- Department of the Interior, Office of Policy Analysis, Washington, DC, United States
Wildfire is an integral part of many ecosystems, and wildland fires also have the potential for costly impacts to human health and safety, and damage to structures and natural resources. Public land managers use various strategies for managing landscape conditions that can affect wildfire, broadly: fuel treatment (and other pre-fire risk mitigation), fire suppression, and post-fire landscape rehabilitation. However, with any of these strategies there is considerable uncertainty in the outcomes that managers can obtain, and thus on the societal costs and benefits associated with wildland fire management. Managers address that uncertainty by using available information to inform their strategy choices. The value of information (VOI) to the land manager is defined as the expected gains from improved wildland fire management outcomes that result from using the information. This paper discusses estimating the value of information using two approaches: a microeconomic theoretical approach, and the Bayesian decision-tree approach frequently used in the VOI literature. These approaches could be used for valuing specific pieces of information (with absolute values), and for prioritizing (with relative values) which areas to focus on for future research.
Introduction
Information is valuable when it can reduce risk and improve decision-making. Macauley (2005) notes that value of information (VOI) largely depends on four factors: the decision-maker’s uncertainty; what is at stake with the decision; any (complementary) cost to using the information; and the cost of the next-best substitute for the information.
Wildland fire managers rely on information on fire behavior, fire impacts, and firefighting resources. Wildfire is an integral part of many ecosystems, though wildland fires also have the potential for costly impacts to human health and safety, and can damage structures and natural resources1. Land managers use available information—often subject to uncertainty—and land management strategies such as: deciding where and when to suppress wildfires, and when to use wildfire to meet resource objectives (like maintaining habitat or reducing fuels); rehabilitating burned areas to restore landscapes and avoid further impacts like mudslides or introduction of invasive species; and mitigating risk with fuel treatments to reduce the effects of undesirable fires. Risk mitigation strategies may also encourage private actions, such as maintaining defensible space, avoiding risky behavior, and insuring valuable structures2. Three broad classes of activity—pre-fire risk mitigation, fire suppression, and post-fire rehabilitation—can reduce the costs and maximize benefits associated with wildland fire. Costs include those associated with management activities and those associated with wildfire damages (North et al., 2012; Chung, 2015; Fernandes, 2015; Jones et al., 2017; Kolden, 2019; Hunter and Robles, 2020).
A recurring challenge is developing information on how these activities can best work together, and using this information in multi-objective decision-support systems (Plucinski, 2019). These activities can also interact as substitutes and complements. We currently lack robust information on these interactions, though researchers have started to address these questions (Mercer et al., 2008; Pacheco and Claro, 2018).
VOI analyses are typically conducted ex post, determining how the availability of certain information changed behavior, and valuing a resulting outcome that is more beneficial or less costly. Such an analysis could be performed for information related to wildland fire as well, however this paper discusses an ex-ante framework for valuing information about how management effort affects the costs and benefits of management. This approach can identify the information that would be most valuable for decision-makers to have, indicating where researchers and land managers should focus their efforts.
We start by considering the manager’s actions as an economic-optimization problem. This approach is adapted from the literature on the marginal abatement cost of reducing carbon emissions, as summarized by Grubb et al. (1993). We describe how reducing the uncertainty in the benefits of management creates the potential for efficiency gains. We then apply the Bayesian decision-tree approach frequently used in the VOI literature to explore how managers could prioritize future research to develop the most valuable information. We discuss some options for developing information, and we close by discussing limitations of this approach, and next steps for future research.
Background
Wildfire is a natural feature of many ecosystems. Historically, land managers had a policy of suppressing fires rather than allowing them to burn. During the 1960s forest ecologists demonstrated the importance of fire in allowing fire-adapted plant species to reproduce and sustaining animal populations that depend on these species. Starting in the 1970s suppression policy shifted toward allowing fires to burn when possible, for example in wilderness areas. Nevertheless, the increase in fuels from decades of suppression, along with the spread of urban areas into the “wildland-urban interface” has led to fires of increasing intensity and cost.
Recent fire seasons have been relatively long and intense. For example, the United States entered National Wildfire Preparedness Level 5 on 14 July 2021, the second earliest date on record for reaching this highest level of national fire activity, and remained at this level for 69 days, the longest period on record (USDA 2021).
A fundamental question connected with expenditures of public funds is whether the results are “worth it” for the public. Wildfire management is no exception. Justifications of the large expenditures related to managing and suppressing wildfire typically focus on identifying direct expenditures and avoided costs. In the context of economic analysis, this is an incomplete description of social costs, which would ideally include values for all resources at their opportunity cost. One of the greatest challenges with comparing costs and benefits is in characterizing everything in terms of a common metric, such as dollars, especially as nonmarket goods and services often are difficult to quantify and monetize.
Previous efforts have attempted to estimate costs and avoided losses for certain fires, though decision makers may never have comprehensive, succinct ledger entries to show the economic value of protecting resources like human health, cultural sites, and wildlife habitat. Nevertheless, benefit-cost analysis and cost effectiveness analysis can help inform decision makers about the tradeoffs they face. Moreover, relative valuation (i.e., relative importance and relative extent of high valued resources and assets) has gained widespread acceptance in the risk assessment and fire planning realms (Scott et al., 2013).
The benefits of wildland fire management activities are mostly avoided costs, in terms of monetized damage to “assets” such as property, environmental and cultural resources, and human health3. While most wildfire risk assessment literature separates natural resources from human assets (e.g., Scott et al., 2013) the distinction is somewhat artificial. Assets differ in terms of value, distribution, size, density on the landscape, and susceptibility to damage by fire. As a result, the rational land manager must prioritize activities to minimize loss of value due to wildfire, which may differ from minimizing physical damage due to wildfire. Land managers also face uncertainty regarding the timing, location, and behavior of wildfires, so their objective is minimizing expected losses.
Management activities are costly, and a land manager faces a dynamic constrained minimization problem: choose the optimal levels of effort to minimize expected wildfire-related (value) losses, subject to the budget constraint for management activities. We characterize these activities broadly into pre-fire fuel treatment, fire suppression, and post-fire rehabilitation4. In practice, wildfire-related management activities (fuel treatment, suppression, and rehabilitation) are typically budgeted for and funded individually, rather than in a combined optimization across all three activities. It is also challenging to coordinate the efforts of managers with overlapping and adjacent jurisdictions.
Various management activities can be both substitutes and complements. Studies such as Thompson et al., 2013 and Sanchez et al., 2019 have attempted to assess the interactions among management activities, though information on these interactions remains limited. Houtman et al. (2013) examined how not suppressing a fire can reduce fuel loads and suppression costs for subsequent fires. Hand et al. (2014) note that early research into fire expenditures focused on the optimal level for suppression once given a set budget for “pre-suppression” (procuring and maintaining equipment). Fried et al., 2006 examined pre-positioning suppression resources to minimize wildfire damage and suppression spending. To our knowledge, there is no current approach dynamically optimizing simultaneously across all three management activities. Optimizing over these activities separately (or not at all) likely results in a suboptimal (i.e., more costly) outcome: excessive wildfire-related losses for a given level of management spending, or equivalently, excessive management expense to limit wildfire-related losses to a given level. There are also constraints on management activities, for example by the time needed to implement treatments (which includes time for planning, NEPA compliance, securing funding, adding personnel) and the availability of suppression resources.
Literature Review
Costs and Benefits of Wildland Fire Management
Since 1975, agencies with fire protection responsibilities have been called on to improve the economic efficiency of their fire management programs (Gonzalez-Caban et al., 1984). Economic analyses of fire management over the years have sought to balance the marginal cost of treatment with the marginal benefits to be gained. A central challenge is measuring society’s full valuation of resources at risk of fire. Even if values are quantifiable, there is considerable uncertainty as to how potential losses respond to various wildfire management options (Gorte and Gorte, 1979; Rhodes and Baker, 2008; Barnett et al., 2016; Ager et al., 2018). An additional challenge lies in balancing the tradeoffs inherent in managing all fire-prone lands, as when treatments to reduce fire threat also impact natural resources nearby (e.g., wildlife, water bodies) and farther off (e.g., potentially harmful smoke from prescribed burns traveling to undesired areas).
A number of authors have attempted to measure the costs and benefits of wildland fire management. Simard (1976) argues that at no time in fire management history has the importance of losses and costs been overlooked, citing Pinchot (1903)’s discussion of wildfire-related human and economic losses, and Greeley (1911)’s discussion balancing fire risks and property values (Pinchot 1903; Greeley 1911; Simard 1976). Early modeling of benefits and costs related to wildfire include Sparhawk (1925), Hornby (1936), and Headley (1943). A survey of these early studies is provided by Gorte and Gorte (1979), who found that early writers developed a “least-cost-plus-loss” model, positing an optimal level of fire management effort that minimizes the sum of firefighting costs and fire-related damages. The actual choice variable differed by author: Sparhawk (1925) used pre-suppression costs, Hornby (1936) used acres burned, Rideout et al. (2008), Rideout and Pamela, (2008) used attack time and attribute-based methods (ABM) for fire management, and Simard (1976) used fire management effort. Sparhawk (1925) identified a range of “indirect” resource values, including watersheds, soils, recreation, and wildlife. However, for analysis, Sparhawk (1925) tallied only the stumpage value of the timber, citing the “paucity of data” and his estimation that “such damage is less than the probable error in estimating damage to timber.” Since those early efforts, newer techniques have been used to value these indirect resource values and there is growing recognition that non-timber values dominate the economic losses from wildfire. Contingent valuation (CV) has been used to value outdoor recreation resources affected by fire, for example, by Hesseln et al. (2004), Loomis et al. (2001), Loomis and Richardson 2001.
