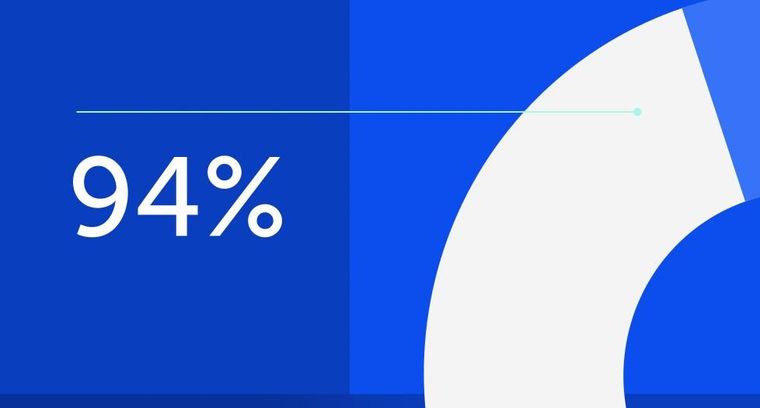
94% of researchers rate our articles as excellent or good
Learn more about the work of our research integrity team to safeguard the quality of each article we publish.
Find out more
ORIGINAL RESEARCH article
Front. Environ. Sci., 05 January 2023
Sec. Drylands
Volume 10 - 2022 | https://doi.org/10.3389/fenvs.2022.1053797
This article is part of the Research TopicBiodiversity Protection and Pollution Control on the Mongolian PlateauView all 7 articles
A clear understanding of the spatiotemporal evolution and driving factors of ecosystem service value (ESV) and the landscape ecological risk index (ERI) can effectively link human well-being and ecosystem security, which is essential for sustainable ecosystem management. Based on multitemporal land use data (1990, 2000, 2010, and 2018), the spatiotemporal evolution of ESV and the ERI in the Ulan Buh Desert was evaluated. The driving forces of ESV and the ERI were quantitatively evaluated by the Geodetector model. The results show that 1) from 1990 to 2018, total ESV in the Ulan Buh Desert increased from 7.00×108 yuan to 11.09×108 yuan. Low-ESV areas accounted for approximately 72.28% of the study area. High-ESV and moderate-high-ESV areas were mainly distributed along the Yellow River and the northeastern region. 2) During the study period, the ecological risk of the Ulan Buh Desert generally decreased; only the ecological risk of the northeastern region improved significantly, and high-risk areas were mainly distributed across sand dunes located in the middle of the desert. 3) There was a negative correlation between ESV and the ERI in the study area, and the main relationship was low value-high risk. 4) Driving force analysis results show that natural and human impact factors jointly affected the spatiotemporal differentiation of ESV and the ERI in the Ulan Buh Desert. Among the influencing factors, the interaction between the distance to a highway and annual precipitation had the strongest impact. The implementation of relevant policies in the study area should be guided by ESV and the ERI, and the protection and restoration of various ecosystems in the study area must be strengthened.
Ecosystem services represent the benefits that human populations derive from ecosystems (Costanza et al., 1997; Daily, 1997; Millennium Ecosystem Assessment, 2005). While providing services, ecosystems also face various ecological risks themselves (Xie et al., 2015; Cao et al., 2019). Ecological risk refers to the likelihood of adverse effects of disturbances on ecosystems (Mann et al., 2021). However, with the development of the social economy, landscape patterns and ecological processes have changed profoundly, which has impacted ecosystem services and ecological risks to different degrees, seriously threatening human well-being (Li et al., 2016). Ecosystem service value (ESV) and the ecological risk index (ERI) quantify the capacity of an ecosystem to provide services and the extent to which a region is exposed to ecological risks, respectively. Therefore, comprehensive consideration of ESV and the ERI provides a basis for the prevention of regional ecological risk and the improvement of ecosystem functions and plays an important role in the construction of regional ecological security patterns (Zhu and Chen., 2022).
In recent years, there has been increasing interest in introducing ESV and the ERI into environmental policies and governance (Gong et al., 2021). Scholars have conducted a series of scientific studies on the quantitative assessment, spatiotemporal evolution and influencing factors of ESV and ecological risk. At present, research on ESV has mainly concentrated on river basins (Woldeyohannes et al., 2020; Pan et al., 2021), cities (Wang et al., 2019; Hou et al., 2020; Rahman and Szabo, 2021), natural reserves (Sannigrahi et al., 2019; Yan et al., 2022) and so on. The main research methods used include the equivalent factor method (Xie et al., 2003; Jia et al., 2021; Liu et al., 2021) and material conversion method (Goldstein et al., 2012; Fadaei et al., 2020; Wang J et al., 2020). The obtained results contribute to the construction of regional ecological civilizations. Studies on landscape ecological risk have mostly focused on cities (Wang D et al., 2021; Al-Hameedi et al., 2022), river basins (Tian et al., 2019; Zhang et al., 2021), mining areas (Wu et al., 2021; Xu et al., 2021) and so on. The spatiotemporal distribution of regional ecological risk is explored by constructing a relative ecological risk assessment model (Bartolo et al., 2012; Heenkenda and Bartolo, 2016) to provide a basis for landscape ecological risk management. However, the abovementioned studies fail to consider the link between ESV and ecological risk. Most studies on ESV consider the benefits brought only by ecosystems while neglecting the potential risks faced by ecosystems under the disturbances of human activities. In contrast, special assessments of ecological risk have considered only the loss degree of an ecosystem when it is disturbed and have neglected the benefits ecosystems provide for human beings. At the same time, there is currently a lack of comprehensive consideration of ESV and the ERI together (Jr et al., 2009; Forbes and Calow, 2013; Xu et al., 2016; Wang, 2021; Karimian et al., 2022), especially for desert ecosystems. Due to the natural conditions of drought and a lack of rain, desert ecosystems are extremely fragile and face ecological risks such as sandstorms and landslides. The comprehensive evaluation of the ESV and ERI of desert ecosystems and the exploration of their driving forces can provide a theoretical basis for the protection and restoration of desert ecosystems.
