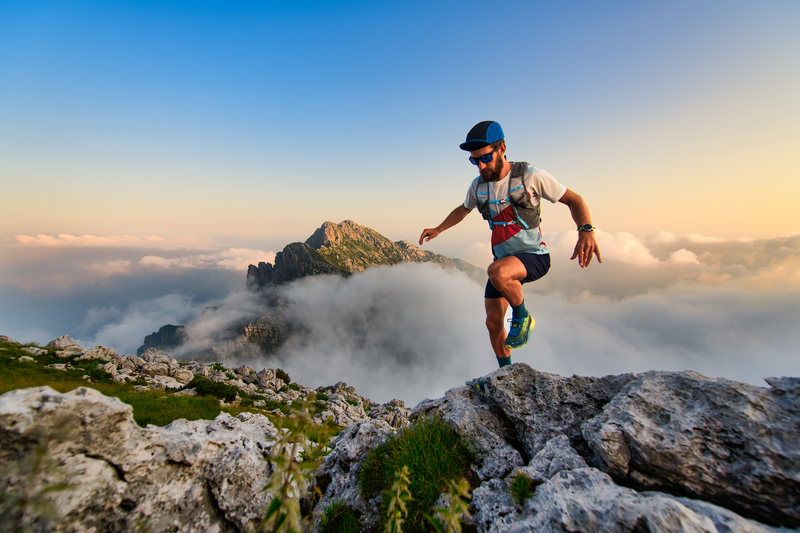
94% of researchers rate our articles as excellent or good
Learn more about the work of our research integrity team to safeguard the quality of each article we publish.
Find out more
BRIEF RESEARCH REPORT article
Front. Environ. Sci. , 31 March 2022
Sec. Land Use Dynamics
Volume 10 - 2022 | https://doi.org/10.3389/fenvs.2022.795197
This article is part of the Research Topic Conservation and Restoration of the Tropical Landscape: Governance and Multidisciplinary Approaches View all 13 articles
The settlement growth is potentially increased by the rural-to-urban perspective change due to the new era of aerotropolis in Kulon Progo. Land-use planning evaluation is required, especially settlement, which has a significant impact on the environment. However, land-use evaluation studies are currently focused on conforming analysis of official land-use planning (OLUP) toward existing or predicted land use partially or in combination with the performance assessment unit. Consequently, it affects the quality of policy products by disregarding crucial considerations of diverse conditions at points of time and aspects of ideality, reality, and regulation. Therefore, the objective of this study was to design a comprehensive policy allocation for settlements using a matrix allocation rule that integrates conformity and performance analysis in three aspects of planning simultaneously. Land allocation was proposed using a geographic information system (GIS) of land capability, settlement suitability, and agricultural and forest land protection. The current land use/land cover (LULC) was classified using visual interpretation of SPOT 7 satellite imagery and a multi-layer perceptron neural network (MLPNN) to predict the LULC in 2035. The result indicated that the stock of land allocation for settlement development is sufficient to meet the demands in 2035. However, there is a problem in the settlement distribution pattern in which 64.3% of existing settlements are located in non-recommended allocation. That number is predicted to increase by 1,145.8 ha. Land-use control instruments need to be conducted to prevent extensive settlement growth in non-recommended allocation. Conversely, zoning allocation should be directed to trigger the growth of settlements in recommended allocation.
Kulon Progo faces an aerotropolis era of regional development due to the construction of Yogyakarta International Airport (YIA). Aerotropolis is a concept of city development centered on the airport (airport city) and emphasizes the surrounding aviation-linked business and residential areas (Kasarda, 2019). The emergence of new airports creates accessibility (Tveter, 2017; Aguirre et al., 2019; Fernandes et al., 2019; Hubbard et al., 2019) and offers added value to the region (Blonigen and Cristea, 2015). Accessibility could trigger development and attract population growth (Baum-Snow, 2010; Chi, 2012; McGraw, 2020). The increasing activity intensity and the growing population stimulate built-up growth, especially settlements (He and Xie, 2019); land use accommodates urban activities such as trade, housing, and services.
The progress in the dynamics of urban the growth of build-up area potentially causes sprawl, which is a big challenge in realizing sustainable development (Bovet et al., 2018). Urban sprawl brings physical impact such as increased emissions (Han, 2020), mass consumption of resources (He et al., 2017), numerous changes in the structure and functioning of landscape (Solon, 2009), conversion of agricultural and forests (Kurnianti et al., 2015; Rustiadi et al., 2020), and socioeconomic problems (Giyarsih, 2010) such as the emergence of slum areas, poverty, and unemployment (Wang and Maduako, 2018).
The evaluation of land-use planning, especially settlements, should be conducted in the early phase of the aerotropolis, considering there is perspective change on regional development to mitigate the emergence of urban sprawl. Land-use planning is an important instrument to prevent urban sprawl (Wilson and Chakraborty, 2013; Bovet et al., 2018). However, the current study (Kassis et al., 2021; Mallma, 2021) has focused its evaluation only on the official land-use plan (OLUP) using performance indicators and on the existing land use (Lyu et al., 2022) to provide recommendations on land-use planning, or evaluated predicted land use in every scenario (Shi et al., 2021; Nijhum et al., 2021) separately. The study of Widiatmaka et al. (2016a) combines the evaluation of OLUP and presents land use using performance analysis. However, the analysis has not included future considerations and has no access to the planning instruments to conduct the policy. The detached analysis has shortcomings that affect the quality of policy product since it did not consider key information at different points in time. Moreover, the policy recommendation of the study that is not linked to the land-use planning instruments is probably difficult to operationalize. Consequently, the evaluation should integrate performance and conformance analysis of the present and future conditions, as well as regulation to provide a policy that accommodates the ideality, reality, and regulation aspect.
