- 1National Research Council, Water Research Institute (CNR IRSA), Verbania Pallanza, Italy
- 2Department of Earth and Environmental Sciences DSTA, University of Pavia, Pavia, Italy
Lake Maggiore is a site of the Long-Term Ecosystem Research (LTER) network, belonging to the deep subalpine Lake District in Northern Italy. Studies on the physical, chemical, and biological features of the lake have been performed continuously since the 1980s. The lake recovered from eutrophication reaching the present oligotrophic condition. In the last decade, climate change represents the main driving factor for the long-term evolution of the lake, affecting its hydrodynamics, nutrient status, and biological communities. In 2020 a high-frequency monitoring (HFM) system was deployed, with the aim to integrate long-term monitoring based on discrete sampling and analysis. The system consists of a buoy equipped with sensors for limnological variables and algal pigments. The high-frequency monitoring program is part of a cross-border project between Italy and Switzerland focusing on lake quality monitoring as a critical input for successful lake management. In this paper we focus on Chlorophyll-a data, with the aim to test whether in-situ fluorescence measurements may provide a reliable estimate of lake phytoplankton biovolume and its seasonal dynamic. Sensor’s performance was regularly tested comparing chlorophyll-a data taken by the in-situ fluorescent sensors (Cyclops7, Turner Design), data from laboratory fluorescence analysis (FluoroProbe, BBE Moldaenke), values obtained from chlorophyll-a analysis by UV-VIS spectrophotometry and data from phytoplankton microscopy analysis. We found a general good agreement between the Chlorophyll-a data obtained with the different methods, confirming the use of in-situ sensors as a reliable approach to measure algal pigments, especially to assess their variability in the short-term, but also to describe the seasonal pattern of phytoplankton biovolume. However, phytoplankton community composition played a substantial role in the performance of the different methods and in the reliability of in-situ data as a tool to assess algal biovolume. This study demonstrates that high-frequency monitoring (HFM), used in conjunction with discrete chemical and biological monitoring, represents an important advance and support in the long-term monitoring of freshwaters and is a useful tool to detect ecological changes. Regular checking and validation of the sensor readings through laboratory analyses are important to get trustworthy data.
1 Introduction
Monitoring the surface water quality is compulsory for the current environmental policies under the EU Water Framework Directive (WFD, 2000/60/EC) (European Commission, 2000). This directive commits EU member countries to achieve good qualitative status for their water bodies and prevent further deterioration. The first step to reaching these ambitious goals is to assess the ecological status of aquatic environments through monitoring and assigning them to specific quality classes. This is central to the operation of the WFD, though the monitoring itself also has other objectives such as increasing system understanding and designing mitigation options (Skeffington et al., 2015).
Traditionally, freshwater monitoring involves fieldwork for sample collection and subsequent laboratory work. Samples are collected manually from selected waterbody stations and depth at scheduled intervals throughout the year. The amount of manual work required makes these methods costly and time-consuming. Moreover, because of the low sampling frequency, this approach often fails to capture the dynamics of biotic and abiotic processes within freshwater ecosystems. Despite the usefulness of traditional monitoring programs, the discrete nature of sampling means it is vital to fill the knowledge gaps related to short-lived, extremely episodic, or unpredictable events, and in general to any process with a characteristic temporal scale shorter than the sampling frequency (Jennings et al., 2012; Meinson et al., 2016).
The WFD requires the classification of the ecological status of surface waters in an integrative way, by using multiple taxonomic groups (biological quality elements, BQEs) together with supporting physico-chemical and hydro-morphological variables (Caroni et al., 2013). For lake monitoring, phytoplankton is one of the required BQEs to be assessed. Phytoplankton biomass is widely used as an indicator of the status assessment of surface waters (Salmaso et al., 2006; Boyer et al., 2009). Microscopy identification and enumeration of phytoplankton performed in the laboratory are essential to provide reliable data for assessing the ecological state of lakes. However, phytoplankton microscopy analysis is a time-consuming method, requiring specialized scientific personnel to determine taxonomy and to make biomass calculations of phytoplankton communities in lakes.
Chlorophyll-a (hereinafter Chl-a) concentration is often used as a proxy for phytoplankton biomass (Vörös, and Padisák, 1991; Kasprzak et al., 2008). It represents one of the key indicators of water quality for lakes, in particular concerning eutrophication-related problems (e.g., deterioration of water quality, development of algal blooms). Chl-a is traditionally quantified in the laboratory from water samples using ethanol or acetone extraction followed by spectrophotometric measurement (Lorenzen, 1967; ISO 10260, 1992). Although it is nowadays a well-established technique, Chl-a extraction protocols may have some shortcomings as they require the manual collection of large water sample volumes, sample transportation, and laboratory personnel to perform extraction and spectrophotometric analyses.
A global increase in the frequency, intensity, and duration of cyanobacterial blooms is raising concerns as many bloom-forming species produce harmful compounds that pose a risk to human and animal health (Taranu et al., 2015; Harke et al., 2016). To evaluate the threat posed by cyanobacteria bloom formations and to assist in the understanding of bloom dynamics, the biomass of cyanobacteria is required to be quantified. The identification of taxa and estimation of the abundance of cyanobacteria, as well as of pigment and cyanotoxin concentrations, are important tasks given the increase in the frequency, intensity, and duration of cyanobacterial blooms that are raising concerns worldwide. However, laboratory determination procedures for the quantification of cyanobacterial pigments, such as phycocyanin, are labour-intensive and time-consuming (Sarada et al., 1999; Randolph et al., 2008).
Laboratory analysis to assess phytoplankton biomass and algal pigments have been extensively used in long-term monitoring programs of lakes and the time series produced have contributed to extended datasets and synoptic studies across different ecosystems (e.g., Salmaso et al., 2003; Morabito et al., 2018a; Stockwell et al., 2020). However, both phytoplankton microscopy analysis and Chl-a determination in the laboratory do not allow the monitoring of lake productivity and phytoplankton variations in real time or at high frequency: these methods are indeed not able to detect accurately phytoplankton successions and bloom formation with a sufficient temporal resolution. In particular, the short-lived nature of cyanobacterial blooms makes traditional laboratory methods difficult to be used for their early detection and monitoring (Hunter et al., 2009; Stumpf et al., 2012; Bertone et al., 2018).
The limited spatial and temporal coverage of discrete monitoring methods prompted the development of alternative and complementary monitoring techniques. As regards algal pigment, during the last 2 decades, field fluorometers for in-situ measurement have become increasingly common worldwide (Marcé et al., 2016; Meinson et al., 2016). Fluorometric quantification of Chl-a and other common algal pigments such as phycocyanin and phycoerythrin is generally cost-effective and allows frequent observations during sudden phenomena such as mixing events or short-lived algal blooms (Jennings et al., 2012; Klug et al., 2012). These sensors have been incorporated into in-situ monitoring systems across the globe (McQuaid et al., 2011; Hamilton et al., 2014; Hodges et al., 2018), showing potential for assessment of phytoplankton biomass spatially and temporally.
