- 1Ocean Sciences Department, University of California, Santa Cruz, Santa Cruz, CA, United States
- 2Department of Biology, University of Massachusetts Boston, Boston, MA, United States
Kelp forests dominate autotrophic biomass and primary productivity of approximately 30,000 to 60,000 km of shallow temperate and Arctic rocky reef coastline globally and contribute significantly to carbon cycling in the coastal ocean. Rapid biomass turnover is driven by very high growth rates and seasonal environmental drivers. As a result, kelp biomass varies greatly with time, space, and by species. In the northeast Pacific region, bull kelp (Nereocystis leutkeana) and giant kelp (Macrocystis pyrifera) form extensive floating surface canopies with a distinct spectral signature compared to the surrounding water. Studies have shown that remote sensing is advantageous for deriving large-scale estimates of floating surface canopy biomass, which comprises more than 90% of bull and giant kelp standing stock. However, development and validation of remotely derived kelp canopy biomass is lacking because existing approaches are time intensive and costly. This study attempted to close that gap by developing a rapid survey design utilizing diver and unmanned aerial vehicle (UAV) imagery across six sites in northern and central California. Kelp sporophytes were collected and measured for morphometric characteristics and genera-specific allometry to canopy biomass. Kelp density was measured using in situ diver surveys and coupled with UAV imagery to quantify kelp canopy biomass at a range of ground sampling distances. We successfully estimated kelp canopy biomass from UAV imagery at 33% (2/6) of the survey sites, but consistently determining canopy biomass via this approach was challenged by both survey design and kelp patch-specific spatial characteristics. The morphologies of bull kelp in Monterey were significantly different than other regions measured, but further work should be conducted to fully characterize differences in canopy biomass at the regional and sub-regional scale. We use this opportunity to suggest survey design strategies that will increase the success of future methodological development of UAV biomass retrieval. We also recommend developing long-term, annual genera-specific monitoring programs across the northeast Pacific region and beyond to validate remote sensing derived biomass estimates beyond the small number of existing well-characterized sites.
Introduction
Kelp forests are highly productive and diverse nearshore ecosystems that thrive along shallow temperate and Arctic rocky reefs (Steneck et al., 2002) and they dominate autotrophic biomass and primary productivity across approximately 25% of the world’s coastlines (Reed and Brzezinski, 2009; Wernberg et al., 2019). As a result, kelp forests are a biogenic habitat that support a range of goods and services of ecological (three-dimensional habitat structure, biodiversity, nutrient cycling, etc.) and economic (coastline defense, recreational and commercial fisheries, harvestable biomass) value (Teagle et al., 2017). Kelps contribute significantly to nutrient and carbon cycling in the coastal ocean (Reed and Brzezinski, 2009), where rapid biomass turnover is driven by very high growth rates and seasonal environmental drivers (Reed et al., 2008). However, deriving large-scale estimates of kelp standing stock is challenging because kelp forests and macroalgal habitats are spatially and temporally dynamic (Dayton et al., 1999). Distribution of biomass is driven by species, environmental conditions, and physical disturbance such as waves and swell (Ebeling et al., 1985), and patch-level biological and physical factors such as grazing, spore dispersal, and substrate type (Dayton et al., 1984). These challenges influence our ability to determine accurate regional and global rates of kelp net primary productivity, carbon cycling, and carbon sequestration. Determining kelp biomass based carbon metrics is imperative as kelp forests are threatened by the influence of anthropogenic factors such as climate change (Wernberg et al., 2016; Rasher et al., 2020), increasing frequency and intensity of marine heatwaves (Oliver et al., 2017; Cavanaugh et al., 2019; Straub et al., 2019; Dexter et al., 2020; McPherson et al., 2021), and overfishing (Ling et al., 2009).
In the northeast Pacific region (Aleutians Islands, Alaska to Baja California, Mexico) giant kelp (Macrocystis pyrifera) and bull kelp (Nereocystis luetkeana) are the dominant surface canopy forming kelps. Their canopies are easily observed using remote sensing techniques, such as plane-based aerial surveys, spaceborne satellites, and unmanned aerial vehicles (UAVs) because the spectral signature of the surface canopy is distinct from the surrounding water. This is advantageous for determining estimates of standing stock because nearly all (>90%) of bull and giant kelp biomass and, thereby primary production, is contained within the surface canopy. Historically, the most common kelp mapping approaches have evaluated kelp canopy area rather than biomass because 1) many mapping campaigns are conducted by state natural resource departments that have prioritized canopy extent as a metric for kelp as a harvestable and managed natural resource (e.g. California Department of Fish and Wildlife (CDFW), Oregon Department of Fish and Wildlife (ODFW), Washington Department of Natural Resources (WDNR), Alaska Department of Fish and Game, etc.), and 2) species- or region-specific relationships between pixel spectral characteristics and biomass have not been widely developed nor validated. While there is significant value in monitoring kelp canopy area, estimating large-scale rates of primary productivity and carbon sequestration will continue to be limited unless region and genera-specific biomasses can be derived from remote sensing data.
Studies reporting validation of remote sensing derived estimates of kelp canopy biomass are limited, but the general approach is to establish location specific empirical relationships between in situ measurements of kelp biomass and image-based kelp classification methods. Allometry is primarily used to derive in situ biomass from kelp morphology such as blade length, bulb diameter (Stekoll et al., 2006), or a combination of multiple morphometric features (Rassweiler et al., 2008). Stekoll et al. (2006) created the first remote estimates of kelp canopy biomass for bull kelp and Allaria fistulosa in southeast Alaska using high spatial resolution (0.5–2 m) multispectral (4 band) airborne data and ground truthing techniques in 2002 and 2003. Normalized difference [(
In addition to the applicability of biomass predictions, there is increasing motivation to apply higher spatial resolution (<30 m) imagery to kelp mapping efforts in regions where complex shoreline topography exists (Nijland et al., 2019) and significant kelp canopy declines have occurred (McPherson et al., 2021). To date, a range of platforms with varying spatial resolutions have been applied to kelp mapping efforts (Schroeder et al., 2019). Multispectral USGS Landsat imagery (30 m spatial resolution) has been widely used because of the large temporal and spatial scales at which data are freely available (Cavanaugh et al., 2010; Cavanaugh et al., 2011; Bell et al., 2015; Young et al., 2015; Beas-Luna et al., 2020; Bell et al., 2020b; Friedlander et al., 2020; McPherson et al., 2021; Houskeeper et al., 2022). Studies comparing the suite of sensors (Landsat TM, ETM+, and OLI) to higher spatial resolution aerial imagery (e.g. CDFW/ODFW) have found that though false positives (water pixels mis-identified as kelp) by Landsat are uncommon, the sensor often misses pixels containing less than 20% kelp (Hamilton et al., 2020; Finger et al., 2021). Furthermore, the difference between relatively high (∼2 m) and moderate (30 m) spatial resolution is pronounced when canopy coverage is low (Finger et al., 2021; kelp reflectance signals are lower than Landsat’s detection capabilities) or coastline features (large tidal range and complex topography) limit detection of fringing kelp beds within a 30 m buffer to the shore (Nijland et al., 2019). Mora-Soto et al. (2020) and Huovinen et al. (2020) were the first studies to use European Space Agency (ESA) Sentinel-2 (10 m) imagery to map giant kelp. The creation of a global kelp map by Mora-Soto et al. (2020) was validated against previously surveyed or observed beds, but the approach was not effective at detecting bed sizes <1 ha (10,000 m2) and results were limited to kelp canopy area rather than biomass or productivity.
Increasingly, scientists and managers are using UAV platforms to customize and validate kelp mapping efforts, despite the tradeoffs in the total area covered using UAVs versus other platforms and logistical challenges. UAVs offer flexibility for studying kelp beds at local, and potentially sub-regional, scales and have applicability in offshore aquaculture (Bell et al., 2020a), satellite remote sensing validation (Mora-Soto et al., 2020), physiology (Bell et al., 2020a), and may supplement expensive aerial surveys by resource management/monitoring efforts (Hohman et al., 2019). UAVs are also optimal for observations of local scale kelp bed variability; Cavanaugh et al. (2021) illustrated the significance of tidal height and current velocity on changes in kelp canopy area and the effect across sites.
Given the flexibility of UAV platforms, developing effective field surveys is more accessible than with satellite imagery and the potential for quantifying fine-scale physiological metrics using UAVs mounted with multispectral or hyperspectral sensors is possible given sufficient understanding of biomass. Due to the limited number of validation studies deriving kelp canopy biomass using remote sensing data we designed a rapid sampling approach where morphology measurements and diver surveys were used to estimate in situ kelp canopy biomass and a UAV platform was used to map floating kelp canopy. Though the surveys were designed with the aim to develop and scale regional estimates of giant and bull kelp canopy biomass, this was challenged by several factors. Key outcomes include 1) an investigation of the regional differences in allometric relationships for canopy biomass derivation, and 2) characterizing the features of both the survey design and kelp patch that influenced our ability to reliably retrieve estimates of canopy biomass from the UAV imagery. Finally, we suggest in situ sample designs to improve outcomes for future approaches to deriving canopy biomass using remote sensing platforms.
