- 1U.S. Geological Survey, Science and Decisions Center, Reston, VA, United States
- 2Consortium for Science, Policy and Outcomes, Arizona State University, Washington, DC, United States
- 3Applied Economics Office, Engineering Laboratory, National Institute of Standards and Technology, Gaithersburg, MD, United States
- 4U.S. Geological Survey, National Land Imaging Program and Social and Economic Analysis Branch, Fort Collins Science Center, Fort Collins, CO, United States
There is growing interest within and beyond the economics community in assessing the value of information (VOI) used in decision making. VOI assessments often do not consider the complex behavioral and social factors that affect the perception, valuation, and use of information by individuals and groups. Additionally, VOI assessments frequently do not examine the full suite of interactions and outcomes affecting different groups or individuals. The behavioral and social factors that we mention are often (but not always) innately-derived, less-than-conscious influences that reflect human and societal adaptations to the past. We first discuss these concepts in the context of the recognition and use of information for decision making. We then find fifteen different aspects of value and information pertinent to VOI assessments. We examine methodologies and issues related to current VOI estimation practices in economics. Building on this examination, we explore the perceptions, social factors, and behavioral factors affecting information sharing, prioritization, valuation, and discounting. Information and valuation issues are then considered in the context of information production, information trading and controls, and information communication pathologies. Lastly, we describe issues relating to information useability and actionability. Our examples mention the value and use of geospatial information, and more generally concern societal issues relating to the management of natural resources, environments, and natural and anthropogenic hazards. Our paper aims to be instrumentally relevant to anyone interested in the use and value of science.
1 Introduction
1.1 The Ancestral, the Unconscious, and Some Defining Questions
Humans, like many other animals, have evolved to be inherently curious, shaped by ancestral priorities reflecting a diversity of behavioral and social factors (New et al., 2007; Cosmides et al., 2010). This curiosity drives humans to (consciously and unconsciously) observe and collect data about the world around them, in turn generating information used in decision making. This information has value to individuals and, more broadly, to society. If it did not, efforts to seek information would be absent. Interest in quantifying the value of information (hereafter “VOI”) has existed for decades as economists, other scientists, and decision makers have sought to better understand the benefits derived from certain types of information (Howard, 1966; Howard, 1968; Keisler et al., 2014).
Value of information theory posits that information has potential value when it is used to inform decisions that lead to improved outcomes (Macauley, 2006). Further, information is valuable when it reduces uncertainty, although the value of a new piece of information may decline as overall knowledge surrounding a decision becomes sufficient or has decreasing marginal returns (Howard, 1966; Howard, 1968; Bernknopf and Shapiro, 2015). The estimation of VOI commonly involves comparing the difference in value between outcomes (real or hypothetical) with and without additional information (Howard, 1966). Attempts to estimate VOI, however, lead to a range of important questions, such as:
• Value(s) according to whom, and for whom?
• Conscious or unconscious value(s)?—realizing that no sharp delineation easily separates the conscious and the unconscious mind, or defines free will.
• Value(s) assessed from the past, present, and/or future?
• Do all information users benefit and if so, who are they?
• Will anyone be negatively impacted?
• Do different information users have the same perception of what might be an improved outcome?
• If many users find value in the same information, then it is a social good. But can the value of that social good be estimated? Philosophers have long realized that what is good and rational to pursue for one individual, may differ from what is good and rational to pursue for that individual’s family, or for the individual’s community, or for the individual’s country, or for another country, or for the global community (MacIntyre, 2016).
1.2 Information, Knowledge, and Human Modalities Affecting Decisions
It is important to recognize that information is not the same as knowledge and should not, therefore, be used interchangeably (Glynn et al., 2017). Knowledge is internalized information (tacit or explicit) that aligns with given beliefs (conscious or unconscious) and/or acquired behaviors, and that thereby enables decisions and actions. Given our definitions, information does not have any value unless it can become internalized and transformed into knowledge. But at the same time, human recognition, seeking, and structuring of data and observations into information implies that some potential value was, at a minimum, innately expected given the human agency in the creation and consideration of information. We recognize that the topic of value has great complexity going beyond the scope of the present paper (e.g., Kenter et al., 2019; Christie et al., 2021). Glynn et al. (2022) examine in greater depth the formation of knowledge from data and information, and the consequent transition of knowledge into decisions. Hereafter we use the word “decisions” to generally imply that an actor (individual, institution, or collectivity) or a group of actors decides to act (or not act) based on newly generated knowledge. We use “action” only when the nature or consequence of a specific action is relevant.
To put some of the questions posed above into perspective and to highlight the difference between information and knowledge, consider the analogy of a book read by different people. A page in this book may have useful information for each reader, but the value of the information may differ among readers because each may have different purposes (or “goods”) they are pursuing by reading the page. Additionally, each reader brings a unique set of beliefs, biases, heuristics, or acquired values (BBHV, including norms), lived experiences, learned expertise, processing capabilities, and many other framings that can affect how they look at, prioritize, and ingest information on the page, possibly transforming it into usable knowledge (Glynn, 2014; Glynn, 2017; Glynn et al., 2017; Glynn et al., 2018). (In this paper, we will refer to the complete set of framings as “human modalities,” and the narrower set as BBHV). These human modalities will also affect how the reader uses their newfound knowledge from the page. That use of knowledge, and therefore of information, may differ from that of another reader. Furthermore, as one reader continues reading the rest of the book and perhaps many other books, additional information is gathered for potential use.
As we already mentioned, some information may be innately (i.e., intrinsically) valuable to individuals and society (Bennett et al., 2016), whereas other information will be instrumentally valuable. The “innate value” of information is difficult to define and measure because it primarily concerns intrinsically derived human prioritizations that can affect human decisions and valuations, including the seeking of information. In contrast, “instrumental value” can be explicitly, consciously, recognized as having some instrumental purpose. Although instrumental value may be assessed more easily than innate value, doing so is still difficult because it involves estimating variable potential VOI for a range of information users rather than simply totaling the same value across a uniform set of representative individuals (Howard, 1966; Keisler et al., 2014).
All of these questions and considerations related to valuing information beg the question, “How appropriate is it to offer an absolute single estimate of VOI?” We argue not very, because seeking such answers often depends on answering a wide range of other difficult (if not impossible) to answer questions, as highlighted above. Do we think that it is possible and meaningful to measure an intrinsic, innate, or absolute value of information, without considering its users, uses, BBHV, human modalities, purposes, and context? We have doubts that this can be done, or at least done meaningfully. Nonetheless, requests or desires for single measures (usually monetary) of VOI exist—in the policy and management community (cf. Glynn et al., 2022), and also in the science community (e.g., ESA-ESRIN, 2019).
Economists have realized that human judgments, choices, decisions, and actions, are controlled by a complex diversity of behavioral and social factors; and that the idea that individuals always act rationally in pursuit of their self-interest is a false assumption underlying many mainstream economic and financial models (Thaler, 2000; Thaler, 2015). The fields of behavioral economics and behavioral finance have examined many of the biases and heuristics that influence individuals’ behaviors (Tversky and Kahneman, 1974; Kahneman and Tversky, 1979). Social scientists more generally have also looked at social influences on individual and group behavior, such as the roles of moral and social norms, in-group/out-group processes, and intra-group dynamics (Elster, 1989; Akerlof, 1997; Boudon, 2003; Lindner and Strulik, 2008; Everett et al., 2015). Progress has been made in the behavioral, economic, and social sciences. As new findings are brought forward, there is a continuing need to synthesize advances in knowledge to improve understanding of human and societal decision-making. Of particular interest is illuminating some of the ways that social and behavioral factors can affect data to decision pathways (DDPs) used to translate information into societal actions (cf. Section 2 below; also, Glynn et al., 2022, this issue).
1.3 Aims and Structure of This Article
In this paper, we elaborate on some of the social and behavioral factors and influences that affect the valuation and use of information by individuals or communities. Section 2 discusses some stages that are essential in the human recognition of a need for information, and in the follow through to decisions and actions. Examples are provided that frame the discussion in terms of perceived information familiarity and perceived urgency for decisions. Our analysis provides context for understanding how individuals, policymakers, and collectivities may develop, collect, evaluate, and use information. (We use the term “collectivities” to refer to any social affiliations, identities, groups, communities, or organizations that link people together in some way). Section 3 then provides a more detailed discussion of the factors and influences affecting information perception, prioritization, processing, and use through the lens of fifteen value explorations (VEs).
In Section 3.1, we first address how VOI is typically estimated. In both this paper and our companion paper (Glynn et al., 2022, this issue), we use the term VOI more generally to refer to the value provided by information to each of its users when it is used in decisions and improves the outcomes of those decisions. This is an instrumental definition and perspective on VOI. We are generally not seeking to assess an innate or absolute value broadly applicable to—or summed over—all users and purposes and contexts. Next, we examine under what conditions it is or is not possible to assess VOI through presence versus absence of information comparisons. We argue that the use of information automatically requires the use of an associated “model”—by which we mean all mental, conceptual, or scientific models associated with the processing and use of a given set of information. Next, we discuss the situation where an addition of information may invalidate prior held knowledge, and thereby may increase the uncertainty considered by the users of information. The idea that additional information always reduces uncertainty tends to support confirmation bias and framing and anchoring biases in the evaluation and use of information (Nickerson, 1998; Spiegelhalter et al., 2011). These are just a few of the BBHV that affect human thinking, judgments, and the valuation and use of information (Glynn, 2014; Glynn, 2017; Glynn et al., 2017; Glynn et al., 2018).
The remainder of Section 3 further expands the consideration of the behavioral and social factors affecting VOI. Our companion paper (Glynn et al., 2022, this issue) frames the VEs provided here in the context of DDPs and provides examples of different types of DDPs. Both of our papers seek to improve the science, management, and policy of complex societal issues and “wicked problems.” Wicked problems are problems that are dynamic and difficult to define and whose initially considered solutions create new problems (Rittel and Webber, 1973). We focus especially on the use of geospatial information and on societal issues relating to the management of natural resources, environments, and natural and anthropogenic hazards. We caution the reader that some of the examples that we use are hypothetical in nature, presented to provoke thoughts and reactions, or perhaps further questions, rather than well-documented matters of fact.
2 From Recognition to Use of Information
Better understanding of how human modalities affect data collection, information syntheses, and knowledge development would allow better understanding of how information and knowledge are used (or not) for societal applications, including planning for the future. Use (and valuation) of information by the general public can differ from that of policy makers, practitioners, and researchers (Mercier and Sperber, 2017; Rathje, 2018). Acknowledging and understanding this reality, and improving ways to deal with it, may allow for vast improvements in data use, and in the resultant decision pathways that are forged and followed by actors.
So, how are data and information used to get to decisions and actions? Following Klein (1999), we posit that the drive or urgency that leads an actor to produce, transmit, receive, and/or use information requires an ability to progressively:
1. Recognize (consciously or unconsciously) a need or use for information.
2. Internalize the information and transform it into knowledge, by seeking to align it with existing beliefs and other innately held forms of knowledge.
a. Associate (consciously or unconsciously) the information with mental or conceptual models of use.
b. Assess (consciously or unconsciously) the value of the information (VOI), and the associated models, in the context of their possible use.
3. Evaluate, and potentially compare, a multiplicity of possible decisions to move into the future, respecting the complexities of an information-rich world in which actors may differ by their beliefs, cultures, norms, and motivations. Here, we recognize that comparing options or alternatives to determine the best possible one is often not what happens, especially when decisions are urgent and/or when decision makers feel sufficiently experienced. Heuristic and experiential strategies are most commonly used instead, often starting with and adapting to the first easily envisioned possible decision path (Klein, 1999; Kahneman and Klein, 2009; Kruglanski and Gigerenzer, 2011).
4. Advance (or not) along one or more DDPs, thereby possibly adapting to an issue, including through the actions and interactions of connected actors.
The “recognize, internalize, evaluate, advance” (RIEA) decision sequence above is a structured progression linking recognition of an issue, and the incorporation of information and associated models (mental, conceptual, and/or scientific), to some evaluation of one or more choices and possible action by an actor. We recognize that there are large differences in how individuals, institutions, and communities recognize needs for information, acquire knowledge, and make decisions using that knowledge. Our RIEA sequence is a greatly abstracted simplification.
A somewhat different way of thinking about information and the drive for action is illustrated in Figure 1. Here an actor’s sense of urgency to act is related to their recognition and perceived familiarity with the issue. Familiarity combines different forms of knowledge that the actor may have or may acquire. Specifically, the actor may have tacit knowledge acquired unconsciously through repeated lived experiences. The actor may have also developed consciously held scientific knowledge, also known as justified true belief (cf. Glynn et al., 2022, this issue), or the actor may have acquired some learned or otherwise socially transferred knowledge. Our definition of familiarity combines all these forms of knowledge.
