- 1West Chester University, West Chester, PA, United States
- 2Community College of Philadelphia, Philadelphia, PA, United States
Microorganisms play a critical role in the structure and functioning of soil ecosystems. Within acidic soil across the northeastern United States and Canada, we have little understanding of the microbial diversity present and its relationship to the biochemical cycles. The current study is aimed at understanding the taxonomical and functional diversities in the acidic soil obtained from near various types of trees, how the diversities change as a function of depth, and the linkage between taxonomical and functional diversities. From eight sampling locations, soil samples were collected from three horizons (depths). The three depths were 0–10 cm (A), 11–25 cm (B), and 26–40 cm (C). Results indicate that across all the samples analyzed, Bradyrhizobium and Candidatus Solibacter are the most abundant bacteria in the soil microbiome. The differences in the soil microbiome across the samples were attributed to the abundance of individual organism’s present in the soil and not to the presence or absence of individual organisms. Subsystem level analysis of the soil microbiome sequences indicate that there is higher level of abundance of genes attributed to regulation and cell signaling. A low level of sequences were detected for sulfur metabolism, potassium metabolism, iron acquisition and metabolism, and phosphorous metabolism. Structure-functional analysis indicate that Bradyrhizobium, Rhodopseudomonas, and Burkholderia are the major organisms involved in the nutritional ecosystem functioning within acidic soil. Based on the results, we propose utilizing a consortium of these organisms as an environmentally friendly alternative to the use of chemicals to maintain soil fertility and ecosystem functioning.
Introduction
Soil microorganisms are the largest biodiversity pool on earth, with more than 1030 microbial cells, 104–106 species, and nearly 1,000 Gbp of microbial genome per gram of soil (Vogel et al., 2009; Mendes and Tsai, 2018). They are the primary factors that affect the soil ecosystem functioning and play key roles in forming and maintaining a multitude of soil characteristics including integrity, fertility, ecology, and overall soil function (Shah et al., 2011). Soil microorganisms are also vital for decomposition, pollutant removal, recycling of essential elements, suppressing plant diseases found in soil, and promoting growth for vegetation (Garbeva et al., 2004). Much is known of the microbial taxa present in soils from across the planet and the impact of perturbation of soil conditions. A Google Scholar search for the term “soil microbial diversity” reveals over 1.6 million hits. Nevertheless, our understanding of how microbial diversity and ecosystem functions are linked, and how each of the microbial taxa present in the soil are linked to the individual ecological functions remain limited.
Increased use of 16s rDNA metagenomic methodology using pyrosequencing and Illumina Miseq and Hiseq techniques, has increased our understanding of the taxonomy of soil microorganisms by orders of magnitude. However, the 16S rDNA sequencing method has numerous limitations including differentiating closely related species (Hasan et al., 2014), non-uniform distribution of sequence dissimilarity among taxa, presence of multiple copies of the 16S rRNA gene (Garrity et al., 2009), failure of target amplification of polymerase chain reaction (PCR) primers (Venter et al., 2004), and generation of chimeric sequences (Quince et al., 2009). Further, in majority of these research, the role of individual microorganisms in the soil remains at the level of hypothesis based on prior literature (examples include our own prior research: Kumar et al., 2011; Collins et al., 2012). Methods such as Biolog, Fungilog, and soil enzyme activity are many times used in studies as indicators of the ecosystem functioning and correlation to the taxonomic data (Nannipieri et al., 2002; Sobek and Zak, 2003; Rutgers et al., 2016). While a step forward, these methods are primarily predictive of soil microbial functional dynamics (Bell et al., 2009).
Whole genome shotgun metagenomics provide a better approach for obtaining the taxonomic and functional aspects of the entire soil microbial genome. This method yields millions to billions of short reads, providing necessary sequencing depth as needed. It also offers an opportunity to identify organisms present in the microbiome and the biochemical pathway information present at the genomic level in each of the identified organisms. Shotgun metagenomic study has been used to elucidate the microbiome present in soil (Enagbonma et al., 2019; Edwards et al., 2013), rhizosphere (Babalola et al., 2021; Akinola et al., 2021), waste water and sludge samples (Delforno et al., 2017; Ibarbalz et al., 2016) and samples from international space station (Singh et al., 2018). In this study, we employ shotgun metagenomic approach to identify and quantitate bacterial species present in the acidic soil and elucidate the major ecological functions of major organisms.
Acidic soil typically has a pH range of 4.0–4.5, is high in iron and aluminum, and is often considered nutrient-poor. Across the eastern United States and southeastern Canada, soil is primarily acidic (Bruulsema, 2006). The acidic conditions in the soil of the region is primarily attributed to the parent materials of the soil and increased precipitation that leaches cations from the soil. The soil is optimal for the growth of trees like Apple, Beech, Dogwood, Oak, and Magnolia, and Pears. In addition, plants from heath family (huckleberries, blueberries, and cranberries) do well in acidic soil. Literature search indicates that no reports are available studying the structure–function relationship in the natural acidic soil from the region. Such information is critical to understand how within such soil is the ecosystem functioning and how to ensure the ecosystem remains functional for optimal agricultural productivity.
The current study focused on understanding the taxonomical and functional diversities in the acidic soil obtained from near various types of trees, how the diversities change as a function of depth, and the linkage between taxonomical and functional diversities. We address three questions in the study: 1) what are the major microorganisms that are common to acidic soil types and depth? 2) what are the biochemical pathways that can be generalized across all acidic soil types and depth? 3) What role do each of these organisms play in the ecosystem functioning in the soil?
Methodology
Sample Collection
Samples were collected initially from eight sampling locations across the West Chester University Campus in West Chester, PA. No permit was required to obtain samples. Table 1 describes the analyzed eight locations, the types of vegetation present at each sampling location, as well as sampling coordinates. Sampling coordinates were recorded using Google map app downloaded on an android smart phone. The sampling locations were selected based on the vegetation present and initial sampling of 25 different locations. The final locations were selected based on the similarity of the vegetation between sampling locations, pH levels, and the quantity/quality of the DNA isolated. The protocols for the safety of data collection were strictly followed as recommended by the U.S. Fish and Wildlife Services and the Foundation for Ecological Research in the Northeast (Batcher, 2005). At each location, soil samples were collected from three horizons: 0–5 cm (Horizon A); 6–15 cm (Horizon B) and 16–30 cm (Horizon C). All distinguishable debris and pebbles were removed using sterile forceps, and the soil was mixed thoroughly prior to analysis to yield a homogenized sample.
