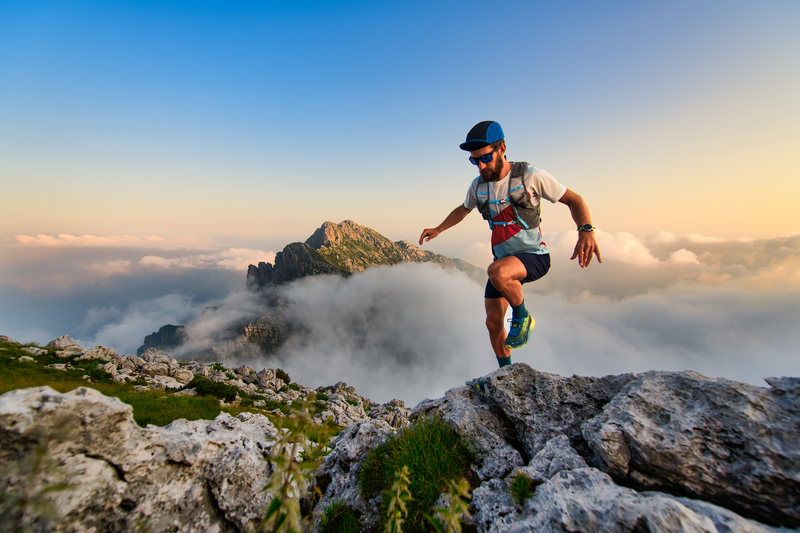
95% of researchers rate our articles as excellent or good
Learn more about the work of our research integrity team to safeguard the quality of each article we publish.
Find out more
ORIGINAL RESEARCH article
Front. Environ. Sci. , 01 March 2023
Sec. Environmental Economics and Management
Volume 10 - 2022 | https://doi.org/10.3389/fenvs.2022.1051636
This article is part of the Research Topic Fuzzy Mathematical Model and Optimization in Digital Green Innovation for Industry 5.0 View all 14 articles
Management ability improves organizational learning and innovation ability, thereby improving enterprise performance. Higher-ability management has more flexible and adaptive strategic thinking and ability, allowing it to quickly capture market opportunities, formulate long-term digital transformation strategies, gain competitive advantage through product and service innovation, and thus promote enterprise innovation performance. The research sample for this paper is A-share listed companies in the manufacturing industry from 2008 to 2019, and it integrates management capabilities with digital economy and green technology innovation. Our research shows that management ability can significantly boost manufacturing enterprises’ green technology innovation. Furthermore, regardless of whether the enterprise is state-owned or non-state-owned, management ability is positively related to green technology innovation performance, and internal control has a significant positive regulating effect on the relationship between them. Given these findings, this study offers important insights for strengthening the integration of the digital economy and green transformation, emphasizing the rationality of management capabilities, and developing policies for various enterprises.
It is necessary to investigate the digital transformation of the manufacturing industry against the backdrop of the digital economy. In 2016, the CPC Central Committee and the State Council issued the Outline of National Innovation-Driven Development Strategy, stating that innovation-driven development is the core support of national power, the core strategy of international competition, and the new trend of economic development, and that innovation-driven development should be included in the national priority strategy. According to data, China’s digital economy will be worth 39.2 trillion yuan in 2020, up 3.3 trillion yuan from last year, accounting for 38.6% of GDP, up 2.4 percentage points year on year. Both the “14th Five-Year Plan” and the long-term goal of 2035 propose that we seize the strategic opportunities of the new round of scientific and green technological revolutions and industrial revolutions, actively accelerate digital development, promote digital transformation, promote deep integration of the Internet, big data, artificial intelligence, and the real economy, and build a digital power with international competitiveness. Green innovation is gradually assuming a dominant position as a result of the rapid advancement of digital technology and the continuous advancement of high-quality development. Green innovation can not only directly generate economic value, but it can also have an impact on the ecological environment, achieve environmental performance, and ultimately realize high-quality development for the entire society and economy (Guo and Ji, 2022). China has a new understanding of the digital economy and green innovation as it enters a new era. This research proposes a novel concept of digital green innovation in manufacturing enterprises. It differs from green innovation, which is based on technological advancement, and digital innovation, which is based on artificial intelligence (Gunasekeran et al., 2021; Stahl, 2021; Yao et al., 2022). Green innovation is the process by which digital technologies such as 5G and the Internet of things are integrated into current green innovation and development (H. H. Zhou et al., 2021). Digital and green innovation are no longer separated from these two dimensions, but instead combine to form a more appropriate innovative form for current economic development. The incorporation of digital technology into the process of green innovation not only improves the efficiency of resource integration and the ability of environmental monitoring, but also serves as a key link between green production, life, and a beautiful environment, thereby realizing high-quality green innovation development (Xu and Zhang, 2020). Green manufacturing aims to save energy and reduce consumption through digital technology, intelligent manufacturing, improving production efficiency, and producing the same or even higher output value with less consumption and emissions (Acquah et al., 2021; Mandal et al., 2021). At the same time, the demand for energy-saving products and technologies in green manufacturing coincides with the demand for new products and technologies in intelligent manufacturing (Zhou et al., 2021; Yin et al., 2022a).
The factors that influence enterprise green technology innovation are broadly classified as government factors and market factors. Most studies concentrate on government factors such as environmental regulations (Berrone et al., 2013; Yu et al., 2017; Qu et al., 2022) and government support (Xie et al., 2015; Wu et al., 2022); however, the positive impact of environmental oversight on enterprise green transformation is gradually fading (Leiter et al., 2011). To some extent, environmental regulation will raise enterprise operating costs (Berrone et al., 2013; Qu et al., 2022), discouraging some businesses from pursuing green transformation. Furthermore, while government assistance partially subsidizes the resources required for green technological innovation, this may lead to a problem of dependence. In reality, businesses must be more dynamic and adaptable in their allocation of innovative resources (Diewert et al., 2018; El-Kassar and Singh, 2019; Feng et al., 2022). Due to the limitations of government assistance, some scholars began to investigate the impact of market factors on enterprise green innovation. Lin et al. (2013) used Vietnam as an example to demonstrate that market demand correlates positively with green product innovation. Cuerva et al. (2014) believe that the advancement of external technology promotes the dynamic capability of businesses, thereby enhancing green innovation. Cao et al. (2021) and Feng et al. (2022) stated that digital finance can solve the green innovation financing problem, avoiding over-reliance on the government and putting enterprises in a better position to promote green technological innovation; however, market factors cannot be ignored (Wei et al., 2015; Cao et al., 2021; Yin et al., 2022b).