The Ulan Buh Desert is among the eight largest deserts in China. It is located in the core area of the northern wind-sand belt of the national ecological restoration security pattern (Jin, 2022). The ecological security of this desert is related to people’s well-being, to the socioeconomic development of the Yellow River Basin and even to that of the Beijing-Tianjin region. This paper aims to solve the following scientific problems through analysis and research: 1) to evaluate the spatial and temporal evolution of ESV and ERI values in the Ulan Buh Desert and 2) analyze the driving factors affecting the spatiotemporal distribution of ESV and ERI values. This study provides a scientific basis for formulating scientific risk prevention and ecological remediation countermeasures for the Ulan Buh Desert.
The Ulan Buh Desert (39° 17′-40° 46′N, 105° 24′-107° 01′E) straddles the Alxa League and the Bayan Nur of Inner Mongolia, covering a total area of 1.3×108 hm2. It is bounded by Langshan Mountain in the northwest, the Hetao Plain in the northeast, the Yellow River in the east and Helan Mountain in the south (Wang X et al., 2020). The region has a temperate arid climate with an annual precipitation level of 151 mm, an annual average temperature of 7.8°C and a large difference in temperature between the daytime and nighttime (Cao et al., 2022). The plant community is dominated by xerophytes. The topography of the study area is low in the middle and high in the surrounding regions. Soil types mainly include gray desert soil, cumulated irrigated soil, aeolian sand soil, solonchak and light brown calcic soil (Ji and Guo., 1995). The main land use types are sand dunes (Figure 1).
Land use data collected from July to September in 1990, 2000, 2010 and 2018 were taken from Landsat five Thermal Mapper (TM) and Landsat eight Operational Land Imager (OLI) images of the United States Geological Survey (USGS; https://www.usgs.gov/). With the support of ENVI 5.3, radiometric calibration, atmospheric correction and mosaic processing were carried out successively. Land use maps of the study area from 1990 to 2018 were obtained using the object-oriented classification method and visual interpretation. The overall classification accuracy level was 85.13% (Song et al., 2021). According to the interpretation results, land use types were divided into grasslands, cultivated land, water bodies, building land, forests, mobile dunes, fixed dunes, semifixed dunes, saline-alkali land and other land. Mobile, fixed and semifixed dunes were merged into dunes during the ESV calculation process. Socioeconomic data were selected from the 1990–2018 statistical yearbooks of Inner Mongolia and the China grain yearbook (Inner Mongolia Bureau of Statistics, 2022).
Annual precipitation, digital elevation model (DEM), soil type and distance to highway data were provided by the Data Center for Resources and Environmental Sciences, Chinese Academy of Sciences (https://www.resdc.cn/) with spatial resolutions of 1 km, 30 m, 1 km and 1 km, respectively. The median normalized difference vegetation index (NDVI) with masked clouds and cloud shadows based on the red and near infrared bands of the surface reflectance collections for Landsat five and 8 (USGS Landsat Surface Reflectance Tier 1) for July to September in 1990, 2000, 2010 and 2018 were derived from the Google Earth Engine (GEE; https://code.earthengine.google.com/), a cloud-based computing platform. Slope and aspect data were calculated from DEM data with a spatial resolution of 30 m.
The study area was divided into 1716 3-km × 3-km square grids with the support of ArcMap 10.2. First, ESV and the ERI were calculated based on the grid units and assigned to the central points of each grid cell. Then, ordinary kriging interpolation was performed based on the central points to obtain the spatial distributions of ESV and the ERI in the study area.
Based on the value equivalent factor method proposed by Xie (Xie et al., 2015) and the actual willingness to pay of Inner Mongolia, the equivalent factor of the ESV of the Ulan Buh Desert was found to be 253.31 yuan·hm−2 (Song et al., 2021) after adjusting the coefficient and removing the effect of inflation. The ESV per unit area of the Ulan Buh Desert is shown in Supplementary Table S1.
Based on the geometric interval classification method of ArcGIS, the ecosystem value of the study area was divided into five grades: low (0, .0026], moderate-low (.0026, .0055], moderate (.0055, .0085], moderate-high (.0085, .0180] and high (.0180, ∞) (× 108 yuan).
Based on the research of Zeng (Zeng and Liu., 1999), the ERI model was constructed by using the landscape disturbance index and landscape fragility index:
where
Based on the geometric interval classification method of ArcGIS and the actual conditions of the Ulan Buh Desert, the ERI values of the study area were divided into five grades: low (0, .0650), moderate-low (.0650, .0710), moderate (.0710, .0760), moderate-high (.0760, .0840) and high (.0840, ∞).
In this paper, a bivariate local autocorrelation model is used to reflect the degree of correlation between local ESV and the ERI by using Moran’s I index. The formula for calculating Moran’s I index is as follows (Qiao et al., 2020; Jin et al., 2021; Li C et al., 2022):
where
Geodetector is a set of statistical methods that test the spatial heterogeneity of variables and reveal the driving force behind variations (Wang and Xu., 2017).
The factor detector uses the q value to express the explanation degree of an independent variable to the spatial heterogeneity of the dependent variable. The formula is as follows:
where h is the number of layers of variables x or y,
The interaction detector is applied to analyze interactions between the independent variables X1 and X2. The detection is performed as follows: first, the q values of independent variables X1 and X2 are calculated; then, the interaction between X1 and X2 is calculated as q (X1∩X2), and q (X1), q (X2) and q (X1∩X2) are compared to analyze their interactive behaviors (Table 2).
