- 1Hubei Provincial Academy of Eco-Environmental Science, Wuhan, China
- 2State Environmental Protection Key Laboratory of Soil Health and Green Remediation, Wuhan, China
The identification and effective control of pollution sources is essential because heavy metal pollution in agricultural soil is associated with food safety and public health. Industrial wastewater, waste gas, and residues generated from pharmaceutical manufacturing are important sources of heavy metal pollutants in soil, but the research of their risk for surrounding agricultural soil is inadequate. In this study, the typical pharmaceutical manufacturing complex and its surrounding farmland in Hubei Province, China was employed to systematically and comprehensively assess its environmental risk and source apportionment. The results revealed the potential risk of cadmium (Cd), lead (Pb), arsenic (As), and mercury (Hg) from pharmaceutical production for farmland soil around, and among these heavy metals, As and Cd were observed to have the higher pollution level. The accumulated Cd and As had contribution to a series of risks, including comprehensive pollution risk, geo-accumulation risk, potential ecological risk, and the carcinogenic and non-carcinogenic risk. Positive matrix factorization (PMF) source analysis combining with the geographic distribution of heavy metal surrounding pharmaceutical manufacturing confirmed that there were three main heavy metal pollution sources, including pharmaceutical wastewater, traffic, and agricultural chemicals, which had the 52.37%, 16.49%, and 31.14% contributions to the surrounding agricultural soil. The present study provided systematic strategies of environment risk assessment and source apportionment, and can be referred for casual analysis and prevention strategies for farmland soil surrounding pharmaceutical manufacturing complex.
1 Introduction
The heavy metal pollution in soil has been causing great risk for public health. National survey report of China’s soil environmental quality in 2014 showed that 16.1% of total soil samples and 19.4% of agricultural soils were polluted by heavy metals, particularly for Cd, Pb, As, Hg, Cr, with over-standard rates of 7.0%, 1.5%, 2.7%, 1.6%, 1.1%, respectively (MEE, 2014). China, with a large population base, has created a huge demand for public medical care (Hu et al., 2019). Pharmaceutical companies scattered throughout China that produce massive amounts of industrial by-products such as wastewater, gas, and residues. Just as previous reported, heavy metals, such as cadmium (Cd) and mercury (Hg), are key potential typical pollutants of the pharmaceutical industry (Wang et al., 2019; Yang et al., 2020; Luo et al., 2021; Duan et al., 2022). Therefore, pharmaceutical manufacturing complex might have potential pollution risk surrounding agricultural soil. Although the emission of industrial by-products has been regarded as the major potential sources of heavy metal pollution in agricultural soil, the potential risk of heavy metal pollution for surrounding agricultural soil during pharmaceutical production has not been systematically explored.
The assessment approaches such as single factors, the Nemerow integrated pollution index, geo-accumulation index, potential ecological risk, and human health risk are commonly employed to evaluate the risk of heavy metal pollution in soil (Kang et al., 2020; Li et al., 2014; Ren et al., 2021; Chen et al., 2022). The Nemerow integrated pollution index reflects the combined pollutive effects of various heavy metals, while the geo-accumulation index reflects the risk of heavy metal accumulation in soil. Moreover, the potential ecological risk assessment determines the ecological risk of heavy metal pollution in soil, and the human health risk assessment focuses on risk to human health caused by heavy metals pollution in soil (Guston et al., 2014; Health, U.S.E.P.A.O.o. and Group, E.A.E.A., 1989; Muller, 1979; Nemerow, 1974; Zhou et al., 2022; Chen et al., 2021). However, the systematic risk of heavy metals cannot be effectively reflected only by referring to a single risk assessment method described above, it is essential to integrate these approaches for evaluating the environmental risk of heavy metals.
