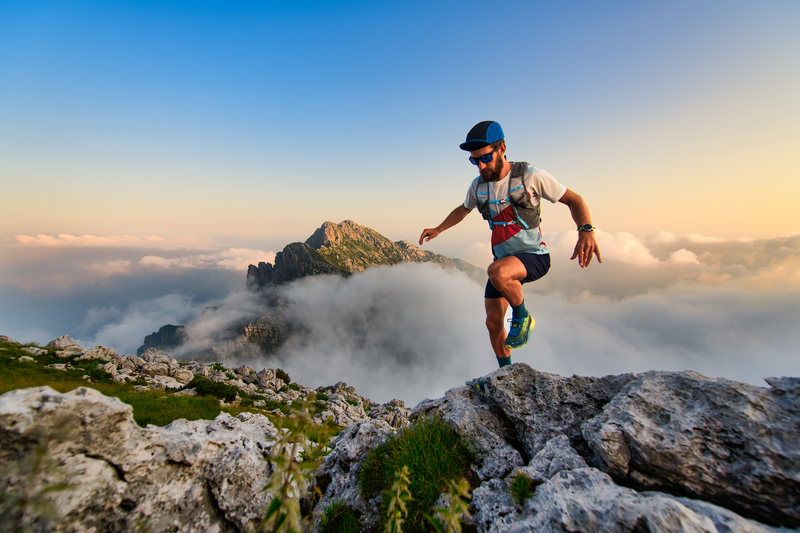
94% of researchers rate our articles as excellent or good
Learn more about the work of our research integrity team to safeguard the quality of each article we publish.
Find out more
ORIGINAL RESEARCH article
Front. Environ. Sci. , 04 January 2023
Sec. Environmental Economics and Management
Volume 10 - 2022 | https://doi.org/10.3389/fenvs.2022.1065000
This article is part of the Research Topic Clean Energy Transition and Load Capacity Factors: Environmental Sustainability Assessment through Advanced Statistical Methods View all 18 articles
This research investigates the nexus existing between agricultural productivity and CO2 emissions under the moderating effect of transportation within the context of China. The data for this study are drawn from the World Bank and cover the period 1991–2019. The data is analyzed using an autoregressive distributed lag approach (ARDL). Agricultural productivity is measured in terms of crop and livestock production. The goal of this research is to make some contributions, as crop production has a negative impact on carbon dioxide emissions in the long and short run. Carbon dioxide emissions are positively influenced by livestock production in the long run, but negatively in the short run. As for the moderation effect, the results indicate that transportation significantly impacts agricultural productivity and CO2 in both the long and short term. The study provides in-depth insights to policy makers for designing more suitable policies regarding the necessity of decreasing CO2 emissions. In addition to discussing the crucial implications, future directions are also discussed.
In China’s economic development, the agriculture sector plays a vital role. As per, Zheng et al. (2019), China is one of the most growing economies in the world. China is dominant in producing vegetables, meat and different kinds of cereals. Further, China is in the first position regarding the largest population ranking. Thus, it is crucial to understand how agricultural activity affects CO2 emissions and also how transportation moderates this relationship. The main objective of this study is to determine how China’s agricultural sector is affecting the environmental ecosystem.
Further, the economic development and climate change have been in line for the last two decades. As a result of economic growth, carbon dioxide emissions contribute to the worldwide climate crisis, such as floods, storms, extreme temperatures, melting glaciers and rising sea levels (Fei, et al., 2011). One of the greatest threats fronted by humanity on this globe is climate change (Lu et al., 2018). In many parts of the world, rising global average surface temperatures are melting glaciers and altering precipitation, severely eroding regional water supply advantages (Nie et al., 2021). In recent decades, many experimental studies have proclaimed that climate change and global warming are the causes of environmental deterioration and the researchers are highlighting the significance of sustainable environment (Lu et al., 2018).
The sustainable environment denotes securing the climate for posterity without compromising the present speed of economic flourishment. Sustainable progression is essential to form this globe into a livable space for not only the current generation but also for posterity. Minimizing carbon discharges is the major target to achieve the sustainable evolution (Barroso, 2020). Many analysts have examined the relationship between climate and sustainability, while many examined other variables that may affect this relationship from this perspective. One of the key variables in evaluating the relationship between environment and growth (Nathaniel et al., 2021), is energy consumption as it is the primary contributor to CO2 emissions.
As all economic sectors are accountable for their growth, so all have some primary or secondary influence on carbon dioxide emissions, and likely its accurate for the agricultural sector (Khan et al., 2021). The agricultural sector accounts for 14%–30% of global GHG emissions because of its reliance on fossil fuels (Zhang et al., 2020). Indeed, the use of gasoline-powered farm equipment, pumping water for irrigation, indoor livestock care, and the use of nitrogen-rich fertilizers all contribute to agriculture’s extraordinary greenhouse gas emissions. According to the Food and Agriculture Organization of the United Nations (FAO), agricultural sector CO2 emissions will likely to decrease by almost 80%–88% between now and 2020 (Zhang et al., 2020). Indeed, it can be attained by replacing the agronomics sector’s layout and alternating the energy mix utilized in the agricultural zone by using sustainable sources. This also highlights the significance of the agricultural sector that it is a dominant root of earnings, especially in growing countries (Jebli et al., 2020). Further, agriculture is also in charge of supplying food to masses of humans all over the world. Chien et al. (2022) stated that agronomy could have a constructive or obstructive influence on the standard of the environment. Thus, through energy, and utilization, agriculture can influence the environment adversely.
