- 1Department of Environmental Science & Management, Portland State University, Portland, OR, United States
- 2Samara Group LLC, Portland, OR, United States
- 3Department of Geography Portland State University, Portland, OR, United States
- 4Oregon Department of Fish & Wildlife, Salem, OR, United States
- 5Department of Math and Statistics, Portland State University, Portland, OR, United States
- 6Oregon Department of Forestry, Portland State University, Portland, OR, United States
Roads are not the only determining factor for wildlife movement across the landscape, but due to the extensive distribution of the road network their impact can be dramatic. Although it has been well documented that roads decrease habitat connectivity for wildlife due to animal-vehicle collisions, habitat fragmentation, and avoidance behavior, approaches for identifying connectivity across the landscape often do not fully examine the barrier effect of roads. Here, we explored the extent of the impact of roadways on wildlife connectivity by using Omniscape to model connectivity including and without the barrier effect of roads, then evaluating the difference between these two models. We created these connectivity models for three organisms that represent different taxa, movement types, and habitat requirements: northern red-legged frog, Pacific-slope flycatcher, and Columbian black-tailed deer. We found that roads had a strong impact on connectivity for all three species. Change in flow was most pronounced on the roads, especially where they ran through permeable habitat for a species. Roads also influenced connectivity well beyond the footprint of the roadway, affecting flows intersecting the roads and diffusely around them. The extent and nature of this impact depended on the species, road density, and surrounding habitat. The different effects across species highlight the importance of considering different taxa simultaneously while planning. Moreover, the ability to assess modeled wildlife habitat connectivity in the absence of existing widespread linear infrastructure allows for critical evaluation of where mitigation activities, such as wildlife crossing structures and fencing, may be most beneficial. Hence, this novel approach has practical application for increasing connectivity for wildlife across roads.
Introduction
The extensive network of roads affects animals in a variety of ways, including acting as a barrier to animal movement (Forman et al., 2003). Overall connectivity for wildlife is reduced by roadways as a result of habitat fragmentation, reduction in available habitat or its quality, injury and mortality as a result of animal-vehicle collisions, and behavioral avoidance, among other factors (Forman et al., 2003; Fahrig and Rytwinski, 2009; D’Amico et al., 2016; Teixeira et al., 2017). The impacted area extends beyond the road surface due to noise, air, water and other pollution from roads, and this road effect zone extends at least 100 m to either side of the road (Forman and Deblinger, 1998). The cumulative impacts of roads can greatly decrease the ability of organisms to move among habitat patches in order to successfully find food, water, shelter, and mates, impacting genetic diversity, population size and structure, metapopulation structure, and species diversity (Trombulak and Frissell, 2001; Forman et al., 2003; Fahrig and Rytwinski, 2009; Clark et al., 2010; Patrick and Gibbs, 2010). Most species are susceptible to at least some level of mortality or fragmentation from roads, including flighted species like birds, which are often overlooked in studies of road network impacts on wildlife (Kociolek et al., 2011; Loss et al., 2014). Although it has been well documented that roads decrease habitat connectivity for wildlife due to animal-vehicle collisions and behavioral avoidance, approaches for identifying locations most suitable for connectivity mitigation across the landscape often do not fully examine both the barrier effect of road networks and the permeability of the surrounding landscape.
The impacts of roads on wildlife vary based not only on the characteristics of the road (e.g. road width, speed limit, traffic volumes) but also on individual- and species-level characteristics (Forman and Alexander, 1998; van der Ree et al., 2015). Species-specific movement characteristics, life history traits, behavioral drivers, and habitat needs and diet, as well as the frequency with which individuals encounter roads as barriers on the landscape as they migrate, search for breeding locations, or move about their home ranges, may determine the extent to which the road network affects wildlife connectivity (Rytwinski and Fahrig, 2012; Beyer et al., 2013; Cook and Blumstein, 2013; Grilo et al., 2014). Animals that migrate seasonally or have large home ranges will face an increased chance of experiencing habitat fragmentation due to the road effect zone. Crepuscular animals may be most threatened by mortality from vehicle strikes due to the combination of reduced human sight lines at these low light movement times and the fact that traffic is often heaviest at dawn and dusk (Bliss-Ketchum et al., 2016). Further, different species perceive roadways and risk associated with roadways differently, which can affect the extent to which roads may serve as a barrier on the landscape (Jacobson et al., 2016). Hence, efforts to understand how to reduce the impact of roads will likely be most effective if they use species-specific approaches and also consider multiple species across taxonomic and behavioral groups.
Some of the impacts of roadways can be mitigated, but determining and implementing effective mitigation techniques is a difficult task. Public and government willingness to spend limited funds on mitigation measures is greatest when the risks to human safety are most apparent, typically in cases where large bodied animals like deer are frequently involved in collisions. When animal-vehicle collisions do not threaten loss of human life or damage to vehicles, such as when collisions occur with smaller-bodied animals, the ability to enact mitigation can be incredibly difficult. In addition, data on road-associated mortality and connectivity impacts are generally lacking for most animals, especially small species, thwarting the realization of urgency to address the problem. However, even in cases where road mortality hotspots are well documented, the siting of crossing structures aimed both at reducing the collision rate and increasing habitat connectivity for these (and other) species is not straightforward, as the locations along the roadway where mortality is highest might not coincide with the best location to support habitat connectivity (Teixeira et al., 2017). Roadkill hotspots may not indicate where crossing structures should be located to best facilitate animal movement because animals may move a long distance away from their preferred path to an area with lower traffic in order to find a place they can attempt to cross (Jackson et al., 2011). Even telemetry studies may not highlight an individual’s preferred path unless locations are collected at very fine timescales. As a result, there is a need for modeling approaches to aid in identifying the best sites for mitigating road effects.
Here, we use a novel habitat modeling approach, along with application of circuit theory-based connectivity modeling using the Omniscape algorithm, to quantify the impact of roads on habitat connectivity and to examine where natural movements of wildlife would be likely to occur if roads were absent from the landscape. We examine this change in connectivity for three different species, including an amphibian, a bird, and a large-bodied, generalist mammal. In addition to evaluating connectivity both with and in the absence of roads, we also quantify the impact of roadways on wildlife by examining road density and distance from roads in the study area.
The examination of habitat permeability and connectivity for wildlife, both including and in the absence of roadways, can support better choices in implementation of road mitigation techniques. For example, modeling the locations that wildlife are most likely to move in the absence of the influences of the current road network can support management by identifying ideal locations for the placement of crossing structures, habitat enhancement, or deterrence in order to improve habitat connectivity and decrease the risk of wildlife-vehicle collisions. This approach can also be used to identify where new development can be situated in order to avoid blocking key natural pathways.
Methods
Study Area
Our study area encompasses a HUC 8 watershed in southwestern Oregon, USA, spanning from the coast inward across the Coast Range and Klamath Mountains to the west slope of the Cascade Mountains, between Coos Bay, OR and Crater Lake National Park (Figure 1). The coordinates of the center of the study area are 123°23′35″W 43°15′54″N. Major roadways in this region include US 101 and Interstate 5, as well as OR 138, 42, and 227, among others. The dominant habitat types are westside lowland conifer-hardwood forest and montane mixed conifer forest. The area includes two small cities, Coos Bay (population 16,300) and Roseburg (population 23,080).
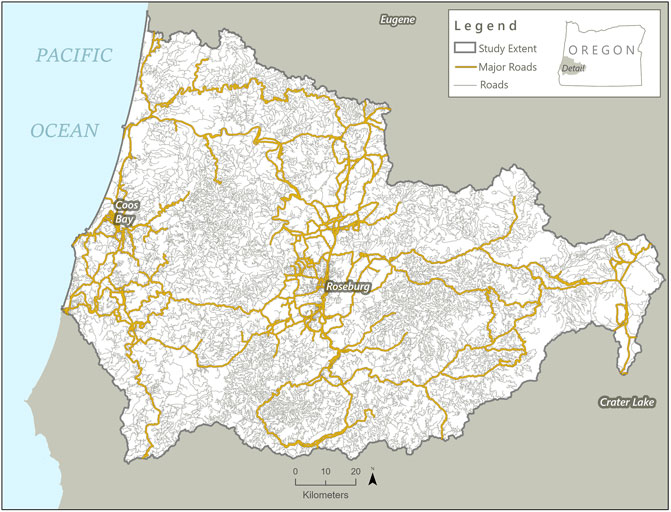
FIGURE 1. Map of study area highlighting all of the roads present in the region. Roads highlighted in orange are the primary roads (Interstate 5, large arterial roadways, and connecting regional roads) that were used in analysis; other roads are indicated in brown.