Lake Maggiore is a deep oligomictic lake belonging to the “IT-08 Southern Alpine Lake” LTER site. The lake has been studied for its physical, chemical, and biological aspects since the 1980s. It recovered from eutrophication thanks to the reduction of catchment loads and reached a stable oligotrophic status by the end of the 1990s. Nowadays, dissolved oxygen and nutrient dynamics are mainly driven by in-lake processes, in particular, the stratification and mixing regime, which in turn are affected by climate change (Rogora et al., 2018, Rogora et al., 2021). Water temperature increased, at different rates according to depth and season, causing increased stability of the water column and a decreasing frequency of deep mixing events, the last full turnover having occurred in 2006 (Fenocchi et al., 2018). As an effect, oxygen is steadily decreasing and phosphorus, nitrate, and silica are accumulating in the deep layers, with limited replenishment of the trophogenic layers (Rogora et al., 2021). These changes are affecting phytoplankton composition and seasonal succession (Morabito et al., 2012; Tanentzap et al., 2020), with a slightly increasing trend of Chl-a concentrations and the evidence of short-lived phytoplankton blooms triggered by heavy precipitation events (Morabito et al., 2018b).
To integrate the discrete monitoring of the lake, in particular, to get information on short-term lake dynamics, an HFM of Lake Maggiore was started in 2020 within the cross-border cooperation project SIMILE (Italian acronym for “Integrated monitoring system for knowledge, protection, and valorization of the subalpine lakes and their ecosystems”). Its general objectives are the improvement/optimization of the monitoring of the deep subalpine lakes (Lugano, Maggiore, and Como) in the so-called “Insubric” region of Northern Italy and the strengthening of the coordinated management of water through an intensification of stakeholders’ participation in the processes of knowledge gain and monitoring of the water resource. The first aim of SIMILE is the testing and developing of an innovative monitoring approach, integrating satellite data, in-situ high-frequency sensor data, and user-contributed georeferenced data (Brovelli et al., 2019).
Within the SIMILE project, an assessment of the high-frequency data quality was started, with a focus on algal pigment data. This paper presents a comparison of the in-situ high-frequency measurements of Chl-a provided by the sensors with those obtained from laboratory analysis on water samples collected in 2020–21. We also measured phytoplankton abundance and composition in each sample by microscopy analysis. Finally, we aimed to critically discuss HFM as a complementary approach to the discrete monitoring of lakes, highlighting both its strong points and major drawbacks, focusing on the contribution that HFM may provide in long-term ecological research.
2 Methods
2.1 Study site
Lake Maggiore is the second deepest (370 m) and largest (212.5 km2) lake in Italy. It belongs to the subalpine lake district, and together with lakes Lugano, Como, Garda, and Iseo, it contributes to almost 80% of the total volume of freshwater in Italy. These lakes altogether are an invaluable water resource for several uses including hydroelectric power production, potabilization, irrigation, as well as they are key elements for the tourist economy of the Alpine region (Salmaso et al., 2020). Lake Maggiore is oligotrophic by nature; a eutrophication process started in the 1960s, when nutrient concentration, mainly phosphorus, started to rise, followed by an increase in phytoplankton abundance, biovolume, and primary production. After reaching a eutrophic state in the late 1970s-early 1980s, a recovery process started, thanks to the reduction of catchment loads and the lake gradually returned to an oligotrophic condition, with total phosphorus concentrations around 10 μg P L−1 (Ruggiu et al., 1998; Morabito et al., 2012). Since the late 1980s, changes in phytoplankton species diversity and composition appeared, with a decrease in average cell size, Chl-a, and total biovolume, as well as of annual primary production (Ruggiu et al., 1998).
Lake Maggiore watershed (about 6,600 km2) is shared almost equally between Italy and Switzerland, so issues related to the lake water quality and its restoration and management have been afforded through cooperation between the two countries. In particular, lake monitoring has been performed monthly since the 1980s at the deepest point (Ghiffa station), within the limnological campaigns funded by the International Commission for the Protection of Italian-Swiss Waters (CIPAIS; www.cipais.org). The monitoring covered several physical, chemical, and biological variables, and these long-term data series allowed us to evaluate the lake trophic evolution i.e., oligotrophication, as well as its response to climate drivers (Tanentzap et al., 2020; Rogora et al., 2021). However, until recently, only discrete data, based on regular field campaigns, have been collected, except for meteo-hydrological data for which high-frequency monitoring stations have been established in the lake watershed (Ciampittiello et al., 2021).
As regards phytoplankton, integrated samples (0–20 m) have been regularly collected and analyzed by microscopy to identify taxa at the species level (Morabito et al., 2012) and assess biovolume of the main groups (Ruggiu et al., 1998). Chl-a concentration was also measured on integrated samples by spectrophotometric technique in the period 1984–2009 (APAT-IRSA-CNR, 2003), while from 2010 measurements were taken using a vertical profiling instrument (FluoroProbe, BBE Moldaenke) after a careful check of the comparability of the two methods.
2.2 LM1 buoy
The monitoring buoy LM1 was deployed in Lake Maggiore in the Pallanza basin (about 50 m from the shoreline, anchored at a depth of about 40 m; Figure 1). The buoy was developed in-house and conceived as a low-cost modular system (Tiberti et al., 2021). It is equipped with sensors for pH, conductivity, dissolved oxygen—surface (1.5 m depth) and deep (10 m depth)—and algal pigments (Chl-a, both at surface and deep, phycocyanin (PC) and phycoerythrin (PE) at surface), a thermistor chain, a weather station, and a live webcam (https://www.meteolivevco.it/boa-limnologica-sul-lago-maggiore/). Sensors used for pigment monitoring (Cyclops7, Turner Design) include two Chl-a sensors (surface and deep) which were deployed in 2020, and one PC and one PE sensors which were added in March 2021. Each sensor is wire connected to the electronic control unit, which has been specifically designed within the project for signal acquisition from the sensors, data storage, basic data elaboration, and wireless transfer. The open-source data management software istSOS (http://istsos.org/) was selected for managing and dispatching the observations from the monitoring sensors (Strigaro et al., 2022). Further details on the system hardware and software are provided by Tiberti et al. (2021).
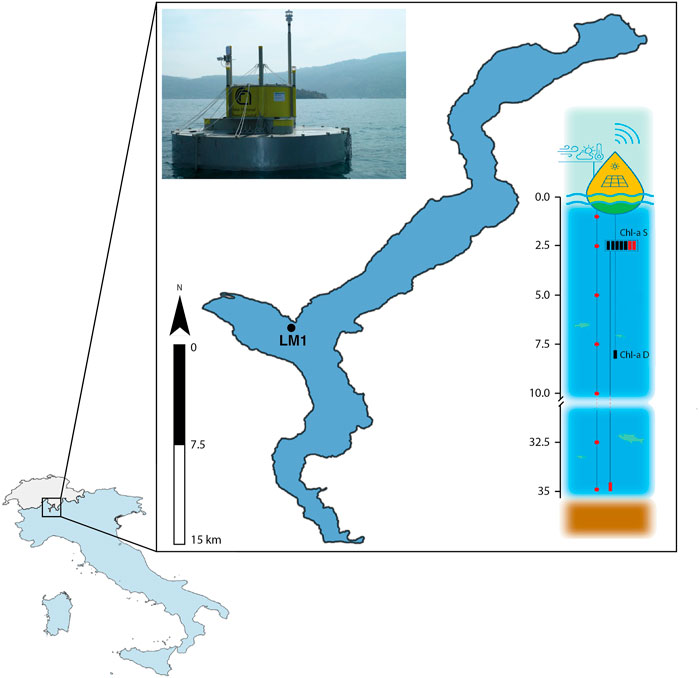
FIGURE 1. Location and main characteristics of the monitoring buoy LM1 in Lake Maggiore (see Tiberti et al., 2021 for the details). On the bottom-left map, Italy is coloured blue while Switzerland is grey.
