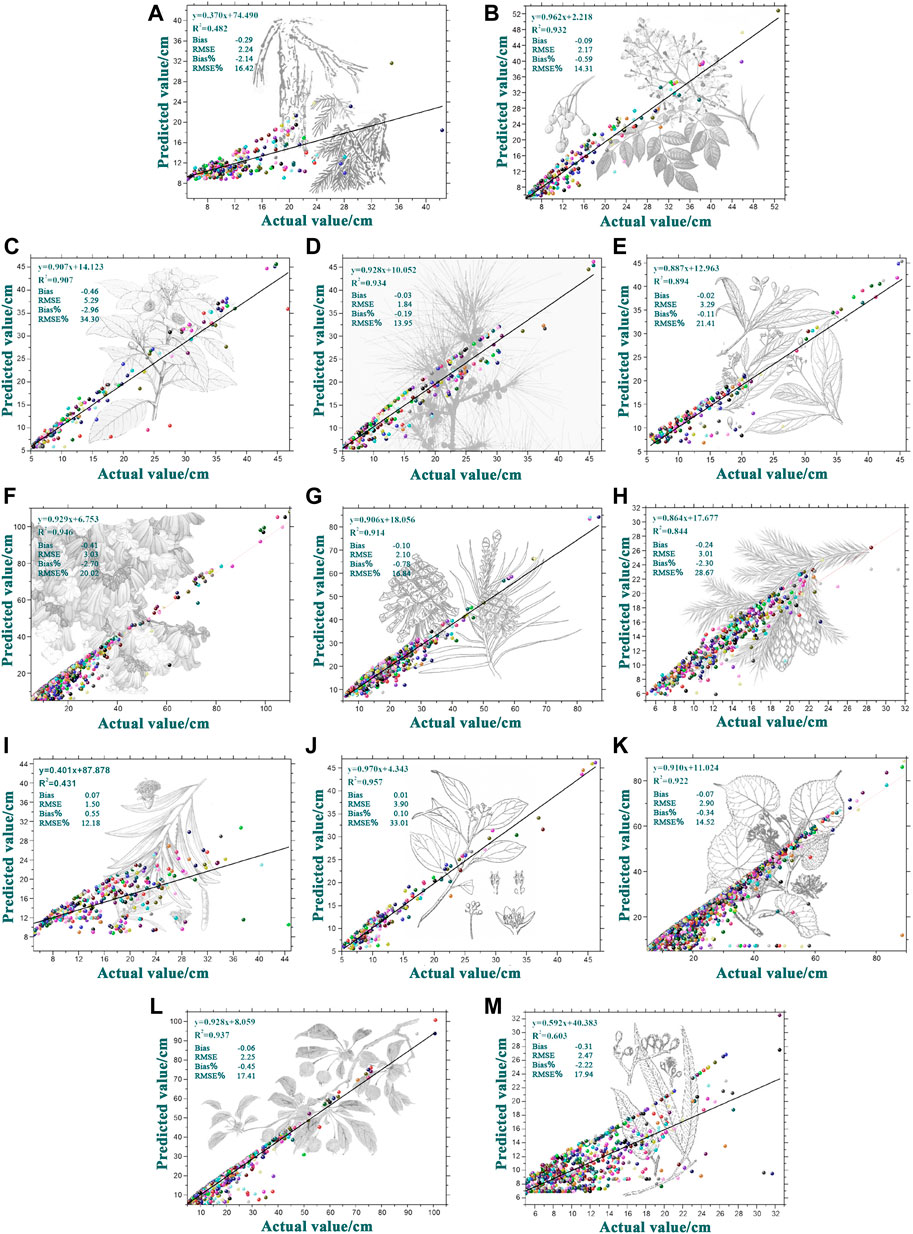
FIGURE 4. Reference value and predicted value distribution of DBH growth of 13 major tree species groups (A) Melia; (B) Casuarina; (C) Acacia; (D) Cunninghamia; (E) Schima; (F) Phoebe; (G) Quercus; (H) Eucalyptus; (I) Cinnamomum (J) other Pine (1) (K) other Pine (2) (L) other hard broadleaf trees (M) other soft broadleaf trees.