A simple decision matrix provides an integrated analysis of policy generations. The simple decision model has the robustness to conduct a policy that involves multiple stakeholders on frequently repeated decisions compared to the complex ones (Katsikopoulos et al., 2018). It is a suitable tool to generate recommendations on spatial planning policy in Indonesia, which is regularly conducted every 5 years with the participatory paradigm involving multiple stakeholders. The geographic information system (GIS) helps analyze the data input by arranging the policy spatially. GIS application has been widely used in spatial planning (AbuSada and Thawaba, 2011; Omar and Raheem, 2016; Ustaoglu and Aydınoglu, 2020). Therefore, the objective of this study was to design policy allocation for settlement development comprehensively using a matrix allocation rule and a geographic information system that integrates unit analysis of evaluation, existing and predicted land use, and the OLUP simultaneously to conduct remediation in OLUP.
The study was located in Kulon Progo Regency, Province of Yogyakarta, Indonesia, with a land area of 57,326.1 ha and a total population of 436,395 in 2020 (Statistic Bureau of Kulon Progo, 2021). The airport development is under the national strategic projects mentioned in Presidential Regulation Number 3 of 2016 and 98 of 2017. The airport construction entered its first phase in 2016–2021, which could accommodate 10 million passengers per year. The ultimate capacity can accommodate 20 million passengers per year, by 2031–2041 (Minister of Transportation, 2013).
Kulon Progo is classified into three types of development areas. First, the north cluster that has an undulating topography with the existence of Menoreh Hills. The Bedah Menoreh project that aims to develop geotourism and create a nature-based tourism village (Local Government Regulation No 2 of 2021) is indicated by the yellow line in Figure 1. Second, the east cluster has a hilly topography accentuated by the new development of the Sentolo industrial estate according to Kulon Progo Regional Spatial Plan 2012–2032, as indicated by the shaded area in Figure 1. The third is the south cluster of Kulon Progo, which is an area with flat topography. The cluster has become the center of settlement growth and infrastructure development.
The data of SPOT 4 of 2010, SPOT 6 of 2015, and SPOT 7 of 2020 satellite imageries were obtained through Indonesia’s National Institute of Aeronautics and Space. Furthermore, base maps and infrastructure facilities were obtained from the Geospatial Information Agency. OLUP data for Kulon Progo 2012–2032 and forest area were downloaded through http://geoportal.kulonprogokab.go.id/. The soil map with a 1:50,000 scale was acquired from the Indonesian Center for Agricultural Land Resources Research and Development. Social, economic, and population data were collected through Statistics Indonesia, while the primary data were obtained through ground check and AHP questionnaire.
The research dissects three processes: analysis of present and future condition of land uses, analysis of the land allocation using multi-criteria decision analysis and a geographic information system, and arrangement of the policies using a matrix allocation rule.
Visual interpretation by on-screen digitizing based on the interpretation key (Lillesand et al., 2015) was conducted to build land use/land cover (LULC) at three time points: 2010, 2015, and 2020. Meanwhile, land use was classified using SNI 7645–1:2014. The ground check verification of 96 spots was held on December 3–10, 2020. The Kappa accuracy of 2010, 2015, and 2020, which validates LULC with the field data, was 81.2%, 81.5%, and 81.9%, respectively.
Several land-use prediction models have been used to model forecasting LULC, such as Cellular Automata (CA) (Aljoufie, 2014; Aljoufie et al., 2016), Cellular Automata-Markov (CA-Markov) (Mitsova et al., 2011; Kurnianti et al., 2015; Gong et al., 2019), SLEUTH (Saxena and Jat, 2020), and Artificial Neural Network (Tayyebi et al., 2011; Morgado et al., 2014; Gong et al., 2014; Bhatti et al., 2015). The CA and ANN combination model is provided by the multi-layer perceptron neural network (MLPNN) found in Modules for Land Use Change Simulation (MOLUSCE) (https://plugins.qgis.org/plugins/molusce/) and has been used by Hossain et al. (2021), Hossain and Moniruzzaman (2021), and Kafy et al. (2021). The forecasting LULC in this research used MOLUSCE in QGIS software 2.18.23 version. This module provided transitional potential modeling using an artificial neural network algorithm that has a high accuracy in building transitional models compared to other processes (Hossain et al., 2021), cellular automata for prediction, and Kappa accuracy for validation.
The prediction used the year 2035 to accommodate the gap of year implementation of the plan, which will be reviewed as an input in the next spatial plan. The prediction was carried out using two models: the simulation model used to validate LULC in 2020 and the simulation model to project the LULC of 2035. The LULC of 2010 and 2015 was selected to generate the first model. The modeling used a 5 × 5 pixel neighborhood to set maximum iteration and pixels for modeling. The iteration of data learning was used 10,000 times to gain stability (Eastman, 2012). The validation process for modeling predictions was conducted by comparing the simulation model LULC of 2020 and the actual land use using the validation panel. The model is said to have good accuracy when it has a Kappa value of at least 0.80 (Eastman, 2012). Furthermore, after validation, it can be used to build projected land use of 2035 with four iterations. One iteration represents 5 different time points (years) considering the trend of LULC change.
The allocation area for settlement development is constructed by considering the component of land capability as a factor of carrying capacity and suitability, which indicates the suitable strategic location of the settlement. Land capability and suitability are useful tools for building management units (De Feudis et al., 2021). Furthermore, paddy fields and forest areas are counted as limiting factors for settlement development. The island of Java, including Kulon Progo, is a national food barn. However, Kulon Progo has not delineated an official food security area and has been faced with massive urbanization, interrupting agricultural land sustainability. On the other hand, settlements are prohibited in the protected area under the Ministry of Environment and Forestry authorities.
