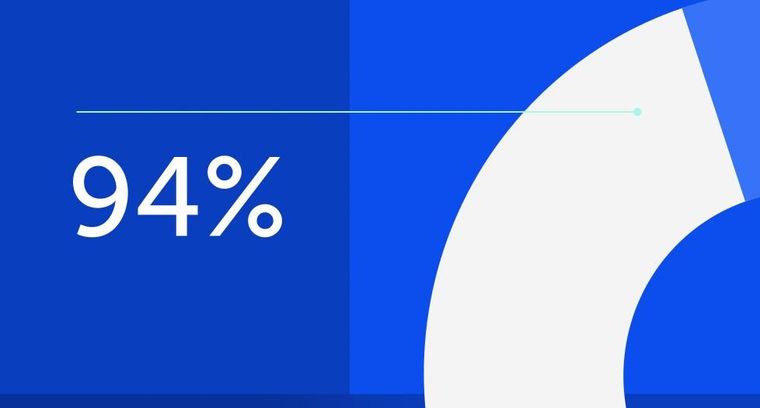
94% of researchers rate our articles as excellent or good
Learn more about the work of our research integrity team to safeguard the quality of each article we publish.
Find out more
ORIGINAL RESEARCH article
Front. Environ. Sci., 28 November 2022
Sec. Environmental Informatics and Remote Sensing
Volume 10 - 2022 | https://doi.org/10.3389/fenvs.2022.1072180
This article is part of the Research TopicHydrology, Water Resources, and Ecosystem Sustainable DevelopmentView all 10 articles
With the deterioration of environmental pollution, resource security and climate crisis, transforming the mode of economic development and developing a green economy have turned into an international consensus. However, environmental regulations (ERs) can help facilitate technological innovation. As an important financial support for the green innovation transformation of China’s manufacturing industry, it is crucial to exploit the policy synergy between green credit (GC) and ERs at the regional level to stimulate technological innovation effects. GC, as a financial instrument, can play a unique role in ERs; therefore, the relationship between ERs and the level of green technology innovation (GTI) based on the GC perspective deserves an in-depth study. Using a spatial Durbin model (SDM) for the panel data of 30 Chinese provinces from 2006 to 2016, this paper explores the spatial effects of ERs and GC on GTI in manufacturing and the moderating effects of GC policies on ERs affecting GTI. The research finds that ERs exert a negative impact on local manufacturing GTIs and undermine the innovation effect in neighboring locations through spatial spillover effects. The development of GC helps stimulate the transformation of GTI in local manufacturing industries with further attention on the effects of policy instruments and their combinations. The moderating effect of GC suggests that its development can weaken the inhibiting effect of ERs on GTI in local and neighboring areas and is particularly significant in coastal areas. Our study provides a theoretical basis and policy insights for coordinating government external intervention and market operation laws at the regional level to bring into play the incentive effect of technological innovation.
China’s industrial supply capacity has grown to the point of being able to support economic development (Bressanelli et al., 2019; Bressanelli et al., 2022). However, the development model at the expense of the ecological environment at the expense of the ills of increasingly prominent institutional barriers, technical factor shortcomings, and phase conversion resistance is still accumulating and has become a real obstacle to the process of green and sustainable development (Geng et al., 2022; Jayachandran, 2022). The new situation of a tight time window for achieving carbon peaking and carbon neutrality presents high requirements for China’s industrial low-carbon transformation and green development, and realizing the coordinated development of industrial transformation and ecological civilization has become a major strategic issue (Kolkiş et al., 2020; Zhao et al., 2021; Zhao et al., 2022). The 19th National Congress report of the Communist Party of China proposed the building of a market-oriented green technology innovation (GTI) system, focusing on the change from factor inputs to green system change and technology innovation-driven, from the scale advantage to innovation development advantage of the development path, has become an important support to achieve industrial green transformation (de Oliveira et al., 2018; Li and Gao, 2022). It can be observed that green technology progress has become the optimal means to achieve a “win-win” for environmental pollution control and regional economic growth.
As the core driving force and important support for green development transformation, GTI combines the concepts of “green” and “innovation,” updating product processes and market services through technological innovation to reduce pollution emissions, improve resource utilization efficiency (Lv et al., 2021; Suki et al., 2022), and directly optimize the green industrial structure to promote regional green development transformation (Behera and Sethi, 2022). Environmental regulation (ERs) policy is a common way to stimulate enterprises to engage in GTI, and it plays a significant role in the pollution treatment cost expenditure of the front-end support of enterprises and the pollution emission of the end-end regulation of enterprises (Hassan et al., 2022). Green credit (GC) is an important instrument of green finance and a market-based instrument included in the broad ERs. GC accounts for over 90% of the existing green financial instruments in China and effectively promotes GTI by enterprises (Zhang et al., 2022).
Compared with traditional non-clean technology areas, the profit advantage of GTI is relatively weak (Shen et al., 2021); relying on only market forces is insufficient to support the transformation of China’s industrial economy into green technology-oriented innovation (Wei et al., 2020). There is an urgent need for the government to effectively implement ecological, green, and scientific environmental economic policies and regulatory instruments. According to the “Porter hypothesis”, the role of ERs in promoting innovation is constrained by the financial status of the enterprises (Liu et al., 2021). The micro-subjects of regional green technology innovation are enterprises, and enterprises require continuous and stable financial support to conduct GTI. If ERs are strong in a certain region, it leads to an increase in the demand for funds for pollution control and innovation investment, which may cause polluting enterprises to adopt the avoidance strategy of relocating nearby. This leads to a significant difference in the technological innovation effect of ERs between local and neighboring regions (Mbanyele and Wang, 2022), resulting in the phenomenon of “ERs failure” at the regional level (Zhong and Peng, 2022). Therefore, green financial support is the key for the government to fully play its role in ERs.