One of the major causes behind CO2 emissions is energy consumption which is increasing due to the utilization of machinery in production and transport activities, agricultural igniting procedure, soared demand for unprocessed materials, raised use of land along with dangerous cooling and heating mechanisms. Therefore, environmental sustainability is the need of the hour. Thus, now a days, biological farming is getting attention as it also enhances ecological standards by reducing the part of bug spray and reduces the amount of high-energy feed (Chien et al., 2022; Zhou et al., 2022) Therefore, agricultural activity need to be based on green sustainable methods in order to reduce CO2 emissions. In this research, the main focus is on CO2 emissions due to agricultural activities. The main motivation of this research is based on the campaigns about green agricultural practices, climate change awareness efforts, and efforts for reducing CO2 emissions due to agricultural processes. Although past studies have investigated the link between CO2 emissions and agriculture but they fail to consider the moderating effect of transportation. The contributions of this study are manifold, first, this study aims to examine do agriculture activities impact on CO2 emissions under the moderating effect of transportation which will help stakeholders and policy makers in improving the agriculture sector by designing appropriate policies. Second, this study will provide in-depth insights to researchers and practitioners about how livestock and crop production can be enhanced. Third, the findings of this research will provide crucial strategies which other countries can follow to achieve agricultural sustainability along with a decrease in CO2 emissions.
The necessity to reduce global warming and CO2 emissions has received much attention from all countries across the globe. Therefore, the government and policy makers has called for empirical studies and practical measures to achieve sustainable environment. This issue of sustainability is the main priority for environmentalists, researchers, policy makers and government. However, it seems nearly impossible to achieve the targeted goals because of the increase in economic growth that degrades the environment due to various activities like agriculture, forestry and manufacturing products. In view of past studies, the existing research on environmental quality has considered a number of key variables that can influence the quality of the environment, most notably urbanization, globalization, tourism, industrialization, direct expenditures, literacy, public expenses, and inclusive finance (Usman et al., 2022). However, there is a dearth of literature about the moderating role of transportation. Therefore, this research mainly focuses on providing new perspective on the existing environment literature. This research seeks to provide answers to following key research questions: 1) Is there any effect of agricultural activities on environmental degradation in China? 2) What sort of moderating effect do transportation has on the relationship between agriculture processes and CO2 emissions ? 3) What is the role of transportation sector in increasing CO2 emissions in China ? In this way this research aims to recommend policymakers about pragmatic initiatives needed in the agricultural sector of China.
This study will pursue three precise objectives to fill the existing research gaps in the literature. The first goal is to evaluate the role of transportation in figuring out the carbon dioxide emissions of the transport sector. The second objective is to empirically examine the effect of agricultural productivity on carbon dioxide emissions. The study’s third and last goal is to calculate the transportation sector’s emissions of CO2. In this paper, we explicitly include transportation in imagining the relationship with carbon emissions.
In the past, several experimental studies have documented the relationship between agricultural productivity and emissions of carbon dioxide, and these studies have been discussed below. However, literature has shown a dearth of studies taking transportation as the moderator. It is generally accepted i.e. there is a relationship between agricultural productivity, CO2 discharge with the subsequent temperature change.
As per literature, the agriculture accounts for a quarter to a third of worldwide CO2 emissions (World Bank, 2013; Pickson et al., 2020). Agricultural activities account for about one-tenth to 12th percentage of total global emissions. Meanwhile, land use and land cover converted to agriculture account for about twelve-twenty % of worldwide carbon discharges (Villoria & Nelson, 2019). Agriculture’s share of worldwide carbon dioxide emissions is relatively smaller than that of the thermodynamic industry. To decrease carbon dioxide emissions due to agricultural activity, there is a high demand to introduce low-carbon farming methods that encourage economic development and safeguard the environment, (Dufour et al., 2009; Zhou et al., 2022; Jiang et al., 2020; Koondhar et al., 2020).
In another study, Reynolds et al. (2015) determined the link between agronomical product yield and CO2 emissions for South Asia and sub-Saharan Africa. The model estimates confirm a link between agricultural yield and CO2 emissions. Likewise Wang H. et al. (2020) studied the effect of crops, crop byproducts, and harvesting procedures on CO2 discharges. This research hypothesizes that harvest residues either raise CO2 discharges or degrade environmental quality. Similarly a study found that the effects of imitated CO2 emissions effects agriculture production and household well-being (Edoja et al., 2016). The simulation results of this study admitted the negative correlation between CO2 emissions and agricultural productivity. The relationship between climate change and the production of agriculture, and the difference in agricultural production to global warming was found smaller than the response of production to CO2 fertilization are determinant by (Nwaka et al., 2020).
Since a few decades, China has been gradually improving as a country. Several agricultural machinery subsidies tend to promote agricultural progression in the Chinese Government. As global warming becomes more serious, actual CO2 emissions have become a key research field in recent years (Shin et al., 2014, Zeng et al., 2019; Zhu et al., 2021). Through photosynthesis (plants) agriculture contributes to reduce CO2 emissions on one hand, while on the one hand, it adds to its metabolism and endurance from the energy-related CO2 discharges that are unavoidable in the production process because input elements lead to increased CO2 emissions. A study by Yang et al. (2020a) discussed the energy linked CO2 emissions due to using coal and electricity. Another study highlighted that CO2 emissions are linked to the utilization of energy per unit (Chi et al., 2021) and it is also found that CO2 emissions has a strong relationship with the gross domestic product (Wang R. et al., 2020; Yan et al., 2020; Aslam et al., 2021). Considering these facts, based on total factor output and factor replacement, the data envelopment analysis (DEA) method is broadly applied to measure environmental and energy performance, especially carbon emission performance.