The least-cost-plus-loss approach has since been refined in a variety of ways. For example, Donovan and Rideout (2003) address a long-standing issue with the “cost plus net value change” (C + NVC) approach, allowing independent levels of pre-suppression and suppression effort, and modeling their dependence via a net cost function (costs minus benefits). Mason et al. (2006) considered the specific costs and benefits related to fuel removals for forest fire prevention. Houtman et al. (2013) used the least-cost- plus-net-value-change model to find that allowing a fire to burn reduces fuels and suppression costs for future fires in the same area5. Bayham and Yoder (2020) used an econometric model of suppression-resource allocation and combined their results with projected housing growth estimates in California to show that suppression expenditures may rise by nearly $24 million per year. Bowman et al. (2013) argue for more comprehensive models accounting for the complexity of wildland fire, offering pyrogeography as a framework to consider the atmospheric, biological, and socioeconomic dimensions of wildland fire. The authors state that more data on the costs and benefits of different approaches to fire management are required for such a framework to identify optimal wildland fire management solutions.
Calkin et al. (2010) and Bowman (2018) discuss some of the manager’s information challenges and needs. For example, there are challenges in acquiring and using local data for national analysis purposes and management applications. Data on resources that might be burned (e.g., threatened and endangered species habitat, natural and cultural resources, energy resources, and valuable structures) are often incomplete., though there has been progress in this area6. Data standards, types, and completeness vary or may conflict from one entity or level of government to another. The Wildland Fire Decision Support System (WFDSS) is an interagency, web-based application that helps agency administrators and fire managers make risk-informed decisions for all types of wildland fires. WFDSS integrates various applications used to manage fire incidents into a single system, assisting fire managers and analysts in making and documenting strategic and tactical decisions7
Categorizing Costs and Benefits
Federal agencies do not comprehensively track wildland fire cost data. Previous research estimating the economic costs of wildfires (i.e., costs beyond direct budget costs of suppression and rehabilitation) is limited and does not allow for a systematic and consistent comparison of results (Bowman 2018)8. Federal agencies can use regional Stratified Cost Index models (Gebert et al., 2007) to predict (suppression) cost for a given fire based on data from past fires: acres burned, fuel type, other fire characteristics, and values at risk (housing, wilderness). Some authors have used case studies to estimate wildfire losses for specific wildfire incidents, while other studies have used data on insurance losses to estimate wildfire costs (Lynch, 2004; Calkin et al., 2014). Gebert and Black (2012) assessed the effect of different federal wildland fire management objectives and strategies on expenditures. Analyses of 1,330 US Forest Service and US Department of Interior fires from 2006 to 2008 indicate that management objectives and strategies do affect costs, but the results vary both by agency and by the cost metric used. Although most of this research is based on case studies, a few studies have produced estimates at a regional or national level (Young et al., 2019; Wang et al., 2021).
Much of the literature is focused on understanding wildfire occurrence (e.g., likelihood of ignition and wildfire extent). The National Institute of Standards and Technology (NIST, 2017) summarized assessed losses and costs from a sample of wildland fire literature, and estimated total annualized wildfire costs and losses at $71-$348 billion (NIST 2017). Yale University’s Global Institute of Sustainable Forestry (Morton et al., 2003) assessed costs and losses resulting from 10 selected wildfires and found that although the majority of wildfire impacts are negative, positive aspects include improved habitat for certain species and short-term economic impacts from wildfire suppression activities. Colavito et al. (2021) presented an approach for estimating the full cost of a fire, including costs to government agencies, cost of cleanup, loss of life, loss in property value and personal property, structural damage, fire evacuation costs, value of unpaid labor, armoring, and the value of habitat. Zybach et al. (2009) developed a comprehensive ledger to collect information about fire costs and damages, and found that the US Fire Service’s suppression costs account for no more than 10% of C + NVC totals. The remainder includes other expenses, preparedness measures, reduced aesthetics, and long-term losses to society. In evaluating the benefits and costs associated with health effects, the U.S. Environmental Protection Agency (EPA) has used a value of $7.4 million (in $2006) for the value of a “statistical life” (EPA 2014). Mason et al. (2006) report an average of 4.8 deaths per million acres of wildland fires over the 1990s. This implies a value of avoided fatalities in the range of $5 to $10 per acre (Mason et al., 2006).
Economic Optimization Framework
In this section we consider the manager’s actions as an economic-optimization problem. This approach is adapted from the literature on marginal abatement costs of reducing carbon emissions, as summarized by Grubb et al. (1993). We describe how reduced management uncertainty creates the potential for efficiency gains. The remaining sections explore how those potential gains can be used to estimate the value of information on the benefits of management.
Stating the Problem
We consider a single land manager (with no possibilities of coordinating with other landowners) choosing a level of generic management “effort” to minimize total costs (C + NVC) for a single acre, subject to a budget constraint. For simplicity, we frame the problem in a static context and do not address the dynamic nature of the problem9.
Land managers have a variety of decisions, as well as limited information, when faced with the possibility of a wildfire. At a high level, these decisions include determining the level of suppression, whether to implement fuels treatments, and the scope and magnitude of post fire rehabilitation. Often these decisions are made by different agents acting independently of each other. Better outcomes (e.g., lower losses to society) may result from considering interactions among these decisions, that is, if the decisions about each management strategy were made together based on information about effectiveness and cost. The behavioral response of land managers is an important part of the problem which we do not attempt to explicitly model in this paper.
The landowner’s problem can be stated as:
subject to C(E) ≤ F, where
1) TC is Total Cost;
2) E is the level of management effort, which we define to include fuel treatment, fire suppression, post-fire rehabilitation, and risk-mitigation activities. Effort can encompass the use of wildfire to meet management objectives like fuel reduction;
3) C is the (budgetary) cost of management effort, assumed to increase with E (we assume C is deterministic);
4) NVC is the “net value change”: wildfire-related damages, i.e., reduced value of “assets” (structures, resources, human health, etc.) net of (ecological) benefits. We assume that NVC decreases with E: management effort can reduce damages and increase benefits. Given conditions expected to hold in the near term (high fuel loads, expanding WUI, warming climate), we assume that for low levels of effort, damages exceed benefits, while at higher levels of effort benefits exceed damages. We assume that NVC is probabilistic, with an uncertain response to changes in E;
5) I is fire intensity and extent, which may be affected by management effort (e.g., fuel treatments, suppression); and
6) F is the available funding, that is the manager’s budget. In our stylized formulation of the problem, there is a single budget constraint for all types of management effort, though there may separate budget constraints for the various forms of effort.
The management costs, C, are the costs for applying management “effort,” which may include carrying out fuel treatment, fire suppression, and burned-area rehabilitation10. Net value change, NVC or net wildfire-related losses, reflects the monetized effects of wildfire on “asset values”: property, buildings, and infrastructure; natural resources such as areas for recreation, cultural practices and resources, wildlife habitat, and commercial harvest; and human health and safety (i.e., costs of evacuation, health impacts from smoke, and wildfire-related injuries). The “net” part of NVC reflects the subtracting out the various benefits of wildfire, e.g., clearing brush, aiding in forest and grassland regeneration, etc.
The TC function is an envelope curve that combines costs of management actions (the C in C + NVC) and damages (NVC). This “cost plus net value change” (C + NVC) approach is similar to the approach taken by Donovan and Rideout (2003). They make the point that “pre-suppression and suppression should be modeled as independent inputs…related through the NVC function.” For simplicity we do not distinguish between pre-suppression and suppression, instead using the generic variable “effort.” Similarly, “effort” also includes post-fire rehabilitation. We assume that we can derive a “unit cost” for effort: e.g., a cost per acre or person-hour of carrying out fuel treatment, suppression, or rehabilitation activity11.
The loss or damage function is a key component of our model. In our framework, the losses are a function of the Suppression (S), Treatment (T), and Rehabilitation (R), and exogenous factors (α) that are outside control of the manager, where losses, L, are defined as:
The partial derivatives of this function show how changing the level of “effort” for each management action will affect the L. The other components of the total cost function are the costs associated with the management activities. These costs are conceptually much more straightforward. However, especially for suppression costs it is not clear the budget constraint is binding, since land managers may be able to draw on additional resources to suppress a given wildfire. Also, budgets for rehabilitation often depend on the magnitude of damages.
Ideally, the manager would choose the optimal level of effort, E*, such that the marginal cost of the last “unit” of effort is exactly offset by the marginal benefit of that last unit12. Applying a level of effort greater than E* would lead to higher costs that are not fully offset by additional benefits. Conversely, applying a level of effort less than E* would mean failing to realize some of the potential benefits of management.
Differentiating (1) with respect to E gives the first-order condition for this cost minimization:
As NVC is equivalent to wildfire damages minus benefits, –NVC(E) is the reverse: benefits minus damages, or net benefits. Setting–NCV’(E) equal to B(E), we can rewrite (2) as
We assume that the manager has full information on the budgetary cost of effort (C), and that there is uncertainty related to the benefits of effort (B). In particular, the manager may over- or underestimate the effectiveness of effort. Stated another way, the manager may under- or overestimate the amount of effort required to achieve a given level of net benefits. We discuss the probabilities of over-or underapplying effort in the section below on the formalized VOI framework.
Graphical Representation
Figures 1, 2 provide a graphical representation of the problem in a static framework. Figure 1 show total benefits and costs. Figure 2 shows marginal benefits and marginal costs. The solid-line curves indicate the “true” benefit and cost functions, which the manager knows only under the full-information scenario. We assume that the manager has full information on costs, and imperfect information on benefits, though both benefits and costs could be subject to uncertainty.