For the present study, pigment raw data (in Volt) were stored in daily text files at 1 minute frequency. Successively, the data were processed by applying quality assurance/quality control (QA/QC) procedures and aggregated on an hourly basis. Sensor signal at the surface was clearly affected by quenching during daylight, as shown by the daily cycle in fluorescence data (Tiberti et al., 2021). As suggested by McBride and Rose (2018), night measurements should be considered the most indicative of Chl-a concentration. In particular, for the comparison with laboratory data, we selected fluorescence sensor data between 00:00 and 05:00 a.m. on the day of the sampling. The conversion from signal data (in Volt) to Chl-a concentrations (µg L−1) was done by applying a measured factor obtained from laboratory calibration (Tiberti et al., 2021).
2.3 Chl-a analysis
During the first 2 years of the HFM (2020-2021) we collected 54 water samples within 37 sampling dates, approximatively fortnightly, during the periods of high biological activity, and monthly in the other months. Samples were collected close to the buoy using a Ruttner bottle. Part of the samples were taken at 1 m depth (n = 37), and part at 10 m depth (n = 17), where the surface and deep Chl-a sensors are placed, respectively. Data covered the periods January-September 2020 and March-December 2021. Unfortunately, no data were available between October 2020 and February 2021 because a violent storm seriously damaged the buoy and the sensors on 2 October 2020 and the repairing operation took several months before the monitoring could be restarted.
All the samples were analyzed as follows:
- acetone extraction followed by UV-VIS spectrophotometrical reading (APAT IRSA 9020) to quantify Chl a, b, c, according to APAT—IRSA/CNR, 2003.
- fluorometric determination of total Chl-a and of the contribution by the main algal classes through the multi-wavelength probe FluoroProbe (bbe Moldaenke GmbH), used as a Workstation benchtop unit. The instrument can detect four phytoplankton classes: green algae, xanthophyll-containing brown algae (dinoflagellates, diatoms, chrysophyceae), blue-green algae (PC-rich cyanobacteria), and red algae (PE-rich cyanobacteria, cryptophyceae) (Callieri et al., 2021).
On a subset of the surface samples (n = 23), microscopy analysis of phytoplankton based on the inverted microscopy techniques (Utermöhl, 1931; Lund et al., 1958) and the CEN guidance (CEN, 2004) was performed. Taxa were classified at species or genus level by an inverted microscope (Zeiss) at intermediate (200x) and high (×400) magnification. Community composition was estimated as taxa relative abundance. The total biovolume and biovolume of each group were estimated from density data and original measurements of cell volume (Morabito et al., 2012).
To evaluate how well in-situ sensor data reflect the results of laboratory techniques, we used a series of linear regressions, with the average in-situ night values (from 00:00 and 05:00 a.m.) of Chl-a as the dependent variable and the laboratory measures of Chl-a (by UV-VIS and FP) as independent variables, both considering all samples together (n = 54) and surface and deep samples separately (n = 37 and n = 17, respectively). In addition, we described the linear relationship between the in-situ Chl-a measures and the estimated algal biovolume (available only for 23 surface samples) with a further regression analysis. All analyses were performed in the R environment for statistical computing (R Core Team, 2022).
3 Results
3.1 Evaluation of Chl-a estimation by in-situ sensors
Chl-a concentrations measured in Lake Maggiore during the study period through HFM ranged between 2 and 13 μg L−1 at the surface and 1 and 12 μg L−1 at the deep sample points, with average values of 4.7 and 3.3 μg L−1, respectively. These values are typical of mesotrophic lakes; however, they lie in the low range of Chl-a concentrations characterizing freshwater lakes worldwide (Filazzola et al., 2020). Some peaks (above 10 μg L−1) were detected in spring and summer, usually in correspondence with diatom blooms, such as in May 2021.
Altogether the results of the linear regression indicate a good fit between the values recorded by the buoy and both UV-VIS and FP data (Table 1; Figure 2). The slopes of the regression lines are frequently significantly lower than 1 (1 is not included in the 95% Confidence Intervals), which indicates that laboratory calibration, based on dilutions of algal cultures, may be inaccurate under natural conditions, i.e., natural algal communities. Because of this, discrete laboratory measures from discrete samples could be used to recalibrate the sensors.

TABLE 1. Results from linear regressions between chlorophyll-a concentrations measured by in-situ sensors (buoy) and by UV-VIS spectrophotometry or FluoroProbe (FP). The intercept (a), slope (b), coefficient of determination (R2), and p-values are reported; lower and upper 95% confidence intervals of a and b are reported in brackets.
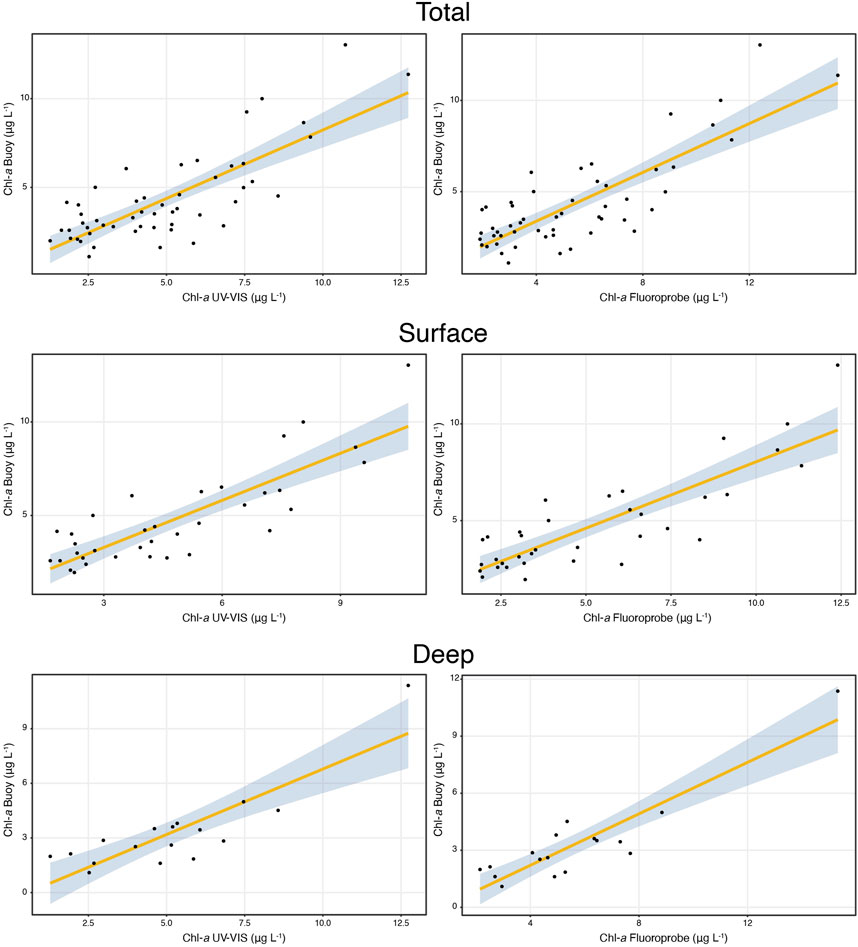
FIGURE 2. Comparison between Chl-a concentrations obtained from the in-situ sensors (buoy) and laboratory analysis UV-VIS (left panels) and FP (right panels). In the panels data are shown for all the samples, surface and deep samples. Statistics of the linear regressions are provided in Table 1.