Heavy metal pollution risk assessment describes the current status of heavy metal pollution in soil, and pollution source analysis is regarded as necessary to identify and quantify heavy metal pollution sources. Due to the limited data about heavy metal pollution emission sources in China and the difficulty of accurately describing heavy metal migration, the receptor model of pollutants has been widely applied to source analyses of heavy metals in soil because it does not require either information about the composition of various pollution sources or specification of how emission factors are transported (Li et al., 2014; Huang et al., 2018). The reportedly main source analytical methods of the receptor model included chemical mass balance (CMB), positive matrix factorization (PMF), and the UNIX model. Among them, PMF was the most frequently applied to analyze sources of heavy metal pollution in agricultural soil (Lee et al., 2016; Jiang et al., 2017; Liang et al., 2017). In order to fully understand the current pollution level and source of heavy metal in agricultural soil surrounding pharmaceutical manufacturing complexes, it is essential to perform systematic assessment and source analysis in agricultural soil around pharmaceutical manufacturing complexes.
Here, we analyzed the heavy metal contents of soil samples in pharmaceutical manufacturing complexes and its surrounding farmland soil in Hubei Province, China. The main objectives were to determine the accumulation risk and potential ecological risk of heavy metal, characterize the human health risk, and identify the potential sources of heavy metal pollution. We explored the relationship between heavy metal pollution in pharmaceutical manufacturing complexes and its surrounding agricultural soil, and assessed the potential risk of heavy metal pollution to agricultural soil caused by a typical pharmaceutical manufacturing complex. The findings of this study aimed to provide a reference methodology for risk assessment and pollution sources identification of heavy metal in agricultural soil surrounding typical pharmaceutical manufacturing complexes.
2 Materials and methods
2.1 Survey area information and soil sampling
A typical pharmaceutical complex in Hubei Province established in 2009 mainly produced chemical intermediates and active pharmaceutical ingredients (APIs). Due to the poor management of production during the early stage and the lack of environmental monitoring, industrial wastewater and waste residue generated by the complex were not appropriately treated. Surface soil in some areas existed heavy metal pollution. The pharmaceutical plant covers an area of ∼188,304 m2 surrounded by ∼ 680,000 m2 of southwest plain glacial lake tidal soil, where rice paddy fields are irrigated with water from a local river.
In this study, 554 soil samples with different soil depth were collected from 157 soil sites in pharmaceutical complex (Figure 1). For detailed investigation, 40 m × 40 m grid points were used for the soil exceedance areaof preliminary investigation, and 20 m × 20 m grid points were used for the isolated exceedance points and abnormal depths in the results. 38 soil samples were collected from 0 to 20 cm surface layer in agricultural land. The soil samples were air-dried, sieved, and stored at temperature lower than 4°C.
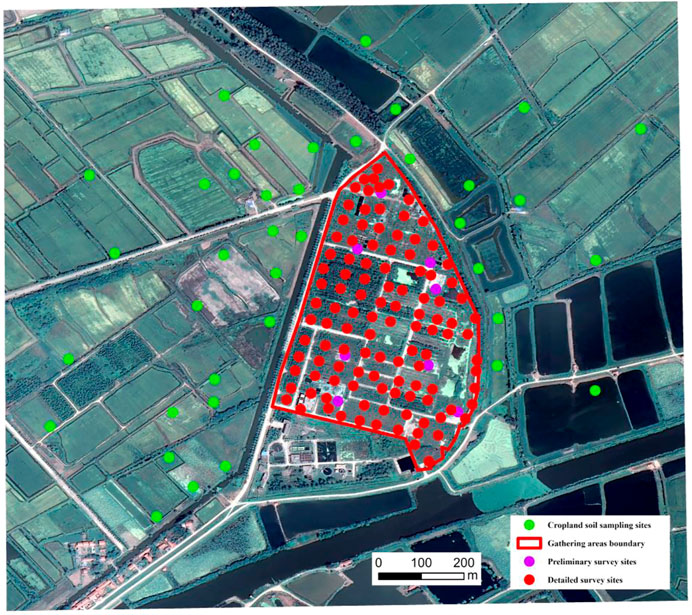
FIGURE 1. Distribution of sampling points within pharmaceutical complex and surrounding agricultural soil.