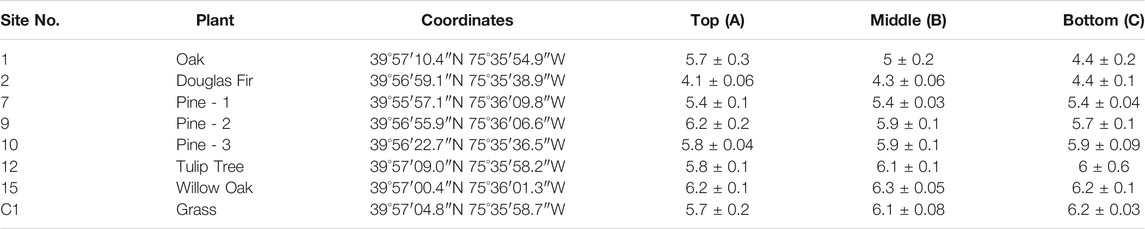
TABLE 1. Vegetation type, coordinates, and pH for the locations from where the soil samples were obtained along with the pH values at each of the three sampling depths for each location. (0–5 cm, Horizon A; 6–15 cm, Horizon B; 16–30 cm, Horizon C).
pH Measurement
5 g soil samples were mixed with 10 ml d/w and vortexed for 10 min. The solution was allowed to sit for 1 h and pH measured of the settled solution. All measurements were done in triplicates.
DNA Extraction and Shotgun Metagenomics
DNA extraction from each soil sample was carried out using the Qiagen DNeasy PowerSoil DNA Isolation Kit, according to the manufacturer’s protocol. DNA isolation was performed in triplicates for each sampling location and the samples were pooled prior to analysis. DNA concentration in all samples were determined using the Qubit three Fluorometer (Invitrogen Technologies). All the samples were diluted to 100 ng/μL and used for the library preparation, using the Nextera Dna Flex Library Preparation kit (Illumina, San Diego, CA), according to the manufacturer’s protocol. Cluster generation and sequencing were performed with the MiSeq Reagent Kit v2 500-cycles Paired-End in a MiSeq instrument at West Chester University. Samples were sequenced in a batch of 24 samples on a single flow cell. DNA sequences were annotated with Metagenomics Rapid Annotation (MG-RAST) pipeline version 4.0 for downstream analyses. Taxonomic and functional profiles were generated using the normalized abundance of sequence matches to the Refseq and Subsystems databases, respectively. All settings were set at default values prior to analysis (maximum e-value cutoff, 1e−5; minimum % identity cutoff, 60%; minimum alignment length cutoff, 15). The sequences have been deposited and are available through the NCBI BioProject Database ID: PRJNA719140.
Clustering Analysis
Clustering analysis were performed using Statistica (release 14.0) software. The tree cluster analysis was performed using Ward’s method as the amalgamation rule and the distance measured as the Euclidean distances. The method was chosen as the cluster membership is assessed by calculating the total sum of squared deviations from the mean of a cluster (Shah et al., 2011). Prior to clustering analysis, data obtained from MG-RAST were log2 transformed and DSeq normalized.
Results
Soil pH
pH for all the soil samples analyzed in this study were in the acidic range of 4.1–6.3 (Table 1). Results show that the type of tree clearly influences the soil pH, with soil around Douglas Fir being the most acidic soil amongst all the types studied. No significant difference in pH was observed across the depths, except for soil obtained around the Oak tree (Table 1), where a stark drop in pH was observed as we go from depth A (pH, 5.7) to depth C (pH, 4.4).
Sequencing Analyses and Microbial Community Diversity
A total of 22,745,412 raw sequence reads were generated for the 24 samples using the Illumina Miseq sequencing platform. 96.2% of the sequences passed the Illumina Chasity Filter for a total of 21,880,208 PF reads. MG-RAST analysis of the submitted reads yielded 8,219,706 total sequences (Supplementay Table S1). Over 99% of the sequences were annotated, with almost equal distribution of known proteins (4,073,051) and proteins of unknown function (4,073,868) (Supplementay Table S1).
The rarefaction curves indicate high genetic diversity, with no complete saturation observed even after almost 8 million sequences (Supplementary Figure S1). For all the samples, the curve has slowly begun to flatten, indicating a reasonable number of species have been sampled. The mean alpha diversity observed was 479, with the range from 417 to 547 species (Supplementay Table S1).
Taxonomic Characterization of Soil Microbiome
Taxonomically, all soil samples had bacterial populations from 50 to 57 phylum. Bacteria belonging to Proteobacteria and Actinobacteria were the most predominant bacteria, comprising over 60% of the total microbial community in each of the samples analyzed (Figure 1)
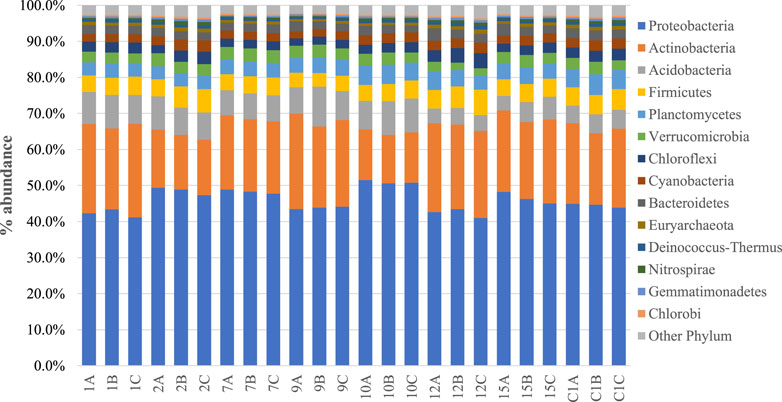
FIGURE 1. Phyla comparison of soil microbiomes collected from eight sampling locations at three different horizons (A, 0–10 cm; B, 10–20 cm; C, 20–30 cm).