Agricultural production extensively uses machinery and transportation that preoccupies bio-fuels and releases carbon emissions (Sardar, 2022). Transport and transport exercise in the sector of agriculture, such as elevating crops, animal husbandry, forest ranging, fishing, sources of pollution of the environment leads to the emission of CO2 (Gomez et al., 2020). Machines used in agriculture, for instance land tilling, yielding crops, and groundwater pumping, emit CO2 (Rahman et al., 2021). Transport of agricultural products, livestock and fishing, forest timber, etc. Are primarily done using bio-fuel-based modes of transport, causing pollution through carbon emissions (Zhan et al., 2018). Two types of emissions are seen in agricultural production processes (Yang et al., 2018). This includes direct and indirect discharges of CO2 gases. Direct carbon emissions arise from the use of fossil fuels in various agricultural and transportation activities (Bhatti et al., 2021). While the indirect carbon emissions are due to the various processes used in agricultural activities. The carbon emissions that are embodied are larger than those produced in course of production of agriculture (Niu et al., 2020; Khursheed et al., 2021). In addition, emissions of CO2 released during international and domestic transport of agricultural activities are an important part of CO2 emissions (Wang et al., 2019).
In this research, transport competitiveness adjustment is used as a moderator to determine changes in agricultural trends. This suggests that the high competitiveness of the transport sector plays a value-adding role for the agricultural sector in reducing CO2 emissions in the transport sector. This research has the theoretical underpinning of the environmental Kuznets curve (EKC). This theory is selected because it is used in several past empirical studies. This theory is also validated by several past researchers (Hanif, 2018; Sharma et al., 2021). This research using the theoretical foundation of EKC proposes that agricultural productivity effects on CO2 emissions and the transportation positively moderates this relationship. Based on the literature reviewed, we propose the following hypothesis;
H1: A positive relationship exists between agricultural productivity and CO2 emissions.
H2: Transportation moderates the relationship between agricultural productivity and CO2 emissions.
The purpose of the study described above is to identify whether transportation has a moderating effect on agricultural productivity and carbon emissions. In order to test the relationship between study variables, the ARDL method was applied to time series data spanning 1991 to 2019. World Development Indicators (http://data.worldbank.org) provided the data sets of the selected variable for this study in order to accomplish the study objectives. Here, CO2 emissions represent a dependent variable, and livestock production represents an independent variable. Table 1 provides a comprehensive overview of the variables, their definitions, and symbols, as well as the source of the data and the descriptive analysis. Kilotons are used to measure CO2 emissions. Based on 2014–2016 = 100, the livestock and crop production indices are calculated. From Figure 1 to Figure 3, it is illustrated that there has been a trend in the variables, LP, CO2, CP, and TP in China during the period 1990 to 2019.
Figure 2 shows the trend in variables (i.e., CO2, LP, CP, and TP) as a function of time. As shown in Figure 4 and Table 1 contain all the details regarding the variables and sources of the data in regard to the description of the variables.
Most observed and hypothetical studies consider the agricultural and transport sectors the most important factors affecting CO2 emissions (Ullah et al., 2020). An important source of income, agriculture, has contributed to increased environmental pollution. As the agricultural sector subsidizes to economic development, it is also responsible for the emission of CO2 and environmental deprivation from land use, deforestation and livestock use of machinery, fertilizers, stump burning and fossil fuels. An analysis of the impact of AP on the environment is conducted using Borlaug’s hypothesis (Angalsen et al., 2001). This hypothesis suggests that the early stages of AP reduce eco-friendly value. As a result of agricultural productivity, environmental value is enhanced through increased demand for goods and services produced per environmental regulations. In general, the econometric model of our study can be characterized as follows:
Where CO2t represents CO2 emissions, APt represents agricultural productivity, t denotes time series and ε is the error term. The estimate of β1 is likely to lead to the conclusion that agriculture productivity reduces environmental pollution by reducing the number of dirty activities in the economy (Ullah et al., 2022).
Based on the findings of this study, it is suggested that Transportation (TP) might act as a moderating factor in the case of China since it directly affects CO2. Consequently, this study seeks to discover the role of TP in moderating the effect of LP on CP and CO2 emissions. An interaction variable was presented by (Cohen et al., 2014) to check the moderating effects of the interaction variables in this setting. According to (Chen and Myagmarsuren, 2013), as a second step, we construct two models to verify moderating factors' effects. First, we added a proxy for transportation (lnTP) to Equation 2 in order to estimate the main effect as presented below:
Added interaction variables to Eq. 2 as an independent variable in the second model after which we get new Eq. 3 to evaluate transportation’s moderating effect.
An interaction variable will be considered moderating if it shows statistically significant relationships with the other variables, according to Cohen et al. (2014). The coefficients should also be statistically significant, thus confirming that transportation plays a moderating role in our study. Thus, TP is an important moderating variable in statistical methodology since it affects the direct relationship between CO2 emissions and the regressors (LP and CP).
Whenever a long-term relationship is to be estimated, it is necessary to determine the order of integration by testing the unit root of each variable. Appropriate methods for the study are determined by factors such as integration order or stationarity characteristics. In the results and discussion section, we present the results of the analyses of Dickey and Fuller (1979), Dickey and Fuller (1981) and Phillips and Perron (1988) using the ADF and PP tests, respectively. In order to answer research questions empirically, we consider the order in which our study variables are integrated. For the examination of asymmetry relationships among fundamental variables, we used recently advanced econometric methodology, namely, the autoregressive distributed lag (ARDL) bounds-testing approach to co-integration, presented by (Pesaran et al., 2001) for symmetric co-integration, and the ARDL bounds-testing approach. A variety of techniques have been used to investigate long-run associations between variables in literature in the related field (Engle and Granger, 1987; Johansen, 1991), but as indicated in a recent study (Liu et al., 2020) and based on the following superior characteristics, this technique has been selected: The use of ARDL models is recommended only when the underlying variables have mixed integration orders of I (0), and I (1), and none of the series have been integrated at the second level. Alternatively, other conventional co-integration methods are limited to series in the I (0) and I (1) states (Pesaran et al., 2001; Satti et al., 2014). The second benefit of the ARDL framework is its ability to provide efficient results, as it is free of serial correlations and endogeneity issues, as well as when endogenous explanations are present (Liu et al., 2020). Lastly, both techniques produce solid results, even when the sample size is small (Hsueh et al., 2013).