Land capability is assessed by matching criteria of land characteristics including topography, drainage, soil texture, erosion, adequate soil depth, and particular factors such as rocks and flooding (Hardjowigeno and Widiatmaka, 2007). It is derived from USDA that was quantified by Arsyad (2012). The land capability class is divided into eight based on limiting factors and conditions in every class. The high to medium classes consist of classes I to IV, which can support cultivation activity. Classes V to VIII belong to the moderate to poor classes, which are prioritized for conservation due to severe inhibiting factors (Arsyad, 2012; Widiatmaka et al., 2015).
Land suitability analysis was conducted using GIS to carry out multi-criteria decision analysis (MCDA) and analytical hierarchy process (AHP) to weight the criteria (Widiatmaka et al., 2016b; Saxena and Jat, 2020). The criteria used include biophysical parameters such as slope, elevation, distance to rivers, distance to the coast, rainfall, and socioeconomic parameters. This consists of distance to road access, city center, trade facilities, transportation facilities, and health and education facilities. Weighting assessment involves experts to achieve an objective approach indicated by a consistency ratio (CR) of less than 10% (Saaty, 2008). Furthermore, the criteria that the expert has scored are measured to determine their influence on other criteria. This is conducted to obtain a relative ranking between criteria through pairwise comparison (Saaty, 2008). The scale of the relative ranking between variables ranged from 1 to 9. The overlay analysis using ArcGIS was calculated following the weight for every criterion to obtain suitability scores. The suitability of settlement is divided into four classes, namely, S1 (very suitable), S2 (fairly suitable), S3 (marginally suitable), and N (not suitable) using natural break.
Land allocation for settlement development is categorized into recommended and non-recommended allocations. Recommended allocation is the area that satisfies the following prerequisites: has high to medium class (I–IV) of land capability, is located in a suitable class (S1, S2, and S3), and is located in non-agricultural land (wetland agriculture) and forest area. Recommended allocation consists of Allocation I, II, and III, determined by their suitability class. Allocation I is the area that has S1 suitability class, and so on. Meanwhile, the area that does not meet the prerequisites is included in the non-recommended allocation.
Policy allocation is decided by a simple decision matrix, considering the land allocation of settlement development, existing LULC of 2020, LULC prediction of 2035, and OLUP of 2012–2032. Furthermore, the policies are prepared using a simple decision matrix rule to be easily applied by stakeholders (Katsikopoulos et al., 2018; Pérez et al., 2020). Land allocation of settlement development represents the ideal area for developing settlements. This allocation has functioned as a performance unit analysis that will evaluate existing, predicted land use, and OLUP. Land uses are divided into three major classes: settlement (S), cultivated (C), and protected area (P), depending on the degree of environmental influence. The evaluation of predicted land use focused on settlement. The matrix integrated with conformance analysis between existing, predicted land use, and OLUP, which represents the regulation aspect. The review of OLUP was dependent on the conformance and performance analysis.
The policy allocation for settlement development is divided into two rules: the rule in the recommended area indicated by Supplementary Figure S1 in which the main policy is to trigger settlement development, and the rule in the non-recommended area indicated by Supplementary Figure S2 involving settlement growth prevention because of the shortage in allocation requirement. The rule follows the combination condition of existing (S/C/P), predicted (S), and OLUP (S/C/P). The combination condition link to the instrument of planning control policy is provided in Local Government of Kulon Progo Regency no. 1 of 2012, including zoning regulation, disincentive and incentive, land permit, and law enforcement. The alternative policy is to review the OLUP due to the irrelevant allocation or the potential threat to the environment.
The Kappa accuracy of the 2020 simulation map that validates the simulation map 2020 with LULC of 2020 is 0.95. The prediction results in 2035 showed that the settlement area would increase from 4,129.3 ha to 5,863.5 ha, indicated by the bar of settlement in Figure 2A. The newly built area indicated by settlement in this research tends to grow near the existing one in accordance with the study of Luo and Wei (2009) and Zhang et al. (2011). It caused the neighborhood land use to have a more significant conversion probability. The mixed garden has a spatial distribution correlation with the settlement that causes the more extensive conversion of the mixed garden. The conversion of wetland agriculture also increased due to the low land rent. Rondhi et al. (2018) reveal that agricultural land rent is lower than housing rent, especially in the peri-urban area that encourages agricultural land conversion. The southern part of Kulon Progo is experiencing peri-urban conditions with the perspective change. The number of conversions tends to increase in the second period shown in Figure 2B, indicating the process of settlement expansion. However, the massive urbanization in the early phase of aerotropolis from the perspective of settlement growth rate remains unnoticed. The growth rate in the first period was only 113 ha and was increased to 168.6 ha in the second one. Compared with the immediate mature stage urban area, the Yogyakarta Urbanized Area (YUA) settlement growth was 329 ha per year in 2003–2013 (Wijaya and Umam, 2016).
Even though the growth rate of settlement is still small, the process of regional perspective change can be identified by the deviation direction of new settlement location growth. The new settlements in 2015–2020 are primarily distributed between W-SW (West-Southwest) or the surrounding airport area. The E-SE (East-Southeast), S-SW (South-Southwest), and SW-W (Southwest-West) areas also experienced an increase, although not as much as the W-SW. Generally, the area on the southern part of settlement’s mean center [indicated by the red dot in Figure 2C, which was adopted from Litasari et al. (2022)] experienced an increase during the aerotropolis period shown by the orange net in Figure 2D and spatially can be seen in Figure 2C. The result is in accordance with the study of Litasari et al. (2022), which applied perspective change using spatial mean center in three time points of settlement development. Pratiwi and Rahardjo (2018) also found increasing land price around the airport area, especially the area with good accessibility near the Daendeles and National road. It indicated that the emergence of the airport as a new growth center potentially triggered the activity shown by the deviation in the settlement’s growth location. The study conducted by Wang et al. (2019) shows that the emergence of transportation infrastructure (in the case of the high-speed railway) could deviate the spatial structure.