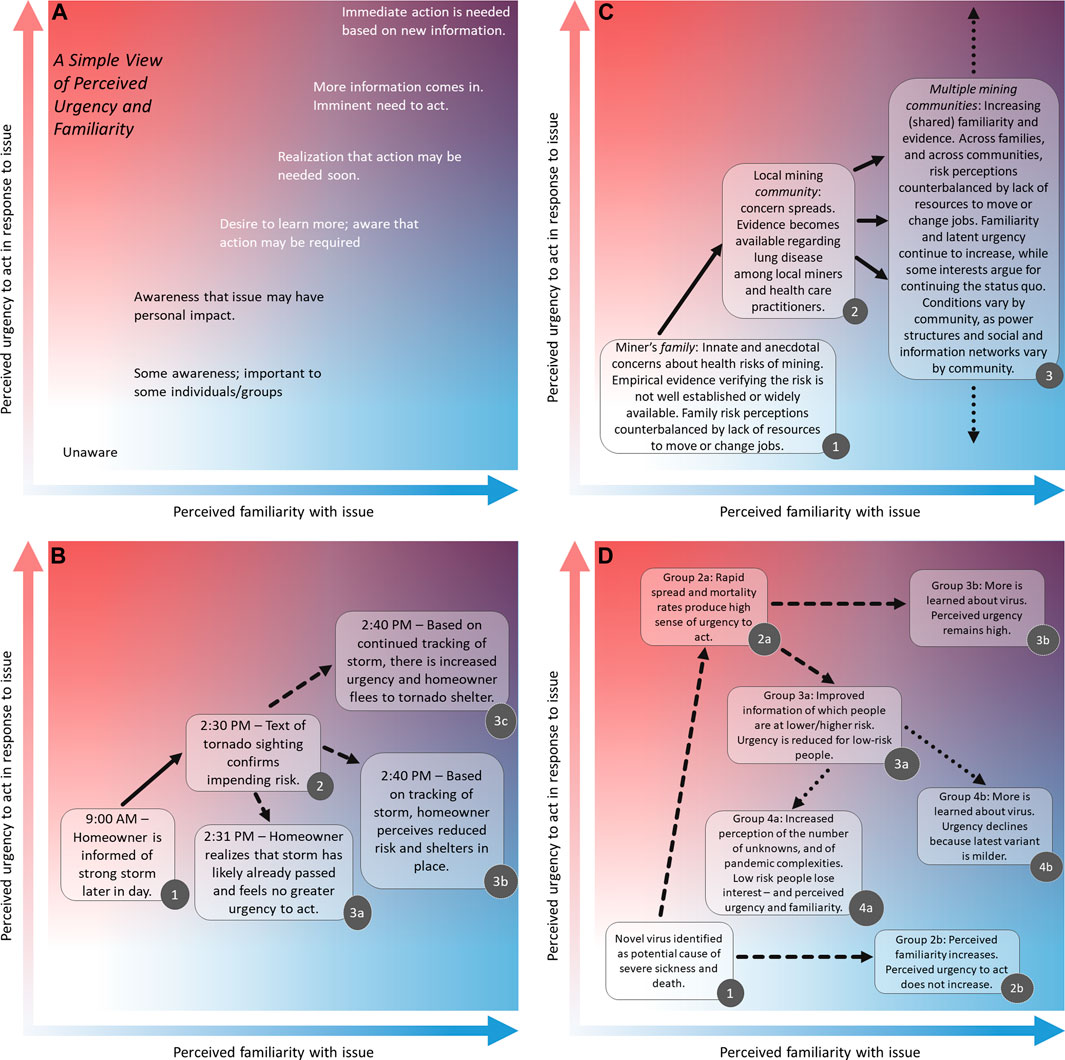
FIGURE 1. Four panels (A–D) illustrating how actors (one or many) may react to information, and perceived familiarity with (X axes) and urgency to act on (Y axes) an issue. Panel (A) illustrates a simple view of some possible states of mind of an actor in the midst of a two-dimensional spectrum of perceived urgency versus familiarity states. Panel (B) depicts a more complex hypothetical example where a homeowner is confronted by a severe storm, and information may lead to different decisions and ensuing states of mind. Panel (C) shows how information about a health risk of mining combines with a diversity of social factors (and a progression in time) to affect perceived urgency versus familiarity states of mind across different social levels—from that of a family unit to a mining-dependent community to a collectivity of communities with mining experience. Panel (D) portrays possible progressions and splintering (in perceived urgency versus familiarity) of different actor mind states responding to information (and mis-/dis-information) about a pandemic.
Figure 1 (with 4 panels) seeks to illustrate, for one or for multiple actors, how perceived familiarity might combine with perceived urgency to act, in response to information. The panels attempt to describe the states of mind of an actor(s) as they come to and make possible decisions.
Figure 1A—A simple view: Some states of mind are described for a handful of familiarity–urgency-to-act combinations. Color gradients indicate that a spectrum of such perceived states is also possible, extending beyond the depicted states. The states of mind depicted suggest a correlation between perceived urgency and perceived familiarity. However, real situations exhibit greater complexity, may have horizontal or near vertical shifts (in either upward or downward directions), or show possible discontinuities in actors’ decisions and states of mind in response to both behavioral and social factors. We seek to illustrate just a few of these complexities through some hypothesized examples (Figures 1B–D).
Figure 1B—A Storm is Coming: At 9 a.m., a homeowner realizes that a storm with heavy winds is predicted for later in the day (box 1). At 2:30 p.m., the homeowner gets a text from a local agency that a tornado has been sighted and is expected to touch down in the area soon (box 2). For the homeowner, this might then mean their urgency to act increases rapidly. Depending on the perceived quality of the information, perceived needs and conditions, timing, and available resources, the homeowner may decide (possibly in concert with associated family and friends) to either: do nothing, perhaps because the information seems stale or poor, and/or the homeowner realizes that the storm has likely already passed (box 3a); or based on further improved information to open the windows a bit and actively shelter-in-place (box 3b); or based on further tracking of the storm, to rapidly relocate to a more tornado-proof shelter in the immediate area (box 3c).
Figure 1C—Health Risks at a Dangerous Job: Consider coal miner families during the Great Depression, aware that miners who are already subject to mine collapses and other serious injuries may be developing black lung disease. Black lung disease was not medically well understood until the 1950s, but symptoms were known to mine workers well before that time. Despite their concern, a given mining family might feel the need to keep mining, given a lack of resources to change their situation and their perception of the risks of mining (box 1).
With time, stronger evidence (causal and statistical as well as anecdotal) becomes available and deeper knowledge of mining risks grows in the local community. The disease finds a formal name and local health care providers see patterns and bring them up with colleagues and patients. Social forces combine with improved information and lead to both increased familiarity and increased urgency to act in the local community (box 2). Actions to mitigate or eliminate mining risks may still not be taken because: 1) perceived risks remain below some threshold, and/or 2) are not easily implementable, and/or 3) are not pursued due to family identity and traditions (a type of behavioral inertia), and/or 4) a greater social context comes in where the perceived risks of continuing mining employment do not exceed the fears and risks of changing the situation (in a Great Depression or sub-regional job environment). Braveman et al. (2011) provide an overview of these social factors and others as they affect decisions and the health of communities. Our use of the term “behavioral inertia” is meant to cover all forms of inertia affecting decisions, such as cognitive inertia, psychological inertia, social inertia, and even knowledge inertia or learning inertia.
Across multiple communities and over more time, empirical evidence continues to grow, but forces for change also face countervailing powers as well as institutional inertia (box 3). The level of trust in new information and perceived urgency to act vary significantly across communities (demonstrated by the vertical spread and variability of box 3). Note: Figure 1C does not explicitly consider limitations on resources, such as monetary or attention resources, that may need to be considered to incentivize acquiring more information or taking action.
Figure 1D—A Pandemic Virus: Our third example explores features of how epidemics have historically affected societal structures and human behaviors, including with the COVID-19 pandemic beginning from early 2020 (Choi and Hogg, 2010; Kachanoff et al., 2020; Rosenfeld et al., 2021). Communities socially and geographically distant from the area where the pandemic was first detected may not have reacted strongly, initially (box 1), to information about the new spreading virus, including because of previous experience with the 2002–2004 SARS outbreak. However, the rapidly perceived possibility of catastrophic repercussions, despite low levels of familiarity, quickly led to a high perceived urgency to act for some groups (box 2a), together with a broadly perceived need for greater knowledge about the virus and its spread. As time went on, with greater familiarity and knowledge of how the virus operated and how to counter it, for some the need to act became less urgent (box 3a). There were also segments of the population who felt that the urgency to act needed to remain high (box 3b). Following possible paths beyond box 3a, some segments of the population then felt a decline in their perceived need to act, even as their perception of “familiarity” decreased as it became clearer that there were great unknown complexities regarding the virus and its variants (box 4a). For others, perceived knowledge and familiarity increased over many months, and this led them to feel reduced urgency for action (box 4b). Lastly, there were also some actors whose perceived urgency to act remained constant from the start, despite their own perception of increasing familiarity (box 2b).
The Figure 1 examples show that familiarity and urgency do not necessarily correlate linearly with each other. Inertia, and all sorts of behavioral and social factors can intervene. And there are many points where new information may fail to translate to knowledge, and knowledge to decisions.
There are some critical elements running through our presentation in this section, first of the RIEA sequence, and then of Figure 1. First, recognition of a need for data and information likely depends on some pre-existing knowledge or beliefs. Second, an actor’s progression—from a recognition of a need for data and information to the internalization and transformation into knowledge needed for actionable decisions—involves perceptions and the actor’s unconscious or less-than-conscious processes. The reader will also have noticed differences between 1) the stages provided initially in the decision sequence verbally illustrating the actor’s progression from recognition of an issue to decision/action, and 2) the perceived urgency/familiarity statements graphically presented in Figure 1. Because of the framing provided and the words used, the figure conveys an emotional urgency in the progression to decisions that is not present in our initial REIA decision sequence. Actors represented in Figure 1 may also seem more in the mode of reacting to possible hazards. In comparison, an actor going through the progression stages in the verbally articulated RIEA sequence may seem more analytical. That actor may be thinking ahead (perhaps more consciously than in the figure examples) and using data and information proactively, taking the time to assess VOI and associated models, to make choices, to consider the role of beliefs, social norms and cultures, and motivations held by themselves and others.
Human perceptions and processing abilities, and a wide diversity of behavioral and social factors, affect both the conscious and the unconscious valuation and use of information (Thaler and Sunstein, 2008; Cialdini, 2009; Ariely, 2010; Chabris and Simons, 2010; Kahneman, 2011; Akerlof and Shiller, 2015). In our view, this is true of individuals, but also of social collectivities of all types.
3 Value of Information Explorations
Here, we explore how social and behavioral factors affect the selection, communication, and reception of information—and of associated processing and use models. More generally, we examine the valuation, useability, and actionability of information for societal benefit. Fifteen value explorations (VEs) were developed as part of this paper. They are used to frame our discussions of VOI; and in our companion paper (Glynn et al., 2022, this issue) to examine the broader context of DDPs.
We group our 15 VEs as follows. A first subsection (3.1) discusses some methodologies and issues related to current VOI estimation practices in economic studies (VE1 to VE3). A second subsection (3.2) discusses perceptions and social and behavioral factors affecting information sharing, prioritization, valuation, and discounting (VE4 to VE9). A third subsection (3.3) discusses information and valuation issues in the context of information production, information trading and controls, and information communication pathologies (VE10 to VE12). A last subsection 3.4 discusses issues relating to information useability and actionability (VE13 to VE15).
3.1 Estimation of value in VOI methodologies
• Information for model refinement (VE1)
• VOI determination by comparison with the counterfactual (VE2)
• Information that challenges or disproves a model or hypothesis (VE3)
3.2 Perceptions and other influences on information valuation, sharing, and discounting
• Issues of information and value perception, or lack thereof (VE4, VE5)
o Information with clearly perceived direct impacts on individuals and communities (VE4)
o Information with poorly perceived indirect impacts (VE5a)
o Information with no perceived or actual relevance (VE5b)
• Sharing and use of information by communities and collectivities (VE6, VE7)
o The sharing of information (VE6)
o The wise and discerned use of information (VE7)
• Prioritization, discounting, and responsibility feedbacks (VE8)
• Stated and revealed preference valuations (VE9)
3.3 Other forms of values and valuations
• Use of information production expenditures in VOI assessments (VE10)
• Use of exchange values for assessing information value (VE11)
• Mis-/dis-information and other information communication pathologies (VE12)
3.4 Information useability and actionability
• Value ascribed through statistical analyses or other community or population assessments (VE13)
• Resource and equity issues in relation to information valuation and use (VE14)
• Importance of dependent information, and “future-found” value of information (VE15)
Table 1 provides an organized summary of our 15 VEs, providing some considerations of behavioral and societal factors that can affect valuation processes, use of information, and thus have relevance to VOI assessment methodologies. Various aspects of scope are discussed as well as some identified opportunities and challenges, and some probing questions that may help improve VOI and address valuation issues.