Agriculturalization leads to a shift from green to blue economic growth. We can estimate CO2 emissions in the long run using Eq. 4. We will then assemble our model into an error-correction format, which will enable us to assess agricultural productivity over the short and long term using the same equation. According to (Pesaran et al., 2001), the authors describe the ARDL bounds testing approach for exploring long-run and short-run linear relationships in Eq. 4.
Calculating lags in the short and long run requires consideration of several factors: 1) the first difference operator, 2) the intercept or continuous parameter, 3) the optimal number of lags, 4) and 5) the short and long-run coefficients. An alternative hypothesis of linear co-integration among variables (H0: γ1 = γ2 = γ3 = γ4 = 0) is compared with the null hypothesis of no co-integration among the underlying variables (H0: γ1 = γ2 = γ3 = γ4 ≠ 0). Testing for the null hypothesis of no co-integration among the underlying variables is conducted by applying the ARDL bounds approach. These hypotheses are tested based on the nonstandard F-test proposed by (Pesaran et al., 2001), famously known as FPSS. The authors provide critical bound values at different levels of significance. A null hypothesis that no co-integration exists is rejected if the calculated value of the test statistics is higher than the upper bound’s critical value. The null hypothesis is accepted if the test statistics decrease below the inferior bound critical value. We could conduct another co-integration test if the test statistics fall between the upper and lower bound critical values. Additionally, ARDL assumes that all variables explain the dependent variable in an asymmetric manner.
All variables in the study are described in Table 2, including mean and standard deviation, maximum and minimum values, and p-values for Cramer von Mises, Shapiro-Wilk, and anderson Darling tests. As a result, Co2 and both interaction variables of AP have significantly higher means. In both the interaction terms of AP and the standard deviation of Co2 there is a high level of volatility compared to the rest of the variables.
The results in Table 3 indicate that TP correlates negatively with CO2, LP, and CP. On the other hand, it has a positive correlation with the other variables. The moderating term (I. LP, CP) correlates positively with the other variables, except for the correlation of I. LP with LP.
We must ensure that our variables are stationary when using time series data. This is because estimations based on non-stationary data can be misleading. Therefore, before we perform long-run estimations (through ARDL), we apply the unit root test to ensure that variables involved are stationary. Table 4 presents the unit root test results as described by (Ng and Perron, 2001).
In Table 5, it is stated that the ARDL bounds test could only be applied to variables with mixed integration orders I (0) or I (1). We used the ADF test, developed by (Dickey and Fuller, 1978; Dickey and Fuller, 1981), as well as the PP test, developed by (PHILLIPS and PERRON, 1988) to determine the stationary properties of the variables; the results are presented in Table 5. Based on this, both LP, I. LP, the interactive term (i.e. the interaction variable that results from multiplying the TP and I. LP in order to examine the moderating effect of TP), CO2, and CP are non-stationary at the level, however, as per ADF, they are stationary when the first difference is taken. ADF results are also confirmed by the PP test. At both the level and the first difference, the TP and LP are found to be stationary. The results obtained from unit roots are supported by both tests, demonstrating their robustness.
Based on the results of the linear ARDL bound test on the stationarity of the variables, first, we applied the co-integration test using the linear ARDL bound test, which is shown in Table 5. In all models from Equations (1)–(3), ARDL F-statistics are greater than the upper bond critical values given by (Pesaran et al., 2001) at all significance levels 1%, 5%, and 10%. In the case of China, the co-integration between the AP, the Co2, the TP, and the interactive term was established over the period of study. A diagnostic and stability test was performed to ensure the robustness of the results; as a result, ARDL bounds test of (Pesaran et al., 2001) proved to be robust, reliable, and useful for the formulation of policy recommendations. This study also demonstrates a strong long-term co-integration among variables (significant F-statistics) found in the linear ARDL model (Table 5).
Also, we apply some robust analysis to the diagnostic test, and the results are also provided at the bottom of Table 5. It is demonstrated that the residuals are normally distributed in the BJarque-Bera test, BD-Watson test, and BAmsey’s RESET test that the data is homoscedastic under the given model, whereas BD-Watson test reveals no autocorrelation. Finally, the BAreush Pagan Godfrey test demonstrates that data is homoscedastic within the given model.
ARDL model is implemented in a suitable manner using the cumulative sum of the square of recursive residuals (CUSUMsq) tests, as Brown 1976) suggested. As shown in Figure 5, the CUSUMsq test graphs lie within the red colored critical bounds at a 5% confidence interval. The blue line in the middle of the graph represents the measurements of the cumulative sum of the squares of the residuals. According to the CUSUMsq graphs, our model appears to be well-stable.
Using ARDL approach, Table 6 provides the results of short-run and long-run estimations. Carbon dioxide emissions (CO2) in panel 1) are positively and statistically significantly related to livestock productivity and transportation, while negatively and statistically significantly related to crop productivity. In the short run, the coefficient of LP is 32907.8, while in the long run, the coefficient is 4829.8. This indicates that a 1% increase in LP will result in a 98% increase in carbon emissions per capita.