Study Species
Northern red-legged frog (Rana aurora) is a pond-breeding amphibian with a fully terrestrial adult life stage. It is listed as a species of greatest conservation need in Oregon but is also found from California to Canada (Oregon Department of Fish and Wildlife Oregon Conservation Strategy, 2016). It migrates distances up to 5 km from breeding sites, selecting small (∼0.8–1.5 ha) habitat patches containing moist understory (Chan-McLeod and Moy, 2007; Hayes et al., 2007; Hayes et al., 2008). This species and its close relatives may be indicators for habitat fragmentation, climate resiliency, ecological health, and disease vectors. There are still questions on how land use, habitat availability and aquatic-terrestrial fragmentation affect amphibian mobility and chances for survival, especially in developed or urbanizing areas (Hayes et al., 2008). The availability and connectivity of upland terrestrial habitat has been identified as a major conservation gap for this species across managed environments in the Pacific Northwest (Grand et al., 2017). Roadways in close proximity to water bodies and wetlands used for breeding can both cause habitat loss and become a source of direct mortality by vehicle collision for breeding adults and newly emerged juveniles (Hayes et al., 2008). Due to their relatively long-distance movements, red-legged frogs are at greater risk of road effects than other amphibians in the region (Carr and Fahrig 2001).
Pacific-slope flycatcher (Empidonax difficilis) is an avian old-growth associate that frequents dense Douglas fir-western hemlock and mixed coniferous-deciduous forests, often within riparian growth along the edge of openings and nearby to water (Marshall et al., 2006). It is a sensitive species that avoids noise, traffic, and other human disturbances on road crossings, particularly where the species utilizes bridge crossings over riparian areas (Campbell et al., 1997). It migrates and breeds across Mexico, USA, and Canada, traveling up to 6,700 km along the coast (Lowther et al., 2020). Within California and Canada the minimum patch size ranges from 0.89 to 4.25 ha (Ainsley, 1992; Lowther et al., 2020).
Columbian black-tailed deer (Odocoileus hemionus columbianus) is a wide-ranging ungulate generalist and, in Oregon, is typically found from the coast to the Cascade Mountains (Oregon Department of Fish and Wildlife, 2021). They are edge-adapted species using dense forest cover to hide during the day and more open, early successional forest to feed at dawn and dusk. During 2009, approximately 260,000 animal-vehicle collisions were reported in the United States, causing 12,000 human injuries and 173 human fatalities (National Highway Traffic Safety Administration, 2011). The animals involved in police-reported collisions are predominantly deer (Odocoileus spp.) (Centers for Disease Control, 2004; Williams and Wells, 2005). Road characteristics such as number of lanes and traffic volume influence the level of road avoidance by deer (Alexander and Waters, 2000; Ager et al., 2003; Dodd et al., 2007). The movement distances travelled by deer can be fairly small and localized, based on seasonal availability of food in the Coast Range Mountains, but their habitat patch size can range from 2 to 260 ha (Leckenby et al., 1982).
Modeling Approach
Currently, the four most common approaches to modeling habitat connectivity draw on cost distance (LaRue and Nielsen, 2008; Richard and Armstrong, 2010), resistant kernel (Compton et al., 2007; Wasserman et al., 2012), current flow (Koen et al., 2014; Pelletier et al., 2014), and network flow (Carroll et al., 2012) approaches. Most applications of cost distance and resistant kernel methods, while frequently utilized, suffer from several shortcomings. Both resistant kernel and cost distance approaches assume that organisms have perfect knowledge of the landscape and move with the intent to minimize travel costs, assumptions which are likely not representative of natural systems for most species. For example, dispersing individuals would not have had any exposure to the landscape outside their natal area, and some migrating ungulates move to maximize energy intake rather than to minimize travel costs (Bischof et al., 2012).
Many of the existing approaches to connectivity analysis, including cost distance, resistant kernel, and network flow models, assess connectivity between discrete patches, in the form of terminals or core area polygons. Patch selection, however, can strongly influence connectivity modeling results (Baldwin et al., 2010; Perkl et al., 2016). Decisions about how patches are defined (e.g., minimum size requirements for core areas), as well as placement, directly impact the spatial outputs of the connectivity assessment. Without a complete understanding of the effects of patch selection within a study area, any given set of patches may lead to erroneous conclusions about connectivity that translate into negative consequences for subsequent management. One application of current flow (Circuitscape) also relies on patch selection, and is susceptible to similar issues.
Our analyses utilize current flow in a wall-to-wall framework using the Omniscape algorithm (McRae et al., 2016; Landau et al., 2021). Omniscape is a newer application of circuit theory that does not rely on definition of core habitat patches; instead, connectivity is assessed continuously across the landscape. The current flow approach of Omniscape simultaneously evaluates the contribution of multiple movement pathways, rather than identifying a single least-cost corridor. Representation of connectivity as a gradient more accurately represents natural systems—movement patterns found in nature are rarely restricted to single, discrete corridors, and many sub-optimal paths likely still serve as functional connections for wildlife.
Omniscape is parameterized using a resistance surface, developed here as a raster layer with a 30 m pixel size with relative resistance values assigned to different landscape features. In order to define resistance layers for each species, our first step was to model habitat permeability for each species. Using available habitat models, published literature, and expert opinion of a particular species’ needs (e.g., the animal requires wetland habitat, will not travel further than 500 m from a water source, etc.), we developed models identifying how permeable different landscape features are expected to be for each of our target species. These species-specific models take into account not only what is optimal habitat for the species, but also habitat that is suboptimal but does not significantly impair the ability of the species to move through that landscape, as well as landscape features that act as barriers to species movement. For each of our three target species, each landscape feature was assigned a score, ranging from −3 to 3, with higher values attributed to higher quality habitat, and negative values attributed to barriers to movement. The individual component scores were then summed within each pixel to create the Habitat Permeability Model. Relatively high scores are indicative of areas of the landscape that are expected to facilitate species movement, whereas relatively low scores are indicative of areas of the landscape that are expected to impede species movement. These Habitat Permeability Models differ from species distribution or habitat suitability models in that they are designed to illustrate how easy or difficult it will be for a given species to move across the landscape. These models are less restrictive than traditional species distribution or habitat suitability models, recognizing that wildlife will often move through otherwise unsuitable habitat.
To evaluate the impacts of roadways on species movement, we created two permeability models for each species, one including a road layer, and one without roads considered. Data layers and parameters are provided in Supplementary Table S1. We designed our road layer using data from Open Street Maps (www.openstreetmap.org). Open Street Maps is an open-source database that combines information from many different regional and local agencies and contains location and attribute information for road types ranging from interstates to trails. For this project, we edited the dataset to include only interstates (I-5), large arterial roadways, and connecting regional roads. Each of these road types has relatively high traffic volume and/or speed limits in excess of 64 kph (40 mph), which represent roads we expect to pose the greatest threat to animal movement across the landscape (Visintin et al., 2018). We did not include residential roads, rural roads, or logging roads; although these road types are numerous on this landscape and certainly fragment habitat, they are less likely to significantly affect the movements of our target species. We buffered the centerline of each road by 90 m to account for both the road itself and the road effect zone (Forman and Deblinger, 1998).
After draft Habitat Permeability Models were completed for each species, we initiated outreach to local experts for review. A minimum of four experts per species were invited to review the model outputs and accompanying parameter tables. Species experts were able to add comments directly to the model parameter table, as well as comment and draw polygons on areas of the map where they felt the model was performing well, or poorly, in regards to the individual species presence and movement capabilities. Proposed changes from reviewers were compiled and evaluated by the project team and adjustments to model parameters were tracked. Changes were then applied to the models and these revised Habitat Permeability Models were passed to Omniscape for the connectivity analysis. While species experts provided valuable feedback that we feel improved our parameterization of component layers and overall quality of our Habitat Permeability Models, we acknowledge that until confronted against empirical data these models serve primarily as hypotheses about where we expect movement for each species will be facilitated or impeded. Given that the effort described here was a conceptual exercise to explore the potential of this method, we elected not to validate for this publication. However, we strongly encourage that draft models undergo a formal validation process using species occurrence data to test model performance prior to real-world application.