The results (Figure 4) showed that the DBH growth prediction’s bias ranged from -0.46 to 0.07 cm, RMSE ranged from 1.50 to 5.29 cm, the bias% ranged from −2.96% to 0.55%, and RRMSE ranged from 12.18% to 34.30%. The predicted values were evenly distributed on both sides of the reference values, indicating that the DBH growth prediction accuracy of major tree species groups was good.
3.2 Geospatial Environmental Influence Indicators of Tropical Forest Growth
In this study, the “interpolation” function of the “spatial analyst tool” in ArcMap 10.2 was selected, and the “inverse distance weight method” was used to deal with the geospatial environmental influence indicators’ change of the tropical forest in Hainan Island. The geospatial environmental influence indicators for tropical forest growth of Hainan Island were subdivided into a 0.05° × 0.05° grid. The growth pattern of the tropical forest in Hainan Island is shown in Figure 5. From the west to the east of Hainan Island, the growth of geospatial environmental influence indicators of the tropical forest increased gradually. The southwestern part of Hainan Island, such as Dongfang City, Ledong Li Autonomous County, Sanya City, and Changjiang Li Autonomous County had lower geospatial environmental indexes, ranging from 1.0014 to 1.6708. The geospatial environmental index of Baisha Li Autonomous County, Wuzhishan City, Baoting Li, Miao Autonomous County, and Danzhou City ranged from 1.6708 to 1.9433. The geospatial environmental index of Lingshui Li Autonomous County, Qiongzhong Li and Miao Autonomous County, and Lingao County ranged between 1.774 and 1.9433. The cities in the eastern part of Hainan Island, such as Wenchang, Qionghai, Wanning, and Tunchang counties, had higher geospatial environmental indexes, ranging from 1.9433 to 2.5120. Northern cities such as Chengmai County, Ding’an County, and Haikou City had a low to high geospatial environmental index ranging from 1.3272 to 2.0381.
3.3 Trends in Tropical Forest Carbon Storage
In this study, without considering the total consumption of forest stands, the carbon storage of tropical forests in Hainan Island was statistically predicted according to the National Forest Management Plan (2016–2050) (Figure 6). From 2003 to 2008, tropical forest area decreased slightly, while from 2008 to 2013 it increased slightly. From 2013 to 2018, the tropical forest area significantly increased by 7.66 × 104 ha and is expected to increase by 6.45 × 104 ha in the next 30 years. From 2003 to 2018, the volume of the forest increased slowly, and the forest carbon storage increased slowly after a slight decrease from 2003 to 2008. The tropical forest volume increased by 2,403.11 × 104 m3, and the forest carbon storage increased by 13.07 TgC in these 15 years. It is estimated that in the next 30 years, the tropical forest volume will increase by 23,188.33 × 104 m3, and the forest carbon storage will increase by 106.68 TgC; the forest volume per unit area and forest carbon density increased slowly before 2018, and then increased rapidly after a slight decrease in 2018. It is estimated that in next 30 years, the tropical forest volume per unit area will increase by 119.26 m3/ha changing to an increase by 392.34 m3/ha; the forest carbon density will increase by 69.60 Mg/ha changing to an increase by 194.08 Mg/ha. The annual forest carbon sink was −0.06 TgC/yr from 2003 to 2008. Due to large-scale afforestation, there was a slight increase to 1.78 TgC/yr from 2008 to 2013 and slightly increased to 0.89 TgC/yr from 2013 to 2018. In the next 30 years, the forest carbon sink is expected to grow annually and then will tend to be stable.