2.2 Soil physiochemical properties analysis
The pH was determined at a soil-water ratio of 1:2.5. Soil samples were digested with nitric-hydrochloride-hydrofluoric acid in a microwave digestion system. Cd and Pb were determined by graphite furnace atomic absorption spectrophotometry. As was determined by inductively coupled plasma mass spectrometry, and Hg was determined by atomic fluorescence spectroscopy. Analysis methods and detection limit of heavy metals are shown in Table 1. To ensure the quality assurance and quality control (QA/QC), certified reference materials and blank samples were included.
2.3 Soil environmental risk assessment
2.3.1 Nemerow integrated multi-factor pollution index
The Nemerow integrated pollution index of multiple heavy metals (Nemerow, 1974) is calculated as follows:
where,
2.3.2 Geo-accumulation index
The impact of human activity on the environment is identified by the geo-accumulation index with reference to the natural variability of heavy metals (Müller, 1979) calculated as:
where, Igeo is the geo-accumulation index of heavy metal n, Cn is the concentration of heavy metal n in soil, Bn is the background value of heavy metal according to local soil parent material conditions, and K is a coefficient reflecting fluctuating changes for the background value due to differences in parent material across regions. The geo-accumulation index was divided into seven levels of pollution from none to extreme (Supplementary Table S2).
2.3.3 Potential ecological risk assessment
The potential ecological risk index was proposed in 1980 to integrate the potential ecological impact of heavy metals (Hakanson, 1980). It considers the type, toxic effects, concentration, and sensitivity of aquatic organisms to heavy metal pollution and is calculated as:
where RI is the comprehensive potential ecological risk index,
The intensity of pollution and the magnitude of toxicity are indicated by RI values. Supplementary Table S3 shows the classification criteria for
2.3.4 Assessment of human health risk
We analyzed human non-carcinogenic risk and carcinogenic risk (CR) using the United States Environmental Protection Agency (USEPA)-recommended health risk assessment model (USEPA, 2011; Zhou et al., 2022). The non-carcinogenic hazard quotient and total carcinogenic risk (TCR) were calculated in sensitive populations grouped based on behavioral and physiological differences. Daily intake was calculated using the following equations:
Values >1 for HQ and HI indicates that a population is at potential non-carcinogenic risk. Values for CR or TCR of > 1E-04, 1E-04‒1E-06, or < 1E-06 represents carcinogenic, acceptable, and negligible risk to human health, respectively. Supplementary Table S4 shows the values and parameters involved in the equations, and Supplementary Table S5 lists the reference dose (RfD) for chronic disease and slope factors.
2.4 Source apportionment
The major sources of heavy metal pollution and their contributions were identified and quantified using the PMF model based on a weighted least squares iterative calculation with non-negative constraints on the decomposition matrix and standard deviation (SD) for optimization (Norris et al., 2014; Liu et al., 2022). The PMF decomposed the original matrix into three parts by continuously decomposing receptor matrix X to obtain optimal factor scores and loadings of matrices G and F. The criterion for determining the optimal was the minimal value of the objective function Q, which can be calculated as:
Where Xij is the content of element j in sample i (mg/kg), gik is the contribution of source factor k to sample i, fkj is the content of element j in factor k, eij is the residual for every sample, p is the number of source factors, and Uij is the uncertainty of element j in sample i; MDL is the detection limit, and the error fraction is the ratio (%) of measurement uncertainty. The uncertainty of the PMF model was measured using the classical bootstrap (BS) method, and calculations followed the USEPA PMF 5.0 User Guide.
2.5 Statistical analysis
Data were statistically analyzed and diagrams were created in R language. Positive matrix factorization was calculated by EPA PMF version 5.0 (USEPA, Washington, DC, United States). The spatial distribution of HMs in the study area was produced using ArcGIS pro 3.0 (Environmental Systems Research Institute, Redlands, CA, United States).