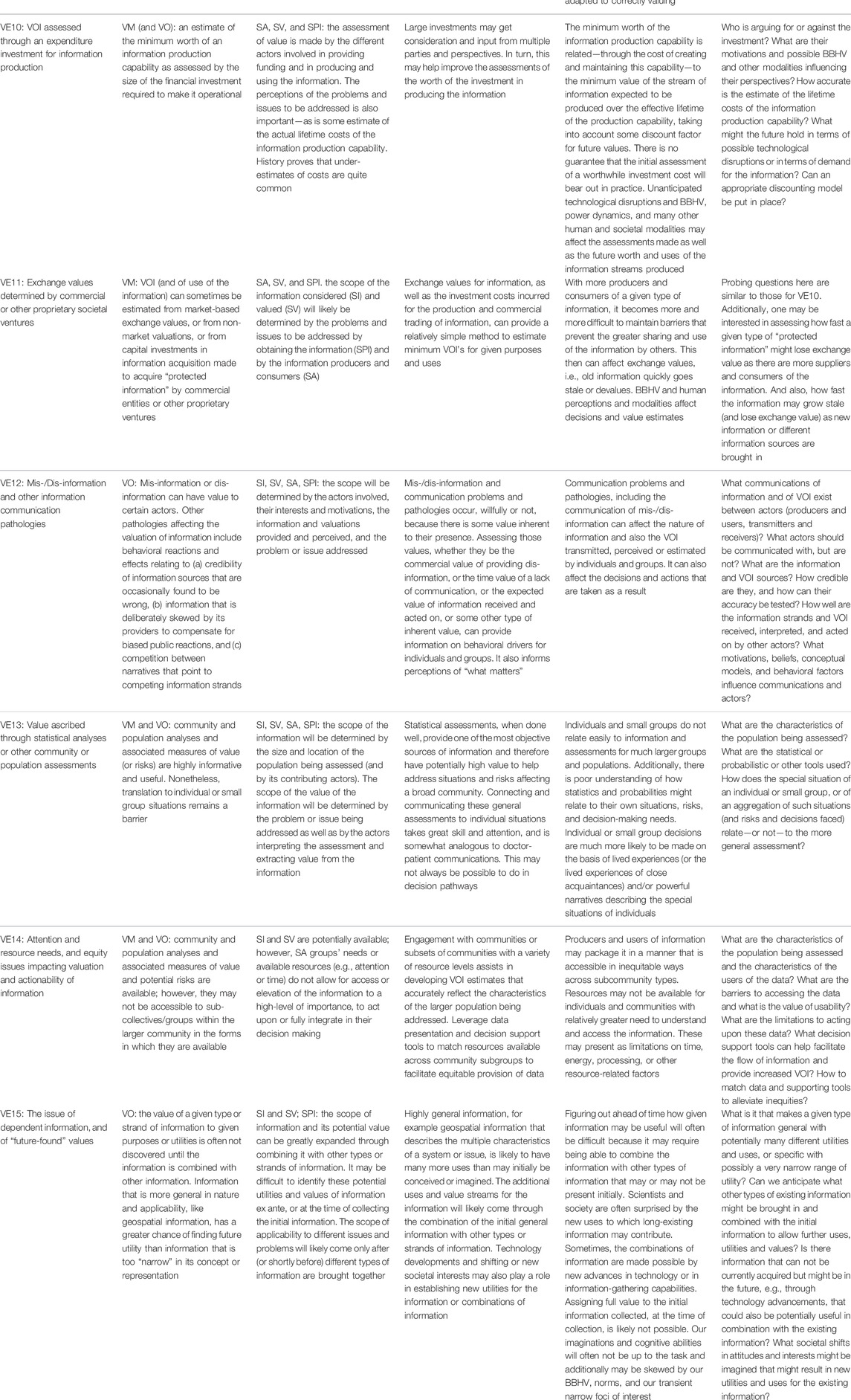
TABLE 1. Summary of fifteen “value explorations” of VOI methodologies and of behavioral and social factors influencing information and VOI. See accompanying article by Glynn et al. (2022, this issue) for complementary analyses.
The 15 VE discussions below complement the information provided in Table 1. Illustrative examples and supporting references are also given for the social and behavioral factors that we consider important to the valuation and use of information (including for DDPs).
3.1 Estimation of Value in VOI Methodologies
3.1.1 Information for Model Refinement (VE1)
VOI studies have sometimes claimed (Howard, 1966; Harris, 2002; Macauley, 2006; Bernknopf et al., 2018) that the value arises largely from the opportunity to refine or improve an existing mathematical model by decreasing the uncertainty associated with the model results. Usually what is meant is that while the model was constructed using some information, having additional information may allow for model refinement such that it has higher resolution or greater predictive or explanatory power—with consequently reduced model uncertainty. The advantage of bringing additional information to the model—without drastically changing the model—is that the value of information added to the initial “model + information” construct may now be more easily quantified. The additional information is certainly of value to the modelers or model creators: their model now has greater fidelity to observed data and/or greater explanatory or predictive power. Will the model, however, and its new associated information be used? Do the model and the additional information obtained have value for decision makers or other users, aside from the model developers and information providers?
3.1.2 VOI Determination by Comparison With the Counterfactual (VE2)
Determination of VOI through comparison with a counterfactual often assumes that the counterfactual is characterized only by an absence of the information whose value is being assessed. The determinations typically ignore the presence or absence of models (conceptual, mathematical, mental, or otherwise) that may be associated—quite possibly in distinct and diverse ways—with either the presence, or with the absence, of the information. The benefits to decision makers (and any other users of the information) of having additional information is commonly explained without describing how the decision procedures and models may change in the presence or absence of the additional information. The additional information is typically described as consisting of additional (or improved) data and/or observations. Nonetheless, models are likely present that either explicitly or innately embed several assumptions, include causal or functional relationships, and therefore possibly change how information may be used. These models affect decision processes, and potentially, could change drastically depending on the presence or absence of the additional information. Does additional information ever really have value in the absence of a model of how to use it? Probably not. Explicitly describing the different applicable model(s) in both the presence and absence of additional information is critical. For both situations, this means describing not only the model purpose(s) and scope(s) of applicability but also the involved actors—and making sure that they remain consistent.
3.1.3 Information That Challenges or Disproves a Model or Hypothesis (VE3)
VOI studies rarely consider the value of additional information that ends up invalidating a model or set of beliefs, or disproving a hypothesis (Howard, 1966; Keisler et al., 2014; Resources for The Future, 2022). And yet, such information is valuable. It allows existing concepts, beliefs, or ideas to dramatically evolve: for new theories and models to replace, or at least, supplement old ones. This type of information, potentially, has great value for the advancement of science and societal knowledge. Nonetheless, information that ends up overturning a model is not always perceived as having value, perhaps especially by actors less interested in knowledge advancement than in the preservation of existing beliefs. Indeed, anchoring of pre-existing beliefs, even in the face of contradictory information, is well established by behavioral scientists (Anderson et al., 1980; Anderson, 1982; Anderson, 1983), and has even been studied in the domain of artificial intelligence (Fagin and Halpern, 1987). Everyone is affected to some degree by an anchoring of beliefs, and therefore a desire to preserve former conceptions or models of reality.
History documents many examples of the delaying of knowledge advancement and science acceptance due to the need by some actors (including institutions) to preserve established models. Galileo Galilei’s trial by the Roman Inquisition in 1632 as a result of his defense of Copernican heliocentrism, a model published in 1543, serves as one such example (Finocchiaro, 2010).
Additionally, when new information challenges or disproves a model more quickly than actors can absorb and process, confusion and uncertainty may result. Familiarity (cf. Figure 1) may decrease. Human abilities and inabilities to perceive, process, communicate, and integrate information in timely and useful ways for decision making are a running theme throughout this paper (cf. VEs 5, 7, 8, 12, 13, 14). We leave it to the reader to assess whether the rapid advances in knowledge regarding the behavior and transmission of corona viruses and variants during the recent COVID-19 pandemic—and the resulting rapidity of communications, and the associated disparate and changing recommendations—resulted in a decrease, or an increase, in the states of certainty or uncertainty held by affected populations.
In summary, the invalidation of an existing model (conceptual, numerical, or mental) by new information does not necessarily result in a decrease in uncertainty, at least initially, regarding the system described or conceptualized by the model, especially if the perspectives of different types of interested actors are considered. Our use of the term “uncertainty” includes both epistemic and aleatoric uncertainties (Di Baldassarre et al., 2016). We suggest that epistemic uncertainty may initially increase when new information invalidates an established model. The interested reader may refer to Voinov et al. (2016) for a discussion of uncertainty in the context of participatory modeling (i.e., with multiple actors and perspectives involved).
3.2 Perceptions and Other Influences on Information Valuation, Sharing and Discounting
3.2.1 Information That Has Direct or Indirect Impacts on Individuals and Communities (VE4 and VE5)
What kind of information can humans most easily perceive and appropriately process for their personal or social benefit or to avoid harm? What type of information are humans innately well equipped to use? What type of information requires considerable and explicitly conscious efforts for humans to use? How does information that has no perceived or actual relevance impact human behavior and society? These are some of the questions that we address here. Some economic models portray humans as being infinitely and near instantly capable of perceiving, processing, and responding to all the information that matters (Stigler, 1957; Gibbard and Varian, 1978; McDermott, 2015). The behavior of these model individuals is also assumed to be always controlled by rational maximization and material self-interest. Homo economicus refers to this type of model human being (Thaler, 2000). It is important to look beyond such reductionist models of human behavior and information accessibility and use; even as it is acknowledged that the Homo economicus model retains value (Kirchgässner, 2008; Levitt and List, 2008; Schreck et al., 2020). Humans are better equipped to respond to information that they can most directly perceive, and that they have been well adapted (genetically, culturally, experientially) to perceive and respond to (VE4). They are not anywhere near as well equipped to respond to information that lies outside of their adaptation history, and/or for which they have limited capabilities to sense, perceive, process, communicate, prioritize, and respond to (VE5a). Adaptive processes have also helped humans and society make decisions to ignore information (usually unconsciously or sub-consciously) that is not perceived to be relevant (New et al., 2007) for use (VE5b). This is an essential capability for human survival in a world awash with information. Not all information can realistically be paid attention to, and prioritization of attention is critical. We explore these interconnected issues below, in the order mentioned.
3.2.1.1 Information with Clearly Perceived Direct Impacts (VE4)
It is human nature to constantly seek a balance between 1) innate curiosity (a driver of innovation and learning) and 2) prioritizing attention to information that facilitates decisions and actions (Berlyne, 1954; Loewenstein, 1994; Kobayashi et al., 2019). The latter is particularly important in a world that offers ever-increasing access to information for our attention-limited lives and capacity-limited brains. While individuals differ considerably in how they seek an appropriate balance, their prioritizations are generally well adapted for situations commonly, and acutely experienced in the past (Gigerenzer and Brighton, 2009; Marewski et al., 2010; Kruglanski and Gigerenzer, 2011). By acute, we refer to situations that have direct and immediate impacts on their lives, i.e., where there is no doubt about cause-and-effect relationships, or the impact of perceived information. People consequently place appropriately high value on information pertaining to these types of direct-impact frequently experienced situations. They do not need explicit calculations to understand the value of such information: they are likely to innately, tacitly, know it. The urgency of the situation and of the need for information is well perceived (cf. Figure 1).
3.2.1.2 Information with Poorly Perceived Indirect Impacts (VE5a)
However, the VOI developed and integrated into knowledge largely tacitly, through experiences and biocultural adaptation mechanisms (Carroll et al., 2017; Waring and Wood, 2021), does not necessarily apply well under situations such as the following (Tversky and Kahneman, 1974; Tversky and Kahneman, 1981; Kahneman and Tversky, 1979; Kahneman and Tversky, 1984; Stanovich, 2010; Spiegelhalter et al., 2011; Thaler, 2015; Glynn, 2017; Glynn et al., 2017):
• when people have limited cognitive or perception abilities;
• for new or infrequently experienced situations;
• when there is no clear and direct causal relationships between perceived information and consequent impacts (i.e., where we do not have a clear mental model); or
• when compounding or cascading impacts occur, are too diverse, or do not have needed feedbacks to allow actors to accurately process and integrate information, or adapt to it.