ERs are implemented by the state and targeted at individual or organizational enterprises. It has been widely used as a traditional tool for environmental protection and is a key external driver of GTI, but its incentive effect on micro-individual technology innovation remains controversial (Böcher, 2012; Karmaker et al., 2021; Zhao et al., 2022).
Relevant studies have shown that green finance, with economic and environmental benefits, has become an emerging environmental governance tool for achieving market-oriented GTI (Liu et al., 2017; Irfan et al., 2022; Sharif et al., 2022). However, under the constraints of ERs, the potential for green finance to provide financial support for enterprises to engage in GTI activities to alleviate the contradiction between environmental protection and economic development requires in-depth exploration. First, the relationship between ERs and technological innovation exhibits an inverted “U" curve; when the intensity of ERs is weak, it promotes technological innovation activities. When the intensity of ERs exceeds a certain threshold, ERs restrict technological innovation, i.e., it is difficult for innovation compensation to cover the cost of compliance (Zhang and Wei, 2014). The interregional gradient in the intensity of the enforcement of ERs in China has led to regions with more lenient environmental policies, thereby providing opportunities for neighboring firms to circumvent the high-cost business practices of pollution abatement and technological innovation (Zhang et al., 2022). Second, as a new environmental economic policy, the essence of GC policy is that commercial banks provide differentiated loan pricing and credit lines for energy-saving, clean production, and environmental enterprises with high energy consumption and high pollution (Soundarrajan and Vivek, 2016; Su et al., 2022; Wang et al., 2022), which can effectively alleviate the financial dilemma of GTI in regional manufacturing industries (Nabeeh et al., 2021). On the one hand, green credit compresses the financing space of highly polluting enterprises and increases the financing cost, forcing enterprises to carry out methods of technological innovation and transformation of production. On the other hand, green credit provides a wider range of external financing channels for polluting enterprises to achieve green innovation and transformation, which can effectively relieve the financial difficulties of green technology innovation in regional manufacturing industries. However, it is difficult to rationally allocate the limited credit funds among different regions based on the principle of parity. This results in a large difference in the level of GC development on the impact of GTI in local and neighboring regions. Third, in the field of environmental policy, government mechanisms have long dominated ecological and environmental governance, while the constraints of financial scarcity and government failure have hindered government mechanisms from matching the demand for ecological and environmental improvement. However, as the ecological environment is a quasi-public good, green finance, as a new market-based instrument for ecological governance, can exert a multiplier effect in a more effective, equitable, and sustainable manner when coordinated with the traditional environmental regulatory system (Falcone, 2020). Finally, geographically, there are differences between coastal and inland regions in terms of economic development level, government control, regional ecological environment, and financial market development, which leads to interregional differences in ERs and GC for GTI in manufacturing. On the one hand, it lies in the fact that ERs instruments in coastal areas are more effective in generating “innovation compensation” than those in inland areas (Ren et al., 2018; Nie et al., 2022). On the other hand, the incentive-matching effect of GC and ERs is more pronounced in coastal areas than that in inland areas, which exerts a positive moderating effect on GTI (He and Yan, 2020).
There is spatial variability in the impact of ERs intensity on GC. When ERs are weak, the implementation of GC can effectively guide enterprises to develop GTI by limiting financing to “three high” enterprises. When ERs are strong, i.e., when the cost of green technology research and development and the difficulty of financing seriously hinders the green transformation of enterprises, more capital supply in GC is used to solve part of the financing loan problem, thereby effectively promoting the GTI of enterprises. However, existing studies on GTI are mostly from a single perspective of ERs and GC, and fail to analyze their synergistic effects and spatial spillover effects. To fill this gap, this paper expands the spatial analysis framework of GTI by verifying the synergistic effect of GC and ERs on GTI and its regional differences. In terms of research content, this paper explores the synergies between green credit as a new environmental governance tool and traditional environmental regulation instruments on GTI in manufacturing. In terms of study dimensions, heterogeneity analysis is enriched by grouping by region.
Based on this, the key questions that our study addresses are: 1) Is there a local effect or spatial spillover effect of GC on the GTI of enterprises? 2) In terms of interregional equity, is there a crowding-out effect on GTI in neighboring regions due to a shortage of financial resources and competition from local governments? 3) As a new type of environmental governance tool, how will the integration of GC into an integrated framework of ERs and GTI create synergies with traditional ERs instruments? Therefore, our study examined 30 provinces (including municipalities and autonomous regions) in China (excluding Hong Kong, Macao, Taiwan, and the Tibet Autonomous Region) as the research sample, employed a spatial econometric model to explore the effects of ERs and GC on the role of GTI in manufacturing, and used a moderating effect model to test the role of GC in the process of ERs affecting manufacturing technology innovation (Figure 1). Based on the aforementioned theoretical analysis, this paper proposes the following hypotheses:
To investigate the spatial spillover effects of ERs and GC, we employed spatial econometric empirical analysis. The spatial Durbin model (SDM) based on panel data can effectively solve the possible endogeneity problem of interregional ERs variables. Drawing on the existing scholarly works (Ni et al., 2020), the model is constructed as shown in Model (1).
To test the moderating effect of GC on ERs and GTI in manufacturing in Hypothesis 3, we constructed spatial econometric models with moderating effects for the extended analysis in this paper. The model is constructed as shown in Model (2).
where i is a province (i = 1, 2, ... 30); t is the year (t = 2006, 2007, ... 2016); GTIit is the GTI intensity; ERsit is the ERs intensity; GCit is GC; ERsit*GCit is the cross term of GC and ERs; Xit is a control variable, including urbanization level (URB), innovation human resource input (HRI), and fiscal decentralization (FE); and εit is an unpredictable error term.