Kulon Progo is dominated by non-recommended allocation covering 79% of the total area, which is distributed mainly in Menoreh Hills. According to the study of Hadmoko et al. (2010), the area of Menoreh covering 26,100 ha (45.5% total area of Kulon Progo) is prone to landslide (moderate to high risk) and, thus, is not suitable for settlement. The Bedah Menoreh project shown with a yellow line in Figure 3A will provide access, potentially triggering settlement growth. This area is grouped in Class VII and VIII with the slope as the main obstacle and an area with N suitability class shown in Figures 3B,C.
The remaining percentage is contributed by other obstacles like coarse soil texture in the southern part of Kulon Progo. Soil texture affects the ability of the soil to hold and pass water (Arsyad, 2012). Water can easily pass (and thus difficult to store) in soil with a coarse texture (Lu et al., 2021). In addition, soil with a coarse texture is a poor filtering material and can cause groundwater pollution (Hardjowigeno and Widiatmaka, 2007). Such conditions raise the potential for sanitation problems and water pollution. It is a big challenge due to the development of aerotropolis that stimulates the activities in the southern part of Kulon Progo. The wetland agriculture (Figure 3D) and forest area (Figure 3E) promote to be protected and included in non-recommended allocation. Meanwhile, the areas of recommended allocation I, II, and III are 1,856.11 (3.23%), 4,796.01 (8.37%), and 5,386.36 (9.40%) ha, respectively. The recommended area is sufficient to meet the demands of the settlement in 2035, which covers 5,863.5 ha according to the LULC prediction.
The evaluation indicates that 2,656.53 ha (64.33%) of existing settlement belong to non-recommended allocation and are predicted to increase by 1,145.83 ha in 2035. Widodo et al. (2015) also pointed out that only 22.73% of villages have good land resource carrying capacity in YUA. The number of areas with good carrying capacity in YUA smaller than Kulon Progo is probably affected by the degree of urban growth. Consequently, the development of settlements needs to be conducted carefully according to carrying capacity. The development in Kulon Progo is in the early stages; the supply land is adequate and can still meet the demand. The main problem of settlement development lies in spatial distribution.
It is difficult to demolish or relocate settlements located in non-recommended allocation. The implementation of the policies requires data such as land ownership, cost–benefit analysis, and multidimensional analysis to measure social and economic effects. Demolition may be the alternative when there is a public interest regulated in Law Number 2 of 2012, which provides appropriate and fair compensation. The law can be enforced in the area defined as a protected area in the OLUP following the S-S-P rule in Figure 4A. The rule shows a good performance of the OLUP that conforms to land allocation. However, the existing land use does not conform to land allocation and OLUP. The condition represents land-use infringement. The area included 458.83-ha settlements distributed in Menoreh Hills shown in red color in Figure 4B.
The growth of the rest of the existing settlement outside the protected area is supposed to be limited using the disincentive mechanism. The rule follows the condition of S-S-S/C in Figure 4A. The condition shows conformity between the existing, predicted, and OLUP, but it has a bad performance due to the location that distribute in non-recommended allocation. It is strengthened by the study of Shen et al. (2019), which stated that good conformity does not always represent good performance. The disincentives, in this case, can be given in the form of the imposition of high tax levies, adjusted to the number of costs required to overcome the land-use impacts, restrictions on infrastructure provision, imposition of compensation, and penalties.
The disincentive mechanism can be implemented to the cultivated area potentially converted into settlements. Even though the OLUP and existing land use have conformity and good performance, it potentially can be converted into settlement in the future. The rule follows the condition of C-S-C/P. A study of Widiatmaka et al. (2016) concludes that the condition is fairly without problem because the study obviates the dynamic change of future conditions. The settlement does not exist but potentially will grow in non-recommended allocation, so the policy is required to prevent conversion. Dadashpoor and Malekzadeh (2020) found that disincentives affect the spatial structure by preventing the growth of metropolitan areas through social welfare, rule, and regulation. The mechanism that can be used is to reject the proposed permit of settlement utilization and restrict the infrastructure provision. The area recommended proposed a disincentive policy covering the entire settlement in Kulon Progo indicated by dark purple in Figure 4B with a cover area of 2,730.5 ha.
The lack of a spatial plan cannot be tackled due to the changing condition that needs to be evaluated and revised (Faludi, 2000). The policy to revise the zoning allocation is required when the existing condition has a good performance, whereas the OLUP has a poor performance. It is indicated by the condition of C-S-S or P-S-S/C. Indeed, it has a high probability that it will be converted into settlement in the future. The settlement allocation adjusted in that area will burden the environment due to the exceeded land capacity. Meanwhile, the condition of P-S-P has good conformance and performance for both the OLUP and the existing condition, although it has potentially converted into settlement. Thus, it required incentive policies to preserve the allocation of land use. The incentive is an effective tool to protect the landscape and is better applied in privately owned lands (Wainaina et al., 2021). Using this allocation matrix rule, approximately 168.5 ha of protected area and 400.8 ha of wetland agriculture can be preserved. The settlement that potentially grows in the non-recommended allocation can be triggered to grow in the stock area. The amount of allocation stock until 2035 covers 9.968,08 ha, indicated by the yellow polygon in Figure 4B.