The high frequency (HF) data collected in 2020 and 2021 (hourly average) allowed us to describe the seasonal pattern of Chl-a concentrations in great detail (Figure 3): sensor readings were able to describe the annual Chl-a dynamics, its main peaks (e.g., March, late April and June 2020 and May 2021) and a number of short-lived peaks of Chl-a (Figure 3) that would have passed unnoticed or poorly described by discrete data. Some discrepancy was observed between HF and laboratory data in late summer 2020, when the in-situ data slightly underestimated the Chl-a values.
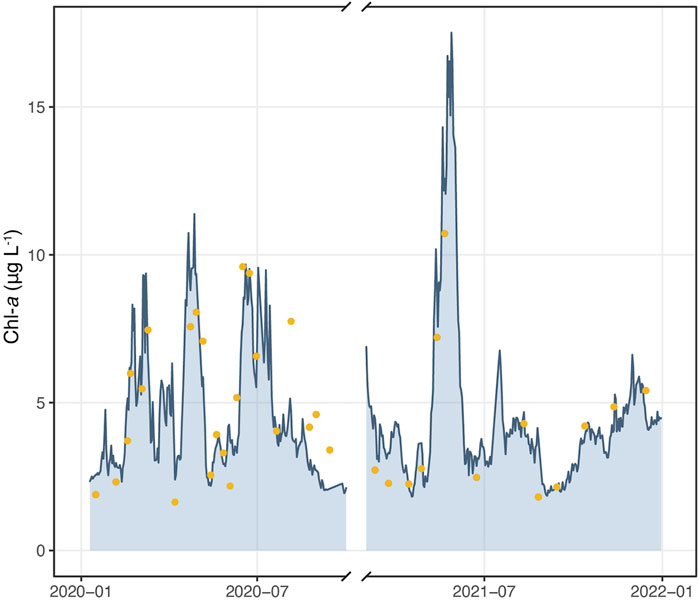
FIGURE 3. Hourly average data of Chl-a concentrations from in-situ sensors (bluish area) and Chl-a concentrations in the surface samples collected in 2020–21 and analysed by UV-VIS spectrophotometry (yellow dots).
3.2 Algal groups and their role in Chl-a detection
To get additional data on phytoplankton biovolume and composition, a subset of surface samples were counted by microscope (Figure 4). As regards the phytoplankton community composition, our results confirmed those obtained from the long-term monitoring of Lake Maggiore (Morabito et al., 2012; Rogora et al., 2021). We found a limited presence of cyanobacteria, which were present in small amounts mostly in late summer (e.g., 290 mm3 m−3 in August 2020). Bacillariophyceae were dominant in almost all the samples, representing between 50 and 80% of the total biovolume. The second most important group was that of cryptophyceae, which were dominant in a few spring samples (e.g., about 500 and 750 mm3 m−3 in March and May 2021).
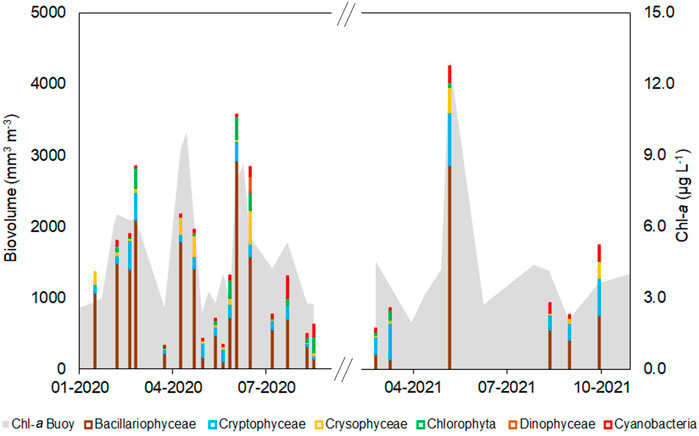
FIGURE 4. Biovolume of the main phytoplankton groups based on microscopy analysis of surface samples (n = 23) collected in 2020 and 2021. The grey area shows the pattern of HF Chl-a data from the in-situ sensor.
The Chl-a values, both from the buoy and UV-VIS analysis, closely followed the pattern of the Bacillariophyceae group (Figure 4). Diatoms are indeed the dominant taxon in Lake Maggiore, and their blooms in spring and summer are the main responsible for the Chl-a peaks observed in the lake, especially in recent years (Rogora et al., 2021).
When considering total biovolume, the highest values were measured in early March 2020 (2,800 mm3 m−3), June 2020 (2,500 and 2,800 mm3 m−3 respectively on the 16 and 30 of June), and May 2021 (4,200 mm3 m−3). Despite the limited number of samples available in 2021, the seasonal pattern in both years was similar to the long-term monitoring of the lake, with the highest biovolume measured in spring (Rogora et al., 2021). The pattern of total biovolume followed well that of Chl-a concentrations resulting from the HFM (Figure 4). The linear regression parameters (R2 = 0.72, p < 0.001; a = 1.82 (0.77–2.87 95% CI); b = 0.002 (0.001–0.003 95% CI)) indicate a good fit between in-situ Chl-a sensor and the biovolume estimates from the microscopic analysis, as shown in Figure 5.
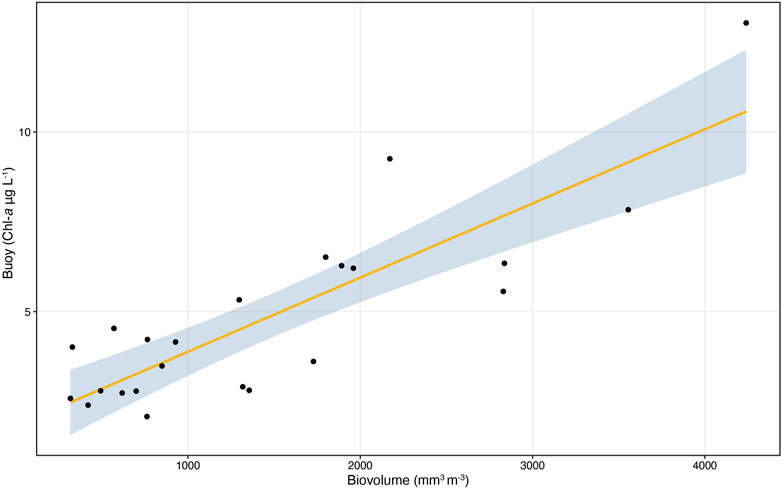
FIGURE 5. Linear regressions between Chl-a concentrations obtained from the in-situ sensors (buoy) and total phytoplankton biovolume by microscopy analysis (n = 23).