The spatial econometric model reflects the spatial relationship between economic variables by setting a spatial weight matrix, and different spatial weight matrices represent different forms of spatial distances between variables, reflecting the different ways of influencing regional spatial effects (Zhang et al., 2020). Here, we selected the spatial adjacency matrix and the economic distance spatial weight matrix.
1) Spatial adjacency matrix. The most commonly used spatial weight matrix is the spatial adjacency matrix. According to Ansenlin and Griffith (Anselin and Griffith, 2010), a 0–1 weight matrix was constructed for the spatial adjacency of 30 provinces (autonomous regions and municipalities directly under the central government) in China as follows, where the matrix was assigned the value of one when two regions are adjacent and 0 when two regions were not adjacent. The calculation formula is shown in Model (3).
2) Economic distance matrix. In addition to considering the influence of geographical distance on economic variables, economic distance is an important factor in portraying regional economic differences. The weight calculation formula is shown below.
where
The sample interval was 2006–2016, and the paper covered 30 provinces (including municipalities directly under the Central Government and autonomous regions) (excluding data from Hong Kong, Macao, Taiwan, and Tibet Autonomous Region). Definition of Main Variables and their data sources were related to the statistical yearbooks of previous years (Table 1).
(1)GTI. Since the number of green invention patent applications (GIPA) can be time-sensitive to directly examine green technology innovation activities of enterprises, GIPA was adopted as the main characterization indicator of GTI (Bai et al., 2019). Specifically, according to the “Green List of International Patent Classifications” launched in 2010, we used patent classification numbers to search for GIPAs and calculate the total number of green patent applications per year by region.
(2)GC. Considering that interest expense can reflect the size of credit, the interest expense ratio of non-six energy-consuming industries was chosen to indirectly measure the degree of GC development according to the research method of Guo et al. (2019) and Jiang et al. (2020), where the amount of green credit is the interest expenses of industrial industries in each province total interest expenditure minus the six major energy-consuming industries interest expenditure.
(3)ERs. Most previous studies measured the intensity of ERs from two perspectives: environmental inputs (pollution control investment, government environmental fiscal expenditure, and abatement costs) and environmental performance (sewage charges, sewage taxes, and disposal rates of pollutants). Considering that economic ERs are more likely to internalize external environmental costs, our study focused on economic ERs and measured the intensity of ERs using a composite index of expenditure and regulatory indicators (Michael, 2012; Chen et al., 2022).
①Expenditure indicators focus on governance inputs, using the ratio of industrial investment in pollution control to industrial value added to measure.
②Regulatory indicators are based on the regulatory strength of governmental departments in implementing ERs system policies. Based on the previous research method (Yang et al., 2008; Guo et al., 2017; Huang et al., 2020), the amount of unit emission fee revenue was used as a regulatory-type index.
Drawing from the research of Peng and Yuan (2018), this study employed the min-max standardization method to calculate the composite index of ERs and set Model 5) for calculation.
where ERsij is the composite index of ERs in the jth province in year i; ERSMij and ERRIij are the proportion of ERs investment amount and the average income of ERs in the jth province in year i, respectively. max(ERSMi) and min(ERSMi) denote the maximum and minimum values of the proportion of ERs investment amount in each province of the country in year i, respectively; max(ERRIi) and min(ERRIi) denote the maximum and minimum values of the average ERs income of each province in the country in year i, respectively.
Here, the SDM is selected as the optimal choice by combining the Lagrange Multiplier (LM) and Wald tests, and the results of the Hausman test are used to select the fixed effects estimation results and set the time fixed model. As shown in Table 2, Models (1)–3) are the results of the maximum likelihood estimation of the SDM with the inclusion of the cross terms of GC, ERs, and GC in turn.
As can be seen from Table 2, ERs hinder GTI in China’s manufacturing industry. The analysis of the coefficient estimates for the explanatory variables shows that the regression coefficient of ERs is significantly negative under the three models, indicating that there is a significant negative relationship between the intensity of ERs and GTI in manufacturing in the region. This implies that all provinces in the country are in the primary stage of cost saving, with weak innovation support, such as technological improvement and optimization of management models. Innovation compensation can hardly compensate for the high production costs caused by ERs, and supporting funds and policies also lead to the effect of industrial GTI transformation being difficult to show. Thus, it is difficult to offset the negative impact of ERs on the crowding-out effect of innovation input.
Second, Table 2 shows that GC policy could play the “Porter effect” and positively promote GTI in China’s manufacturing industry. From the estimated coefficients of the variables in Model (2), the incentive effect of GC for technological innovation can be effectively brought into play in the policy context of green finance booming. The reason may be that, by the type of enterprise, high energy-consuming enterprises such as “two high and one leftover” face financing constraints, which need to be eliminated, or they are prompted to improve the efficiency of GTI and adjust their industrial structure to reduce undesirable output (Hsu et al., 2014). Simultaneously, enterprises in the clean industry or promoting green projects are the first to develop environmentally friendly technologies and products with the financial support of GC and achieve value-added business benefits with the “first-mover advantage” achieved in market competition, thereby generating an “innovation compensation effect.”
