- 1Integrated Research on Energy, Environment and Society (IREES), University of Groningen, Groningen, Netherlands
- 2Aquatic Ecology and Environmental Biology, Radboud Institute for Biological and Environmental Sciences (RIBES), Radboud University, Nijmegen, Netherlands
- 3Department of Earth Sciences, Vrije Universiteit Amsterdam, Amsterdam, Netherlands
Estimating annual CO2 budgets on drained peatlands is important in understanding the significance of CO2 emissions from peatland degradation and evaluating the effectiveness of mitigation techniques. The closed-chamber technique is widely used in combination with gap-filling of CO2 fluxes by parameter fitting empirical models of ecosystem respiration (Reco) and gross primary production (GPP). However, numerous gap-filling strategies are available which are suitable for different circumstances and can result in large variances in annual budget estimates. Therefore, a need for guidance on the selection of gap-filling methodology and its influence on the results exists. Here, we propose a framework of gap-filling methods with four Tiers following increasing model complexity at structural and temporal levels. Tier one is a simple parameter fitting of basic empirical models on an annual basis. Tier two adds structural complexity by including extra environmental factors such as grass height, groundwater level and drought condition. Tier three introduces temporal complexity by separation of annual datasets into seasons. Tier four is a campaign-specific parameter fitting approach, representing highest temporal complexity. The methods were demonstrated on two chamber-based CO2 flux datasets, one of which was previously published. Performance of the empirical models were compared in terms of error statistics. Annual budget estimates were indirectly validated with carbon export values. In conclusion, different gap-filling methodologies gave similar annual estimates but different intra-annual CO2 fluxes, which did not affect the detection of the treatment effects. The campaign-wise gap-filling at Tier four gave the best model performances, while Tier three seasonal gap-filling produced satisfactory results throughout, even under data scarcity. Given the need for more complete carbon balances in drained peatlands, our four-Tier framework can serve as a methodological guidance to the handling of chamber-measured CO2 fluxes, which is fundamental in understanding emissions from degraded peatlands and its mitigation. The performance of models on intra-annual data should be validated in future research with continuous measured CO2 flux data.
1 Introduction
Covering only 3% of the global land surface, peatland contains over 600 Gt of carbon, which is nearly 30% of all global soil carbon (Parish et al., 2008; Yu et al., 2010). However, centuries of peatland drainage for agriculture and forestry have changed large peatland areas from carbon sinks into sources. At present, around 10% of global peatland is degraded due to drainage or exploitation (Joosten, 2010). Drained peatlands are estimated to annually emit 1.91 Gt CO2-equivalents without further exploitation (Leifeld and Menichetti, 2018). This comprises an estimated 12–41% of the greenhouse gas (GHG) emission budget for keeping global warming below +1.5 to +2°C (Leifeld et al., 2019). Given the importance of emissions from degraded peatlands, numerous studies have focused on the measurement of the net ecosystem exchange (NEE), and, subsequently, estimation of the annual CO2 budgets. These studies helped understanding the magnitude of emissions among different peatland systems (Campbell et al., 2014; Tiemeyer et al., 2016), influences of environmental factors (Järveoja et al., 2016; Hoyt et al., 2019), effects of land use and management (Beetz et al., 2013; Günther et al., 2015; Renou-Wilson et al., 2016), and peatland’s contribution to large-scale CO2 emission inventories (Wilson et al., 2016a; Tiemeyer et al., 2020). Annual CO2 budgets provide straightforward information that could be easily adopted in policy- or decision-making regarding peatland degradation.
Closed-chamber methods represent an inexpensive and easy-to-use technique that is suitable for use on a wide range of ecosystems (Heng, 2021). At larger scale, however, estimation of annual CO2 budgets is heavily dependent on spatial and temporal interpolation, i.e., gap-filling of chamber measurement data. The most frequently-used gap-filling methodologies include parameter fitting of empirical models, such as the simple temperature and PAR (photosynthetically active radiation) dependent functions of ecosystem respiration (Reco) and gross primary production (GPP), respectively. The most frequently used relations are the Arrhenius-type Reco relation (Lloyd and Taylor, 1994) and rectangular hyperbolic light response equation of GPP (Michaelis and Menten, 1913).
A wide variety of models with different complexity can be applied for better interpretation of the processes. In model structure, various environmental factors can be incorporated to improve model performance. For example, hydrological regimes (e.g., soil moisture content, groundwater level) regulates Reco by establishing aerobic and anaerobic zones within the soil profile (Juszczak et al., 2013). Plant composition, biomass and phenology can reflect the temporal variations on the contributions from microbial heterotrophic and plant autotrophic respiration to Reco (Järveoja et al., 2020) and the photosynthesis capacity of the plants to GPP (Peichl et al., 2018). An extensive summary of commonly used model structures and environmental factors are presented in Supplementary Table S1. Parameter fitting of these models are applied with time scales from campaign-specific (e.g., Beetz et al., 2013) to seasonal (Waddington and Roulet, 2000) or annual (Wilson et al., 2016b), adding a temporal dimension of the model’s complexity.
Such diversity of gap-filling methodology creates variations in the annual CO2 budget estimation, which may lead to uncertainties on the ability of the methodology to reach budget estimates closest to the real CO2 fluxes. Such uncertainties affect upscaling of the CO2 emission and conclusions in field trials and comparative studies (Hoffmann et al., 2015). Huth et al. (2017) found strongly diverse CO2 budgets (−7.3 to 15.6 t ha−1) across gap-filling options with different pooling methods of measured data (temporal complexity). Karki et al. (2019) calculated widely variable NEE estimates, ranging from −9.35 to −2.08 t ha−1 yr−1 on one plot, when combining eight Reco and eight GPP models (structural complexity). Previous studies attempted to mitigate such uncertainties by standardizing the data acquisition and processing approaches. Hoffmann et al. (2015) proposed a standardized automatic data processing algorithm for campaign-specific modeling. Huth et al. (2017) provided options of the timing of the flux measurements, strategies of data pooling and methods of flux partitioning. However, the methodological diversity of gap-filling and the subsequent uncertainties still complicates the application and interpretation of chamber-based flux data.
Manual closed chamber measurements require human resources to carry out the measurements. Which makes that the current state of the method may be limited due to practical issues, such as accessibility of the sites, equipment deficiency, and/or unexpected influence from management or weather events (e.g., in Weideveld et al., 2021), resulting in flux datasets with potentially low measurement frequency, prolonged data gaps, and/or large variations. The standard campaign-specific method may not be possible under such circumstances, requiring additional procedures in the gap-filling data processing and modeling.
The above-mentioned methodological challenges regarding diversity of the methods, uncertainties, and data deficiency confounds the handling of chamber-measured flux data, as well as the subsequent data analysis of comparative studies or field trails. A streamlined framework for the gap-filling strategy is timely needed that contributes to the current methodological standards. The main objectives of this study are: 1) present a systematic framework of gap-filling methodologies following a gradient of structural and temporal model complexity; 2) demonstrate and compare the statistical performances of the gap-filling methods using multiple datasets; 3) investigate the potential influence of gap-filling method selection on the detection of treatment effects in a field trail.
2 Materials and Methods
2.1 CO2 Flux Dataset
Selected gap-filling methodologies were tested on an existing chamber-based CO2 flux dataset from the province of Friesland, the Netherlands, published in Weideveld et al. (2021). CO2 exchanges were monitored from January 2017 till December 2018 at four farms. All farm locations have large fields with deep drainage (ditch water levels from 60 to 90 cm below surface) and intensive fertilization (>230 kg N ha−1 yr−1). The peat soils have a thickness of 0.8–2.0 m, some of which are covered with a carbon-rich clay layer of 20–40 cm. The grasslands are dominated by Lolium perenne. Each farm was set up with a control site containing traditional drainage and infiltration ditches, and a treatment site containing sub-soil irrigation (SSI) drains. The SSI system functions by installing permeable drainage/irrigation pipes at around 10 cm below ditch water level. It was proposed to elevate groundwater level in the dry summer season to reduce CO2 emissions, while fulfilling a drainage function when the groundwater level is above the ditch water level (van den Akker et al., 2010; Querner et al., 2012 as cited in; Weideveld et al., 2021).