In addition to the challenges brought about through human perception thresholds and cognitive processing limits, three realities of the modern world can help illustrate problems and issues originating from conditions that we may not be well adapted to: 1) an increasing global human population density; 2) the hyper-connectedness of our world (in the transport of ideas, people, biota, materials, and goods); and 3) the ever-increasing sophistication of our technologies (cf. Glynn et al., 2017).
The fact that we, as individuals and communities, have fallibilities that affect our perceptions, minds, and behaviors is not new. Francis Bacon (Bacon, 1620) understood many of these issues 400 years ago when he discussed “Idols of the Mind” (i.e., of the Tribe, of the Cave, of the Marketplace, and of the Theater). For example, here is his description about the Idols of the Tribe:
The Idols of Tribe have their foundation in human nature itself, and in the tribe or race of men. For it is a false assertion that the sense of man is the measure of things. On the contrary, all perceptions as well of the sense as of the mind are according to the measure of the individual and not according to the measure of the universe. And the human understanding is like a false mirror, which, receiving rays irregularly, distorts and discolors the nature of things by mingling its own nature with it.
It seems that scientists (and everyone else) have spent over 400 years largely failing to incorporate this fundamental lesson: what matters according to people’s perceptions is not necessarily what really should matter to them. Who among us could be unfamiliar with people catering to their own perceptions and to the perceptions of others as if these were all that mattered? How many of us reside in the delusion that we are always acting rationally and with supreme logic? Human hubris and the beliefs that humans generally have in their simplifications and rationalizations about the world (Akinci and Sadler-Smith, 2019; Sadler-Smith and Akstinaite, 2021) are evolutionarily-derived adaptations that prevent human decision making from being frozen through a complexity of choices (Schwartz, 2004). The authors recognize the potential usefulness of simplifications and the need for some degree of hubris. In contrast to the present paper, an online Explainer Series by Resources For the Future (Resources for The Future, 2022) offers a more rationalistic perspective on VOI and human behavior.
For all the situations where innate perceptions and reactions are inappropriate (i.e., where evolutionary/experiential processes have not yet allowed a needed filtering), properly assessing VOI generally requires a much greater explicitly conscious effort—and calculations and assessments of VOI may still be problematic or inaccurate. Despite best efforts, we may still be influenced by our BBHV, including social and moral norms. What is the value of information that we do not properly process? Information that is not properly processed may lead to decisions that are later deemed bad. By chance, it may also lead to decisions that are later deemed good. Assessing the value of poorly processed information may be difficult to impossible to assess in this context.
3.2.1.3 Information With No Perceived or Actual Relevance (VE5b)
Lastly, we can ask what is the value of information that we do not use? Does it have any value? Although we often do not recognize it, information that is presented to us but for which we do not have any use at the time of presentation, comes at a cost to our attention and therefore may even have a negative value, at least in that moment. Such information distracts or detracts our attention from information pertinent to the needs of the moment. In other words, there are opportunity costs to accessing any information, and those costs may be especially important when the information is not critical at the time it presents itself. Herbert Simon (1971) introduced this issue well:
[I]n an information-rich world, the wealth of information means a dearth of something else: a scarcity of whatever it is that information consumes. What information consumes is rather obvious: it consumes the attention of its recipients. Hence a wealth of information creates a poverty of attention and a need to allocate that attention efficiently among the overabundance of information sources that might consume it.
It is also important to consider that information with no perceived or actual relevance at a given moment in time may eventually find relevance (cf. “future-found value”: VE15).
3.2.2 The Sharing, and Wise and Discerned Use, of Information by Communities and Collectivities—or Lack Thereof (VE6 and VE7)
Value Exploration 5b brought up the issue of information with no perceived or actual relevance, and the need to prioritize information seeking in an attention economy (Simon, 1971). Here, we build on that discussion by considering some aspects of information communication and sharing in, and between, communities and collectivities (VE6). We then provide some thoughts and examples on some of the controls on value judgments of information by communities and collectivities, and what affects the wise and discerned use of communicated information (VE7).
3.2.2.1 The Sharing of Information (VE6)
Humans have limits in their abilities to perceive and process information. Those limits vary amongst individuals, due to differences in their experiences and learning, access to human-augmentation tools and technologies (Raisamo et al., 2019), and other situational and framing factors. Ideally, we can exploit our individual differences to enhance our ability to collectively perceive and process information, i.e., to create a “wisdom of the crowds” (Surowiecki, 2005). Indeed, modern society has developed organizational structures and civic governance systems that allow the specialization of individuals, or of different private and public entities, and the sharing of the information they produce. We now have easier access to much more information than ever before; however, discerning which information is of value and which actors may be trusted is increasingly difficult (Karlova and Fisher, 2013). And our communities have often used that information to enhance quality of life, increase efficiencies, and facilitate information production through new technologies (e.g., remote sensing), specializations, and fields of learning (e.g., artificial intelligence). The resulting increase in information production and dissemination has resulted in remarkable accomplishments. For example, earthquake risks in the U.S. have been greatly decreased through the enforcement of building codes supported by advances in scientific knowledge and monitoring technologies (Burby and May, 1999; Bernknopf and Shapiro, 2015; Tanner et al., 2020).
3.2.2.2 The Wise and Discerned Use of Information (VE7)
Nonetheless, the greatly increased availability and use of information has in some cases by its own volume undermined “justified belief” and trust systems. As a result, social trust in information has often faltered or been mis-placed, and knowledge systems have splintered (Wardle and Derakhshan, 2018; Rubin, 2019), even for systems where trust is empirically justified (cf. also Figure 1 discussion). Innate reactions (by individuals or their tribes) to new information (and new situations) often represent efficiencies and habits developed over the course of human and cultural evolution (Damasio, 1994; Gigerenzer, 2007). These innate reactions often do not yield gently to our ability to think carefully as individuals—especially in today’s information-rich environments.
In critical situations where experientially based tacit knowledge is not appropriate (see Value Explorations 4 and 5), innate judgment may not only be less effective, it can actively mis-guide us away from more explicitly, thoughtfully considered, choices. The value individuals place on information is often influenced by social proofing and trust in the various tribes or social groups that they identify with (Cialdini and Goldstein, 2004). Social proofing means that we tend to believe what a group (that we identify with) believes in, and we may place prioritized attention (and value) on information provided by the group or key members. Unfortunately, the VOI a social group or tribe perceives may not accurately reflect what science and logic determine matters for addressing a given situation. On the other hand, errors of judgment by a social group are rarely called to account, and it is almost always safer for individuals to adopt a group’s values and social norms than to stick out and articulate differences of perspective and valuation. The increasing volume, and speed of exposure, of information can aggravate, rather than ameliorate, this problem. In a complex, dynamic, information-rich world, social proofing provides a key mechanism for simplifications, beliefs perceived as essential, and for the prioritizing of attention and of information.
For example, there is an ever-increasing amount of information relating to contaminant concentrations in waters across the United States (Bagstad et al., 2020). Nonetheless, communities (i.e., social groups and tribes) often do not pay attention or are not motivated to react to that information until a critical threshold is reached, an acute change in shared community perception occurs, and the issue can no longer be ignored. The threshold is often visual, such as in the case of the burning Cuyahoga River, which after more than a dozen river fires suddenly became an important factor in the establishment of the Clean Water Act (Boissoneault, 2019). A threshold may also be crossed when a clear and present danger to children is recognized, as in the case of the Flint water crisis (Butler et al., 2016; Krings et al., 2019; Nowling and Seeger, 2020). Our sensory perceptions, fallible as they are, often dictate our community responses and the value that we place on information (Krings et al., 2019; Nowling and Seeger, 2020). The value of homes next to a water body that has an increasing concentration of nutrients or contaminants (e.g., endocrine-disrupting chemicals) will generally not be affected by this increase. A threshold may be reached however, when our senses can no longer ignore the degradation in water quality: the river burns or turns orange, algal blooms develop, the lake smells bad, charismatic biota are grossly visibly deformed, etc. A sharp discontinuity occurs in the type of information available and human and community evaluations of the information also sharply react—then the exchange value of lakeside homes plummets precipitously (Kuwayama, 2018). Is this a timely rational reaction by Homo economicus? In some cases, our senses may be tricked by a transient and relatively benign phenomenon, such as a river turning orange due to the precipitation of iron oxyhydroxide. But even when our senses do appropriately detect a problem, would it not have been better to value and make earlier use of previously available information to remediate or mitigate the issue? Can we train our belief and trust systems, especially at the social tribe and communal-decision levels, to improve our ability to properly value information? Does the price of homes neighboring a lake properly reflect the value of water quality information about the lake—or does it represent delusions and misperceptions of a community?
3.2.3 Prioritization, Discounting, and Responsibility Feedbacks (VE8)
The prioritization (and use) of information in an attention economy (Simon, 1971) has similarities with the prioritization of money and investment expenditures. Any prioritization comes with associated uncertainties and opportunity costs. The greater the perceived uncertainties and potential opportunity costs, the greater the discounting that is applied to the prioritization. Social discounting relates to our preference to invest in our own present communities rather than in others (Jones and Rachlin, 2006; Locey et al., 2013). Knowledge discounting relates to our propensity to use knowledge (beliefs especially but also information) that we already hold rather than to seek to understand and use unfamiliar knowledge (Rao and Sieben, 1992). Delay discounting or temporal discounting relates to our perception that a present value (of information or money) means more to us than a future value which carries uncertainty and risks (Loewenstein and Thaler, 1989; van Dijk and Zeelenberg, 2003). All these forms of discounting are affected by social proofing (Cialdini and Goldstein, 2004).
Temporal discounting affects spending decisions that have present costs and for which the potential future benefits carry some uncertainty with respect to their nature, magnitude, and the timing of their accrual (Loewenstein and Thaler, 1989). In a highly dynamic and uncertain world, why should we place a high value on information for which the future costs and benefits carry significant uncertainty? Moreover, how motivated are we to pay present costs when we may not be around to reap the future benefits (or avoided costs)? Economists often use constant discount rates to model normal human evaluative behavior, but it has been argued that mental evaluative processes and behaviors are often discontinuous or highly non-linear when it comes to our valuation of information (Loewenstein and Thaler, 1989; Zeckhauser and Viscusi, 2008; Kahneman, 2011). We often prefer to ignore information that requires present actions (and costs) that are outside of our normal individual experiential knowledge and that of the people that we feel closest to (Loewenstein and Thaler, 1989).
A building collapse in Florida on 24 June 2021, provides an example of this phenomenon (LaForgia et al., 2021). Information provided by experts was available noting serious problems affecting the structural stability of the building, but it was difficult for non-experts to understand, and much easier to ignore or delay action that would have come with very high immediate costs to the condominium inhabitants.
Our understanding and valuation of information regarding the risks posed by natural hazards is likely worse than our understanding and valuation of risks associated with human constructions. At the level of communities and individuals, we are more likely to understand something that we build than something that nature has managed and dynamically altered over much longer time scales than our lived experiences (Wachinger and Renn, 2010; Wachinger et al., 2013). Consequently, all over the world, we often ignore the risks associated with building towns on flood plains, or in coastal areas prone to hurricanes, storm surges, and sea-level rise (Di Baldassarre et al., 2013; Ciullo et al., 2017). We also often disregard the risks of building our houses in wildfire or earthquake areas, or on potentially unstable slopes and cliffs. We ignore the pandemic risks associated with living in highly populated areas, and those associated with our disruption of ecosystems and the direct and indirect killing of countless species (Campbell-Arvai, 2019).
When catastrophes occur that we deem to be natural, we seek refuge in the exceptionalism of the events, and in our self-ascribed lack of possible control (Linkov et al., 2014; Salazar et al., 2016; Ciullo et al., 2017). Although blame attribution in the face of disasters is a complex topic, scapegoating and the shifting of responsibility beyond our own actions and decisions to others (or to other forces) is a common societal coping mechanism (Drabek, 1986; Girard, 1989; Arceneaux and Stein, 2006; Lauta, 2014; Straub, 2021; Raju et al., 2022). There are incentives to ignore or improperly value the information that we had that could have led us to take pathways to mitigate or prevent the catastrophes. For example, the reaction of the officials and inhabitants following the flooding of towns built on flood plains is instructive (cf. discussion in Glynn et al., 2022, this issue). Reacting to recent catastrophes (e.g., July 2021 floods in Germany), officials and inhabitants argued that floods recently experienced had never occurred in the history of their town. Is that the right metric to assess the possibility of catastrophic risk? Geological and hydrological processes often operate with timescales and controls distinct from those of lived human experience. But communities and policymakers can control local, regional, and national decisions to build on floodplains, or not; to alter or remove wetlands and other areas that could help absorb flood waters, or not; to drastically increase impermeable surfaces, or not; to cause differential subsidence in the built environment, or not. These controls are ignored, and blame is laid instead on the whims of nature or on climate change (Sterman, 2008; Sterman, 2012; Meyer and Kunreuther, 2018). Relevant and actionable information about the floods, floodplains, and the impacts of human infrastructure is largely ignored with dire societal consequences (Barry, 1997).