In the low-information scenario, the manager will over- or underestimate the benefit function. These possibilities are shown in Figure 1 as dashed curves, the “anticipated” or uncertain benefits. The vertical position of the benefits curves is determined by exogenous shifters related to fire-behavior and inventories of resources. For example, a given level of effort E will result in higher benefits for more easily managed fires, or lower benefits for more difficult-to-manage fires. Likewise, a given level of effort E will result in higher benefits in areas with higher-valued resources, and lower benefits in areas with lower-valued resources.
The manager with full information would achieve the optimal level of benefits B* by applying E* level of effort (point c). If imperfect information causes the manager to overestimate the effectiveness of treatment, they would under-apply effort. Thinking that B0,L is the true benefit function, to target the same level B*, this manager would apply effort of EL (point b), and the result would be BL, below B* (point e). Likewise, if imperfect information causes the manager to underestimate the effectiveness of treatment, they would over-apply effort. Thinking that B0,H is the true benefit function, to target the same level B*, this manager would apply effort of EH (point d), and the result would be BH, above B* (point a).
Figure 2 shows marginal cost and benefit functions, the slopes of the curves from Figure 1. Over- or under applying effort is costly, and additional information about the true benefit function would reduce the deviation from E*. The value of this additional information is demonstrated by the shaded areas A and B in Figure 2, which tally the total cost of over- or under-applying effort13. The total cost is a weighted average of these two areas, with the weights equal to the probability of the manager choosing EL or EH. The magnitude of potential efficiency losses is governed by the position and slopes of the marginal benefit and cost curves. The steepness of the curves reflects willingness to pay for increments of benefits (avoided costs). Obtaining additional information about the benefit curves will move the dashed curves closer to the solid curves, reducing the efficiency losses14.
Note that even with full information on the effect of management effort, there would still be some stochastic variation in the benefit function related to unpredictable variables like the weather. For simplicity the graphs do not show this potential variation in the benefits function.
The potential for reducing total costs (wildfire-related losses plus management costs) is related to the value of improved information on the interactions among the three areas of wildland fire management. Additional information about any of these interactions can improve decision-making about management activities. Hence, improved decisions would potentially lower C + NVC; it is this value differential that facilitates valuing information. Perfect information on all interactions would allow the manager to choose the cost-minimizing optimum, if all other issues were fully addressed (e.g., adopting a global process for making funding decisions).
We have described the value of information problem in a static setting. However, the problem is really a dynamic problem. The dynamic optimization problem requires specifying the various components of effort (treatment, suppression, and rehabilitation), say as T, S, and R, and identifying an optimal time path for T, S, and R. Solving the dynamic optimization problem would involve balancing the costs of changing each management strategy (T, S, and R) against the future benefits of changes. The first order conditions for a solution (as in Eq. 3) would involve setting the partial derivatives of the objective function equal to each other.
Value of Information Framework
The value of information (VOI) is defined as the expected gains from making more optimal decisions, as a result of acquiring additional information in the presence of uncertainty. There is an extensive literature on the value of information (Kuhn, 1953; Bratvold, 2009; Forney et al., 2012; NASA, 2013; Bernknopf et al., 2020, 2021a, 2021b). The analytical framework for this approach relies on Bayesian decision-tree analysis, along with various market- and non-market techniques used to monetize the outcomes. The value of information is given by the difference in the values between (1) the “without information” or “low information” and (2) “full information” (or at least “improved information”) cases. In our case, the difference is the change in total costs with and without additional information. NASA (2013) lists three requirements for information to have economic value: 1) there must be a linkage between the applied sciences information, a decision, an action, and a socially valuable set of outcomes or benefits; and 2) the socially valuable benefits must be measurable in some meaningful way and must differ depending on which program action is taken; and 3) information must have the potential to cause a different decision alternative to be chosen. To motivate what follows in this section, we start by providing a brief summary of the theoretical framework described in Bernknopf et al. (2020), illustrated by an example.
A Stylized Example: Deciding Where to Apply Fire-retardant
Consider a land manager who has signed a $10,000 contract to apply fire retardant today to an area to the north of a nearby fire15. If the fire is expected to spread to the north, the manager has no reason to alter the contract. However, if the fire changes course, the manager would be faced with either changing the contract or spending another $10,000 to apply retardant to a different area16. Assume the manager could pay a lower change-fee of $5,000 to reschedule and reroute the contractor. If the manager believes a change in the fire’s course is sufficiently unlikely (say 40%), they will be willing to risk applying the retardant today. If they believe a change in the fire’s course is sufficiently likely (say 60%), they will reschedule.
The threshold for “sufficiently likely” depends on the ratio of the costs of the two options:
A) Pay $15,000 (with certainty): $10,000 for the contracted service, plus $5,000 to reschedule and reroute the retardant (though there is no guarantee that the fire will continue in the new direction); or
B) Pay an uncertain amount if they apply retardant today: $10,000 for the original contract (if the fire holds course), or $20,000 if the fire changes course and they must reapply elsewhere tomorrow.
If the manager believes that the chance of the fire changing course is less than 50%, then the expected cost of Option B is less than $15,000, and a risk-neutral group would choose to proceed with applying retardant today17. For example, if the manager believes that the chance of a course-change is 40% and no course-change is 60%, the expected cost of applying retardant today would be 60% x $10,000 + 40% x $20,000 = $14,000.
Another way to avoid the uncertainty would be for the manager to buy a perfectly accurate fire forecast for tomorrow (if such a forecast were possible). Thus, a third, theoretical option is:
C) Buy a fire forecast that will accurately predict where a fire will spread tomorrow, and then:
1) if the fire is forecast to change course, reschedule and reroute (at a cost of $15,000); or
2) if the fire is forecast not to change course, proceed with applying retardant today (at a cost of $10,000).
If there truly is a 40% chance of a course-change tomorrow, there is a 40% chance that the fire forecast will advise rescheduling, and a 60% chance that the forecast will advise proceeding. The expected cost of Option C would be 40% x $15,000 + 60% x $10,000 = $12,000 (plus the cost of the forecast)18. A risk-neutral manager would prefer Option C to Option B. In fact, Option C would save the manager $2,000 over Option B, so they would be willing to pay up to $2,000 for this information. As discussed in Macauley (2005), this value of information varies with the probability of the fire changing course. This example illustrates the basis for VOI.
The Formalized Value of Information Framework
This framework can be formalized as in Bernknopf et al. (2020). Following Bernknopf’s notation, the land manager must make a decision (e.g., how much retardant to apply in a given area) that involves a random outcome, X (e.g., the effectiveness of fuel treatments, suppression, or rehabilitation for avoiding damage to resources in that area). Referring to our example in the previous section, X represents the effectiveness of retardant in protecting resource values in “the north”. X is uncertain in part due to incomplete information about where the fire is going to go (stochastic variation also plays a role, e.g., interactions between fire and weather, fire behavior, etc.). Prior to receiving additional information, the manager believes that the probability of X taking on the value x (e.g., retardant will be “highly” effective in protecting resources in an area to the north) is equal to (x). This is the manager’s prior belief about the probability that X = x.
If there is a correlated random variable Y (e.g., the direction of fire spread), and the manager receives some new information that Y has taken on the value y (e.g., an accurate forecast that the fire will spread to the north), we can use Bayes’ theorem to express the probability of X = x occurring, given that Y = y (i.e., the probability of retardant being “highly” effective in protecting resources in the north, given that the fire is spreading to the north):
Let d be the level of management effort (e.g., retardant) the manager chooses to apply. Before receiving information about the direction of fire spread, the land manager’s prior belief about net benefits is equal to:
Given these expectations, it is rational for the land manager to choose the decision d0 that maximizes expected net benefits (or minimizes expected net loss):
New information on the value of y (the accurate forecast of the direction of fire spread) may allow the land manager to adjust the level of retardant useThe manager’s posterior belief about net benefits, incorporating the (now known) value of y, is equal to:
In this case, the land manager will choose the decision dy such that
We expect the benefits to increase with the availability of the new information (y). The expected value of information is equal to this increase:
The VOI framework can reveal the extent to which improved information is valuable. Information can influence management actions (i.e., amount and location of fuel treatments, fire suppression, and post-fire rehabilitation), and these management actions affect the probability and extent of losses from wildfire. Managers could use this information to decide how much “effort” to expend on management actions, potentially resulting in lower losses (or lower management costs, or both). Information on how management actions affect the probability and extent of losses would allow managers to adjust the level and distribution of effort across potential management activities.
Information Required to Solve the Land Manager’s Optimization Problem
The key information for the manager’s problem developed above (Eq. 1) is the parameterization of the loss function, and how this function responds to different allocations of effort across the management activities. Specifically, one would need a joint damage (loss) function for fuel treatment, suppression, and rehabilitation. We posit that this function is a smooth function (like the MB curve in Figure 2), with damages decreasing as additional resources (i.e., effort) are applied, up to a point when additional resources do not mitigate additional damages. A full treatment of the problem would account for all major sources of uncertainty for this function; this is beyond the scope of this paper.
The information we seek to value is data on how management actions (fuel management, suppression, rehabilitation) affect the expected value of fire-related losses. The information includes the direct and indirect effects of each management activity19. Given enough data, one would be able to trace out or approximate the damage function with respect to each of the inputs, and identify the optimal levels of inputs to apply to a given wildfire. In the context of the static model presented in Figures 1, 2, information is needed on the position and slopes of the Marginal Benefit (MB) curves. Land management agencies currently collect some of the necessary data (though agency budgets constrain management actions), and these could be used to approximate the damage function20. At least in concept, land managers would be willing to pay to obtain more comprehensive data if such data could reduce total costs to society21.