4 Discussion
The comparison between field and laboratory methods to estimate Chl-a concentrations in Lake Maggiore showed an overall good agreement. In particular, in-situ fluorimetric sensors provided a reliable estimate of Chl-a values and captured peaks occurring during algal blooms in spring and summer. Linear regressions were statistically significant between the data from the buoy and those from laboratory analyses. As expected, we found a higher correlation coefficient with the buoy sensor and FP data since both estimate Chl-a concentrations through a fluorimetric measure. A better fit with laboratory data emerged for the deep sensor, with respect to the surface one, possibly due to the more limited variability of deep Chl-a concentrations. A fair agreement was also found between the in-situ fluorometric data and the total phytoplankton biovolume estimated by cell counting, suggesting in-situ HF data as a tool to assess phytoplankton biomass variability in the short term. However, the phytoplankton community composition should be considered in this evaluation.
In this study, during most of the year, the phytoplankton community of Lake Maggiore was dominated by diatoms, and their biovolume variation during the 2-year period had a fair agreement with the in-situ HF Chl-a sensor trend. However, during the summer, cyanobacteria started to be an important part of the phytoplankton community. In particular, in late summer and early autumn small cell-sized (Chroococcales, cell sizes 2–4 µm) colonial cyanobacteria taxa such as Aphanoteche sp. and Aphanocapsa sp. reached considerable density values. During this period, Chl-a concentration by in-situ sensors recorded lower values with respect to laboratory methods and phytoplankton counting. A similar discrepancy was observed by other studies that highlighted how at low Chl-a concentrations (approx. below 10 μg L−1), the percentage contribution of picocyanobacteria to total phytoplankton biomass can be high (Voros et al., 1998). PE-rich picocyanobacteria are commonly found in oligotrophic waters where green and blue-green light is available, as in the case of Lake Maggiore (Callieri et al., 2021). Picocyanobacteria are characterized by a low Chl:biomass ratio with respect to other phytoplankton groups, so their presence could lead to an underestimate of total phytoplankton biomass based on in-situ Chl-a data.
Our results confirmed what was highlighted by other studies about the limitations of sensor use to estimate Chl-a variations, including interference from water turbidity and the different contribution by phytoplankton classes (Zamyadi et al., 2012; Kong et al., 2014; Kasinak et al., 2015). In fact, in-situ fluorescence estimation mainly depends on phytoplankton biomass, its community composition, and its physiological state (Richardson et al., 2010). However, other physical and chemical factors such as water temperature, water column stratification, underwater light, back light scattering, and light absorption by organic and inorganic particles can affect the final measure of chlorophyll and phycobiliprotein values (Proctor & Roesler 2010; Ostrowska 2012).
A limitation of our study consists in the narrow range of Chl-a values considered in the method comparison: Chl-a in Lake Maggiore is in the low range of concentrations for freshwater, with most of the data between 2 and 3 μg L−1 and only a few data above 10 μg L−1, during spring algal density increases. Moreover, the phytoplankton community was dominated by diatoms, which also contributed to the highest Chl-a values recorded, followed by green algae. PC-containing cyanobacteria were rarely detected in the lake, and this prevented us from assessing the use of phycocyanin in-situ sensors as early warning systems for potentially toxic cyanobacteria blooms.
A further limitation of our assessment lies in the uneven distribution of samples (more frequent sampling in 2020 and a higher number of surfaces with respect to deep samples) and in the lack of replicates, which prevented the evaluation of random errors. However, we tested the agreement between methods on a total of 54 samples covering the seasonal pattern of Chl-a and the results clearly demonstrate an overall agreement between in-situ sensor data and extracted Chl-a.
A further problem we encountered was the presence of gaps in the HF data due to technical issues (e.g., sensor damages, fouling, lack of data transmission), the most relevant being a 5-month interruption between 2020 and 2021 due to the damages caused to the buoy by a severe storm. However, these issues are not rare in HFM applications and should be considered when designing the monitoring system and evaluating the costs and benefits of the system itself (Seifert-Dähnn et al., 2021). Despite these drawbacks, the HFM systems in Lake Maggiore performed well in depicting the seasonal pattern of Chl-a concentrations, which in turn proved to be a good proxy of phytoplankton biovolume. We still need to test the performance of the PC and PE sensors deployed in 2021. However, we think these sensors will be a useful integration to the HFM of Lake Maggiore, for a more comprehensive evaluation of the phytoplankton succession. Overall, our results confirmed that in-situ sensors may be useful in measuring diagnostic pigments and estimating algae abundance in near real-time, especially when sensors for different pigments are combined (Pace et al., 2017; Bertone et al., 2018; Chegoonian et al., 2022). These data may be relevant in planning field campaigns for institutional monitoring purposes, e.g., targeting the sampling and analysis effort on bringing information on critical or representative periods in terms of algal blooms.
It is worth mentioning that in some periods of 2020 (March-May and November-December) the field campaigns for the monitoring of Lake Maggiore had to be delayed due to the lockdowns caused by the outbreak of the COVID-19 pandemic. In those situations, the HF data provided by the buoy were the only data available and, even though limited to a few variables, provided useful information on the lake dynamics. Some relative maxima in Chl-a concentrations recorded by the buoy (e.g., 7.8–8.6 μg L−1 on 16 and 23 of June 2020) were not detected by the discrete monitoring program based on monthly surveys (3.1 μg L−1 for June 2020; CIPAIS, 2022) confirming that monthly or even lower-frequent sampling is likely to miss the short-term variability of some lake processes. HF data may not only detect short-term events but also improve the estimate of key parameters for water quality assessment, such as Chl-a concentrations (Bresciani et al., 2011). Further, the HF Chl-a data may be employed in assessing the performance of the monitoring systems based on satellite data, also under development within the SIMILE project (Luciani et al., 2021; Bratic et al., 2022).
Long-term monitoring has proved to disproportionately contribute to a better understanding of ecosystems as well as to support management and restoration policy (Hughes et al., 2017). However, a multi-scale approach is required in ecological study, combining monitoring at different temporal and spatial scales (Sparrow et al., 2020). In-depth information on lake productivity and phytoplankton biomass, such as those provided by microscopy and laboratory analysis of discrete samples, cannot be replaced by sensor data. Nevertheless, HFM, even when limited to a few basic variables, may supplement time series of highly detailed data, contributing to a deeper knowledge of ecosystem dynamics, especially as regards short-term and highly variable processes (Carpenter et al., 2020).