In this study, human influence factors, such as the distance to a highway, and natural factors, such as the NDVI, precipitation, slope, aspect and soil type, are selected as independent variables, while ESV and the ERI are selected as dependent variables. According to the data distribution characteristics, precipitation, slope, aspect and soil type data were classified into 6, 6, 6 and 7 categories, respectively, using the natural breaks method, and the NDVI and distance to a highway data were divided into 6 and 9 categories, respectively, using the geometric interval classification method. Based on the 3 km × 3 km grid, the variables were extracted to represent the center of each grid cell and were input into the Geodetector for the driving force analyses of ESV and the ERI.
From 1990 to 2018, dunes were the main land use type in the Ulan Buh Desert, accounting for more than 70% of the area (Figure 2). The area of mobile dunes decreased significantly over this period, while the areas of fixed dunes, semifixed dunes and cultivated land increased gradually during the study period. The shifting areas of mobile dunes to semifixed dunes were 11.70×104 hm2, 13.99×104 hm2 and 19.63 ×104 hm2 from 1990–2000, 2000–2010 and 2010–2018, respectively. The shifting areas of grasslands to cultivated land were 1.57×104 hm2 and .63×104 hm2 from 1990–2000 and 2000–2010, respectively. The change rates of building land, water bodies and forest areas were small during the study period. In general, the main form of land use type transfer in the study area was flow between dune types.
The total ESV in the Ulan Buh Desert showed a fluctuating and increasing trend from 7.00 × 108 yuan in 1990 to 6.17 × 108 yuan in 2000 and then continued to increase to 11.09 × 108 yuan by 2018, exhibiting a change rate of 58.41%, indicating that the ESV of the study area improved from 1990–2018 (Table 3). According to the results obtained for the four periods, the ESVs provided by different land use types could be ranked as follows: dunes > water bodies > cultivated land > other land > grasslands > forests > saline-alkali land > building land. Among them, the ESV of dunes first decreased and then increased with a change rate of -2.88% before further decreasing with a change rate of -4.46% between 2010 and 2018, while the values of water bodies and grassland first decreased and then increased. The change rate of water bodies was 142.73% from 1990 to 2018. In addition, the ESVs of cultivated land, forests and building land increased gradually.
In terms of its spatial distribution, the ESV of the Ulan Buh Desert was high in the northeast and low in the southwest (Figure 3). The study area mainly consisted of moderate-low-value areas and low-value areas. Portions of the moderate-high and high-value areas were intensively distributed in clusters in the northeastern part of the study area, while the other portions were located along the Yellow River in the eastern region. The area of moderate-high and high-value areas first decreased and then increased from 1990–2018. The areas of cultivated land and water bodies were relatively large, so the ESVs they provided were high. Low-value and moderate-low-value areas were mainly distributed in dune regions in the middle of the study area. The moderate-value area was distributed in the shape of a circle around the moderate-high-value area, and this area decreased gradually, potentially because the transfer of grasslands to cultivated land led to a continuous change from the moderate-value area to the moderate-high-value area.
In general, the ERI of the study area was low in the northeast and high in the southwest (Figure 4). The study area was dominated by a moderate-high-risk area, which was mainly distributed in the dune area. However, the area of moderate-high risk decreased from 99.83 × 104 hm2 to 90.88 × 104 hm2 from 1990 to 2018. The ecological risk status of the northeast plain region gradually improved, and moderate-risk and moderate-high-risk areas gradually changed to low-risk and moderate-low-risk areas.
Based on the bivariate local spatial autocorrelation method of GeoDa software, the correlation between the ESV and ERI of the Ulan Buh Desert was analyzed. The results show that the Moran’s I index values for 1990–2018 ranged from -.103, -.209, and -.217 to -.136, indicating that there was a certain negative correlation between ESV and the ERI in the Ulan Buh Desert. As shown in Figure 5, the areas where ESV and the ERI were significantly correlated were mainly distributed in other land areas in the western Ulan Buh Desert and along the northeastern border with the Hetao Plain. The areas were divided into five patterns with regard to correlation behavior: high value-high risk, low value-low risk, low value-high risk, high value-low risk and non-significant correlation (Figure 5).
Low value-high risk areas refer to areas with low ESVs per unit area but high ERIs; these areas were mainly distributed in the town of Jilantai and the eastern area of the town of Aolunbulage and accounted for 19.64%, 20.22%, 19.99%, and 19.35% of the overall area in 1990, 2000, 2010 and 2018, respectively. High value-low risk areas refer to areas with high ESVs per unit area but low ERIs; these regions were mainly distributed in the northeastern part of the study area. Their areal proportion showed a fluctuating growth trend, increasing from 8.10% in 1990 to 9.97% in 2018. High value-high risk areas refer to areas with high ESVs and ERIs. These regions were distributed along the Yellow River in the eastern part of the study area, accounting for approximately .52% of the study area in 1990. There was no clustered distribution of high value-high risk areas from 2000–2018. Low value-low risk areas refer to areas with low ESVs and ERIs. These regions were scattered in the northeastern part of the study area but concentrated in the building land area. The non-significant correlation area accounted for 64.39–67.54% of the total study area and was dominated by various types of sand dunes that were continuously distributed over a large area.
A factor detector can detect the explanatory degree of different driving factors to dependent variables, and the higher the q value is, the greater the explanatory degree is. Figures 6A, B show the contributions of different factors to the spatial differentiation of ESV and the ERI. In terms of the q mean, the explanatory power of each ESV driver could be ranked in descending order as follows: the NDVI (.233) > distance to a highway (.200) > soil type (.197) > precipitation (.082) > slope (.014) > aspect (.005). The NDVI, distance to a highway and soil type were the main driving factors of ESV spatial differentiation, while precipitation, slope and aspect were secondary factors. The explanatory powers of the ERI drivers, in descending order, were as follows: the NDVI (.347) > distance to a highway (.292) > soil type (.283) > precipitation (.075). The NDVI, distance to a highway and soil type were the main driving factors of the spatial distribution of ERI, while precipitation, slope and aspect were secondary factors. On the whole, the influence of natural factors on the spatial differentiation of ESV and the ERI was greater than that of human impact factors.