Hierarchical structure analysis was performed on the normalized genus level abundance data using Ward’s linkage method to investigate the link between soil microbiota and plant type/soil pH and depth. Results indicate that the soil samples analyzed can be divided into six major clusters, after which the linkage distance separating the sub-clusters is small (Figure 2). Table 2 describes the members of each cluster and K-means clustering confirms the results. While overall the samples from individual locations from each of the horizon are clustered together or are in close clusters, samples from horizon B of location 10 (Pine tree vegetation) and horizon A of location 12 (Tulip tree) have unique microbiota to form its own cluster. ANOVA analysis indicates that the mean abundance for all the genera within a cluster are statistically different between clusters (p < 0.05), except for the abundance of seven genera (Supplementary Table S2). The seven genera whose abundance are not statistically different between clusters (p > 0.05) are Nitrosopumilus, Carboxydothermus, unclassified genera derived from Deltaproteobacteria, Pelotomaculum, Oceanicola, Thermotoga, and Bdellovibrio (Supplementary Table S2).
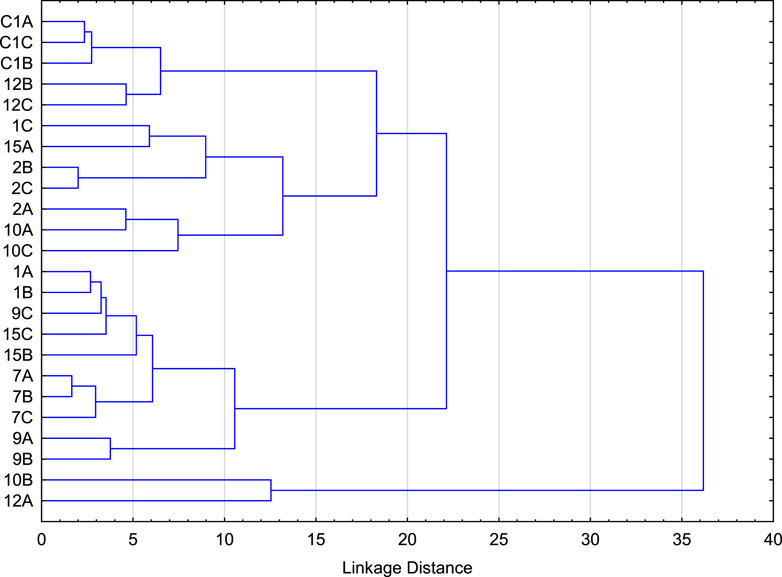
FIGURE 2. Hierarchical structure analysis used to identify the number of major clusters and the cluster members based on the normalized genus level abundance data.
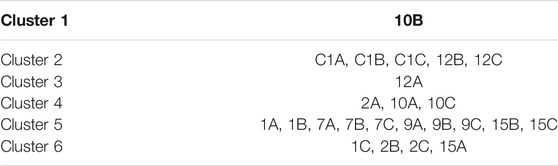
TABLE 2. Clustering of the soil samples based on the hierarchical structure analysis of taxonomic data at genera level. Soil samples were collected from eight sampling locations at three different horizons (A, 0–10 cm; B, 10–20 cm; C, 20–30 cm).
Table 3 shows the abundance of the top 30 microbial genera in the representative samples from each of the clusters and the average abundance of the organisms across all the 24 samples analyzed. Results show that Bradyrhizobium and Candidatus Solibacter, both Gram-negative bacteria, are the most abundant microorganism in the soil samples analyzed. Streptomyces and Mycobacterium are the two most abundant Gram-positive bacteria found in the soil samples.
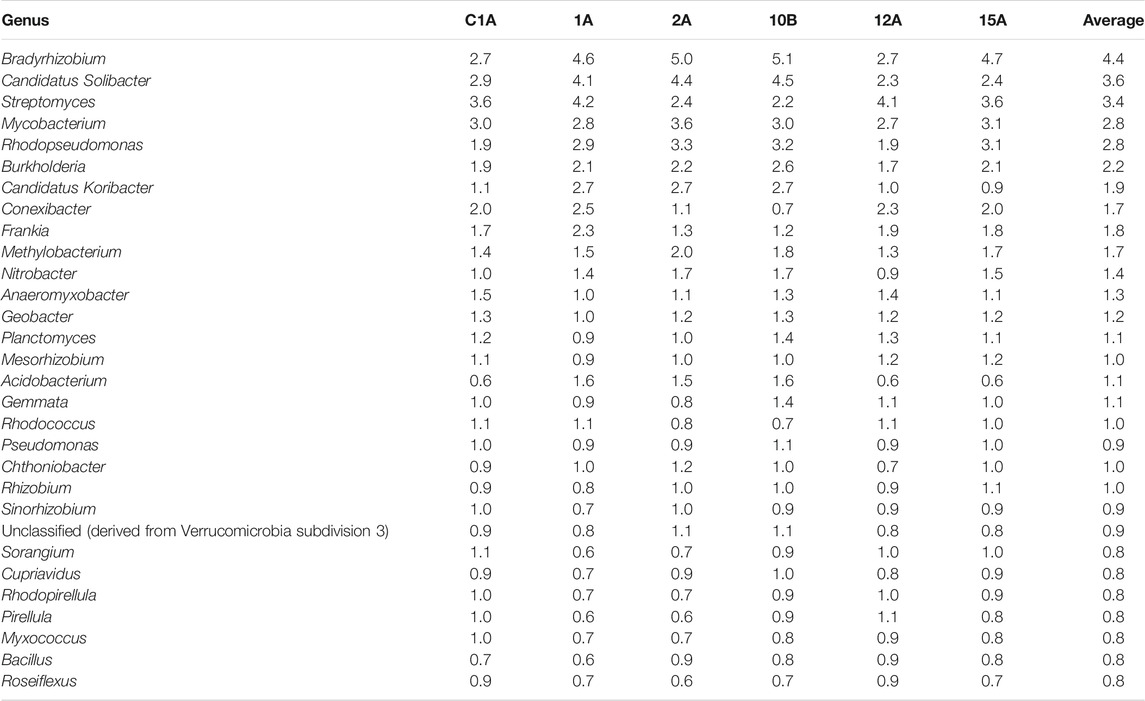
TABLE 3. Relative Abundance of the top 30 bacterial genera in the representative soil samples from each cluster and average values across all the soil samples analyzed.
Functional Characterization of Soil Microbiome
A heat map illustrating the functional annotation of sequence reads containing predicated proteins of known functions across all the 24 soil samples is shown in Figure 3. Variation was observed between samples primarily related to proteins involved in virulence, disease and defense; cell wall and capsule; membrane transport; DNA metabolism; and respiration. Among the functional categories identified by MG-RAST, the five most dominant categories based on the relative abundance of assigned reads were carbohydrates (13.3 ± 0.4%), the clustering-based subsystems (functional coupling evidence but unknown function; 12.9 ± 0.2%), amino acids and derivatives (9.6 ± 0.3%), miscellaneous (6.8 ± 0.2%), and protein metabolism (7.7 ± 0.3%).