4 Discussion
4.1 Analysis of Tropical Forest Growth
Longitudes and latitudes are used as the impact indicators of the regional microclimate in each plot. Hainan Island is located in the Northern hemisphere. Thus, the higher the latitude in the Northern hemisphere, the shorter the day. In areas with higher latitudes, photosynthesis slows down, and tree growth cycles increase, resulting in more organic matter accumulation. Therefore, tropical forest trees grow best in high latitudes. Due to the longitudinal zonality of Hainan Island, the water content in the east to west direction was significantly different. Therefore, the higher the longitude, the better the tropical forest grows. From the perspective of topography, the smaller the slope, the more aboveground biomass (De Castilho et al., 2010), the better the plant root system (Stokes et al., 2009), and consequently, the better the tree growth. The north slope was shady with less evaporation, and the soil moisture content was better there than on the south slope. Hainan Island is located in the tropics; it has a diverse mountain terrain, a large number of microclimates (Jiang et al., 2016), and uneven precipitation, and these important factors limit tree growth. Therefore, the tree growth status on the north slope was better than that on the south slope. The tropical forest in the downhill area was heavily deforested and replaced with economically important tree crops such as rubber; however, the Areca and Cassava will remove the nutrients from the area for tree growth (Wang et al., 2007). Therefore, the growth status of the tree in the uphill area was better than that in the downhill area. From a meteorological and climatic point of view, rainfall through the canopy layer could increase the elements in the water and soil nutrients (Chen et al., 2020). Therefore, the greater the annual average rainfall, the better the tropical forest growth status. Tree growth requires specific temperature conditions, and they generally grow in areas where the average monthly temperatures exceed 6°C (Körner, 1998). In the Hainan tropical forest, the annual average minimum temperature (19.873°C), and the annual average maximum temperature (29.432°C) over the past 20 years were not found to limit tree growth. On the contrary, large temperature differences could improve the photosynthetic efficiency of the tree. Therefore, the trees in the tropical forest could grow better with lower annual average minimum air temperatures and higher annual average maximum air temperatures. The overburden soil layer in Hainan Island was thin, and the differences between the soil layer thicknesses were small, which could explain why the relationship between the tree growth status and soil layer thickness was not evident.
Notably, the higher the altitude on Hainan Island, the better the growth of the tropical forest, as this was different from previous studies (Yuliya et al., 2006; David and Robert, 2007). In general, the vertical growth of the mountain tree was hump curved, and a combination of water and heat was the best at the middle altitudes. This phenomenon can be explained by the water–energy balance hypothesis (O'Brien, 2006). However, most of the altitudes of Hainan Island were below 1,200 m, which did not reach the middle altitude for the mid-domain effect (Syfert et al., 2018). In addition, in the tropical and subtropical mountainous areas, tree lines only appeared in the places where the summer isotherm was as low as 3–6°C (Körner, 1998). In the tropical forest of Hainan Island without tree lines, the vertical zonality of the tree growth was not obvious. On the contrary, the species diversity at higher altitudes of the tropical forest was abundant. Therefore, the tree growth status of the tropical forest in the higher altitude areas of Hainan Island was better.
4.2 Feasibility and Deviation Analysis of the Tropical Forest Carbon Storage Prediction Method
According to the Ninth Inventory of Forest Resources in Hainan (2003–2018), this study used the annual increase in DBH to accurately estimate the forest carbon sink potential and follow two assumptions. First, this study assumes that the forest area data of the Ninth Inventory of Forest Resources in Hainan represent the distribution of the tropical forest area in Hainan in 2003, 2008, 2013, and 2018. Moreover, there will be no large-scale deforestation and death in the next 30 years. Secondly, this study assumes that the area proportion of existing man-made forests can approximately reflect the area proportion of newly built forests in the future. According to the proportion of existing man-made forests among various forest types of the Hainan tropical forest in the Ninth Inventory of Forest Resources, the total area of new man-made forests in the future will be allocated to various forest types of the Hainan tropical forest in proportion. After deducting the total deforestation of forest stands, total mortality of forest stands and total consumption of forest stands, we calculate the total area of tropical forests in Hainan Island in the next 30 years. The total consumption of forest stands deducted is shown in Table 4. These data include the impact of manual management measures on forest biomass. Therefore, the prediction results given in this study consider the impact of human factors and historical processes on the forest biomass carbon pool in Hainan Island to a certain extent. The prediction results can truly reflect the forest carbon pool and its change.