Proximity Effects and Coordinated Management Activities
A related concept is “proximity effects”: suppressing a wildfire in one area reduces the risk of the wildfire spreading to adjacent areas; or implementing a fuels management project in one area may have some spillover effects for adjacent areas (Thompson et al., 2013) 22. Coordination across land ownerships will affect the slope and shape of the damage function. In our simple formulation of the single landowner’s management problem, we formalize coordination as an exogenous factor that scales the damage functions. As an extension of our approach, one could model the manager’s decisions in a game-theoretic framework. This could be either a non-cooperative or cooperative game.
Finding the Value of Information
As discussed above, VOI is found as the expected difference in valuation between outcomes with and without information:
Figure 3 illustrates the concept applied to a single-variable example of optimizing wildland fire management. The lower (green) portion of the figure illustrates the expected value under the full-information scenario: with full (improved) information on how effort affects total costs (C + NVC), the manager chooses the optimal level of effort, E*, minimizing total costs (C + NVC). In contrast, the upper (blue) portion of the figure illustrates the low-information scenario: with imperfect information about the expected effect of effort, the manager either applies too much effort (i.e., incurring overly high management costs) or too little effort (i.e., incurring overly high losses from fire). The expected value of total costs under the low-information scenario is the weighted average of these two possibilities, with the weights being the likelihood of the manager over- or under-applying effort.
VOI is given by the difference between the (expected) total cost under the low-information scenario, and the total cost under the full-information scenario:
Given that with imperfect information either suppression costs or fire-related losses (or both) will be higher than optimal, in this setup VOI is greater than zero. Without full information, the manager must guess at the right level of effort to apply. The farther this guess could potentially be from the optimal level, the larger the VOI23.
Table 1 provides more detail on the conceptual framework presented in Figure 3. As before, the manager’s decision is the level of management effort to provide. As shown in the first column “Inputs”, the manager makes this decision based on available information, including available inventories of resources, relative valuation for resources at risk, fire-related risks (ignition, fire spread, damage to resources), and effectiveness of (and interactions among) management actions. As before, the level of available information varies from “low” to “full”.
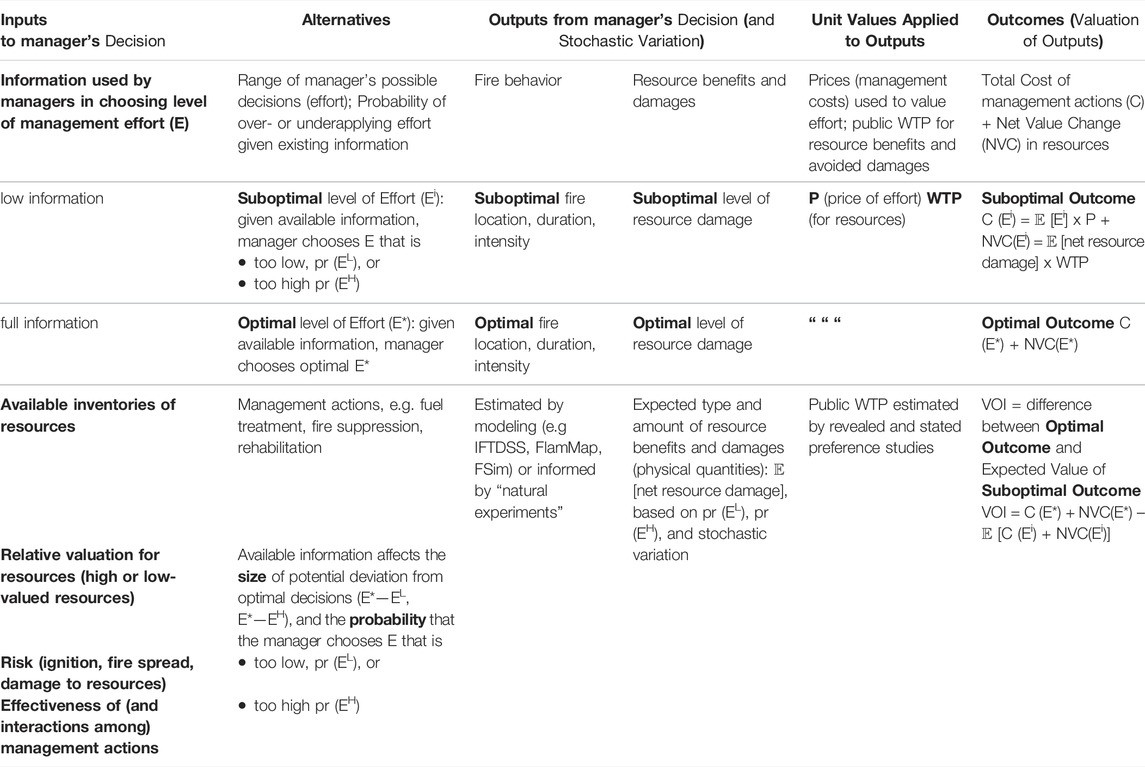
TABLE 1. Conceptual components of VOI for a wildland fire manager’s decision (level of management effort).
In the second column “Alternatives”, we see how the available information affects the manager’s decision: with full information they choose the optimal level of effort (E*); otherwise they choose a suboptimal level of effort that is either too low (EL) or too high (EH). Available information can affect how far off these suboptimal levels are from E*. Available information also affects probability of under- or over-applying effort.
The third column “Outputs” captures what happens on the landscape as a result of the manager’s choice. Fire behavior (e.g., location, duration, intensity) responds to management effort (as well as to stochastic variations like weather). Fire behavior in turn affects the fate of resources on the landscape, and these will either end up in their “optimal” state (the best that can be achieved, assuming optimal management of fire’s beneficial and harmful effects) or a suboptimal state. In this framework, the fate of these resources is determined by the available information driving management decisions (along with stochastic interactions).
The columns described so far sketch out how the information available to fire-managers affects the state of resources on the landscape. The remaining two columns value that information. The “Outcomes” column applies the unit cost of management effort (e.g., the cost of hiring a tanker for a day) from the “Unit Values” column to the level of effort the manager chose in the “Alternatives” column (e.g., the total number of tanker-days). This gives the total cost for the chosen level of effort, the “C” in the C + NVC framework. This column also applies willingness-to-pay values in the “Unit Values” column to the state of the resources determined in the “Outputs” column, giving the public’s value for the resulting state of the resources. As in the other columns, these values are affected by available information: “full” information leads to the “optimal” outcome (with the highest net value), and “low” information leads to a “suboptimal” outcome (with lower net value). Note that the suboptimal outcome is a result of the manager choosing too much or too little management effort, and the expected value of the suboptimal C + NVC depends on the probability of the manager over- or underapplying effort. As noted in the “Alternatives” column, these probabilities are affected by the available information.
Thus the existence of a difference between the optimal and suboptimal outcomes in the rightmost column is the result of a difference in available information. The dollar value of this difference in outcomes gives us the value of the differential information.
Examples of Using the Framework to Estimate Value of Information
This section considers the probability of the manager over- and under-applying effort. The framework to consider this question involves modeling the outcomes of past fires to explore alternative management scenarios. While this approach is beyond the scope of this paper we provide some examples of how a future. researcher might proceed. Conceptually, an alternative approach could involve conducting landscape-scale and long-duration fire simulations to estimate the benefits of management effort (suppression, fuels treatment, rehabilitation). Future work could take advantage of more sophisticated models better suited to the task than the examples provided here.
A Fire-suppression Example
Modeling can inform how varying the levels and type of fire suppression activities affects fire perimeter or intensity. Repeated modeling of different fire events could inform an aggregate or general wildland fire damage function. For example, Figure 4 shows the results of using FSPro24 to model the effect of suppression on the Indiahoma Wye fire, an F-class fire25 that burned 2,621 acres in Oklahoma in 2011. The map shows the possible extent of the Indiahoma Wye fire in the absence of suppression, with areas color-coded by probability range.
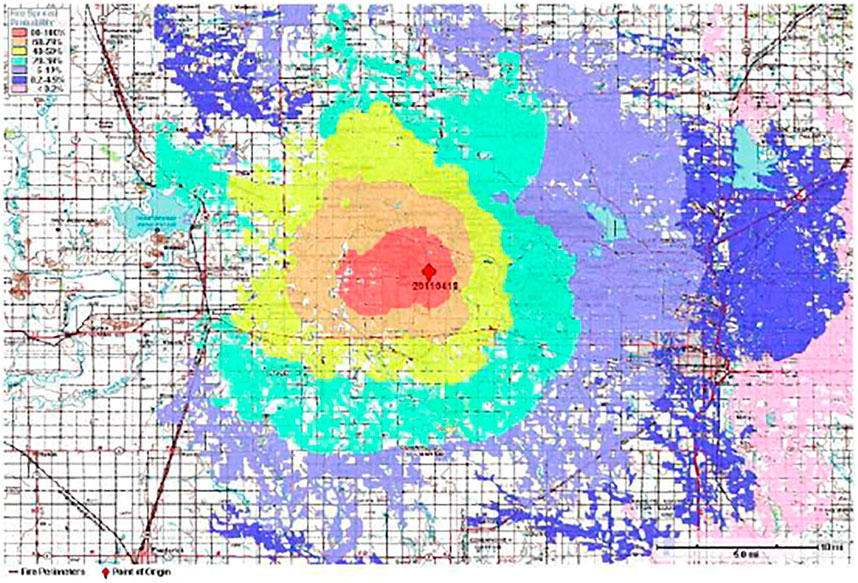
FIGURE 4. Probabilistic fire perimeter under no-suppression scenario (Indiahoma Wye Fire, 2011) (source: FSPro output by Erin Noonan-Wright, U.S. Forest Service, Rocky Mountain Research Station, 2016).