Methods
Survey locations–Six sites along the California coastline were selected for in situ diver and UAV surveys in July and August 2019 (Figures 1, 2; Table 1). Three of the sites consisted of pure bull kelp, two in Mendocino County in northern California (Figure 1 magenta box; Figures 2A, B) and one along the northeastern side of the Monterey Peninsula in central California (Figure 1 green box; Figure 2C). The other three consisted of pure giant kelp along the northeastern side of the Monterey Peninsula (Figure 1 green box; Figures 2D–F). The length of time between conducting the dive and UAV surveys for sites did not exceed 30 days. Site locations were chosen based on accessibility to kelp beds, protection from large swell, sporophyte collection and transport to shore, and ease of accessibility for UAV flight operations.
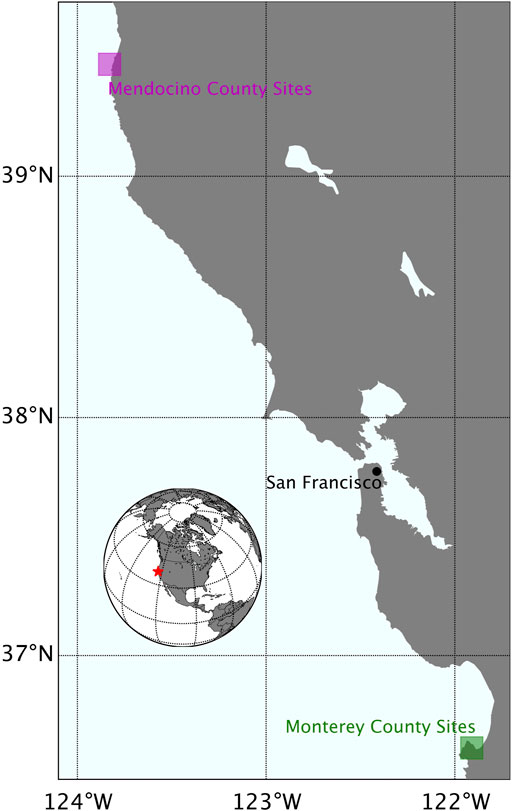
FIGURE 1. A map of the California coastline illustrating specific areas where survey sites were located in Mendocino County (magenta box) and Monterey County (green box).
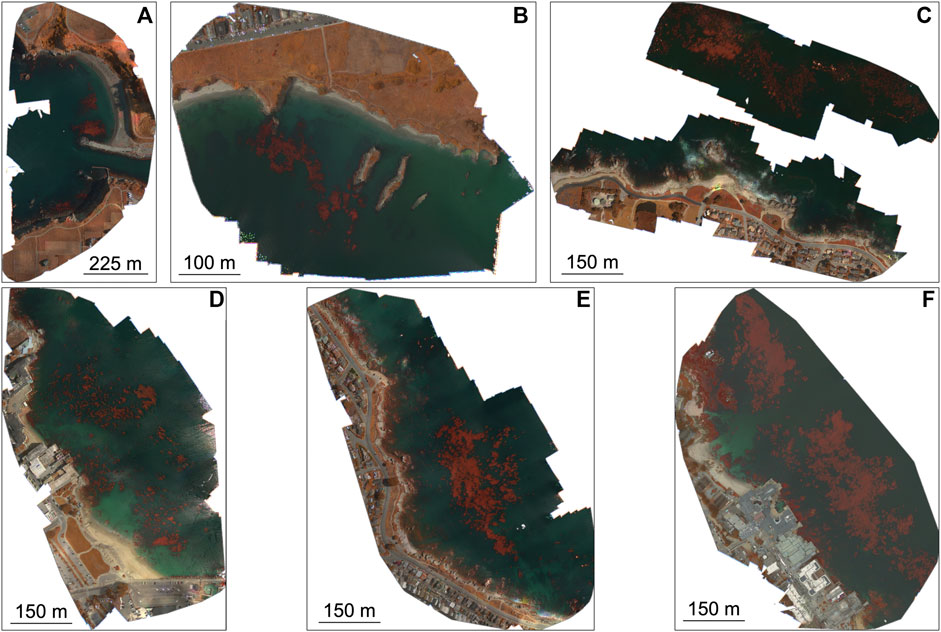
FIGURE 2. Micasense RedEdge-M false color orthomosaics of the six survey sites. (A) Noyo Harbor (NH), Mendocino County; (B) Portuguese Beach (PB), Mendocino Peninsula; (C) Point Piños (PP), Monterey Peninsula; (D) Otter Cove (OC), Monterey Peninsula; (E) San Carlos Beach (SCB), Monterey Peninsula; (F) Hopkins Marine Station (HMS), Monterey Peninsula.
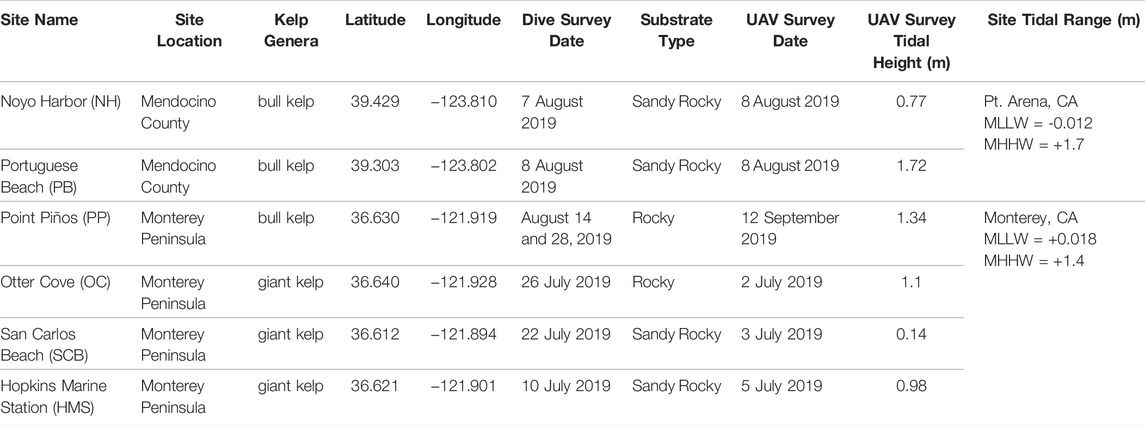
TABLE 1. Detailed description of each survey location including region (Mendocino County or Monterey Peninsula), kelp genera, dive site coordinates, dive survey date, substrate type, UAV survey date, and mean tidal height during each UAV survey, survey site mean state (MLLW and MHHW) from Pt. Arena, CA and Monterey, CA (tidesandcurrents.noaa.gov).
UAV data acquisition and processing–A graphical overview describing the processing scheme for UAV imagery (top panel) and in situ surveys (bottom panel) is described in Figure 3. High resolution multispectral imagery were obtained at each survey site (Table 1) using a DJI Matrice 100 quadcopter mounted with a MicaSense RedEdge-M pointed nadir to the water surface. The RedEdge-M simultaneously captures data in five spectral bands, the blue (475 nm), green (560 nm), red (668 nm), red-edge (717 nm), and near-infrared (NIR; 840 nm) (See Supplementary Table S1 for FWHM). The RedEdge-M was equipped with a downwelling light sensor (DLS) for all flights. To calibrate reflectance for each flight, we imaged a spectral calibration panel with known reflectance during the middle of each mission when UAV batteries were swapped. Our along-track overlap between consecutive images was 80% and side-track overlap between consecutive flight lines was 75%. Sun glint can distort the reflectance of pixels when imagery is collected when the Sun is at or close to zenith (90°). To avoid glint contamination, we conducted flights at or close to optimal Sun angle conditions (∼45° to zenith).