TABLE 4. Trends in the total consumption of tropical forest stands in Hainan Island from 2003 to 2050.
In addition, the factors affecting the accuracy of the prediction results in this study mainly include the following aspects: First, in the next 30 years, it is assumed that there will be no large-scale deforestation and tropical forest death. According to the model, tropical forest biomass will increase naturally. However, some forests are still dead or cut down in the process of growth. Young growth forests with low biomass density replace mature forests with high biomass density, which will make the estimation of tropical forest carbon storage too large. Assuming that the current mortality ratio of forest stands, and the deforestation of forest stands are maintained for the next 30 years (Table 4), according to the relationship between the current tropical forest volume and carbon storage, the tropical forest in Hainan Island will lose about 24.63 TgC in the next 30 years. Then, the forest carbon storage in 2050 will be reduced from 169.96 TgC to 145.33 TgC. Second, various influencing factors in the future may also reduce the accuracy of newly increased man-made forest estimation. With the change of policies on Hainan Island, human activities, trade, and other factors may also affect the area proportion of newly built forests. Third, factors such as climate and environmental change, natural disasters and CO2 concentration may also affect the accumulation process of forest biomass density in the future. Fourth, whether China’s forestry sustainable development strategic objectives can be achieved will directly affect the prediction results of this study. Fifth, the method of calculating the tropical forest carbon storage using the Chinese forest average carbon content will cause deviations. Therefore, it is urgent to establish tropical forest carbon content models of different tree species.
Based on the existing defects of the prediction results’ accuracy, we will improve the research from the following two aspects in the future. First, our forest DBH growth prediction model only introduces the relationship between DBH and environmental information. In the future, we want to introduce the relationship between DBH, tree age, and environmental information into the models to predict the DBH change more accurately. Then, we can estimate the forest carbon sequestration potential generated by tree growth more accurately. Second, our existing models have limitations. Our models put forward the aforementioned assumptions under the condition that there will be no extreme climate in the future. However, the future climate is unpredictable. Extreme climate may occur in the future. Therefore, we intend to introduce CMIP6 into the future models. CMIP6 refers to the monthly values of minimum temperature, maximum temperature, and precipitation that were processed for nine global climate models (GCMs): BCC-CSM2-MR, CNRM-CM6-1, CNRM-ESM2-1, CanESM5, GFDL-ESM4, IPSL-CM6A-LR, MIROC-ES2L, MIROC6, MRI-ESM2-0, and for four Shared Socio-economic Pathways (SSPs): 126, 245, 370, and 585. In this way, we can assess the relationship between climate, man-made, trade openness, and forest carbon sequestration potential in different future scenarios more clearly.
4.3 Analysis of Tropical Forest Carbon Sequestration Potential
The growth process of the tropical forest trees is primarily through respiration and photosynthesis to fix carbon dioxide, which is linked to tree growth. Therefore, accurately predicting the growth process of tropical forests to predict their forest carbon sequestration potential is of huge importance. The forest carbon sequestration potential was different for different forest ecosystems. Compared with temperate forests, the forest carbon sequestration potential of tropical forests was more effective (Terakunpisut et al., 2007). In the case of considering the total consumption of forest stands, the change trend of the tropical forest carbon sequestration potential in Hainan Island from 2003 to 2050 is shown in Figure 7. In 2020, the area of China’s forest was 1.75 × 108 ha, and the forest carbon sink generated by the growth of China’s forest in the next 30 years is estimated to be 4,667.87 TgC (Qiu et al., 2020). The tropical forest area in Hainan Island only accounted for 0.88% of China’s forest area. However, in the next 30 years, the forest carbon sink generated by tree growth in Hainan Island's tropical forest will account for 1.8% of China’s forest carbon sink. Therefore, Hainan Island's tropical forest has huge forest carbon sequestration potential in the next 30 years. From 2020 to 2050, the CO2 emissions from fossil fuel combustion in China are conservatively estimated to be 91.43 PgC, and China’s forest vegetation (tree, economic, shrub, and bamboo forests) will absorb 22.14% of the CO2 emission from fossil fuel combustion (Qiu et al., 2020). The growth of tropical forests in Hainan Island will absorb 0.34% of China’s CO2 emissions. Therefore, although Hainan Island’s tropical forest area is small, its contribution to the absorption of CO2 emissions is huge. It is roughly estimated that in the next 30 years, the total carbon sink of the tropical forest in Hainan Island will be 83.59 TgC.