We used the inverse of these Habitat Permeability Models as the resistance surface for Omniscape, rescaled to 1–100 (100 being the most resistant and 1 being the least). Omniscape also requires a moving window size. We tested moving window sizes that were directly related to species home range, but found that these only highlighted small, local patterns of connectivity. We ultimately used moving windows that were 870 pixels, approximately 1/8th the study area. Using Omniscape this way allowed us to model movement across the landscape in a way that created regional visualizations of connectivity for these species without being spatially or temporally bounded by home range or daily range.
To compare the effects of roads on species movement, we examined the cumulative current flow output produced by Omniscape for the models including and in the absence of major roadways. We also applied a change detection function between the models with and in the absence of roads for each species, to evaluate the change in current flow when roads are removed from the landscape. In this case, a change detection is the subtraction of the “roads” model from the “no roads” model, using raster calculator (ESRI ArcGIS Pro 2.8.1), to identify where there was a positive difference, which indicates a higher connectivity value due to the absence of the road; a negative difference is indicative of lower connectivity due to the absence of the road. This is analogous to a common change detection method used in landscape-scale analyses of vegetation change using NDVI values.
Road Density
Using ESRI ArcGIS Pro (2.8.1) we intersected a 10 km2 grid “fishnet” with the all roads layer (primary roads, secondary roads, and pedestrian/non-motor vehicle trails), as well as the primary roads layer (those expected to have a substantial effect on connectivity: Interstate 5, large arterial roadways, and connecting regional roads; Figure 1). We summarized the shape length of roads per 10 km2 grid and combined these values to obtain the total and average length of roads in the study area. We also calculated the distance from all roads and primary roads using Euclidean geometry. This produces a continuous scale of distance (km) from a road, which we classified into categories for distant from, near, and on road locations. Using this categorization, we identified the farthest areas in the study from roads. We created the road density outputs using focal statistics and a moving window size of 1 km2 (radius of 0.56 km). The road density scale for all roads and for primary roads were symbolized using 3-class quantiles for simplicity to designate low density, medium density, and high density.
Results
Road Density
The study area had an intermediate density of roads as judged by distance from roads and road length per area. The greatest Euclidean distance away from primary roads was 18.8 km, with a mean of 3.4 km away (Figure 2A). The summed road length for primary roads, those expected to have the largest effect on connectivity, was 3,174.4 km. The average road density of primary roads was 4.8 km of linear road per 10 km2, and the highest road density was 24.8 km of linear roadway per 10 km2. The I-5 highway corridor and coastal road network had the highest densities among primary roadways (Figure 2B).
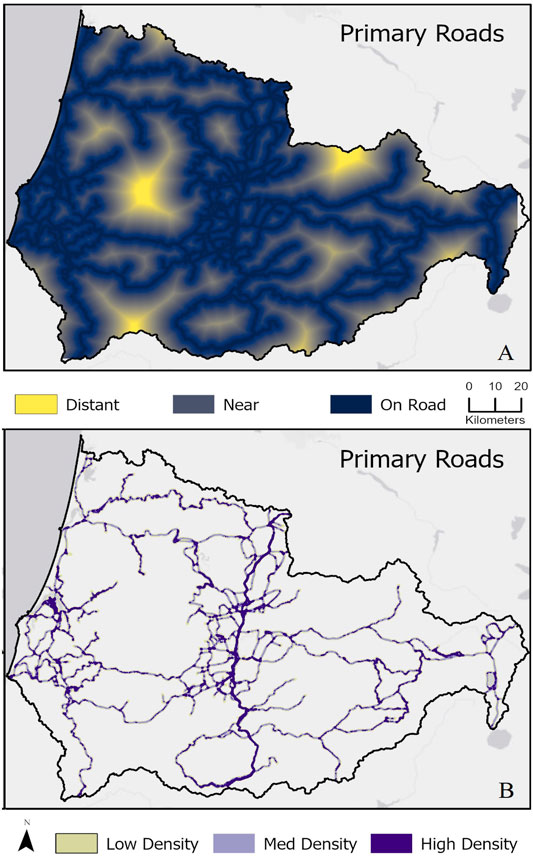
FIGURE 2. Road density maps illustrating (A) the greatest Euclidian distance from the road for primary roads and (B) primary road densities per 1 km2 area categorized as low, medium, and high density. For (A) yellow shows areas that are furthest from roads whereas dark blue is adjacent to roads; outputs were calculated using Euclidean distance geometry, symbolized using 3-class categories for simplicity (distant from roads, near/adjacent to roads, on the road). For (B) outputs used focal statistics to calculate the relative density, symbolized using 3-class quantiles for simplicity (low, medium, and high-density roads). Raw values ranged from 1 to 221.
When considering all roads in the study area, densities were, of course, higher. The greatest distance away from any road type, including primary roads, secondary roads, and pedestrian/non-motor vehicle trails, was 3.8 km, with a mean of 0.3 km (Supplementary Figure S1A). Small pockets of contiguous non-road land cover can be found in the eastern portion of the study area along the west slope of the Cascades and in the western portion along the coast. The summed road length for all roads was 30,857.3 km. This averaged 16.9 km of linear road per 10 km2, and the highest road density of all roads in the area was 117 km of linear roadway per 10 km2. When all road types were considered, high densities of roadways were widespread throughout the study area and areas far from primary roads still encompassed high densities of other roads (Supplementary Figure S1B). In addition, the highest densities of all road types often occurred in and surrounding developed areas (Supplementary Figure S1B).
Effect of Roads on Modeled Connectivity
In our study area, roadways had a substantial impact on connectivity for all three target species (Figure 3). In some cases, primary roads directly overlap the target species’ most permeable habitat (Figure 3). This overlap between roads and areas expected to best facilitate wildlife movement greatly reduces the possibility of high-quality connectivity in some portions of the study area. Modeled connectivity along roadways increased considerably for all three species when roads were removed from the models (Figure 3). However, the effect of roads on permeability differed among the species as well as across different road densities and habitats.
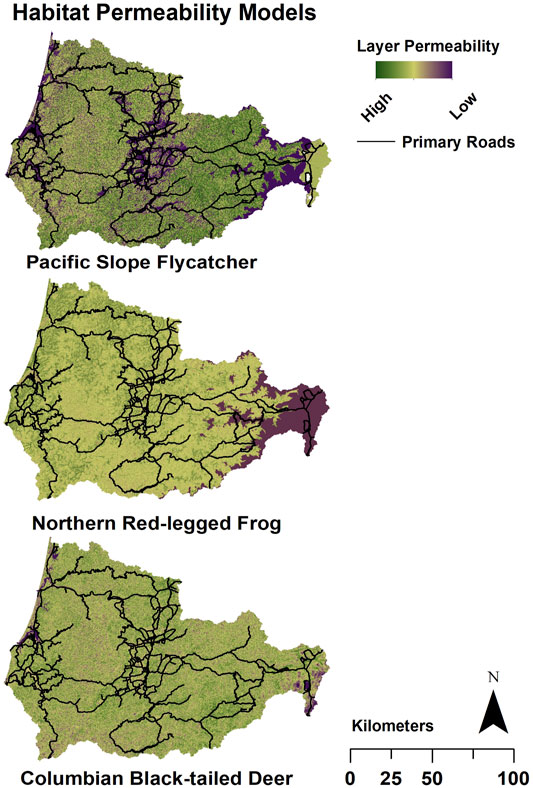
FIGURE 3. Example Habitat Permeability Models for Pacific slope flycatcher, northern red-legged frog, and Columbian black-tailed deer. The primary roads (black lines) are superimposed on the model for reference. Plum indicates the lowest permeability of each 30 m grid cell; yellow indicates moderate permeability, and green indicates high permeability. The inverse of the permeability models was used as the resistance layers in Omniscape.