CO2 fluxes were measured on all four farms twice a month during the growing season (April–September) and once a month during the rest of the year. On each site, three 15 cm-deep soil collars for flux measurements with chambers (80 × 80 × 50 cm) were installed as replicates. An opaque (dark) chamber was used to measure ecosystem respiration (Reco) and a transparent (light) chamber for the measurement of net ecosystem exchange (NEE). Gross primary production (GPP) was directly derived from NEE using measured daytime Reco, which could avoid propagating Reco modeling errors into GPP models (Huth et al., 2017), while light and dark measurements were performed in sequence to minimize errors due to deviations in temperature over time. During each campaign, per field flux measurements were performed from sunrise to noon, or from noon to sunset. Depending on the duration of sunlight, an average of nine light and 10 dark measurements during winter, and 18 light and 20 dark measurements were achieved. An average of 383 measured CO2 fluxes were collected per site and year (Weideveld et al., 2021; Supplementary Table S2). Grass height and groundwater table (GWT) were measured at the start of every field campaign. Photosynthetically active radiation (PAR) and soil temperature at 5 and 10 cm depth were measured during the campaigns. PAR, soil temperature and air temperature were also continuously recorded every 5 min during and between field campaigns. The recorded data was allocated into hourly averages to simplify the computation in the following gap-filling extrapolation. According to Hoffmann et al. (2015), the use of hourly average PAR and temperature could induce systematic bias in the gap-filled annual budget estimates. The positively skewed distribution pattern of the GPP functions could result in an overestimation of over 1 t CO2 ha−1 yr−1 in the GPP estimates (Hoffmann et al., 2015). Meanwhile, bias in the Reco estimates in our case was presumably small since soil temperature as the driving environmental factor for the Reco functions changes slower than air temperature. Grass yield inside soil collars was harvested eight times in 2017 and five times in 2018. Total carbon was measured in dry plant material (t C ha−1 yr−1) to determine C-export via harvest. Slurry manure was applied four times per year at rates of 119–181 kg N ha−1 yr−1 with a C/N ratio of 16.3 ± 1.3 (mean±SD) to simulate C-import via farm management. Export and import of carbon were converted from carbon content to CO2 for calculating the CO2 balance. More details on the experimental design, flux measurements and data treatment are available in Weideveld et al. (2021).
In the previous analysis in Weideveld et al. (2021), a campaign-wise gap-filling strategy was applied and no effect from the SSI technique was detected. In 2018, the experimental farms suffered from an extreme drought. Parameter fitting of the data therefore faced difficulties and led to uncertainties in the CO2 budget estimates, which was presumably due to drought effect that could not be explained by the measured environmental factors such as soil moisture and GWT. This extensive dataset combining multiple years and locations with the unexpected drought events provided opportunities to test the performances of gap-filling strategies and exemplify their differences.
2.2 Gap-Filling Methodological Tiers
A framework of four Tiers of gap-filling methodologies was constructed (Figure 1). A Tier represents a level of methodological complexity, which has been used in the IPCC GHG inventory reporting methods (IPCC, 2019). Tier 1 is the basic method applying the original form of the empirical models without extra independent variables on annual datasets. Higher Tiers are more demanding in terms of model complexity and data requirements. Within each Tier, the most commonly used empirical models of Reco and GPP were selected from Supplementary Table S1. Parameter fitting was performed using non-linear least square (NLS) models with the R package lme4 (Bates et al., 2015). Model complexity was determined by two aspects: model structure and temporal scale. Structural complexity was increased by adding extra parameters to the basic empirical models. Temporal complexity was increased by shortening modeling periods by separating the dataset according to seasonality or applying campaign-specific models. For both Reco and GPP, multiple model structures were tested at each Tier. Significance of the parameters was determined by the p-value and t-statistic of the parameter estimate from the NLS model. Models that performed poorly (non-converging regressions, insignificant/abnormal parameter values, etc.) were discarded while the best performing model combinations (see Section 2.3) were selected to be used in the next Tiers and for further statistical analysis.
2.2.1 Tier One: Basic Annual Models
From Supplementary Table S1, the most widely-used empirical models were selected as the basic annual models. For Reco, temperature-dependent functions including the Lloyd-Taylor modified Arrhenius (Table 1, Eq. 1) and Van’t Hoff exponential (Table 1, Eq. 2) models were selected. Air temperature, soil temperature at 5 and 10 cm depth were tested in the parameter fitting. Soil temperature at 5 cm depth consistently provided better performances in different models and was therefore selected to be used in other Tiers. For GPP, rectangular hyperbolic functions including the Michaelis-Menten’s (Table 1, Eq. 3), Smith’s (Table 1, Eq. 4) and Mitscherlich’s (Table 1, Eq. 5) light response curves were selected. For the parameter fitting, flux measurement data was pooled over the entire year. The Lloyd-Taylor modified Arrhenius and Michaelis-Menten’s hyperbolic functions were selected to be used in the higher Tiers.
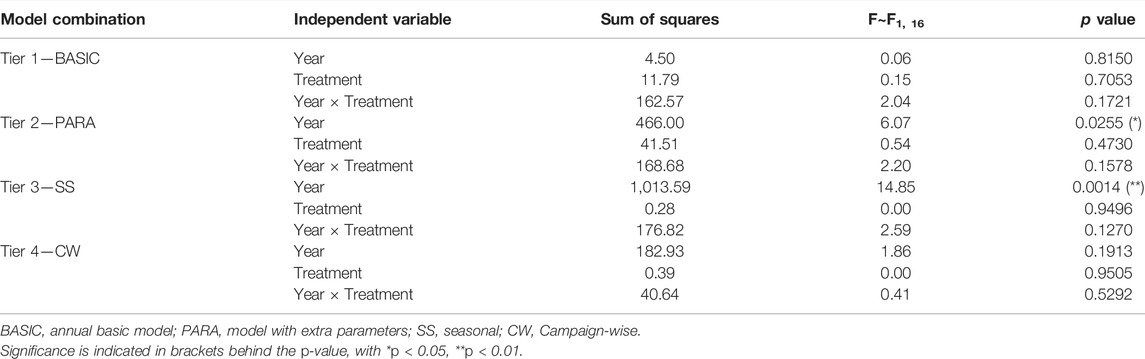
TABLE 2. Summary of the analysis of variance (ANOVA) for the mixed-effects models fitted on the gap-filled NEE for each gap-filling strategy. Year, treatment and their interaction are independent variables, and farm location is included as a random effect.
2.2.2 Tier Two: Annual Models With Extra Parameters
2.2.2.1 Inclusion of Extra Environmental Factors
Extra environmental factors were introduced into the Tier one models for an increased model structural complexity. Multiple mathematical forms of the environmental factors were tested and compared for the best model performance (Supplementary Table S1B). Grass height was used as a vegetation index since it is correlated to a wide range of vegetation indexes, such as Normalized Difference Vegetation Index (NDVI), Leaf Area Index (LAI) and Difference Vegetation Index (DVI) (Payero et al., 2004), although rarely used directly. The GPP model with grass height modified GPPmax (Karki et al., 2019, Table 1, Eq. 7) was selected for further calculations. Reco models with grass height were not included due to frequently insignificant parameter fitting, indicating a weak correlation. A temperature function (Supplementary Table S1B) was introduced to the GPP function with grass height (Karki et al., 2019, Table 1, Eq. 8) to account for the influence of low temperature during winter and spring (Yamori et al., 2014). Groundwater table, although frequently used in other studies (see references in Supplementary Table S1B), was discarded from the models due to its generally poor model performance in this study. Soil moisture was not tested due to lack of continuous measurements.
2.2.2.2 Introducing a Drought Index
In order to describe the contrasting climate conditions in the studied years, a drought index was defined based on drought events (any period with more than three consecutive days without precipitation, Jassey and Signarbieux, 2019) and the cumulative atmospheric water flux (precipitation minus evapotranspiration, P-ET, Stagge et al., 2015). The daily precipitation and evapotranspiration data were collected from the nearest official KNMI weather station (weather station Leeuwarden, 18–30 km distance from research sites, Weideveld et al., 2021). The accumulated P-ET was reset to zero at the beginning of both years. Pulses of water from small precipitation events can stimulate the carbon flux (Munson et al., 2010; Shen et al., 2015). Munson et al. (2010) found on a semiarid grassland that water is less limiting to carbon fluxes after rain events above 5 mm. No such study was found for peat meadows. Therefore, we reset the accumulated P-ET also after rain events above 5 mm, assuming a reduced drought effect. The drought index was then calculated as absolute values of the accumulated P-ET that remained negative with resets after rain events (Supplementary Figure S1). The drought index was then tested in different forms and included as a residual term in both the Reco and GPP models (Table 1, Eqs 6, 9) as only this form resulted in significant model parameters.
2.2.3 Tier Three: Seasonal Gap-Filling
The annual flux datasets were split into two seasonal subsets, adding a level of temporal complexity. Here, seasonality was defined based on drought conditions, whereas a drought season had a positive drought index, and non-drought seasons had a drought index equal to zero. The best performing Tier one and Tier two models were tested in the parameter fitting of two seasonal data sets. For non-drought periods, an Arrhenius Reco model was combined with a Michalis-Menten GPP model with grass height and temperature function. For drought periods, both models were applied with addition of a drought index. The drought period covered only 37 days in 2017 (151 days in 2018), which led to insufficient data points for the model fitting. Therefore, linear regressions were applied to the modeled Reco for the drought period in 2017 (Table 1, Eq. 10); and the parameter of light response function adopted fixed GPPmax and α from the annually fitted Tier two models.