The digital economy, as the integration of information technology and economic production mode, offers a new strategic option for China’s industrial transformation (Yang et al., 2021). It opens up a new research avenue for green technology innovation by releasing social productive forces, altering knowledge transfer methods, and lowering transaction costs (He et al., 2021; Li et al., 2022). Dou and Gao. (2022) believe that cloud computing and big data will accelerate the transformation of production modes in areas with a high level of digital economy, requiring enterprises to update their equipment (Wang and Fan, 2020), eliminate the traditional production mode (Cao et al., 2021), and achieve a balance between economic and environmental development. Because green technology innovation is more risky than general innovation, sufficient resources are required to support green technology innovation (Wu et al., 2022). Because green technology innovation in enterprises is expensive, risky, and profitable, enterprise managers will not consider green innovation technology when making business decisions. As a result, green technology innovation will be hampered if manufacturing enterprises cannot rationally allocate limited resources. The digital economy can break time and space constraints with digital technologies, leading to the sharing and transfer of labor and capital resources, thereby contributing to green total factor growth rates (Song et al., 2020; Li et al., 2022). Furthermore, it is worth noting that the digital economy is a driver of economic structural transformation, which will aid in the elimination of “highly polluting, energy-intensive” industries and their transformation into green industrial factories (Lu et al., 2021). Based on the above analysis, we have introduced digital transformation as an intermediary between management capabilities and green technology innovation. Management ability is an important human capital of an enterprise that can create a sustainable competitive advantage for the enterprise, play a critical role in the enterprise’s survival and development, and have a direct impact on the enterprise’s green technological innovation. Currently, research on the economic consequences of managerial competence focuses primarily on corporate performance, earnings management, tax avoidance activities, and investment efficiency, with few empirical studies on managerial competence and enterprise innovation, and the findings are inconsistent. Based on the above analysis, we introduced management ability as a mediating force between the digital economy and green technology innovation.
The level of digitalization has an increasing impact on the performance of green technological innovation. Currently, research on the impact of digital transformation on enterprise innovation performance has been delayed; previously, empirical research on the impact of digital transformation on enterprise innovation performance focused on the macro-level impact of regional innovation level and factor allocation efficiency, while micro-level research on the relationship between digital transformation and enterprise innovation was limited. There have been numerous studies on innovation investment and patent application, but there have been few studies on innovation performance. To summarize, can management ability improve the performance of innovation? How can traditional manufacturing management effectively improve green technological innovation through digital transformation in the post-epidemic era, when the global economic situation is complicated and severe, and the domestic economic growth rate is slowing? Does internal control promote or inhibit management ability and technological innovation in China, given the late implementation of internal control and the imperfect system? What are the distinctions between the functions and influences mentioned above in China’s special property rights? All of these are critical issues that require immediate attention.
This paper’s main contributions are as follows: First, this paper identifies the text of sample companies’ reports, and then determines the degree of digital transformation by dividing the frequency of indicators in the reports from the application of digital technology, which is useful for expanding and enriching relevant theories of digital transformation measurement. Second, this paper employs the DEA BCC model to improve Demerjian’s model for measuring management competence by excluding the influence of production scale and by controlling time, bringing the influence of fiscal and monetary policy into the model, in order to more accurately measure the management competence of Chinese listed companies. Third, from the standpoint of digital transformation, this paper establishes a theoretical framework of management ability to promote green technological innovation of enterprises via the action path of “digital transformation,” thereby expanding the research boundary of related theories. Fourth, it investigates the moderating effect of internal control on the relationship between managerial ability and green technological innovation performance, providing support for the theory of internal control promotion.
Green technological innovation, as a driving force for economic development, is an important source of competitive advantage for enterprises, which is critical to China’s economic development. Sehmookler (1966) believed that “innovation efficiency” is essentially the input-output ratio of enterprise innovation activities when it comes to measuring green technological innovation performance. Charnes and Cooper (1978) proposed using the CCR model of the data envelopment method to measure innovation efficiency, and Banker (1984) broke the traditional constant return on scale limitation by using the BBC model to accurately reflect innovation efficiency. Many academics use financial indicators to investigate and analyze the innovation efficiency of businesses. Hirshleifer et al. (2013) calculated an enterprise’s green technological innovation efficiency by dividing the number of patents granted by the sum of R&D expenditures over the previous 2 years. In terms of research on the influencing factors of enterprises’ innovation activities, some scholars believe that preferential tax policies, financial subsidies, and intellectual property protection provided by the government can help stimulate enterprises’ innovation; enterprises with higher corporate tax rates have less R&D expenditure; the higher the proportion of institutional investors, the higher the proportion of enterprise innovation investment; and the improvement of CEO competiveness.
Management, as an enterprise’s most important human capital, plays a critical role in its daily operations and major decisions. The “Top echelon theory” was proposed by Hambrick and Mason (1984), who argued that top managers have different background characteristics and will inevitably be influenced by these characteristics when making decisions. Bertrand and Schoar (2003) believe that management’s background characteristics will influence organizational behavior more than time and company specific characteristics. A large number of behavioral research findings show that competent management can develop better strategies and correctly predict competitor behavior. Some scholars measure management ability using the manager’s fixed effect, historical rate of return, stock price fluctuation, media attention, and executive compensation, but these methods have limitations. Demerjian et al. (2012) distinguished management competence from corporate efficiency and proposed a DEA-Tobit two-stage analysis method to assess overall management competence. Scholars in the United States and elsewhere began to investigate the economic consequences of management competence, such as corporate profits, investment activities, tax avoidance activities, financing constraints, corporate strategy, earnings management, on-the-job consumption, and so on.