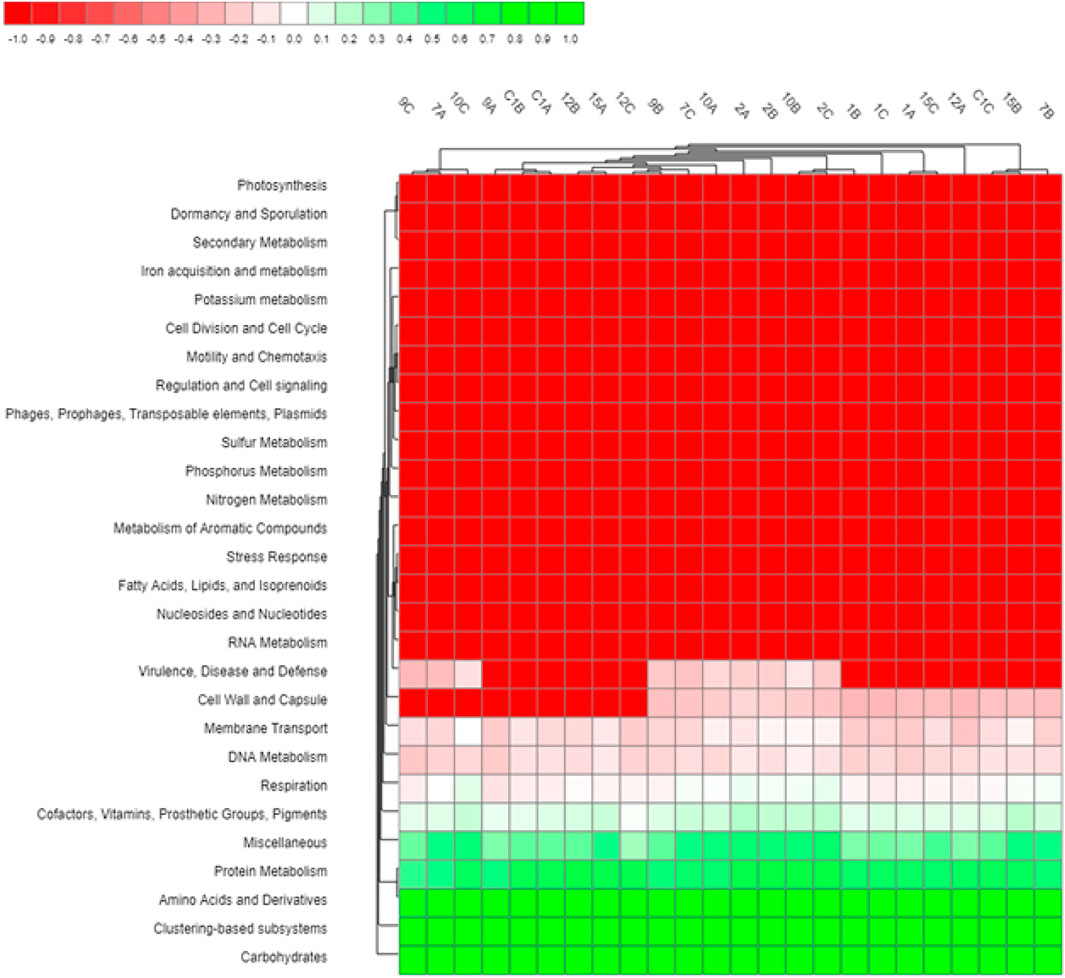
FIGURE 3. Heat map showing the differential abundance of functional categories (subsystem Level 1) between different soil samples.
Relative abundance of the predicated proteins annotated at subsystem level 2 for each of the soil samples is presented in the supplemental table (Supplementary Table S3). Hierarchical structure analysis was performed on the normalized values, similar to that performed for taxonomic data. Results indicate that the soil samples can be divided into 5 clusters, after which the linkage distance separating the sub-clusters is small (Figure 4). Table 4 describes the members of each cluster and K-means clustering confirms the results. Similar to taxonomic clustering, samples from horizon B of location 10 (Pine tree vegetation) and horizon A of location 12 (Tulip tree) have unique composition of functional proteins to form its own cluster.
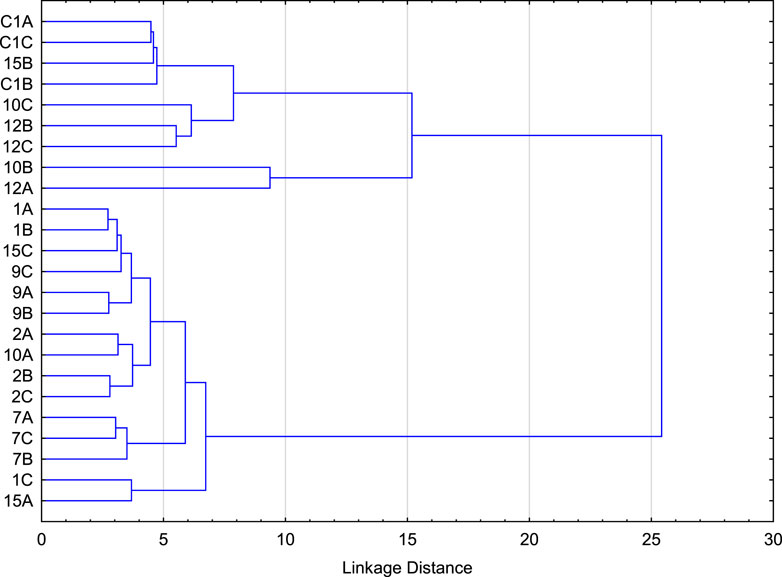
FIGURE 4. Hierarchical structure analysis used to identify the number of major clusters and the cluster members based on the normalized predicated proteins annotated at subsystem level 2.
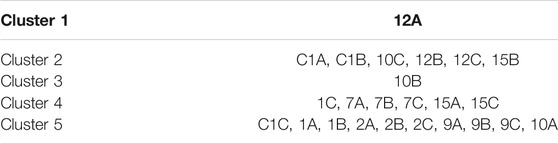
TABLE 4. Clustering of the soil samples based on the functional annotation of the sequence reads by MG-RAST at subsystem level 2. Soil samples were collected from eight sampling locations at three different horizons (A, 0–10 cm; B, 10–20 cm; C, 20–30 cm).