2.2.4 Tier Four: Campaign-Wise Gap-Filling
Campaign-wise gap-filling refers to parameter fitting of flux data measured on an individual measurement date. The modeling and gap-filling procedure is adopted from Weideveld et al. (2021): Per campaign, Arrhenius and Michalis-Menten functions were fitted for Reco and GPP, respectively. Pooling of data from two or more adjacent campaigns was applied when the range of PAR did not cover a complete light response curve including the light-limited part and after the light saturation point. This occurred in both years during winter from January to March, and occasionally in 2017 during summer from June to September when data was collected on rainy and cloudy days (see campaign-wise parameters in Supplementary Dataset). Gap-filling between two adjacent campaigns are averages of the CO2 flux estimates from these two campaign-wise models, weighted by the temporal distances of the gap-filled moment to each of the measurements.
2.3 Model Performance
Performances of the fitted models were evaluated by comparing measured and modeled values based on a series of model indicators following Moriasi et al. (2007) as used by Hoffmann et al. (2015). Mean absolute error (MAE), RMSE (root mean square error)—observations standard deviation ratio (RSR), coefficient of determination (r2), modified index of agreement (md), and Nash–Sutcliff’s model efficiency (NSE) were calculated for each model. Goodness of the fit was determined on a set of thresholds rating the indicators (Hoffmann et al., 2015).
2.4 Gap-Filling and Validation
Hourly CO2 fluxes (Reco, GPP and NEE) were calculated by feeding the environmental variables (hourly average soil temperature at 5 cm depth, air temperature, PAR, and interpolated grass height) to the selected gap-filling models. Grass height was linearly interpolated between measurement campaigns using the R package zoo (Zeileis and Grothendieck, 2005). More accurate interpolation was not possible due to the lack of high frequency measurement or empirical models for grass height. Depending on the growth stage of the plants, linear interpolation may induce overestimation at short grass after harvest due to the plants’ recovery and underestimation at relatively long grass when plant growth is reaching the maxima.
Annual Reco, GPP and NEE budgets were calculated by summing these hourly fluxes. Model errors and extrapolation errors are the most important sources of uncertainty in the gap-filling of annual CO2 budgets (Beetz et al., 2013). Only model errors were estimated, since extrapolation errors were partly related to, and therefore discussed by, the selection of different extrapolation methods (Weideveld et al., 2021). A Monte Carlo simulation was included in each gap-filling run for model error estimation (Beetz et al., 2013; Leiber-Sauheitl et al., 2014; Hoffmann et al., 2015; Berger et al., 2019; Zhao et al., 2020) using R package nlstools (Baty et al., 2015). Parameter fitting of Reco and GPP models was bootstrapped with 1,000 iterations. Annual Reco and GPP budgets were calculated with all the bootstrapped model parameter sets, from which the standard deviation (SD) was calculated to represent the uncertainties of the estimates. Uncertainty of annual NEE budgets was subsequently calculated by combining the SDs of Reco and GPP following the law of error propagation.
An independent quantitative validation of the annual budget estimates was also needed alongside the proposed model performance and uncertainty assessments, in order to evaluate the accuracy of the gap-filled CO2 budgets. However, a direct validation was not possible in absence of a known true value of annual CO2 budgets. Therefore, only an indirect validation was performed using the positive correlations between GPP and plant biomass presented in previous studies (Otieno et al., 2009; Hirota et al., 2010; Weideveld et al., 2021). Derived annual GPP budgets were linearly correlated with C-export per year and method Tier as a quality check of the estimates by examining the significance of the regression.
2.5 Applicability Demonstration
Our Tier list framework was developed mainly in consideration of the drought effect during our measured period that caused large variances in the fluxes data. Meanwhile, the ever-growing number of chamber-based flux measurements set up on global peatlands are performed under influence from various conditions other than drought events. For example, differences in equipment, operational techniques, environmental and weather conditions, etc. Our framework and insights on method selection also need to be tested under other types of variations or data gaps. Therefore, the framework was further demonstrated and evaluated on an unpublished CO2 flux dataset from the fifth farm location over 2018 and 2019. The fifth farm location was close to the other four main locations in Friesland, with similar soil type and management regime. The farm followed the same experimental setup as the other four farms, with a control site and a treatment site installed with SSI. At this location, the SSI treatment was enhanced by installing a pressure well connected to the drainage ditch. Water level inside the well could be raised by a pump to provide increased inflow pressure, therefore keeping the groundwater level stable throughout the year (van den Akker et al., 2019). Measurement procedures were in line with the previous experiments. The measurement frequency, however, was significantly lower than in the other locations, with in total less than ten campaigns: once per month during the growing season (April–September) and twice during the non-growing season (in October and November/December) (Supplementary Table S2). Parameter fitting of gap-filling models followed the four methodological Tiers. Drought index was not used as a parameter here, since the drought effect was not as pronounced as in the four main locations due to extra irrigation in 2018. Grass height was used instead of the drought index in the Reco model from the Tier two method. Model performances and the gap-filled annual budgets were evaluated and compared. Budget estimates under data scarcity with large gaps to fill could further test the applicability of our framework on different datasets and evaluate the strengths and limitations of each Tier.
2.6 Statistics
The effect of gap-filling methodology on annual budget estimation and detection of treatment effects were investigated. Correlation of model performance ratings against the Tier position of gap-filling methodologies was tested by a simple linear regression. Linear mixed-effects models were fitted using R package lme4 (Bates et al., 2015) with gap-filled CO2 fluxes (Reco, GPP and NEE) as dependent variables; year, treatment, their interaction and gap-filling methodology as fixed effects; and farm location as random effect. Type III analysis of variance tables of the linear mixed-effects models were computed with Satterthwaite’s method using the anova function from the R basic package stats. The post-hoc Tukey’s HSD test was used with R package emmeans (Lenth et al., 2020) to further detect significant differences between gap-filling methodologies. To test whether selection of different gap-filling methodology would generate a treatment effect, linear mixed-effects models were also fitted separately for all gap-filling methodologies with annual NEE as dependent variable, year, treatment, and their interaction as independent variables, and farm location as random effect. All data was processed and analyzed using R version 4.0.2 (R Core Team, 2020).
3 Results and Discussion
3.1 Model Performances and Annual Budget Estimates
The model performances under different gap-filling strategies improved significantly (p < 0.001) with higher complexity (Figure 2, Supplementary Table S3). However, such improvement did not lead to systematic differences among annual CO2 budget estimates from the four Tiers (Figure 3). In general, all gap-filling strategies resulted in CO2 emissions situated in the upper range of emissions from productive grasslands on organic soils (Grønlund et al., 2008; Tiemeyer et al., 2016, 2020). Large variances can be observed between the gap-filled annual budgets (Supplementary Table S4) and daily fluxes (Supplementary Figure S2) resulting from different methods. The basic empirical models from Tier one were not suitable for annual parameter estimates (Figure 2) due to limited explanatory power regarding the environmental and temporal variations. Gap-filled daily fluxes showed mild fluctuations without reflections on rapid changing conditions such as harvest. This resulted in significantly larger annual GPP estimates from the Tier one method in 2017 (p < 0.001, Figure 3). Influence from environmental changes can be accounted for by higher Tier methodologies with either inclusion of additional variables or season-/campaign-specific modeling. For example, inclusion of grass height and the temperature function in Tier two substantially improved GPP estimates in all aspects, which is in close agreement with other studies stressing the importance of accounting for plant growth and harvest (Eickenscheidt et al., 2015; Huth et al., 2017; Kandel et al., 2013). This, however, could lead to drastic fluctuations and contradicting trends in the daily fluxes (Supplementary Figure S2). The lower soil respiration (Davidson et al., 1998) and photosynthetic CO2 uptake (Fu et al., 2020; Koebsch et al., 2020) under desiccation stress may have been oversimplified by the drought index proposed in our study, leading to unrealistic peaks and dips of the daily fluxes in Tier two and three. Tier three seasonal gap-filling substantially improved the model performances by circumventing the lack of good predictors for the drought period that altered important drivers of CO2 fluxes. However, it is more prone to influences of individual high Reco measurements under abrupt environmental changes, such as rain-induced soil respiration pulses (Lee et al., 2004; Ma et al., 2012). Such data “outliers” may have confounded the models when fitted seasonally, leading to less representative temperature-respiration relationships, and may have resulted in the significantly higher Reco from Tier three comparing to Tier four in 2017 (p < 0.05, Figure 3). Tier four campaign-wise model fitting further reduced model errors (Figure 2). The ability of the Tier four campaign-wise method to reflect environmental changes without complicated parameterization demonstrated its robustness and reliability when applied with sufficient measurement frequency. However, it is subject to over-extrapolation, since a narrow measurement range of temperature from a single campaign could introduce bias when extrapolated outside of that range (Hoffmann et al., 2015; Huth et al., 2017). Pooling of all gap-filling results over locations and treatment sites showed an absence of consistently significant differences (Figure 3) in spite of the above-mentioned variances. This implies a strong influence from divergence among years and locations. Gap-filling method selection should therefore be considered case-specifically in light of characteristics of the dataset, such as the time scale and frequency of the measurements and abrupt or abnormal environmental changes.