There are few literatures on managerial competence and green technological innovation at the moment, and the conclusions about their relationship are contradictory. Some academics believe that management ability can promote enterprise green technological innovation. For the first time, Chen et al. (2015) demonstrated that management competence can promote enterprise innovation, and that companies with higher management competence can create more valuable products and have a competitive advantage. According to Yung and Chen (2018), managers with higher ability are generally more willing to take risks, which contributes to an increase in enterprise R&D investment. Based on data from publicly traded companies in the manufacturing industry, Cheng and Wang (2019) discovered that management capabilities are positively related to enterprise green technological innovation. Mishra (2019) discovered a U-shaped relationship between business management capability and enterprise R&D, which means that as business management capability improves, enterprise innovation capability decreases and then increases. According to Yung and Chen (2018) research, management capability is negatively related to enterprise innovation investment, with this relationship being stronger in state-owned enterprises. Furthermore, Zhou and Peng (2019) demonstrated empirically, using data from China’s A-share listed companies, that high-capacity management would inhibit enterprise innovation input and output for the purpose of risk prevention.
Management plays a critical role in the process of enterprise innovation, and its ability becomes the key to enterprise innovation success. According to Barker and Mueller (2002), management, as the primary source of strategic decision-making, has a direct impact on enterprise green technological innovation. Based on data from publicly traded companies in the manufacturing industry, Cheng and Wang (2019) discovered that management capabilities are positively related to enterprise green technological innovation. The following four aspects highlight management’s role in promoting green technological innovation. To begin with, more capable management has a higher risk tolerance and control ability. In the course of their innovation activities, businesses are bound to face a variety of risks. Because higher-level management has greater risk-taking ability, they are more willing to take risks and pursue high-risk innovation projects. At the same time, more capable management can control risks more calmly and be adept at identifying opportunities in risks, allowing them to make sound decisions and promote the improvement of innovation performance. Second, more capable management can better identify investment opportunities and make sound strategic decisions, thereby improving enterprise innovation performance. According to relevant research, high-capacity management can better understand the current company strategy and the industry’s development trend, more accurately predict market demand, look for favorable investment opportunities, evaluate the potential investment value, and choose projects with higher NPV to invest in, making them more likely to invest in high-value innovative projects. Third, improved management skills can effectively alleviate financial constraints and provide financial security for enterprise innovation. According to Andreou et al. (2015), higher management ability can reduce the degree of information asymmetry by effectively communicating enterprise value and thus raising more funds. According to Chemmanur et al. (2010), higher management ability has a stronger signal transmission function, which can effectively avoid friction in information communication between inside and outside the enterprise, lower the risk premium of external financing, and thus alleviate the financing constraint problem. Fourth, competent management has strong integration and allocation abilities, allowing it to fully absorb and utilize idle enterprise resources, fully exploit the innovative advantages of researchers, and better implement innovative projects. According to Barney (1991), management ability is an important human capital of an enterprise that can create a sustainable competitive advantage. According to Lee et al. (2018)’s research, high-capacity managers have more experience in enterprise resource management, which allows them to make better use of enterprise resources and implement innovative projects. Furthermore, Chang et al. (2015) discovered that competent managers can influence innovation outcomes by establishing the best framework for R&D personnel, thereby maximizing their innovation potential. According to Zacher (2015), complementary management capabilities can stimulate employees’ innovative ability, thereby promoting the team’s innovative performance. As a result, we propose the following assumptions:
H1. There is a positive correlation between managerial competence and green technological innovation performance, that is, higher managerial competence can improve the technological innovation performance of enterprises.
The impact of digital level on the performance improvement of green technological innovation is increasing as the digital economy develops, and more and more scholars are beginning to pay attention to digital transformation and green technological innovation of enterprises. Abrell et al. (2016), from the perspective of innovation results, believed that digital application improved the operation status of small and medium-sized enterprises, enhanced product performance and service quality, and thus enhanced the innovation performance of enterprises; The research of Jahanmir and Cavadas (2018) found that the continuous improvement of digitalization level had a profound impact on enterprise organization mode, information transmission efficiency, information utilization efficiency and information decision-making management, thus improving the innovation performance level of enterprises to some extent; The research of Nambisan et al. (2017) pointed out that “internet plus” has a positive impact on innovation efficiency, and economic development and government funding have a regulatory effect; The research of Barrett et al. (2015) proves that the digitalization level of enterprises is positively related to the operational innovation performance and market innovation performance of enterprises, and it is found that the digitalization level of different industries has different influences on innovation performance, and there is an optimal threshold for the improvement of innovation performance, so the digital transformation needs to be further deepened; Aslam et al. (2020) has proved that dynamic capability plays an intermediary role in the relationship between digital transformation and enterprise innovation performance.
Management ability is primarily manifested in learning ability, opportunity identification, networking, strategic positioning, business innovation, organization and management, and so on. Enterprise digital transformation cannot be separated from continuous improvement and advancement of management capabilities, which promotes enterprise green technological innovation. First and foremost, the ability of management to identify opportunities and build networks can lead to the acquisition of more key resources required for digital transformation, thereby improving enterprise green technological innovation performance. Premaratne (2002) discovered that resource acquisition has a positive impact on small business performance; that is, small businesses can only improve their performance by establishing entrepreneur networks to acquire key resources. According to Andreou et al. (2015), strong management ability can effectively improve the efficiency of obtaining key resources, thereby promoting enterprise growth. Second, the strong learning ability of management can assist enterprises in better analyzing, forecasting, and evaluating in the complex digital environment, avoiding the risks of the enterprise’s digital transformation period, and avoiding the negative impact brought by the innovative performance of digital applications. Third, the management’s strong organizational management ability can promote the mutual transmission and sharing of digital knowledge and ability among organization members, and continuously improve the innovation performance with digital resources as the core, thus improving the innovation performance. Enterprises can improve their innovation performance through organizational collaboration and innovation, according to Subramanian (1996) and Hubert and Xuereb (1997). According to Vial (2019), entrepreneurship improves organizational learning and innovation ability, there by improving enterprise performance. Finally, higher-ability management has more flexible and adaptive strategic thinking and ability, allowing it to quickly capture market opportunities, formulate long-term digital transformation strategies, gain competitive advantage through product and service innovation, and thus promote enterprise innovation performance. Li (2020) discovered that entrepreneurs’ strategic and management abilities contributed significantly to the growth of businesses. Simultaneously, increased management ability can significantly improve the company’s performance by implementing relevant diversification strategies. As a result, we propose the following assumptions:
H2. The management ability can improve the green technological innovation performance of enterprises through digital transformation, that is, digital transformation plays an intermediary role.