Table 5 shows the abundance of top 30 predicated proteins in the representative samples from each of the clusters. Results show that unidentified proteins involved in regulation and cell signaling comprise nearly 1 in 5 proteins predicated from the sequences. Nearly 6% of the predicated proteins are from the miscellaneous SEED category comprising a diverse set of genes identified during investigation of plant-prokaryote interactions by a project at the Department of Energy (DOE), USA (Thureborn et al., 2016). Protein biosynthesis, central carbohydrate metabolism, and resistance to antibiotics and toxic compounds were the other top predicated functions of the proteins.
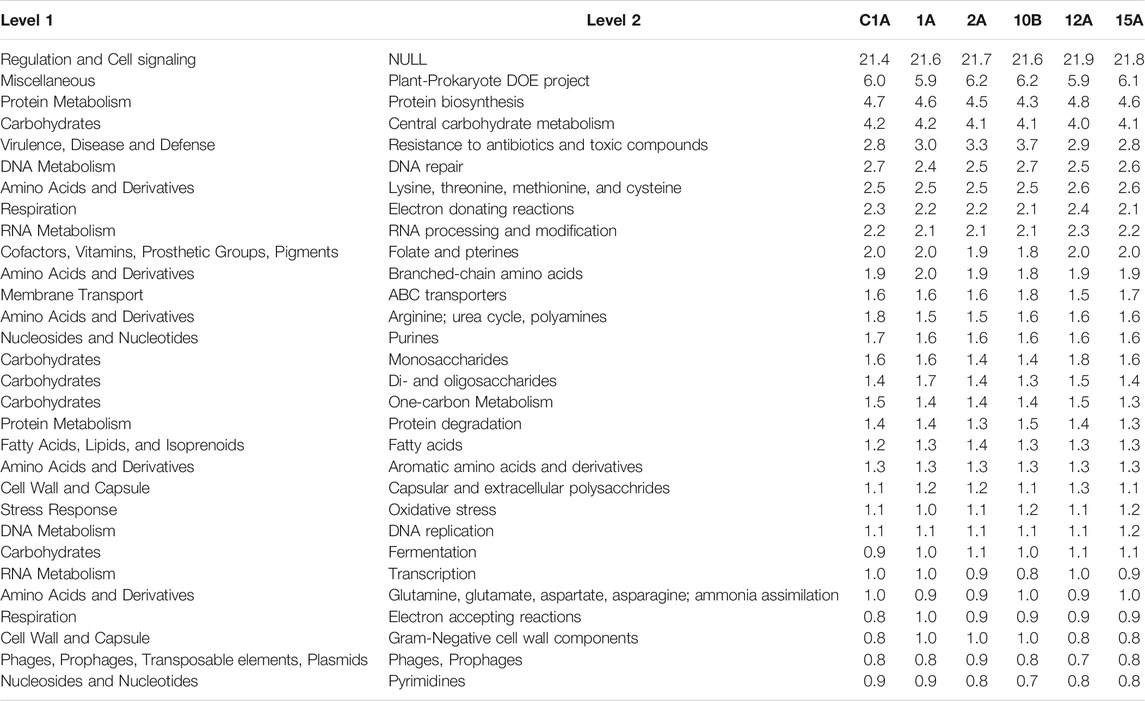
TABLE 5. Relative Abundance of the top 30 functional genes (level 2) bacterial genera in the representative soil samples from each cluster.
Linking Diversity to Function
To identify the key microorganisms playing significant role in the biochemistry of soil, Refseq and Subsystems analysis were performed together on MGRAST platform. The Subsystem analysis was performed at level 3 wherever possible. Top 5 genera having the largest quantity of annotated reads within each of the metabolic class were identified (Supplementary Table S4). Data indicates that Bradyrhizobium, Rhodopseudomonas, and Burkholderia are the key bacteria within the soil microbiota. Both, Bradyrhizobium and Rhodopseudomonas are top contributors in 24 of the 44 metabolic classes analyzed (Figure 5). Burkholderia is a top organism in 16 of the metabolic classes (Figure 5).
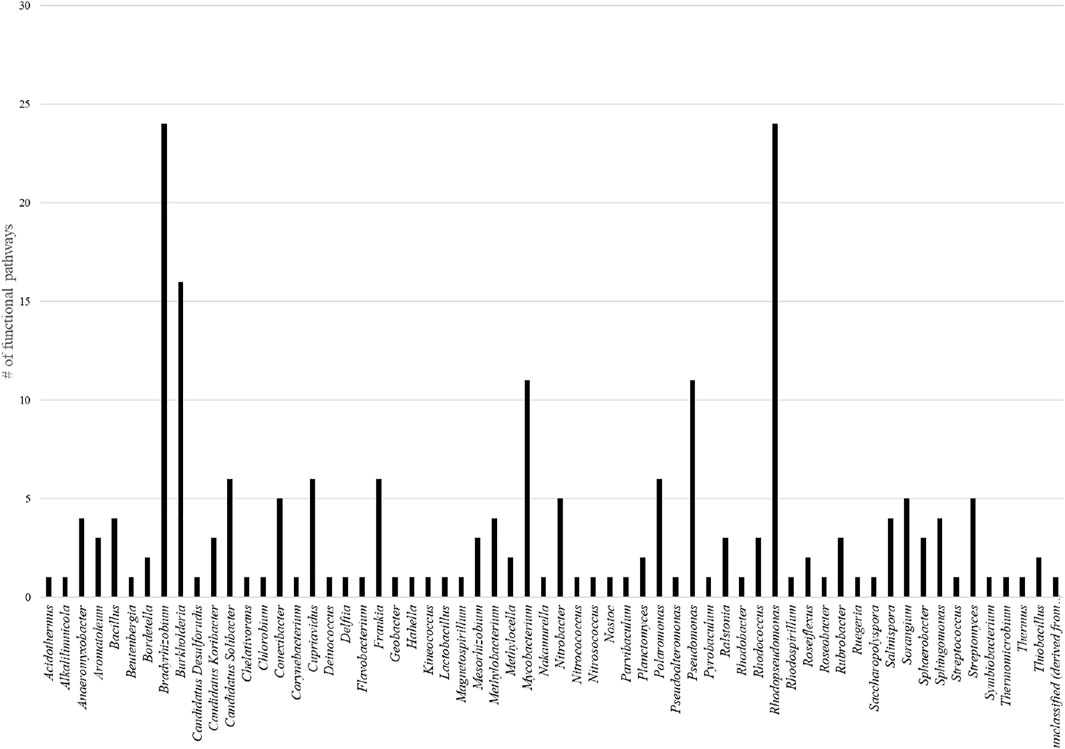
FIGURE 5. Total number of functional pathways top microbial genera are involved in within the acidic soil samples analyzed.