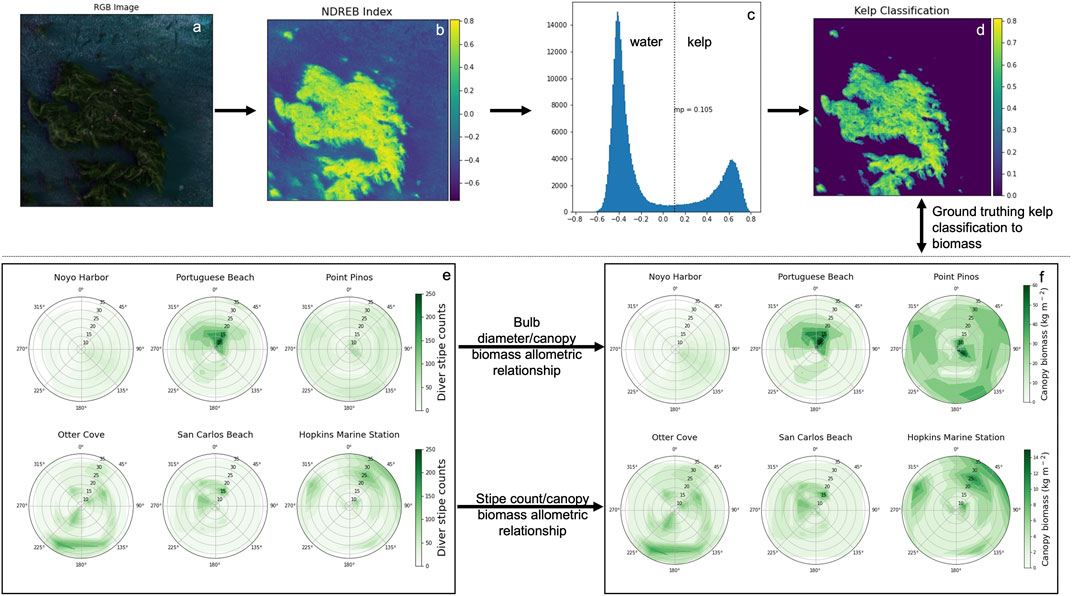
FIGURE 3. A flow chart of the methodology used in the detection of kelp in each 10 cm pixel (top panel) and deriving in situ kelp canopy biomass via diver and morphometric surveys (bottom panel).
RedEdge-M imagery was processed in the photogrammetric software Pix4D Mapper (Pix4D, 1,008 Prilly, Switzerland). Raw images with pixel values in digital numbers (DN) were converted to radiance (L; W m−2 nm−1 sr−1) using a standard MicaSense radiometric calibration model. Radiance values were converted to unitless reflectance (
and
Pixel based kelp detection–A vegetation index termed ‘Normalized Difference Red-edge Blue’ (NDREB) developed by Cavanaugh et al., 2021 was used to classify kelp pixels in UAV reflectance images (Figures 3B–D). Cavanaugh et al., 2021 showed that NDREB was superior at separating kelp and water compared to other multispectral indices. In brief, we calculated the NDREB [(
In situ surveys of kelp density and biomass–In situ surveys were used to develop spatially resolved estimates of canopy biomass for bull and giant kelp across six sites via 1) assessment of subtidal stipe (frond) density (Figure 3E), 2) sporophyte collection and morphometric measurement for development of genera-specific allometry and conversion of diver stipe (frond) density to canopy biomass (Figure 3 bottom panel arrows), and the resulting derived biomass (Figure 3F). Results from field-based data were related to NDREB kelp classification results from UAV surveys with the aim to acquire image-based estimates of kelp canopy biomass (Figure 3). For both genera, sporophyte lengths were measured to the nearest mm using a diver transect tape and weights were measured to the nearest 0.01 kg using a portable electronic balance.
Diver survey design - Subtidal surveys were designed to rapidly assess spatial distribution and patterns of stipe (frond) density across many kelp beds. Dive sites consisted of 3,600 m2 square plots surveyed in a radial configuration (Figure 4). At five of the six sites (Portuguese Beach, Point Piños, Otter Cove, San Carlos Beach, and Hopkins Marine Station; Figures 4B–F), eight separate transects were conducted. At Noyo Harbor (Figure 4A), only four of the eight transects were conducted because ocean conditions limited dive operations. Point Piños (Figure 4C) was surveyed across two separate days (Table 1) because ocean conditions limited dive operations after the first four transects were conducted on 14 August 2019.
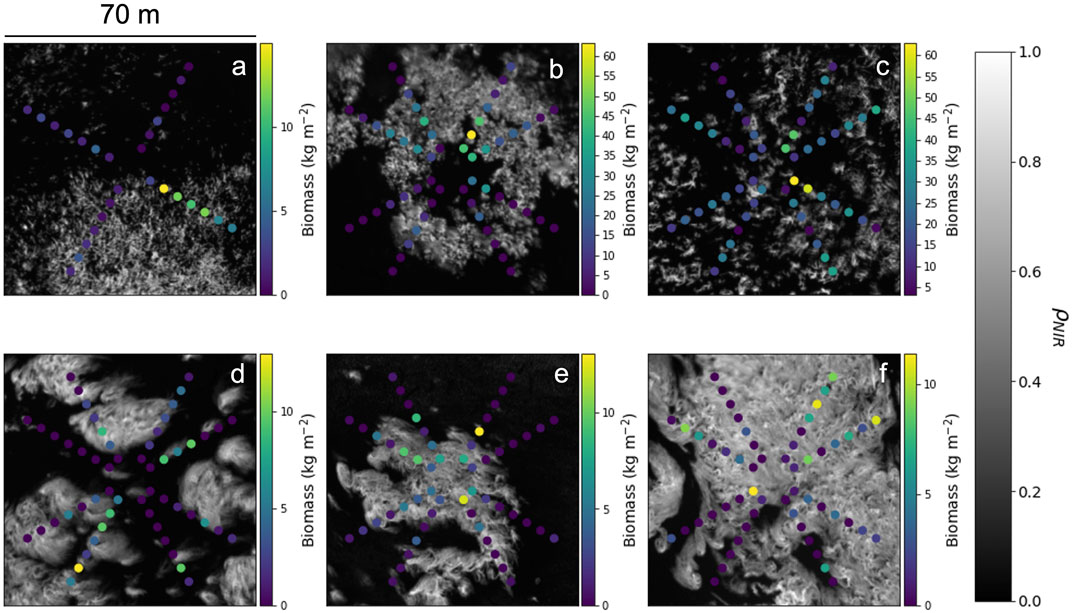
FIGURE 4. MicaSense RedEdge-M near-infrared reflectance
Survey teams consisted of two divers. One navigated each compass heading (30°, 60°, 120°, 150°, 210°, 240°, 300°, and 330°) and reeled out the transect tape to 40 m, while the other counted stipes (fronds) within a 2-m swath along the transect tape. Stipe (frond) counts were recorded for every 5-m interval (area = 10 m2), which we have termed the ‘transect interval’. Each individual transect began at the 5-m mark to avoid overlap of stipe (frond) counts at the center of the survey area. As a result, each complete dive survey consisted of a series of 4 or 8, 70 m2 transects or a total dive survey area of 280 m2 (Noyo Harbor) or 560 m2 (Portuguese Beach, Point Piños, Otter Cove, San Carlos Beach, and Hopkins Marine Station). The geospatial location of each transect interval was trigonometrically determined using the GPS location (UTM) of the center buoy and the transect distance from the center buoy.
Morphology and allometry for canopy biomass - Adult sporophytes (Figure 5), defined as the mature stage of the bull and giant kelp diploid lifecycles, were indiscriminately collected for morphometric measurement and canopy biomass determination across multiple locations in central and northern California (Table 2) in 2018 and 2019, including five of the six dive sites surveyed in 2019 (Noyo Harbor, Point Piños, Otter Cove, Hopkins Marine Station, and San Carlos Beach). Specifically, we measured morphology at four sites for giant kelp (total of 11 sporophytes) in 2019 and six sites for bull kelp (total of 86 sporophytes) in 2018 and 2019 (Table 2). Divers removed sporophytes from the substrate manually by cutting the primary stipe just above the holdfast (Figure 5; Supplementary Figure S1A), brought them to the surface and then to shore where morphometric measurements were conducted on a clean surface (Supplementary Figures S1B–F). When tissue hydration could not be maintained using fresh seawater, a portable pop-up tent was used to shade samples (Supplementary Figures 1C, D).
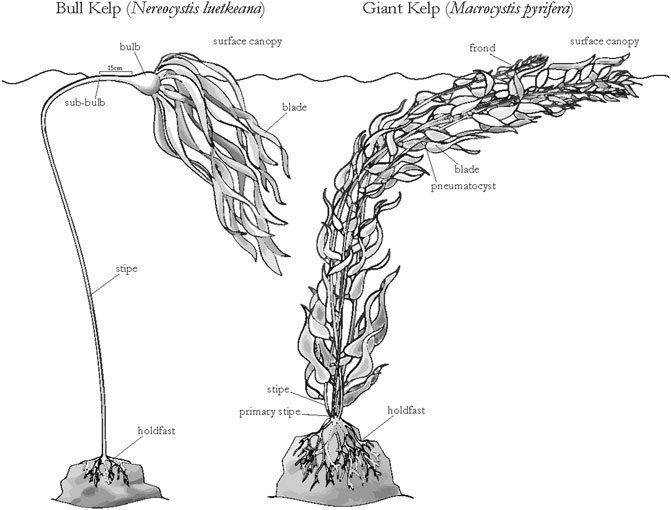
FIGURE 5. Relevant morphometric characteristics of bull kelp (Nereocystis leutkeana) and giant kelp (Macrocystis pyrifera) adult sporophytes (image credit: Niky Taylor; UCSC).