Faced with increasingly fierce market competition and a more complex business environment, higher-level management has a deeper understanding and grasp of the internal control system of enterprise business activities, and can flexibly respond to changes in the internal and external environment of enterprises, effectively design and operate internal controls, dynamically and timely adjust internal controls of enterprises, and improve implementation. Demerjian et al. (2013) discovered through questionnaire research that the more attention management pays, the more effective the enterprise’s internal control is. According to Bikkil et al. (2015)’s research, higher management ability can better identify the company’s internal control defects, thereby improving the quality of internal control.
In 2008, the Basic Standards of Enterprise Internal Control stated unequivocally: “Internal control is a process carried out by the board of directors, the board of supervisors, the managers, and all enterprise employees with the goal of achieving the control objectives. The manager is in charge of organizing and overseeing the enterprise’s internal control system on a daily basis ". As more academics become interested in enterprise internal control, two opposing viewpoints emerge. Some academics believe that internal control is beneficial in promoting enterprise green technological innovation. Cheng and Wang (2019) assessed the internal control quality of enterprises using the internal control index of Chinese listed companies and discovered that internal control can improve enterprise innovation. Internal control and R&D subsidy are significantly positively correlated with enterprise innovation and performance, according to research by Chen et al. (2015). According to Hall’s (2002) research, the quality of an enterprise’s internal control plays a significant role in promoting its innovation performance. Other scholars believe that overly strict institutionalization frequently leads to rigid management, which is incompatible with the implementation of flexible green technological innovation. Jensen (2010) estimated the productivity of 432 companies’ enterprise capital expenditure and R&D expenditure items, revealing that many companies had significant inefficiency, demonstrating the failure of internal control systems. Furthermore, Hu and Jefferson (2004) demonstrates that a strict internal control system increases management’s innovation risk, and the innovation environment is not guaranteed, limiting management’s innovation efficiency. However, because the implementation of internal control in China has been delayed and an overly strict system has yet to be established, the theory of internal control promotion may be more appropriate for China’s national circumstances.
The following three aspects primarily reflect the role of internal control in promoting the relationship between management capabilities and green technological innovation. First, high-quality internal control can effectively control enterprise risks and provide assurance for high-risk innovation activities undertaken by management. Through risk assessment and control activities of internal control, enterprises can analyze the scientificity and feasibility of innovative projects, carry out combined development and innovation, and improve the risk warning system, reducing uncertainty in the innovation process, effectively preventing the company’s innovation risks, and thus improving the efficiency of green technological innovation. Second, effective internal control can create a good platform for information and communication, promote the transmission of internal and external information, and improve the reliability of financial reports, thereby increasing investors’ trust in financial reports, reducing the degree of internal and external information asymmetry, alleviating enterprise financing constraints, and raising more funds for enterprises’ innovative activities. Third, internal control can be an effective supervision mechanism in the innovation process. High-level internal control can effectively supervise enterprise management’s behavior, prevent management from taking more private benefits in order to “maximize personal benefits,” and increase excessive investment caused by agency conflicts, reducing enterprise innovation efficiency. As a result, the following assumptions are advanced in this paper:
H3. Internal control plays a positive regulatory role in the relationship between management capabilities and green technological innovation.
From 2008 to 2019, China’s A-share manufacturing listed companies in Shanghai and Shenzhen were used as research samples in this paper. The original data are from the CSMAR database, the DIB internal control and risk management database, the RESSET database, and the Osiris database, and are manually collected from reports published by listed companies. The samples are screened based on the research needs: first, financial companies with accounting standards that differ significantly from those of other industries are excluded; second, companies with special treatment, such as ST and *ST, are excluded; and finally, companies with missing data are deleted. Following screening, 2084 unbalanced panel data are obtained. To avoid the influence of outliers, the main continuous variables are truncated at the 1% and 99% levels.
Green technological innovation (IE). According to Sehmookler (1966), “innovation efficiency” is essentially the input-output ratio of enterprises to innovation activities. According to Mao and Zhang (2018), when a patent is in the application stage, it indicates that the enterprise’s innovation activities have yielded results, so the number of patent applications is regarded as the most accurate and direct indicator of innovation output. This paper, based on the research method of Hirshleifer et al. (2013), focuses on the ratio of innovation output to R&D investment as a measure of green technological innovation performance (IE). The precise method is to divide the number of patent applications by the total R&D investment over the previous 3 years. Because the value of green technological innovation performance is small, this paper has done some treatment to facilitate observation, and the detailed calculation method is shown in Table 1.
Management abilities (MA). Demerjian et al. (2012) proposed the DEA-Tobit two-stage analysis method in this paper, which separated management ability from company efficiency and then measured management ability as a whole. The BCC model of Data Envelopment Analysis (DEA) is used in the first stage to estimate the company’s efficiency (FE) by industry, as shown in model (2). Operating income (Sales) is the output variable, while the input variables are operating cost (CoGS), sales and management expenses (SG&A), net assets, plant and equipment (PPE), net R&D expenditure (R&D), Goodwill, and net Intangible assets (intangibles). The variables Sales, CoGS, and SG&A are current year data; the variables PPE, R&D, Goodwill, and Intangible are end-of-year data, because management’s past decisions on these assets are expected to affect current income.