From an agricultural perspective, Nitrogen, Phosophorus, Sulphur and Iron metabolic pathways are significant. In the Nitrogen, Iron and Sulphur pathways, beyond the three genera identified, Mycobacteria also plays a significant role. Organisms from Anaeromyxobacter and Aromatoleum genera are key contributors in the nitrosative stress and dissimilatory nitrile reductase pathways respectively (Supplementary Table S4). Organisms from Sorangium genera have the most genes coding for Sulphate reduction associated complexes. Similarly, organisms from Cupriavidus and Pseudomonas genera are other top bacteria involved in Phosphate pathways (Supplementary Table S4). In Iron pathways, Bacillus, Frankia, and Pseudomonas were the top genera involved (Supplementary Table S4). The catabolic genes related to the degradation of xenobiotics were also annotated and linked to the microbial genera. Beyond Bradyrhizobium, Rhodopseudomonas, and Burkholderia, bacteria from Pseudomonas and Cupriavidus play a key role in degradation of xenobiotic compounds (Supplementary Table S4). Results indicate that for each of the biochemical functions, there is redundancy within the soil microbiome.
Discussion
We investigated the microbial structural and functional diversity within the top acidic soil associated with a wide variety of plants. Results indicate that irrespective of the level of acidity in the soil, most of the microorganisms type associated with the soil generally remains the same. The differences observed between soil samples, could be attributed to the abundance of individual organisms present in the soil. Soil chemistry and the vegetation present guides the abundance of individual organisms. The change in microbial abundance results in change in the abundance of functional genes within the soil microbiome. Literature is replete with scientific studies showing soil microbiome changes with the structure of the soil (eg. Fierer and Jackson, 2006; Fierer et al., 2012; Mendes and Tsai, 2018; Shah et al., 2021). Based on our results, we suggest that one needs to consider whether the type of organisms present in the soil are different or if the abundance of individual organisms is different before reaching the conclusions related to microbiome difference amongst different soil samples. Further, current methods of calculating alpha and beta diversity may not capture the true similarities in the microbiome from different soil types. As further advances are made in the next-generation sequencing techniques, we believe similarities in the microbiome across soil type could become more evident.
Taxonomically, prior research has shown that Gram-negative organisms are predominant organisms present in the soil (Shah and Subramaniam, 2018). Results obtained in the current study supports the prior observation. When one considers similar observations in microbiome studies conducted in marine environments, and even in human, fish and animals, a theme starts to emerge–in the microbial communities across the matrices, Gram-negative bacteria are the predominant organisms.
Functionally, high levels of genes attributed to regulation and cell signaling (level 1) appear to be an identifying indicator for acidic soils. cAMP is a major gene annotated to this category. Delmont et al. (2012) reported abundance of cAMP related annotation within the soil metagenome. Considering acidic soil is poor in nutrition and the northeast region of the United States has varying weather patterns, soil bacteria might be required to deal with constantly fluctuating substrates and environmental conditions. cAMP is a universal cell energy and metabolism regulator. Higher level of this and other genes involved in regulation and cell signaling can be attributed to the requirement of bacteria to adapt to the changing soil chemistry. Surprisingly, we noticed low levels of the abundance of genes related to nutrient cycling (sulfur metabolism, potassium metabolism, iron acquisition and metabolism, and phosphorous metabolism). Genes annotated to virulence disease and defense were significantly prevalent in the soil samples analyzed. The cluster-based subsystems contain diverse functions, such as resistance to antibiotics and toxic compounds, and pathogenicity islands.
Results of our study indicate that in the acidic soil, Bradyrhizobium, Rhodopseudomonas, and Burkholderia are the major organisms in the soil involved in the nutritional ecosystem functioning. Bradyrhizobium and Candidatus solibacter are taxonomically the most abundant organisms in the soil samples analyzed. Collectively, it is evident from taxonomic and functional analysis of the soil microbiome, bacteria from Bradyrhizobium are highly critical to maintaining soil fertility, irrespective of soil type. Analyzing the microbiota present in 52 soil samples from different countries, Shah and Subramaniam (2018) found that bacteria from Bradyrhizobium genera were the most abundant organisms in the microbiota. The structure-function linkage results indicate that the organism is not only responsible for nitrogen fixation and other pathways in N cycle, but also plays a key role in S and Fe cycles, and degradation pathways of xenobiotic compounds. Bradyrhizobium bacteria are present as symbiotic and non-symbiotic organisms in the soil, and literature is replete with the importance of the organism in the Nitrogen cycle (Ormeño-Orrillo and Martínez-Romero, 2019). Many strains of Bradyrhizobium are used commercially to improve crop production (Environmental Protection Agency, n.d.). We suggest that the beneficial impact of the organism in improving soil fertility could also be attributed to its role in other biochemical pathways.
Acidic soils provide a unique environment for soil microorganisms due to iron, manganese and aluminum toxicity, low nitrogen, phosphorus, and molybdenum levels, toxic levels of phenolic acids, and hydrogen ion toxicity (Kidd and Proctor, 2001; Shah et al., 2011). Often to overcome this issue, nitrogen fertilizers and other chemicals are used to improve soil fertility, but these methods can cause other environmental issues including increase in nitrous oxide emissions (Xu et al., 2014). As a substitute to the use of chemicals for improving soil fertility and crop production, we suggest to the scientific community to study the possibility of using consortia of organisms including Bradyrhizobium, Rhodopseudomonas, and Burkholderia. Considering the importance and ubiquity of these organisms in the soil, the consortia could be used by farmers across the globe, irrespective of soil chemistry and geographical location.
Next-generation sequencing methods are increasingly used to study how the soil microbiome responds to changes in environmental conditions or to addition of contaminants in the soil. We suggest that in addition to analyzing general community-based diversity changes, scientists should specifically look for changes in the Bradyrhizobium, Rhodopseudomonas, and Burkholderia population to understand the impact. Our results suggest that changes in abundance of these organisms may greatly impact the soil fertility.