We have verified the role of ERs and GC for GTI in manufacturing based on independent perspectives. Next, this paper explores the role of the combination of GC and ERs on GTI from the perspective of coordination and cooperation. Table 2 shows the regression results of ERs and GC. Among them, model 3) contains the regression results of the interaction term with the inclusion of GC and ERs. The main effect and spatial lag coefficients of the interaction term, ERs*GC, are negative, indicating that the combination of GC development and ERs inhibits GTI behavior in manufacturing. These results suggest the negative GC effect as a new type of environmental governance instrument in combination with traditional ERs instruments in terms of driving effects on technological innovation. The reason may be that, after a certain level of ERs intensity, the combination of GC and ERs exerts a greater negative effect on GTI. High-intensity ERs contributed to a rapid increase in production costs for companies in a short time, leading to great social and economic pressure on these companies and hindering the development of GTI. Although GC provides financial support to green enterprises and projects, the interaction term between GC and ERs is negative because GTI is characterized by high risks and long-term lags, and the economic benefits to enterprises are highly uncertain. Therefore, GC does not significantly moderate this negative effect.
The previous section outlines the verification of the applicability of the empirical analysis using time-fixed effects SDM, but we consider possible errors in the spillover effects using point estimation tests, and this section highlights our use of partial differential methods to estimate the direct, indirect, and total effects of ERs and GC on technological innovation (Table 3). First, from the spatial decomposition term, the effect of ERs on local and neighboring GTI is significantly positive, and the technology innovation due to the strengthening of ERs may have had the same promotion effect on other regions (Qu, 2018).
As can be seen from Table 3, there is a positive spillover effect of ERs on GTI in manufacturing in surrounding areas. First, according to the “pollution refuge hypothesis”, if the intensity of ERs in the region increases, polluters will choose to move out of the region because of the rising cost. However, for other regions, the relaxed regulatory policies afford the region a comparative cost advantage, and polluting industries move in. Under the effect of the transfer of polluting industries, the industrial structure of the transferred areas gradually tends toward a lower level. Under the “race to the bottom” effect of local governments, there are ERs policy games and GDP competition in each region. When a certain location implements a more stringent environmental access policy, other regional governments adopt the “race to the bottom” strategy of ERs to achieve GDP growth and attract the inflow of resources by not raising or lowering environmental standards, thereby inhibiting industrial restructuring (Wheeler, 2001). In addition, certain studies have pointed out that in the context of high-quality economic development, the central environmental protection inspectors require the upgrading of ERs policies in each region, and the current competition model between governments is more of a “race to the top” model, where there is a demonstration learning effect when a certain location upgrades their ERs intensity (Holzinger and Sommerer, 2011). However, recently, the central government has abandoned the past “GDP-only” performance appraisal system and fully incorporated green development indicators, which, to an extent, has promoted the GTI process.
Recently, driven by policies such as ecological civilization construction and high-quality urban development, intergovernmental competition in pursuit of mobility elements has gradually weakened, and the concept of green development has increasingly become an important part of the assessment. Technology-intensive industries have become the driving force of regional development and exert a pivotal impact on economic development, government financial growth, the attraction of investment, raising the share of green innovation inputs, and evolving the industrial structure toward cleanliness to an extent. Our model results may provide theoretical implications for designing effective environmental regulatory policies and avoiding inefficiency losses from government intervention.
Second, Table 3 shows that GC exerted significant positive direct effects and negative spillover effects on manufacturing technology innovation. GC development improves the mismatch between the cost and benefit of GTI through the transmission mechanisms of the “financial support effect,” “capital allocation effect,” and “risk diversification effect” and promotes the transformation of the local manufacturing industry into green and clean innovation by achieving technological progress. A significant negative spillover relationship exists between GC and GTI in neighboring regions, mainly because the scarcity property of GC, as a financial resource, leads to an increase in credit funds in the region accompanied by a decrease in credit funds in other regions, which reinforces the status quo of interregional competition for resource elements.
China is a vast country, and there are significant differences in resource endowments, economic development levels, and historical and cultural factors between coastal and inland regions. To further investigate regional ERs, GC, and their heterogeneous effects on technological innovation, we divide the sample into the two aforementioned regions and conduct an empirical analysis based on the geographical adjacency matrix (Tables 4, and 5).
The study shows that the local and spatial spillover effects of ERs on GTI in coastal areas are significantly negative, which in turn undermines the GTI process in neighboring areas. If there is no reasonable environmental compensation mechanism among local governments, it is difficult to bridge the benefit gap caused by the governance costs and opportunity costs paid by each region for green transformation, which in turn restricts the equity of regional manufacturing innovation development. The impact of ERs in inland areas on GTI is significantly negative, and the impact on GTI in surrounding areas is insignificant, which is mainly constrained by resource endowment, industrial structure, and institutional culture, thereby hindering the stimulation of the dynamics of regional GTI activities.
GC in coastal areas exerts a positive effect on green technologies in the region and a negative effect on GTI in neighboring regions. At the firm level, this can be explained by the fact that the strict credit granting policy of GC, by setting environmental access thresholds, induces heavily polluting firms to focus on investment in production factors at the end of pollution reduction, which in turn inhibits local GTI activities. As GC resources become the focus of competition between regions, the abundance of local GC resources further exacerbates the innovation financing dilemma of neighboring regions, thereby inhibiting the GTI level in neighboring regions (Zhou et al., 2021). The local effect of GC on GTI in inland regions is significantly positive, while the spatial spillover effect does not pass the significance test, indicating that the competition for GC resources in inland regions does not intensify. The reason for this phenomenon is that coastal regions have advantages in terms of economic conditions, market system formations, and technological innovation resource reserves. In particular, the pace of economic green transformation has always been at the forefront for China, with more complete ERs policy measures and mature pollution control experience. Therefore, GC can be combined with traditional ERs instruments to create a gaining effect of regional technological innovation activities. However, the impact of the cross-sectional term of ERs and GC on local technological innovation in inland regions is insignificant, indicating that GC policies and traditional ERs instruments in inland regions have not yet produced incentive-matching effects.
To better verify the mechanism of action between ERs, GC, and GTI, we use the following methods to test the robustness of the empirical results and the results are reported in Table 6.