FIGURE 7. Trends in the potential of tropical forest carbon sink in Hainan Island from 2003 to 2050.
5 Conclusion
By establishing the relationship between tropical forest growth and the changes in the geospatial environment, we could assess the geospatial environment influence mechanisms and predict the carbon sequestration potential generated by forest growth. This has huge significance for tropical forest growth and carbon sink predictions and is a breakthrough in the theoretical research of tropical forest response mechanisms to climate change. Through the forest growth geospatial environment indicators, the impact mechanisms of forest growth and forest carbon sequestration potential were effectively analyzed, which can significantly guide the formulation of future forest management plans and forest protection policies. Notably, in the next 30 years, China will increase its area of afforestation by 2.25 × 107 ha. Among them, the tropical forest in Hainan Island will increase by 6.45 × 104 ha. It is almost impossible to increase the afforestation area in Hainan Island tropical forest due to insufficient land area, economic development, residents’ life, and other limiting reasons, which is also a common problem faced by tropical forests worldwide. Although tropical forests cannot augment their forest carbon sequestration capacity by expanding their area, they can increase it as they grow older. Therefore, protecting the existing tropical forest ecosystems is critical.
Data Availability Statement
The original contributions presented in the study are included in the article/Supplementary Material, further inquiries can be directed to the corresponding author.
Author Contributions
ZQ, ML, and YS conceived the research route; ZQ, ML, YS, and DL designed and performed the experiments; and ZQ, ML, and Y S analyzed the data and wrote the main manuscript.
Funding
This research was funded by the “Hainan Provincial Key Research and Development Plan of China (Grant number ZDYF2021SHFZ110),” “National Natural Science Foundation of China (Grant number 32160364),” “Hainan Provincial Natural Science Foundation of China (Grant number 320QN185),” “Scientific Research Staring Foundation of Hainan University (Grant number KYQD (ZR)20056),” and “Science and Technology Project of Haikou City, China (Grant number 2020-057).”
Conflict of Interest
The authors declare that the research was conducted in the absence of any commercial or financial relationships that could be construed as a potential conflict of interest.
Publisher’s Note
All claims expressed in this article are solely those of the authors and do not necessarily represent those of their affiliated organizations, or those of the publisher, the editors, and the reviewers. Any product that may be evaluated in this article, or claim that may be made by its manufacturer, is not guaranteed or endorsed by the publisher.
Supplementary Material
The Supplementary Material for this article can be found online at: https://www.frontiersin.org/articles/10.3389/fenvs.2022.807105/full#supplementary-material
Abbreviations
BEF, biomass expansion factor; DBH, diameter at breast height; RMSE, root mean square error; RRMSE, relative root mean square error; bias%, relative bias.