Considering that the soil samples analyzed were from the West Chester, PA region only, further studies are warranted using acidic soil samples from across the globe to validate the observations. Nevertheless, the metagenomic data reported here furthers our knowledge on the acidic soil microbial communities at structural and functional level. There is a large degree of similarity in the soil microbiome associated with different vegetation and soil pH. Increasing our attention to similarities in soil microbiome may allow us to further the biotechnological potential of microbial based products to improve soil fertility in the future.
Data Availability Statement
The datasets presented in this study can be found in online repositories. The names of the repository/repositories and accession number(s) can be found below: https://www.ncbi.nlm.nih.gov/bioproject/719140.
Author Contributions
VS conceived the idea; MJ, SF, SS, VS were responsible for experimentation, data analysis and manuscript writing.
Funding
This research is based upon work supported by the National Science Foundation under Grant No. CBET-1748439 and West Chester University Sustainability Grant.
Conflict of Interest
The authors declare that the research was conducted in the absence of any commercial or financial relationships that could be construed as a potential conflict of interest.
Publisher’s Note
All claims expressed in this article are solely those of the authors and do not necessarily represent those of their affiliated organizations, or those of the publisher, the editors and the reviewers. Any product that may be evaluated in this article, or claim that may be made by its manufacturer, is not guaranteed or endorsed by the publisher.
Supplementary Material
The Supplementary Material for this article can be found online at: https://www.frontiersin.org/articles/10.3389/fenvs.2022.766302/full#supplementary-material
References
Akinola, S. A., Ayangbenro, A. S., and Babalola, O. O. (2021). The Diverse Functional Genes of maize Rhizosphere Microbiota Assessed Using Shotgun Metagenomics. J. Sci. Food Agric. 101 (8), 3193–3201. doi:10.1002/jsfa.10948
Babalola, O. O., Omotayo, O. P., and Igiehon, N. O. (2021). Survey of Maize Rhizosphere Microbiome Using Shotgun Metagenomics. Microbiol. Resour. Announc 10 (1), e01309–20. doi:10.1128/MRA.01309-20
Batcher, M. S. (2005). Monitoring Protocols for Central Pine Barrens Field Plots, V. 1.01. Prepared for Upton Ecological Research Reserve. Upton, New York, United States: Brookhaven National Laboratory.
Bell, C. W., Acosta-Martinez, V., McIntyre, N. E., Cox, S., Tissue, D. T., and Zak, J. C. (2009). Linking Microbial Community Structure and Function to Seasonal Differences in Soil Moisture and Temperature in a Chihuahuan Desert Grassland. Microb. Ecol. 58 (4), 827–842. doi:10.1007/s00248-009-9529-5
Collins, D., Luxton, T., Kumar, N., Shah, S., Walker, V. K., and Shah, V. (2012). Assessing the Impact of Copper and Zinc Oxide Nanoparticles on Soil: a Field Study. PLoS One 7 (8), e42663. doi:10.1371/journal.pone.0042663
Delforno, T. P., Lacerda Júnior, G. V., Noronha, M. F., Sakamoto, I. K., Varesche, M. B. A., and Oliveira, V. M. (2017). Microbial Diversity of a Full-Scale UASB Reactor Applied to Poultry Slaughterhouse Wastewater Treatment: Integration of 16S rRNA Gene Amplicon and Shotgun Metagenomic Sequencing. MicrobiologyOpen 6 (3), e00443. doi:10.1002/mbo3.443
Delmont, T. O., Prestat, E., Keegan, K. P., Faubladier, M., Robe, P., Clark, I. M., Pelletier, E., Hirsch, P. R., Meyer, F., Gilbert, J. A., Le Paslier, D., Simonet, P., and Vogel, T. M. (2012). Structure, Fluctuation and Magnitude of a Natural Grassland Soil Metagenome. Isme J. 6 (9), 1677–1687. doi:10.1038/ismej.2011.197
Edwards, A., Pachebat, J. A., Swain, M., Hegarty, M., Hodson, A. J., Irvine-Fynn, T. D. L., Rassner, S. M. E., and Sattler, B. (2013). A Metagenomic Snapshot of Taxonomic and Functional Diversity in an alpine Glacier Cryoconite Ecosystem. Environ. Res. Lett. 8 (3), 035003. doi:10.1088/1748-9326/8/3/035003
Enagbonma, B. J., Aremu, B. R., and Babalola, O. O. (2019). Profiling the Functional Diversity of Termite mound Soil Bacteria as Revealed by Shotgun Sequencing. Genes 10 (9), 637. doi:10.3390/genes10090637
Environmental Protection Agency (). TSCA Experimental Release Application Approved for Bradyrhizobium Japonicum Strains (Fact Sheet). Web Citation. Available at: https://www.epa.gov/regulation-biotechnology-under-tsca-and-fifra/tsca-experimental-release-application-approved (Accessed on June 10, 2021).