Considering the inteslrraction of ERs strategies and the spillover effects of GTI levels occurring in neighboring regions, as well as multiple factors, geographical and cognitive proximity also exert an impact. Therefore, here, the economic spatial weight matrix is used instead of the 0–1 geographical neighborhood weight matrix, and the results of the study do not show a significant change in the sign and significance of the regression coefficients of the core variables, confirming that our findings are extremely robust.
The combined index of GIPAs and green utility patent applications is chosen as the measure of GTI, based on which GTI was calculated. Through the model analysis, we observe that the regression results are very robust for the green innovation variables and the replacement of the weight matrix, which further validates the scientific nature of our findings.
By incorporating both environmental regulation and green credit policies into the analytical framework of GTI, this paper empirically tests the joint effect of GC and ERs on GTI. Based on inter-regional interaction strategies and differences, this paper also explores the local effects and spatial spillover effects of environmental regulation and green credit at the regional level, and empirically examines the uneven locational characteristics of the technological innovation effects of ERs and GC. The main findings of this paper include the following: 1) the local impact and spatial spillover effect of ERs on GTI in manufacturing industries at the national level is mainly reflected in the inhibitory effect. The negative effect of “following cost” is greater than the “compensation effect” of technological innovation; that is, environmental regulation policies that raise firms’ production costs and lack economic incentives are ineffective in promoting innovation. GC policies can stimulate the transformational development of GTI in local manufacturing industries, but the scarcity of resources weakens the innovation level of green technology in manufacturing industries in neighboring provinces through spatial spillover effects. 2) The combination of GC development and ERs can produce synergistic and complementary incentive-coordinated technology innovation driving effects. The complementary effects of GC fund supply and product, process, and emission reduction are significant, making the economic performance of ERs for technology innovation prominent. Considering the ecological environment has quasi-public goods characteristics, GTI is a complex systemic project, and green finance, as a new market-based instrument for ecological and environmental governance, can play a complementary role with traditional environmental regulatory systems in a more effective, equitable and sustainable manner. However, it is difficult to promote green innovation transformation by purely relying on the regulation means of GC. The traditional ERs means are fundamental in stimulating technology innovation despite the hard constraint of commercial banks and emission enterprises (Feng and Liang, 2022). 3) The eastern coastal regions with strong innovation vitality and endogenous dynamics. For inland regions with little experience in environmental governance and a weak innovation base, a “circular-coordination” mechanism for GC resources should be constructed. By region, there are regional differences in the technological innovation effects of ERs and GC. The local effect of ERs on GTI in coastal areas is significantly positive; that is, strong innovation vitality and endogenous dynamics of coastal regions enable environmental regulation measures and green financial development produces incentive-matching effects. Although environmental regulation measures for inland areas with little experience in environmental governance and a weak innovation base are significantly negative for local GTI and insignificant for neighboring GTI, GC effectively stimulates the dynamic role of local GTI activities and exerts a significant marginal effect on the financial dilemma of local manufacturing investment in research and development (R&D) green technology.
The following shortcomings exist for this study: 1) It is appropriate to use prefecture-level city data to explore the spatial effects of ERs, GC, and GTI in manufacturing based on regional scales. Given the availability of GC-related data, provincial panel data, and the large error in measuring GC variables by indirect methods, there are limitations in the generalizability of the study findings. 2) The “Porter hypothesis” and the regulatory role of GC are closely related to the type of ERs, which include formal ERs instruments (command and control and market incentives) and informal ERs instruments (information disclosure, public participation, and voluntary regulation) (Zhou et al., 2022). However, the influence factors considered in this study are relatively single, and different types of ERs tools can be included in the same model in future studies to examine their differential impacts on GTI in manufacturing. 3) Considering that the spatial spillover effect follows the law of distance decay, there is a certain bias in the empirical test based on the entire domain only, and the local spatial matrix of different distance ranges should be set in the future to explore the GTI effect.
Based on the empirical findings of our study, the following policy implications about the integration of ERs and green finance policies are proposed accordingly.
First, based on the spatial dependence of interprovincial manufacturing GTI, local governments should establish a good competitive relationship with each other, strengthen strategic interoperability and positive interaction with neighboring regions, and stimulate local green development with the implementation of green innovation and transformation strategies by regional industries holistically.
Second, the central government should abandon the traditional approach in the design of policies, systems, and processes for environmental and social risk management, and coordinate a regionally differentiated GC system instead. GC policies should be made on a “person-by-person” basis; that is the eastern coastal should leverage the role of commercial banks and other financial sectors in promoting the supply of GC funds to meet the financial needs of high-level technological innovation. It is necessary to improve the green policy system, such as the environmental information disclosure of enterprises, information sharing between environmental protection departments and banks, and strengthen the prescreening and post-supervision mechanism to weaken the influence of information asymmetry. For inland regions, local governments can create an innovative atmosphere through financial support, construction of innovative subjects, and strengthening intellectual property protection, so as to construct a cooperative mechanism and innovation-supportive for interregional credit policies (Liu and Nie, 2022) in the place of the interregional competition for resources with a cooperative mechanism for interregional credit resources to support GTI (Liu and Nie, 2022).
Third, as GTI is a complex systemic project, it is difficult to promote green innovation transformation by purely relying on the regulation means of GC. The traditional ERs means are fundamental in stimulating technology innovation despite the hard constraint of commercial banks and emission enterprises (Feng and Liang, 2022). The ERs system can be planned in a unified manner by establishing a coordinating management institution for GTI, GC, and ERs to coordinate green development data, such as scientific and technological innovation, capital loans, and pollution emissions, and make joint efforts from the market investment and financing and government supervision levels (Chen et al., 2022).