Conversely, the existing and projected settlements located in the recommended allocation are supposed to be stimulated to grow. The zoning allocation is targeted to an area of 1,053.47 to trigger the settlement growth. It only needs details on the priority hierarchy to follow the rule in Figure 4C. The hierarchy of prioritization utilization in recommended allocation is divided into the following:
• Priority I: located in Allocation I and prioritized for the designation of settlements with high density.
• Priority II: distributed in Allocation II and allocated for settlements with medium to low density because of some obstacles that need some management.
• Priority III: areas that can be used for settlement expansion that will be used when the area in Allocations I and II has been exceeded. This area is located in Allocation 3.
The existing settlements that have been regulated in zoning allocation cover an area of 751.24 ha distributed in Allocation I, II, and III. However, 721.53 ha is not adjusted to settlements in OLUP. On the other hand, the total predicted settlement located in recommended allocation is 595.34 ha, most of which is distributed in Allocation II. However, only 292.23 ha have been regulated in zoning allocation. Although this area has a good performance in supporting activity, the policy to allocate using the stipulation settlement zoning allocation is required. A zoning allocation is a tool that can manage the distribution of settlements to prevent sprawling (Chadchan and Shankar, 2012).
The evaluation reveals that the present settlements are dominantly distributed in non-recommended allocation and are predicted to increase in 2035. The stock of land allocation for settlement development is adequate to fill the settlement demand in 2035, which is represented by the area of predicted settlements. The problem lies in the distribution pattern rather than the lack of appropriate area for settlement development. The policy allocation matrix rule fills the gap linking policy recommendation to the planning control instruments by managing the distribution of settlement growth. The rule integrates the performance and conformance between the land allocation for settlement development accommodating the ideality aspect, existing and projected settlement that represents the reality aspect, and OLUP as an aspect of regulation. Using this method, the potential conversion of the area with low carrying capacity, wetland agriculture, and forest area can be mitigated and triggered to grow in recommended allocation. The research confined the evaluation to build a policy allocation only on the settlement. A broader analysis and framework can be developed to accommodate all land-use evaluation in OLUP.
The original contributions presented in the study are included in the article/Supplementary Material, further inquiries can be directed to the corresponding author.
Conceptualization, UL, WW, and KM; methodology, UL, WW, KM, and MM; manuscript review, WW, KM, and MM; formal analysis, data curation, and writing original draft preparation, UL.
This study was fully funded by the Indonesian Ministry of Education, Culture, Research, and Technology contract number 6568/IT3.L1/PN/2020 in the scheme of Master’s Education Program Towards a Doctorate for Excellent Undergraduates (PMDSU).
The authors declare that the research was conducted in the absence of any commercial or financial relationships that could be construed as a potential conflict of interest.
All claims expressed in this article are solely those of the authors and do not necessarily represent those of their affiliated organizations, or those of the publisher, the editors, and the reviewers. Any product that may be evaluated in this article, or claim that may be made by its manufacturer, is not guaranteed or endorsed by the publisher.
The authors are grateful to the IPB University for providing facilities to support the study. Furthermore, we are grateful to Indonesia’s National Institute of Aeronautics and Space and Central Bureau of Statistics for providing the raw data.
The Supplementary Material for this article can be found online at: https://www.frontiersin.org/articles/10.3389/fenvs.2022.795197/full#supplementary-material
AbuSada, J., and Thawaba, S. (2011). Multi Criteria Analysis for Locating Sustainable Suburban Centers: A Case Study from Ramallah Governorate, Palestine. Cities 28 (5), 381–393. doi:10.1016/j.cities.2011.05.001
Aguirre, J., Mateu, P., and Pantoja, C. (2019). Granting Airport Concessions for Regional Development: Evidence from Peru. Transport Policy 74, 138–152. doi:10.1016/j.tranpol.2018.12.003
Aljoufie, M., Brussel, M., Zuidgeest, M., van Delden, H., and van Maarseveen, M. (2016). Integrated Analysis of Land-Use and Transport Policy Interventions. Transport. Plann. Techn. 39 (4), 329–357. doi:10.1080/03081060.2016.1160578
Aljoufie, M. (2014). Toward Integrated Land Use and Transport Planning in Fast-Growing Cities: The Case of Jeddah, Saudi Arabia. Habitat Int. 41, 205–215. doi:10.1016/j.habitatint.2013.08.010
Baum-Snow, N. (2010). Changes in Transportation Infrastructure and Commuting Patterns in US Metropolitan Areas, 1960-2000. Am. Econ. Rev. 100 (2), 378–382. doi:10.1257/aer.100.2.378
Bhatti, S. S., Tripathi, N. K., Nitivattananon, V., Rana, I. A., and Mozumder, C. (2015). A Multi-Scale Modeling Approach for Simulating Urbanization in a Metropolitan Region. Habitat Int. 50, 354–365. doi:10.1016/j.habitatint.2015.09.005