The issue of human responsibility for the consequences of human actions was well stated by the philosopher Jean-Jacques Rousseau (Rousseau et al., 1990) in a letter to his contemporary, the philosopher and writer Francois Voltaire, who had written a moving poem following the Lisbon earthquake of 1755 on All Saint’s day (Mendonça et al., 2019):
Moreover, I believe I have shown that with the exception of death, which is an evil almost solely because of the preparations which one makes preceding it, most of our physical ills are still our own work. Without departing from your subject of Lisbon, admit, for example, that nature did not construct twenty thousand houses of six to seven stories there, and that if the inhabitants of this great city had been more equally spread out and more lightly lodged, the damage would have been much less, and perhaps of no account. All would have fled at the first disturbance, and the next day they would have been seen twenty leagues from there, as gay as if nothing had happened; but it is necessary to remain, to be obstinate around some hovels, to expose oneself to new quakes, because what one leaves behind is worth more than what one can bring along.
3.2.4 Value Ascribed Through Stated Preferences or Revealed Preferences (VE9)
The values that we claim to place on information (stated preferences) often differ from the values that we exhibit through our actions (revealed preferences)—and both claims and actions are affected by the presence, size, and nature of the audiences that are watching us, as well as by the communication modes through which the values are expressed (Carson and Hanemann, 2005; Loomis, 2011; Hausman, 2012). Some studies have nonetheless found conditions where stated and revealed preference values agree with each other (Brookshire et al., 2021). These methods are essential to the valuation of non-market goods, such as existence values or environmental preferences. [Non-market goods are goods and services that people consume but that cannot be traded in formal markets. Existence values are a type of intrinsic value or non-use value that reflect the value that people place on knowing that a particular thing (e.g., a species, a culture, a place) exists.]
VOI studies of stated preferences or revealed preferences are useful not only because of their estimates of VOI, but also because they provide insights into the behavioral and societal factors affecting the valuation and use of information. They may therefore help in the effective design of DDPs, and associated policy and government decisions—especially when VOI estimates can be compared across time and decisions, and/or across VOI estimation approaches. In that regard, it is interesting to consider how estimates of the value of Landsat satellite information have evolved. Following an earlier multi-method estimate of Landsat VOI (Miller et al., 2013), Straub et al. (2019) describe how willingness-to-pay (stated preference) varied over time and as a function of Landsat technologies and a diversity of other factors, including the transition of Landsat imagery into the public domain. More recently, Molder et al. (2022) used a qualitative approach to provide a better understanding of the value and value chains for Landsat information. The authors created a mapping of the Landsat data ecosystem that included the diversity of actors and uses of the information, and thereby provided a useful system’s perspective on Landsat VOI.
3.3 Other Forms of Values and Valuations
3.3.1 VOI Assessed Through an Expenditure Investment for Information Production (VE10)
The willingness of an entity or actor to spend funds and invest in producing information may provide an indication of the minimum worth assessed for the production of information by the funders, and/or by the producers and users of information. For example, the sum of the costs of launching and operating a satellite needed to produce useful geospatial information may imply the minimum expected value of the information it produces—over its operational lifetime. Congress—following a possible request from the Office of Management and Budget—may provide the funds for the entire effort, but its assessment of the value proposition probably also receives input from agencies involved in the information production effort (i.e., NASA and USGS in the case of Landsat), as well as past and potential users of the information (e.g., other governmental agencies, private sector entities). This process is part of the Federal Government budget process in the United States; it also frequently involves the Congressional Research Service (Normand, 2021), and accesses information from scientific journals (Wu et al., 2019). Nonetheless as for any social enterprise, BBHV, constituency pressures, power dynamics, and other human modalities may affect decisions regarding an information production investment, and therefore its resulting VOI.
3.3.2 Exchange Values Determined by Commercial or Other Proprietary Societal Ventures (VE11)
Information is sometimes treated and traded as a commodity or material good. This means that access to information—or to its effective use—can be prevented through protection barriers that once in place allow the information (or its effective use) to be excludable and therefore worth trading. In such cases, the value of the information can be estimated from the exchange values determined by the trading entities. A formal market may allow sellers and buyers to set their own exchange values for the information, but this becomes less likely as the number of sellers and buyers increase, as the chance for leakage increases, which would undercut the sellers’ price. More likely, given that information that is sold is often novel and under tight controls by one seller or very few, power asymmetries and other market failures will influence exchange values (McConnell and Brue, 2005). Exchange values for information will also reflect not just the cost of providing the information but can also reflect a value in using the information for given purposes. Consider non-open-source academic publishing, where the publishing house or journal is the only official source for an article that runs $45 or more, but still may not cover the journal’s costs. There is one seller, buyers who must use an original copy, and costs supplemented by unpaid article reviewers. Commercial or other entities providing the marketed information (and assisting with its use) will have made decisions at some point on the worth of investing in obtaining or producing the information (cf. VE10), as well as in managing and controlling access to it, in relation to the potential capital returns to be realized. Human dynamics and perceptions will affect these estimates and business decisions (Kahneman, 2011).
3.3.3 Mis-/Dis-Information and Other Information Communication Pathologies (VE12)
Due to commercial or political self-interests, strongly held beliefs or perceptions, actors often alter, mis-represent, or communicate information that is factually wrong. This means that value is found in the production, communication, and consumption of mis-information or dis-information—whether done willfully or unconsciously (Akerlof and Shiller, 2015). The value of mis-information and dis-information could therefore potentially be assessed by determining market or non-market exchange values, or by assessing the value of the communication enterprises distributing the questionable information, or by assessing the value of the mis-/dis-information in preventing or delaying a negative consequence (e.g., commercial or political) to a given entity or individual.
More generally, the communication of information affects the access to, as well as the useability and actionability of information—and therefore it affects VOI. Various issues and pathologies can potentially affect the transmission and reception of information. The credibility that we assign to information is one factor that affects information communication and use, although credibility may be difficult to assess. Indeed, while we may at times be critical thinkers capable of examining the facts and likelihood of truth in communicated information, we are also sometimes subject to responding to its “truthiness, i.e., a truthful or seemingly truthful quality that is claimed for something not because of supporting facts or evidence but because of a feeling that it is true or a desire for it to be true (Merriam-Webster online definition, Accessed March 16, 2022). Another issue is that regardless of its truth or factual content, information is always competing with other information for attention. Not all information can be absorbed into knowledge, and behavioral processes affect the selection of both what is transmitted and what is received.
Also, information and data usually have associated uncertainty, and measures of error may or may not be communicated or properly interpreted. Information uncertainties generally increase when the information is not the result of direct observation but is instead obtained through transformation and modeling of other data and information (cf. VE3 discussion, and references therein), such as when predictions are given for some future state of the world (e.g., weather predictions). Information that is provided but found erroneous can negatively impact the credibility of the information provider. And a negative judgment on the provider’s credibility may persist and get enshrined in the minds of potential information users, potentially to their detriment if the information providers prove to be more accurate or truthful later on or under different conditions. This reality is highlighted in Aesop’s story of the shepherd who cried “wolf” too many times. Cash et al. (2003) review some of the social and behavioral influences affecting credibility and legitimacy of information sources and uses. Lastly, credibility and perception issues may also skew how information is presented by providers, or the information itself may be deliberately biased as information providers attempt to judge possible consumer reactions. For example, the phenomenon of “wet bias” in weather predictions has been documented and attributed to weather prediction entities (especially commercial ones) wanting to compensate for the greater consequences of not predicting “bad” (i.e., wet) weather relative to those of not predicting “good” (i.e., dry) weather (Silver, 2012).
3.4 Information Useability and Actionability
3.4.1 Value Ascribed Through Statistical Analyses or Other Community or Population Assessments (VE13)
Statistical measures of value, such as the “value of a statistical life” (VSL), are useful at the level of the populations or communities that provide the basis for the statistical distributions and evaluations (Kniesner and Viscusi, 2019). There are many behavioral and social factors that impede the actual use and application of statistical measures, especially by non-experts (Kniesner and Viscusi, 2019; Broughel, 2020). Having to decipher or understand probabilities or statistics is an important barrier to the use of effective information (Hoffrage et al., 2000; Gigerenzer, 2014). The application of community or population statistics to individual, or small-group, or small sample-size situations is also a problem that non-experts have problems dealing with (Hoffrage et al., 2000).
Indeed, individuals and groups within the evaluated communities typically find it difficult to relate the statistical information to their own individual (or small group) “special” situation (Meder and Gigerenzer, 2014). They are more likely to make decisions based on hearing about the experiences of a close friend or family member, or even through the compelling story of an unrelated individual, than through knowledge of a general assessment made at the level of a community or population (Cutting, 1987; Bergin, 2016). The greater the size of the community or population being assessed, the less personal the assessment becomes, and the smaller the perception of relevance (Akerlof, 1997).
It is our nature as individuals and members of given social tribes to believe that we—and our tribes—are exceptional (Akerlof, 1997; Kranton, 2010; Huettel and Kranton, 2012; Sadler-Smith and Akstinaite, 2021). Information that is presented to us, that is de-personalized or not especially reflective of our own lived experiences, identities, and the groups that we associate with is easily discarded or ignored. Statistical measures of value can or could play a role in making decisions for the communities and populations that form the basis of the measures, but those measures will likely be understood, and decisions more effectively made, by people in trusted positions who do understand the relevance of community and population assessments, and their statistical and probabilistic aspects. An ability for trusted people to communicate the reasons clearly and continually for their assessments and how those assessments relate to individual situations will also be important. In essence, this is often what a doctor does in diagnosing and devising treatments for patients: they use general information and assessments and make it individually relevant.
3.4.2 Attention and Resource Needs, and Equity Issues Impacting Valuation and Actionability of Information (VE14)
Failure to manage issues at the needed time can affect the VOI and vice versa—failure to appreciate the VOI can lead to a lack of needed timely action. In determining the VOI, methods are needed that address issues that are relevant when there are resource issues that affect prioritizations. There are cases where associated measures of value and potential risks are available. However, that information, in the forms initially made public or available, may not be understandable or accessible to subgroups within the larger community (Wildavsky and Dake, 1990; Rosenfeld et al., 2021). Davenport and Beck (2001) wrote that “Attention is focused mental engagement on a particular item of information. Items come into our awareness, we attend to a particular item, and then we decide whether to act.”
In any moment, attention is a limited resource (VE4, VE5), and typically attention capacity correlates with measures of social equity (Frederickson et al., 2010) and vulnerability. [Social equity relates to the degree of impartiality, fairness, and justice as applied in social policy.] Those who work in lower income jobs are likely to have fewer resources, and possibly less time to access, interpret, and use information that could benefit their situation and financial resources (Macaulay, 2021). Furthermore, in a knowledge economy it may be the case that the presentation of information does not align well with the knowledge and understanding of those who may have more attention resources. [Powell and Snellman (2004) define the knowledge economy as “production and services based on knowledge-intensive activities that contribute to an accelerated pace of technological and scientific advance as well as equally rapid obsolescence”]. There are many issues that affect the economics of attention (Franck, 2002; Franck, 2016; van Krieken, 2019; Straub, 2021). Default assumptions that socio-economic status may correlate with attention resources are not always correct (Schmitt and Schlatterer, 2021). In our consumer age, attention economics share many of the same properties as market economics. Attention is being sought as well as being consumed, and the nature and type of information and other factors affect “attention transactions” (van Krieken, 2019).
The scope of information and valuation are potentially available; however, individuals or groups may be so constrained in attention or time that they do not have a realistic opportunity to access or elevate the information to a high-enough level of importance to act upon or fully integrate it in their decision making. Producers and users of information may also package it in a manner that is accessible in unfair or unjust ways across subcommunity types (van Krieken, 2019). Resources may not be available for individuals and communities in relatively greater need to understand and access the information. These may present as limitations on time, energy, processing, or other resource-related factors (van Krieken, 2019). Engagement with communities (or subsets of communities) with a variety of resource levels can aid in the development of VOI estimates that accurately reflect the characteristics of the population being addressed (Chiavacci et al., 2020). Information presentation and decision support tools can be used to meet different resource availabilities across community subgroups to facilitate equitable provision of data (Gray et al., 2017; Sterling et al., 2019).