5 Conclusion
Our study confirmed that in-situ sensors measuring in vivo fluorescence are an interesting approach for monitoring algal blooms in lakes and are an effective tool to detect and track bloom formation, complementing the more classical laboratory analysis. HFM is not limited to Chl-a, but also includes other algal pigments (i.e., PC and PE) that may improve the evaluation of lake productivity and phytoplankton succession: used in conjunction with discrete chemical and biological monitoring, it represents an important advance in the monitoring of freshwaters and a useful tool to detect ecological changes. However, regular check and validation of the sensor readings through laboratory analyses is needed to get reliable data.
Automated in-situ monitoring can warn water managers and local authorities when measures against blooms are required and data can be used for successive selection of sites to be sampled and analyzed in the laboratory. In summary, lake monitoring, programs based on discrete sample collection and analysis may be successfully integrated by HFM and other approaches, such as the use of satellite data and of observations provided by citizens: this can hold both for lakes subject to operational monitoring under the WFD, where early warning systems could be needed to assess critical issue such as algal blooms, but also for lakes under the surveillance monitoring, to supplement and validate long-term discrete data.
Data availability statement
The raw data supporting the conclusion of this article will be made available by the authors, without undue reservation.
Author contributions
MR conceptualised the study and prepared the original draft, with the support of AL and LK. AL, SM, RC, and LK performed the laboratory analyses. TC and DM were in charge for the buoy data management. TC and RT led the data analysis for the manuscript. All the authors contributed to writing, reviewing, and editing.
Funding
The development of the LM1 buoy and all the activities related to the evaluation of pigment data have been supported by SIMILE, an Interreg Italian-Swiss project funded by the European Regional Development Fund (ERDF) ID: 523544. Long-term limnological research on Lake Maggiore is partly funded by the International Commission for the Protection of Swiss-Italian Waters (CIPAIS).
Acknowledgments
Investigations on Lake Maggiore are also carried out in the framework of the LTER (Long Term Ecological Research) Italian network, site “IT-08 Southern Alpine lakes” (http://www.lteritalia.it/). We want to thank the other partners and collaborators of the SIMILE project: Lombardy Region, Ticino Canton, Fondazione Politecnico di Milano, IREA CNR, and the Regional Agency for Environmental Protection (ARPA) of the Lombardy Region, for the fruitful cooperation within the project. We also wish to thank the technicians, postdocs and students who have contributed to the fieldwork and laboratory analysis described in this study.
Conflict of interest
The authors declare that the research was conducted in the absence of any commercial or financial relationships that could be construed as a potential conflict of interest.
Publisher’s note
All claims expressed in this article are solely those of the authors and do not necessarily represent those of their affiliated organizations, or those of the publisher, the editors and the reviewers. Any product that may be evaluated in this article, or claim that may be made by its manufacturer, is not guaranteed or endorsed by the publisher.
References
Bertone, E., Burford, M. A., and Hamilton, D. P. (2018). Fluorescence probes for real-time remote cyanobacteria monitoring: A review of challenges and opportunities. Water Res. 141, 152–162. doi:10.1016/j.watres.2018.05.001
Boyer, J. N., Kelble, C. R., Ortner, P. B., and Rudnick, D. T. (2009). Phytoplankton bloom status: Chlorophyll a biomass as an indicator of water quality condition in the southern estuaries of Florida, USA. Ecol. Indic. 9, S56–S67. doi:10.1016/j.ecolind.2008.11.013
Bratic, G., Carrion, D., Cannata, M., Rogora, M., Strigaro, D., and Brovelli, M. A. (2022). lake water quality monitoring tools. Int. Arch. Photogramm. Remote Sens. Spat. Inf. Sci., 599–606. doi:10.5194/isprs-archives-XLIII-B4-2022-599-2022
Bresciani, M., Stroppiana, D., Odermatt, D., Morabito, G., and Giardino, C. (2011). Assessing remotely sensed chlorophyll-a for the implementation of the Water Framework Directive in European perialpine lakes. Sci. Total Environ. 409, 3083–3091. doi:10.1016/j.scitotenv.2011.05.001
Brovelli, M. A., Cannata, M., and Rogora, M. (2019). SIMILE, a geospatial enabler of the monitoring of sustainable development goal 6 (ensure availability and sustainability of water for all). Int. Arch. Photogramm. Int. Arch. Photogramm. Remote Sens. Spat. Inf. Sci.XLII-4/W20, 3–10. doi:10.5194/isprs-archives-xlii-4-w20-3-2019
Callieri, C., Hernández-Avilés, J. S., Eckert, E. M., Rogora, M., Tartari, G., Sforzi, T., et al. (2021). Transparent exopolymer particles (TEP), phytoplankton and picocyanobacteria along a littoral-to-pelagic depth-gradient in a large subalpine lake. J. Limnol 80 (3). doi:10.4081/jlimnol.2021.2021
Caroni, R., Van de Bund, W., Clarke, R. T., and Johnson, R. K. (2013). Combination of multiple biological quality elements into waterbody assessment of surface waters. Hydrobiologia 704 (1), 437–451. doi:10.1007/s10750-012-1274-y
Carpenter, S. R., Arani, B. M., Hanson, P. C., Scheffer, M., Stanley, E. H., and Van Nes, E. (2020). Stochastic dynamics of Cyanobacteria in long-term high-frequency observations of a eutrophic lake. Limnol. Oceanogr. Lett. 5 (5), 331–336. doi:10.1002/lol2.10152
CEN (2004). Water quality – guidance standard for the routine analysis of phytoplankton abundance and composition using inverted microscopy (Utermöhl technique) CEN TC 230/WG 2/TG 3/N83, May 2004.