Transformation to digital (DT). At the moment, scholars both at home and abroad use two main measurement methods for digital transformation. The first step is to create a digital transformation questionnaire and assess it using a Likert7-point scale. The second step is to recognize the text of the sample company’s report, and finally to determine the degree of digital transformation by calculating the frequency of the breakdown indicators of artificial intelligence technology, blockchain technology, cloud computing technology, big data technology, and digital technology application in the report. Based on the practice of Flammer (2021), this paper employs the Robert frequency of digital transformation indicators in sample company reports and the natural logarithm plus 1 as digital transformation proxy variables. The greater the value of this indicator, the greater the enterprise’s degree of digital transformation.
Internal control (IC) (IC). Domestic scholars typically assess the quality of internal control based on the internal control defects disclosed by enterprises or the development of comprehensive internal control evaluation indicators. Shenzhen Dibo Company’s internal control index (since 2000) comprehensively, objectively, and comprehensively reflects the internal control quality of enterprises across seven dimensions: internal environment, control activities, risk assessment, information communication, supervision and inspection, whether the accounting firm issues an assessment report, and whether independent directors and the board of supervisors express their opinions. As a result, the natural logarithm of “internal control index” is used as a proxy variable for internal control quality in this paper (IC). The higher the value of this index, the more effective the enterprise internal control system and the higher the quality of internal control.
In order to control other factors that may affect the green technological innovation performance of enterprises, this paper mainly selects company Size (size), established years (Age), asset-liability ratio (Lev), return on assets (ROA), total asset turnover rate (ATO) and R&D intensity (RD) as control variables, and controls the annual dummy variables. See Table 1 for definitions of variables.
Multiple regression analysis is widely used in fields such as econometrics, ecology and finance (Dormann et al., 2013). Many studies often use a set of predictor variables to measure the response to a particular variable. In view of Wei et al. (2015) and He et al. (2021), multiple regression models have been used to measure the relationship between digital technology, management ability, and green innovation. To test the relationship between regional digital economy and green technology innovation, we constructed the following regression models (see model Eq. 1 and model Eq. 2).
The BCC model of Data Envelopment Analysis (DEA) is used in the first stage to estimate the company’s efficiency (FE) by industry, as shown in model Eq. 2. Operating income (Sales) is the output variable, while the input variables are operating cost (CoGS), sales and management expenses (SG&A), net assets, plant and equipment (PPE), net R&D expenditure (R&D), Goodwill, and net Intangible assets (intangibles). The variables Sales, CoGS, and SG&A are current year data; the variables PPE, R&D, Goodwill, and Intangible are end-of-year data, because management’s past decisions on these assets are expected to affect current income.
In the above equation,
In the second stage, this paper employs the Tobit regression model for regression, with the residual error representing the company’s management capability (MA), as shown in model Eq. 3. Size denotes the size of the company; Market denotes the market share; F denotes the level of free cash flow; Age denotes the number of years listed; BSC denotes the diversified management level of enterprises, as measured by the Herfindal index of operating income; and FCI denotes foreign business. If there is income from overseas business, the value will be 1; otherwise, it will be 0.
Based on the above variable design, we develop model Eq. 4 to investigate the impact of management capabilities on enterprise green technological innovation performance. We use the explained variables that lag by one period because the innovation output has a certain lag. Using the method of Baron and Kenny (1986), this paper develops models Eqs. 5, 6 to test the intermediary effect of digital transformation. We developed a model to investigate the moderating effect of internal control on management ability and green technological innovation performance Eq. 7.
where IE is the dependent variable, representing the green technology innovation of enterprises. i represents individual enterprises, and t represents years. Control represents all control variables. In the models, we also control year fixed effects yeart. ɛ is random error term. β1, β2, β3,β4, β5, β6 and β7 represent the coefficients, indicating the coefficient of digital finance development and control variables. β0 represent the model intercept terms.
The descriptive statistical results of the main variables are shown in Table 2. The highest value of green technological innovation (IE) among them is 27.8173, the lowest value is 4.3108, the average value is 7.9812, and the standard deviation is 3.6174, demonstrating that there are significant differences in green technological innovation performance among enterprises. The average management competence (MA) is −0.0713, indicating that management competence in China’s manufacturing industry is generally low and needs to be improved further. The minimum value is −0.4061, the maximum value is 0.3461, and the standard deviation is 0.1621, indicating that different company managers have varying abilities to use existing assets to generate output, with significant differences. The highest digital transformation degree (DT) is 3.9942, the lowest is 0.6717, and the average is 1.6102, indicating that the digital transformation degree of China’s manufacturing industry is low and needs to be popularized and applied further. The highest internal control (IC) is 7.0134, the lowest is 6.0972, the average is 6.4371, and the standard deviation is 0.1191, indicating that there is not a significant difference in internal control quality among listed Chinese manufacturing companies.
According to the regression results in Table 3, there is a 1% significant positive correlation between management ability and enterprise innovation performance (coefficient is 1.382, t value is 2.81), indicating that the stronger the management ability, the higher the enterprise innovation performance, and H1 is supported. To further test the impact of different managers’ abilities on enterprise innovation performance, we divided the samples into two groups based on the median of managers’ abilities: High-ability and Low-ability, and performed regression using model Eq. 5, as shown in the results. Managers’ abilities (MA) are significantly positively correlated with enterprises’ innovation performance (IE) at the 1% level (coefficient is 3.643), indicating that the higher the management ability, the stronger its promotion effect on innovation performance.
Models Eqs. 4–6 are constructed in this paper using the intermediary utility test method developed by Baron and Kenny (1986) as a reference to determine whether management capabilities can improve enterprise innovation performance through digital transformation. The results of model Eq. 4 in Table 3 show that managerial competence (MA) is significantly positively correlated with innovation performance (IE) at the 1% level (coefficient is 1.382, t value is 2.81); model Eq. 5 in Table 3 shows that there is a significant positive correlation between management competence (MA) and digital transformation (DT) at the 5% level (coefficient is 0.317, t value is 2.19); (coefficient is 1.416, t value is 2.71). As a result, we believe that digital transformation has a partial intermediary effect and is managed.