Table 6, also provides the results of the estimation with interaction variables (see panel b). The analysis results are still significant after the inclusion of interaction variables, except livestock productivity (LP). The coefficient of crop productivity is significant but its sign is negative (β = -13890.14, p < 0.05). Further, when interaction terms (variables) are included in the analysis, LP and CP have a greater impact on carbon emissions than before. The main difference between model 1) and model 2) is that the inverted U-shaped relationship has disappeared and the interaction variables (lnLP x TP and CP x TP) have become statistically significant. It is evident that Transportation acts as a moderator in the relationship between Agriculture Productivity (AP) and Carbon Dioxide (CO2). Nasir and Rehman found similar results as described in Table 6. Overall, the long-run results suggest that transportation contributes significantly to CO2 emissions as a moderator.
In the second portion of Table 6, the short-run estimates are presented for both cases: the main effect (without an interaction term) and the main effect with an interaction or moderating effect. Crop productivity error correction terms are statistically significant at 1% and have a negative coefficient.
To determine the robustness of the model, the pairwise Granger causality test is estimated between two variables simultaneously, which elaborates the directional linkages between them. In Table 7, we demonstrate the results of the pairwise Granger causality analysis. The estimations of the pairwise Granger causality shows unidirectional causality between LnLP to LnCO2, LnLP to LnCP, ln (LP*TP) to LnCO2, Ln (CP*TP) to LnCO2, lnLP to Ln (CP*TP) and LnTP to Ln (CP*TP).
China is one of those countries which are progressively improving its agricultural methods for achieving environmental sustainability. Further, China produces energy from coal, a non-renewable source, this research recommends using a renewable source to control CO2 emissions. Further, as per Abdoli et al. (2018) latest biofuels, including biogas and other agricultural deposits such as hazelnut, wheatgrass etc, are the key source of renewable energy in the agriculture sector. Therefore, the increase in global warming due to CO2 emissions is one of the most investigated research topics. Although several studies have analyzed the factors leading to pollution, in this research a new comprehensive framework is developed and tested on Chinese economy. The findings of this study reveal a significant relationship between agriculture and CO2 emissions under the moderating effects of transportation. Thus, this study recommends that the government of China should organize trainings for farmers regarding the latest harvesting techniques. This study’s results give policymakers direction to focus more on research and development activities. This study also has a few limitations, including limited inclusion of variables and sample size. Thus, future researchers can increase the variables with a huge sample to analyze this key relationship. As the effects of agricultural productivity on CO2 may differ among different economies. Further, all categories of green activities can reduce the level of CO2 emissions as the energy generally used in the agriculture sector is highly dependent on fossil fuels. Therefore, the agriculture sector of China should increase the photosynthesis process to increase the oxygen supply for the environmental sustainability. Thus, organic farming will not only positively affect human health but also help environmental sustainability. This study also deserve consideration from policy makers in China for designing policies regarding the significance of transportation in understanding the relationship between agricultural activities and CO2 emissions. Additionally, the policy makers should design policies to create the awareness about the necessity of decreasing CO2 emissions through the understanding the importance agriculture processes. Thus, it is crucial to develop the rural areas focused on modern and green agriculture policies. Therefore, this study will help policy makers in designing more suitable policies for reducing the emissions of CO2 considering the characteristics of that country.
In this study, the impact of agricultural activity on CO2 emissions is investigated under the moderating effects of transportation. For the empirical results, we have used very widely applicable econometric methodology, ARDL applied on a data from 1991 to 2019. For the presentation of agricultural productivity, crop and livestock production are used. The findings revealed that the crop production negatively affects carbon dioxide emissions in the long and short run. While livestock production positively affects the carbon dioxide emissions and negatively in the short run, the moderator (transportation) shares a negative relationship with the dependent variable, that is CO2. Therefore, both hypotheses are accepted. As a result, the Granger Causality test confirms that agricultural productivity contributes to CO2 emissions in a bidirectional fashion, as opposed to the one-way causality between transportation and CO2, which does not show a causal relationship with CO2. Hence, the findings of this study reveal a significant relationship between agriculture and CO2 emissions under the moderating effects of transportation.
In case of with moderation effect, the results reveal a significant and positive moderation effect of transportation on the relationship between agricultural productivity and CO2 in the long and short run. This result is consistent with past studies (Adams et al., 2020; Kocak et al., 2020). Thus, the results highlight the significant role of agricultural productivity, and transportation in understanding the environmental degradation due to CO2 and the research objectives are achieved. The study concludes with highlighting significant role of agriculture and transportation in understating the control on CO2 emissions. Further, the study also provides empirical implications, as the moderation of transportation confirms its effect on the relationship between agricultural activity and CO2 emissions. Therefore, this study recommends that government should strengthen the control of transportation and should regulate the use of green agricultural methods. Further, if the grip on transportation is strengthened then CO2 emissions will be reduced leading to achieve environmental sustainable goals. In the presence of strict government regulations, green agricultural practices should be adopted and resultantly, the CO2 emissions will be decreased. This study gives a new perspective to researchers and practitioners for understanding the significance of reducing CO2 emissions by considering the role of agriculture sector along with transportation sector. Thus, this study recommends that China should adopt organic farming techniques and the latest technological and innovative farming techniques and the farmers should be introduced to new farming techniques.
The raw data supporting the conclusions of this article will be made available by the authors, without undue reservation.
DW wrote the paper draft and collected the data. RH helped in formatting and also managed statistical issues. IA ensured grammar checks and overall structure of the paper.