Blonigen, B. A., and Cristea, A. D. (2015). Air Service and Urban Growth: Evidence from a Quasi-Natural Policy Experiment. J. Urban Econ. 86, 128–146. doi:10.1016/j.jue.2015.02.001
Bovet, J., Reese, M., and Köck, W. (2018). Taming Expansive Land Use Dynamics - Sustainable Land Use Regulation and Urban Sprawl in a Comparative Perspective. Land Use Policy 77, 837–845. doi:10.1016/j.landusepol.2017.03.024
Chadchan, J., and Shankar, R. (2012). An Analysis of Urban Growth Trends in the post-economic Reforms Period in India. Int. J. Sustain. Built Environ. 1, 36–49. doi:10.1016/j.ijsbe.2012.05.001
Chi, G. (2012). The Impacts of Transport Accessibility on Population Change across Rural, Suburban and Urban Areas: A Case Study of Wisconsin at Sub-county Levels. Urban Stud. 49 (12), 2711–2731. doi:10.1177/0042098011431284
Dadashpoor, H., and Malekzadeh, N. (2020). Driving Factors of Formation, Development, and Change of Spatial Structure in Metropolitan Areas: A Systematic Review. J. Urban Manage. 9, 286–297. doi:10.1016/j.jum.2020.06.005
De Feudis, M., Falsone, G., Gherardi, M., Speranza, M., Vianello, G., and Vittori Antisari, L. (2021). GIS-based Soil Maps as Tools to Evaluate Land Capability and Suitability in a Coastal Reclaimed Area (Ravenna, Northern Italy). Int. Soil Water Conserv. Res. 9 (2), 167–179. doi:10.1016/j.iswcr.2020.11.007
Faludi, A. (2000). The Performance of Spatial Planning. Plann. Pract. Res. 15 (4), 299–318. doi:10.1080/713691907
Fernandes, V. A., Pacheco, R. R., Fernandes, E., and da Silva, W. R. (2019). Regional Change in the Hierarchy of Brazilian Airports 2007-2016. J. Transport Geogr. 79, 102467. doi:10.1016/j.jtrangeo.2019.102467
Giyarsih, S. R. (2010). Urban Sprawl of the City of Yogyakarta, Special Preference to the Stage of Spatial Transformation. Indones. J. Geogr. 42 (1), 47–58. doi:10.22146/ijg.1576
Gong, J., Chen, W., Liu, Y., and Wang, J. (2014). The Intensity Change of Urban Development Land: Implications for the City Master Plan of Guangzhou, China. Land Use Policy 40, 91–100. doi:10.1016/j.landusepol.2013.05.001
Gong, X., Bian, J., Wang, Y., Jia, Z., and Wan, H. (2019). Evaluating and Predicting the Effects of Land Use Changes on Water Quality Using SWAT and CA-Markov Models. Water Resour. Manage. 33 (14), 4923–4938. doi:10.1007/s11269-019-02427-0
Hadmoko, D. S., Lavigne, F., Sartohadi, J., Hadi, P., and Winaryo, W. (2010). Landslide Hazard and Risk Assessment and Their Application in Risk Management and Landuse Planning in Eastern Flank of Menoreh Mountains, Yogyakarta Province, Indonesia. Nat. Hazards 54, 623–642. doi:10.1007/s11069-009-9490-0
Han, J. (2020). Can Urban Sprawl Be the Cause of Environmental Deterioration? Based on the Provincial Panel Data in China. Environ. Res. 189, 109954. doi:10.1016/j.envres.2020.109954
Hardjowigeno, S., and Widiatmaka, W. (2007). Evaluasi Kesesuaian Lahan Dan Perencanaan Tataguna Lahan. Yogyakarta: Gadjah Mada University Press.
He, Y., and Xie, H. (2019). Exploring the Spatiotemporal Changes of Ecological Carrying Capacity for Regional Sustainable Development Based on GIS: A Case Study of Nanchang City. Technol. Forecast. Soc. Change 148, 119720. doi:10.1016/j.techfore.2019.119720
He, Q., Song, Y., Liu, Y., and Yin, C. (2017). Diffusion or Coalescence? Urban Growth Pattern and Change in 363 Chinese Cities from 1995 to 2015. Sustain. Cities Soc. 35, 729–739. doi:10.1016/j.scs.2017.08.033
Hossain, F., and Moniruzzaman, D. M. (2021). Environmental Change Detection through Remote Sensing Technique: A Study of Rohingya Refugee Camp Area (Ukhia and Teknaf Sub-district), Cox's Bazar, Bangladesh. Environ. Challenges 2, 100024. doi:10.1016/j.envc.2021.100024
Hossain, M. T., Zarin, T., Sahriar, M. R., and Haque, M. N. (2021). Machine Learning Based Modeling for Future Prospects of Land Use Land Cover Change in Gopalganj District, Bangladesh. Phys. Chem. Earth, 103022. doi:10.1016/j.pce.2021.103022
Hubbard, J. R., Mitra, S., and Miller, C. R. (2019). Analyzing Human Capital as a Component of the Aerotropolis Model. J. Air Transport Manage. 78, 63–70. doi:10.1016/j.jairtraman.2019.04.008
Kafy, A. A., Dey, N. N., Al Rakib, A., Rahaman, Z. A., Nasher, N. M. R., and Bhatt, A. (2021). Modeling the Relationship between Land Use/land Cover and Land Surface Temperature in Dhaka, Bangladesh Using CA-ANN Algorithm. Environ. Challenges 4, 100190. doi:10.1016/j.envc.2021.100190
Kasarda, J. D. (2019). “Aerotropolis,” in The Wiley Blackwell Encyclopedia of Urban and Regional Studies, 1–7. New York: John Wiley & Sons Inc. doi:10.1002/9781118568446.eurs0436
Kassis, G., Bertrand, N., and Pecqueur, B. (2021). Rethinking the Place of Agricultural Land Preservation for the Development of Food Systems in Planning of Peri-Urban Areas: Insights from Two French Municipalities. J. Rural Stud. 86, 366–375. doi:10.1016/j.jrurstud.2021.07.003
Katsikopoulos, K. V., Durbach, I. N., and Stewart, T. J. (2018). When Should We Use Simple Decision Models? A Synthesis of Various Research Strands. Omega 81, 17–25. doi:10.1016/j.omega.2017.09.005
Kurnianti, D. N., Rustiadi, E., and Baskoro, D. P. T. (2015). Land Use Projection for Spatial Plan Consistency in Jabodetabek. Indones. J. Geogr. 47, 124–131. doi:10.22146/ijg.9249
Lillesand, T. M., Kiefer, R. W., and Chipman, J. W. (2015). Remote Sensing and Image Interpretation. United States: Petra Recter.