FIGURE 6. Factor detection of the spatial differentiation of ESV (A) and ERI (B). Note: DTH, distance to highway; AP, annual precipitation; ST, soil type.
The interaction results of various ESV-influencing factors show that the interaction between any two factors was stronger than that of any single factor, indicating that ESV in the study area was affected by the interactions among multiple factors (Figure 7A). The interaction between the distance to a highway and precipitation was the strongest (41.6%). The interactions between the distance to a highway and other factors were significantly stronger than the interactions among other factors, further proving the important effect of the distance to a highway on the spatial differentiation of ESV. This result also shows that natural and human impact factors affected spatial patterns of ESV in the study area. Among the ERI-influencing factors, the interaction of any two factors had a greater influence on ERI than any single factor itself (Figure 7B). Among them, the interactive interpretation of the distance to a highway and precipitation was the strongest, reaching 60.9%. The interaction of any two factors significantly enhanced the influence of each factor on the ERI, indicating that the spatial differentiation of the ERI in the study area was jointly affected by multiple factors.
FIGURE 7. Factor interaction detection for the spatial differentiation of ESV (A) and ERI (B). Note: DTH, distance to highway; AP, annual precipitation; ST, soil type.
ESV showed a trend of first decreasing and then increasing, while the ERI decreased year by year from 1990 to 2018. The driving force results show that the distance to a highway and NDVI are the main factors affecting the spatiotemporal differentiation of ESV and the ERI in the study area, while the interaction of distance to a highway and annual precipitation has the strongest effect on these variables in the study area (Yu et al., 2020; Sun, et al., 2021). ESV showed a trend of first decreasing and then increasing from 1990–2018. Coincidentally, the annual change in precipitation in the Ulan Buh Desert is similar to that of ESV, which also indirectly indicates the strong influence of precipitation on ESV (Sánchez-Canales et al., 2012). ESV in the study area reached its peak in 2018. This may be attributed to the increase in precipitation, which provided sufficient water for the growth of vegetation and crops (Long et al., 2022). At the same time, the construction of new roads also enabled the transmission of human and material resources, which was also conducive to the growth of vegetation (Klarenberg et al., 2018; Carvalho and Szlafsztein, 2019). This will further lead to the improvement of ecological functions such as food production and raw material supply. The ERI decreased year by year from 1990 to 2018. As the main factor affecting the distribution of the ERI, the NDVI showed an increasing trend during the study period. The increase in vegetation coverage plays an important role in suppressing sand dust storms and soil wind erosion (Yu, 2018), reducing ecological risks faced in the study area. After 2010, the construction of highways increased ecological risk in the study area to a certain extent. However, in general, the increase in precipitation and NDVI has offset the negative impact of highway construction on ecological risk, so ecological risk in the study area is decreasing year by year.
Spatially, previous studies (Wang, 2019) and this study found that higher ESV was mainly distributed across the northeast plain and Yellow River shore with lower risk levels. The areas of lower ESV were mainly concentrated in dunes in middle and other land areas in the west with higher risk. Forests, grassland and cultivated land are widely distributed in the northeast plain and along the Yellow River with relatively high vegetation coverage. The types of ecosystem services provided are complex and diverse, and ecosystem functions are relatively stable. Meanwhile, the transportation of human and material resources is very convenient due to the short distance to a highway, which is conducive to expanding the planting range of crops and the degree of water use. This will lead to the improvement of food production, raw material supply and other functions. The high vegetation coverage in the northeast plain helps suppress sand dust storms, thus reducing the possibility of ecological risks. Various sand dunes are widely distributed in the middle of the study area with relatively low vegetation coverage. The ESV provided is low due to the presence of relatively simple ecosystem functions. At the same time, the bare surface is also vulnerable to natural disasters such as sandstorms and landslides. The interaction of distance to a highway and precipitation had the strongest effect on the ERI, meaning that the closer an area is to a highway, the higher the degree of ecological risk faced by the arid region is. The northwestern part of the town of Jilantai and the town Aolunbulage are located close to a highway and experience relatively little annual precipitation, so they face high levels of ecological risk (Li L et al., 2022). In addition, the distributions of ESV and the ERI were related to annual precipitation. However, the overall difference in precipitation in this study area was not significant, so it had a limited influence.
In general, the total ESV in the Ulan Buh Desert increased from 1990 to 2018 but still presented high landscape ecological risk. To prevent the spread and transfer of high-ecological-risk areas, corresponding governance measures should be taken based on the zoning results of the spatial correlation between ESV and the ERI: the study area was dominated by low value-high risk areas, accounting for approximately 19.8% of the entire study area. Landscape types in these regions mainly include the Gobi Desert, mountainous regions and other land types. While the ESVs were low in these regions, they faced high levels of ecological risk. The protection and restoration of such land use types should be strengthened, and attention should be given to reducing damage to the ecological environments in these areas. Landscape types in the high value-low risk areas mainly included grasslands and cultivated land, and the ecosystem services provided by these land types were relatively significant. It is necessary to strengthen the protection and restoration of cultivated land ecosystems in this area and at the same time reduce human disturbances to the grassland ecosystems. Resources should be rationally used to maintain normal ecosystem functions; in addition, the Yellow River shore was identified as the main center of high value-high risk areas. While serving as the main supply area for ecosystem services in the study area, it also faced risks of drying up and being converted by other land types. Therefore, supplemental ecological water projects should be reasonably carried out to improve the utilization efficiency of water resources, optimize the use of Yellow River water resources and alleviate the discrepancy between the supply and demand of local water resources. Low value-low risk areas were scattered in the northeastern plain region, and landscape types in these areas mainly included dunes and cultivated land. All kinds of dunes present are very likely to expand to surrounding areas, resulting in enlarged land desertification areas. The “Three North Shelter Forest” project should be continued to prevent the transfer and expansion of these dunes. Meanwhile, ecological agriculture and deserticulture should be carried out in a concentrated manner to increase vegetation coverage and achieve the effects of windbreaks and sand fixation, thereby reducing ecological risk and improving ecosystem functions.