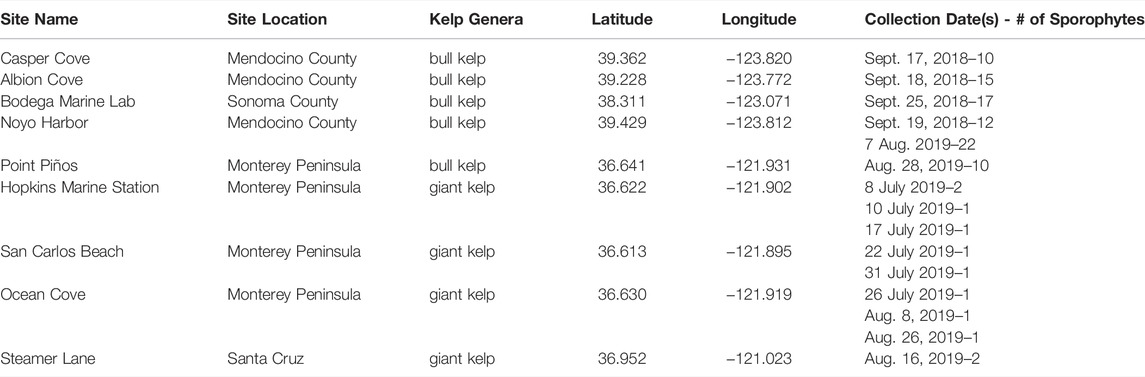
TABLE 2. Detailed information of site-specific sporophyte collection including site name, region, kelp genera, latitude/longitude, and collection dates.
Bull kelp morphometric measurements were made for stipe length and width, bulb diameter, sub-bulb diameter (15 cm below the base of the bulb), longest blade length/width, longest blade weight, number of blades, canopy weight (top 1 m of stipe including all the blade biomass), and total sporophyte weight (Figure 5). Central and northern California data from 2018 to 2019 (n = 86) were combined with data collected on the western coast of Prince of Wales Island, Alaska in 2018 (Pearson and Eckert, 2019; n = 55).
Bulb diameter was randomly measured within the 3,600 m2 dive survey plot. The site-specific mean bulb diameter was used to estimate the mean canopy biomass per sporophyte at each bull kelp site. The total wet biomass for each transect interval (10 m2) was calculated by taking the product of the stipe counts within each transect interval and the site-specific mean canopy biomass per sporophyte derived from allometry.
Giant kelp sporophytes were divided into two sections, the sub-surface canopy and surface canopy (Figure 5). The surface canopy was determined by measuring the depth of the holdfast prior to collection. Within each section, morphometric measurements were made for total tissue weight, number of blades, and number of fronds. Central California giant kelp data were combined with SBC LTER measurements of canopy biomass and frond counts from 2002 to 2003 (Reed and Rassweiler, 2018; n = 36). At each giant kelp dive site, the canopy biomass per transect interval was estimated using the allometric relationship developed between the total number of stipes (fronds)/sporophyte and the canopy biomass. We counted within each transect interval to calculate the corresponding canopy biomass. An ANCOVA was run using the Python 3.1 pingouin statistics module (Vallat, 2018) to determine if region (and season) had an influence on the slope and intercepts of the relationship between the dependent variable (bulb diameter or frond count) and canopy biomass.
Retrieving canopy biomass from NDREB–A spatial analysis was conducted to compare in situ derived canopy biomasses to mean NDREB kelp classification at four different radii around the transect interval (0.5, 1.5, 2.5, 5 m). We chose these radii because they relate to relevant spaceborn sensor’s ground sampling distances - PlanetScope (3 m), Planet RapidEye (5 m), ESA Sentinel-2 (10 m) (spectral characteristics are described in Supplementary Table S1). All pixels that fell within the radius were averaged, resulting in a single mean NDREB value per transect interval location. A full site level comparison of mean biomass and mean NDREB was also conducted with relevance to deriving canopy biomass from the Landsat suite (30 m) and SBC LTER retrievals of canopy biomass with Landsat (Cavanaugh et al., 2011; Bell et al., 2015).
Semi-variogram analysis–A geostatistical approach was used to describe kelp patch spatial autocorrelation and patch characteristics by calculating the semi-variance of each survey site’s NDREB (Figure 3D) and in situ biomass measurements (Figure 3F). Prior to conducting the variogram analysis, in situ biomass data were interpolated into a 2-D grid with the MATLAB function griddata, using a triangulation-based linear interpolation method. We used the Python 3.1 Variogram and DirectionalVariogram classes within the SciKit-GStat (skgstat) module to determine the semi-variance (
where NDREB (or in situ biomass) are the observations at locations xi and xi + h and N(h) is the number of point pairs at that lag. Semi-variance parameters were estimated by fitting spherical models to the empirical semivariograms:
where
Results
Allometric relationships for canopy biomass were developed for bull kelp and giant kelp based on morphometric measurements and their relationship to canopy biomass (Figure 5). Longest blade length was the strongest predictor of bull kelp canopy biomass (r2 = 0.78; Table 3). Sub-bulb diameter, bulb diameter, and number of blades were also significant predictors of canopy biomass (r2 = 0.72, 0.64, and 0.48, respectively; Table 3). Our results were consistent with Stekoll et al. (2006) for bull kelp in Alaska. Bulb diameter was selected to use for deriving canopy biomass in this study because bulb diameter can be quickly measured by divers or from a boat at the surface of the kelp canopy. The strongest predictor of giant kelp canopy biomass was the number of canopy blades (r2 = 0.81; Table 3). Additionally, mean canopy frond length and number of fronds at the base of the sporophyte were statistically significant predictors of canopy biomass (r2 = 0.57 and 0.48, respectively; p < 0.05). Our sample size of sporophytes was relatively small, collected in July and August 2019 (n = 11). However, combining our dataset with SBC LTER measurements of stipe (frond) count and canopy biomass collected monthly in 2002 and 2003 increased the sample size to n = 40, increased the F-statistic 23.1 (p < 0.05), but reduced the overall coefficient of determination to 0.32 (Table 3).

TABLE 3. Linear regression statistics for the different morphometric measurements for estimates of wet weight.
All in situ diver measurements of bull kelp bulb diameter displayed a Gaussian probability distribution (Figures 6A–C). The range of bulb diameter at Point Piños (Figure 6C; 7.06
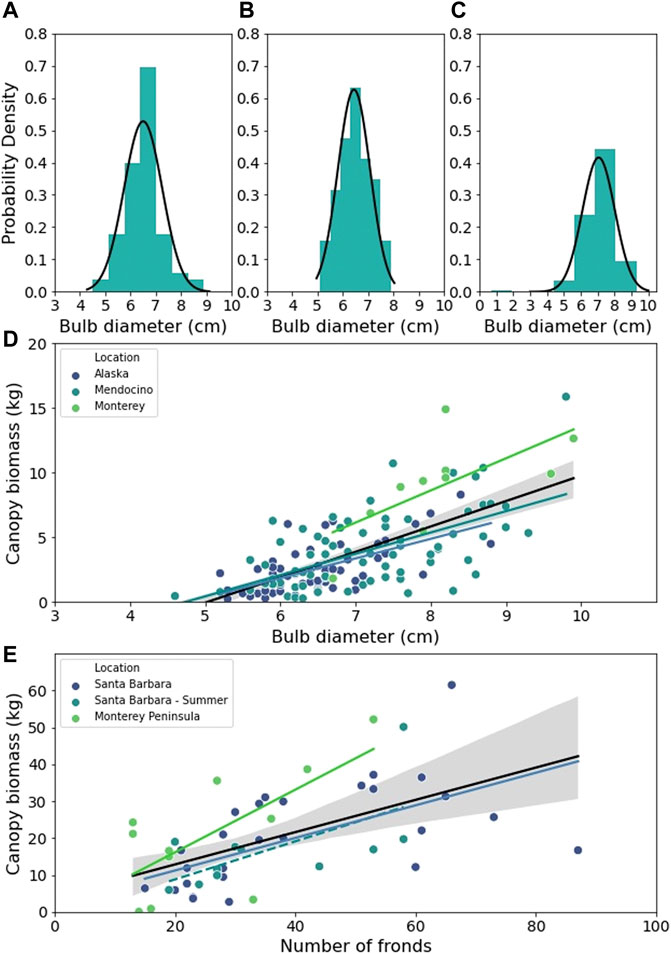
FIGURE 6. The probability distribution of bull kelp bulb diameter for (A) Noyo Harbor, (B) Portuguese Beach, (C) Point Piños. (D) Scatter plot of wet bull kelp canopy biomass against bulb diameter fitted with OLS linear regressions for Alaska (blue line), Mendocino (teal line), Monterey (green line), and all regions combined (black line). (E) Scatter plot of wet giant kelp canopy biomass against frond number fitted with OLS linear regressions for all data from Santa Barbara, CA (blue line), summer data from Santa Barbara (blue dashed line), Monterey, CA (green line), and all regions combined (black line). Regression details listed in Supplementary Table S1. Grey shaded areas represent regression 95% CI.