3 Results and discussion
3.1 Statistical description of heavy metal pollution in soil
Table 2 shows that the mean pH at the surface of surrounding agricultural soil was 6.56 ± 0.96, with a small coefficient of variation (CV). The mean values for Cd, Pb, As, and Hg in surface soil ranged from 0.16 to 2.22, 23.30–524.00, 8.26–6920.00, and 0.17–1.96, respectively, with CVs of 1.04, 1.52, 5.12, and 0.79, respectively. Among them, the mean values for Cd and As exceeded their corresponding background values, which need to be considered as pollutants of concern. Meanwhile, the CVs of Cd, Pb, and As were higher than 100%, indicating that the existence of influence of anthropogenic activities (Fei et al., 2019).
3.2 Soil heavy metal pollution risk assessment
3.2.1 Assessment of single-factor pollution and Nemerow integrated pollution index
The single-factor and Nemerow integrated pollution indexes reflect the single and comprehensive pollution levels of heavy metals, respectively (Figure 2). The single-factor pollution indices for Cd, Pb, As, and Hg ranged from 0.33 to 7.40, 0.16–7.49, 0.33–230.67, and 0.22–3.92 mg kg−1, respectively, and with the exceedance rate of 5.26%, 23.68%, 34.21%, and 3%, respectively. The Nemerow integrated pollution index ranged from 0.52 to 166.89, with 23.68%, 52.63%, 15.79%, and 7.89% of points, which corresponded to clean, relatively clean, light, and heavy pollution, respectively. The spatial distribution of the single-factor pollution index (Figure 2) indicated a wide distribution of Cd and As pollution, whereas that of Pb and Hg pollution existed lesser extent. Based on the distribution of heavy metals distant from potential pollution sources, the pollution levels of all four heavy metals were closely associated with the pharmaceutical complex. The superimposed Cd and As pollution made Nemerow pollution index a higher level, which was also closely correlated with distance from the pharmaceutical complex (Figure 3). Comprehensively speaking, a pond in the southwest section of the complex was a hotspot for heavy metal pollution. Remarkably, except for Cd and Hg (Wang et al., 2019; Yang et al., 2020), Pb and As were also observed to be the concerned potential pollutant in this study, which were obviously different from previous reports.
3.2.2 Geo-accumulation assessment
The geo-accumulation index reflects the extent of heavy metal pollution in soil by external inputs (Figure 4). As shown in Figure 4, The geo-accumulation indices for Cd, Pb, As, and Hg ranged from −0.69 to 3.11, −0.78 to 3.71, −1.16 to 8.55, and 0.51 to 4.03, respectively, and their corresponding ratios of polluted points were 47.37, 47.37, 78.95, and 100%, respectively. The geo-accumulation indices of heavy metals showed obvious accumulation risk of Cd, Pb, As, and Hg. Among them, Cd and As accumulation were obviously influenced by the distance from the pharmaceutical complex (Figure 4). Hg as a potential pollutant byproduct of pharmaceutical manufacturing had been proven in previous report (Wang et al., 2019), which was also found herein around the sewage ponds. However, in this study, it was found to have no obvious pollution risk of pharmaceutical Hg byproducts to the surrounding agricultural soil.
3.2.3 Potential ecological risk assessment
The potential ecological risk index (EI) reflects the potential hazard level of heavy metal pollution to soil ecology (Figure 5). The EI of Cd, Pb, As, and Hg ranged from 10 to 222, 0.78 to 37.43, 3.30 to 2,306.67, and 8.76 to 156.80, respectively, and their corresponding ratios (%) of points existing ecological risk were 10.53, 0, 7.89, and 2.63%, respectively. These findings indicated that the accumulation of all four heavy metals might pose a risk to the regional ecosystem (Xiao et al., 2019). Figure 6 shows that Pb and Hg confer potential ecological risk only in the hotspot area, whereas Cd and As have further spillover ecological risk. The geographic distribution of potential ecological risk RI was consistent with the EIs for Cd and As.
