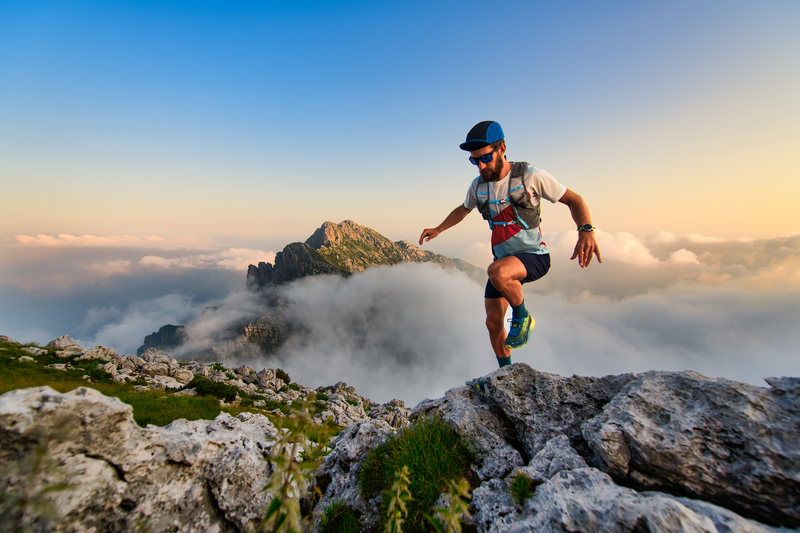
94% of researchers rate our articles as excellent or good
Learn more about the work of our research integrity team to safeguard the quality of each article we publish.
Find out more
EDITORIAL article
Front. Environ. Sci. , 11 January 2023
Sec. Environmental Citizen Science
Volume 10 - 2022 | https://doi.org/10.3389/fenvs.2022.1071665
This article is part of the Research Topic Social Media, Artificial Intelligence and Carbon Neutrality View all 10 articles
Editorial on the Research Topic
Social media, artificial intelligence and carbon neutrality
In recent years, more ambitious climate targets have been announced by countries as knowledge of the magnitude and rate of climate change has increased. Terminology like carbon neutrality has become more prevalent (Majava et al., 2022). Carbon neutrality refers to net-zero carbon emission achieved by either raising carbon adsorption or lowering carbon emissions. Many places have set out their road map to achieve the carbon neutrality goal. For example, the European Green Deal, a new growth strategy for the EU, was introduced by the EU Commission in 2019. The fundamental objective of this policy is to make the EU climate neutral by 2050 (Wyrwa et al., 2022). China, the greatest developing nation in the world, has pledged to attain carbon neutrality by 2060 and a peak in carbon dioxide emissions before 2030 to reduce the greenhouse effect (Liu et al., 2022). By 2035, Finland’s government intends to be carbon neutral. The creation of low-carbon roadmaps by industry sectors has been one policy tool towards this goal. The government started developing low-carbon roadmaps in 2019, where industry sectors had to state when and how they would achieve carbon neutrality (Majava et al., 2022).
To achieve the goal of carbon neutrality with government policies, problems should be identified. Social media offers effective platforms for disseminating carbon neutrality-related information (Yao et al., 2022) and advancing environmentally friendly living practices. At the same time, AI helps us analyse big data that can be used to make policy recommendations to governments and understand how nations and governments responded to a high-risk event or an environmental disaster by looking at historical data. Given the above, this Research Topic aims to cover carbon neutrality, artificial intelligence and social media.
Given that previous research has overlooked social media’s potential to exert pressure on corporations to disclose their carbon emissions, Shao and He examined the influence of social media pressure on corporate carbon disclosure based on legitimacy theory, using data from 3,656 Chinese listed businesses between 2009 and 2019. Computer programs classified positive, neutral, and negative sentiments comments. This study employed the Janis-Fadner coefficient (J-F) (legitimacy) to assess the legitimacy pressure on social media. It was found that the legitimacy pressure from social media considerably improved corporate carbon disclosure. Companies should put more effort into effective carbon management strategies and disclosure to achieve consistent carbon management practices.