Answering the following questions may help address resource and equity issues (Jordan et al., 2018): What are the characteristics of the population being assessed and the characteristics of the users of the information? What are barriers to access and usability? What are limitations to acting on the information? What decision support tools may facilitate flow of information and provide increased VOI?
3.4.3 The Issue of Dependent Information, and of “Future-Found” Values (VE15)
Information is usually produced, obtained, or used with some purpose (and associated mental or conceptual model) in mind. Sometimes, other possible uses for the information may also be imagined or perhaps less-consciously intuited. Commonly however, information is found—at some point in the future—to have many more uses (and therefore value) than was initially conceived or imagined (Glynn et al., 2022, this issue). This is especially true of information that describes the characteristics of a system or an issue that has broad interest (Straub et al., 2019; Molder et al., 2022). For example, geologic (and associated topographic) information may have initially been generated with the goal of understanding the distribution and economic availability of a particular geological resource (i.e., mineral or energy). But perhaps these maps contain much more information than just the distribution of a single resource. For example, Chiavacci et al. (2020) document the economic value of health benefits resulting from the use of geologic maps as a tool for communicating radon risk potential in Kentucky. The original developers of the geologic maps likely never thought of this possible use. Technology developments and shifting or new societal interests may also play a role in establishing new utilities for the information or combinations of information. For example, societal interest in radon indoor-air concentrations arose after the advent of relatively cheap and accessible monitoring technologies; and after the societal realization that elevated radon levels in air could cause lung cancer.
The full value of collected information can become clear as it is combined with other information. While we can imagine the potential value of some of these combinations, it is quite likely that we often miss some. Our limited cognitive abilities, and our often-skewed perceptions are reasons we often suffer from failures of imagination and perception—and from more optimally deciding what types of information may be more useful or valuable to collect (not just in the present but also over the longer term). Highly general information such as geospatial information (including land imagery and remote sensing) is likely to have many more possible value streams than can be imagined at one moment in time. Additionally, our human ability to discern new and useful combinations of information may soon be assisted by artificial intelligence.
We expect that consideration of behavioral and social factors will continue to be warranted as society develops new sources, types, combinations, and uses of information.
4 Conclusion
Humans are complex social beings. Our ability to discern what information truly matters for decision making is often limited and skewed by a wide diversity of factors. Recognizing this fact—specifically for situations and issues where these factors are important—is a critical first step in addressing these limitations, and in seeking to improve our perceptions, abilities, and decision making.
The expanding complexity of the world and diversity of information sources directly impacts how we use and value information (individually and collectively). The value of the information we are exposed to, as it relates to informing our decisions, is influenced by our biases, beliefs, heuristics, values, influencers around us, and so on. Estimating and understanding the value of information in regards to its useability and actionability brings a need to consider: 1) the different users and applications of the information; 2) the timing of when information might be used relative to when it is available; 3) the controls, social barriers, or filters that affect valuations and useability; and 4) the credibility and trustworthiness of the information, and of the actors involved in information production, communication, and use.
The value of information used in decision making is also of interest to economists and others because information is costly to collect, process, and transform into applicable knowledge. Thus, stakeholders often want to understand if investments in information collection should be undertaken, continued, or ceased, as part of managing a balance of scarce resources. Such situations are where VOI studies can be beneficial: they can help decision makers better understand the role information could potentially play in a decision, or how information influenced an action or outcome.
Traditional economic studies of VOI—which often assume that Homo economicus defines the nature and decision making of human actors—may be appropriate for certain situations and issues for which humans and their communities are well adapted. However, there are many circumstances where human limitations and more complex behaviors and social factors need to be considered. These circumstances are something Homo economicus’s fully rational thinking, at the level of individuals or of communities, fails to properly address. Recognizing this issue, the goal of our paper was to highlight such circumstances and provide thought-provoking questions and examples related to the assessment of VOI.
Table 1 provides a structured summary of the 15 Value Explorations that we discuss. Probing questions are included that may be used to analyze, critique, and improve the design of “data to decision pathways” (DDPs) preceding and following VOI assessments. As discussed here, these pathways, and the useability and actionability of VOI require careful consideration of:
• the treatment, value, and applicable scope of the information;
• the characteristics of the models (mental, conceptual, or numerical) associated with the information;
• the actors involved in providing and consuming the information and models; and
• the decision makers who are expected to be acting on the information and associated models at different points along the “data to decision pathways.”
These elements, and their role in affecting DDPs, are the subject of a further examination in our companion article (Glynn et al., 2022, this issue). We hope that the present article (and Table 1 summary) will provide a basis and impetus for future research into the behavioral and social factors affecting the value and use of information by individuals and collectivities.
Better recognition of when human perceptions, judgments and reactions are well-adapted—and when they are not—will help modern society make more informed and effective use of information in managing itself, and in addressing complex issues, such as those related to natural resources, environments, and natural and anthropogenic hazards. Due to the breadth of scales that it can cover, and to the visual understanding that it provides, geospatial information plays an essential part in this societal evolution. Economic studies, like those exploring VOI, are critical to evaluating the role and importance of information in decision making, as they provide explicit models that can be critiqued—allowing for the iterative, usefully curated, expansion of human and societal understanding. Such studies could become even more beneficial to society through more thorough consideration of the complexities of human nature.
Author Contributions
All authors listed have made a substantial, direct, and intellectual contribution to the work and approved it for publication.
Funding
The authors were supported by funds and resources from their affiliated institutions.
Conflict of Interest
The authors declare that the research was conducted in the absence of any commercial or financial relationships that could be construed as a potential conflict of interest.
Publisher’s Note
All claims expressed in this article are solely those of the authors and do not necessarily represent those of their affiliated organizations, or those of the publisher, the editors and the reviewers. Any product that may be evaluated in this article, or claim that may be made by its manufacturer, is not guaranteed or endorsed by the publisher.
Acknowledgments
Any use of trade, firm, or product names is for descriptive purposes only and does not imply endorsement by the U.S. Government. We owe a debt of gratitude in preparing this manuscript to the insightful comments provided by the two journal reviewers, and also by our colleagues David Butry (NIST), Patricia McKay (MSU), Meredith Nevers (USGS), and Paul White (GNS Science).
References
Akerlof, G. A., and Kranton, R. (2010). Identity Economics. Am. Econ. Rev. 7. doi:10.2202/1553-3832.1762
Akerlof, G. A., and Shiller, R. J. (2015). Phishing for Phools: The Economics of Manipulation and Deception. United States: Princeton University Press.
Akerlof, G. A. (1997). Social Distance and Social Decisions. Econometrica 65, 1005. doi:10.2307/2171877
Akinci, C., and Sadler-Smith, E. (2019). Collective Intuition: Implications for Improved Decision Making and Organizational Learning. Br. J Manage 30, 558–577. doi:10.1111/1467-8551.12269
Anderson, C. A. (1983). Abstract and concrete Data in the Perseverance of Social Theories: When Weak Data lead to Unshakeable Beliefs. J. Exp. Soc. Psychol. 19, 93–108. doi:10.1016/0022-1031(83)90031-8
Anderson, C. A. (1982). Inoculation and Counterexplanation: Debiasing Techniques in the Perseverance of Social Theories. Soc. Cogn. 1, 126–139. doi:10.1521/soco.1982.1.2.126
Anderson, C. A., Lepper, M. R., and Ross, L. (1980). Perseverance of Social Theories: The Role of Explanation in the Persistence of Discredited Information. J. Personal. Soc. Psychol. 39, 1037–1049. doi:10.1037/h0077720
Arceneaux, K., and Stein, R. M. (2006). Who Is Held Responsible when Disaster Strikes? the Attribution of Responsibility for a Natural Disaster in an Urban Election. J. Urban Aff. 28, 43–53. doi:10.1111/j.0735-2166.2006.00258.x
Ariely, D. (2010). Predictably Irrational: The Hidden Forces that Shape Our Decisions. New York, New York, USA: Harper Perennial.
Bacon, F. (1620). “The New Organon (Or True Directions Concerning the Interpretation of Nature),” in eBooks@Adelaide.edu.com (From Taggard and Thompson 1863 Edition) (South Australia: The University of Adelaide). Available at: http://ebooks.adelaide.edu.au/b/bacon/francis/organon/complete.html.
Bagstad, K. J., Ancona, Z. H., Hass, J., Glynn, P. D., Wentland, S., Vardon, M., et al. (2020). Integrating Physical and Economic Data into Experimental Water Accounts for the United States: Lessons and Opportunities. Ecosystem Serv. 45, 101182. doi:10.1016/j.ecoser.2020.101182
Barry, J. M. (1997). Rising Tide: The Great Mississippi Flood of 1927 and How it Changed America. New York: Simon & Schuster.
Bennett, D., Bode, S., Brydevall, M., Warren, H., and Murawski, C. (2016). Intrinsic Valuation of Information in Decision Making under Uncertainty. Plos Comput. Biol. 12, e1005020. doi:10.1371/journal.pcbi.1005020
Bergin, D. A. (2016). Social Influences on Interest. Educ. Psychol. 51, 7–22. doi:10.1080/00461520.2015.1133306
Berlyne, D. E. (1954). A Theory of Human Curiosity. Br. J. PsychologyGeneral Section 45, 180–191. doi:10.1111/j.2044-8295.1954.tb01243.x
Bernknopf, R., Brookshire, D., Kuwayama, Y., Macauley, M., Rodell, M., Thompson, A., et al. (2018). The Value of Remotely Sensed Information: The Case of a GRACE-Enhanced Drought Severity Index. Weather, Clim. Soc. 10, 187–203. doi:10.1175/WCAS-D-16-0044.1
Bernknopf, R., and Shapiro, C. (2015). Economic Assessment of the Use Value of Geospatial Information. Ijgi 4, 1142–1165. doi:10.3390/ijgi4031142
Boissoneault, L. (2019). The Cuyahoga River Caught Fire at Least a Dozen Times, but No One Cared until 1969. Smithsonian Magazine. Available at: https://www.smithsonianmag.com/history/cuyahoga-river-caught-fire-least-dozen-times-no-one-cared-until-1969-180972444/.
Boudon, R. (2003). Beyond Rational Choice Theory. Annu. Rev. Sociol. 29, 1–21. doi:10.1146/annurev.soc.29.010202.100213
Braveman, P., Egerter, S., and Williams, D. R. (2011). The Social Determinants of Health: Coming of Age. Annu. Rev. Public Health 32, 381–398. doi:10.1146/annurev-publhealth-031210-101218
Brookshire, D. S., Thayer, M. A., Schulze, W. D., and Arge, R. C. D. (2021). Valuing Public Goods: A Comparison of Survey and Hedonic Approaches. Available at: https://www.jstor.org/stable/pdf/1808583.pdf.14
Broughel, J. (2020). Rethinking the Value of Life: A Critical Appraisal of the Value of a Statistical Life. Logan, UT: Center for Growth and Opportunity at Utah State University. Available at: https://www.thecgo.org/research/rethinking-the-value-of-life/(Accessed February 22, 2022).
Burby, R. J., and May, P. J. (1999). Making building codes an e!ective tool for earthquake hazard mitigation. Environ. Hazards 11.
Butler, L. J., Scammell, M. K., and Benson, E. B. (2016). The Flint, Michigan, Water Crisis: A Case Study in Regulatory Failure and Environmental Injustice. Environ. Justice 9, 93–97. doi:10.1089/env.2016.0014
Campbell-Arvai, V. (2019). Visits from the Ghost of Disturbance Past: Information about Past Disturbance Influences Lay Judgments of Ecosystems. J. Environ. Manage. 232, 438–444. doi:10.1016/j.jenvman.2018.11.068
Carroll, J., Clasen, M., Jonsson, E., Kratschmer, A. R., McKerracher, L., Riede, F., et al. (2017). Biocultural Theory: The Current State of Knowledge. Evol. Behav. Sci. 11, 1–15. doi:10.1037/ebs0000058
Carson, R. T., and Hanemann, W. M. (2005). “Chapter 17 Contingent Valuation,” in Handbook of Environmental Economics (Elsevier), 821–936. doi:10.1016/S1574-0099(05)02017-6
Cash, D., Clark, W. C., Alcock, F., Dickson, N., Eckley, N., and Jager, J. (2003). Salience, Credibility, Legitimacy and Boundaries: Linking Research, Assessment and Decision Making. Kennedy School of Government Working Papers Series. Boston, MA: SSRN. doi:10.2139/ssrn.372280
Chiavacci, S. J., Shapiro, C. D., Pindilli, E. J., Casey, C. F., Rayens, M. K., Wiggins, A. T., et al. (2020). Economic Valuation of Health Benefits from Using Geologic Data to Communicate Radon Risk Potential. Environ. Health 19, 1. doi:10.1186/s12940-020-00589-8
Choi, E. U., and Hogg, M. A. (2010). Who Do You Think You Are? Ingroup and Outgroup Sources of Identity Validation. J. Theo. Soc. Psychol. 4, 125–134. doi:10.1002/jts5.66
Christie, M., Kenter, J. O., Bullock, C., Bruen, M., Penk, M., Feld, C., et al. (2021). Evaluating the Multiple Values of Nature – ESDecide: From an Ecosystem Services Framework to Application for Integrated Freshwater Resources Management. Wexford, Ireland: Irish Environmental Protection Agency. Available at: www.epa.ie.