Chegoonian, A. M., Zolfaghari, K., Leavitt, P. R., Baulch, H. M., and Duguay, C. R. (2022). Improvement of field fluorometry estimates of chlorophyll a concentration in a cyanobacteria-rich eutrophic lake. Limnol. Ocean. Methods 20 (4), 193–209. doi:10.1002/lom3.10480
Ciampittiello, M., Manca, D., Dresti, C., Grisoni, S., Lami, A., and Saidi, H. (2021). Meteo-hydrological sensors within the lake Maggiore catchment: System establishment, functioning and data validation. Sensors 21 (24), 8300. doi:10.3390/s21248300
European Commission. Brussels: European Commission.Directive 2000/60/EC of the European parliament and of the council of 23 october 2000 establishing a framework for community action in the field of water policyOff. J. L327/1
Fenocchi, A., Rogora, M., Sibilla, S., Ciampittiello, M., and Dresti, C. (2018). Forecasting the evolution in the mixing regime of a deep subalpine lake under climate change scenarios through numerical modelling (Lake Maggiore, Northern Italy/Southern Switzerland). Clim. Dyn. 51 (9), 3521–3536. doi:10.1007/s00382-018-4094-6
Filazzola, A., Mahdiyan, O., Shuvo, A., Ewins, C., Moslenko, L., Sadid, T., et al. (2020). A database of chlorophyll and water chemistry in freshwater lakes. Sci. Data 7 (1), 310–10. doi:10.1038/s41597-020-00648-2
Hamilton, D. P., Carey, C. C., Arvola, L., Arzberger, P., Brewer, C., Cole, J. J., et al. (2014). A global lake ecological observatory network (GLEON) for synthesising high-frequency sensor data for validation of deterministic ecological models. Inland Waters 5, 49–56. doi:10.5268/iw-5.1.566
Harke, M. J., Steffen, M. M., Gobler, C. J., Otten, T. G., Wilhelm, S. W., Wood, S. A., et al. (2016). A review of the global ecology, genomics, and biogeography of the toxic cyanobacterium, Microcystis spp. Harmful algae. 54, 4–20. doi:10.1016/j.hal.2015.12.007
Hodges, C. M., Wood, S. A., Puddick, J., McBride, C. G., and Hamilton, D. P. (2018). Sensor manufacturer, temperature, and cyanobacteria morphology affect phycocyanin fluorescence measurements. Environ. Sci. Pollut. Res. 25 (2), 1079–1088. doi:10.1007/s11356-017-0473-5
Hughes, B. B., Beas-Luna, R., Barner, A. K., Brewitt, K., Brumbaugh, D. R., Cerny-Chipman, E. B., et al. (2017). Long-term studies contribute disproportionately to ecology and policy. BioScience 67 (3), 271–281. doi:10.1093/biosci/biw185
Hunter, P. D., Tyler, A. N., Gilvear, D. J., and Willby, N. J. (2009). Using remote sensing to aid the assessment of human health risks from blooms of potentially toxic cyanobacteria. Environ. Sci. Technol. 43 (7), 2627–2633. doi:10.1021/es802977u
ISO 10260, 1992. Water quality — measurement of biochemical parameters — spectrometric determination of the chlorophyll-a concentration
Jennings, E., Jones, S., Arvola, L., Staehr, P. A., Gaiser, E., Jones, I. D., et al. (2012). Effects of weather-related episodic events in lakes: An analysis based on high-frequency data. Freshw. Biol. 57 (3), 589–601. doi:10.1111/j.1365-2427.2011.02729.x
Kasinak, J. E., Holt, B. M., Chislock, M. F., and Wilson, A. E. (2015). Benchtop fluorometry of phycocyanin as a rapid approach for estimating cyanobacterial biovolume. J. Plankton. Res. 37, 248–257. doi:10.1093/plankt/fbu096
Kasprzak, P., Padisák, J., Koschel, R., Krienitz, L., and Gervais, F. (2008). Chlorophyll a concentration across a trophic gradient of lakes: An estimator of phytoplankton biomass? Limnologica 38, 327–338. doi:10.1016/j.limno.2008.07.002
Klug, J. L., Richardson, D. C., Ewing, H. A., Hargreaves, B. R., Samal, N. R., Vachon, D., et al. (2012). Ecosystem effects of a tropical cyclone on a network of lakes in northeastern North America. Environ. Sci. Technol. 46 (21), 11693–11701. doi:10.1021/es302063v
Kong, Y., Lou, I., Zhang, Y., Lou, C. U., and Mok, K. M. (2014). Using an online phycocyanin fluorescence probe for rapid monitoring of cyanobacteria in Macau freshwater reservoir. Hydrobiologia 741, 33–49. doi:10.1007/s10750-013-1759-3
Lorenzen, C. J. (1967). Determination of chlorophyll and pheo-pigments: Spectrophotometric equations. Limnol. Oceanogr. 12, 343–346. doi:10.4319/lo.1967.12.2.0343
Luciani, G., Bresciani, M., Biraghi, C. A., Ghirardi, N., Carrion, D., Rogora, M., et al. (2021). Satellite Monitoring system of Subalpine lakes with open source software: The case of SIMILE Project. Baltic J. Mod. Comput. 9, 135–144. doi:10.22364/bjmc.2021.9.1.08
Lund, J. W. G., Kipling, C., and Le Cren, E. D. (1958). The inverted microscope method of estimating algal numbers and the statistical basis of estimations by counting. Hydrobiologia 11, 143–170. doi:10.1007/bf00007865
Marcé, R., George, G., Buscarinu, P., Deidda, M., Dunalska, J., de Eyto, E., et al. (2016). Automatic high frequency monitoring for improved lake and reservoir management. Environ. Sci. Technol. 50 (20), 10780–10794. doi:10.1021/acs.est.6b01604
McBride, C. G., and Rose, K. C. (2018). “Automated high-frequency monitoring and research”, K. Collier, J. Quinn, and C. Howard-Williams (Hamilton: Springer), 419–461. doi:10.1007/978-3-319-93043-5Lake restoration handbook
McQuaid, N., Zamyadi, A., Prévost, M., Bird, D. F., and Dorner, S. (2011). Use of in vivo phycocyanin fluorescence to monitor potential microcystin-producing cyanobacterial biovolume in a drinking water source. J. Environ. Monit. 13 (2), 455–463. doi:10.1039/c0em00163e
Meinson, P., Idrizaj, A., Nõges, P., Nõges, T., and Laas, A. (2016). Continuous and high-frequency measurements in limnology: History, applications, and future challenges. Environ. Rev. 24 (1), 52–62. doi:10.1139/er-2015-0030
Morabito, G., Mazzocchi, M. G., Salmaso, N., Zingone, A., Bergami, C., Flaim, G., et al. (2018a). Plankton dynamics across the freshwater, transitional and marine research sites of the LTER-Italy Network. Patterns, fluctuations, drivers. Sci. Total Environ. 627, 373–387. doi:10.1016/j.scitotenv.2018.01.153
Morabito, G., Oggioni, A., and Austoni, M. (2012). Resource ratio and human impact: How diatom assemblages in Lake Maggiore responded to oligotrophication and climatic variability. Hydrobiologia 698, 47–60. doi:10.1007/s10750-012-1094-0
Morabito, G., Rogora, M., Austoni, M., and Ciampittiello, M. (2018b). Could the extreme meteorological events in Lake Maggiore watershed determine a climate-driven eutrophication process? Hydrobiologia 824 (1), 163–175. doi:10.1007/s10750-018-3549-4
Ostrowska, M. (2012). Model of the dependence of the sun-induced chlorophyll a fluorescence quantum yield on the environmental factors in the sea. Opt. Express 20 (21), 23300–23317. doi:10.1364/oe.20.023300
Pace, M. L., Batt, R. D., Buelo, C. D., Carpenter, S. R., Cole, J. J., Kurtzweil, J. T., et al. (2017). Reversal of a cyanobacterial bloom in response to early warnings. Proc. Natl. Acad. Sci. U. S. A. 114 (2), 352–357. doi:10.1073/pnas.1612424114
Proctor, C. W., and Roesler, C. S. (2010). New insights on obtaining phytoplankton concentration and composition from in situ multispectral Chlorophyll fluorescence. Limnol. Oceanogr. Methods 8, 695–708. doi:10.4319/lom.2010.8.0695
R Core Team (2022). R: A language and environment for statistical computing, Found. Stat. Comput. Vienna: Austria. https://www.R-project.org/.