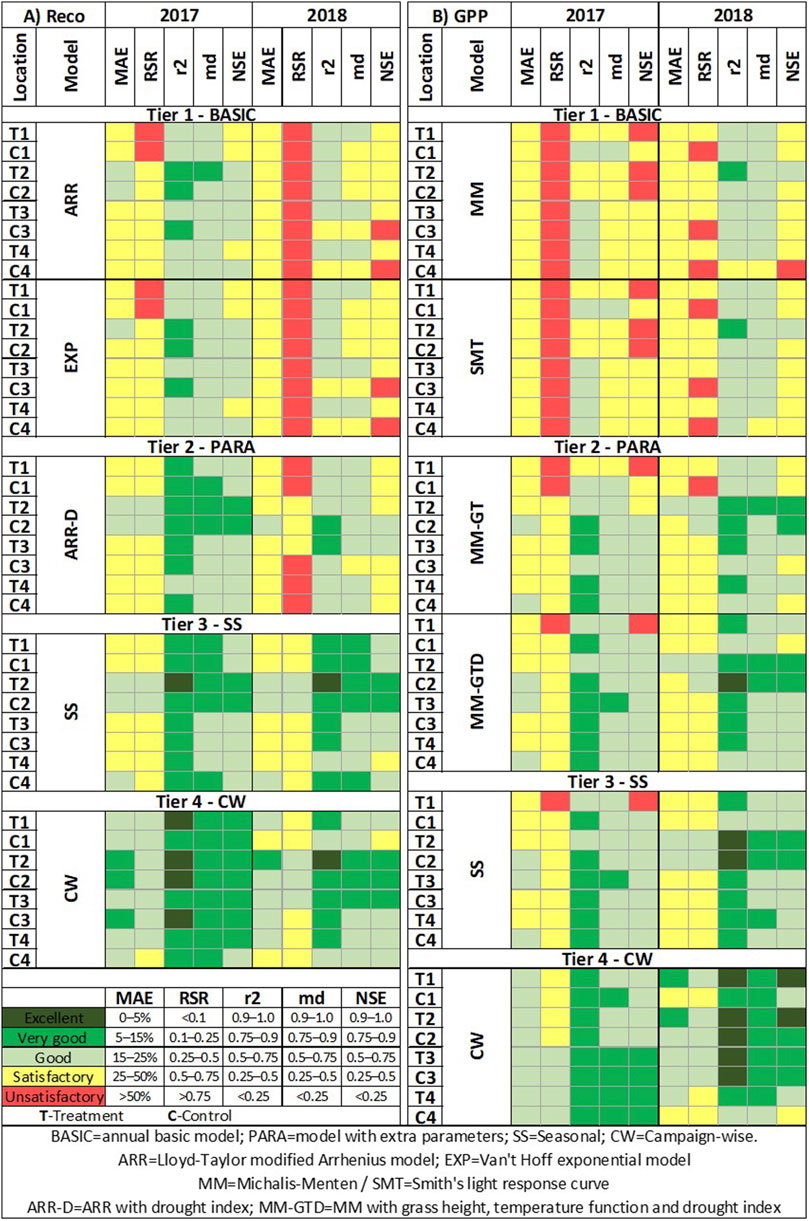
FIGURE 2. Heatmap of model performance indicators per farm and treatment site following the four Tiers of the gap-filling guiding framework. (A) Reco, and (B) GPP. Statistical quality criteria were adopted from Moriasi et al. (2007) as used by Hoffmann et al. (2015): MAE = mean absolute error, RSR = root mean square error (RMSE)-observations standard deviation ratio, r2 = coefficient of determination, md = modified index of agreement, NSE = Nash-Sutcliff model efficiency.
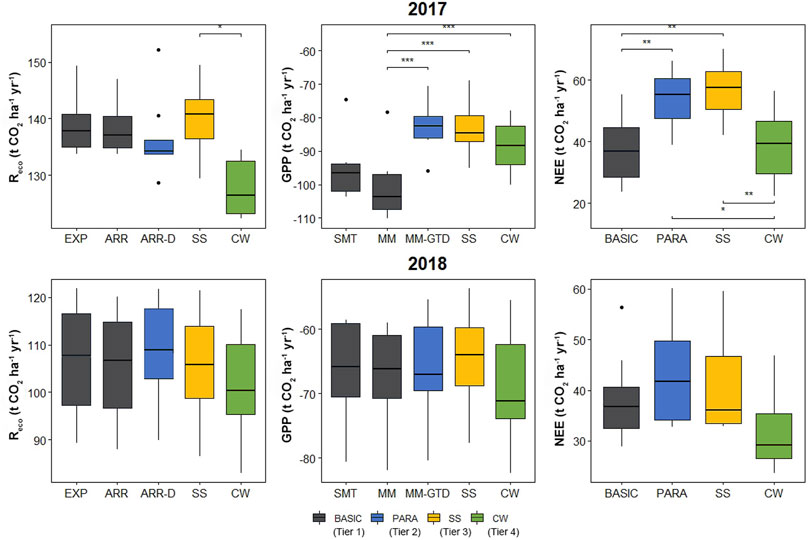
FIGURE 3. Gap-filled annual Reco, GPP and NEE budgets from different model selections and combinations in both years. The box indicates the median and the upper/lower quartiles. The whiskers represent the maximum and minimum values. Filled points are the outliers. The asterisks between boxes indicate significant differences between models based on linear mixed-effects (LME) models (*p < 0.05; **p < 0.01; ***p < 0.001).
3.2 Sensitivity of Treatment Effect to the Choice of Gap-Filling Method
Despite the seemingly large differences between CO2 budget estimates of treatment and control plots observed in individual farms (Supplementary Table S4), we did not find any significant treatment effect when pooling the data of the four locations (Table 2). The differences found in individual locations could be due to random variations in the site-specific biotic and/or abiotic processes between treatment and control plots, such as differences in vegetation growth, microbial activities, and GWT fluctuations. These processes could be highly variable due to the complexity of in-situ environmental conditions. Statistical results from the pooled data were less likely to be affected by such random effects. Therefore, testing of treatment effects from field trials requires a combination of multiple locations and a longer timespan (e.g., Maljanen et al., 2007; Elsgaard et al., 2012) to avoid confounding factors from site or year differences. Annual budget estimates from a single location are more consistent in representing the magnitude of CO2 emissions.
3.3 Applicability Demonstration: Gap-Filling Under Data Scarcity
Parameter fitting and gap-filling for the dataset from the fifth farm location was carried out for demonstration purposes, following the methodological Tier list. The low frequency of the measurement campaigns (<10 per year) in this dataset insufficiently captured the temporal dynamics in CO2 fluxes. As a result, Tier four campaign-wise gap-filling, although being the most commonly used standard approach, led to high uncertainties and poor fit of gap-filling model parameters (Table 3). Tiers containing models with lower temporal complexity at annual or seasonal levels were proven more applicable, as GPP modeling using Tier one (Michalis-Menten function) and Tier two (Michalis-Menten function with grass height) resulted in smaller uncertainties of budget estimates (Table 3). However, annual Reco modeling methodologies from Tier one and two (Arrhenius function and with drought index) resulted in poor or even unsatisfactory performances (Table 3), mainly due to the large variances in the raw flux data. The best model performances were achieved by the Tier three seasonal method (Table 3). Meanwhile, gap-filled daily fluxes (Figure 4) occasionally showed large fluctuations despite the satisfactory error statistics. For example, Reco and GPP fluxes from Tiers one, two and four showed potential overestimations due to low representativeness of the dataset in the winter season. Extreme peaks and dips can be observed in the Reco fluxes, especially from the Tier four campaign-wise method, showing strong effects of individual high and low measurements when extrapolated over a prolonged time period (Huth et al., 2017). The extremely low Reco fluxes observed in summer 2018 from Tier two method can be imputed to the low explanatory power of the drought index leading to unrealistic outputs from the model. The satisfactory model error statistics achieved by Tier three and four methods (Table 3) demonstrated the potential of generating annual budget estimates even from scarce data. However, large uncertainties should be acknowledged given the varying behavior of the models throughout the year (Figure 4). It is therefore necessary to test multiple gap-filling methods when the quality and quantity of raw flux data is limited. The resulting annual budget estimates should be interpreted with caution, merely as an indication for the order of magnitude of the emissions.