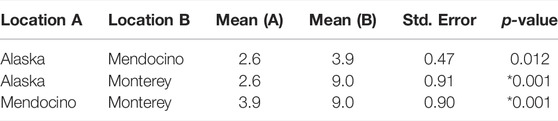
TABLE 4. Results from pairwise Tukey’s HSD post-hoc test for region specific bull kelp bulb diameters. *denotes significance.

TABLE 5. ANCOVA results for the effect of region (and seasons for giant kelp) on the slope and intercepts of the relationship between the dependent variable (bulb diameter or frond count) and canopy biomass. Bull kelp regions are Monterey, Mendocino, and Alaska. Giant kelp regions/seasons are Santa Barbara (all), Santa Barbara (summer), and Montery. *denotes significance.
Though measured canopy biomass values ranged significantly across site and genera, NDREB values were generally within range of each other (Figure 7; top row = bull kelp sites; bottom row = giant kelp sites). A predictive relationship between canopy biomass and NDREB was observed at two of the study sites, Portuguese Beach (bull kelp; Figure 7B) and San Carlos Beach (giant kelp; Figure 7E) for all sampling radii (0.5, 1.5, 2.5 m; Table 6). Regression statistics for these two sites was characterized by high F-statistic and r2 values relative to the other four sites. However, across all sites, there was no influence of averaging radii on the predictive power of the relationship between canopy biomass and NDREB. Portuguese Beach (Figure 7C) and Point Piños exhibited the largest range in measured canopy biomass (0 to >60 kg-ww m−2; Figure 7) that were associated with high diver recorded stipe densities (Figures 3E, F). Conversely, giant kelp stations had a smaller ranged in measured canopy biomass as a result of lower diver measured stipe densities (0–15 kg-ww m−2; Figures 7D–F).
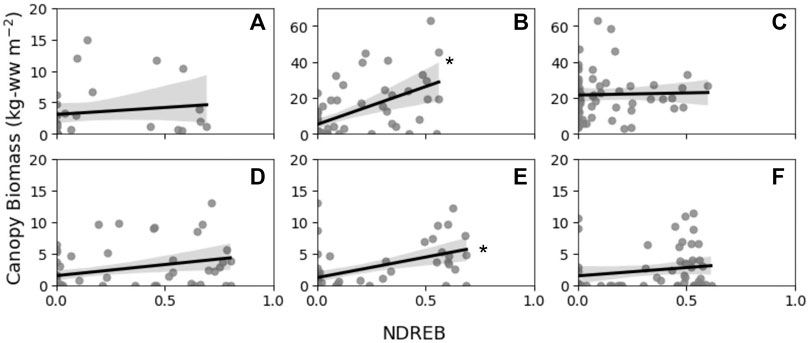
FIGURE 7. Transect interval canopy biomass in kg wet weight (kg-ww) against average NDREB values from a 1.5 m radius around each transect interval (A) Noyo Harbor; (B) Portuguese Beach; (C) Point Piños; (D) Otter Cove; (E) San Carlos Beach; (F) Hopkins Marine Station with OLS regression (black lines) and 95% confidence intervals (grey shading). Significance of the OLS regression is indicated with an asterisk. See Table 6 for each sampling radii and site’s regression statistics.
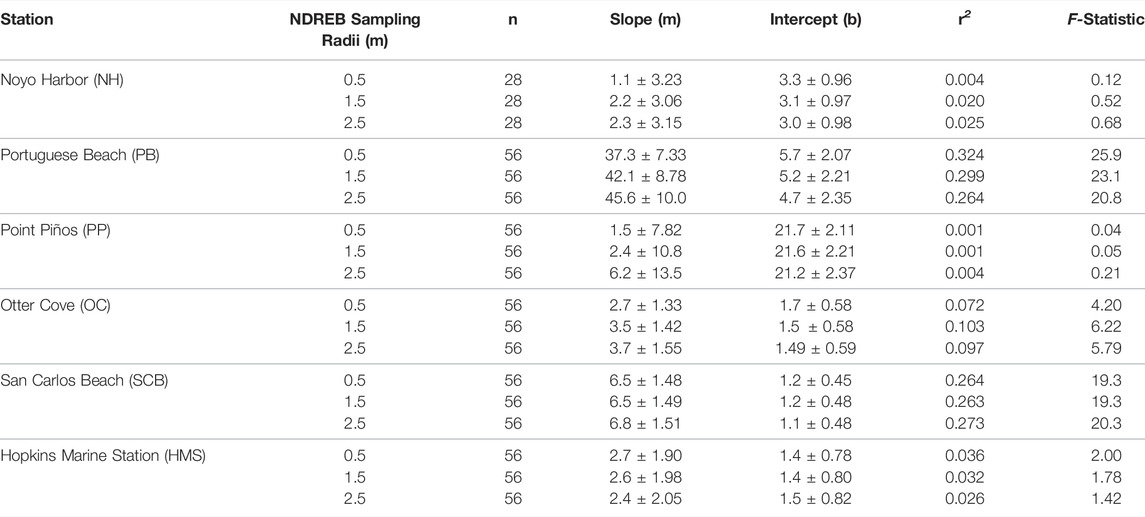
TABLE 6. Original Least Squared (OLS) regression statistics from Figure 7 with slope and intercept values
No significant relationship was observed when site level means of NDREB and in situ canopy biomass were compared (Figure 8). Portuguese Beach and Point Piños showed high mean canopy biomass relative to the mean station NDREB reflecting higher diver measured stipe densities for these two bull kelp sites (Figures 3E, F). The remaining four stations were characteristic of relatively low mean canopy biomass and a range of mean NDREB values from 0.18 to 0.39 reflecting sparser diver measured stipe densities at those four stations.
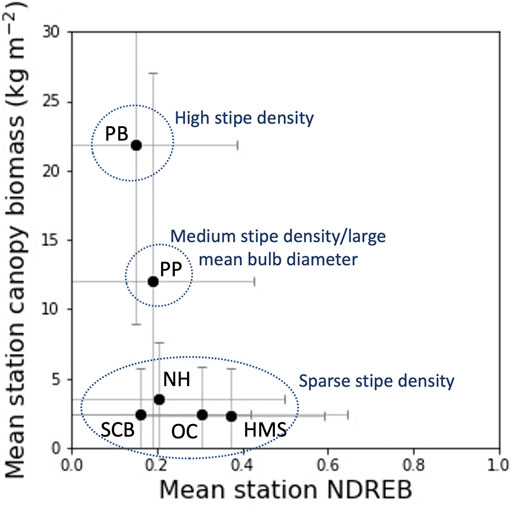
FIGURE 8. Mean station canopy biomass plotted again mean station NDREB with
The descriptive variogram analysis conducted for NDREB and diver measured canopy biomass indicated differences in patch level spatial patterns between Portuguese Beach, San Carlos Beach and the other survey sites (Figure 9). Although we were not able to consistently develop predictions of canopy biomass from NDREB, the patterns of semi-variance across the site’s spatial extents were similar between the diver surveys and NDREB, indicating that similar spatial patterns were being observed with both methods. For Portuguese Beach and San Carlos Beach (the two sites where robust relationships of canopy biomass were achieved), a dominant ‘u-shaped’ pattern was visible in the semi-variograms, where variability rapidly rises to the edge of the patch (the peak of the ‘u-shape’) and falls outside of the patch beyond approximately 30 m from the center of the survey site.