The purpose of this study was to explore the spatiotemporal variation characteristics of ESV and the ERI, along with their driving mechanisms, in the Ulan Buh Desert to provide a theoretical basis for the scientific management and optimization of land use patterns. However, this work has some limitations. First, the basic data used are insufficient. Only four-phase remote sensing image data for 1990, 2000, 2010 and 2018 are used, and these data cannot accurately express specific spatiotemporal changes in ESV and the ERI from 1990–2018. Second, there is an obvious scale effect in the spatiotemporal distribution characteristics of the ESV and landscape ERI results (Yang et al., 2021). This paper analyzes driving factors only for the entire study area and cannot fully explain differences or influencing factors in local areas. Therefore, further identifications should be made in the future, priority areas for ecological security should be further identified in the future, and the influencing factors of these priority areas should be explored in a more targeted way to provide more detailed scientific guidance for local ecological security governance.
Based on four-phase land use data of the Ulan Buh Desert for 1990 to 2018 and with the technical support of ArcGIS 10.2 and Geodetector, in this paper, we evaluated spatiotemporal differences in ESV and the ERI for the Ulan Buh Desert. The driving mechanism behinds these variations were revealed using the Geodetector model, and the following results were obtained:
1) The ESV of the Ulan Buh Desert showed a fluctuating growth trend, ranging from 7.00 × 108 yuan in 1990 to 6.17 × 108 yuan in 2000 and then increasing to 11.09 × 108 yuan in 2018; the change rate was approximately 58.41%. The study area was dominated by high-risk areas, which were mainly distributed in dune regions. The high-risk area decreased year by year from 1990–2018.
2) There was a certain negative correlation between the ESV and ERI of the Ulan Buh Desert from 1990–2018.
3) The Geodetector results show that the interaction between the distance to a highway and precipitation was the main factor affecting the spatiotemporal differentiation of ESV and the ERI in the Ulan Buh Desert.
The original contributions presented in the study are included in the article/Supplementary Material, further inquiries can be directed to the corresponding authors.
HL and RW wrote the manuscript. HS and WC helped design the structure of the manuscript and discussed the methods. JS collected the data. LWe, YZ, XZ, and LWa were involved in the study design and critically revised the manuscript. All authors contributed to the article and approved the submitted version.
This work is funded by the Science and Technology Major Project of Inner Mongolia (Grant Nos. 2022YFHH0024, 2022YFHH0017, 2021ZD0011 and 2021ZD12) and Yinshanbeilu Grassland Eco-hydrology National Observation and Research Station, China Institute of Water Resources and Hydropower Research (No. YSS202108) and the National Science Funds, P.R. China (Nos. 32160279 and 32161143025).
We acknowledge all the producers of the datasets used in this study. We are also grateful to the reviewers for their helpful comments on the manuscript.
The authors declare that the research was conducted in the absence of any commercial or financial relationships that could be construed as a potential conflict of interest.
The handling editor JW is currently organizing a Research Topic with the author LW.
All claims expressed in this article are solely those of the authors and do not necessarily represent those of their affiliated organizations, or those of the publisher, the editors and the reviewers. Any product that may be evaluated in this article, or claim that may be made by its manufacturer, is not guaranteed or endorsed by the publisher.
The Supplementary Material for this article can be found online at: https://www.frontiersin.org/articles/10.3389/fenvs.2022.1053797/full#supplementary-material
Al-Hameedi, W., Chen, J., Faichia, C., Nath, B., Al-Shaibah, B., and Al-Aizari, A. (2022). Geospatial analysis of land use/cover change and land surface temperature for landscape risk pattern change evaluation of baghdad city, Iraq, using CA–markov and ANN models. Sustainability 14 (14), 8568. doi:10.3390/su14148568
Bartolo, R., Dam, R., and Bayliss, P. (2012). Regional ecological risk assessment for Australia's tropical rivers: Application of the relative risk model. Hum. Ecol. Risk Assess. 18 (1), 16–46. doi:10.1080/10807039.2012.631467
Cao, Q., Xiao, H., Liu, T., Sun, J., Xia, J., Wang, D., et al. (2022). Soil organic carbon density and its influencing factors in croplands with different cultivation years in the Northeastern Ulan Buh Desert, China. Chin. J. Appl. Ecol. 33 (10), 2628–2634. doi:10.13287/j.1001-9332.202210.017
Cao, Q., Zhang, X., Lei, D., Guo, L., Sun, X., Wu, J. J. J. o. C. P., et al. (2019). Multi-scenario simulation of landscape ecological risk probability to facilitate different decision-making preferences. J. Clean. Prod. 227, 325–335. doi:10.1016/j.jclepro.2019.03.125
Carvalho, R., and Szlafsztein, C. (2019). Urban vegetation loss and ecosystem services: The influence on climate regulation and noise and air pollution. Environ. Pollut. 245, 844–852. doi:10.1016/j.envpol.2018.10.114
Costanza, R., d'Arge, R., Groot, R. D., Farber, S., Grasso, M., Hannon, B., et al. (1997). The value of the world's ecosystem services and natural capital. Nature 387, 253–260.