Cialdini, R. B., and Goldstein, N. J. (2004). Social Influence: Compliance and Conformity. Annu. Rev. Psychol. 55, 591–621. doi:10.1146/annurev.psych.55.090902.142015
Cialdini, R. B. (2009). Influence: The Psychology of Persuasion. Revised edition. New York, NY: First Collins Business Essentials.
Ciullo, A., Viglione, A., Castellarin, A., Crisci, M., and Di Baldassarre, G. (2017). Socio-hydrological Modelling of Flood-Risk Dynamics: Comparing the Resilience of green and Technological Systems. Hydrological Sci. J. 62, 880–891. doi:10.1080/02626667.2016.1273527
Cosmides, L., Barrett, H. C., and Tooby, J. (2010). . Proc. Natl. Acad. Sci. U S A 107 (Suppl 2), 9007–9014. doi:10.1073/pnas.0914623107
Cutting, J. E. (1987). Perception and Information. Annu. Rev. Psychol. 38, 61–90. doi:10.1146/annurev.ps.38.020187.000425
Damasio, A. R. (1994). Descartes’ Error: Emotion, Reason, and the Human Brain. New York, NY: Putnam.
Davenport, T. H., and Beck, J. C. (2001). The Attention Economy: Understanding the New Currency of Business. Boston, Mass: Harvard Business School Press.
Di Baldassarre, G., Brandimarte, L., and Beven, K. (2016). The Seventh Facet of Uncertainty: Wrong Assumptions, Unknowns and Surprises in the Dynamics of Human-Water Systems. Hydrological Sci. J. 61, 1748–1758. doi:10.1080/02626667.2015.1091460
Di Baldassarre, G., Viglione, A., Carr, G., Kuil, L., Salinas, J. L., and Blöschl, G. (2013). Socio-hydrology: Conceptualising Human-Flood Interactions. Hydrol. Earth Syst. Sci. 17, 3295–3303. doi:10.5194/hess-17-3295-2013
Drabek, T. E. (1986). Human System Responses to Disaster: An Inventory of Sociological Findings. 1st ed. Springer-Verlag.
Elster, J. (1989). Social Norms and Economic Theory. J. Econ. Perspect. 3, 99–117. doi:10.1257/jep.3.4.99
ESA-ESRIN (2019). There Is Value in Earth Observations: Can You Measure it?. Paris, France: European Space Agency. Available at: http://earsc.org/Sebs/workshop-2019.
Everett, J. A. C., Faber, N. S., and Crockett, M. (2015). Preferences and Beliefs in Ingroup Favoritism. Front. Behav. Neurosci. 9. doi:10.3389/fnbeh.2015.00015
Fagin, R., and Halpern, J. Y. (1987). Belief, Awareness, and Limited Reasoning. Artif. Intelligence 34, 39–76. doi:10.1016/0004-3702(87)90003-8
Finocchiaro, M. A. (2010). Defending Copernicus and Galileo: Critical Reasoning in the Two Affairs. Dordrecht: Springer.
Franck, G. (2002). The Scientific Economy of Attention: A Novel Approach to the Collective Rationality of Science. Scientometrics 55, 3–26. doi:10.1023/A:1016059402618
Frank, R. H., and Sunstein, C. R. (2008). Nudge: Improving Decisions about Health, Wealth, and Happiness. New Haven, CT: Yale University Press, 202–208. doi:10.1086/592313
Frederickson, H. G. (2010). Social Equity and Public Administration Origins, Developments, and Applications. New York: Routledge.
Gibbard, A., and Varian, H. R. (1978). Economic Models. J. Philos. 75, 664–677. doi:10.5840/jphil1978751111
Gigerenzer, G., and Brighton, H. (2009). Homo Heuristicus: Why Biased Minds Make Better Inferences. Top. Cogn. Sci. 1, 107–143. doi:10.1111/j.1756-8765.2008.01006.x
Girard, R. (1989). The Scapegoat. Johns Hopkins paperbacks ed. Baltimore: Johns Hopkins University Press.
Glynn, P. D. (2017). “Integrated Environmental Modelling: Human Decisions, Human Challenges,” in Integrated Environmental Modelling to Solve Real World Problems: Methods, Vision and Challenges. Editors A. T. Riddick, H. Kessler, and J. R. A. Giles (London, United Kingdom: Geological Society of London). doi:10.1144/SP408.9
Glynn, P. D., Rhodes, C. R., Chiavacci, S. J., Helgeson, J. F., Shapiro, C. D., and Straub, C. L. (2022). Value of Information and Decision Pathways: Concepts and Case Studies. Front. Environ. Sci. 10, 805214. doi:10.3389/fenvs.2022.805214
Glynn, P. D., Voinov, A. A., Shapiro, C. D., and White, P. A. (2017). From Data to Decisions: Processing Information, Biases, and Beliefs for Improved Management of Natural Resources and Environments. Earth's Future 5, 356–378. doi:10.1002/2016EF000487
Glynn, P. D., Voinov, A. A., Shapiro, C. D., and White, P. A. (2018). Response to Comment by Walker et al. on "From Data to Decisions: Processing Information, Biases, and Beliefs for Improved Management of Natural Resources and Environments". Earth's Future 6, 762–769. doi:10.1002/2018EF000819
Glynn, P. D. (2014). “W(h)ither the Oracle ? Cognitive Biases and Other Human Challenges of Integrated Environmental Modeling,” in 7th Intl. Congress on Env. Modelling and Software. Editors D. P. Ames, N. W. T. Quinn, and A. E. Rizzoli (San Diego: International Environmental Modelling and Software Society), 8. doi:10.13140/2.1.3919.8089
Gray, S., Singer, A., Schmitt-Olabisi, L., Introne, J., and Henderson, J. (2017). Identifying the Causes, Consequences, and Solutions to the Flint Water Crisis through Collaborative Modeling. Environ. Justice 10, 154–161. doi:10.1089/env.2017.0016
Harris, R. (2002). Earth Observation and UK Science Policy. Space Policy 18, 205–213. doi:10.1016/S0265-9646(02)00020-6
Hausman, J. (2012). Contingent Valuation: From Dubious to Hopeless. J. Econ. Perspect. 26, 43–56. doi:10.1257/jep.26.4.43
Hoffrage, U., Lindsey, S., Hertwig, R., and Gigerenzer, G. (2000). Communicating Statistical Information. Science 290, 2261–2262. doi:10.1126/science.290.5500.2261
Howard, R. A. (1968). The Foundations of Decision Analysis. IEEE Trans. Syst. Sci. Cyber. 4, 211–219. doi:10.1109/TSSC.1968.300115
Howard, R. (1966). Information Value Theory. IEEE Trans. Syst. Sci. Cyber. 2, 22–26. doi:10.1109/TSSC.1966.300074
Huettel, S. A., and Kranton, R. E. (2012). Identity Economics and the Brain: Uncovering the Mechanisms of Social Conflict. Phil. Trans. R. Soc. B 367, 680–691. doi:10.1098/rstb.2011.0264
Jones, B., and Rachlin, H. (2006). Social Discounting. Psychol. Sci. 17, 283–286. doi:10.1111/j.1467-9280.2006.01699.x
Jordan, R., Gray, S., Zellner, M., Glynn, P. D., Voinov, A., Hedelin, B., et al. (2018). Twelve Questions for the Participatory Modeling Community. Earth's Future 6, 1046–1057. doi:10.1029/2018EF000841
Kachanoff, F. J., Bigman, Y. E., Kapsaskis, K., and Gray, K. (2020). Measuring Realistic and Symbolic Threats of COVID-19 and Their Unique Impacts on Well-Being and Adherence to Public Health Behaviors. Soc. Psychol. Personal. Sci. 12, 14. doi:10.1177/1948550620931634
Kahneman, D., and Klein, G. (2009). Conditions for Intuitive Expertise: A Failure to Disagree. Am. Psychol. 64, 515–526. doi:10.1037/a0016755
Kahneman, D., and Tversky, A. (1984). Choices, Values, and Frames. Am. Psychol. 39, 341–350. doi:10.1037/0003-066x.39.4.341
Kahneman, D., and Tversky, A. (1979). Prospect Theory: An Analysis of Decision under Risk. Econometrica 47, 263–292. doi:10.2307/1914185
Karlova, N., and Fisher, K. E. (2013). A Social Diffusion Model of Misinformation and Disinformation for Understanding Human Information Behaviour. Inf. Res. 18.
Keisler, J. M., Collier, Z. A., Chu, E., Sinatra, N., and Linkov, I. (2014). Value of Information Analysis: the State of Application. Environ. Syst. Decis. 34, 3–23. doi:10.1007/s10669-013-9439-4
Kenter, J. O., Raymond, C. M., van Riper, C. J., Azzopardi, E., Brear, M. R., Calcagni, F., et al. (2019). Loving the Mess: Navigating Diversity and Conflict in Social Values for Sustainability. Sustain. Sci. 14, 1439–1461. doi:10.1007/s11625-019-00726-4
Kirchgässner, G. (2008). Homo Oeconomicus: The Economic Model of Behaviour and its Applications in Economics and Other Social Sciences. New York, NY: Springer New York. doi:10.1007/978-0-387-72797-4
Kniesner, T. J., and Viscusi, W. K. (2019). “The Value of a Statistical Life,” in Oxford Research Encyclopedia of Economics and Finance (USA: Oxford University Press). doi:10.1093/acrefore/9780190625979.013.138
Kobayashi, K., Ravaioli, S., Baranès, A., Woodford, M., and Gottlieb, J. (2019). Diverse Motives for Human Curiosity. Nat. Hum. Behav. 3, 587–595. doi:10.1038/s41562-019-0589-3
Krings, A., Kornberg, D., and Lane, E. (2019). Organizing under Austerity: How Residents' Concerns Became the Flint Water Crisis. Crit. Sociol. 45, 583–597. doi:10.1177/0896920518757053
Kruglanski, A. W., and Gigerenzer, G. (2011). Intuitive and Deliberate Judgments Are Based on Common Principles. Psychol. Rev. 118, 97–109. doi:10.1037/a0020762
Kuwayama, Y. (2018). The Value of Water Quality: Separating Amenity and Recreational Benefits. Available at: https://ostromworkshop.indiana.edu/pdf/seriespapers/2018spr-colloq/olmstead-paper.pdf.
LaForgia, M., Playford, A., and Gamio, L. (2021). Lax Enforcement Let South Florida Towers Skirt Inspections for Years. New York, NY: The New York Times. Available at: https://www.nytimes.com/2021/07/04/us/south-florida-condo-maintenance-violations.html (Accessed October 27, 2021).
Lauta, K. C. (2014). New Fault Lines? on Responsibility and Disasters. Eur. J. Risk Regul. 5, 137–145. doi:10.1017/s1867299x0000355x
Levitt, S. D., and List, J. A. (2008). Homo Economicus Evolves. Science 319, 909–910. doi:10.1126/science.1153640
Lindner, I., and Strulik, H. (2008). Social Fractionalization, Endogenous Appropriation Norms, and Economic Development. Economica 75, 244–258. doi:10.1111/j.1468-0335.2007.00614.x
Linkov, I., Fox-Lent, C., Keisler, J., Sala, S. D., and Sieweke, J. (2014). Risk and Resilience Lessons from Venice. Environ. Syst. Decis. 34, 378–382. doi:10.1007/s10669-014-9511-8
Locey, M. L., Safin, V., and Rachlin, H. (2013). Social Discounting and the Prisoner's Dilemma Game. J. Exp. Anal. Behav. 99, 85–97. doi:10.1002/jeab.3
Loewenstein, G., and Thaler, R. H. (1989). Anomalies: Intertemporal Choice. J. Econ. Perspect. 3, 181–193. doi:10.1257/jep.3.4.181
Loewenstein, G. (1994). The Psychology of Curiosity: A Review and Reinterpretation. Psychol. Bull. 116, 75–98. doi:10.1037/0033-2909.116.1.75
Loomis, J. (2011). What's to Know about Hypothetical Bias in Stated Preference Valuation Studies? J. Econ. Surv. 25, 363–370. doi:10.1111/j.1467-6419.2010.00675.x
Macaulay, A. (2021). The Attention Trap: Rational Inattention, Inequality, and Fiscal Policy. Eur. Econ. Rev. 135, 103716. doi:10.1016/j.euroecorev.2021.103716
Macauley, M. K. (2006). The Value of Information: Measuring the Contribution of Space-Derived Earth Science Data to Resource Management. Space Policy 22, 274–282. doi:10.1016/j.spacepol.2006.08.003
MacIntyre, A. (2016). Ethics in the Conflicts of Modernity: An Essay on Desire, Practical Reasoning, and Narrative. Cambridge: Cambridge University Press. doi:10.1017/9781316816967Ethics in the Conflicts of Modernity
Marewski, J. N., Gaissmaier, W., and Gigerenzer, G. (2010). Good Judgments Do Not Require Complex Cognition. Cogn. Process. 11, 103–121. doi:10.1007/s10339-009-0337-0
McConnell, C. R., and Brue, S. L. (2005). Microeconomics: Principles, Problems, and Policies. 16th ed. Boston: McGraw-Hill/Irwin.