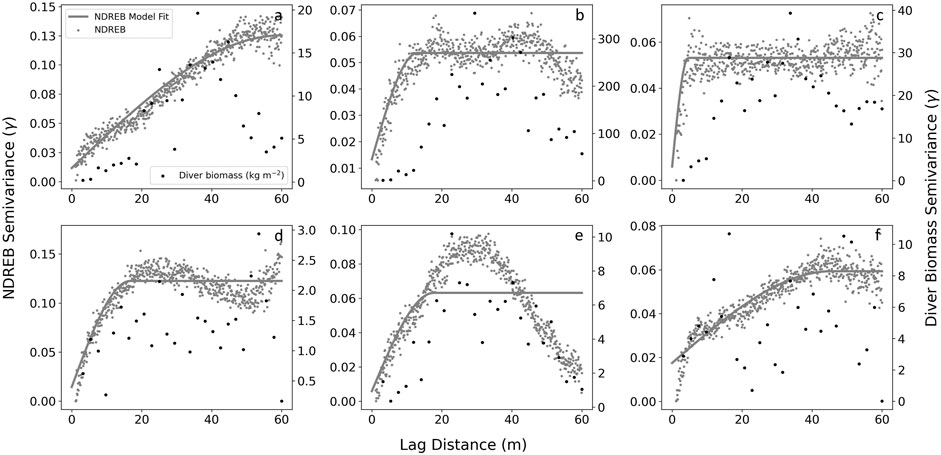
FIGURE 9. Semi-variogram for each station NDREB (grey) and diver measured canopy biomass (black). NDREB are overlayed with a spherical model fit. (A) Noyo Harbor; (B) Portuguese Beach; (C) Point Piños; (D) Otter Cove; (E) San Carlos Beach; (F) Hopkins Marine Station.
Discussion
In the northeast Pacific region, bull kelp and giant kelp form the base of an ecologically and economically important temperate nearshore coastal ecosystem with extensive floating surface canopies. Remote sensing is advantageous for detecting their floating canopy and deriving large-scale estimates of surface canopy biomass, which comprises more than 90% of bull and giant kelp standing stock (Rassweiler et al., 2008). Development and validation of remotely derived kelp canopy biomass is lacking because the associated methodology is time intensive and costly, but important because biomass is necessary for determining rates of net primary productivity and quantifying carbon cycling in temperate nearshore regions. In this study, we compiled bull and giant kelp morphometric measurements from different regions in the NE Pacific to assess the validity of applying a single biomass conversion relationship across disparate regions. We also surveyed six spatially distinct sites (3 bull kelp and 3 giant kelp) with varying stipe and canopy characteristics for measurements of in situ canopy biomass via diver and UAV surveys with the aim to retrieve canopy biomass estimates from remote sensing imagery. The results exhibited that 1) kelp morphology differed slightly across the regions we examined but didn’t strongly influence in situ estimates of canopy biomass, and 2) reliable, consistent remote sensing retrieval of canopy biomass was difficult using the survey approach developed here and dependent on specific kelp patch characteristics across sites.
Kelp allometry for canopy biomass measurements–Deriving and utilizing allometric relationships for canopy biomass is a key component of developing methods for canopy biomass retrieval from spaceborne and aerial platforms (Stekoll et al., 2006; Cavanaugh et al., 2010). In this study, we compared the regional differences in bull kelp bulb diameter and giant kelp stipe count as predictors of canopy biomass and found that across all regions, these metrics were robust predictors of canopy biomass. The effect of region on the slopes and intercept of the linear relationships were small for both genera (Figure 6; Table 4 and Table 5) and indicates that it may be generally acceptable to apply a relationship derived from one region to others across the NE Pacific. It is possible that the morphological differences we observed were a result of local-scale hydrodynamics influencing tissue morphology, rather than regional-scale processes (Koehl and Alberte, 1988; Koehl, 2022).
However, the sample sizes for some of our regions were relatively low (e.g. giant kelp from the Monterey region). Sample sizes of studies collecting sporophyte morphology is influenced by the per sporophyte effort it requires to collect this information. A single giant kelp sporophyte can take up to 15 person-hours to conduct a complete morphometric survey. Bull kelp is less labor intensive, on the order of two person-hours per sporophyte. Both genera require SCUBA (from shore or boat) to collect and deliver sporophytes that can weigh up to 60 kg to the lab for processing. While the logistical challenges may be the primary reason for the lack of data, the collection of this data have not been prioritized by funding and management agencies in the past. We advocate for allocating resources into developing predictive relationships for canopy biomass to further improve accuracy of kelp canopy retrieval from spaceborne sensors via long-term monitoring and targeted studies with UAVs.
The influence of kelp patch characteristics on canopy biomass retrievals–Descriptive results from the semi-variogram analysis indicated that kelp bed characteristics play a role in accurately determining canopy biomass retrievals. If a kelp patch was larger than the spatial area of the dive survey (i.e. a breakdown in autocorrelation did not occur within the survey area), we were limited in our ability to develop a robust working relationship between canopy biomass and NDREB. If the kelp patch was smaller than the spatial area of the dive survey (i.e., pixel autocorrelation broke down within the dive survey area), we were able to identify a relationship between canopy biomass and kelp fraction. This pattern in the semi-variance results described sites with a distinct kelp patch surrounded by water and biomass at these sites also had a robust correlation with NDREB. Out of the six sites, Portuguese Beach (Figure 4B; Figure 7) and San Carlos Beach (Figure 4E; Figure 7) were clear examples of this pattern, exhibiting dense, relatively homogenous stipe (frond) counts inside the kelp bed and a relatively homogenous absence of stipes (fronds) outside of the kelp bed. This indicates a potential mismatch between the scale of measurements by diver survey and remote sensing because 1) surveying large patches is quantified best by satellite remote sensing but is not feasible for in situ surveys, and 2) very high spatial resolution measurements of canopy can be observed with UAVs, but diver survey methods are limited in the coarseness of their observations.
The influence of kelp genera on canopy biomass retrievals–Bull and giant kelp have distinctly different morphologies and surface canopy characteristics which influenced accurate retrievals of canopy biomass estimates from UAV based observations. Bull kelp has a single gas-filled stipe and pneumatocyst (Figure 5) with long blades (length
Diver estimates of canopy biomasses ranged significantly depending on the density of bull kelp sporophytes resulting in area normalized canopy biomasses at Portuguese Beach and Point Piños that were approximately 7 times greater than the giant kelp sites (Figure 7). Although bulb diameter can predict an individual sporophyte’s canopy biomass with relative accuracy, using stipe counts did not translate well to area normalized canopy biomass at interval locations, either because the sporophytes fanned out and distributed at the surface or because blades were below the water surface and were not detected by the sensor on the UAV. Further consideration should be given to the deepest survey site (Point Piños; ∼30 m) where it is likely that a portion of the sporophytes counted by divers didn’t reach the surface, resulting in an overestimation of in situ canopy biomass.
Giant kelp blades grow along the entire frond from the base to the growing tip (meristem; Figure 5). More of the canopy biomass is floating on the water surface or just below because the base of each giant kelp blade (length
The influence of survey design on canopy biomass retrievals - The in situ diver survey approach developed in this study was similar to methodology for Landsat derived giant kelp canopy biomass (Rassweiler et al., 2008; Cavanaugh et al., 2011) and aerial survey derived Alaska bull kelp biomass (Stekoll et al., 2006) in that disparate kelp beds were measured to quantify canopy biomass and then associated with spectral characteristics of kelp canopy. However, the goal of our survey design was to rapidly assess as many large sites as possible by only collecting stipe (frond) counts within each 5-m section of the transect. The radial survey pattern allowed us to have eight transects of reasonable length (35 m) within each survey site. By assessing a large area, we could increase the sample size, and therefore, the number of matchup locations between in situ canopy biomass and UAV imagery. However, it is possible that persistent errors and offsets were introduced to the transect interval’s geospatial locations via this approach. Despite SBC LTER’s robust prediction of Santa Barbara giant kelp canopy biomass estimates from 30 m Landsat (Cavanaugh et al., 2011; Bell et al., 2020b), we had little success developing a similar relationship using mean site level (60 × 60 m) NDREB and canopy biomass, likely due to limitations in the number of sites that could be sampled within the given timeframe of the study.
Our findings shed light on the advantages of site-specific long-term monitoring for retrieving canopy biomass from remote sensing platforms at the kelp bed scale (∼10–30 m), allows for the development of temporally and spatially robust relationships. However, this approach is not always feasible for subtidal monitoring programs (which, in itself, are lacking). We recognize there should be alternative methods to retrieving canopy biomass. UAVs provide flexibility for survey design and approach to adjust measurement temporal frequency, the spatial extent of the survey (a single bed to multiple), and postprocessing pixel spatial binning (pixel sizes from 10 cm +). If given a long enough timeseries or enough data points, UAV’s can collect the required information, but as demonstrated here, it requires multiple measurements (i.e. a timeseries) to build reliable relationships.