Daily, G. (1997). Nature's services: Societal dependence on natural ecosystems. Washington, DC: Island Press. doi:10.2307/3244191
Fadaei, E., Mirsanjari, M., and Amiri, M. (2020). Modeling of ecosystem services based on land cover change and land use using invest software in jahannama conservation area (case: Carbon sequestration ecosystemservice). Town Ctry. Plan. 12, 153–173. doi:10.22059/JTCP.2020.294342.670051
Forbes, V., and Calow, P. (2013). Use of the ecosystem services concept in ecological risk assessment of chemicals. Integr. Environ. Assess. Manag. 9 (2), 269–275. doi:10.1002/ieam.1368
Goldstein, J., Caldarone, G., Duarte, T., Daily, G., Hannahs, N., Mendoza, G., et al. (2012). Integrating ecosystem-service tradeoffs into land-use decisions. Proc. Natl. Acad. Sci. U. S. A. 109 (19), 7565–7570. doi:10.1073/pnas.1201040109
Gong, J., Cao, E., Xie, Y., Xu, C., Li, H., and Yan, L. (2021). Integrating ecosystem services and landscape ecological risk into adaptive management: Insights from a Western mountain-basin area, China. J. Environ. Manage. 281, 111817. doi:10.1016/j.jenvman.2020.111817
Heenkenda, M., and Bartolo, R. (2016). Regional ecological risk assessment using a relative risk model: A case study of the Darwin harbour, Darwin, Australia. Hum. Ecol. Risk Assess. 22, 401–423. doi:10.1080/10807039.2015.1078225
Hou, L., Wu, F., and Xie, X. J. E. I. (2020). The spatial characteristics and relationships between landscape pattern and ecosystem service value along an urban-rural gradient in Xi’an city, China. Ecol. Indic. 108, 105720. doi:10.1016/j.ecolind.2019.105720
Inner Mongolia Bureau of Statistics (2022). Inner Mongolia statistical yearbook. Beijing: China Statistics Press.
Ji, M., and Guo, Y. (1995). Discussion on the countermeasures of forestry development and utilization in the northeast of Ulan Buh Desert. J. Inn. Mong. For. Sci. Technol. (04), 43–47.
Jia, Y., Liu, Y., and Zhang, S. (2021). Evaluation of agricultural ecosystem service value in arid and semiarid regions of northwest China based on the equivalent factor method. Environ. Process. 8 (2), 713–727. doi:10.1007/s40710-021-00514-2
Jia, Y., Tang, X., and Liu, W. J. S. (2020). Spatial–temporal evolution and correlation analysis of ecosystem service value and landscape ecological risk in Wuhu City. Sustainability 12 (7), 2803. doi:10.3390/su12072803
Jin, T., Zhang, Y., Zhu, Y., Gong, J., and Yan, L. (2021). Spatiotemporal variations of ecosystem service values and landscape ecological risk and their correlation in forest area of loess plateau, China: A case study of ziwuling region. J. Appl. Ecol. 32 (5), 1623–1632. doi:10.13287/j.1001-9332.202105.030
Jin, Z. (2022). Ecohydrographic effects and sustainability of grain for green program in a small basin of loess plateau. J. Earth Environ. 13 (02), 121–131.
Jr, W., Helm, R., Adams, W., Clements, W., Cramer, M., Curry, M., et al. (2009). Translating ecological risk to ecosystem service loss. Integr. Environ. Assess. Manag. 5 (4), 500–514. doi:10.1897/IEAM_2009-009.1
Kanwar, P., Bowden, W., and Greenhalgh, S. (2015). A regional ecological risk assessment of the Kaipara Harbour, New Zealand, using a relative risk model. Hum. Ecol. Risk Assess. 4, 1123–1146. doi:10.1080/10807039.2014.976046
Karimian, H., Zou, W., Chen, Y., Xia, J., and Wang, Z. (2022). Landscape ecological risk assessment and driving factor analysis in Dongjiang river watershed. Chemosphere 307, 135835. doi:10.1016/j.chemosphere.2022.135835
Klarenberg, G., Muñoz-Carpena, R., Campo-Bescós, M., and Perz, S. (2018). Highway paving in the southwestern Amazon alters long-term trends and drivers of regional vegetation dynamics. Heliyon 4 (08), e00721. doi:10.1016/j.heliyon.2018.e00721
Li, B., Chen, D., Wu, S., Zhou, S., Wang, T., and Chen, H. (2016). Spatio-temporal assessment of urbanization impacts on ecosystem services: Case study of Nanjing City, China. Ecol. Indic. 71, 416–427. doi:10.1016/j.ecolind.2016.07.017
Li, C., Zhang, J., Philbin, S., Yang, X., Dong, Z., Hong, J., et al. (2022). Evaluating the impact of highway construction projects on landscape ecological risks in high altitude plateaus. Sci. Rep. 12, 5170. doi:10.1038/s41598-022-08788-8
Li, L., Tang, H., Lei, J., and Song, X. J. E. I. (2022). Spatial autocorrelation in land use type and ecosystem service value in Hainan Tropical Rain Forest National Park. Ecol. Indic. 137, 108727. doi:10.1016/j.ecolind.2022.108727
Li, W., Wang, Y., Xie, S., Sun, R., and Cheng, X. J. E. I. (2020). Impacts of landscape multifunctionality change on landscape ecological risk in a megacity, China: A case study of beijing. Ecol. Indic. 117, 106681. doi:10.1016/j.ecolind.2020.106681
Liu, C., Hou, Y., Chen, W., and Cui, H. (2021). Research on ecological risk characterization methods for urbanized areas based on ecosystem services. Acta Ecol. Sin. 41 (9), 3343–3353. doi:10.5846/stxb202003250686
Long, X., Lin, H., An, X., Chen, S., Qi, S., and Zhang, M. J. E. I. (2022). Evaluation and analysis of ecosystem service value based on land use/cover change in Dongting Lake wetland. Ecol. Indic. 136, 108619. doi:10.1016/j.ecolind.2022.108619
Mann, D., Anees, M., Rankavat, S., and Joshi, P. (2021). Spatio-temporal variations in landscape ecological risk related to road network in the Central Himalaya. Hum. Ecol. Risk Assess. 27 (2), 289–306. doi:10.1080/10807039.2019.1710693
Millennium Ecosystem Assessment (2005). Ecosystems and human well-being: A framework foe assessment. Washington, DC: Island Press.