Randolph, K., Wilson, J., Tedesco, L., Li, L., Pascual, D. L., and Soyeux, E. (2008). Hyperspectral remote sensing of cyanobacteria in turbid productive water using optically active pigments, chlorophyll a and phycocyanin. Remote Sens. Environ. 112 (11), 4009–4019. doi:10.1016/j.rse.2008.06.002
Richardson, T. L., Lawrenz, E., Pinckney, J. L., Guajardo, R. C., Walker, E. A., Paerl, H. W., et al. (2010). Spectral fluorometric characterization of phytoplankton community composition using the Algae Online Analyser®. Water Res. 44, 2461–2472. doi:10.1016/j.watres.2010.01.012
Rogora, M., Austoni, M., Caroni, R., Giacomotti, P., Kamburska, L., Marchetto, A., et al. (2021). Temporal changes in nutrients in a deep oligomictic lake: The role of external loads versus climate change. J. Limnol., 80(3). doi:10.4081/jlimnol.2021.2051
Rogora, M., Buzzi, F., Dresti, C., Leoni, B., Lepori, F., Mosello, R., et al. (2018). Climatic effects on vertical mixing and deep-water oxygen content in the subalpine lakes in Italy. Hydrobiologia 824 (1), 33–50. doi:10.1007/s10750-018-3623-y
Ruggiu, D., Morabito, G., Panzani, P., and Pugnetti, A. (1998). Trends and relations among basic phytoplankton characteristics in the course of the long-term oligotrophication of Lake Maggiore (Italy). Hydrobiologia 369, 243–257. doi:10.1023/a:1017058112298
Salmaso, N., Buzzi, F., Capelli, C., Cerasino, L., Leoni, B., Lepori, F., et al. (2020). Responses to local and global stressors in the large southern perialpine lakes: Present status and challenges for research and management. J. Gt. Lakes. Res. 46, 752–766. doi:10.1016/j.jglr.2020.01.017
Salmaso, N., Morabito, G., Buzzi, F., Garibaldi, L., Simona, M., and Mosello, R. (2006). Phytoplankton as an indicator of the water quality of the deep lakes south of the alps. Hydrobiologia 563, 167–187. doi:10.1007/s10750-005-0003-1
Salmaso, N., Morabito, G., Mosello, R., Garibaldi, L., Simona, M., Buzzi, F., et al. (2003). A synoptic study of phytoplankton in the deep lakes south of the Alps (lakes Garda, Iseo, Como, Lugano and Maggiore). J. Limnol. 62 (2), 207–227. doi:10.4081/jlimnol.2003.207
Sarada, R. M. G. P., Pillai, M. G., and Ravishankar, G. A. (1999). Phycocyanin from spirulina sp: Influence of processing of biomass on phycocyanin yield, analysis of efficacy of extraction methods and stability studies on phycocyanin. Process Biochem. 34 (8), 795–801. doi:10.1016/s0032-9592(98)00153-8
Seifert-Dähnn, I., Furuseth, I. S., Vondolia, G. K., Gal, G., De Eyto, E., Jennings, E., et al. (2021). Costs and benefits of automated high-frequency environmental monitoring–The case of lake water management. J. Environ. Manage. 285, 112108. doi:10.1016/j.jenvman.2021.112108
Skeffington, R. A., Halliday, S. J., Wade, A. J., Bowes, M. J., and Loewenthal, M. (2015). Using high-frequency water quality data to assess sampling strategies for the EU Water Framework Directive. Hydrol. Earth Syst. Sci. 19 (5), 2491–2504. doi:10.5194/hess-19-2491-2015
Sparrow, B. D., Edwards, W., Munroe, S. E., Wardle, G. M., Guerin, G. R., Bastin, J. F., et al. (2020). Effective ecosystem monitoring requires a multi-scaled approach. Biol. Rev. 95 (6), 1706–1719. doi:10.1111/brv.12636
Stockwell, J. D., Doubek, J. P., Adrian, R., Anneville, O., Carey, C. C., Carvalho, L., et al. (2020). Storm impacts on phytoplankton community dynamics in lakes. Glob. Change Biol. 26 (5), 2756–2784. doi:10.1111/gcb.15033
Strigaro, D., Cannata, M., Lepori, F., Capelli, C., Lami, A., Manca, D., et al. (2022). Open and cost-effective digital ecosystem for lake water quality monitoring. Sensors 2, 6684. doi:10.3390/s22176684
Stumpf, R. P., Wynne, T. T., Baker, D. B., and Fahnenstiel, G. L. (2012). Interannual variability of cyanobacterial blooms in Lake Erie. PLoS ONE 7 (8), e42444. doi:10.1371/journal.pone.0042444
Tanentzap, A. J., Morabito, G., Volta, P., Rogora, M., Yan, N. D., and Manca, M. (2020). Climate warming restructures an aquatic food web over 28 years. Glob. Change Biol. 26 (12), 6852–6866. doi:10.1111/gcb.15347
Taranu, Z. E., Gregory-Eaves, I., Leavitt, P. R., Bunting, L., Buchaca, T., Catalan, J., et al. (2015). Acceleration of cyanobacterial dominance in north temperate-subarctic lakes during the Anthropocene. Ecol. Lett. 18 (4), 375–384. doi:10.1111/ele.12420
Tiberti, R., Caroni, R., Cannata, M., Lami, A., Manca, D., Strigaro, D., et al. (2021). Automated high frequency monitoring of Lake Maggiore through in situ sensors: System design, field test and data quality control. J. Limnol. 80 (2). doi:10.4081/jlimnol.2021.2011
Utermöhl, von H. (1931). Neue Wege in der quantitativen Erfassung des Planktons. (Mit besondere Beriicksichtigung des Ultraplanktons). SIL Proc. 1922-2010 5, 567–596. doi:10.1080/03680770.1931.11898492
Vörös, L., Callieri, C., Balogh, K. V., and Bertoni, R. (1998). Freshwater picocyanobacteria along a trophic gradient and light quality range. Hydrobiologia 369, 117–125. doi:10.1023/a:1017026700003
Vörös, L., and Padisák, J. (1991). Phytoplankton biomass and chlorophyll-a in some shallow lakes in central Europe. Hydrobiologia 215, 111–119. doi:10.1007/bf00014715
Keywords: chlorophyll, fluorimetry, high frequency monitoring, LTER, phytoplankton
Citation: Rogora M, Cancellario T, Caroni R, Kamburska L, Manca D, Musazzi S, Tiberti R and Lami A (2023) High-frequency monitoring through in-situ fluorometric sensors: A supporting tool to long-term ecological research on lakes. Front. Environ. Sci. 10:1058515. doi: 10.3389/fenvs.2022.1058515
Received: 30 September 2022; Accepted: 01 December 2022;
Published: 04 January 2023.
Edited by:
Robert Ptacnik, Wasser Cluster Lunz, AustriaReviewed by:
Arda Özen, Cankiri Karatekin University, TurkeyJoaquim I. Goes, Columbia University, United States
Copyright © 2023 Rogora, Cancellario, Caroni, Kamburska, Manca, Musazzi, Tiberti and Lami. This is an open-access article distributed under the terms of the Creative Commons Attribution License (CC BY). The use, distribution or reproduction in other forums is permitted, provided the original author(s) and the copyright owner(s) are credited and that the original publication in this journal is cited, in accordance with accepted academic practice. No use, distribution or reproduction is permitted which does not comply with these terms.
*Correspondence: Michela Rogora, bWljaGVsYS5yb2dvcmFAY25yLml0