Suggested survey design for future UAV methods–Based on the results presented here and lessons learned, we provide a recommended survey design aimed at retrieving canopy biomass estimates from remote sensing methods. Primarily, we believe measurements should focus directly on the surface canopy rather than subtidal stipe counts or biomass (Figure 10). This allows for direct comparison of in situ surface biomass measurements to pixel-based kelp classification metrics. Specifically, we recommend conducting in situ canopy measurements across a variety of densities (either within a single bed or multiple different beds) within a bounding box marked by buoys/markers in the center and corners (Figure 10 white and orange dots). Buoys should remain deployed for the extent of the UAV survey for ground truthing and geospatial positioning of the in situ site. Quadrat sampling points for surface measurements within the bounding box can be randomized by heading/distance to remove bias associated with using underwater compass headings for calculation of GPS locations. As such GPS measurements at the center of each quadrat location can be taken. Within each 1 m2 quadrat, measurements of the bulb diameter (bull kelp), sub-bulb diameter (bull kelp), mean canopy stipe length (giant kelp), and stipe count (bull and giant kelp) can be used to estimate canopy biomass (Table 3). Taking subtidal stipe counts is a good comparison to other surveys being conducted by relevant monitoring organizations (i.e. Reef Check California, CDFW, Humble State University, etc.), but as indicated by the results presented here can be unreliable for developing fine spatial scale estimates of biomass. We recommend conducting UAV surveys on the same day just before or after in situ canopy measurements or at a similar tidal height.

FIGURE 10. Suggested survey design for future in situ validation studies of canopy biomass using a UAV platform.
Conclusion
Estimation of kelp canopy area has rapidly advanced, leading to regional-scale (e.g Bell et al., 2020b) to global-scale (e.g. Mora-Soto et al., 2020) estimates, and assessments of kelp canopy area in very remote locations (Friedlander et al., 2020; Houskeeper et al., 2022). Despite these advances, it is desirable in some cases to move beyond canopy area to assess biomass, and then primary productivity, as that provides information on kelp bed health, carbon sequestration, and viability. Validation studies of kelp canopy biomass retrievals via remote sensing are lacking because the data is difficult to collect, but this data is important because accurate large-scale estimates of productivity and carbon cycling cannot be made without them. We attempted to close the data gap by developing a rapid and thorough approach to gathering the in situ and remote sensing data that are required to retrieve biomass from remote sensing platforms. Although, specific kelp patch configuration and survey design limited the number of sites where we successfully retrieved kelp canopy biomass, we consider our findings valuable to the kelp remote sensing community, to whom there is very little of this data available. Understanding the challenges in determining remotely derived kelp canopy biomass is important and our general conclusions can be used to extrapolate to other regions and potentially other kelp genera. To aid in future efforts, we outline an improved survey design for future in situ validation studies using UAVs. Finally, we strongly recommend funding/implementation of long-term monitoring programs, such as those used by the SBC LTER, across the region for both giant and bull kelp. Critically, this requires both standardization of methodology and consistent funding by management agencies for such efforts. The alternative is to rely solely on canopy area which can be a poor proxy for biomass and productivity, limiting our understanding of how kelp beds respond to short- and long-term environmental conditions.
Data Availability Statement
The raw data supporting the conclusion of this article will be made available by the authors, without undue reservation.
Author Contributions
All authors contributed to this manuscript including conceptualization, MLM and RMK; methodology, MLM and RMK; writing—original draft preparation, MLM; writing—review and editing, MLM and RMK.
Funding
Financial support was provided by the Dr. Earl H. Myers and Ethel M. Myers Oceanographic and Marine Biology Trust and the UCSC Department of Ocean Sciences. Vessel support and lodging was provided by Laura Rogers-Bennett’s Marine Invertebrate Fisheries and Conservation Laboratory. Funding for the UAV was supported by the Ida Benson-Lynn Ocean Health endowment. The MicaSense RedEdge-M and Pix4D software was supported by the Packard Science and Technology endowment to the Institute of Marine Sciences.
Conflict of Interest
The authors declare that the research was conducted in the absence of any commercial or financial relationships that could be construed as a potential conflict of interest.
Publisher’s Note
All claims expressed in this article are solely those of the authors and do not necessarily represent those of their affiliated organizations, or those of the publisher, the editors and the reviewers. Any product that may be evaluated in this article, or claim that may be made by its manufacturer, is not guaranteed or endorsed by the publisher.
Acknowledgments
Many thanks to the all the people who made this work possible, including Heidi Hirsh (Stanford University), scientific divers (Logan Grady, Jeannette Peters, and Sara Hamilton), field volunteers (alphabetically: Dennis Finger, Aimee Foy, Megan Gallagher, Kyle Hughes, Kevin Hum, Josie Iselin, Quinn Letitia, John Mizell, Rich Muller, Brynn O'Hara, and Jezabel Powers), and sensitive wildlife monitors (Herrick Hanks, Teri Nicholson, Jennifer Parkin, Judi Romero, and Bill Standley). Development and testing of the MicaSense RedEdge-M was provided by Ben Erwin and Brent Roman. Helpful feedback on this manuscript was provided by Tom Bell (University of California Santa Barbara/Woods Hole Oceanographic Institute) and Niky Taylor (University of California Santa Cruz).
Supplementary Material
The Supplementary Material for this article can be found online at: https://www.frontiersin.org/articles/10.3389/fenvs.2022.690963/full#supplementary-material
References
Beas-Luna, R., Micheli, F., Woodson, C. B., Carr, M., Malone, D., Torre, J., et al. (2020). Geographic Variation in Responses of Kelp Forest Communities of the California Current to Recent Climatic Changes. Glob. Chang. Biol. 26, 6457–6473. doi:10.1111/gcb.15273
Bell, T. W., Allen, J. G., Cavanaugh, K. C., and Siegel, D. A. (2020b). Three Decades of Variability in California's Giant Kelp Forests from the Landsat Satellites. Remote Sens. Environ. 238, 110811. doi:10.1016/j.rse.2018.06.039
Bell, T. W., Cavanaugh, K. C., and Siegel, D. A. (2015). Remote Monitoring of Giant Kelp Biomass and Physiological Condition: An Evaluation of the Potential for the Hyperspectral Infrared Imager (HyspIRI) Mission. Remote Sens. Environ. 167, 218–228. doi:10.1016/j.rse.2015.05.003
Bell, T. W., Nidzieko, N. J., Siegel, D. A., Miller, R. J., Cavanaugh, K. C., Nelson, N. B., et al. (2020a). The Utility of Satellites and Autonomous Remote Sensing Platforms for Monitoring Offshore Aquaculture Farms: A Case Study for Canopy Forming Kelps. Front. Mar. Sci. 7, 520223. doi:10.3389/fmars.2020.520223
Cavanaugh, K. C., Cavanaugh, K. C., Bell, T. W., and Hockridge, E. G. (2021). An Automated Method for Mapping Giant Kelp Canopy Dynamics from UAV. Front. Environ. Sci. 8, 587354. doi:10.3389/fenvs.2020.587354
Cavanaugh, K. C., Reed, D. C., Bell, T. W., Castorani, M. C. N., Beas-luna, R., and Barrett, N. S. (2019). Spatial Variability in the Resistance and Resilience of Giant Kelp in Southern and Baja California to a Multiyear Heatwave. Front. Mar. Sci. 6, 1–14. doi:10.3389/fmars.2019.00413
Cavanaugh, K., Siegel, D., Kinlan, B., and Reed, D. (2010). Scaling Giant Kelp Field Measurements to Regional Scales Using Satellite Observations. Mar. Ecol. Prog. Ser. 403, 13–27. doi:10.3354/meps08467
Cavanaugh, K., Siegel, D., Reed, D., and Dennison, P. (2011). Environmental Controls of Giant-Kelp Biomass in the Santa Barbara Channel, California. Mar. Ecol. Prog. Ser. 429, 1–17. doi:10.3354/meps09141
Dayton, P. K., Currie, V., Gerrodette, T., Keller, B. D., Rosenthal, R., and Tresca, D. V. (1984). Patch Dynamics and Stability of Some California Kelp Communities. Ecol. Soc. Am. 54, 253–289. doi:10.2307/1942498
Dayton, P. K., Tegner, M. J., Edwards, P. B., and Riser, K. L. (1999). Temporal and Spatial Scales of Kelp Demography: The Role of Oceanographic Climate. Ecol. Monogr. 69, 219–250. doi:10.1890/0012-9615(1999)069[0219:tassok]2.0.co;2
Dexter, K. F., Wernberg, T., Grace, S. P., Thormar, J., Fredriksen, S., Narvaez, C. N., et al. (2020). Marine Heatwaves and the Collapse of Marginal North Atlantic Kelp Forests. Sci. Rep. 10, 13388. doi:10.1038/s41598-020-70273-x
Ebeling, A. W., Laur, D. R., Rowley, R. J., and Barbara, S. (1985). Severe Storm Disturbances and Reversal of Community Structure in a Southern California Kelp Forest. Mar. Biol. 84, 287–294. doi:10.1007/bf00392498
Finger, D. J. I., McPherson, M. L., Houskeeper, H. F., and Kudela, R. M. (2021). Mapping Bull Kelp Canopy in Northern California Using Landsat to Enable Long-Term Monitoring. Remote Sens. Environ. 254, 112243. doi:10.1016/j.rse.2020.112243
Friedlander, A. M., Ballesteros, E., Bell, T. W., Caselle, J. E., Campagna, C., Goodell, W., et al. (2020). Kelp Forests at the End of the Earth: 45 Years Later. PLoS One 15, e0229259. doi:10.1371/journal.pone.0229259
Hamilton, S. L., Bell, T. W., Watson, J. R., Grorud‐Colvert, K. A., and Menge, B. A. (2020). Remote Sensing: Generation of Long‐Term Kelp Bed Data Sets for Evaluation of Impacts of Climatic Variation. Ecology 101 (7), e03031. doi:10.1002/ecy.3031
Hohman, R., Hutto, S., Catton, C. A., and Koe, F. (2019). Sonoma-Mendocino Bull Kelp Recovery Plan. San Francisco, CA: Plan for the Greater Farallones National Marine Sanctuary and the California Department of Fish and Wildlife.