Fierer, N., and Jackson, R. B. (2006). The Diversity and Biogeography of Soil Bacterial Communities. Proc. Natl. Acad. Sci. 103 (3), 626–631. doi:10.1073/pnas.0507535103
Fierer, N., Leff, J. W., Adams, B. J., Nielsen, U. N., Bates, S. T., Lauber, C. L., Owens, S., Gilbert, J. A., Wall, D. H., and Caporaso, J. G. (2012). Cross-biome Metagenomic Analyses of Soil Microbial Communities and Their Functional Attributes. Proc. Natl. Acad. Sci. 109 (52), 21390–21395. doi:10.1073/pnas.1215210110
Garbeva, P., Van Veen, J. A., and Van Elsas, J. D. (2004). Microbial Diversity in Soil: Selection of Microbial Populations by Plant and Soil Type and Implications for Disease Suppressiveness. Annu. Rev. Phytopathol. 42, 243–270. doi:10.1146/annurev.phyto.42.012604.135455
Garrity, G. M., Thompson, L. M., Ussery, D. W., Paskin, N., Baker, D., Desmeth, P., Schindel, D. E., and Ong, P. S. (2009). Studies on Monitoring and Tracking Genetic Resources: an Executive Summary. Stand. Genomic Sci. 1 (1), 78–86. doi:10.4056/sigs.1491
Hasan, N. A., Young, B. A., Minard-Smith, A. T., Saeed, K., Li, H., Heizer, E. M., McMillan, N. J., Isom, R., Abdullah, A. S., Bornman, D. M., Faith, S. A., Choi, S. Y., Dickens, M. L., Cebula, T. A., and Colwell, R. R. (2014). Microbial Community Profiling of Human Saliva Using Shotgun Metagenomic Sequencing. PLoS One 9 (5), e97699. doi:10.1371/journal.pone.0097699
Ibarbalz, F. M., Orellana, E., Figuerola, E. L. M., and Erijman, L. (2016). Shotgun Metagenomic Profiles Have a High Capacity to Discriminate Samples of Activated Sludge According to Wastewater Type. Appl. Environ. Microbiol. 82 (17), 5186–5196. doi:10.1128/aem.00916-16
Kidd, P. S., and Proctor, J. (2001). Why Plants Grow Poorly on Very Acid Soils: Are Ecologists Missing the Obvious? J. Exp. Bot. 52 (357), 791–799. doi:10.1093/jexbot/52.357.791
Kumar, N., Shah, V., and Walker, V. K. (2011). Perturbation of an Arctic Soil Microbial Community by Metal Nanoparticles. J. Hazard. Mater. 190 (1-3), 816–822. doi:10.1016/j.jhazmat.2011.04.005
Mendes, L. W., and Tsai, S. M. (2018). Distinct Taxonomic and Functional Composition of Soil Microbiomes along the Gradient forest-restinga-mangrove in southeastern Brazil. Antonie Van Leeuwenhoek 111 (1), 101–114. doi:10.1007/s10482-017-0931-6
Nannipieri, P., Kandeler, E., and Ruggiero, P. (2002). “Enzyme Activities and Microbiological and Biochemical Processes in Soil,” in Enzymes in the Environment. Editors R. G. Burns, and R. P. Dick (New York: Marcel Dekker), 1–33.
Ormeño-Orrillo, E., and Martínez-Romero, E. (2019). A Genomotaxonomy View of the Bradyrhizobium Genus. Front. Microbiol. 10, 1334. doi:10.3389/fmicb.2019.01334
Quince, C., Lanzén, A., Curtis, T. P., Davenport, R. J., Hall, N., Head, I. M., ..., , Read, L. F., and Sloan, W. T. (2009). Accurate Determination of Microbial Diversity from 454 Pyrosequencing Data. Nat. Methods 6 (9), 639–641. doi:10.1038/nmeth.1361
Rutgers, M., Wouterse, M., Drost, S. M., Breure, A. M., Mulder, C., Stone, D., Creamer, R. E., Winding, A., and Bloem, J. (2016). Monitoring Soil Bacteria with Community-Level Physiological Profiles Using Biolog ECO-Plates in the Netherlands and Europe. Appl. Soil Ecol. 97, 23–35. doi:10.1016/j.apsoil.2015.06.007
Shah, A., Shah, S., and Shah, V. (2021). Impact of Flooding on the Soil Microbiota. Environ. Challenges 4, 100134. doi:10.1016/j.envc.2021.100134
Shah, V., Shah, S., Kambhampati, M. S., Ambrose, J., Smith, N., Dowd, S. E., et al. (2011). Bacterial and Archaea Community Present in the Pine Barrens Forest of Long Island, NY: Unusually High Percentage of Ammonia Oxidizing Bacteria. PLoS One 6 (10), e26263. doi:10.1371/journal.pone.0026263
Shah, V., and Subramaniam, S. (2018). Bradyrhizobium Japonicum USDA110: a Representative Model Organism for Studying the Impact of Pollutants on Soil Microbiota. Sci. total Environ. 624, 963–967. doi:10.1016/j.scitotenv.2017.12.185
Singh, N. K., Wood, J. M., Karouia, F., and Venkateswaran, K. (2018). Succession and Persistence of Microbial Communities and Antimicrobial Resistance Genes Associated with International Space Station Environmental Surfaces. Microbiome 6 (1), 204–223. doi:10.1186/s40168-018-0585-2
Sobek, E. A., and Zak, J. C. (2003). The Soil FungiLog Procedure: Method and Analytical Approaches toward Understanding Fungal Functional Diversity. Mycologia 95 (4), 590–602. doi:10.1080/15572536.2004.11833063
Thureborn, P., Franzetti, A., Lundin, D., and Sjöling, S. (2016). Reconstructing Ecosystem Functions of the Active Microbial Community of the Baltic Sea Oxygen Depleted Sediments. PeerJ 4, e1593
Venter, J. C., Remington, K., Heidelberg, J. F., Halpern, A. L., Rusch, D., Eisen, J. A., Wu, D., Paulsen, I., Nelson, K. E., Nelson, W., Fouts, D. E., Levy, S., Knap, A. H., Lomas, M. W., Nealson, K., White, O., Peterson, J., Hoffman, J., Parsons, R., Baden-Tillson, H., Pfannkoch, C., Rogers, Y.-H., and Smith, H. O. (2004). Environmental Genome Shotgun Sequencing of the Sargasso Sea. science 304 (5667), 66–74. doi:10.1126/science.1093857
Vogel, T. M., Simonet, P., Jansson, J. K., Hirsch, P. R., Tiedje, J. M., Van Elsas, J. D., Bailey, M. J., Nalin, R., and Philippot, L. (2009). TerraGenome: a Consortium for the Sequencing of a Soil Metagenome. Nat. Rev. Microbiol. 7 (4), 252. doi:10.1038/nrmicro2119
Keywords: acidic soil, microbial ecology, shotgun metagenome, microbiome, bradyrhizobium, rhodopseudomonas, burkholderia
Citation: James MT, Farrisi ST, Shah S and Shah V (2022) Identification of Major Organisms Involved in Nutritional Ecosystem in the Acidic Soil From Pennsylvania, USA. Front. Environ. Sci. 10:766302. doi: 10.3389/fenvs.2022.766302
Received: 28 August 2021; Accepted: 21 February 2022;
Published: 09 March 2022.
Edited by:
El-Sayed Salama, Lanzhou University, ChinaReviewed by:
Swapnil Pandey, University of Florida, United StatesMuhmmad Israr Khan, Kohat University of Science and Technology, Pakistan
Copyright © 2022 James, Farrisi, Shah and Shah. This is an open-access article distributed under the terms of the Creative Commons Attribution License (CC BY). The use, distribution or reproduction in other forums is permitted, provided the original author(s) and the copyright owner(s) are credited and that the original publication in this journal is cited, in accordance with accepted academic practice. No use, distribution or reproduction is permitted which does not comply with these terms.
*Correspondence: Vishal Shah, vshah@ccp.edu