Zeng et al. analysed Weibo and LinkedIn to learn about the public’s and professionals’ interest in carbon-neutral cities by comprising 533 postings (3,733 sentences) on LinkedIn and 1908 microposts (14,668 sentences) on Weibo, which is the first of its kind. The research found that organisations and the government Weibo users in the Weibo platform are key opinion leaders in this area, while the co-director of the Alliance for Carbon Neutral Cities was the most influential person on LinkedIn. As for the most popular posts, this study utilised the clustering approach, an artificial intelligence method for analysis. The most influential cluster on Weibo centred on low-carbon city development, while the largest cluster on LinkedIn was related to climate change action. In general, users on Weibo and LinkedIn focused on “energy” and related topics. A slight difference was that Weibo users concerned about green development in the building industry more, whereas LinkedIn users focused more on climate and sustainability.
Because of the importance of carbon-neutral regulations and supervision, this Research Topic consists of three articles about regulations and carbon neutrality.
Gao and Gao (2022)’s study pinpointed waste disposal aids in achieving “double carbon”. The most feasible waste treatment is incineration; however, the right choice of location and the legal compensation system are necessary. This project offered a dynamic environmental monitoring system for waste incineration power facilities and addressed the health risk to inhabitants based on a Gaussian model. It computed the pollutants around the waste incineration power plant by considering topography, wind direction, and other effects on pollutants’ diffusion. Monitoring stations were installed, and the waste incineration’s environmental monitoring system was constructed to study the concentration distribution. This study also designed economic compensation plans to inform future regulation by policymakers by considering the power plant’s income, economy, government compensation, and pollution level.
Liu B. et al. suggested that through the use of media, informal environmental control has gradually shown a positive impact on green innovation as information technology has advanced. Using panel data from 285 prefecture-level Chinese cities between 2008 and 2019, this study examined how environmental legislation affected urban green innovation. Using two-way fixed-effect and mediation-effect models, they studied the impact of heterogeneous environmental legislation on urban green innovation. A negative U-shaped relationship existed between market-based and voluntary environmental regulation, whereas an inverted non-linear U-shaped relationship existed between command-based environmental regulation and urban green innovation. Their findings indicated that China’s urban green innovation development was sluggish and national policies affected it. This study concluded that China needs to increase environmental regulatory efficiency to meet the country’s carbon neutrality target.
Ying et al. investigated the heavy polluting-listed companies’ innovation behavioural changes under the tightening environmental regulations following the “smog explosion” event as a “quasi-natural experiment” by using a differences-in-differences approach. By examining the variations in innovation behaviour of firms with varying R&D intensities and varied property rights, this study identifies the contradictory “Porter hypothesis”, which proposes that polluting businesses can gain from environmental policies. The quantile regression results demonstrated a U-shaped relationship between enterprise R&D intensity and the haze treatment effect. Compared to privately owned heavy-polluting enterprises, state-owned heavy-polluting firms had a more significant decline in innovation investment.
The remaining four articles in this Research Topic are concerned with the influence of the digital economy on carbon emissions. Wu et al. contributed to the existing scholarship by examining the regional variability and threshold effects of the influence of the digital economy on carbon emissions in addition to measuring the spatial impact of carbon emissions. It evidenced that the digital economy could reduce carbon emissions.
In the second article, Gao et al. reviewed the information in Xinhuanet, a site for Xinhua News Agency’s news releases, one prominent media for reporting on China’s carbon issues. Using computational algorithm coding, the results found that digital economy transformation in reducing carbon emissions was more effective in China’s central area. The effect coefficient of the digital economy was significant when the time lag of carbon emission intensity was included. In addition, local efforts to reduce carbon emissions were severely hampered by those in nearby places. It was challenging for low-tech regions to benefit from the digital economy’s emission reduction benefits. Stricter environmental laws in the digital economy accelerated regional carbon emission reductions. To unleash the carbon emission reduction effect of the digital economy, China should enhance its digital infrastructure and encourage reform and innovation (Gao et al.).