References
Aguilos, M., Hérault, B., Burban, B., Wagner, F., and Bonal, D. (2018). What Drives Long-Term Variations in Carbon Flux and Balance in a Tropical Rainforest in French Guiana? Agric. For. Meteorol. 253-254, 114–123. doi:10.1016/j.agrformet.2018.02.009
Beer, C., Reichstein, M., Tomelleri, E., Ciais, P., Jung, M., Carvalhais, N., et al. (2010). Terrestrial Gross Carbon Dioxide Uptake: Global Distribution and Covariation with Climate. Science 329, 834–838. doi:10.1126/science.1184984
Bonan, G. B. (2008). Forests and Climate Change: Forcings, Feedbacks, and the Climate Benefits of Forests. Science 320, 1444–1449. doi:10.1126/science.1155121
Boothroyd, I. M., Worrall, F., and Allott, T. E. H. (2015). Variations in Dissolved Organic Carbon Concentrations across Peatland Hillslopes. J. Hydrol. 530, 372–383. doi:10.1016/j.jhydrol.2015.10.002
Chen, B., Yun, T., Ma, J., Kou, W., Li, H., Yang, C., et al. (2020). High-Precision Stand Age Data Facilitate the Estimation of Rubber Plantation Biomass: A Case Study of Hainan Island, China. Remote Sens. 12, 3853. doi:10.3390/rs12233853
Cheng, W., Feng, Z., and Yu, J. (2017). Development of Generic Standard Volume Model and Derived Form Factor Model for Major Tree Species in China. Trans. Chin. Soc. Agric. Mach. 48, 245–252. doi:10.6041/j.issn.1000-1298.2017.03.031
David, A. C., and Robert, B. A. (2007). Effects of Size, Competition and Altitude on Tree Growth. J. Ecol. 95, 1084–1097. doi:10.1111/j.1365-2745.2007.01280.x
De Castilho, C. V., Magnusson, W. E., de Araújo, R. N. O., and Luizão, F. J. (2010). Short-Term Temporal Changes in Tree Live Biomass in a Central Amazonian Forest, Brazil. Biotropica 42, 95–103. doi:10.1111/j.1744-7429.2009.00543.x
Dong, K., Sun, R., and Dong, X. (2018). CO2 Emissions, Natural Gas and Renewables, Economic Growth: Assessing the Evidence from China. Sci. Total Environ. 640-641, 293–302. doi:10.1016/j.scitotenv.2018.05.322
Fang, J., Chen, A., Peng, C., Zhao, S., and Ci, L. (2001). Changes in Forest Biomass Carbon Storage in China between 1949 and 1998. Science 292, 2320–2322. doi:10.1126/science.1058629
Fang, J., Yu, G., Liu, L., Hu, S., and Chapin, F. S. (2018). Climate Change, Human Impacts, and Carbon Sequestration in China. Proc. Natl. Acad. Sci. U.S.A. 115, 4015–4020. doi:10.1073/pnas.1700304115
Hao, L.-N., Umar, M., Khan, Z., and Ali, W. (2021). Green Growth and Low Carbon Emission in G7 Countries: How Critical the Network of Environmental Taxes, Renewable Energy and Human Capital Is? Sci. Total Environ. 752, 141853. doi:10.1016/j.scitotenv.2020.141853
He, Q., Zeng, C., Xie, P., Liu, Y., and Zhang, M. (2018). An Assessment of Forest Biomass Carbon Storage and Ecological Compensation Based on Surface Area: A Case Study of Hubei Province, China. Ecol. Indic. 90, 392–400. doi:10.1016/j.ecolind.2018.03.030
Jaenicke, J., Rieley, J. O., Mott, C., Kimman, P., and Siegert, F. (2008). Determination of the Amount of Carbon Stored in Indonesian Peatlands. Geoderma 147, 151–158. doi:10.1016/j.geoderma.2008.08.008
Jiang, Y., Zang, R., Letcher, S. G., Ding, Y., Huang, Y., Lu, X., et al. (2016). Associations between Plant Composition/diversity and the Abiotic Environment across Six Vegetation Types in a Biodiversity Hotspot of Hainan Island, China. Plant Soil 403, 21–35. doi:10.1007/s11104-015-2723-y