McDermott, J. F. M. (2015). Perfect Competition, Methodologically Contemplated. J. Post Keynesian Econ. 37, 687–703. doi:10.1080/01603477.2015.1050335
Meder, B., and Gigerenzer, G. (2014). “Statistical Thinking: No One Left behind,” in Probabilistic Thinking Advances in Mathematics Education. Editors E. J. Chernoff, and B. Sriraman (Dordrecht: Springer Netherlands), 127–148. doi:10.1007/978-94-007-7155-0_8
Mendonça, D., Amorim, I., and Kagohara, M. (2019). An Historical Perspective on Community Resilience: The Case of the 1755 Lisbon Earthquake. Int. J. Disaster Risk Reduction 34, 363–374. doi:10.1016/j.ijdrr.2018.12.006
Mercier, H., and Sperber, D. (2017). The enigma of Reason. Cambridge, Massachusetts: Harvard University Press.
Meyer, R., and Kunreuther, H. (2018). An Experimental Analysis of Learning from Experience about Natural-Hazards. Retrieved from: https://repository.upenn.edu/marketing_papers/330.
Miller, H. M., Richardson, L., Koontz, S. R., Loomis, J., and Koontz, L. (2013). Users, Uses, and Value of Landsat Satellite Imagery—Results from the 2012 Survey of Users. Reston, VA: U.S. Geological Survey. doi:10.3133/ofr/20131269
Molder, E. B., Schenkein, S. F., McConnell, A. E., Benedict, K. K., and Straub, C. L. (2022). Landsat Data Ecosystem Case Study: Actor Perceptions of the Use and Value of Landsat. Front. Environ. Sci. 9, 805174. doi:10.3389/fenvs.2021.805174
New, J., Cosmides, L., and Tooby, J. (2007). Category-specific Attention for Animals Reflects Ancestral Priorities, Not Expertise. Proc. Natl. Acad. Sci. U.S.A. 104, 16598–16603. doi:10.1073/pnas.0703913104
Nickerson, R. S. (1998). Confirmation Bias: A Ubiquitous Phenomenon in Many Guises. Rev. Gen. Psychol. 2 (2), 175–220. doi:10.1037/1089-2680.2.2.175
Normand, A. E. (2021). Landsat 9 and the Future of the Sustainable Land Imaging Program. Available at: https://crsreports.congress.gov (Accessed February 11, 2022).
Nowling, W. D., and Seeger, M. W. (2020). Sensemaking and Crisis Revisited: the Failure of Sensemaking during the Flint Water Crisis. J. Appl. Commun. Res. 48, 270–289. doi:10.1080/00909882.2020.1734224
Powell, W. W., and Snellman, K. (2004). The Knowledge Economy. Annu. Rev. Sociol. 30, 199–220. doi:10.1146/annurev.soc.29.010202.100037
Raisamo, R., Rakkolainen, I., Majaranta, P., Salminen, K., Rantala, J., and Farooq, A. (2019). Human Augmentation: Past, Present and Future. Int. J. Human-Computer Stud. 131, 131–143. doi:10.1016/j.ijhcs.2019.05.008
Raju, E., Boyd, E., and Otto, F. (2022). Stop Blaming the Climate for Disasters. Commun. Earth Environ. 3, 1. doi:10.1038/s43247-021-00332-2
Rao, A. R., and Sieben, W. A. (1992). The Effect of Prior Knowledge on price Acceptability and the Type of Information Examined. J. Consumer Res. 19. doi:10.1086/209300
Rathje, S. (2018). Why People Ignore Facts. New York, NY: Psychology Today. Available at: https://www.psychologytoday.com/us/blog/words-matter/201810/why-people-ignore-facts (Accessed January 23, 2022).
Resources for The Future (2022). Value of Science 101: What Is “Value”? Available at: https://www.rff.org/publications/explainers/what-is-value/(Accessed February 13, 2022).
Rittel, H. W. J., and Webber, M. M. (1973). Dilemmas in a General Theory of Planning. Policy Sci 4, 155–169. doi:10.1007/BF01405730
Rosenfeld, D. L., Balcetis, E., Bastian, B., Berkman, E. T., Bosson, J. K., Brannon, T. N., et al. (2021). Psychological Science in the Wake of COVID-19: Social, Methodological, and Metascientific Considerations. Perspect. Psychol. Sci. 17, 311–333. doi:10.1177/1745691621999374
Rousseau, J. J., Masters, R. D., and Kelly, C. (1990). The Collected Writings of Rousseau. New England: Dartmouth College by University Press.
Rubin, V. L. (2019). Disinformation and Misinformation triangle. JD 75, 1013–1034. doi:10.1108/JD-12-2018-0209
Sadler-Smith, E., and Akstinaite, V. (2021). Human Hubris, Anthropogenic Climate Change, and an Environmental Ethic of Humility. Organization & Environment. Thousand Oaks, CA: Sage Publications, 108602662110390. doi:10.1177/10860266211039000
Salazar, G. P., Diaz, A. O., and Lopez, G. G. (2016). Natural Disasters and Poverty: Understanding the Systemic Complexity. Available at: https://proceedings.systemdynamics.org/2016/proceed/papers/P1096.pdf.18
Schmitt, S. Y., and Schlatterer, M. G. (2021). Poverty and Limited Attention. Econ. Hum. Biol. 41, 100987. doi:10.1016/j.ehb.2021.100987
Schreck, P., van Aaken, D., and Homann, K. (2020). "There's Life in the Old Dog yet": The Homo Economicus Model and its Value for Behavioral Ethics. J. Bus Econ. 90, 401–425. doi:10.1007/s11573-019-00964-z
Silver, N. (2012). The Signal and the Noise: Why So Many Predictions Fail -- but Some Don’t. Penguin Press HC.
Simon, H. A. (1971). “Designing Organizations for an Information-Rich World,” in Computers, Communications, and the Public Interest (Baltimore, MD: John Hopkins Press).
Spiegelhalter, D., Pearson, M., and Short, I. (2011). Visualizing Uncertainty about the Future. Science 333, 1393–1400. doi:10.1126/science.1191181
Sterling, E. J., Zellner, M., Jenni, K. E., Leong, K., Glynn, P. D., BenDor, T. K., et al. (2019). Try, Try Again: Lessons Learned from success and Failure in Participatory Modeling. Elementa 7. doi:10.1525/elementa.347
Sterman, J. D. (2008). Risk Communication on Climate: Mental Models and Mass Balance. Science 322, 532–533. doi:10.1126/science.1162574
Sterman, J. D. (2012). “Sustaining Sustainability: Creating a Systems Science in a Fragmented Academy and Polarized World,” in Sustainability Science. Editors M. P. Weinstein, and R. E. Turner (New York, NY: Springer), 21–58. doi:10.1007/978-1-4614-3188-6_2
Stigler, G. J. (1957). Perfect Competition, Historically Contemplated. J. Polit. Economy 65, 1–17. doi:10.1086/257878
Straub, A. M. (2021). "Natural Disasters Don't Kill People, Governments Kill People:" hurricane Maria, Puerto Rico-recreancy, and 'risk Society'. Nat. Hazards 105, 1603–1621. doi:10.1007/s11069-020-04368-z
Straub, C. L., Koontz, S. R., and Loomis, J. B. (2019). Economic Valuation of Landsat Imagery. Reston, VA: U.S. Geological Survey. doi:10.3133/ofr20191112
Tanner, A., Chang, S. E., and Elwood, K. J. (2020). Incorporating Societal Expectations into Seismic Performance Objectives in Building Codes. Earthquake Spectra 36, 2165–2176. doi:10.1177/8755293020919417
Thaler, R. H. (2000). From Homo Economicus to Homo Sapiens. J. Econ. Perspect. 14, 133–141. doi:10.1257/jep.14.1.133
Tversky, A., and Kahneman, D. (1974). Judgment under Uncertainty: Heuristics and Biases. Science 185, 1124–1131. doi:10.1126/science.185.4157.1124
Tversky, A., and Kahneman, D. (1981). The Framing of Decisions and the Psychology of Choice. Science 211, 453–458. doi:10.1126/science.7455683
van Dijk, E., and Zeelenberg, M. (2003). The Discounting of Ambiguous Information in Economic Decision Making. J. Behav. Decis. Making 16, 341–352. doi:10.1002/bdm.450
van Krieken, R. (2019). Georg Franck's 'The Economy of Attention': Mental Capitalism and the Struggle for Attention. J. Sociol. 55, 3–7. doi:10.1177/1440783318812111
Voinov, A., Kolagani, N., McCall, M. K., Glynn, P. D., Kragt, M. E., Ostermann, F. O., et al. (2016). Modelling with Stakeholders - Next Generation. Environ. Model. Softw. 77, 196–220. doi:10.1016/j.envsoft.2015.11.016
Wachinger, G., Renn, O., Begg, C., and Kuhlicke, C. (2013). The Risk Perception Paradox-Implications for Governance and Communication of Natural Hazards. Risk Anal. 33, 1049–1065. doi:10.1111/j.1539-6924.2012.01942.x
Wachinger, G., and Renn, O. (2010). Risk Perception and Natural Hazards. Stuttgart: DIALOGIK Non-Profit Institute for Communication and Cooperative Research. Available at: http://caphaz-net.org/outcomes-results/CapHaz-Net_WP3_Risk-Perception.pdf.
Wardle, C., and Derakhshan, H. (2018). Thinking about ‘information Disorder’: Formats of Misinformation, Disinformation, and Mal-Information. Available at: https://en.unesco.org/node/296051.12
Waring, T. M., and Wood, Z. T. (2021). Long-term Gene-Culture Coevolution and the Human Evolutionary Transition. Proc. R. Soc. B. 288, 20210538. doi:10.1098/rspb.2021.0538
Wildavsky, A., and Dake, K. (1990). Theories of Risk Perception: Who Fears what and Why? Daedalus 119, 41–60. doi:10.2307/20025337
Wu, Z., Snyder, G., Vadnais, C., Arora, R., Babcock, M., Stensaas, G., et al. (2019). User Needs for Future Landsat Missions. Remote Sensing Environ. 231, 111214. doi:10.1016/j.rse.2019.111214
Keywords: value of information, biases, hazards, environmental risks, decisions, natural resources, behavioral economics, wicked problems
Citation: Glynn PD, Chiavacci SJ, Rhodes CR, Helgeson JF, Shapiro CD and Straub CL (2022) Value of Information: Exploring Behavioral and Social Factors. Front. Environ. Sci. 10:805245. doi: 10.3389/fenvs.2022.805245
Received: 30 October 2021; Accepted: 30 March 2022;
Published: 13 May 2022.
Edited by:
Elena Moltchanova, University of Canterbury, New ZealandReviewed by:
Joseph Conran, National Oceanic and Atmospheric Administration (NOAA), United StatesJames Jarvis, Canadian Space Agency, Canada
Copyright © 2022 Glynn, Chiavacci, Rhodes, Helgeson, Shapiro and Straub. This is an open-access article distributed under the terms of the Creative Commons Attribution License (CC BY). The use, distribution or reproduction in other forums is permitted, provided the original author(s) and the copyright owner(s) are credited and that the original publication in this journal is cited, in accordance with accepted academic practice. No use, distribution or reproduction is permitted which does not comply with these terms.
*Correspondence: Pierre D. Glynn, cGdseW5uQHVzZ3MuZ292, cGdseW5uMkBhc3UuZWR1