Modeled connectivity for Pacific-slope flycatcher was highest in the eastern portion of the study region, due to the presence of higher quality habitat for the species (Figures 3, 4A). In this area, modeled removal of roads revealed strong increases in connectivity, not only along the roadways but also between them (Figures 5A,B). In contrast, connectivity was lower in the middle and western portions of the study area, so removal of roads provided only moderate improvements for flycatcher connectivity in these regions (Figure 5A). At a finer scale, the change detection function illustrates that connectivity increased in areas both overlapping with and perpendicular to roadways when roads were removed (Figure 5B). While increased connectivity is expected along the former roadway, more surprising is the improvement in connectivity in areas surrounding and between roadways, suggesting that roadways are bisecting multiple potential movement pathways, even for this flighted species (Figures 4A, 5A,B).
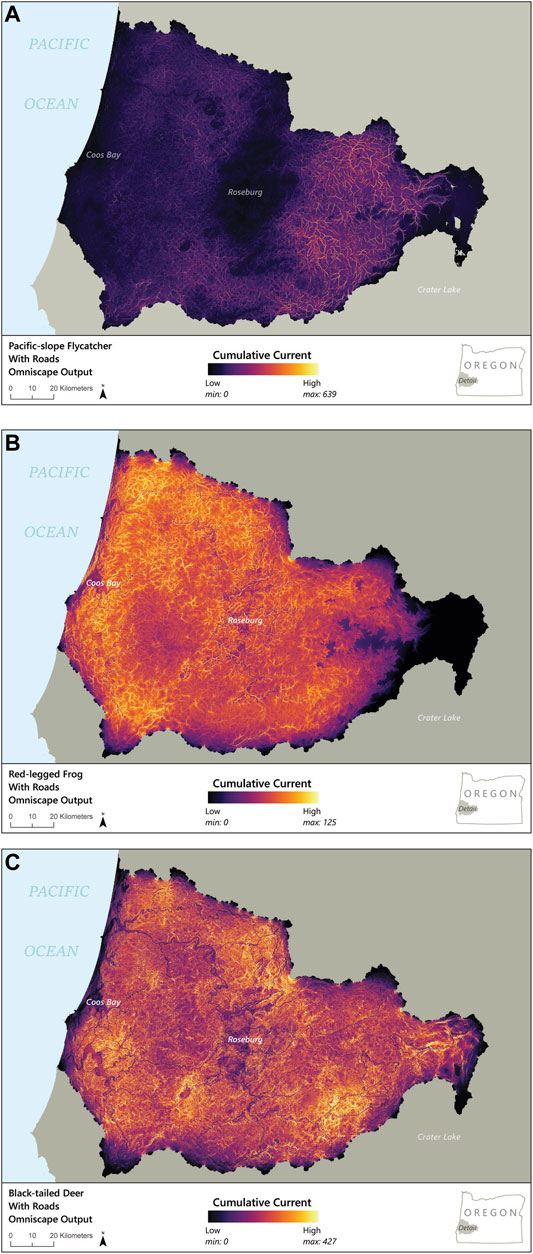
FIGURE 4. Maps of Omniscape-modeled connectivity across the entire study area, with roads included for (A) Pacific-slope flycatcher, (B) red-legged frog, and (C) Columbian black-tailed deer. Black and purple represent low current flow and connectivity, whereas yellow represents high modeled current flow and therefore connectivity.
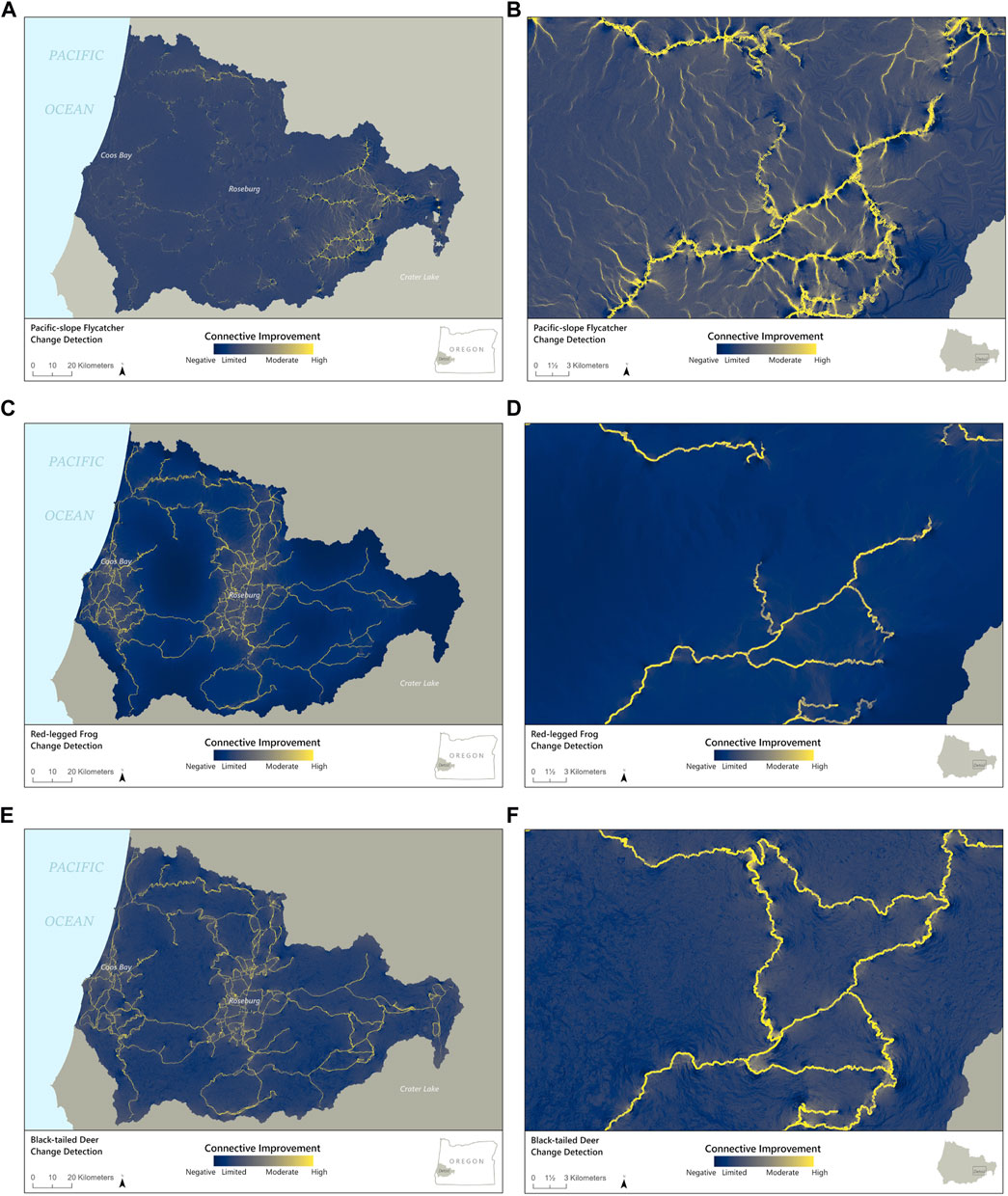
FIGURE 5. Maps showing the difference in detected connectivity between Omniscape models with roads subtracted from models without roads for (A) Pacific-slope flycatcher throughout the study area and (B) zoomed in on a portion of the eastern side of the study area; (C) red-legged frog for the whole study area and (D) zoomed in on the eastern portion, and (E) Columbian black-tailed deer for the whole study area and (F) zoomed in on the eastern portion. The dark blue areas represent low improvement from modeled removal of roads whereas, at the other end of the color scheme, yellow represents high current improvement for the “No Road” scenario compared to the “Road ” scenario.
Omniscape output for northern red-legged frog illustrates moderate to high current flow at a broad scale throughout most of the study area, but reduced current flow from larger roads and urban areas (Figure 4B). The change detection function illustrating changes in current flow as a result of modeled removal of roadways also highlights improvements in connectivity both along and between road corridors, particularly in the areas that have the highest densities of primary roads (Figure 5C). At a finer scale, examining an area with a moderate density of roads, we see only minimal increase in connectivity beyond roadways, suggesting that roadways have an uneven impact on connectivity for northern red-legged frog, possibly based on the density and configuration of the road network (Figure 5D).