Johnson, D. J., Condit, R., Hubbell, S. P., and Comita, L. S. (2017). Abiotic Niche Partitioning and Negative Density Dependence Drive Tree Seedling Survival in a Tropical Forest. Proc. R. Soc. B 284, 20172210. doi:10.1098/rspb.2017.2210
Körner, C. (1998). A Re-assessment of High Elevation Treeline Positions and Their Explanation. Oecologia 115, 445–459. doi:10.1007/s004420050540
Liang, Q., Xinxiao, Y. U., Pang, Z., and Wang, C. (2010). Study on Soil Organic Carbon Density of Different Forest Types (In Chinese). Ecol. Environ. Sci. 19, 889–893. doi:10.1088/1674-1056/19/8/080512
Malhi, Y., Wood, D., Baker, T. R., Wright, J., Phillips, O. L., Cochrane, T., et al. (2006). The Regional Variation of Aboveground Live Biomass in Old-Growth Amazonian Forests. Glob. Change Biol. 12, 1107–1138. doi:10.1111/j.1365-2486.2006.01120.x
McRoberts, R. E., Næsset, E., and Gobakken, T. (2013). Inference for Lidar-Assisted Estimation of Forest Growing Stock Volume. Remote Sens. Environ. 128, 268–275. doi:10.1016/j.rse.2012.10.007
Mitchard, E. T. A. (2018). The Tropical Forest Carbon Cycle and Climate Change. Nature 559, 527–534. doi:10.1038/s41586-018-0300-2
Navarrete-Segueda, A., Martínez-Ramos, M., Ibarra-Manríquez, G., Cortés-Flores, J., Vázquez-Selem, L., and Siebe, C. (2017). Availability and Species Diversity of Forest Products in a Neotropical Rainforest Landscape. For. Ecol. Manag. 406, 242–250. doi:10.1016/j.foreco.2017.08.037
O'Brien, E. M. (2006). Biological Relativity to Water?energy Dynamics. J. Biogeogr. 33, 1868–1888. doi:10.1111/j.1365-2699.2006.01534.x
Poorter, L., van der Sande, M. T., Thompson, J., Arets, E. J. M. M., Alarcón, A., Álvarez-Sánchez, J., et al. (2015). Diversity Enhances Carbon Storage in Tropical Forests. Glob. Ecol. Biogeogr. 24, 1314–1328. doi:10.1111/geb.12364
Qiu, Z., Feng, Z., Jiang, J., Lin, Y., and Xue, S. (2018a). Application of a Continuous Terrestrial Photogrammetric Measurement System for Plot Monitoring in the Beijing Songshan National Nature Reserve. Remote Sens. 10, 1080. doi:10.3390/rs10071080
Qiu, Z., Feng, Z.-K., Wang, M., Li, Z., and Lu, C. (2018b). Application of UAV Photogrammetric System for Monitoring Ancient Tree Communities in Beijing. Forests 9, 735. doi:10.3390/f9120735
Qiu, Z., Feng, Z., Song, Y., Li, M., and Zhang, P. (2020). Carbon Sequestration Potential of Forest Vegetation in China from 2003 to 2050: Predicting Forest Vegetation Growth Based on Climate and the Environment. J. Clean. Prod. 252, 119715. doi:10.1016/j.jclepro.2019.119715
Rajashekar, G., Fararoda, R., Reddy, R. S., Jha, C. S., Ganeshaiah, K. N., Singh, J. S., et al. (2018). Spatial Distribution of Forest Biomass Carbon (Above and below Ground) in Indian Forests. Ecol. Indic. 85, 742–752. doi:10.1016/j.ecolind.2017.11.024
Rawat, M., Arunachalam, K., Arunachalam, A., Alatalo, J., and Pandey, R. (2019). Associations of Plant Functional Diversity with Carbon Accumulation in a Temperate Forest Ecosystem in the Indian Himalayas. Ecol. Indic. 98, 861–868. doi:10.1016/j.ecolind.2018.12.005
Sharma, M., and Parton, J. (2007). Height-diameter Equations for Boreal Tree Species in Ontario Using a Mixed-Effects Modeling Approach. For. Ecol. Manag. 249, 187–198. doi:10.1016/j.foreco.2007.05.006
Sheikh, M. A., Kumar, M., Todaria, N. P., and Pandey, R. (2020). Biomass and Soil Carbon along Altitudinal Gradients in Temperate Cedrus Deodara Forests in Central Himalaya, India: Implications for Climate Change Mitigation. Ecol. Indic. 111, 106025. doi:10.1016/j.ecolind.2019.106025