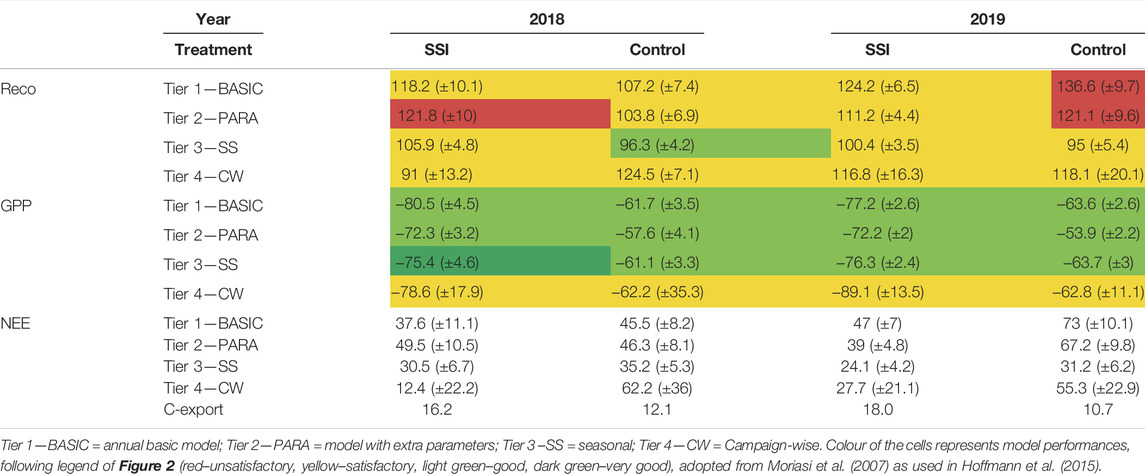
TABLE 3. Annual CO2 budgets of the fifth farm location as a demonstration of the framework applicability. Data are CO2 budget estimates in t ha−1 yr−1 (±SD). C-export was translated from grass yield in t CO2-eq ha−1 yr−1.
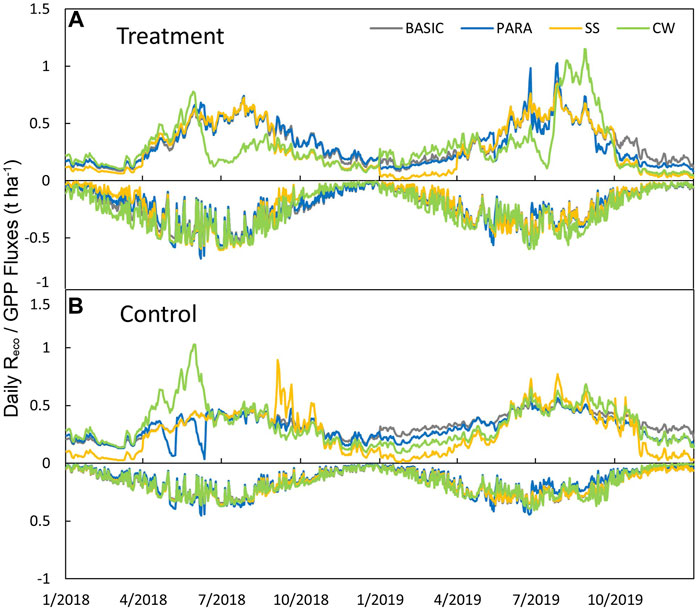
FIGURE 4. Gap-filled daily summed CO2 fluxes (Reco and GPP are depicted as the positive and negative values, respectively) from different models at (A) treatment and (B) control site of the demonstration location 5.
3.4 Validation of the Annual Budget Estimates
Given the lack of known true values of carbon budgets for locations in this study, independent validation of the derived CO2 exchange was not possible. Nonetheless, an indirect cross-validation of derived annual GPP budgets with C-export via biomass harvest was performed (Figure 5). A similar validation for Reco was not feasible in our analysis due to lack of comparable environmental datasets. In general, Annual GPP showed strong correlation with C-export when pooling data from all five locations and 3 years from 2017 to 2019 (p < 0.001, R2 = 0.42). GPP estimates in 2018 (p < 0.001, R2 = 0.52) and 2019 (p = 0.007, R2 = 0.69) were roughly in agreement with the general trend. Previous meta-analysis from Tiemeyer et al. (2016) gave average values of GPP and C-export from nutrient-rich deep-drained grassland on organic soils overlapping the range of our estimates (Figure 5), also indicating realistic estimation of the GPP values. However, GPP estimates in 2017 showed poor correlation with C-export values (p = 0.598, R2∼0) and strong deviation to the general trend, especially with results from the Tier one method. This is in accordance with the significant overestimation of Tier one GPP values identified (Section 3.1, Figure 3), and is reflected in the Tier one daily fluxes (Supplementary Figure S2) that failed to represent changes of CO2 exchange due to plant biomass growth and removal. Accuracy of GPP estimates in 2017 should therefore be given low confidence. Within-day timing and frequency of the measurements (Huth et al., 2017; Gana et al., 2018; Järveoja et al., 2020) could also be a cause of this low accuracy. Our midday measurements at a fixed timeframe in 2017 resulted in poor coverage of different PAR ranges. Meanwhile, results from the four Tiers all showed strong correlation with C-export (p < 0.01, R2 = 0.38–0.43) when tested separately, including the Tier one method that also produced GPP values in good agreement with the GPP∼C-export correlation in 2018 and 2019 (Figure 5). Therefore, the general feasibility of all four method Tiers can be concluded. However, Tiers one and two methods are more prone to bias when analyzing raw flux datasets with poor representativeness for the environmental conditions and management events, leading to potentially large variations in the GPP estimates.
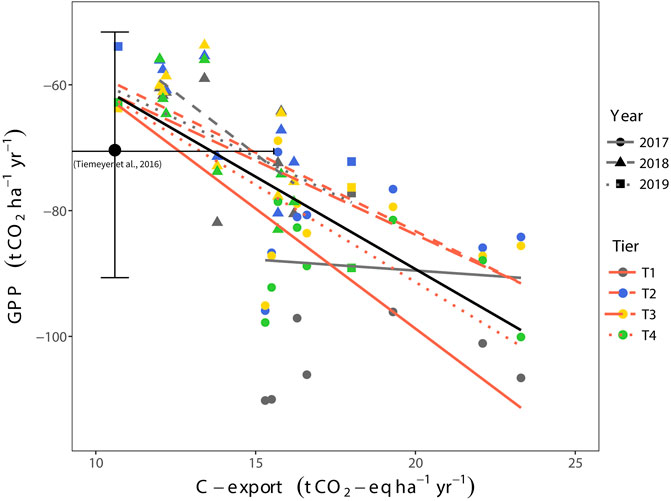
FIGURE 5. Indirect validation by comparing derived annual GPP with C-export per method Tier and year. The black solid line is the result of general linear regression of the entire dataset (p < 0.001, R2 = 0.42). Grey lines are regression lines per year (2017, p = 0.598, R2∼0; 2018, p < 0.001, R2 = 0.52; 2019, p = 0.007, R2 = 0.69). Red lines are regression lines per method Tier (Tier one, p = 0.002, R2 = 0.42; Tier two, p = 0.002, R2 = 0.43; Tier three, p = 0.002, R2 = 0.0.42; Tier four, p < 0.001, R2 = 0.38). Black point with error bars represents average values of GPP and C-export from nutrient-rich deep-drained grassland adopted from Tiemeyer et al. (2016).
Better estimates of carbon pools including plant biomass could serve as a valid data source for indirect cross-validation. For example, a process-based growth model for plant biomass could better correlate with the derived GPP values by avoiding uncertainties introduced by the harvest practice. However, such models are normally parameterized only for mineral soils and requires extensive growth data for local calibration (e.g., Barrett et al., 2005). Long-term carbon fluxes monitoring can also be cross-validated with soil carbon pool. Hoffmann et al. (2017) validated chamber-derived net ecosystem carbon balance by resampling of soil organic carbon. Net carbon losses could be proxied by monitoring soil subsidence (Couwenberg and Hooijer, 2013), while such estimated emissions are highly uncertain due to the complicated processes involved in soil surface changes including the oscillation of peat soil (Fritz et al., 2008).
It is still challenging to sufficiently validate chamber-based annual budgets estimates given the limitations of the above-mentioned indirect validation approaches. Paired comparison of the discrete manual chamber fluxes with continuous flux measurements from eddy-covariance and automatic chamber techniques could potentially enable independent validation of the gap-filling methods and budget estimates. Lucas-Moffat et al. (2018) presented highly correlated fluxes between manual chamber and eddy-covariance fluxes from a cropland. Meanwhile, Cappoci and Vargas, 2022) discovered up to 60% underestimation of annual CO2 efflux by manual chamber compared to automatic chamber in a tidal marsh. More continuous measurements with higher resolution in long term are needed for the improvement of the validation and therefore the refinement of the gap-filling methods. An ongoing year-round automatic chamber measurement including farm locations of this study (Erkens, 2020) could provide opportunities for robust cross-validation of the gap-filling approaches in the near future. However, not without acknowledgement of the specific shortcomings in each method. For example, while manual chamber provides low temporal resolution and lack of night-time fluxes; eddy-covariance measurements have a variable footprint and insufficient energy closure; automatic chambers could lead to heating up to the vegetation and upper soil layer, and are subject to disturbance of the precipitation/evapotranspiration process. Therefore, our statistical comparison provides insights for the selection of available gap-filling methods to meet the growing number of chamber measurements as well as the need to derive more annual carbon balances in drained peatlands. Despite the fact that a “best” approach could not be identified.