Similar to northern red-legged frog, Omniscape output for Columbian black-tailed deer showed moderate to high current flow throughout much of the study area, largely due to the generalist nature of the species and the extent of permeable habitat for deer in the region. However, the model did suggest lower current flow in areas within and surrounding larger roadways and urban areas (Figures 4C, 5E). The change detection function comparing connectivity with and in the absence of roadways illustrates both the effect of the roadways themselves on decreased habitat connectivity for deer as well as a cumulative impact from the overall road network (Figure 5E). In the developed areas, modeled removal of primary roads illustrated moderate increases in diffuse connectivity for deer, suggesting the overall road network has an impact on movement in addition to the effect of individual roads. At a finer scale, the change detection function highlights that the effect of roads on connectivity for deer clearly extended beyond the road corridor, creating patches with reductions in diffuse connectivity for over 1 km beyond the roadways in many cases (Figure 5F). In areas with higher road densities, modeled removal of roads illustrated a moderate improvement in connectivity for 2–5 km beyond any specific road; this improvement extended only ∼0.5 km beyond the road corridor where large roads exist in isolation (Figure 5E).
Discussion
All species need habitat connectivity, yet constructed linear features such as roads can greatly decrease habitat permeability for some species (Forman et al., 2003; D’Amico et al., 2016). Indeed, ubiquitous in most countries and habitats, roads can decrease survival and movement of a variety of animals, causing population-level effects (Rytwinski and Fahrig, 2015). At least 110.9 million km of roads divide the habitat of most countries into smaller fragments, and the United States accounts for 6.6 million km of these roads, including 76,334 km of expressways (Central Intelligence Agency, 2021). Because of this extensive and important network, road density in the USA is at least 1.2 km/km2 for public roads (Forman, 2000). Our study area in southwestern Oregon averaged a low to moderate road density of just under 1.7 km/km2. However, road density impacts animal behavior, occurrence, diversity, and mortality (e.g., Trombulak and Frissell, 2001; Beyer et al., 2013; Leblond et al., 2013).
Even at these relatively moderate densities, the road network created potential for decreased wildlife connectivity in our study area. The effect of roads, as measured by modeled connectivity in the absence of roadways, varied among low and high road-density areas. For all three of our species, the amount of area with improved connectivity from removing the barrier effect of roads increased with the density of roadways (Figure 3). This finding is in line with previous research using individually based movement models that illustrated that elk (Cervus canadensis) similarly experienced increased mortality risk and decreased emigration rates in areas where road effects saturated the landscape because of road densities above 1.25 km/km2 (Frair et al., 2008). Road density also has been found to affect black bear (Ursus americanus) connectivity and number of road crossings (Zeller et al., 2021), turtle dispersal (Patrick and Gibbs, 2010), and recolonization of habitats by wolves (Mladenoff et al., 1999).
The extent to which modeled wildlife connectivity was improved as a result of the modeled removal of roads varied substantially among the three species we examined, as well as among different regions within the study area. While this approach does not allow for direct comparisons of the change in connectivity across species, we can see that for all three species the benefits of road “removal” only accrued if the habitat already facilitated movement of the species. Unsurprisingly, improvement in connectivity was greatest for all three species along the removed roadways (Figure 5). More interesting are the nuanced ways connectivity changed between these road corridors and based on road density.
In some locations, removal of roadways had a substantial impact on species connectivity beyond the roadway itself. For example, for Pacific-slope flycatcher, modeled removal of roads in higher quality habitat opened up connectivity flows across and between roads in areas of moderate road density. In contrast, connectivity across roads for northern red-legged frog increased only in the areas of highest road density (Figure 5C). This suggests that high densities of primary roads might have a greater impact on connectivity for the northern red-legged frog, whereas even moderate densities of roads appear to limit connectivity for Pacific slope-flycatcher. Birds can benefit from roads (Morelli et al., 2014) or face minimal road effects, but the literature documenting negative road impacts to birds is growing (Kociolek et al., 2011; Loss et al., 2014). For example, barn owls (Tuto alba) can face high mortality from collisions with cars (Grilo et al., 2014).
For Columbian black-tailed deer, changes to connectivity beyond roadways were more diffuse than for either northern red-legged frog or Pacific-slope flycatcher. Since deer are highly mobile habitat generalists, movement of this species is not restricted to particular habitat types, so removal of roads permits a diffuse increase in movement across former roadways. This improvement in diffuse connectivity, particularly within developed areas, suggests that for deer, the overall network of roads has an effect on movement in addition to the effect of individual roads, and this effect can extend for several kilometers beyond the road corridor.
Whereas our study highlights the negative effects of roadways on connectivity for three species, representing birds, mammals, and amphibians, for some species, roads do not necessarily create strong barriers to movement. In fact, for a variety of mammals, connectivity, including connectivity across natural barriers, has been documented to be enhanced by roads, at least in some ways (Hill et al., 2021). Even in cases where roadways improve connectivity, however, roads might serve as an ecological trap as well, leading to wildlife-vehicle collisions and mortality (Hill et al., 2021).
Road impacts vary across species for several reasons. First, animal startle responses and traffic volume interact to determine whether a species will avoid roads, risk mortality, or be less affected (Jacobson et al., 2016). Avoidance can be mitigated using strategic vegetation management or specific design features to limit noise and light disturbance, though these ideas need further testing. Second, roads may be most permeable for nocturnal animals as fewer vehicles are on the road at night than during the day and the road surface typically cools through the night. However, headlights may cause animals to freeze and driver line of sight is diminished at night, both adding to the risk faced by animals that are on the road.
These varying responses highlight the need for modeling or measuring habitat connectivity for multiple species rather than just one or a few. Some efforts use a generalist or wide-ranging species, like deer, to represent connectivity broadly for all wildlife. Movement of generalist species, however, may not adequately capture movement and habitat needs for more specialist species (Krosby et al., 2015; Meurant et al., 2018; Costanza et al., 2020). Based on differences within taxonomic groups identified in the literature (e.g., Loss et al., 2014; Fensome and Mathews, 2016; Jacobson et al., 2016; Hill et al., 2021) as well as differences among taxonomic groups, as illustrated here and elsewhere (e.g., Tessier et al., 2020), it is clearly important to consider connectivity for multiple different types of taxa when considering both road impacts and siting for mitigation. Similarly, as was found with Pacific-slope flycatcher here, consideration should extend to taxa not traditionally thought of as facing decreased connectivity due to roads, such as for birds and other flying organisms, including bats and butterflies (e.g., Fensome and Mathews, 2016; Zielin et al., 2016).
Mitigation techniques can aid in decreasing the negative impacts of roadways on wildlife connectivity. Wildlife crossing structures are generally, though not always, effective for animal passage (Denneboom et al., 2021). Omniscape outputs suggest pathways where modeled connectivity across roads can be maximized by adding a crossing structure meant to facilitate movement of multiple wildlife species. Given that building wildlife crossing structures is an expensive endeavor, we should be locating and ultimately constructing structures so they will be effective for as many species as possible (Denneboom et al., 2021). The approach developed here can be used experimentally to model improvement in connectivity when adding crossing structures in different places on the landscape. This technique can be applied in conjunction with, or in place of, the use of roadkill hotspots, which are generally limited to larger-bodied species such as deer and elk and do not necessarily align with best places for improving connectivity (Eberhardt et al., 2013; Teixeira et al., 2017; Lee et al., 2021), or standard GIS modeling approaches, which may not consider what connectivity would be like in the absence of the road barrier.
While modeled connectivity was greatly increased directly along roadways, the amount of improvement from removing roads was not uniform throughout the study area, due to variation in the permeability of the surrounding landscape for each species. The areas where removing roads created the greatest overall improvement were the areas where crossing structures would be expected to have the greatest overall benefit for connectivity. Conversely, some areas showed decreased current flow when roads were removed. These decreases suggest that the species would switch to crossing at alternative locations along the roadway if barriers were reduced as a result of mitigation. Hence, those areas likely would not be the best targets for efforts to improve wildlife passage.