Valuing the damage to assets within this perimeter would provide one point on the “suppression effort” damage function for this fire. Additional modeling (to examine different levels of suppression effort) could allow an analyst to sketch out additional points along the Indiahoma Wye suppression-effort damage function.
Once the analyst has developed the damage function, they could assess whether managers over- or under-applied suppression effort for this fire. Repeating this exercise for additional fires would allow the researcher to build up a profile of characteristics that lead to over- or under-suppression. This would inform the probabilities described in previous sections (e.g., see Figure 3), and allow the researcher to estimate the VOI of additional information.
A Fuel-Treatment Example
An analyst could likewise use modeling to obtain additional information on the potential effects of fuel treatment on fire behavior. This section describes using the Near-Term Fire Behavior (NTFB) model26 to examine the effect of fuel treatments. Future research might instead rely on more recent tools, such as IFTDSS27 or FSim28 for fuel treatment planning, though the concept would be similar. Figure 5 shows the result of using NTFB to model the Garden Creek fire, which burned 2,052 acres in Montana in 2018.
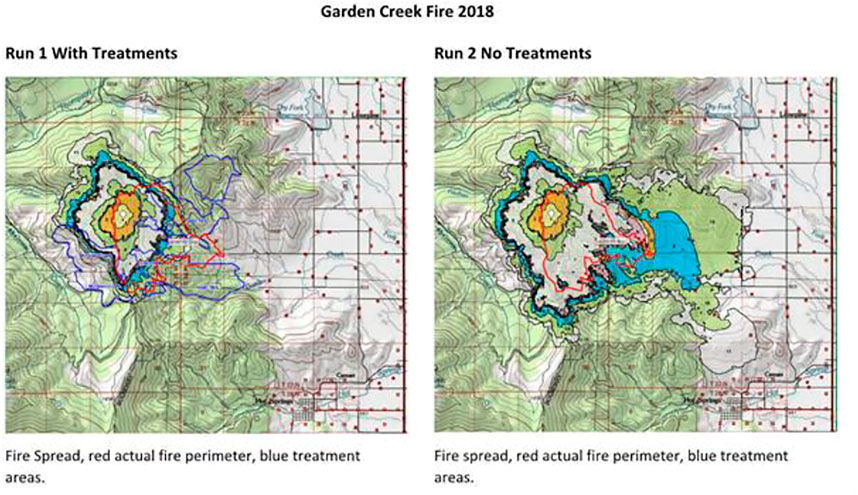
FIGURE 5. Effect of fuel treatments on a probabilistic fire perimeter (actual fire shown in red) (source: analysis by Dan Mindar, National Park Service, 2018).
Fuel treatments completed prior to the fire appear to have limited the spread of the wildfire. The model was used to generate 1) a calibrated wildfire model perimeter (Run 1), which shows the effect of fuel treatments (and excludes the effects of wildfire suppression), and 2) a model perimeter for a wildfire using identical inputs, though without the fuel treatments. Comparing the two runs, it appears that the royal blue treatments located on the East side of the red actual fire perimeter reduced the spread of the wildfire in that direction.29
Valuing the damage to assets within this perimeter would provide one point on the “fuel treatment” damage function for this fire. Additional modeling (to examine different levels of fuel-treatment effort) could allow an analyst to sketch out additional points along the Garden Creek “fuel treatment” damage function.
As for the suppression example described above, the analyst could use the damage function to assess whether managers over- or under-applied treatment effort in this region, given one fire that occurred. One important difference from suppression is that treatment decisions are often made long before a fire occurs, so in addition to other uncertainties, the manager must also consider the probability of a wildfire breaking out in any given area. Nevertheless, this type of modeling could build up a profile of landscape characteristics that lead to over- or under-treatment. This would inform the probabilities described in previous sections (e.g., see Figure 3), and allow the researcher to estimate the VOI of additional information.
Other Approaches
Similar modeling efforts to those described above could inform analysts about the effect of mitigation and post-fire rehabilitation on wildland fire-related damages. Research on optimizing post-fire rehabilitation efforts include McKoy et al. (2016) and Calkin et al. (2008).30 Tools available to researchers include the Burned Area Emergency Response (BAER) database of post-fire rehabilitation costs31 and the Erosion Risk Management Tool (ERMiT).32 Ideally, the researcher would be able to develop functions that allow for interactions among the various stages and forms of management effort. For example, an interactions model would provide insight into how various levels of fuel treatment affect the optimal level of suppression once a wildfire starts. Combining these modeling efforts with cost information (i.e., developing Cost (C) and Marginal Cost (MC) functions) would allow the analyst to determine the (ex post) optimal level of management effort for past fires (as well as the optimal mix across stages and forms of effort). Comparing the optimal effort (mix) to past management actions would show where managers had over- and under-applied various forms of effort. Repeated modeling would allow researchers to estimate the probability of over- and underapplying effort for various management activities.
There are admittedly limitations to this approach. Developing models robust enough to encompass substitution and complement effects will require a large amount of data. Researchers still know little about what affects the level of suppression effort, and idiosyncrasies at the level of the incident management team may overshadow characteristics of the wildfires or resources being studied33. Using data from historical fires implicitly assumes that future fires will be similar, which may not be the case. Also basing the damage function on modeling for single fires may mischaracterize the manager’s decision process. For example, decisions about fuel treatments may address risk over larger areas and longer timeframes than a single wildfire.
Information can also directly affect the manager’s prior probability of over- or under-applying effort. In particular, if available information indicates that very high-value resources are in the path of a wildfire, all else equal, the manager is less likely to under-suppress, and more likely to over-suppress. Thus, information about value inventories, and public willingness-to-pay for protecting these values are amenable to being valued using this approach. This approach is complementary to the microeconomic approach developed above. The microeconomic approach identifies the optimal level of effort by balancing marginal costs and marginal benefits, but it may not properly differentiate among choices based on total costs and total benefits. In contrast the VOI framework includes the manager’s assessment of total costs and total benefits and can identify relatively more valuable information by the effect it could have on the manager’s updated probabilities.
The modeling efforts described here are all comparisons for a single fire, and thus would allow a researcher to sketch out the total and marginal benefit functions for a single fire. As discussed above, these functions depend in part on various characteristics of the fire, such as the region, landcover, terrain, weather, values at risk, etc. Repeating the modeling process for more fires with different characteristics would allow researchers to start to parameterize general total and marginal benefit functions that vary with those characteristics. This is similar to parameterizing a demand curve given income, substitutes, complements, etc. It will also be important to conduct landscape-scale and long-duration fire simulations. This will allow wildland fire management to better match other landscape-scale planning and assessments, and will allow researchers to estimate the benefit of their planned management activities.
The most valuable information would be related to situations with steep marginal benefit and marginal cost curves (where costs and benefits are very sensitive to fire intensity and the level of effort to manage it), or where the low-information manager’s estimates of these curves are far from the true curves (say if there is great uncertainty in effectiveness or as a result of interactions, or if managers know little about the public’s willingness to pay to avoid damage to particular resources). In these cases, additional information is likely to avoid large over- or underestimates of the effect of management effort. Future effort could identify these situations and increase available information (by parameterizing the relevant B, MB, C, and MC functions) through focused research in these areas34.
Parameterized marginal benefit and marginal cost curves could help understand the effectiveness of effort in various fire situations, and the associated marginal costs. That is, a more complete understanding of the shape and position of the marginal benefit and marginal cost curves can help identify areas where a small level of effort is sufficient to avoid damage and areas where costs increase rapidly with additional effort.
There are at least four types of information that could help parameterize the C, B, MC, and MB curves illustrated in Figures 1, 2. This information includes:
1) Fire-behavior modeling (including changes in timing, location, and intensity related to climate change)35.
2) How management actions interact to affect fire behavior and the effect of fire on values at risk.
3) Comprehensive inventories of resources. This information can be important in targeting effort and identifying opportunity costs.
4) WTP to avoid damages for different types of resources (e.g., targeting effort—what is most valued; how much are motorists willing to pay to avoid a detour and how many motorists are likely to be affected?). This information typically comes from stated preference surveys focused on fire-damage “deltas” (e.g., how much is one WTP to avoid damages of Scenario X?)
Conclusion, Limitations, and Next Steps
This paper presents a conceptual approach to estimating the value of information. Moving from concept to application will require collecting data and performing empirical analysis to estimate benefit and damage functions. Including additional complexity like risk preferences would require further developing this conceptual model.
Managers currently lack information on the loss function L (T,S,R) and the management-cost function C (T,S,R). Parameterizing the management-cost function may be a relatively straightforward engineering problem. For example, the cost of a fuel-treatment project depends on the labor and equipment required, the time frame, project location, etc. Costs for suppression or rehabilitation could be estimated in a similar way36. Estimating the loss function is more demanding and may require gathering a large amount of data or focusing on a series of case studies. Future work could build on the approach of Katuwal et al. (2016), comparing the lengths of controlled fire line (imputed from changes in daily fire perimeters) to effort applied (in terms of fire crews, bulldozers, fire engines, helicopters, and air tankers)37.
Fire modeling approaches continue to advance and will likely offer more robust options in future. The current approach to suppression and fuels treatment modeling is akin to assuming a “barrier” on the landscape to limit fire spread, and modeling resource exposure using tools like FSPro and NFTB (or FARSITE)38. Managers also have tools available for modeling post-fire rehabilitation, making use of the known extent and severity of the fire, and addressing uncertainty in rainfall. Combining these approaches would allow modelers to examine how potential losses change with fire spread and intensity, and with varying levels of fuel treatment, suppression, and post-fire rehabilitation (Huffman et al., 2020; Young et al., 2020).