Fourth, considering that GTI activities are characterized by high risks, economies of scale, and innovation spillovers (Wicki and Hansen, 2019), the institutional environment for technology innovation is the key to the “Porter effect.” Local governments can create an innovative atmosphere through financial support, construction of innovative subjects, strengthening intellectual property protection, and other basic systems, which in turn attract the gathering of high-end production factors, such as capital, talents, and knowledge. Concomitantly, the GC policy can also be dovetailed with the science and technology policy, incorporate the green R&D investment and performance of enterprises into the environmental policy assessment, and make joint efforts from the market investment and financing and government supervision levels (Chen et al., 2022).
The original contributions presented in the study are included in the article/supplementary material, further inquiries can be directed to the corresponding author.
Conceptualization, LJ, JZ, and YW; methodology, JZ and YW.; software, YW; writing—original draft preparation, LJ; writing—review and editing, LJ and JZ; project administration, LJ; funding acquisition, LJ. All authors have read and agreed to the published version of the manuscript.
This research was funded by the National Natural Science Foundation of China, grant number 72074238、72074239; the Fundamental Research Funds for the Central University of Finance and Economics; and the Double First-class Discipline Construction Project of Central University of Finance and Economics.
The authors declare that the research was conducted in the absence of any commercial or financial relationships that could be construed as a potential conflict of interest.
All claims expressed in this article are solely those of the authors and do not necessarily represent those of their affiliated organizations, or those of the publisher, the editors and the reviewers. Any product that may be evaluated in this article, or claim that may be made by its manufacturer, is not guaranteed or endorsed by the publisher.
Anselin, L., and Griffith, D. A. (2010). Do spatial effects really matter in regression analysis? Pap. Reg. Sci. 65, 11–34. doi:10.1111/J.1435-5597.1988.TB01155.X
Bai, Y., Song, S. Y., Jiao, J. L., and Yang, R. R. (2019). The impacts of government R&D subsidies on green innovation: Evidence from Chinese energy-intensive firms. J. Clean. Prod. 233 (1), 819–829. doi:10.1016/j.jclepro.2019.06.107
Behera, P., and Sethi, N. (2022). Nexus between environment regulation, FDI, and green technology innovation in OECD countries. Environ. Sci. Pollut. Res. 29, 52940–52953. doi:10.1007/s11356-022-19458-7
Böcher, M. (2012). A theoretical framework for explaining the choice of instruments in environmental policy. For. Policy Econ. 16, 14–22. doi:10.1016/j.forpol.2011.03.012
Bressanelli, G., Perona, M., and Saccani, N. (2019). Challenges in supply chain redesign for the circular economy: A literature review and a multiple case study. Int. J. Prod. Res. 57 (23), 7395–7422. doi:10.1080/00207543.2018.1542176
Bressanelli, G., Visintin, F., and Saccani, N. (2022). Circular economy and the evolution of industrial districts: A supply chain perspective. Int. J. Prod. Econ. 243, 108348. doi:10.1016/j.ijpe.2021.108348
Chen, L., Wang, N., Li, Q. Y., and Zhou, W. J. (2022). Environmental regulation, foreign direct investment and China’s economic development under the new normal: Restrain or promote? Environ. Dev. Sustain., 1–22. doi:10.1007/s10668-022-02239-0
Chen, Z. G., Zhang, Y. Q., Wang, H. S., Ouyang, X., and Xie, Y. X. (2022). Can green credit policy promote low-carbon technology innovation? J. Clean. Prod. 359, 132061. doi:10.1016/j.jclepro.2022.132061
de Oliveira, U. R., Espindola, L. S., da Silva, I. R., da Silva, L. N., and Rocha, H. M. (2018). A systematic literature review on green supply chain management: Research implications and future perspectives. J. Clean. Prod. 187, 537–561. doi:10.1016/j.jclepro.2018.03.083
Falcone, P. M. (2020). Environmental regulation and green investments: The role of green finance. Int. J. Green. Econ. 14 (2), 159–173. doi:10.1504/IJGE.2020.109735
Feng, Y. C., and Liang, Z. (2022). How does green credit policy affect total factor productivity of the manufacturing firms in China? The mediating role of debt financing and the moderating role of environmental regulation. Environ. Sci. Pollut. Res. 29, 31235–31251. doi:10.1007/s11356-021-17984-4
Geng, W. L., Li, Y. Y., Zhang, P. Y., Yang, D., Jing, W. L., and Rong, T. Q. (2022). Analyzing spatio-temporal changes and trade-offs/synergies among ecosystem services in the Yellow River Basin China. Ecol. Indic. 138, 108825. doi:10.1016/J.ECOLIND.2022.108825
Guo, L. L., Qu, Y., and Tseng, M. L. (2017). The interaction effects of environmental regulation and technological innovation on regional green growth performance. J. Clean. Prod. 162, 894–902. doi:10.1016/j.jclepro.2017.05.210
Guo, Q., Zhou, M., Liu, N. N., and Wang, Y. Y. (2019). Spatial effects of environmental regulation and green credits on green technology innovation under low-carbon economy background conditions. Int. J. Environ. Res. Public Health 16 (17), 3027. doi:10.3390/ijerph16173027