The method developed here could be applied to evaluate potential locations for siting wildlife crossing structures. For example, crossing structure locations could be experimentally added and removed from the model, either individually or all together, and the resulting connectivity value outputs compared to both connectivity given current road placement (i.e., with no crossing structures in place) as well as the ideal connectivity scenario (i.e., in the absence of all roads). Note that even when ideal spots are identified, the best place for wildlife to cross may not align with sites that are practical to mitigate. Topography and surrounding habitat, existing infrastructure, land ownership, etc. all may affect the viability of mitigation at a given site (Grilo et al., 2008; Denneboom et al., 2021). The ability to evaluate multiple potential pathways, as with the methods presented here, allows for consideration of mitigation both in the context of maximizing improvements in wildlife connectivity and in ensuring feasibility of implementation. If animal avoidance of roads is a contributing factor towards fragmentation, crossing structures alone may not fully mitigate the problem, and other complementary approaches, such as reduction and mitigation of noise and artificial light, as well as vegetation management, may also be needed (Kociolek et al., 2011).
The work presented here, modeling the removal of roads as a novel approach for analyzing the impact of roadways on wildlife movement, demonstrates how the road network disrupts regional wildlife habitat connectivity. The multiple, diffuse pathways that emerge by removing roads illustrate the extent to which these pervasive linear features impact the permeability of habitat for wildlife. Moreover, this technique helps identify sites that would most improve connectivity if the road barrier were removed in places, such as through the installation of a wildlife crossing structure. While it is infeasible in practice to remove roadways, the methods presented here illustrate the impact of roads on connectivity and allow practitioners to envision where mitigation techniques could be implemented in order to best facilitate regional connectivity.
Data Availability Statement
The original contributions presented in the study are included in the article/Supplementary Material. Further inquiries can be directed to the corresponding author.
Author Contributions
CdR conceived of the idea, drafted the manuscript, and participated in decision-making throughout. LB-K contributed to the development of manuscript ideas, approaches, and writing. ML constructed the habitat permeability and roads layers, contributed to the development of the concept and all methods. AT processed model data layers, and contributed analyses, maps, writing and interpretation. LM-W ran the Omniscape models and created associated maps. RW participated throughout all phases of development, served as a primary editor for the manuscript, and is a PI for development of many of the methods. AS conducted literature reviews of study species and helped write and edit. AH, AR, JS, and DR all aided in idea and methodological development and editing.
Funding
This project was funded by grant no. 161-19 from Oregon Department of Fish and Wildlife, which includes funding from US Fish and Wildlife Service Wildlife and Sport Fish Restoration (grant no. F19AF00226) and Adaptive Science (grant no. F19AC00009) programs.
Conflict of Interest
Authors LB-K, AS, and AT were employed by the company Samara Group LLC.
The remaining authors declare that the research was conducted in the absence of any commercial or financial relationships that could be construed as a potential conflict of interest.
Publisher’s Note
All claims expressed in this article are solely those of the authors and do not necessarily represent those of their affiliated organizations, or those of the publisher, the editors and the reviewers. Any product that may be evaluated in this article, or claim that may be made by its manufacturer, is not guaranteed or endorsed by the publisher.
Acknowledgments
The processes used in this study benefited from discussion with Lori Hennings (Metro), Tom Miewald (NRCS), Alan Yanahan (USFWS), and all supporters of the OCAMP project including the Bureau of Land Management, Burns Paiute Tribe, Defenders of Wildlife, Oregon Department of Fish and Wildlife, Oregon Department of Transportation, Oregon Wildlife Foundation, The Nature Conservancy, and USFWS. We thank the many reviewers of the species Habitat Permeability Models, especially David Helzer and Josh Chapman, who critiqued the Pacific-slope flycatcher model; Laura Guderyahn, Jade Keehn, and Josh Chapman, who critiqued the northern red-legged frog model; and Don Whittaker, DeWaine Jackson, and Steve Niemela, who critiqued the Columbian black-tailed deer model.
Supplementary Material
The Supplementary Material for this article can be found online at: https://www.frontiersin.org/articles/10.3389/fenvs.2022.757954/full#supplementary-material
References
Ager, A. A., Johnson, B. K., Kern, J. W., and Kie, J. G. (2003). Daily and Seasonal Movements and Habitat Use by Female Rocky Mountain elk and Mule Deer. J. Mammal. 84, 1076–1088. doi:10.1644/BBa-020
Ainsley, D. T. J. (1992). Vocalizations and Nesting Behavior of the Pacific-slope Flycatcher, Empidonax difficilus. Master’s thesis. Victoria (BC): University of Victoria.
Alexander, S. M., and Waters, N. M. (2000). The Effects of Highway Transportation Corridors on Wildlife: a Case Study of Banff National Park. Transp. Res. C 8, 307–320. doi:10.1016/S0968-090X(00)00014-0
Baldwin, R. F., Perkl, R. M., Trombulak, S. C., and Burwell, W. B. (2010). “Modeling Ecoregional Connectivity,” in Landscape-Scale Conservation Planning. Editors S. C. Tombulak, and R. F. Baldwin (Netherlands: Springer Netherlands), 349–367. doi:10.1007/978-90-481-9575-6_16
Beyer, H. L., Ung, R., Murray, D. L., and Fortin, M.-J. (2013). Functional Responses, Seasonal Variation and Thresholds in Behavioural Responses of Moose to Road Density. J. Appl. Ecol. 50 (2), 286–294. doi:10.1111/1365-2664.12042
Bischof, R., Loe, L. E., Meisingset, E. L., Zimmermann, B., Van Moorter, B., and Mysterud, A. (2012). A Migratory Northern Ungulate in the Pursuit of Spring: Jumping or Surfing the Green Wave? The Am. Nat. 180, 407–424. doi:10.1086/667590
Bliss-Ketchum, L. L., de Rivera, C. E., Turner, B. C., and Weisbaum, D. M. (2016). The Effect of Artificial Light on Wildlife Use of a Passage Structure. Biol. Conserv. 199, 25–28. doi:10.1016/j.biocon.2016.04.025
Campbell, R. W., Dawe, N., McTaggart-Cowan, I., Cooper, J., Kaiser, G., McNall, M., et al. (1997). The Birds of British Columbia. Vancouver: UBC Press.
Carr, L. W., and Fahrig, L. (2001). Effect of Road Traffic on Two Amphibian Species of Differing Vagility. Conservation Biol. 15 (4), 1071–1078. doi:10.1046/j.1523-1739.2001.0150041071.x
Carroll, C., McRae, B. H., and Brookes, A. (2012). Use of Linkage Mapping and Centrality Analysis across Habitat Gradients to Conserve Connectivity of gray Wolf Populations in Western North America. Conserv. Biol. 26, 78–87. doi:10.1111/j.1523-1739.2011.01753.x
Centers for Disease Control (2004). Nonfatal Motor-Vehicle Animal Crash-Related Injuries - United States, 2001-2002. Morbidity Mortality Weekly Rep. 53 (30), 675–678. doi:10.1016/j.jsr.2004.10.002
Central Intelligence Agency (2021). The World Factbook. Washington, DC: Central Intelligence Agency. Available at: https://www.cia.gov/the-world-factbook/countries/united-states/#transportation (Accessed July15, 2021).