Parameterizing the loss function requires detailed inventories of resources at risk, and information on their values. Some inventory information is currently available, especially for areas receiving wildland fire management. However, we currently lack many estimates of the value of the resources in these inventories, especially resources that are not typically bought and sold in markets. Available estimates could inform a benefit-transfer approach to valuing the costs of wildfire (and the avoided costs of various management actions). Expanding and updating these inventories and value estimates could provide useful information on how resource values vary by region and other attributes. Developing approaches for resources and values not easily valued (e.g., health) is also an important area for future work.
Author Contributions
All authors listed have made a substantial, direct, and intellectual contribution to the work and approved it for publication.
Author Disclaimer
The views expressed in this paper represent the views of the authors and not the Department of the Interior.
Conflict of Interest
The authors declare that the research was conducted in the absence of any commercial or financial relationships that could be construed as a potential conflict of interest.
Publisher’s Note
All claims expressed in this article are solely those of the authors and do not necessarily represent those of their affiliated organizations, or those of the publisher, the editors and the reviewers. Any product that may be evaluated in this article, or claim that may be made by its manufacturer, is not guaranteed or endorsed by the publisher.
Acknowledgments
The authors thank Jason Fallon (DOI Office of Wildland Fire) and James Meldrum (U.S. Geological Survey) for reviewing an early draft of this paper, and two reviewers for their reviews and many helpful suggestions. The authors are responsible for any errors or omissions.
Footnotes
1One specific example is related to wildfire smoke. Information about wildfire smoke is very important to people in areas affected by wildfires, especially where monitoring data are sparse or absent. During the 2021 wildfire season, EPA is helping federal, state, local and tribal organizations monitor and map smoke during wildfire events by sharing equipment in areas that are affected by wildfire smoke.
2NIST, 2017, “The Costs and Losses of Wildfires: A Literature Survey” identifies the following as associated with mitigation: fuels management; defensible space; and insurance; and disaster assistance.
3Direct benefits may also be derived from improved ecosystem services (e.g., water quality, greenhouse gas sequestration) as a result of these management activities. However, quantifying these direct benefits would require measures of consumer preferences and present additional methodological challenges.
4Other pre-fire management activities include risk mitigation measures like education and working with communities to create defensible space, update zoning requirements and fire codes, etc. For pre-fire management actions, we focus on fuel treatment here.
5The authors estimate $2.47 million in suppression cost savings for 178,000-acre study area of ponderosa pine and lodgepole pine in the Deschutes National Forest in central Oregon.
6For example, WFDSS includes many important layers (https://wfdss.usgs.gov/wfdss/wfdss_data_downloads.shtml); HIFLD maps many infrastructure features (https://hifld-geoplatform.opendata.arcgis.com/); there are several data sets documenting where people live (https://wildfirerisk.org/download/and https://github.com/microsoft/USBuildingFootprints; and historic structures/places are also fairly well documented (https://www.nps.gov/subjects/nationalregister/data-downloads.htm).
7https://wfdss.usgs.gov/wfdss/WFDSS_Home.shtml.
8We note that Bowman (2018) is limited to describing the need for a global database of fire behavior and effects.
9Different management strategies may be used at different times: fuels reduction projects may be ordered before any fire occurs, suppression is ordered during a fire, and rehabilitation occurs after a fire is extinguished. Each decision affects decisions in subsequent periods, so finding an optimal level of effort is really a dynamic problem, with potential endogeneity issues.
10We recognize that there are variety of pre-fire mitigation activities that could be considered. For this paper we just focus on fuel treatment.
11In practice these costs vary widely across management strategies, and from one incident to another.
12This is the case for an interior solution, though it is also possible that the optimal level of effort is zero. For example, fuel treatment on steep slopes may be very expensive. A steep area with few assets to protect may have diverging marginal costs and benefits, suggesting an optimal level of zero effort.
13These areas could also be interpreted as the efficiency cost of a decision based on less than full information.
14The marginal cost curve need not be convex; it could feasibly be linear or concave. For convex MC, one could argue that managers implement less-costly actions before more-costly actions.
15To reduce wildfire intensity and rate of spread, managers may use aircraft to coat vegetation with fire retardant, e.g., “red” ammonium phosphate spray, or “blue” titanium dioxide gel.
16For an alternate scenario, instead of information on uncertain fire behavior, the manager could be weighing uncertainty about the resources located within an area, and whether they would be harmed by the application of retardant, say endangered species that were not included on a fire-avoidance map.
17A risk-averse manager would be willing to pay a risk premium to avoid the uncertainty inherent in this decision. Equivalently, they would require a lower chance of a course change to proceed with applying retardant today.
18Note how knowing for certain whether or not the fire will change course tomorrow would remove the costliest possibility of paying $20,000 for applying retardant on the wrong area.
19Information on direct effects allows the land-manager to predict how a given level of expenditure in each activity changes the expected value of wildfire-related losses. Indirect-effect information allows the land-manager to understand the interactions between management activities and how changes in one activity affect optimal levels of the other activities.
20For example, $700 million in wildfire suppression appropriations was provided for FS in FY 2016 and designated as emergency spending. P.L. 114–53, §137.
21Given the public good nature of research, there are advantages to coordinated approaches to gathering data, undertaking research, and sharing results. These coordination activities should in concept span federal, state, and local entities.
22More broadly, this issue is commonly ignored in fuel treatment planning because it increases the dimensionality of the problem, but recent forest planning assessments have begun to address the problem (Yemshanov et al., 2021).
23To reflect the uncertainties in fire behavior, we could relax the assumption that with full information the manager achieves the optimal level of total costs. For example, we could assume that managers are risk averse. Then even if the low-information manager’s choice of effort is very close to the optimal level, VOI is still greater than zero. This is because a risk-averse manager would be willing to pay some amount to reduce the uncertainty in the outcome. So VOI >0 so long as the information reduces uncertainty (outcome variance). However, since the optimal level of effort minimizes total costs, and no manager’s choice could lead to total costs below that optimal level, introducing any uncertainty necessarily increases the expected value of total costs.
24FSPro is the Fire Spread Probability developed for the Wildland Fire Decision Support System (WFDSS). FSPro is a geospatial potential fire growth model. FSPro calculates two-dimensional fire growth and maps the probabilities (over many repeated model runs) of a fire reaching each point on the landscape. https://wfdss.usgs.gov/wfdss/pdfs/fspro_reference.pdf.
25The 7-point size-class scale runs from A (smallest) to G (largest).
26FTB is part of the Wildland Fire Decision Support System (WFDSS). https://wfdss.usgs.gov/wfdss_help/4589.htm.
28https://www.firelab.org/project/fsim-wildfire-risk-simulation-software.
29Additionally, comparing the potential spread of the fire in Run 1 to the actual wildfire perimeter (in red) shows the effect of suppression, which appears to have limited the spread of the wildfire on the West side.
30We are grateful to an anonymous reviewer for providing the information detailed in this paragraph.
31https://www.fs.usda.gov/rmrs/tools/baer-db.
32https://www.fs.usda.gov/treesearch/pubs/26687.
33Hand et al., 2017 examined data for 372 wildland fire incidents between FY 2007 and FY 2011 and found that team-level effects explained about 14% of variation in management effort, measured as meters of daily fire-line production capacity using hand tools, bulldozers and other heavy equipment, fire engines and helicopters.
34For example, it could be instructive to examine the pre-fire mitigation. suppression, and post-fire rehabilitation decisions were made on 2020s Grizzly Creek Fire in Glenwood Canyon, CO. As described by Woodruff (2021), as a result of the fire and subsequent landslides, 40 miles of I-70 was closed for several weeks in 2020 and 2021. As I-70 is Colorado’s only east-west interstate highway, closures result in increased travel time for diverted motorists. Future work could use this fire as an example and include a more detailed discussion of the pre-fire, suppression, and post-fire rehabilitation decisions.
35For example, the 2021 Dixie Fire is California’s largest on record at nearly 1 million acres and also the first known wildfire to run over the Sierra Nevada, where high elevation forests usually too saturated with heavy snow melt to burn. A few days later the Caldor Fire around Lake Tahoe became the second fire to do so. Source: Romero, Ezra David, and Emily Zentner. 2021. “Wildfire torched the Sierra all summer, evading containment. Here’s how Tahoe protected itself.” Cap Radio. Tuesday, 5 October 2021 | Sacramento, CA, https://www.capradio.org/170460.
36For example, the Burned Area Emergency Response Treatments Catalog (BAER) (USDA, 2006) is a U.S. Forest Service handbook for use by managers wishing to estimate management costs.
37Katuwal et al. (2016) found that efficiency of wildland fire suppression effort, as measured by the production of controlled fire line, is affected by weather, landscape and fire characteristics, and that production is increased by the use of bulldozers and fire engines, natural or built breaks (e.g., rivers, roads), and in areas previously burned by wildfires. Adding firefighter crews does not appear to increase production.
38NFTB is the WFDSS Near-Term Fire Behavior module for web-based two-dimensional modeling. FARSITE is a similar desktop application.