The analysis result of model Eq. 7 in Table 3 reflects the influence of internal control quality on the relationship between management capability and innovation performance. The interaction term MA✕IC is significantly positive at 1% level (coefficient is 8.629, t value is 2.82). It shows that the degree of internal control has a positive regulating effect on management ability and innovation performance, that is, the higher the quality of internal control, the more obvious the effect of management ability on improving enterprise innovation performance. The hypothesis is supported.
Table 4 shows that there is a 10% significant positive correlation between management capability and enterprise innovation performance in state-owned enterprises (coefficient is 2.412, t value is 1.89). Management competence (MA) is positively correlated with digital transformation (DT) at the 1% level (coefficient is 0.786, t value is 3.31), at the 5% level (coefficient is 0.397, t value is 2.28), and at the 10% level (coefficient was 11.917, t value was 1.81), indicating There is a significant positive correlation between management capability and enterprise innovation performance in non-state-owned enterprises (coefficient is 1.298, t value is 2.91), and internal control has a significant positive regulating effect (coefficient is 7.013, t value is 1.72), but the intermediary effect of digital transformation is not significant. As a result, when compared to state-owned enterprises, non-state-owned enterprises’ management ability does not make good use of digital transformation to improve enterprise innovation performance.
This paper employs the Chow test and the Permutation test to validate the relationship between managerial competence and innovation performance in model Eq. 4 the difference in the moderating effect of internal control between state-owned enterprises and non-state-owned enterprises in model Eq. 7. The findings show that there is no significant difference in the promotion of managerial competence to innovation performance between state-owned enterprises and non-state-owned enterprises. However, in both state-owned and non-state-owned enterprises, internal control has a significant positive moderating effect on the relationship between managerial competence and innovation performance.
In order to ensure the validity of the research results, this paper further uses two methods to test the robustness. Firstly, this paper uses the method of Goldfarb and Tucker (2019) for reference, and uses the ratio of the sum of R&D investment in the current year and the previous year to the operating income to measure the innovation performance of enterprises, which is recorded as IE1 and regressed again. Secondly, this paper uses the practice of Gurevitch et al. (2018) for reference, and adds some corporate governance factors that may have an impact on the innovation performance of enterprises to make a further robustness test. The added control variables are management Salary, management shareholding ratio (Msharehold), independent director ratio (IndDir), and the concurrent status of chairman and general manager (Dual). It can be seen from the test results in Table 5 that it is completely consistent with the previous empirical results, and the conclusion is robust.
With the rapid development of the digital economy, some scholars have begun to focus on the relationship between digitalization and green development. Few studies, however, have extended the research to green technology innovation and discussed the impact of management capabilities on green technology innovation. In practice, when implementing green technology innovation, active digitalization and deliberate acceleration of digitalization can help it cross the digital divide and realize green technology innovation more quickly. The conclusion of this paper, in theory, expands on previous research and clarifies the complex linear relationship between management capability and green technology innovation. At the same time, we conducted a heterogeneity test, taking into account the unique characteristics of each enterprise. To begin with, the degree of green technology innovation differs greatly between state-owned enterprises and non-state-owned enterprises due to differences in enterprise property rights. The management capabilities of enterprises with varying property rights may have varying effects on their green technology innovation. The reason for this is that, in recent years, the state has paid close attention to green sustainable development, supported green technology behaviors, and provided subsidies and policy incentives for green technology innovation research and development. As a result, in the relationship between internal control and green technology innovation, state-owned enterprises have some advantages over non-state-owned enterprises. External supervision has an impact on the quality of enterprise internal control (Ma et al., 2020). The greater the external supervision, the more self-interested enterprise management will be limited, while the financing environment will be improved and green technology innovation will be encouraged.
Second, the level of green technology innovation varies depending on the enterprise’s management capabilities. To begin, this paper discusses the impact of digitalization on enterprise green technology innovation. This research will help enterprises better understand green technology innovation, accelerate the pace of digitalization, reduce the “risk effect” of digitalization, bridge the digital divide, and realize “profit” as soon as possible. Second, the relationship between management capability and green technology innovation is discovered to vary depending on the nature of property rights, digital level, and internal control. It provides an empirical foundation for various types of businesses to implement digital strategies. It enables businesses and governments to examine digital economic performance from a variety of perspectives. Finally, this study contributes to a better understanding of the feasible mode of Chinese enterprises’ green technology innovation under China’s new normal, as well as the critical role of digitalization in enterprises’ green technology innovation. Simultaneously, it provides a new idea for developing countries and countries that pursue economic growth but ignore environmental problems to carry out green technology innovation at the micro level, as well as a foundation for their green policy formulation. As a result, the research conclusion of this paper is beneficial to enterprises in actively and rapidly meeting the challenge of digitalization, shortening the inhibition effect of digitalization, and thus improving the ability and effect of green governance and realizing green transformation.
Thirdly, Both X inefficiency theory and new institutional economics suggest that the internal management of business organizations is the cause of widespread inefficiencies (Bi et al., 2019). Management ability improves organizational learning and innovation ability, thereby improving enterprise performance. Finally, higher-ability management has more flexible and adaptive strategic thinking and ability, allowing it to quickly capture market opportunities, formulate long-term digital transformation strategies, gain competitive advantage through product and service innovation, and thus promote enterprise innovation performance (Vial, 2019). As firms expand in size, the internal pyramid hierarchy increases, and problems such as information friction arise. As a result, information frictions are more serious in large organizations than in SMEs, internal transaction costs are higher, and resources are more wasted. Initially, digitalization may not promote green transformation or even inhibit it.
Fourthly, Internal control plays a positive regulatory role in the relationship between management capabilities and green technological innovation. High-quality internal control can effectively control enterprise risks and provide assurance for high-risk innovation activities undertaken by management. Through risk assessment and control activities of internal control, enterprises can analyze the scientificity and feasibility of innovative projects, carry out combined development and innovation, and improve the risk warning system, reducing uncertainty in the innovation process, effectively preventing the company’s innovation risks, and thus improving the efficiency of green technological innovation. Internal control can be an effective supervision mechanism in the innovation process. High-level internal control can effectively supervise enterprise management’s behavior, prevent management from taking more private benefits in order to “maximize personal benefits,” and increase excessive investment caused by agency conflicts, reducing enterprise innovation efficiency.