3.2.4 Human health risk assessment
The human health risk assessment model recommended by USEPA was applied to assess the non-carcinogenic and carcinogenic risk of heavy metals for children and adults. Table 3 shows that the hazard quotient (HQ) of As was higher than 1, indicating that it existed non-carcinogenic risk for both children and adults, which caused the total non-carcinogenic Hazard Index (HI) value higher than 1. The analysis of carcinogenic risk (CR) revealed that the CR values of both Cd and As were higher than 1 × 10–6, indicating that these heavy metals had carcinogenic risk. The total carcinogenic risk (TCR) caused by the Cd and As was higher than 1 × 10–6, while As contribution was predominant. We found that the carcinogenic and non-carcinogenic risk of potentially polluted soils was higher for children than for adults (Table 3), which was agreement with previous literature (Zhou et al., 2022). Human health was reported to be more sensitive to As and Cd (Yang et al., 2019). In this study, Cd and As were identified as the main pollution surrounding pharmaceutical complex, which were also observed to be the sensitivity of human health to As and Cd pollution.
3.3 Source analysis of heavy metal pollution in soil
In this study, the main sources of heavy metal pollution were identified and quantified by combining the spatial distribution of heavy metal concentrations in soil of pharmaceutical manufacturing complex and surrounding agricultural soil using the PMF source analysis. The spatial distribution of heavy metals in agricultural soil was closely associated with the pharmaceutical complex (Figure 7). In addition to the hotspot pond on the southwest of the complex, the risk of Cd and As pollution were significantly related with the pharmaceutical complex. Figure 8 shows that the heavy metals were mainly from three pollution sources. The loading of pollution source 1 mainly contained heavy metals of As, Pb, and Cd, while that of pollution source 2 were Hg, Pb, and Cd. The loading of pollution source 3 mainly included Cd, Pb, As, and Hg. Arsenic was mainly from sources 1 and 3, while Hg was from in sources 2 and 3. Remarkably, Pb and Cd were from all the three sources. Considering the overall contribution of the polluted sources in the study area, sources 1, 2, and 3 contributed 52.37%, 31.14%, and 16.49%, respectively. The composition of heavy metal loadings and the geographic distribution of the pollution sources (Figure 9)indicated that source 1 corresponded to irrigation with pharmaceutical wastewater, while source 2 distribution overlapped with road networks and consists of pollution loadings of Hg, Pb, and Cd, which was presumed as traffic pollution (Chai et al., 2021; Yin et al., 2021). Although source 3 had no direct relationship with the geographic distribution of the pharmaceutical plant cluster, it still contributed to all four heavy metals, which was considered to come from the use of pesticides (Hu et al., 2018).
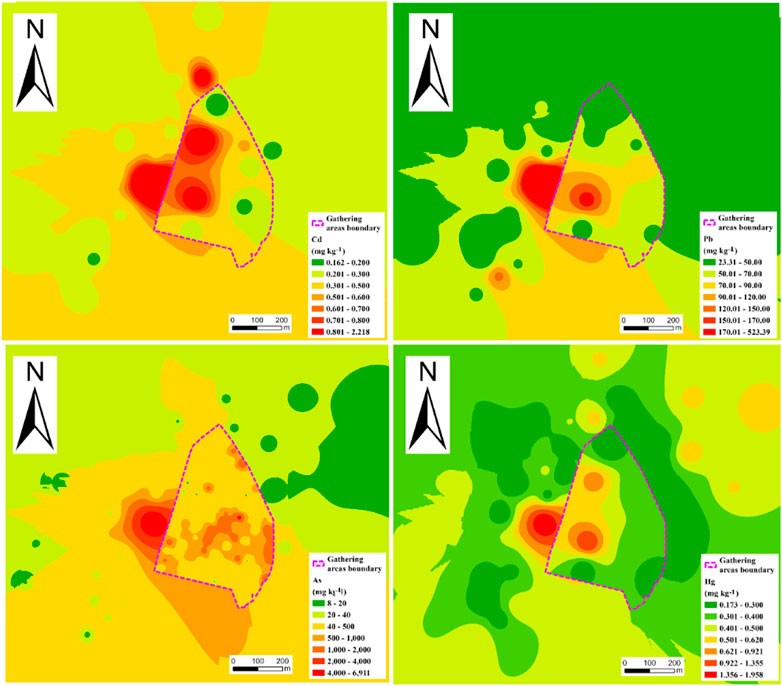
FIGURE 7. Distribution of heavy metal concentrations in pharmaceutical plant cluster and surrounding farmland.