References
Ager, A. A., Barros, A. M. G., Day, M. A., Preisler, H. K., Spies, T. A., and Bolte, J. (2018). Analyzing fine-scale Spatiotemporal Drivers of Wildfire in a forest Landscape Model. Ecol. Model. 384, 87–102. doi:10.1016/j.ecolmodel.2018.06.018
Barnett, K., Parks, S. A., Miller, C., and Naughton, H. T. (2016). Beyond Fuel Treatment Effectiveness: Characterizing Interactions between Fire and Treatments in the US. Forests 7 (10), 237. doi:10.3390/f7100237
Bayham, J., and Yoder, J. K. (2020). Resource Allocation under Fire. Land Econ. 96 (1), 92–110. doi:10.3368/le.96.1.92
Bernknopf, R., Brookshire, D., Macauley, M., Jakeman, G., Kuwayama, Y., Miller, H., et al. (2019). “Societal Benefits - Methods and Examples for Estimating the Value of Remote Sensing Information,” in ASPRS Manual of Remote Sensing. Fourth Edition, 869–910. doi:10.14358/MRS/Chapter8
Bernknopf, R., Kuwayama, Y., Gibson, R., Blakely, J., Mabee, B., Clifford, T. J., et al. (2021a). Monetising the Savings of Remotely Sensed Data and Information in Burn Area Emergency Response (BAER) Wildfire Assessment. Int. J. Wildland Fire 30, 18–29. doi:10.1071/wf19209
Bernknopf, R., Steinkruger, A., and Kuwayama, Y. (2021b). Earth Observations Can Enable Cost-Effective Conservation of Eastern North Pacific Blue Whales: A Value of Information Analysis. Resources for the Future (RFF) Working Paper.
Bowman, D. M. J. S., O'Brien, J. A., and Goldammer, J. G. (2013). Pyrogeography and the Global Quest for Sustainable Fire Management. Annu. Rev. Environ. Resour. 38 (1), 57–80. doi:10.1146/annurev-environ-082212-134049
Bowman, D. (2018). Wildfire Science Is at a Loss for Comprehensive Data. Nat. News Nat. Publishing Group 560, 7. doi:10.1038/d41586-018-05840-4
Bratvold, R. B., Bickel, J. E., and Lohne, H. P. (2009). Value of Information in the Oil and Gas Industry: Past, Present, and Future. SPE Res. Eval. Eng. 12, 630–638. doi:10.2118/110378-PA
Calkin, D., Jones, G., and Hyde, K. (2008). Nonmarket Resource Valuation in the Postfire Environment. J. For. 106 (6), 305–310.
D. E. Calkin, A. A. Ager, and J. Gilbertson-Day (Editors) (2010). “Wildfire Risk and hazard: Procedures for the First Approximation,” Gen. Tech. Rep. RMRS-GTR-235 (Fort Collins, CO: U.S. Department of Agriculture, Forest Service, Rocky Mountain Research Station), 62. Available at: http://www.fs.fed.us/rm/pubs/rmrs_gtr235.pdf.
Calkin, D. E., Cohen, J. D., Finney, M. A., and Thompson, M. P. (2014). How Risk Management Can Prevent Future Wildfire Disasters in the Wildland-Urban Interface. Proc. Natl. Acad. Sci. U.S.A. 111 (2), 746–751. doi:10.1073/pnas.1315088111
Chung, W. (2015). Optimizing Fuel Treatments to Reduce Wildland Fire Risk. Curr. For. Rep. 1 (1), 44–51.
Colavito, M. M., Combrink, T., Hjerpe, E., Edgeley, C., Burnett, J., and Sánchez Meador, A. J. (2021). Full-Cost Accounting Remeasurement of the 2010 Schultz Fire: Understanding the Long-Term Socio- Economic Implications of High-Severity Wildfire and Post-Wildfire Flooding. ERI White Paper— Issues in Forest Restoration. Flagstaff, AZ, USA: Ecological Restoration Institute, Northern Arizona University.
Donvan, G. H., and Rideout, D. B. (2003). A Reformulation of the Cost Plus Net Value Change (C+NVC) Model of Wildfire Economics. For. Sci. 49 (2), 318–323.
Fernandes, P. M. (2015). Empirical Support for the Use of Prescribed Burning as a Fuel Treatment. Curr. For. Rep 1 (2), 118–127. doi:10.1007/s40725-015-0010-z
Forney, W. M., Raunikar, R. P., Bernknopf, R. L., and Mishra, S. K. (2012). An Economic Value of Remote- Sensing Information—Application to Agricultural Production and Maintaining Groundwater Quality: U.S. Geological Survey Professional Paper 1796. Reston, Virginia: U.S. Geological Survey Professional, 60.
Fried, J. S., Gilless, J. K., and Spero, J. (2006). Analysing Initial Attack on Wildland Fires Using Stochastic Simulation. Int. J. Wildland Fire 15, 137–146. doi:10.1071/wf05027
Gebert, K. M., and Black, A. E. (2012). Effect of Suppression Strategies on Federal Wildland Fire Expenditures. J. For. 110 (2), 65–73. doi:10.5849/jof.10-068
Gebert, K. M., Calkin, D. E., and Yoder, J. (2007). Estimating Suppression Expenditures for Individual Large Wildland Fires. West. J. Appl. For. 22 (3), 188–196. doi:10.1093/wjaf/22.3.188
Gonzalez-Caban, A., McKetta, C. W., and Mills, T. J. (1984). Costs of Fire Suppression Forces Based on Cost-Aggregation Approach. Missoula, Montana: USDA Forest Service, Pacific Southwest Forest and Range Experiment Station, 16. Research Paper PSW-171.
Gorte, J. K., and Gorte, R. W. (1979). Application of Economic Techniques to Fire Management – a Status Review and Evaluation. Missoula, Montana: USDA Forest Service Gen. Tech. Rep. INT-53.
Grubb, M., Edmonds, J., ten Brink, P., and Morrison, M. (1993). The Costs of Limiting Fossil-Fuel CO2 Emissions: A Survey and Analysis. Annu. Rev. Energ. Environ. 18, 397–478. doi:10.1146/annurev.eg.18.110193.002145
Hand, M. S., Gebert, K. M., Liang, J., Calkin, D. E., Thompson, M. P., and Zhou, M. (2014). Economics of Wildfire Management, the Development and Application of Suppression Expenditure Models. New York: Springer.
Hand, M., Katuwal, H., Calkin, D. E., and Thompson, M. P. (2017). The Influence of Incident Management Teams on the Deployment of Wildfire Suppression Resources. Int. J. Wildland Fire 26, 615–629. doi:10.1071/wf16126
Headley, R. (1943). Rethinking forest Fire controlRes. Paper M-5123. Northern Rocky Mountain Forest and Range Experimentation Station. Missoula, Montana: USDA Forest Service, 361.
Hesseln, H., Loomis, J. B., and Gonzalez-Caban, A. (2004). Comparing the Economic Effects of Fire on Hiking Demand in Montana and Colorado. Contemp. Econ. Pol. 22, 407–419. doi:10.1016/j.jfe.2004.03.002
Hornby, L. G. (1936). Fire Control Planning in the Northern Rocky Mountain Region. Missoula, Montana: USDA Forest Service, Northern Rocky Mountain Forest and Range Experiment Station, 189. Available at: http://content.lib.umt.edu/utils/getfile/collection/forest/id/308/filename/309.pdf.
Houtman, R. M., Montgomery, C. A., Gagnon, A. R., Calkin, D. E., Dietterich, T. G., McGregor, S., et al. (2013). Allowing a Wildfire to Burn: Estimating the Effect on Future Fire Suppression Costs. Int. J. Wildland Fire 22 (7), 871–882. doi:10.1071/wf12157
Huffman, D. W., Roccaforte, J. P., Springer, J. D., and Crouse, J. E. (2020). Restoration Applications of Resource Objective Wildfires in Western US Forests: a Status of Knowledge Review. Fire Ecol. 16 (1), 1–13. doi:10.1186/s42408-020-00077-x
Hunter, M. E., and Robles, M. D. (2020). Tamm Review: The Effects of Prescribed Fire on Wildfire Regimes and Impacts: A Framework for Comparison. For. Ecol. Manage. 475, 118435. doi:10.1016/j.foreco.2020.118435
Jones, K. W., Cannon, J. B., Saavedra, F. A., Kampf, S. K., Addington, R. N., Cheng, A. S., et al. (2017). Return on Investment from Fuel Treatments to Reduce Severe Wildfire and Erosion in a Watershed Investment Program in Colorado. J. Environ. Manage. 198, 66–77. doi:10.1016/j.jenvman.2017.05.023
Katuwal, H., Calkin, D. E., and Hand, M. S. (2016). Production and Efficiency of Large Wildland Fire Suppression Effort: A Stochastic Frontier Analysis. J. Environ. Manage. 166, 227–236. doi:10.1016/j.jenvman.2015.10.030
Kolden, C. A. (2019). We're Not Doing Enough Prescribed Fire in the Western United States to Mitigate Wildfire Risk. Fire 2 (2), 30. doi:10.3390/fire2020030
Kuhn, H. W. (1953). “11. Extensive Games and the Problem of Information,” in Contributions to the Theory of Games (AM-28), Volume II. Editors H. W. Kuhn, and A. W. Tucker (Princeton, NJ, USA: Princeton University Press), 193–216. doi:10.1515/9781400881970-012
Loomis, J. B., Gonzalez-Caban, A., and Englin, J. (2001). Testing for Differential Effects of forest Fires on Hiking and Mountain Biking Demand and Benefits. J. Agric. Resource Econ. 26, 508–522.
Loomis, J. B., and Richardson, R. (2001). Econ Values of the U.S. Wilderness System. Int. J. Wilderness 7 (1), 31–34. Available at: http://www.wilderness.net/library/documents/loomis1.pdf.
Macauley, M. K. (2005). The Value of Information: A Background Paper on Measuring the Contribution of Space-Derived Earth Science Data to National Resource Management. Discussion Paper 05–26. Resources for the Future. Available at: https://media.rff.org/archive/files/sharepoint/WorkImages/Download/RFF-DP-05-26.pdf.