In this paper, the research samples are listed companies in the Shanghai and Shenzhen A-share manufacturing industries from 2008 to 2019, and the DEA-Tobit two-stage model proposed by Demerjian et al. (2012) is used as a reference to measure managerial competence, and the influence of managerial competence on enterprise green technological innovation performance is empirically tested. It has been discovered that strong management ability can improve enterprise green technological innovation performance; digital transformation serves as a partial intermediary in the relationship between management ability and enterprise green technological innovation performance; the higher the internal control quality, the more obvious the effect of management ability on improving enterprise innovation performance. Simultaneously, this paper examines the heterogeneity of the property right nature of the aforementioned relationship. We discover that managerial competence is positively correlated with green technological innovation performance in both state-owned and non-state-owned enterprises, with no significant difference. Internal control also has a significant positive moderating effect on the relationship between management ability and innovation performance; however, digital transformation plays a significant intermediary role only in state-owned enterprises. It is clear that the management capability of non-state-owned enterprises has not made good use of digital transformation to improve enterprise innovation performance, and the benefits of digital transformation have not been fully utilized. Finally, to ensure the robustness of the research findings, this paper re-measures green technological innovation performance and incorporates control variables related to corporate governance, and the estimated results are completely consistent with the previous empirical findings.
The following conclusions can be drawn:
(1) Because of its high risk tolerance, opportunity discovery, information transmission, and resource integration, management plays a positive role in promoting green technological innovation and enhancing enterprise competitive advantage. As the digital economy develops, enterprise management can provide more key resources for digital transformation through strong comprehensive ability, reasonably avoid digital transformation risks, continuously strengthen the role of digital resources as the core, formulate long-term digital transformation strategies, and improve enterprise green technological innovation performance by improving product performance and service quality.
(2) Against the backdrop of China’s late implementation of internal control and an imperfect system, internal control can effectively control risks, build a good information and communication platform, and exert an effective supervision mechanism, actively promoting the improvement of management’s ability to green technological innovation performance and supporting the theory of internal control promotion.
(3) Non-state-owned enterprises’ management ability has not made good use of digital transformation to improve enterprise innovation performance, and the role of digital transformation has not been well played. Based on the heterogeneity of ownership, environmental policies should be differentiated for different enterprises. Due to the lack of supervision, green transformation should be added to performance appraisals in SOEs. For non-SOEs, governments should provide more support to ensure that funding and talents are sufficient for green technology innovation.
Green technological innovation is critical as a strategic foundation for resolving the dilemma of green economic development and environmental protection. This paper not only provides a different perspective from the existing literature for the analysis of green technology innovation, but it also provides ideas for how to modify green technology innovation decision-making behaviors among manufacturing enterprises under environmental regulation.
The empirical findings of this paper have significant theoretical and practical implications. The empirical evidence presented above may provide some illumination for promoting green technology innovation. For example, the government should appropriately increase subsidies to manufacturing enterprises. First, the government should provide friendly policies for manufacturing enterprises, such as increasing economic incentives, tax incentives, and institutional guarantees, and strengthen support for green R&D of manufacturing enterprises, to increase the time available and motivation for enterprises to carry out green innovation activities.
First, continue to deepen the digital transformation. Enterprises should increase their capital investment in digital transformation, create a reasonable budget, and keep digital transformation costs under control. Simultaneously, everyone should support R&D, promotion, and application activities in the digital transformation process. Actively construct a digital information network cooperation platform, establish an open digital innovation experimental area, and continue to collaborate with the government and other enterprises via the network platform to improve the quality of products and services. Give priority to the introduction of digital talents, as well as the development of digital and intelligent knowledge and skills among employees in this enterprise.
Second, increase government assistance. The government should increase subsidies for green technological innovation activities and provide incentives based on the social benefits of innovation output. At the government level, we should also recruit talents, actively introduce domestic and foreign digital talents, create a digital talent highland, strengthen information infrastructure construction, and provide more accurate services to enterprises by utilizing the national professional concept and technology of digital transformation and upgrading. While developing a digital transformation leader, we should also pay close attention to the digital transformation of non-state-owned enterprises and small and medium-sized businesses. Government agencies should provide targeted subsidies for enterprises of various sizes and economic conditions based on the types of imported technologies, investment amount, and other factors. Furthermore, the government should improve the policy environment for the spread of green technology innovation. First and foremost, the government should improve the system to deal with the risk of green technology innovation spreading. The government should establish a risk response mechanism to reduce the uncertain risk of green technology innovation diffusion in the process of manufacturing enterprises introducing, digesting, absorbing, and applying new technologies, in addition to providing financial and tax policy support. This will assist manufacturing firms in gaining confidence and resolving existing issues related to the development of green innovation. Second, the government should develop appropriate intellectual property policies, such as establishing a green patent and trademark system and managing intellectual property rights confidentially. Its goal is to fully realize the potential of intellectual property rights while also promoting green technology research, application, and popularization.
Then, improve enterprise internal control. Enterprises should strengthen their corporate governance systems, implement a standardized and sound internal control system, and increase the level of internal control. Simultaneously, enterprises should pay attention to the flexibility of their internal control systems in order to avoid impeding the enthusiasm for green technological innovation.
Finally, focus on the development and supervision of management skills. On the one hand, enterprises should fully improve management’s skills, learning ability, and networking ability, as well as improve market acumen and formulate a reasonable incentive mechanism for management, in order to better align their own interests with enterprise goals. On the other hand, improve and optimize the appointment, evaluation, elimination, and other related mechanisms of managers, and raise the default cost of managers; develop corresponding rules and regulations to effectively restrict management’s behavior, paying special attention to the degree of deviation between control and ownership, as well as the concurrent positions of chairman and general manager, etc., so that the supervisory fun can be fully realized.