4 Conclusion and Implication
In this study, we summarized the most commonly-used empirical models of Reco and GPP for gap-filling manual chamber-based CO2 flux into a framework of four Tiers with increasing model complexity. Model performance of the methods from the four Tiers were systematically compared in terms of error statistics. The annual CO2 exchange was in the same order of magnitude with values from literature on similar ecosystems. Detection of treatment effects requires large number of independent observations (locations) and longer period of time (years) to reduce its sensitivity to random variances. Indirect validation of GPP estimates showed good agreement with the GPP∼C-export correlation, except for the year 2017. However, without independent validations using high-resolution long-term continuous flux measurements, the true precision of the methods cannot be discussed but are better suited to determine only the magnitude of the annual CO2 budgets. Nonetheless, specific recommendation could be given for each Tier supporting the selection of suitable methods:
1) Tier one annual basic gap-filling is feasible when higher model complexity is not possible due to deficiency of data required for model input or a low number of measurements. However, Tier one models are not likely to provide robust parameter fitting in most cases, because the variance in CO2 fluxes cannot be sufficiently explained by the limited number of explanatory variables in the models. 2) Tier two methodology introduces higher structural complexity with additional parameterization but remains temporally simple with annual parameter fitting. Robustness of such models depends on the explanatory power of the included variables as well as the representativeness and frequency of the flux measurements. However, the number of variables should be limited to avoid overfitting. 3) Tier three seasonal gap-filling has a moderate overall complexity. It is a potential solution in situations where representativeness of data is an issue, such as with low measurement frequency. The definition of the seasons used to cluster the data is essential when accounting for the unexplained temporal variation in CO2 fluxes. 4) Tier four campaign-wise gap-filling represents the highest temporal complexity by fitting simple relations per measurement campaign. It is the most commonly used procedure in chamber-based CO2 fluxes studies, and the most reliable way of gap-filling when data is adequately available. However, the risk of over-extrapolation should be considered in case the range in temperature or PAR is limited in single measurement campaigns.
Besides in the gap-filling of CO2 fluxes from drained temperate peatlands, similar empirical models of Reco and GPP have been applied also on cropland (e.g., Struck et al., 2020), forest (e.g., Zhao et al., 2020), and tropical peatlands (e.g., Hirano et al., 2014; Gana et al., 2018) with consideration of influences of temperature, groundwater table and plant phenology. The Tier system following an increased model structural and temporal complexity proposed in this study is potentially applicable to these other ecosystems. For example, CO2 and CH4 fluxes from waterbodies can show clear correlations with temperature and water chemistry indicators, and display seasonal differences (Peacock et al., 2021). All in all, the Tier system can provide opportunities in the modeling of various greenhouse gasses in a multitude of ecosystems, though empirical relationships and independent environmental variables should be considered.
Data Availability Statement
The raw data, intermediate results, and R scripts that support the findings of this study are openly available on the DANS platform at https://doi.org/10.17026/dans-xtq-ajzs.
Author Contributions
WL designed the methodology, performed the formal analysis and drafted the manuscript. CF conceived and supervised the study. WL and SW collected and processed the data. All authors contributed to the review and editing of the manuscript and approved the final version.
Funding
WL is supported by the China Scholarship Council (201706350201). The work of MV is funded by NWO-Veni grant 202.053. MB and CF received funding through PEATWISE (NWO ALW-GAS No.4). This research was supported by Interreg-NWE Carbon Connects.
Conflict of Interest
The authors declare that the research was conducted in the absence of any commercial or financial relationships that could be construed as a potential conflict of interest.
Publisher’s Note
All claims expressed in this article are solely those of the authors and do not necessarily represent those of their affiliated organizations, or those of the publisher, the editors and the reviewers. Any product that may be evaluated in this article, or claim that may be made by its manufacturer, is not guaranteed or endorsed by the publisher.
Acknowledgments
We would like to thank all technical staff, students and volunteers who helped in the field and in the laboratory, as well as the landowners who granted access to the measurement sites. Specifically, we are grateful for the help of Sebastian Krosse, Paul van der Ven, staff from the General Instrumentation and Roy Peters for the chemical analyses. We appreciate Reinder Nouta from Wetterskip Fryslân for insightful discussions on the flux data. We acknowledge Peter Cruijsen for his assistance in fieldwork and analyses. We sincerely thank the editor and reviewers for their critical remarks that helped improving the manuscript.
Supplementary Material
The Supplementary Material for this article can be found online at: https://www.frontiersin.org/articles/10.3389/fenvs.2022.803746/full#supplementary-material
References
Barrett, P. D., Laidlaw, A. S., and Mayne, C. S. (2005). GrazeGro: A European Herbage Growth Model to Predict Pasture Production in Perennial Ryegrass Swards for Decision Support. Eur. J. Agron. 23 (1), 37–56. doi:10.1016/j.eja.2004.09.006
Bates, D., Mächler, M., Bolker, B., and Walker, S. (2015). Fitting Linear Mixed-Effects Models Usinglme4. J. Stat. Soft. 67. doi:10.18637/jss.v067.i01
Baty, F., Ritz, C., Charles, S., Brutsche, M., Flandrois, J.-P., and Delignette-Muller, M.-L. (2015). A Toolbox for Nonlinear Regression in R: The Packagenlstools. J. Stat. Soft. 66, 1–21. doi:10.18637/jss.v066.i05
Beetz, S., Liebersbach, H., Glatzel, S., Jurasinski, G., Buczko, U., and Höper, H. (2013). Effects of Land Use Intensity on the Full Greenhouse Gas Balance in an Atlantic Peat Bog. Biogeosciences 10, 1067–1082. doi:10.5194/bg-10-1067-2013
Berger, S., Braeckevelt, E., Blodau, C., Burger, M., Goebel, M., Klemm, O., et al. (2019). A 1-year Greenhouse Gas Budget of a Peatland Exposed to Long-Term Nutrient Infiltration and Altered Hydrology: High Carbon Uptake and Methane Emission. Environ. Monit. Assess. 191. doi:10.1007/s10661-019-7639-1
Campbell, D. I., Smith, J., Goodrich, J. P., Wall, A. M., and Schipper, L. A. (2014). Year-round Growing Conditions Explains Large CO2 Sink Strength in a New Zealand Raised Peat Bog. Agric. For. Meteorology 192-193, 59–68. doi:10.1016/j.agrformet.2014.03.003
Capooci, M., and Vargas, R. (2022). Diel and Seasonal Patterns of Soil CO2 Efflux in a Temperate Tidal Marsh. Sci. Total Environ. 802, 149715. doi:10.1016/j.scitotenv.2021.149715
Couwenberg, J., and Hooijer, A. (2013). Towards Robust Subsidence-Based Soil Carbon Emission Factors for Peat Soils in South-East Asia, with Special Reference to Oil palm Plantations. Mires Peat 12 (1).