Houskeeper, H. F., Rosenthal, I. S., Cavanaugh, K. C., Pawlak, C., Trouille, L., Byrnes, J. E. K., et al. (2022). Automated Satellite Remote Sensing of Giant Kelp at the Falkland Islands (Islas Malvinas). PLoS One 17, e0257933. doi:10.1371/journal.pone.0257933
Huovinen, P., Ramírez, J., Palacios, M., and Gómez, I. (2020). Satellite-Derived Mapping of Kelp Distribution and Water Optics in the Glacier Impacted Yendegaia Fjord (Beagle Channel, Southern Chilean Patagonia). Sci. Total Environ. 703, 135531. doi:10.1016/j.scitotenv.2019.135531
Koehl, M. A. R., and Alberte, R. S. (1988). Flow, Flapping, and Photosynthesis of Nereocystis Leutkeana: A Functional Comparison of Undulate and Flat Blade Morphologies. Mar. Biol. 99, 435–444. doi:10.1007/bf02112137
Koehl, M. A. R. (2022). Ecological Biomechanics of Marine Macrophytes. J. Exp. Bot. 73, 1104–1121. doi:10.1093/jxb/erab536
Ling, S. D., Johnson, C. R., Frusher, S. D., and Ridgway, K. R. (2009). Overfishing Reduces Resilience of Kelp Beds to Climate-Driven Catastrophic Phase Shift. Proc. Natl. Acad. Sci. U. S. A. 106, 22341. doi:10.1073/pnas.0907529106
McPherson, M. L., Finger, D. J. I., Houskeeper, H. F., Bell, T. W., Carr, M. H., Rogers-Bennett, L., et al. (2021). Large-Scale Shift in the Structure of a Kelp Forest Ecosystem Co-Occurs with an Epizootic and Marine Heatwave. Commun. Biol. 4, 298. doi:10.1038/s42003-021-01827-6
Mora-Soto, A., Palacios, M., Macaya, E. C., Gomez, I., Huovinen, P., Perez-Matus, A., et al. (2020). A High-Resolution Global Map of Giant Kelp (Macrocystis Pyrifera) Forests and Intertidal Green. Remote Sens. 12, 1–20. doi:10.3390/rs12040694
Nijland, W., Reshitnyk, L., and Rubidge, E. (2019). Satellite Remote Sensing of Canopy-Forming Kelp on a Complex Coastline: A Novel Procedure Using the Landsat Image Archive. Remote Sens. Environ. 220, 41–50. doi:10.1016/j.rse.2018.10.032
Oliver, E. C. J., Benthuysen, J. A., Bindoff, N. L., Hobday, A. J., Holbrook, N. J., Mundy, C. N., et al. (2017). The Unprecedented 2015/16 Tasman Sea Marine Heatwave. Nat. Commun. 8, 1–12. doi:10.1038/ncomms16101
Pearson, H., and Eckert, G. L. (2019). Morphometrics of Bull Kelp Forests in Southeast Alaska During Summer 2018 [Internet]. Available at: https://knb.ecoinformatics.org/view/urn%3Auuid%3A8463ff07-cc96-4853-bfca-79b3ee1d69e3.
Rasher, D. B., Steneck, R. S., Halfar, J., Kroeker, K. J., Ries, J. B., Tinker, M. T., et al. (2020). Keystone Predators Govern the Pathway and Pace of Climate Impacts in a Subarctic Marine Ecosystem. Science 369, 1351–1354. doi:10.1126/SCIENCE.AAV7515
Rassweiler, A., Arkema, K. K., Reed, D. C., Zimmerman, R. C., and Brzezinski, M. A. (2008). Net Primary Production, Growth, and Standing Crop Ofmacrocystis Pyriferain Southern California. Ecology 89, 2068. doi:10.1890/07-1109.1
Reed, D. C., and Brzezinski, M. A. (2009). “Kelp Forests,” in The Managment of Natural Coastal Carbon Sinks. Editors D. Laffoley, and G. Grimsditch (Gland, Switzerland: IUCN), 31–36.
Reed, D., and Rassweiler, A. (2018). SBC LTER: REEF: Allometric Measurements of Giant Kelp Ver 1. Environmental Data Initiative. Available at: https://doi.org/10.6073/pasta/e880e6b231af718c63f623893c678c86.
Reed, D. C., Rassweiler, A., and Arkema, K. K. (2008). Biomass Rather Than Growth Rate Determines Variation in Net Primary Production by Giant Kelp. Ecology 89, 2493–2505. doi:10.1890/07-1106.1
Schroeder, S. B., Dupont, C., Boyer, L., Juanes, F., and Costa, M. (2019). Passive Remote Sensing Technology for Mapping Bull Kelp (Nereocystis Luetkeana): A Review of Techniques and Regional Case Study. Glob. Ecol. Conserv. 19, e00683. doi:10.1016/j.gecco.2019.e00683
Stekoll, M. S., Deysher, L. E., and Hess, M. (2006). A Remote Sensing Approach to Estimating Harvestable Kelp Biomass. J. Appl. Phycol. 18, 323–334. doi:10.1007/s10811-006-9029-7
Steneck, R. S., Graham, M. H., Bourque, B. J., Corbett, D., Erlandson, J. M., Estes, J. A., et al. (2002). Kelp Forest Ecosystems: Biodiversity, Stability, Resilience and Future. Envir. Conserv. 29, 436–459. doi:10.1017/S0376892902000322
Straub, S. C., Wernberg, T., Thomsen, M. S., Moore, P. J., Burrows, M. T., Harvey, B. P., et al. (2019). Resistance, Extinction, and Everything in Between - The Diverse Responses of Seaweeds to Marine Heatwaves. Front. Mar. Sci. 6, 1–13. doi:10.3389/fmars.2019.00763
Teagle, H., Hawkins, S. J., Moore, P. J., and Smale, D. A. (2017). The Role of Kelp Species as Biogenic Habitat Formers in Coastal Marine Ecosystems. J. Exp. Mar. Bio. Ecol. 492, 81–98. doi:10.1016/j.jembe.2017.01.017
Vallat, R. (2018). Pingouin: Statistics in Python. J. Open Sour. Soft. 3 (31), 1026. doi:10.21105/joss.01026
Wernberg, T., Bennett, S., Babcock, R. C., de Bettignies, T., Cure, K., Depczynski, M., et al. (2016). Climate-Driven Regime Shift of a Temperate Marine Ecosystem. Science 353, 169–172. doi:10.1126/science.aad8745
Wernberg, T., Krumhansl, K., Filbee-dexter, K., and Pedersen, M. F. (2019). “Status and Trends for the World's Kelp Forests,” in World Seas: An Environmental Evaluation (Elsevier), 57–78. doi:10.1016/B978-0-12-805052-1.00003-6
Keywords: nereocystis, macrocystis, bull kelp, giant kelp, canopy biomass, semi-variogram, UAV, UAS
Citation: McPherson ML and Kudela RM (2022) Kelp Patch-Specific Characteristics Limit Detection Capability of Rapid Survey Method for Determining Canopy Biomass Using an Unmanned Aerial Vehicle. Front. Environ. Sci. 10:690963. doi: 10.3389/fenvs.2022.690963
Received: 05 April 2021; Accepted: 06 June 2022;
Published: 14 July 2022.
Edited by:
Wesley Moses, United States Naval Research Laboratory, United StatesReviewed by:
Wiebe Nijland, Utrecht University, NetherlandsTianhai Cheng, Institute of Remote Sensing and Digital Earth (CAS), China
Copyright © 2022 McPherson and Kudela. This is an open-access article distributed under the terms of the Creative Commons Attribution License (CC BY). The use, distribution or reproduction in other forums is permitted, provided the original author(s) and the copyright owner(s) are credited and that the original publication in this journal is cited, in accordance with accepted academic practice. No use, distribution or reproduction is permitted which does not comply with these terms.
*Correspondence: Meredith L. McPherson, TWVyZWRpdGguTWNQaGVyc29uQHVtYi5lZHU=