The authors declare that the research was conducted in the absence of any commercial or financial relationships that could be construed as a potential conflict of interest.
All claims expressed in this article are solely those of the authors and do not necessarily represent those of their affiliated organizations, or those of the publisher, the editors and the reviewers. Any product that may be evaluated in this article, or claim that may be made by its manufacturer, is not guaranteed or endorsed by the publisher.
Abdoli, M. A., Golzary, A., Hosseini, A., and Sadeghi, P. (2018). “Biomass as a source of energy,” in Wood pellet as a renewable source of energy (Cham: Springer), 1–31. doi:10.1007/978-3-319-74482-7_1
Adams, S., Boateng, E., and Acheampong, A. O. (2020). Transport energy consumption and environmental quality: Does urbanization matter? Sci. Total Environ. 744, 140617. doi:10.1016/j.scitotenv.2020.140617
Aslam, B., Hu, J., Shahab, S., Ahmad, A., Saleem, M., Shah, S. S. A., et al. (2021). The nexus of industrialization, GDP per capita and CO2 emission in China. Environ. Technol. Innov. 23, 101674. doi:10.1016/j.eti.2021.101674
Barroso, L. R. (2020). Technological revolution, democratic recession, and climate change: The limits of law in a changing world. Int. J. Const. Law 18 (2), 334–369. doi:10.1093/icon/moaa030
Bhatti, U. H., Sultan, H., Min, G. H., Nam, S. C., and Baek, I. H. (2021). Ion-exchanged montmorillonite as simple and effective catalysts for efficient CO2 capture. J. Chem. Eng. 413, 127476. doi:10.1016/j.cej.2020.127476
Chen, C.-F., and Myagmarsuren, O. (2013). Exploring the moderating effects of value offerings between market orientation and performance in tourism industry. Int. J. Tour. Res., 15(6), 595–610. doi:10.1002/jtr.1900
Chi, Y., Zhou, W., Wang, Z., Hu, Y., and Han, X. (2021). The influence paths of agricultural mechanization on green agricultural development. Sustainability 13 (23), 12984. doi:10.3390/su132312984
Chien, F., Hsu, C. C., Ozturk, I., Sharif, A., and Sadiq, M. (2022). The role of renewable energy and urbanization towards greenhouse gas emission in top Asian countries: Evidence from advance panel estimations. Renew. Energy 186, 207–216. doi:10.1016/j.renene.2021.12.118
Cohen, J. F. W., Richardson, S., Parker, E., Catalano, P. J., and Rimm, E. B. (2014). Impact of the new U.S. department of agriculture school meal standards on food selection, consumption, and waste. Am. J. Prev. Med. 46 (4), 388–394. doi:10.1016/j.amepre.2013.11.013
Dickey, D. A., and Fuller, W. A. (1979). Distribution of the estimators for autoregressive time series with a unit root. J. Am. Stat. Assoc. 74 (366a), 427–431. doi:10.1080/01621459.1979.10482531
Dickey, D. A., and Fuller, W. A. (1981). Likelihood ratio statistics for autoregressive time series with a unit root. Econometrica 49 (4), 1057–1072. doi:10.2307/1912517
Dufour, S., and Piégay, H. (2009). From the myth of a lost paradise to targeted river restoration: forget natural references and focus on human benefits. River. Res. Appl. 25 (5), 568–581. doi:10.1002/rra.1239
Edoja, P. E., Aye, G. C., and Abu, O. (2016). Dynamic relationship among CO2 emission, agricultural productivity and food security in Nigeria. Cogent Econ. Finance 4 (1), 1204809. doi:10.1080/23322039.2016.1204809
Engle, R. F., and Granger, C. W. (1987). Co-Integration and error correction: Representation, estimation, and testing. Econometrica 55 (2), 251–276. doi:10.2307/1913236
Fei, L., Dong, S., Xue, L., Liang, Q., and Yang, W. (2011). Energy consumption-economic growth relationship and carbon dioxide emissions in China. Energy policy 39 (2), 568–574. doi:10.1016/j.enpol.2010.10.025
Gomez-Zavaglia, A., Mejuto, J. C., and Simal-Gandara, J. (2020). Mitigation of emerging implications of climate change on food production systems. Food Res. Int. 134, 109256. doi:10.1016/j.foodres.2020.109256
Hanif, (2018). Impact of economic growth, nonrenewable and renewable energy consumption, and urbanization on carbon emissions in Sub-Saharan Africa. Environ. Sci. Pollut. Res. 25 (15), 15057–15067. doi:10.1007/s11356-018-1753-4
Hsueh, S.-J., Hu, Y.-H., and Tu, C.-H. (2013). Economic growth and financial development in asian countries: A bootstrap panel granger causality analysis. Econ. Model., 32, 294–301. doi:10.1016/j.econmod.2013.02.027
Jebli, M. B., Farhani, S., and Guesmi, K. (2020). Renewable energy, CO2 emissions and value added: Empirical evidence from countries with different income levels. Struct. Change Econ. Dyn. 53, 402–410. doi:10.1016/j.strueco.2019.12.009
Jiang, H., Xu, X., Guan, M., Wang, L., Huang, Y., and Jiang, Y. (2020). Determining the contributions of climate change and human activities to vegetation dynamics in agro-pastural transitional zone of northern China from 2000 to 2015. Sci. Total Environ. 718, 134871. doi:10.1016/j.scitotenv.2019.134871
Johansen, S. (1991). Estimation and hypothesis testing of cointegration vectors in Gaussian vector autoregressive models. Econometrica 59 (6), 1551–1580. doi:10.2307/2938278