Davidson, E. A., Belk, E., and Boone, R. D. (1998). Soil Water Content and Temperature as Independent or Confounded Factors Controlling Soil Respiration in a Temperate Mixed Hardwood forest. Glob. Change Biol. 4, 217–227. doi:10.1046/j.1365-2486.1998.00128.x
Eickenscheidt, T., Heinichen, J., and Drösler, M. (2015). The Greenhouse Gas Balance of a Drained Fen Peatland Is Mainly Controlled by Land-Use rather Than Soil Organic Carbon Content. Biogeosciences 12, 5161–5184. doi:10.5194/bg-12-5161-2015
Elsgaard, L., Görres, C.-M., Hoffmann, C. C., Blicher-Mathiesen, G., Schelde, K., and Petersen, S. O. (2012). Net Ecosystem Exchange of CO2 and Carbon Balance for Eight Temperate Organic Soils under Agricultural Management. Agric. Ecosyst. Environ. 162, 52–67. doi:10.1016/j.agee.2012.09.001
Erkens, G., and Boonman, J. (2020). A New National Research Programme on Greenhouse Gas Emissions from lowland Peat Meadows in The Netherlands. EGU Gen. Assembly, EGU2020–11169. doi:10.5194/egusphere-egu2020-11169
Fritz, C., Campbell, D. I., and Schipper, L. A. (2008). Oscillating Peat Surface Levels in a Restiad Peatland, New Zealand-magnitude and Spatiotemporal Variability. Hydrol. Process. 22, 3264–3274. doi:10.1002/hyp.6912
Fu, Z., Ciais, P., Bastos, A., Stoy, P. C., Yang, H., Green, J. K., et al. (2020). Sensitivity of Gross Primary Productivity to Climatic Drivers During the Summer Drought of 2018 in Europe. Phil. Trans. R. Soc. B 375, 20190747. doi:10.1098/rstb.2019.0747
Gana, C., Nouvellon, Y., Marron, N., Stape, J. L., and Epron, D. (2018). Sampling and Interpolation Strategies Derived from the Analysis of Continuous Soil CO2 Flux. J. Plant Nutr. Soil Sci. 181 (1), 12–20. doi:10.1002/jpln.201600133
Grønlund, A., Hauge, A., Hovde, A., and Rasse, D. P. (2008). Carbon Loss Estimates From Cultivated Peat Soils in Norway: A Comparison of Three Methods. Nutr. Cycl. Agroecosystems 81 (2), 157–167. doi:10.1007/s10705-008-9171-5
Günther, A., Huth, V., Jurasinski, G., and Glatzel, S. (2015). The Effect of Biomass Harvesting on Greenhouse Gas Emissions from a Rewetted Temperate Fen. GCB Bioenergy 7, 1092–1106. doi:10.1111/gcbb.12214
Heng, L. (2021). in Measuring Emission of Agricultural Greenhouse Gases and Developing Mitigation Options Using Nuclear and Related Techniques. Editors M. Zaman, L. Heng, and C. Müller (Cham: Springer International Publishing). doi:10.1007/978-3-030-55396-8
Hirano, T., Kusin, K., Limin, S., and Osaki, M. (2014). Carbon Dioxide Emissions through Oxidative Peat Decomposition on a Burnt Tropical Peatland. Glob. Change Biol. 20 (2), 555–565. doi:10.1111/gcb.12296
Hirota, M., Zhang, P., Gu, S., Shen, H., Kuriyama, T., Li, Y., et al. (2010). Small-scale Variation in Ecosystem CO2 Fluxes in an alpine Meadow Depends on Plant Biomass and Species Richness. J. Plant Res. 123, 531–541. doi:10.1007/s10265-010-0315-8
Hoffmann, M., Jurisch, N., Albiac Borraz, E., Hagemann, U., Drösler, M., Sommer, M., et al. (2015). Automated Modeling of Ecosystem CO2 Fluxes Based on Periodic Closed Chamber Measurements: A Standardized Conceptual and Practical Approach. Agric. For. Meteorology 200, 30–45. doi:10.1016/j.agrformet.2014.09.005
Hoffmann, M., Jurisch, N., Garcia Alba, J., Albiac Borraz, E., Schmidt, M., Huth, V., et al. (2017). Detecting Small-Scale Spatial Heterogeneity and Temporal Dynamics of Soil Organic Carbon (SOC) Stocks: a Comparison between Automatic Chamber-Derived C Budgets and Repeated Soil Inventories. Biogeosciences 14 (4), 1003–1019. doi:10.5194/bg-14-1003-2017
Hoyt, A. M., Gandois, L., Eri, J., Kai, F. M., Harvey, C. F., and Cobb, A. R. (2019). CO2 Emissions from an Undrained Tropical Peatland: Interacting Influences of Temperature, Shading and Water Table Depth. Glob. Change Biol. 25, 2885–2899. doi:10.1111/gcb.14702
Huth, V., Vaidya, S., Hoffmann, M., Jurisch, N., Günther, A., Gundlach, L., et al. (2017). Divergent NEE Balances from Manual‐chamber CO2 Fluxes Linked to Different Measurement and Gap‐filling Strategies: A Source for Uncertainty of Estimated Terrestrial C Sources and Sinks? J. Plant Nutr. Soil Sci. 180, 302–315. doi:10.1002/jpln.201600493
Intergovernmental Panel on Climate Change (IPCC) (2019). 2019 Refinement to the 2006 IPCC Guidelines for National Greenhouse Gas Inventories. Available at: https://www.ipcc-nggip.iges.or.jp/public/2019rf/index.html (Accessed on October 22, 2021).
Järveoja, J., Peichl, M., Maddison, M., Soosaar, K., Vellak, K., Karofeld, E., et al. (2016). Impact of Water Table Level on Annual Carbon and Greenhouse Gas Balances of a Restored Peat Extraction Area. Biogeosciences 13, 2637–2651. doi:10.5194/bg-13-2637-2016
Järveoja, J., Nilsson, M. B., Crill, P. M., and Peichl, M. (2020). Bimodal Diel Pattern in Peatland Ecosystem Respiration Rebuts Uniform Temperature Response. Nat. Commun. 11, 1–9. doi:10.1038/s41467-020-18027-1
Jassey, V. E. J., and Signarbieux, C. (2019). Effects of Climate Warming on Sphagnum Photosynthesis in Peatlands Depend on Peat Moisture and Species‐specific Anatomical Traits. Glob. Change Biol. 25, 3859–3870. doi:10.1111/gcb.14788
Joosten, H. (2010). The Global Peatland CO2 Picture. Peatland Status and Drainage Related Emissions in All Countries of the World. Wetl. Int. 36. Available at: http://scholar.google.com/scholar?hl=en&btnG=Search&q=intitle:The+Global+Peatland+CO+2+Picture+Peatland+status+and+drainage+related+emissions+in+all+countries+of+the+world#0.
Juszczak, R., Humphreys, E., Acosta, M., Michalak-Galczewska, M., Kayzer, D., and Olejnik, J. (2013). Ecosystem Respiration in a Heterogeneous Temperate Peatland and its Sensitivity to Peat Temperature and Water Table Depth. Plant Soil 366, 505–520. doi:10.1007/s11104-012-1441-y
Kandel, T. P., Elsgaard, L., and Laerke, P. E. (2013). Measurement and Modelling of CO2flux from a Drained Fen Peatland Cultivated with Reed Canary Grass and spring Barley. GCB Bioenergy 5, 548–561. doi:10.1111/gcbb.12020
Karki, S., Kandel, T. P., Elsgaard, L., Labouriau, R., and Lærke, P. E. (2019). Annual CO 2 Fluxes from a Cultivated Fen with Perennial Grasses during Two Initial Years of Rewetting. Mires Peat 25, 1–22. doi:10.19189/MaP.2017.DW.322
Koebsch, F., Gottschalk, P., Beyer, F., Wille, C., Jurasinski, G., and Sachs, T. (2020). The Impact of Occasional Drought Periods on Vegetation Spread and Greenhouse Gas Exchange in Rewetted Fens. Phil. Trans. R. Soc. B 375, 20190685. doi:10.1098/rstb.2019.0685
Lee, X., Wu, H.-J., Sigler, J., Oishi, C., and Siccama, T. (2004). Rapid and Transient Response of Soil Respiration to Rain. Glob. Chang. Biol. 10, 1017–1026. doi:10.1111/j.1529-8817.2003.00787.x
Leiber-Sauheitl, K., Fuß, R., Voigt, C., and Freibauer, A. (2014). High CO2 Fluxes from Grassland on Histic Gleysol along Soil Carbon and Drainage Gradients. Biogeosciences 11, 749–761. doi:10.5194/bg-11-749-2014
Leifeld, J., and Menichetti, L. (2018). The Underappreciated Potential of Peatlands in Global Climate Change Mitigation Strategies. Nat. Commun. 9. doi:10.1038/s41467-018-03406-6
Leifeld, J., Wüst-Galley, C., and Page, S. (2019). Intact and Managed Peatland Soils as a Source and Sink of GHGs from 1850 to 2100. Nat. Clim. Chang. 9, 945–947. doi:10.1038/s41558-019-0615-5
Lenth, R., Buerkner, P., Herve, M., Love, J., Riebl, H., and Singmann, H. (2020). Emmeans: Estimated Marginal Means, Aka Least-Squares Means. R package version 1.5.1. Available at: https://cran.r-project.org/package=emmeans.
Lloyd, J., and Taylor, J. A. (1994). On the Temperature Dependence of Soil Respiration. Funct. Ecol. 8, 315–323. doi:10.2307/2389824
Lucas-Moffat, A. M., Huth, V., Augustin, J., Brümmer, C., Herbst, M., and Kutsch, W. L. (2018). Towards Pairing Plot and Field Scale Measurements in Managed Ecosystems: Using Eddy Covariance to Cross-Validate CO2 Fluxes Modeled from Manual Chamber Campaigns. Agric. For. Meteorology 256-257, 362–378. doi:10.1016/j.agrformet.2018.01.023
Ma, S., Baldocchi, D. D., Hatala, J. A., Detto, M., and Curiel Yuste, J. (2012). Are Rain-Induced Ecosystem Respiration Pulses Enhanced by Legacies of Antecedent Photodegradation in Semi-arid Environments? Agric. For. Meteorology 154-155, 203–213. doi:10.1016/j.agrformet.2011.11.007
Maljanen, M., Hytönen, J., Mäkiranta, P., Alm, J., Minkkinen, K., Laine, J., et al. (2007). Greenhouse Gas Emissions from Cultivated and Abandoned Organic Croplands in Finland. Boreal Environ. Res. 12, 133–140.
Michaelis, L., and Menten, M. L. (1913). Die Kinetik der Invertinwirkung. Biochemische Z. 49, 333–369.