Mason, L. C., Lippke, B. R., Zobrist, K. W., Bloxton, T. D., Cedar, K. R., Commick, J. M., et al. (2006). Investments in Fuel Removals to Avoid forest Fires Result in Substantial Benefits. J. For. 2006, 27–31.
McCoy, K., Krasko, V., Santi, P., Kaffine, D., and Rebennack, S. (2016). Minimizing Economic Impacts from post-fire Debris Flows in the Western United States. Nat. Hazards 83, 149–176. doi:10.1007/s11069-016-2306-010.1007/s11069-016-2306-0
Mercer, D. E., Haight, R. G., and Prestemon, J. P. (2008). “Analyzing Trade-Offs between Fuels Management, Suppression, and Damages from Wildfire,” in The Economics of forest Disturbances (Dordrecht: Springer), 247–272. doi:10.1007/978-1-4020-4370-3_13
Morton, D. C., Roessing, M. E., Camp, A. E., and Tyrrell, M. L. (2003). Assessing the Environmental, Social, and Economic Impacts of Wildfire. New Haven, CT: Yale University Global Institute of Sustainable Forestry, Research Paper 001.
National Aeronautics and Space Administration (NASA) (2013). Measuring Socioeconomic Impacts of Earth Observations. Washington, D.C., USA: NASA. Earth Sciences Division. NP-2012-04-856-HQ.
National Institute of Standards and Technology (NIST) (2017). The Costs and Losses of Wildfires. NIST Spec. Publ. 1215 (11). doi:10.6028/NIST.SP.1215
North, M., Collins, B. M., and Stephens, S. (2012). Using Fire to Increase the Scale, Benefits, and Future Maintenance of Fuels Treatments. J. For. 110 (7), 392–401. doi:10.5849/jof.12-021
Pacheco, A. P., and Claro, J. (2018). Operational Flexibility in forest Fire Prevention and Suppression: a Spatially Explicit Intra-annual Optimization Analysis, Considering Prevention, (Pre)suppression, and Escape Costs. Eur. J. For. Res 137 (6), 895–916. doi:10.1007/s10342-018-1147-7
Pinchot, G. (1903). A Primer of Forestry. USDA For. Serv. Bull. 14 (1), 79–83. doi:10.5962/bhl.title.34555
Plucinski, M. P. (2019). Contain and Control: Wildfire Suppression Effectiveness at Incidents and across Landscapes. Curr. For. Rep 5 (1), 20–40. doi:10.1007/s40725-019-00085-4
Rhodes, J. J., and Baker, W. L. (2008). Fire Probability, Fuel Treatment Effectiveness and Ecological Tradeoffs in Western US Public Forests. Open For. Sci. J. 1 (1).
Rideout, D. B., and Pamela, Z. (2008). Three Great Myths of Wildland Fire Management. In Proceedings of the Second International Symposium on Fire Economics, Planning, and Policy: A Global View. Missoula, Montana: USDA Forest Service Gen. Tech. Rep. PSW-GTR-208, 319–325. Available at: http://www.fs.fed.us/psw/publications/documents/psw_gtr208en/psw_gtr208en_319-326_rideout.pdf.
Rideout, D. B., Ziesler, P. S., Kling, R., Loomis, J. B., and Botti, S. J. (2008). Estimating Rates of Substitution for Protecting Values at Risk for Initial Attack Planning and Budgeting. For. Pol. Econ. 10 (2), 205–219. doi:10.1016/j.forpol.2007.10.003
Sánchez, J. J., Loomis, J., González-Cabán, A., Rideout, D., and Reich, R. (2019). Do fuel Treatments in US National Forests Reduce Wildfire Suppression Costs and Property Damage? J. Nat. Resour. Pol. Res. 9 (1), 42–73.
Scott, J. H., Thompson, M. P., and Calkin, D. E. (2013). A Wildfire Risk Assessment Framework for Land and Resource Management. Washington, D.C., USA: United States Department of Agriculture. Available at: https://www.fs.fed.us/rm/pubs_series/rmrs/gtr/rmrs_gtr315.pdf.
Simard, A. J. (1976). Wildland Fire Management: The Economics of Policy Alternatives. Can. For. Serv. Tech. Rep. 15, 52.
Sparhawk, W. N. (1925). The Use of Liability Ratings in Planning forest Fire protection. J. Agric. Res. 30 (8), 693–792.
Thompson, M. P., Vaillant, N. M., Haas, J. R., Gebert, K. M., and Stockmann, K. D. (2013). Quantifying the Potential Impacts of Fuel Treatments on Wildfire Suppression Costs. J. For. 111 (1), 49–58. doi:10.5849/jof.12-027
U.S. Department of Agriculture (USDA) and U.S. Department of the Interior (DOI) (2000). Interior Columbia Basin Supplemental Draft Environmental Impact Statement. Missoula, Montana: USDA Forest Service and DOI Bureau of Land Management. Volume 1, Page 10. Available at: http://www.icbemp.gov/pdfs/sdeis/Volume1/Volume1.pdf.
U.S. Department of Agriculture (USDA), Forest Service (2021). The 2021 Wildland Fire Year: Responding to and Mitigating Threats to Communities. Washington, D.C., USA: Draft testimony of Randy Moore, Chief, U.S. Department of Agriculture-Forest Service, before the U.S. House of Representatives Committee on Agriculture-Subcommittee on Conservation and Forestry. September 29, 2021.
U.S. Department of Agriculture, Forest Service (2006). Burned Area Emergency Response Treatments Catalog. Missoula, Montana: US Forest Service, San Dimas Technology and Development Center. Available at: https://www.fs.fed.us/t-d/pubs/pdf/BAERCAT/lo_res/06251801L.pdf.
U.S. Department of Agriculture, Forest Service, Springerville, Alpine, and Clifton Ranger Districts, Apache-Sitgreaves N. F. (2011). Post Wallow Fire 2011 BAER Trails Assessment Report. Missoula, Montana: USDA. Available at: http://www.fs.usda.gov/Internet/FSE_DOCUMENTS/stelprdb5360159.pdf.
U.S. Environmental Protection Agency (EPA) (1999). The Benefits and Costs of the Clean Air Act, 1990- 2010. Report to Congress. Washington, D.C., USA: EPA, 654. Available at: www.epa.gov/oar/sect812/1990-2010/chap1130.pdf.
U.S. Environmental Protection Agency (2014). Guidelines for Preparing Economic Analyses (Updated May 20214). Washington, D.C., USA: National Center for Environmental Economics, Office of Policy, 18. Available at: yosemite.epa.gov/ee/epa/eerm.nsf/vwAN/EE-0568-52.pdf/$file/EE-0568-52.pdf.
Wang, D., Guan, D., Zhu, S., Kinnon, M. M., Geng, G., Zhang, Q., et al. (2021). Economic Footprint of California Wildfires in 2018. Nat. Sustain. 4 (3), 252–260. doi:10.1038/s41893-020-00646-7
Woodruff, C. (2021). ‘Shocking’ Mudslide Damage Could Keep I-70 through Glenwood Canyon Closed for Weeks. August 2, 2021. Colorado Newsline. Available at: https://coloradonewsline.com/briefs/shocking-mudslide-damage-could-keep-i-70-through-glenwood-canyon-closed-for-weeks/(Accessed February 5, 2022).
Yemshanov, D., Liu, N., Thompson, D. K., Parisien, M.-A., Barber, Q. E., Koch, F. H., et al. (2021). Detecting Critical Nodes in forest Landscape Networks to Reduce Wildfire Spread. PloS one 16 (10), e0258060. doi:10.1371/journal.pone.0258060
Young, J. D., Evans, A. M., Iniguez, J. M., Thode, A., Meyer, M. D., Hedwall, S. J., et al. (2020). Effects of Policy Change on Wildland Fire Management Strategies: Evidence for a Paradigm Shift in the Western US? Int. J. Wildland Fire 29 (10), 857–877. doi:10.1071/wf19189
Young, J. D., Thode, A. E., Huang, C.-H., Ager, A. A., and Fulé, P. Z. (2019). Strategic Application of Wildland Fire Suppression in the Southwestern United States. J. Environ. Manag. 245, 504–518. doi:10.1016/j.jenvman.2019.01.003
Zybach, B., Dubrasich, M., Brenner, G., and Marker, J. (2009). U.S. Wildfire Cost-Plus-Loss Economics Project: The "One-Pager" Checklist. Wildland Fire Lessons Learned Center. Available at: https://www.researchgate.net/publication/237396187_US_Wildfire_Cost-Plus-Loss_Economics_Project_The_One-Pager_Checklist (Accessed Feb 7, 2021).
Keywords: value of information, voi, wildland fire, wildfire, benefits
Citation: Simon B, Crowley C and Franco F (2022) The Costs and Costs Avoided From Wildfire Fire Management—A Conceptual Framework for a Value of Information Analysis. Front. Environ. Sci. 10:804958. doi: 10.3389/fenvs.2022.804958
Received: 29 October 2021; Accepted: 05 April 2022;
Published: 09 May 2022.
Edited by:
Christopher Joseph Lauer, National Oceanic and Atmospheric Administration, United StatesReviewed by:
Benjamin Gannon, Colorado State University, United StatesJesse Young, Rocky Mountain Research Station, United States Forest Service (USDA), United States
Copyright © 2022 Simon, Crowley and Franco. This is an open-access article distributed under the terms of the Creative Commons Attribution License (CC BY). The use, distribution or reproduction in other forums is permitted, provided the original author(s) and the copyright owner(s) are credited and that the original publication in this journal is cited, in accordance with accepted academic practice. No use, distribution or reproduction is permitted which does not comply with these terms.
*Correspondence: Benjamin Simon, YnNpbW9uQGd3dS5lZHU=