Kausar, A., Siddiqui, F., Gadhi, A. K., Ullah, S., and Ali, O. (2022). Measuring the relationship between energy consumption and production of the selected SAARC countries: Panel co-integration and causality analysis. Int. J. Energy Sect. Manag. 16 (2), 284–301. doi:10.1108/IJESM-11-2020-0008
Khan, S. A. R., Ponce, P., and Yu, Z. (2021). Technological innovation and environmental taxes toward a carbon-free economy: An empirical study in the context of COP-21. J. Environ. Manag. 298, 113418. doi:10.1016/j.jenvman.2021.113418
Khursheed, A., Mustafa, F., and Akhtar, A. (2021). Investigating the roles of meteorological factors in COVID-19 transmission in Northern Italy. Environ. Sci. Pollut. Res. 28 (35), 48459–48470. doi:10.1007/s11356-021-14038-7
Koçak, E., Ulucak, R., and Ulucak, Z. Ş. (2020). The impact of tourism developments on CO2 emissions: An advanced panel data estimation. Tour. Manag. Perspect. 33, 100611. doi:10.1016/j.tmp.2019.100611
Koondhar, M. A., Li, H., Wang, H., Bold, S., and Kong, R. (2020). Looking back over the past two decades on the nexus between air pollution, energy consumption, and agricultural productivity in China: A qualitative analysis based on the ARDL bounds testing model. Environ. Sci. Pollut. Res. 27 (12), 13575–13589. doi:10.1007/s11356-019-07501-z
Liu, H., Islam, M. A., Khan, M. A., Hossain, M. I., and Pervaiz, K. (2020). Does financial deepening attract foreign direct investment? Fresh evidence from panel threshold analysis. Res. Int. Bus. Finance, 53, 101198. doi:10.1016/j.ribaf.2020.101198
Lu, Y., Yuan, J., Lu, X., Su, C., Zhang, Y., Wang, C., et al. (2018). Major threats of pollution and climate change to global coastal ecosystems and enhanced management for sustainability. Environ. Pollut. 239, 670–680. doi:10.1016/j.envpol.2018.04.016
Nathaniel, S. P., Murshed, M., and Bassim, M. (2021). The nexus between economic growth, energy use, international trade and ecological footprints: The role of environmental regulations in N11 countries. Energy Ecol. Environ. 6 (6), 496–512. doi:10.1007/s40974-020-00205-y
Ng, S., and Perron, P. (2001). LAG length selection and the construction of unit root tests with good size and power. Econometrica 69 (6), 1519–1554. doi:10.1111/1468-0262.00256
Nie, Y., Pritchard, H. D., Liu, Q., Hennig, T., Wang, W., Wang, X., et al. (2021). Glacial change and hydrological implications in the Himalaya and Karakoram. Nat. Rev. Earth Environ. 2 (2), 91–106. doi:10.1038/s43017-020-00124-w
Niu, J., Guo, F., Ran, J., Qi, W., and Yang, Z. (2020). Methane dry (CO2) reforming to syngas (H2/CO) in catalytic process: From experimental study and DFT calculations. Int. J. Hydro. Energy 45 (55), 30267–30287. doi:10.1016/j.ijhydene.2020.08.067
Nwaka, I. D., Nwogu, M. U., Uma, K. E., and Ike, G. N. (2020). Agricultural production and CO2 emissions from two sources in the ECOWAS region: New insights from quantile regression and decomposition analysis. Sci. Total Environ. 748, 141329. doi:10.1016/j.scitotenv.2020.141329
Pesaran, M. H., Shin, Y., and Smith, R. J. (2001). Bounds testing approaches to the analysis of level relationships. J. Appl. Econ. Chichester. Engl., 16(3), 289–326. doi:10.1002/jae.616
Phillips, P. C. B., and Perron, P. (1988). Testing for a unit root in time series regression. Biometrika 75 (2), 335–346. doi:10.1093/biomet/75.2.335
Pickson, R. B., He, G., Ntiamoah, E. B., and Li, C. (2020). Cereal production in the presence of climate change in China. Environ. Sci. Pollut. Res. 27 (36), 45802–45813. doi:10.1007/s11356-020-10430-x
Rahman, M. M., Aravindakshan, S., Hoque, M. A., Rahman, M. A., Gulandaz, M. A., Rahman, J., et al. (2021). Conservation tillage (CT) for climate-smart sustainable intensification: Assessing the impact of CT on soil organic carbon accumulation, greenhouse gas emission and water footprint of wheat cultivation in Bangladesh. Environ. Sustain. Indic. 10, 100106. doi:10.1016/j.indic.2021.100106
Reynolds, P. D. (2015). Business creation stability: Why is it so hard to increase entrepreneurship? foundations and trends® in entrepreneurship. 10 (5-6), 321–475. doi:10.1561/0300000058
Sardar, M. S., and Rehman, H. u. (2022). Transportation moderation in agricultural sector sustainability—A robust global perspective. Environ. Sci. Pollut. Res. 29, 60385–60400. doi:10.1007/s11356-022-20097-1
Satti, S. L., Farooq, A., Loganathan, N., and Shahbaz, M. (2014). Empirical evidence on the resource curse hypothesis in oil abundant economy. Econ. Model., 42, 421–429. doi:10.1016/j.econmod.2014.07.020
Sharma, R., Sinha, A., and Kautish, P. (2021). Does renewable energy consumption reduce ecological footprint? Evidence from eight developing countries of Asia. J. Clean. Prod. 285, 124867. doi:10.1016/j.jclepro.2020.124867
Shin, Y., Yu, B., and Greenwood-Nimmo, M. (2014). “Modelling asymmetric Co-integration and dynamic multipliers in a nonlinear ARDL framework,” in Festschrift in honor of peter schmidt: Econometric methods and applications. Editors R. C. Sickles, and W. C. Horrace (New York: Springer), 281–314. doi:10.1007/978-1-4899-8008-3_9