Litasari, U. C. N., Widiatmaka, W., Munibah, K., and Machfud, M. (2022). Spatial Pattern Changing Analysis of Built-Up Due to the New Era of Aerotropolis in Kulon Progo, D. I. Yogyakarta. IOP Conf. Ser. Earth Environ. Sci. 950, 012100. doi:10.1088/1755-1315/950/1/012100
Lu, J., Shao, G., Gao, Y., Zhang, K., Wei, Q., and Cheng, J. (2021). Effects of Water Deficit Combined with Soil Texture, Soil Bulk Density and Tomato Variety on Tomato Fruit Quality: A Meta-Analysis. Agric. Water Manage. 243, 106427. doi:10.1016/j.agwat.2020.106427
Luo, J., and Wei, Y. H. D. (2009). Modeling Spatial Variations of Urban Growth Patterns in Chinese Cities: The Case of Nanjing. Landscape Urban Plann. 91, 51–64. doi:10.1016/j.landurbplan.2008.11.010
Lyu, Y., Wang, M., Zou, Y., and Wu, C. (2022). Mapping Trade-Offs Among Urban Fringe Land Use Functions to Accurately Support Spatial Planning. Sci. Total Environ. 802, 149915. doi:10.1016/j.scitotenv.2021.149915
Torres Mallma, S. F. (2021). Mainstreaming Land Use Planning into Disaster Risk Management: Trends in Lima, Peru. Int. J. Disaster Risk Reduct. 62, 102404. doi:10.1016/j.ijdrr.2021.102404
McGraw, M. J. (2020). The Role of Airports in City Employment Growth, 1950–2010. J. Urban Econ. 116, 103240. doi:10.1016/j.jue.2020.103240
Minister of Transportation (2013). Decree of the Minister of Transportation Number: KP.1164 of 2013 Concerning Location Determination of New Airport in Kulon Progo Regency, Yogyakarta Province. Jakarta: Minister of Transportation.
Mitsova, D., Shuster, W., and Wang, X. (2011). A Cellular Automata Model of Land Cover Change to Integrate Urban Growth with Open Space Conservation. Landscape Urban Plann. 99 (2), 141–153. doi:10.1016/j.landurbplan.2010.10.001
Morgado, P., Gomes, E., and Costa, N. (2014). Competing Visions? Simulating Alternative Coastal Futures Using a GIS-ANN Web Application. Ocean Coastal Manage. 101, 79–88. doi:10.1016/j.ocecoaman.2014.09.022
Nijhum, F., Westbrook, C., Noble, B., Belcher, K., and Lloyd-Smith, P. (2021). Evaluation of Alternative Land-Use Scenarios Using an Ecosystem Services-Based Strategic Environmental Assessment Approach. Land Use Policy 108, 105540. doi:10.1016/j.landusepol.2021.105540
Omar, N. Q., and Raheem, A. M. (2016). Determining the Suitability Trends for Settlement Based on Multi Criteria in Kirkuk, Iraq. Open Geospatial Data Soft. Stand. 1 (1), 1–9. doi:10.1186/s40965-016-0011-2
Pérez, W., Arias, R., Taipe, A., Ortiz, O., Forbes, G. A., Andrade-Piedra, J., et al. (2020). A Simple, Hand-Held Decision Support Designed Tool to Help Resource-Poor Farmers Improve Potato Late Blight Management. Crop Prot. 134, 105186. doi:10.1016/j.cropro.2020.105186
Pratiwi, S. E., and Rahardjo, N. (2018). Pemodelan Spasial Harga Lahan Dan Perubahannya Akibat Pembangunan Bandara New Yogyakarta International Airport Di Sekitar Area Bandara. J. Bumi Indones. 7 (3), 1–12.
Rondhi, M., Pratiwi, P. A., Handini, V. T., Sunartomo, A. F., and Budiman, S. A. (2018). Agricultural Land Conversion, Land Economic Value, and Sustainable Agriculture: A Case Study in East Java, Indonesia. Land 7 (4), 1–19. doi:10.3390/land7040148
Rustiadi, E., Pravitasari, A. E., Setiawan, Y., Mulya, S. P., Pribadi, D. O., and Tsutsumida, N. (2020). Impact of Continuous Jakarta Megacity Urban Expansion on the Formation of the Jakarta-Bandung Conurbation over the Rice Farm Regions. Cities 111, 103000. doi:10.1016/j.cities.2020.103000
Saaty, T. L. (2008). Decision Making with the Analytic Hierarchy Process. IJSSCI 1 (1), 83–98. doi:10.1504/ijssci.2008.017590
Saxena, A., and Jat, M. K. (2020). Land Suitability and Urban Growth Modeling: Development of SLEUTH-Suitability. Comput. Environ. Urban Syst. 81, 101475. doi:10.1016/j.compenvurbsys.2020.101475
Shen, X., Wang, X., Zhang, Z., Lu, Z., and Lv, T. (2019). Evaluating the Effectiveness of Land Use Plans in Containing Urban Expansion: An Integrated View. Land Use Policy 80, 205–213. doi:10.1016/j.landusepol.2018.10.001
Shi, X., Zhou, F., and Wang, Z. (2021). Research on Optimization of Ecological Service Function and Planning Control of Land Resources Planning Based on Ecological Protection and Restoration. Environ. Techn. Innovation 24, 101904. doi:10.1016/j.eti.2021.101904
Solon, J. (2009). Spatial Context of Urbanization: Landscape Pattern and Changes between 1950 and 1990 in the Warsaw Metropolitan Area, Poland. Landscape Urban Plann. 93, 250–261. doi:10.1016/j.landurbplan.2009.07.012
Statistic Bureau of Kulon Progo (2021). Kulon Progo Regency in Figures 2020. Available at: https://kulonprogokab.bps.go.id/publication.html (Accessed June 20, 2021).