Moriasi, D. N., Arnold, J. G., Van Liew, M. W., Bingner, R. L., Harmel, R. D., and Veith, T. L. (2007). Model Evaluation Guidelines for Systematic Quantification of Accuracy in Watershed Simulations. Trans. ASABE 50, 885–900. doi:10.13031/2013.23153
Munson, S. M., Benton, T. J., Lauenroth, W. K., and Burke, I. C. (2010). Soil Carbon Flux Following Pulse Precipitation Events in the Shortgrass Steppe. Ecol. Res. 25, 205–211. doi:10.1007/s11284-009-0651-0
Otieno, D. O., Wartinger, M., Nishiwaki, A., Hussain, M. Z., Muhr, J., Borken, W., et al. (2009). Responses of CO2 Exchange and Primary Production of the Ecosystem Components to Environmental Changes in a Mountain Peatland. Ecosystems 12, 590–603. doi:10.1007/s10021-009-9245-5
Parish, F., Sirin, A., Charman, D., Joosten, H., Minayeva, T., Silvius, M., et al. (2008). Assessment on Peatlands, Biodiversity and Climate Change: Main Report. Wageningen: Global Environment Centre, Kuala Lumpur and Wetlands International.
Payero, J. O., Neale, C. M. U., and Wright, J. L. (2004). Comparison of Eleven Vegetation Indices for Estimating Plant Height of Alfalfa and Grass. Appl. Eng. Agric. 20, 385–393. doi:10.13031/2013.16057
Peacock, M., Audet, J., Bastviken, D., Cook, S., Evans, C. D., Grinham, A., et al. (2021). Small Artificial Waterbodies Are Widespread and Persistent Emitters of Methane and Carbon Dioxide. Glob. Change Biol. 27 (20), 5109–5123. doi:10.1111/GCB.15762
Peichl, M., Gažovič, M., Vermeij, I., De Goede, E., Sonnentag, O., Limpens, J., et al. (2018). Peatland Vegetation Composition and Phenology Drive the Seasonal Trajectory of Maximum Gross Primary Production. Sci. Rep. 8, 1–11. doi:10.1038/s41598-018-26147-4
Querner, E. P., Jansen, P. C., van den Akker, J. J. H., and Kwakernaak, C. (2012). Analysing Water Level Strategies to Reduce Soil Subsidence in Dutch Peat Meadows. J. Hydrol. 446-447, 59–69. doi:10.1016/j.jhydrol.2012.04.029
R Core Team (2020). R: A Language and Environment for Statistical Computing. https://www.r-project.org/: R Foundation for Statistical Computing. Vienna, Austria. Available at: https://www.r-project.org/.
Renou-Wilson, F., Müller, C., Moser, G., and Wilson, D. (2016). To Graze or Not to Graze? Four Years Greenhouse Gas Balances and Vegetation Composition from a Drained and a Rewetted Organic Soil under Grassland. Agric. Ecosyst. Environ. 222, 156–170. doi:10.1016/j.agee.2016.02.011
Shen, Z.-X., Yun-Long, L., and Gang, F. (2015). Response of Soil Respiration to Short-Term Experimental Warming and Precipitation Pulses Over the Growing Season in an Alpine Meadow on the Northern Tibet. Appl. Soil Ecol. 90, 35–40. doi:10.1016/j.apsoil.2015.01.015
Stagge, J. H., Tallaksen, L. M., Gudmundsson, L., Van Loon, A. F., and Stahl, K. (2015). Candidate Distributions for Climatological Drought Indices (SPI and SPEI). Int. J. Climatol. 35, 4027–4040. doi:10.1002/joc.4267
Struck, I. J. A., Taube, F., Hoffmann, M., Kluß, C., Herrmann, A., Loges, R., et al. (2020). Full Greenhouse Gas Balance of Silage maize Cultivation Following Grassland: Are No-Tillage Practices Favourable under Highly Productive Soil Conditions? Soil Tillage Res. 200, 104615. doi:10.1016/j.still.2020.104615
Tiemeyer, B., Albiac Borraz, E., Augustin, J., Bechtold, M., Beetz, S., Beyer, C., et al. (2016). High Emissions of Greenhouse Gases from Grasslands on Peat and Other Organic Soils. Glob. Change Biol. 22, 4134–4149. doi:10.1111/gcb.13303
Tiemeyer, B., Freibauer, A., Borraz, E. A., Augustin, J., Bechtold, M., Beetz, S., et al. (2020). A New Methodology for Organic Soils in National Greenhouse Gas Inventories: Data Synthesis, Derivation and Application. Ecol. Indicators 109, 105838. doi:10.1016/j.ecolind.2019.105838
van den Akker, J., Alkemade, H., Van den Berg, M., Bijman, M., Bosma, N., Erkens, G., et al. (2019). Factsheet onderwater- en drukdrainage: Veelgestelde vragen over onderwaterdrainage en drukdrainage (Underwater and pressured drainage factsheet). Available at: https://edepot.wur.nl/547024 (Accessed September 28, 2021).
van den Akker, J., Kuikman, P., De Vries, F., Hoving, I., Pleijter, M., Hendriks, R., et al. (2010). “Emission of CO2 from Agricultural Peat Soils in the Netherlands and Ways to Limit This Emission,” in Proceedings of the 13th International Peat Congress. After Wise Use – The Future of Peatlands, 8–13 June 2008 (Tullamore, Ireland: Oral Presentations), 645–648. Available at: https://edepot.wur.nl/159747.
Waddington, J. M., and Roulet, N. T. (2000). Carbon Balance of a Boreal Patterned Peatland. Glob. Chang. Biol. 6, 87–97. doi:10.1046/j.1365-2486.2000.00283.x
Weideveld, S. T. J., Liu, W., van den Berg, M., Lamers, L. P. M., and Fritz, C. (2021). Conventional Subsoil Irrigation Techniques Do Not Lower Carbon Emissions from Drained Peat Meadows. Biogeosciences 18, 3881–3902. doi:10.5194/bg-18-3881-2021
Wilson, D., Blain, D., Couwenberg, J., Evans, C. D., Murdiyarso, D., Page, S. E., et al. (2016a). Greenhouse Gas Emission Factors Associated with Rewetting of Organic Soils. Mires Peat 17, 1–28. doi:10.19189/MaP.2016.OMB.222
Wilson, D., Farrell, C. A., Fallon, D., Moser, G., Müller, C., and Renou-Wilson, F. (2016b). Multiyear Greenhouse Gas Balances at a Rewetted Temperate Peatland. Glob. Change Biol. 22, 4080–4095. doi:10.1111/gcb.13325
Yamori, W., Hikosaka, K., and Way, D. A. (2014). Temperature Response of Photosynthesis in C3, C4, and CAM Plants: Temperature Acclimation and Temperature Adaptation. Photosynth. Res. 119, 101–117. doi:10.1007/s11120-013-9874-6
Yu, Z., Loisel, J., Brosseau, D. P., Beilman, D. W., and Hunt, S. J. (2010). Global Peatland Dynamics Since the Last Glacial Maximum. Geophys. Res. Lett. 37, a–n. doi:10.1029/2010GL043584
Zeileis, A., and Grothendieck, G. (2005). zoo: S3 Infrastructure for Regular and Irregular Time Series. J. Stat. Softw. 14. doi:10.18637/jss.v014.i06
Keywords: closed-chamber methods, drained peatland, carbon dioxide, CO2 flux modeling, data interpolation
Citation: Liu W, Fritz C, Weideveld STJ, Aben RCH, van den Berg M and Velthuis M (2022) Annual CO2 Budget Estimation From Chamber-Based Flux Measurements on Intensively Drained Peat Meadows: Effect of Gap-Filling Strategies. Front. Environ. Sci. 10:803746. doi: 10.3389/fenvs.2022.803746
Received: 28 October 2021; Accepted: 04 March 2022;
Published: 02 May 2022.
Edited by:
Jörg Luster, Swiss Federal Institute for Forest, Snow and Landscape Research (WSL), SwitzerlandReviewed by:
Mathias Hoffmann, Leibniz Center for Agricultural Landscape Research (ZALF), GermanyMartin Maier, Forstliche Versuchs-und Forschungsanstalt Baden-Württemberg (FVA), Germany
Copyright © 2022 Liu, Fritz, Weideveld, Aben, van den Berg and Velthuis. This is an open-access article distributed under the terms of the Creative Commons Attribution License (CC BY). The use, distribution or reproduction in other forums is permitted, provided the original author(s) and the copyright owner(s) are credited and that the original publication in this journal is cited, in accordance with accepted academic practice. No use, distribution or reproduction is permitted which does not comply with these terms.
*Correspondence: Weier Liu, d2VpZXIubGl1QHJ1Zy5ubA==, bGl1d2VpZXIyQGhvdG1haWwuY29t; Christian Fritz, Q2hyaXN0aWFuLmZyaXR6QHJ1Lm5sIA==