4 Conclusion
In summary, the environmental risk and source apportionment in agricultural soil around a typical pharmaceutical manufacturing complex in Hubei Province were comprehensively assessed. The heavy metals of Cd, Pb, As, and Hg were found to be the potential pollutants in manufacturing complex and had pollution risk for surrounding agricultural soil, especially for Cd and As. PMF source analysis revealed that there were three pollution sources including irrigation with pharmaceutical wastewater, traffic, and agricultural chemicals such as pesticides and fertilizers. We combined environmental risk assessment with source analysis to determine the details of soil pollution within and around a defunct pharmaceutical manufacturing complex. Our study provided a reference for analyzing the causes of heavy metal contamination of agricultural soil associated with pharmaceutical manufacturing, which also helped to develop strategies to prevent and control pollution caused by heavy metal by-products of the pharmaceutical industry.
4.1 Suggestions for managing risks
Relevant departments should organize to carry out risk control of soil pollution promptly. Local government should strengthen source management and further establish farmland irrigation management system to guide farmers not to use sewage for irrigation. It is recommended to track the quality of agricultural products through in-depth investigation and routine monitoring of agricultural quality around the pharmaceutical manufacturing complex.
Data availability statement
The raw data supporting the conclusion of this article will be made available by the authors, without undue reservation.
Author contributions
All authors listed have made a substantial, direct, and intellectual contribution to the work and approved it for publication.
Funding
This work was jointly supported by the National Key Research and Development Program of China (No. 2019YFC1804003), the Environmental Protection Scientific Research Project of Hubei Province (2020) and Knowledge Innovation Program of Wuhan-Basic Research.
Conflict of interest
The authors declare that the research was conducted in the absence of any commercial or financial relationships that could be construed as a potential conflict of interest.
Publisher’s note
All claims expressed in this article are solely those of the authors and do not necessarily represent those of their affiliated organizations, or those of the publisher, the editors and the reviewers. Any product that may be evaluated in this article, or claim that may be made by its manufacturer, is not guaranteed or endorsed by the publisher.
Supplementary material
The Supplementary Material for this article can be found online at: https://www.frontiersin.org/articles/10.3389/fenvs.2022.1105910/full#supplementary-material
References
Chai, L., Wang, Y., Wang, X., Ma, L., Cheng, Z., Su, L., et al. (2021). Quantitative source apportionment of heavy metals in cultivated soil and associated model uncertainty. Ecotoxicol. Environ. Saf. 215, 112150. doi:10.1016/j.ecoenv.2021.112150
Chen, L., Wang, J., Beiyuan, J., Guo, X., Wu, H., and Fang, L. (2021). Environmental and health risk assessment of potentially toxic trace elements in soils near uranium (U) mines: A global met a-analysis. Sci. Total Environ. 816, 151556. doi:10.1016/j.scitotenv.2021.151556
Chen, L., Zhou, M., Wang, J., Zhang, z., Duan, C., Wang, X., et al. (2022). A global met a- analysis of heavy metal (loid)s pollution insoils near copper mines:Evaluation of pollution level and probabilistic health risks. Sci. Tot al Environ. 835, 155441–1. doi:10.1016/j.scitotenv.2022.155441
Chinese soil element background valueCNEMC (1990). Background values of elements in soils of China. Beijing, People's Republic of China: China Environmental Science and Technology Press.
Duan, C., Wang, Y., Wang, Q., Ju, W., Zhang, Z., Cui, Y., et al. (2022). Microbial metabolic limitation of rhizosphere under heavy metal stress: Evidence from soil ecoenzymatic stoichiometry. Environ. Pollut. 300, 118978. doi:10.1016/j.envpol.2022.118978
Fei, X., Christakos, G., Xiao, R., Ren, Z., Liu, Y., and Lv, X. (2019). Improved heavy metal mapping and pollution source apportionment in Shanghai City soils using auxiliary information. Sci. Total Environ. 661, 168–177. doi:10.1016/j.scitotenv.2019.01.149
Guston, D. H., Fisher, E., Grunwald, A., Owen, R., Swierstra, T., and Van der Burg, S. (2014). Responsible innovation: Motivations for a new journal. Taylor & Francis, 1–8.