Chan-McLeod, A. C., and Moy, A. (2007). Evaluating Residual Tree Patches as Stepping Stones and Short-Term Refugia for Red-Legged Frogs. J. Wildl. Manage. 71 (6), 1836–1844. doi:10.2193/2006-309
Clark, R. W., Brown, W. S., Stechert, R., and Zamudio, K. R. (2010). Roads, Interrupted Dispersal, and Genetic Diversity in Timber Rattlesnakes. Conserv. Biol. 24 (4), 1059–1069. doi:10.1111/j.1523-1739.2009.01439.x
Compton, B. W., McGarigal, K., Cushman, S. A., and Gamble, L. R. (2007). A Resistant-Kernel Model of Connectivity for Amphibians that Breed in Vernal Pools. Conserv. Biol. 21, 788–799. doi:10.1111/J.1523-1739.2007.00674.X
Cook, T. C., and Blumstein, D. T. (2013). The Omnivore’s Dilemma: Diet Explains Variation in Vulnerability to Vehicle Collision Mortality. Biol. Conserv. 167, 310–315. doi:10.1016/j.biocon.2013.08.016
Costanza, J. K., Watling, J., Sutherland, R., Belyea, C., Dilkina, B., Cayton, H., et al. (2020). Preserving Connectivity under Climate and Land-Use Change: No One-Size-Fits-All Approach for Focal Species in Similar Habitats. Biol. Conserv. 248, 108678. doi:10.1016/j.biocon.2020.108678
D'Amico, M., Périquet, S., Román, J., and Revilla, E. (2016). Road Avoidance Responses Determine the Impact of Heterogeneous Road Networks at a Regional Scale. J. Appl. Ecol. 53, 181–190. doi:10.1111/1365-2664.12572
Denneboom, D., Bar-Massada, A., and Shwartz, A. (2021). Factors Affecting Usage of Crossing Structures by Wildlife–A Systematic Review and Meta-Analysis. Sci. Total Environ. 777, 146061. doi:10.1016/j.scitotenv.2021.146061
Dodd, N. L., Gagnon, J. W., Boe, S., and Schweinsburg, R. E. (2007). Assessment of elk Highway Permeability by Using Global Position System Telemetry. J. Wildl. Manage. 71 (4), 1107–1117. doi:10.2193/2006-106
Eberhardt, E., Mitchell, S., and Fahrig, L. (2013). Road Kill Hotspots Do Not Effectively Indicate Mitigation Locations when Past Road Kill Has Depressed Populations. J. Wildl. Manage. 77 (7), 1353–1359. doi:10.1002/jwmg.592
Fahrig, L., and Rytwinski, T. (2009). Effects of Roads on Animal Abundance: an Empirical Review and Synthesis. Ecol. Soc. 14 (1), 21. doi:10.5751/ES-02815-140121
Fensome, A. G., and Mathews, F. (2016). Roads and Bats: a Meta-Analysis and Review of the Evidence on Vehicle Collisions and Barrier Effects. Mammal Rev. 46 (4), 311–323. doi:10.1111/mam.12072
Forman, R. T. T., and Alexander, L. E. (1998). Roads and Their Major Ecological Effects. Annu. Rev. Ecol. Syst. 29, 207–231. doi:10.1146/annurev.ecolsys.29.1.207
Forman, R. T. T., and Deblinger, R. D. (1998). “The Ecological Road-Effect Zone for Transportation Planning, and a Massachusetts Highway Example,” in Proceedings of the International Conference on Wildlife Ecology and Transportation 1998, Fort Myers Florida, USA, Febrauary 9, 1998 to Febrauary 12, 1998. Editors G. L. Evink, P. Garrett, D. Zeigler, and J. Berry (Tallahassee: Florida Department of Transportation). Available at: https://trid.trb.org/view/639938.
Forman, R. T., Sperlin, D., Bissonette, J. A., and Clevenger, A. P. (2003). Road Ecology: Science and Solutions. Washington, DC: Island Press.
Forman, R. T. (2000). Estimate of the Area Affected Ecologically by the Road System in the United States. Conserv. Biol. 14 (1), 31–35. doi:10.1046/j.1523-1739.2000.99299.x
Frair, J. L., Merrill, E. H., Beyer, H. L., and Morales, J. M. (2008). Thresholds in Landscape Connectivity and Mortality Risks in Response to Growing Road Networks. J. Appl. Ecol. 45, 1504–1513. doi:10.1111/j.1365-2664.2008.01526.x
Grand, L., Hayes, M., Vogt, K., Vogt, D., Yarnold, P., Richter, K., et al. (2017). Identification of Habitat Controls on Northern Red-Legged Frog Populations: Implications for Habitat Conservation on an Urbanizing Landscape in the Pacific Northwest. Ecol. Process. 6, 44. doi:10.1186/s13717-017-0111-7
Grilo, C., Bissonette, J. A., and Santos-Reis, M. (2008). Response of Carnivores to Existing Highway Culverts and Underpasses: Implications for Road Planning and Mitigation. Biodiv. Conserv. 17 (7), 1685–1699. doi:10.1007/s10531-008-9374-8
Grilo, C., Reto, D., Filipe, J., Ascensão, F., and Revilla, E. (2014). Understanding the Mechanisms behind Road Effects: Linking Occurrence with Road Mortality in Owls. Anim. Conserv. 17 (6), 555–564. doi:10.1111/acv.12120
Hayes, M. P. T., Rombough, C. J., and Hayes, C. B. (2007). Natural History: Rana aurora (Northern Red-Legged Frog), Movement. Herpetolog. Rev. 38, 192–193. doi:10.1161/strokeaha.107.489005
Hayes, M. P., Quinn, T., Richter, K. O., Schuett-Hames, J. P., and Serra Shean, J. T. (2008). “Maintaining Lentic-Breeding Amphibians in Urbanizing Landscapes: The Case Study of the Northern Red-Legged Frog (Rana aurora),” in Urban Herpetology. Editors J. C. Mitchell, R. E. Jung Brown, and B. Bartholomew (Society for the Study of Amphibians and Reptiles).
Hill, J. E., DeVault, T. L., and Belant, J. L. (2021). A Review of Ecological Factors Promoting Road Use by Mammals. Mammal Rev. 51 (2), 214–222. doi:10.1111/mam.12222
Jackson, D., Gregory, S. C., Bjornstrom, G. A., and Larkins, A. N. (2011). Highway Mortality of Mule Deer in Central Oregon. Wildlife Technical Report. Salem, Oregon: Oregon Department of Fish and Wildlife, 22.
Jacobson, S. L., Bliss-Ketchum, L. L., de Rivera, C. E., and Smith, W. P. (2016). A Behavior-Based Framework for Assessing Barrier Effects to Wildlife from Vehicle Traffic Volume. Ecosphere 7 (4), e01345. doi:10.1002/ecs2.1345
Kociolek, A. V., Clevenger, A. P., Clair, C. C. S., and Proppe, D. S. (2011). Effects of Road Networks on Bird Populations. Conserv Biol. 25, 241–249. doi:10.1111/j.1523-1739.2010.01635.x
Koen, E. L., Bowman, J., Sadowski, C., and Walpole, A. A. (2014). Landscape Connectivity for Wildlife: Development and Validation of Multispecies Linkage Maps. Methods Ecol. Evol. 5, 626–633. doi:10.1111/2041-210X.12197
Krosby, M., Breckheimer, I., Pierce, D. J., Singleton, P. H., Hall, S. A., Halupka, K. C., et al. (2015). Focal Species and Landscape “Naturalness” Corridor Models Offer Complementary Approaches for Connectivity Conservation Planning. Landscape Ecol. 30, 2121–2132. doi:10.1007/s10980-015-0235-z
Landau, V. A., Shah, V. B., Anantharaman, R., and Hall, K. R. (2021). Omniscape.jl: Software to Compute Omnidirectional Landscape Connectivity. J. Open Source Softw. 6 (57), 2829. doi:10.21105/joss.02829
LaRue, M. A., and Nielsen, C. K. (2008). Modelling Potential Dispersal Corridors for Cougars in Midwestern North America Using Least-Cost Path Methods. Ecol. Model. 212 (3), 372–381. doi:10.1016/j.ecolmodel.2007.10.036
Leblond, M., Dussault, C., and Ouellet, J. P. (2013). Impacts of Human Disturbance on Large Prey Species: Do Behavioral Reactions Translate to Fitness Consequences? PLoS One 8 (9), e73695. doi:10.1371/journal.pone.0073695
Leckenby, D. A., Sheehy, D. P., Nellis, C. H., Scherzinger, R. J., Luman, I. D., Elmore, W., et al. (1982). “Wildlife Habitats in Managed Rangelands - the Great Basin of Southeastern Oregon,” in Pacific Northwest Forest and Range Experiment Station (USDA Forest Service).