Ullah, A., Arshad, M., Kächele, H., Khan, A., Mahmood, N., and Müller, K. (2020). Information asymmetry, input markets, adoption of innovations and agricultural land use in Khyber Pakhtunkhwa, Pakistan. Land Use Policy 90, 104261. doi:10.1016/j.landusepol.2019.104261
Usman, M., Balsalobre-Lorente, D., Jahanger, A., and Ahmad, P. (2022). Pollution concern during globalization mode in financially resource-rich countries: Do financial development, natural resources, and renewable energy consumption matter? Renew. Energy 183, 90–102. doi:10.1016/j.renene.2021.10.067
Villoria, N. (2019). Technology spillovers and land use change: Empirical evidence from global agriculture. Am. J. Agric. Econ., 14(12), 870. doi:10.1093/ajae/aay088
Wang, H., Wang, S., Yu, Q., Zhang, Y., Wang, R., Li, J., et al. (2020a). No tillage increases soil organic carbon storage and decreases carbon dioxide emission in the crop residue-returned farming system. J. Environ. Manag. 261, 110261. doi:10.1016/j.jenvman.2020.110261
Wang, R., Mirza, N., Vasbieva, D. G., Abbas, Q., and Xiong, D. (2020b). The nexus of carbon emissions, financial development, renewable energy consumption, and technological innovation: What should be the priorities in light of COP 21 agreements? J. Environ. Manag. 271, 111027. doi:10.1016/j.jenvman.2020.111027
Wang, S. L., Huang, J., Wang, X., and Tuan, F. (2019). Are China's regional agricultural productivities converging: How and why? Food Policy 86, 101727. doi:10.1016/j.foodpol.2019.05.010
World Bank (2013). Turn down the heat: Climate extremes, regional impacts, and the case for resilience.
Yan, Z., Zou, B., Du, K., and Li, K. (2020). Do renewable energy technology innovations promote China's green productivity growth? Fresh evidence from partially linear functional-coefficient models. Energy Econ. 90, 104842. doi:10.1016/j.eneco.2020.104842
Yang, D., Liu, Y., Cai, Z., Chen, X., Yao, L., and Lu, D. (2020). First global carbon dioxide maps produced from TanSat measurements. Adv. Atmos. 35 (6), 621–623. doi:10.1007/s00376-018-7312-6
Yang, Z., Li, L., Yuan, H., Dong, Y., Liu, K., Lan, L., and Fang, Y. (2020a October). Evaluation of smart energy management systems and novel UV-oriented solution for integration, resilience, inclusiveness and sustainability, Proceedings of the 2020 5th International Conference on Universal Village (UV), Boston, MA, USA, IEEE, 1–49. doi:10.1109/UV50937.2020.9426217
Zeng, Y., Cao, Y., Qiao, X., Seyler, B. C., and Tang, Y. (2019). Air pollution reduction in China: Recent success but great challenge for the future. Sci. Total Environ. 663, 329–337. doi:10.1016/j.scitotenv.2019.01.262
Zhan, B. J., Xuan, D. X., and Poon, C. S. (2018). Enhancement of recycled aggregate properties by accelerated CO2 curing coupled with limewater soaking process. Cem. Concr. Compos. 89, 230–237. doi:10.1016/j.cemconcomp.2018.03.011
Zhang, L., Ruiz-Menjivar, J., Luo, B., Liang, Z., and Swisher, M. E. (2020). Predicting climate change mitigation and adaptation behaviors in agricultural production: A comparison of the theory of planned behavior and the value-belief-norm theory. J. Environ. Psychol. 68, 101408. doi:10.1016/j.jenvp.2020.101408
Zhou, G., Li, H., Ozturk, I., and Ullah, S. (2022). Shocks in agricultural productivity and CO2 emissions: New environmental challenges for China in the green economy. Economic Research-Ekonomska Istraživanja, United Kingdom, 1–17. doi:10.1080/1331677X.2022.2037447
Keywords: CO2 emission, transportation, ARDL, agriculture, productivity
Citation: Wang D, Hussain RY and Ahmad I (2023) Nexus between agriculture productivity and carbon emissions a moderating role of transportation; evidence from China. Front. Environ. Sci. 10:1065000. doi: 10.3389/fenvs.2022.1065000
Received: 09 October 2022; Accepted: 02 November 2022;
Published: 04 January 2023.
Edited by:
Zeeshan Fareed, Huzhou University, ChinaReviewed by:
Elchin Suleymanov, Baku Enginering University, AzerbaijanCopyright © 2023 Wang, Hussain and Ahmad. This is an open-access article distributed under the terms of the Creative Commons Attribution License (CC BY). The use, distribution or reproduction in other forums is permitted, provided the original author(s) and the copyright owner(s) are credited and that the original publication in this journal is cited, in accordance with accepted academic practice. No use, distribution or reproduction is permitted which does not comply with these terms.
*Correspondence: Rana Yassir Hussain, eWFzc2lyLmh1c3NhaW5AdWUuZWR1LnBr
Disclaimer: All claims expressed in this article are solely those of the authors and do not necessarily represent those of their affiliated organizations, or those of the publisher, the editors and the reviewers. Any product that may be evaluated in this article or claim that may be made by its manufacturer is not guaranteed or endorsed by the publisher.
Research integrity at Frontiers
Learn more about the work of our research integrity team to safeguard the quality of each article we publish.