Pan, N., Guan, Q., Wang, Q., Sun, Y., Li, H., and Ma, Y. J. J. o. C. P. (2021). Spatial differentiation and driving mechanisms in ecosystem service value of arid region: A case study in the middle and lower reaches of shule River Basin, NW China. J. Clean. Prod. 319, 128718. doi:10.1016/j.jclepro.2021.128718
Peng, J., Wang, Y., Zhang, Y., Wu, J., Li, W., and Li, Y. J. E. I. (2010). Evaluating the effectiveness of landscape metrics in quantifying spatial patterns. Ecol. Indic. 10 (2), 217–223. doi:10.1016/j.ecolind.2009.04.017
Qiao, B., Zhu, C.-X., Cao, X.-Y., Xiao, J.-S., Zhaxi, L.-D., Yan, Y.-Q., et al. (2020). Spatial autocorrelation analysis of land use and ecosystem service value in Maduo County, Qinghai Province, China at the grid scale. J. Appl. Ecol. 31 (5), 1660–1672. doi:10.13287/j.1001-9332.202005.014
Rahman, M., and Szabó, G. (2021). Impact of land use and land cover changes on urban ecosystem service value in Dhaka, Bangladesh. Land 10 (8), 793. doi:10.3390/land10080793
Ran, P., Hu, S., Frazier, A. E., Qu, S., Yu, D., and Tong, L. J. E. I. (2022). Exploring changes in landscape ecological risk in the Yangtze River Economic Belt from a spatiotemporal perspective. Ecol. Indic. 137, 108744. doi:10.1016/j.ecolind.2022.108744
Sánchez-Canales, M., Benito, A., Passuello, A., Terrado, M., Ziv, J., Acuña, V., et al. (2012). Sensitivity analysis of ecosystem service valuation in a Mediterranean watershed. Sci. Total Environ. 440, 140–153. doi:10.1016/j.scitotenv.2012.07.071
Sannigrahi, S., Chakraborti, S., Joshi, P. K., Keesstra, S., Sen, S., Paul, S. K., et al. (2019). Ecosystem service value assessment of a natural reserve region for strengthening protection and conservation. J. Environ. Manage. 244, 208–227. doi:10.1016/j.jenvman.2019.04.095
Song, J., Wen, L., Wang, F., Li, K., Wu, C., Zhang, H., et al. (2021). Spatiotemporal dynamics of ecosystem service value in Ulan Buh Desert. Acta Ecol. Sin. 41 (06), 2201–2211. doi:10.5846/stxb202005141228
Sun, M., Niu, W., Zhang, B., Geng, Q., and Yu, Q. (2021). Spatial-temporal evolution and responsesof ecosystem service value under land use change in the Yellow River Basin: A case study of shaanxi-gansu-ningxia region, northwest China. Chin. J. Appl. Ecol. 32 (11), 3913–3922. doi:10.13287/j.1001-9332.202111.016
Tian, P., Li, J., Gong, H., Pu, R., Cao, L., Shao, S., et al. (2019). Research on land use changes and ecological risk assessment in Yongjiang River Basin in Zhejiang Province, China. Sustainability 11 (10), 2817. doi:10.3390/su11102817
Wang, D., Ji, X., Li, C., and Gong, Y. J. S. (2021). Spatiotemporal variations of landscape ecological risks in a resource-based city under transformation. Sustainability 13 (9), 5297. doi:10.3390/su13095297
Wang, F. (2019). “Spatial-temporal dynamics and trade-offs analysis of ecosystem services in Ulan Buh Desert,”. (master's thesis) (Inner Mongolia: Inner Mongolia University).
Wang, H., Liu, X., Zhao, C., Chang, Y., Liu, Y., and Zang, F. J. E. I. (2021). Spatial-temporal pattern analysis of landscape ecological risk assessment based on land use/land cover change in Baishuijiang National nature reserve in Gansu Province, China. Ecol. Indic. 124, 107454. doi:10.1016/j.ecolind.2021.107454
Wang, H. (2021). Regional assessment of human-caused ecological risk in the Poyang Lake Eco-economic Zone using production–living–ecology analysis. PLoS ONE 16 (2), e0246749. doi:10.1371/journal.pone.0246749
Wang, J., Ma, J., Xie, F., and Xu, X. (2020). Improvement of remote sensing ecological index in arid regions: Taking Ulan Buh Desert as an example. Chin. J. Appl. Ecol. 31 (11), 3795–3804. doi:10.13287/j.1001-9332.202011.011
Wang, J., and Xu, C. (2017). Instrumental networking and social network building: How horizontal networking and upward networking create social capital. Acta Geogr. Sin. 72 (01), 116–134. doi:10.3724/sp.j.1041.2017.00116
Wang, J., Zhou, W., Pickett, S. T., Yu, W., and Li, W. J. S. (2019). A multiscale analysis of urbanization effects on ecosystem services supply in an urban megaregion. Sci. Total Environ. 662, 824–833. doi:10.1016/j.scitotenv.2019.01.260
Wang, X., Liu, X., Long, Y., Zhang, Y., Liang, W., Hong, M., et al. (2020). Spatial-temporal changes and influencing factors of ecosystem services in Shanguan City based on improved. Invest. Soil Water Cons. 27 (5), 381–388. doi:10.13869/j.cnki.rswc.2020.05.047
Woldeyohannes, A., Cotter, M., Biru, D., and Kelboro, G. (2020). Assessing changes in ecosystem service values over 1985–2050 in response to land use and land cover dynamics in abaya-chamo basin, southern Ethiopia. Land 9, 37. doi:10.3390/land9020037
Wu, J., Zhu, Q., Qiao, N., Wang, Z., Sha, W., Luo, K., et al. (2021). Ecological risk assessment of coal mine area based on “source-sink” landscape theory–A case study of Pingshuo mining area. J. Clean. Prod. 295, 126371. doi:10.1016/j.jclepro.2021.126371
Xie, G., Lu, C., Leng, Y., Zheng, D., and Li, S. (2003). Evaluation of ecological assets value in qinghai-tibet plateau. J. Nat. Resour. 18 (2), 189–196.