Stokes, E. L., Flecknell, P. A., and Richardson, C. A. (2009). Reported Analgesic and Anaesthetic Administration to Rodents Undergoing Experimental Surgical Procedures. Lab. Anim. 43, 149–154. doi:10.1258/la.2008.008020
Syfert, M. M., Brummitt, N. A., Coomes, D. A., Bystriakova, N., and Smith, M. J. (2018). Inferring Diversity Patterns along an Elevation Gradient from Stacked SDMs: A Case Study on Mesoamerican Ferns. Glob. Ecol. Conserv. 16, e00433. doi:10.1016/j.gecco.2018.e00433
Tang, W., Weng, Y., Zhang, Y., and Cao, X. (2021). Path Analysis of Implementing Carbon Neutral Target in Customer Side of Power Grid Company. IOP Conf. Ser. Earth Environ. Sci. 661, 012020. doi:10.1088/1755-1315/661/1/012020
Terakunpisut, J., Gajaseni, N., and Ruankawe, N. (2007). Carbon Sequestration Potential in Aboveground Biomass of Thong Pha Phum National Forest, Thailand. Appl. Ecol. Env. Res. 5, 93–102. doi:10.15666/aeer/0502_093102
Wang, Z., Tang, Z., and Fang, J. (2007). Altitudinal Patterns of Seed Plant Richness in the Gaoligong Mountains, South-East Tibet, China. Divers. Distrib. 13, 845–854. doi:10.1111/j.1472-4642.2007.00335.x
Wen, D., and He, N. (2016). Forest Carbon Storage along the North-South Transect of Eastern China: Spatial Patterns, Allocation, and Influencing Factors. Ecol. Indic. 61, 960–967. doi:10.1016/j.ecolind.2015.10.054
Yuliya, S., Jacek, O., Peter, B. R., Mark, G. T., Eugene, A. V., and Jerzy, M. (2006). Interannual Growth Response of Norway Spruce to Climate along an Altitudinal Gradient in the Tatra Mountains, Poland. Trees 20, 735–746. doi:10.1007/s00468-006-0088-9
Zapfack, L., Weladji, R. B., Djomo, C. C., Nyako, M. C., Nasang, J. M., Tagnang, N. M., et al. (2020). Biodiversity and Carbon Sequestration Potential in Two Types of Tropical Rainforest, Cameroon. Acta Oecol. 105, 103562. doi:10.1016/j.actao.2020.103562
Zeng, W., Tomppo, E., Healey, S. P., and Gadow, K. V. (2015). The National Forest Inventory in China: History - Results - International Context. For. Ecosyst. 2, 2. doi:10.1186/s40663-015-0047-2
Keywords: tropical forest, forest growth dynamic, geographical information technology, data mining technology, forest carbon sequestration
Citation: Lin M, Song Y, Lu D and Qiu Z (2022) Geospatial Environmental Influence on Forest Carbon Sequestration Potential of Tropical Forest Growth in Hainan Island, China. Front. Environ. Sci. 10:807105. doi: 10.3389/fenvs.2022.807105
Received: 02 November 2021; Accepted: 25 March 2022;
Published: 30 May 2022.
Edited by:
Hans-Peter Schmidt, Ithaka Institute (Switzerland), SwitzerlandReviewed by:
Lijun Cui, South China Sea Institute of Oceanology CAS, ChinaRoger Williams, The Ohio State University, United States
Copyright © 2022 Lin, Song, Lu and Qiu. This is an open-access article distributed under the terms of the Creative Commons Attribution License (CC BY). The use, distribution or reproduction in other forums is permitted, provided the original author(s) and the copyright owner(s) are credited and that the original publication in this journal is cited, in accordance with accepted academic practice. No use, distribution or reproduction is permitted which does not comply with these terms.
*Correspondence: Zixuan Qiu, eml4dWFucWl1QGhhaW5hbnUuZWR1LmNu