Hakanson, L. (1980). An ecological risk index for aquatic pollution control. A sedimentological approach. Water Res. 14, 975–1001. doi:10.1016/0043-1354(80)90143-8
Health, U.S.E.P.A.O.o.Group, E.A.E.A. (1989). Exposure factors handbook. Washington: Office of Health and Environmental Assessment, US Environmental Protection.
Hu, L., Yu, Z., Yuan, Q., Hu, Y., and Ung, C. O. L. (2019). Opportunities and challenges of multinational pharmaceutical enterprises in transforming pharmaceutical market in China. Ther. Innovation Regul. Sci. 53, 207–214. doi:10.1177/2168479018772820
Hu, W., Wang, H., Dong, L., Huang, B., Borggaard, O. K., Hansen, H. C. B., et al. (2018). Source identification of heavy metals in peri-urban agricultural soils of southeast China: An integrated approach. Environ. Pollut. 237, 650–661. doi:10.1016/j.envpol.2018.02.070
Huang, Y., Deng, M., Wu, S., Japenga, J., Li, T., Yang, X., et al. (2018). A modified receptor model for source apportionment of heavy metal pollution in soil. J. Hazard. Mater. 354, 161–169. doi:10.1016/j.jhazmat.2018.05.006
Jiang, Y., Chao, S., Liu, J., Yang, Y., Chen, Y., Zhang, A., et al. (2017). Source apportionment and health risk assessment of heavy metals in soil for a township in Jiangsu Province, China. Chemosphere 168, 1658–1668. doi:10.1016/j.chemosphere.2016.11.088
Kang, Z., Wang, S., Qin, J., Wu, R., and Li, H. (2020). Pollution characteristics and ecological risk assessment of heavy metals in paddy fields of Fujian province, China. Sci. Rep. 10, 12244. doi:10.1038/s41598-020-69165-x
Lee, D. H., Kim, J. H., Mendoza, J. A., Lee, C. H., and Kang, J.-H. (2016). Characterization and source identification of pollutants in runoff from a mixed land use watershed using ordination analyses. Environ. Sci. Pollut. Res. 23, 9774–9790. doi:10.1007/s11356-016-6155-x
Li, Z., Ma, Z., van der Kuijp, T. J., Yuan, Z., and Huang, L. (2014). A review of soil heavy metal pollution from mines in China: Pollution and health risk assessment. Sci. Total Environ. 468, 843–853. doi:10.1016/j.scitotenv.2013.08.090
Liang, J., Feng, C., Zeng, G., Gao, X., Zhong, M., Li, X., et al. (2017). Spatial distribution and source identification of heavy metals in surface soils in a typical coal mine city, Lianyuan, China. Environ. Pollut. 225, 681–690. doi:10.1016/j.envpol.2017.03.057
Liu, Z., Xu, C., Johnson, A. C., Sun, X., Ding, X., Ding, D., et al. (2022). Source apportionment and crop bioaccumulation of perfluoroalkyl acids and novel alternatives in an industrial-intensive region with fluorochemical production, China: Health implications for human exposure. J. Hazard. Mater. 423, 127019. doi:10.1016/j.jhazmat.2021.127019
Luo, L., Wang, B., Jiang, J., Fitzgerald, M., Huang, Q., Yu, Z., et al. (2021). Heavy metal contaminations in herbal medicines: Determination, comprehensive risk assessments, and solutions. Front. Pharmacol. 11, 595335. doi:10.3389/fphar.2020.595335
MEEPRC (2018). Risk control standard for soil contamination of agricultural land. Beijing, China: Standards Press of China Beijing.
Muller, G. (1979). Schwermetalle in den sedimenten des Rheins-Veranderungen seit. Umschav 79, 133–149.