Hassan, T., Khan, Y., He, C. L., Chen, J., Alsagr, N., Song, H. M., et al. (2022). Environmental regulations, political risk and consumption-based carbon emissions: Evidence from OECD economies. J. Environ. Manage. 320, 115893. doi:10.1016/j.jenvman.2022.115893
He, C., and Yan, G. J. (2020). Path selections for sustainable development of green finance in developed coastal areas of China. J. Coast. Res. 104 (1), 77–81. doi:10.2112/JCR-SI104-014.1
Holzinger, K., and Sommerer, T. (2011). ‘Race to the bottom’ or‘race to brussels’? Environmental competition in europe. J. Common Mark. S. 49 (2), 315–339. doi:10.1111/j.1468-5965.2010.02135.x
Hsu, P. H., Tian, X., and Xu, Y. (2014). Financial development and innovation: Cross-country evidence. J. Financ. Econ. 112 (1), 116–135. doi:10.1016/j.jfineco.2013.12.002
Huang, L. X., Liu, S. L., Han, Y. H., and Peng, K. M. (2020). The nature of state-owned enterprises and collection of pollutant discharge fees: A study based on Chinese industrial enterprises. J. Clean. Prod. 271, 122420. doi:10.1016/j.jclepro.2020.122420
Irfan, M., Razzaq, A., Sharif, A., and Yang, X. D. (2022). Influence mechanism between green finance and green innovation: Exploring regional policy intervention effects in China. Technol. Forecast. Soc. Change 182, 121882. doi:10.1016/j.techfore.2022.121882
Jayachandran, S. (2022). How economic development influences the environment. Annu. Rev. Econ. 14, 229–252. doi:10.1146/annurev-economics-082321-123803
Jiang, H. L., Wang, W. D., Wang, L., and Wu, J. H. (2020). The effects of the carbon emission reduction of China’s green finance: An analysis based on green credit and green venture investment. Fin. Forum. 25 (11), 39–48. doi:10.16529/j.cnki.11-4613/f.2020.11.006
Karmaker, S. C., Hosan, S., Chapman, A. J., and Saha, B. B. (2021). The role of environmental taxes on technological innovation. Energy 232, 121052. doi:10.1016/j.energy.2021.121052
Kolkiş, S., Krajacic, G., Duic, N., Rosen, M. A., and Al-Nimr, M. A. (2020). Advances in integration of energy, water and environment systems towards climate neutrality for sustainable development. Energy Convers. Manag. 225, 113410. doi:10.1016/j.enconman.2020.113410
Li, M. Y., and Gao, X. (2022). Implementation of enterprises’ green technology innovation under market-based environmental regulation: An evolutionary game approach. J. Environ. Manage. 308, 114570. doi:10.1016/j.jenvman.2022.114570
Liu, J. Y., Xia, Y., Fan, Y., Lin, S. M., and Wu, J. (2017). Assessment of a green credit policy aimed at energy-intensive industries in China based on a financial CGE model. J. Clean. Prod. 163, 293–302. doi:10.1016/j.jclepro.2015.10.111
Liu, S., Xu, R. X., and Chen, X. Y. (2021). Does green credit affect the green innovation performance of high-polluting and energy-intensive enterprises? Evidence from a quasi-natural experiment. Environ. Sci. Pollut. Res. 28, 65265–65277. doi:10.1007/s11356-021-15217-2
Liu, X. M., and Nie, W. D. (2022). Study on the coupling coordination mechanism of green technology innovation, environmental regulation, and green finance. Environ. Sci. Pollut. Res. 29, 71796–71809. doi:10.1007/s11356-022-20905-8
Lv, C. C., Shao, C. H., and Lee, C. C. (2021). Green technology innovation and financial development: Do environmental regulation and innovation output matter? Energy Econ. 98, 105237. doi:10.1016/j.eneco.2021.105237
Mbanyele, W., and Wang, F. R. (2022). Environmental regulation and technological innovation: Evidence from China. Environ. Sci. Pollut. Res. 29, 12890–12910. doi:10.1007/s11356-021-14975-3
Nabeeh, N. A., Abdel-Basset, M., and Soliman, G. (2021). A model for evaluating green credit rating and its impact on sustainability performance. J. Clean. Prod. 280, 124299. doi:10.1016/j.jclepro.2020.124299
Ni, Y., Chen, B. Y., and Wang, Y. W. (2020). Financial development, environmental regulation and green total factor productivity--an empirical analysis based on spatial Durbin model[J]. J. Guizhou Univ. Fin. Econ. (03), 12–21. doi:10.3969/j.issn.1003-6636.2020.03.002
Nie, G. Q., Zhu, Y. F., Wu, W. P., Xie, W. H., and Wu, K. X. (2022). Impact of voluntary environmental regulation on green technological innovation: Evidence from Chinese manufacturing enterprises. Front. Energy Res. 10, 889037. doi:10.3389/fenrg.2022.889037
Peng, C., and Yuan, P. (2018). Environmental regulation intensity and provincial economic growth in China--a reconstruction based on the intensity of environmental regulation[J]. J. Yunnan Univ. Fin. Econ. 34 (10), 37–51. doi:10.16537/j.cnki.jynufe.000353
Qu, W. B. (2018). Environmental regulation, spatial spillover and regional ecological efficiency: An empirical analysis of Douban Panel Model based on space. J. Beijing Inst. Tech. Soc. Sci. Ed. . 20 (6), 27–33. doi:10.15918/j.jbitss1009-3370.2018.5179
Ren, S. G., Li, X. L., Yuan, B. L., Li, D. Y., and Chen, X. H. (2018). The effects of three types of environmental regulation on eco-efficiency: A cross-region analysis in China. J. Clean. Prod. 173, 245–255. doi:10.1016/j.jclepro.2016.08.113
Shao, S., Li, X., Cao, J. H., and Yang, L. L. (2016). Economic policy options for haze pollution management in China--a perspective based on spatial spillover effects. Econ. Res. 51 (09), 73–88.