Lee, T. S., Creech, T. G., Martinson, A., Nielsen, S. E., Jakes, A. F., Jones, P. F., et al. (2021). Prioritizing Human Safety and Multispecies Connectivity across a Regional Road Network. Conserv. Sci. Pract. 3, e327. doi:10.1111/csp2.327
Loss, S. R., Will, T., and Marra, P. P. (2014). Estimation of Bird‐vehicle Collision Mortality on US Roads. J. Wildl. Manage. 78 (5), 763–771. doi:10.1002/jwmg.721
Lowther, P. E., Pyle, P., and Patten, M. A. (2020). “Cordilleran Flycatcher (Empidonax Occidentalis), Version 1.0,” in Birds of the World. Editor P. G. Rodewald (Ithaca, NY, USA: Cornell Lab of Ornithology). doi:10.2173/bow.corfly.01
Marshall, M. G., Marshall, D. B., and Contreras, A. L. (2006). Birds of Oregon: A General Reference. Oregon State University Press.
McRae, B. H., Popper, K., Jones, A., Schindel, M., Buttrick, S., Hall, K., et al. (2016). Conserving Nature’s Stage: Mapping Omnidirectional Connectivity for Resilient Terrestrial Landscapes in the Pacific Northwest. Portland, OR: The Nature Conservancy.
Meurant, M., Gonzalez, A., Doxa, A., and Albert, C. H. (2018). Selecting Surrogate Species for Connectivity Conservation. Biol. Conserv. 227, 326–334. doi:10.1016/j.biocon.2018.09.028
Mladenoff, D. J., Sickley, T. A., and Wydeven, A. P. (1999). Predicting gray Wolf Landscape Recolonization: Logistic Regression Models vs. New Field Data. Ecol. Appl. 9 (1), 37–44. doi:10.1890/1051-0761(1999)009[0037:pgwlrl]2.0.co;2
Morelli, F., Beim, M., Jerzak, L., Jones, D., and Tryjanowski, P. (2014). Can Roads, Railways and Related Structures Have Positive Effects on Birds?–A Review. Transp. Res. D: Transport Environ. 30, 21–31. doi:10.1016/j.trd.2014.05.006
National Highway Traffic Safety Administration (2011). Traffic Safety Facts 2009: A Compilation of Motor Vehicle Crash Data from the Fatality Analysis Reporting System and the General Estimates System. Washington, DC: U.S. Department of Transportation.
Oregon Department of Fish and Wildlife Oregon Conservation Strategy (2016). Northern Red-Legged Frog. Available at http://oregonconservationstrategy.org/strategy-species/northern-red-legged-frog/ (Last Accessed November 20, 2019).
Oregon Department of Fish and Wildlife (2021). Black-Tailed Deer. Available at: https://myodfw.com/big-game-hunting/species/black-tailed-deer (Accessed July 31, 2021).
Patrick, D. A., and Gibbs, J. P. (2010). Population Structure and Movements of Freshwater Turtles across a Road-Density Gradient. Landscape Ecol. 25, 791–801. doi:10.1007/s10980-010-9459-0
Pelletier, D., Clark, M., Anderson, M. G., Rayfield, B., Wulder, M. A., and Cardille, J. A. (2014). Applying Circuit Theory for Corridor Expansion and Management at Regional Scales: Tiling, Pinch Points, and Omnidirectional Connectivity. PLOS ONE 9, e84135. doi:10.1371/journal.pone.0084135
Perkl, R. M., Baldwin, R. F., Trombulak, S. C., and Smith, G. R. (2016). Landscape Network Congruency at the Ecoregion Scale: Can We Expect Corridor ‘Umbrellas. J. Land Use Sci. 11, 429–449. doi:10.1080/1747423x.2016.1142015
Richard, Y., and Armstrong, D. P. (2010). Cost Distance Modelling of Landscape Connectivity and gap-crossing Ability Using Radio-Tracking Data. J. Appl. Ecol. 47, 603–610. doi:10.1111/j.1365-2664.2010.01806.x
Rytwinski, T., and Fahrig, L. (2012). Do species Life History Traits Explain Population Responses to Roads? A Meta-Analysis. Biol. Conserv. 147, 87–98. doi:10.1016/j.biocon.2011.11.023
Rytwinski, T., and Fahrig, L. (2015). “The Impacts of Roads and Traffic on Terrestrial Animal Populations,” in Handbook of Road Ecology. Editors R. van der Ree, D. J. Smith, and C. Grilo. 1st Edn. (Chichester, UK: John Wiley & Sons), 237–246. doi:10.1002/9781118568170.ch28
Teixeira, F. Z., Kindel, A., Hartz, S. M., Mitchell, S., and Fahrig, L. (2017). When Road-Kill Hotspots Do Not Indicate the Best Sites for Road-Kill Mitigation. J. Appl. Ecol. 54, 1544–1551. doi:10.1111/1365-2664.12870
Tessier, D. L., Maranger, R., and Poisot, T. (2020). Omnidirectional and Omnifunctional Connectivity Analyses with a Diverse Species Pool. bioRxiv. doi:10.1101/2020.02.03.932095
Trombulak, S. C., and Frissell, C. A. (2001). Review of Ecological Effects of Roads on Terrestrial and Aquatic Communities. Conserv. Biol. 14 (1), 18–30. doi:10.1046/j.1523-1739.2000.99084.x
van der Ree, R., Smith, D. J., and Grilo, C. (2015). “The Ecological Effects of Linear Infrastructure and Traffic: Challenges and Opportunities of Rapid Global Growth,” in Handbook of Road Ecology, 1–9. doi:10.1002/9781118568170.ch1
Visintin, C., Golding, N., Van Der Ree, R., and McCarthy, M. A. (2018). Managing the Timing and Speed of Vehicles Reduces Wildlife-Transport Collision Risk. Transp. Res. D: transport Environ. 59, 86–95. doi:10.1016/j.trd.2017.12.003
Wasserman, T. N., Cushman, S. A., Shirk, A. S., Landguth, E. L., and Littell, J. S. (2012). Simulating the Effects of Climate Change on Population Connectivity of American Marten (Martes Americana) in the Northern Rocky Mountains, USA. Landscape Ecol. 27, 211–225. doi:10.1007/s10980-011-9653-8
Williams, A. F., and Wells, J. K. (2005). Characteristics of Vehicle-Animal Crashes in Which Vehicle Occupants Are Killed. Traffic Inj. Prev. 6, 56–59. doi:10.1080/15389580590903186
Zeller, K. A., Wattles, D. W., Conlee, L., and Destefano, S. (2021). Response of Female Black Bears to a High‐density Road Network and Identification of Long‐term Road Mitigation Sites. Anim. Conserv. 24, 167–180. doi:10.1111/acv.12621
Keywords: habitat connectivity, habitat permeability, landscape resistance, Omniscape, road ecology, wildlife connectivity
Citation: de Rivera CE, Bliss-Ketchum LL, Lafrenz MD, Hanson AV, McKinney-Wise LE, Rodriguez AH, Schultz J, Simmons AL, Taylor Rodriguez D, Temple AH and Wheat RE (2022) Visualizing Connectivity for Wildlife in a World Without Roads. Front. Environ. Sci. 10:757954. doi: 10.3389/fenvs.2022.757954
Received: 13 August 2021; Accepted: 24 January 2022;
Published: 16 February 2022.
Edited by:
Wendy Collinson, Endangered Wildlife Trust, South AfricaReviewed by:
Mirko Di Febbraro, University of Molise, ItalyRobert Nathan Fisher, United States Geological Survey (USGS), United States
Copyright © 2022 de Rivera, Bliss-Ketchum, Lafrenz, Hanson, McKinney-Wise, Rodriguez, Schultz, Simmons, Taylor Rodriguez, Temple and Wheat. This is an open-access article distributed under the terms of the Creative Commons Attribution License (CC BY). The use, distribution or reproduction in other forums is permitted, provided the original author(s) and the copyright owner(s) are credited and that the original publication in this journal is cited, in accordance with accepted academic practice. No use, distribution or reproduction is permitted which does not comply with these terms.
*Correspondence: M. D. Lafrenz, bGFmcmVuekBwZHguZWR1
†These authors have contributed equally to this work and share first authorship