Social media has developed into a vital tool for people to learn, work, and live in the era of the mobile internet. In China, there were 832 million consumers of short videos in June 2021 or 85.8% of all internet users. Based on the Stimulus–Organism–Response model, Wang and Yue explored the influence of short science videos on people’s environmental willingness via stimulus response in the third article. This study found that short videos positively influence people’s environmental willingness. This study concluded that we should focus on the emotional resonance of people’s thoughts and make better use of sound and pictures to optimise the persuasive effect of short videos (Wang and Yue).
Finally, most studies neglected the impact of reducing carbon emissions on trade while concentrating primarily on the one-way effect of foreign trade on carbon emissions. In the last article, Zhang et al. (2022) examined the dynamic interactions between global business, technological innovation and carbon emissions via the panel vector autoregressive model. They demonstrated that whereas international trade and carbon emissions were mutually hindering, technological advancement and improving carbon emissions mutually supported each other. While the Chinese doing business abroad faced challenges in overcoming carbon-related trade obstacles, innovations in low-carbon technologies were essential to this procedure.
All authors listed have made a substantial, direct, and intellectual contribution to the work and approved it for publication.
The authors declare that the research was conducted in the absence of any commercial or financial relationships that could be construed as a potential conflict of interest.
All claims expressed in this article are solely those of the authors and do not necessarily represent those of their affiliated organizations, or those of the publisher, the editors and the reviewers. Any product that may be evaluated in this article, or claim that may be made by its manufacturer, is not guaranteed or endorsed by the publisher.
Gao, Y., and Gao, R. (2022). Research and analysis of environmental legal compensation mechanisms related to waste incineration in the context of “double carbon”. Front. Ecol. Evol. 10, 979482. doi:10.3389/fevo.2022.979482
Liu, S., Jiang, Y., Yu, S., Tan, W., Zhang, T., and Lin, Z. (2022b). Electric power supply structure transformation model of China for peaking carbon dioxide emissions and achieving carbon neutrality. Energy Rep. 8, 541–548. doi:10.1016/j.egyr.2022.10.085
Majava, A., Vadén, T., Toivanen, T., Järvensivu, P., Lähde, V., and Eronen, J. T. (2022). Sectoral low-carbon roadmaps and the role of forest biomass in Finland’s carbon neutrality 2035 target. Energy Strategy Rev. 41, 100836. doi:10.1016/j.esr.2022.100836
Wyrwa, A., Suwała, W., Pluta, M., Raczyński, M., Zyśk, J., and Tokarski, S. (2022). A new approach for coupling the short- and long-term planning models to design a pathway to carbon neutrality in a coal-based power system. Energy 239, 122438. doi:10.1016/j.energy.2021.122438
Yao, Q., Li, R. Y. M., and Song, L. (2022). Carbon neutrality vs neutralité carbone: a comparative study on French and English users' perceptions and social capital on Twitter. Frontiers in Environmental Science 10, 969039. doi:10.3389/fenvs.2022.969039
Keywords: social media, artificial intelligence, AI, carbon neutrality, regulation, technology, digital
Citation: Li RYM, Crabbe MJC and Shao X (2023) Editorial: Social media, artificial intelligence and carbon neutrality. Front. Environ. Sci. 10:1071665. doi: 10.3389/fenvs.2022.1071665
Received: 16 October 2022; Accepted: 30 November 2022;
Published: 11 January 2023.
Edited and reviewed by:
Steffen Fritz, International Institute for Applied Systems Analysis (IIASA), AustriaCopyright © 2023 Li, Crabbe and Shao. This is an open-access article distributed under the terms of the Creative Commons Attribution License (CC BY). The use, distribution or reproduction in other forums is permitted, provided the original author(s) and the copyright owner(s) are credited and that the original publication in this journal is cited, in accordance with accepted academic practice. No use, distribution or reproduction is permitted which does not comply with these terms.
*Correspondence: Rita Yi Man Li, cml0YXJlYzFAeWFob28uY29tLmhr
Disclaimer: All claims expressed in this article are solely those of the authors and do not necessarily represent those of their affiliated organizations, or those of the publisher, the editors and the reviewers. Any product that may be evaluated in this article or claim that may be made by its manufacturer is not guaranteed or endorsed by the publisher.
Research integrity at Frontiers
Learn more about the work of our research integrity team to safeguard the quality of each article we publish.