Sharif, A., Saqib, N., Dong, K. Y., and Khan, S. A. R. (2022). Nexus between green technology innovation, green financing, and CO 2 emissions in the G7 countries: The moderating role of social globalisation. Sustain. Dev., 1–13. doi:10.1002/sd.2360
Shen, F., Liu, B., Luo, F., Wu, C. C., Chen, H., and Wei, W. D. (2021). The effect of economic growth target constraints on green technology innovation. J. Environ. Manage. 292, 112765. doi:10.1016/j.jenvman.2021.112765
Soundarrajan, P., and Vivek, N. (2016). Green finance for sustainable green economic growth in India. Agric. Econ. 62, 35–44. doi:10.17221/174/2014-AGRICECON
Su, C. W., Li, W. H., Umar, M., and Lobont, O. R. (2022). Can green credit reduce the emissions of pollutants? Econ. Anal. Policy 74, 205–219. doi:10.1016/j.eap.2022.01.016
Suki, N. M., Suki, N. M., Afshan, S., Sharif, A., Kasim, M. A., and Mohd Hanafi, S. R. (2022). How does green technology innovation affect green growth in ASEAN-6 countries? Evidence from advance panel estimations. Gondwana Res. 111, 165–173. doi:10.1016/j.gr.2022.06.019
Wang, H. T., Qi, S. Z., Zhou, C. B., Zhou, J. J., and Huang, X. Y. (2022). Green credit policy, government behavior and green innovation quality of enterprises. J. Clean. Prod. 331, 129834. doi:10.1016/j.jclepro.2021.129834
Wei, W. D., Cai, W. Q., Guo, Y., Bai, C. Q., and Yang, L. Z. (2020). Decoupling relationship between energy consumption and economic growth in China's provinces from the perspective of resource security. Resour. Policy 68, 101693. doi:10.1016/j.resourpol.2020.101693
Wheeler, D. (2001). Racing to the bottom? Foreign investment and air pollution in developing countries. J. Environ. Dev. 10 (3), 225–245. doi:10.1596/1813-9450-2524
Wicki, S., and Hansen, E. G. (2019). Green technology innovation: Anatomy of exploration processes from a learning perspective. Bus. Strategy Environ. 28 (6), 970–988. doi:10.1002/bse.2295
Yang, H. S., Chen, S. L., and Zhou, Y. Z. (2008). Local government competition and environmental policy-empirical evidence from province’s governments in China. South China J. Econ. (6), 15–30. doi:10.3969/j.issn.1000-6249.2008.06.002
Zhang, H., Liu, Z., and Zhang, Y. J. (2022). Assessing the economic and environmental effects of environmental regulation in China: The dynamic and spatial perspectives. J. Clean. Prod. 334, 130256. doi:10.1016/j.jclepro.2021.130256
Zhang, H., and Wei, X. P. (2014). Green paradox or forced emission-reduction: Dual effect of environmental regulation on carbon emissions. China Pop. Res. Environ. 24 (9), 21–29. doi:10.3969/j.issn.1002-2104.2014.09.004
Zhang, P. Y., Yang, D., Qin, M. Z., and Jing, W. L. (2020). Spatial heterogeneity analysis and driving forces exploring of built-up land development intensity in Chinese prefecture-level cities and implications for future urban land intensive use. Land Use Policy 99, 104958. doi:10.1016/j.landusepol.2020.104958
Zhang, S. L., Wu, Z. H., He, Y. N., and Hao, Y. (2022). How does the green credit policy affect the technological innovation of enterprises? Evidence from China. Energy Econ. 113, 106236. doi:10.1016/j.eneco.2022.106236
Zhao, A. W., Wang, J. Y., Sun, Z. Z., and Guan, H. J. (2022). Environmental taxes, technology innovation quality and firm performance in China—a test of effects based on the porter hypothesis. Econ. Anal. Policy 74, 309–325. doi:10.1016/j.eap.2022.02.009
Zhao, X., Ma, X. W., Chen, B. Y., Shang, Y. P., and Song, M. L. (2021). Challenges toward carbon neutrality in China: Strategies and countermeasures. Resour. Conserv. Recycl. 176, 105959. doi:10.1016/j.resconrec.2021.105959
Zhong, Z. Q., and Peng, B. H. (2022). Can environmental regulation promote green innovation in heavily polluting enterprises? Empirical evidence from a quasi-natural experiment in China. Sustain. Prod. Consum. 30, 815–828. doi:10.1016/j.spc.2022.01.017
Zhou, G. Y., Liu, C., and Luo, S. M. (2021). Resource allocation effect of green credit policy: Based on DID model. Math. (Basel). 9 (2), 159. doi:10.3390/math9020159
Keywords: green credit, environmental regulation, green technology innovation, regulatory effect, spatial effect
Citation: Jiang L, Wang Y and Zhang J (2022) Local-neighborhood effects of environmental regulations on green technology innovation in manufacturing: Green credit-based regulation. Front. Environ. Sci. 10:1072180. doi: 10.3389/fenvs.2022.1072180
Received: 17 October 2022; Accepted: 08 November 2022;
Published: 28 November 2022.
Edited by:
Jay Lee, Kent State University, United StatesCopyright © 2022 Jiang, Wang and Zhang. This is an open-access article distributed under the terms of the Creative Commons Attribution License (CC BY). The use, distribution or reproduction in other forums is permitted, provided the original author(s) and the copyright owner(s) are credited and that the original publication in this journal is cited, in accordance with accepted academic practice. No use, distribution or reproduction is permitted which does not comply with these terms.
*Correspondence: Jian Zhang, empjdWZlQGN1ZmUuZWR1LmNu
Disclaimer: All claims expressed in this article are solely those of the authors and do not necessarily represent those of their affiliated organizations, or those of the publisher, the editors and the reviewers. Any product that may be evaluated in this article or claim that may be made by its manufacturer is not guaranteed or endorsed by the publisher.
Research integrity at Frontiers
Learn more about the work of our research integrity team to safeguard the quality of each article we publish.