Norris, G., Duvall, R., Brown, S., and Bai, S. (2014). EPA positive matrix factorization (PMF) 5.0 fundamentals and user guide. Washington: US Environmental Protection Agency, 1–136. EPA/600/R-14/108.
Ren, Y., Lin, M., Liu, Q., Zhang, Z., Fei, X., Xiao, R., et al. (2021). Contamination assessment, health risk evaluation, and source identification of heavy metals in the soil-rice system of typical agricultural regions on the southeast coast of China. Environ. Sci. Pollut. Res. 28, 12870–12880. doi:10.1007/s11356-020-11229-6
Wang, S., Cai, L.-M., Wen, H.-H., Luo, J., Wang, Q.-S., and Liu, X. (2019). Spatial distribution and source apportionment of heavy metals in soil from a typical county-level city of Guangdong Province, China. Sci. Total Environ. 655, 92–101. doi:10.1016/j.scitotenv.2018.11.244
Xiao, R., Guo, D., Ali, A., Mi, S., Liu, T., Ren, C., et al. (2019). Accumulation, ecological-health risks assessment, and source apportionment of heavy metals in paddy soils: A case study in hanzhong, shaanxi, China. Environ. Pollut. 248, 349–357. doi:10.1016/j.envpol.2019.02.045
Yang, S., Qu, Y., Ma, J., Liu, L., Wu, H., Liu, Q., et al. (2020). Comparison of the concentrations, sources, and distributions of heavy metal (loid) s in agricultural soils of two provinces in the Yangtze River Delta, China. Environ. Pollut. 264, 114688. doi:10.1016/j.envpol.2020.114688
Yang, S., Zhao, J., Chang, S. X., Collins, C., Xu, J., and Liu, X. (2019). Status assessment and probabilistic health risk modeling of metals accumulation in agriculture soils across China: A synthesis. Environ. Int. 128, 165–174. doi:10.1016/j.envint.2019.04.044
Yi, Y., Yang, Z., and Zhang, S. (2011). Ecological risk assessment of heavy metals in sediment and human health risk assessment of heavy metals in fishes in the middle and lower reaches of the Yangtze River basin. Environ. Pollut. 159, 2575–2585. doi:10.1016/j.envpol.2011.06.011
Yin, G., Zhu, H., Chen, Z., Su, C., He, Z., Chen, X., et al. (2021). Spatial distribution and source apportionment of soil heavy metals in Pearl River Delta, China. Sustainability 13, 9651. doi:10.3390/su13179651
Zhou, Y., Jiang, D., Ding, D., Wu, Y., Wei, J., Kong, L., et al. (2022). Ecological-health risks assessment and source apportionment of heavy metals in agricultural soils around a super-sized lead-zinc smelter with a long production history, in China. Environ. Pollut. 307, 119487. doi:10.1016/j.envpol.2022.119487
Keywords: heavy metal, soil, pharmaceutical manufacturing, risk assessment, source apportionment analysis
Citation: Su Q, Zhang X, Zhang Y, Sun G, Li Z, Xiang L and Cai J (2023) Risk assessment of heavy metal pollution in agricultural soil surrounding a typical pharmaceutical manufacturing complex. Front. Environ. Sci. 10:1105910. doi: 10.3389/fenvs.2022.1105910
Received: 23 November 2022; Accepted: 09 December 2022;
Published: 05 January 2023.
Edited by:
Ling Xia, Wuhan University of Technology, ChinaReviewed by:
Jingtao Hou, Huazhong Agricultural University, ChinaLinchuan Fang, Northwest A&F University, China
Copyright © 2023 Su, Zhang, Zhang, Sun, Li, Xiang and Cai. This is an open-access article distributed under the terms of the Creative Commons Attribution License (CC BY). The use, distribution or reproduction in other forums is permitted, provided the original author(s) and the copyright owner(s) are credited and that the original publication in this journal is cited, in accordance with accepted academic practice. No use, distribution or reproduction is permitted which does not comply with these terms.
*Correspondence: Luojing Xiang, eGxqMTk2NTE3MTdAMTYzLmNvbQ==; Junxiong Cai, Y2p4aGJoamt4QDE2My5jb20=