Xie, G., Zhang, C., Zhang, L., Chen, W., and Li, S. (2015). Improvement of the evaluation method for ecosystem service value based on per unit area. J. Nat. Resour. 30 (08), 1243–1254. doi:10.11849/zrzyxb.2015.08.001
Xu, W., Wang, J., Zhang, M., and Li, S. J. (2021). Construction of landscape ecological network based on landscape ecological risk assessment in a large-scale opencast coal mine area. J. Clean. Prod. 286, 125523. doi:10.1016/j.jclepro.2020.125523
Xu, X., Yang, G., Tan, Y., Zhuang, Q., Li, H., Wan, R., et al. (2016). Ecological risk assessment of ecosystem services in the Taihu Lake Basin of China from 1985 to 2020. Sci. Total Environ. 554-555, 7–16. doi:10.1016/j.scitotenv.2016.02.120
Yan, D., Yuan, L., Huang, H., Hu, X., and Yang, Z. (2021). Study on windproof effect of gauze sand barriers on mobile sand dunes in Ulan Buh Desert. Soil Water Cons. 28 (02), 198–202. doi:10.13869/j.cnki.rswc.2021.02.028
Yan, N., Liu, G., Xu, L., Deng, X., and Casazza, M. J. W. R. (2022). Emergy-based eco-credit accounting method for wetland mitigation banking. Water Res. 210, 118028. doi:10.1016/j.watres.2021.118028
Yang, M., Gao, X., Zhao, X., and Wu, P. J. S. o. T. T. E. (2021). Scale effect and spatially explicit drivers of interactions between ecosystem services—a case study from the loess plateau. Sci. Total Environ. 785, 147389. doi:10.1016/j.scitotenv.2021.147389
Yu, N. (2018). “Spatiotemporal patterns of ecosystem services and influencing factors in the Mu Us Sandy Land,”. China(master's thesis) (Beijing: Beijing Forestry University). doi:10.26949/d.cnki.gblyu.2018.000246
Yu, T., Bao, A., Xu, W., Guo, H., Jiang, L., Zheng, G., et al. (2020). Exploring variability in landscape ecological risk and quantifying its driving factors in the Amu Darya Delta. Int. J. Environ. Res. Public Health. 17 (1), 79. doi:10.3390/ijerph17010079
Zeng, H., and Liu, G. (1999). Analysis of regional ecological risk based on landscape structure. China Environ. Sci. 5, 454–457.
Zhang, F., Yushanjiang, A., and Wang, D. J. E. E. S. (2018). Ecological risk assessment due to land use/cover changes (LUCC) in Jinghe County, Xinjiang, China from 1990 to 2014 based on landscape patterns and spatial statistics. Environ. Earth Sci. 77 (13), 1–16. doi:10.1007/s12665-018-7676-z
Zhang, W., Chang, W. J., Zhu, Z. C., and Hui, Z. J. A. G. (2020). Landscape ecological risk assessment of Chinese coastal cities based on land use change. Appl. Geogr. 117, 102174. doi:10.1016/j.apgeog.2020.102174
Zhang, X., Du, H., Wang, Y., Chen, Y., Ma, L., Dong, T. J. H., et al. (2021). Watershed landscape ecological risk assessment and landscape pattern optimization: Take Fujiang River Basin as an example. Hum. Ecol. Risk Assess. 27 (9-10), 2254–2276. doi:10.1080/10807039.2021.1970511
Keywords: Ulan Buh Desert, ecosystem service value, ecological risk, spatiotemporal evolution, driving forces
Citation: Liu H, Wang R, Sun H, Cao W, Song J, Zhang X, Wen L, Zhuo Y, Wang L and Liu T (2023) Spatiotemporal evolution and driving forces of ecosystem service value and ecological risk in the Ulan Buh Desert. Front. Environ. Sci. 10:1053797. doi: 10.3389/fenvs.2022.1053797
Received: 26 September 2022; Accepted: 16 December 2022;
Published: 05 January 2023.
Edited by:
Juanle Wang, Institute of Geographic Sciences and Natural Resources Research (CAS), ChinaCopyright © 2023 Liu, Wang, Sun, Cao, Song, Zhang, Wen, Zhuo, Wang and Liu. This is an open-access article distributed under the terms of the Creative Commons Attribution License (CC BY). The use, distribution or reproduction in other forums is permitted, provided the original author(s) and the copyright owner(s) are credited and that the original publication in this journal is cited, in accordance with accepted academic practice. No use, distribution or reproduction is permitted which does not comply with these terms.
*Correspondence: Lu Wen, d2VubHVAaW11LmVkdS5jbg==; Xuefeng Zhang, eGZ6aGFuZzIwMDNAMTYzLmNvbQ==
†These authors have contributed equally to this work and share first authorship
Disclaimer: All claims expressed in this article are solely those of the authors and do not necessarily represent those of their affiliated organizations, or those of the publisher, the editors and the reviewers. Any product that may be evaluated in this article or claim that may be made by its manufacturer is not guaranteed or endorsed by the publisher.
Research integrity at Frontiers
Learn more about the work of our research integrity team to safeguard the quality of each article we publish.