Tayyebi, A., Pijanowski, B. C., and Pekin, B. (2011). Two Rule-Based Urban Growth Boundary Models Applied to the Tehran Metropolitan Area, Iran. Appl. Geogr. 31 (3), 908–918. doi:10.1016/j.apgeog.2011.01.018
Tveter, E. (2017). The Effect of Airports on Regional Development: Evidence from the Construction of Regional Airports in Norway. Res. Transport. Econ. 63, 50–58. doi:10.1016/j.retrec.2017.07.001
Ustaoglu, E., and Aydınoglu, A. C. (2020). Suitability Evaluation of Urban Construction Land in Pendik District of Istanbul, Turkey. Land Use Policy 99, 104783. doi:10.1016/j.landusepol.2020.104783
Wainaina, P., Minang, P. A., Nzyoka, J., Duguma, L., Temu, E., and Manda, L. (2021). Incentives for Landscape Restoration: Lessons from Shinyanga, Tanzania. J. Environ. Manage. 280, 111831. doi:10.1016/j.jenvman.2020.111831
Wang, J., and Maduako, I. N. (2018). Spatio-temporal Urban Growth Dynamics of Lagos Metropolitan Region of Nigeria Based on Hybrid Methods for LULC Modeling and Prediction. Eur. J. Remote Sensing 51 (1), 251–265. doi:10.1080/22797254.2017.1419831
Wang, S., Wang, J., and Liu, X. (2019). How Do Urban Spatial Structures Evolution in the High-Speed Rail Era? Case Study of Yangtze River Delta, China. Habitat Int. 93, 102051. doi:10.1016/j.habitatint.2019.102051
Widiatmaka, , Ambarwulan, W., Setiawan, Y., Purwanto, M. Y. J., Taryono, T., and Effendi, H. (2015). Land Use Planning for Brackish Water Shrimp Ponds in the North Coast of Tuban, Indonesia. Indones. J. Geogr. 47 (2), 194–211. doi:10.22146/ijg.9268
Widiatmaka, W., Ambarwulan, W., Ambarwulan, Y., and Walter, C. (2016a). Assessing the Suitability and Availability of Land for Agriculture in Tuban Regency, East Java, Indonesia. Appl. Environ. Soil Sci. 2016, 1–13. doi:10.1155/2016/7302148
Widiatmaka, W., Ambarwulan, W., and Sudarsono, S. (2016b). Spatial Multi-Criteria Decision Making for Delineating Agricultural Land in Jakarta Metropolitan Area’s Hinterland: Case Study of Bogor Regency, West Java. Agrivita 38 (2), 105–115. doi:10.17503/agrivita.v38i2.746
Widodo, B., Lupyanto, R., Sulistiyono, B., Harjito, D. A., Hamidin, J., Hapsari, E., et al. (2015). Analysis of Environmental of Carrying Capacity for the Development of Sustainable Settlement in Yogyakarta Urban Area. Proc. Environ. Sci. 28, 519–527. doi:10.1016/j.proenv.2015.07.062
Wijaya, M. S., and Umam, N. (2016). Permodelan Spasial Perkembangan Fisik Perkotaan Yogyakarta Menggunakan Model Cellular Automata Dan Regresi Logistik Biner. Maj. Ilm. Globe 17, 165–172. doi:10.24895/MIG.2015.17-2.227
Wilson, B., and Chakraborty, A. (2013). The Environmental Impacts of Sprawl: Emergent Themes from the Past Decade of Planning Research. Sustainability 5 (8), 3302–3327. doi:10.3390/su5083302
Keywords: evaluation assessment, simple decision matrix, spatial planning, urban growth, zoning regulation
Citation: Litasari UCN, Widiatmaka W, Munibah K and Machfud M (2022) Policy Allocation for Settlement Development Using Simple Allocation Matrix Rules and Geographic Information System. Front. Environ. Sci. 10:795197. doi: 10.3389/fenvs.2022.795197
Received: 14 October 2021; Accepted: 16 February 2022;
Published: 31 March 2022.
Edited by:
Mohamed Kefi, Centre de Recherches et des Technologies des Eaux, TunisiaReviewed by:
Douglas Baker, Queensland University of Technology, AustraliaCopyright © 2022 Litasari, Widiatmaka, Munibah and Machfud. This is an open-access article distributed under the terms of the Creative Commons Attribution License (CC BY). The use, distribution or reproduction in other forums is permitted, provided the original author(s) and the copyright owner(s) are credited and that the original publication in this journal is cited, in accordance with accepted academic practice. No use, distribution or reproduction is permitted which does not comply with these terms.
*Correspondence: Ulfah Choerunnisa Nurul Litasari, Y2hvZXJ1bm5pc2F1bGZhaEBnbWFpbC5jb20=
Disclaimer: All claims expressed in this article are solely those of the authors and do not necessarily represent those of their affiliated organizations, or those of the publisher, the editors and the reviewers. Any product that may be evaluated in this article or claim that may be made by its manufacturer is not guaranteed or endorsed by the publisher.
Research integrity at Frontiers
Learn more about the work of our research integrity team to safeguard the quality of each article we publish.