Some limitations of this study point to areas for future research. First and foremost, the research sample is from China. Future research should include global samples to broaden the results’ applicability. Second, the digital economy is a complex system that is constantly evolving as new digital technology is developed. The indicators of the digital economy must be improved further. Third, the measurement of green technology innovation should be researched further. Although the number of green patent applications is frequently used to measure green technological innovation, the information conveyed by this metric is limited. Additional research could look into the process of green technology innovation, such as the quality of green patents. Finally, the role of management ability in the digital economy and green technology innovation is discussed in this paper. However, this may not be the only path. Further research can investigate the dynamic capabilities and financial constraints of businesses in depth.
The original contributions presented in the study are included in the article/Supplementary Material; further inquiries can be directed to the corresponding author.
All authors listed have made a substantial, direct, and intellectual contribution to the work and approved it for publication.
The authors declare that the research was conducted in the absence of any commercial or financial relationships that could be construed as a potential conflict of interest.
All claims expressed in this article are solely those of the authors and do not necessarily represent those of their affiliated organizations, or those of the publisher, the editors and the reviewers. Any product that may be evaluated in this article, or claim that may be made by its manufacturer, is not guaranteed or endorsed by the publisher.
Abrell, T., Pihlajamaa, M., and Kanto, L. (2016). The role of users and customers in digital innovation: Insights from B2B manufacturing firms. Inf. Manag. 53 (3), 324–335. doi:10.1016/j.im.2015.12.005
Acquah, I. S. K., Essel, D., Baah, C., Agyabeng-Mensah, Y., and Afum, E. (2021). Investigating the efficacy of isomorphic pressures on the adoption of green manufacturing practices and its influence on organizational legitimacy and financial performance. J. Manuf. Technol. Manag. 32, 1399–1420. doi:10.1108/jmtm-10-2020-0404
Andreou, P. C., Ehrlich, D., and Louca, C. (2015). Managerial ability and firm performance: Evidence from the global financial crisis. SSRN Electron. J. 63 (02), 59–78. doi:10.2139/ssrn.2633216
Aslam, H., Blome, C., Roscoe, S., and Azhar, T. M. (2020). Determining the antecedents of dynamic supply chain capabilities. Supply Chain Manag. Int. J. 25 (4), 427–442. doi:10.1108/scm-02-2019-0074
Banker, R. D. (1984). Estimating most productive scale size using data envelopment analysis. Eur. J. Operational Res. 17 (1), 35–44. doi:10.1016/0377-2217(84)90006-7
Barker, V. L., and Mueller, G. C. (2002). CEO characteristics and firm R&D spending. Manag. Sci. 48 (6), 782–801. doi:10.1287/mnsc.48.6.782.187
Barney, J. (1991). Firm resources and sustained competitive advantage. J. Manag. 17 (1), 99–120. doi:10.1177/014920639101700108
Baron, R. M., and Kenny, D. A. (1986). The moderator-mediator variable distinction in social psychological research: Conceptual, strategic, and statistical considerations. J. Pers. Soc. Psychol. 51 (6), 1173–1182. doi:10.1037/0022-3514.51.6.1173
Barrett, M., Davidson, E., Prabhu, J., and Vargo, S. L. (2015). Service innovation in the digital age: Key contributions and future directions. MIS Q. 39 (1), 135–154. doi:10.25300/misq/2015/39:1.03
Berrone, P., Fosfuri, A., Gelabert, L., and Gomez-Mejia, L. R. (2013). Necessity as the mother of ‘green’inventions: Institutional pressures and environmental innovations. Strateg. Manag. J. 34 (8), 891–909. doi:10.1002/smj.2041
Bertrand, M., and Schoar, A. (2003). Managing with style: The effect of managers on firm policies. Q. J. Econ. 118 (4), 1169–1208. doi:10.1162/003355303322552775
Bi, Q., li, H., and Yu, L. (2019). The impact and its mechanisms of the executive with environmental experiences on corporate green transformation. Guangdong Univ. Finance Econ. 34 (05), 4–21.
Bikkil, J., Mitra, S., and Hossain, M. (2015). Earnings quality, internal control weaknesses and industry-specialist audits. Rev. Quantitative Finance Account. 45 (1), 1–32. doi:10.1007/s11156-013-0431-3
Cao, S., Nie, L., Sun, H., Sun, W., and Taghizadeh-Hesary, F. (2021). Digital finance, green technological innovation and energy-environmental performance: Evidence from China's regional economies. J. Clean. Prod. 327, 129458. doi:10.1016/j.jclepro.2021.129458
Charnes, A., and Cooper, W. (1978). Managerial economics-past, present, future. Enterp. Manag. 1, 5–23.
Chemmanur, T. J., Paeglis, I., and Simonyan, K. (2010). Management quality and equity issue characteristics: A comparison of SEOs and IPOs. Financ. Manag. 39 (4), 1601–1642. doi:10.1111/j.1755-053x.2010.01124.x
Chen, Y., and Podolski, E. J. (2015). Veeraraghavan. Does managerial ability facilitate corporate innovative success? J. Empir. Finance 34, 313–326. doi:10.1016/j.jempfin.2015.08.002
Cheng, J., and Wang, Q. (2019). Managerial Ability, enterprise technological innovation and brand value. Friends Account. 04, 79–84.
Cuerva, M. C., Triguero-Cano, Á., and Córcoles, D. (2014). Drivers of green and non-green innovation: Empirical evidence in low-tech SMEs. J. Clean. Prod. 68, 104–113. doi:10.1016/j.jclepro.2013.10.049
Demerjian, P., Lev, B., and McVay, S. (2012). Quantifying managerial ability: A new measure and validity tests. Manage. Sci. 58 (7), 1229–1248. doi:10.1287/mnsc.1110.1487
Demerjian, P. R., Lev, B., Lewis, M. F., and McVay, S. E. (2013). Managerial ability and earnings quality. Account. Rev. 88 (2), 463–498. doi:10.2308/accr-50318
Diewert, W. E., Fox, K. J., and Schreyer, P. (2018). The digital economy, new products and consumer welfare Burkina Faso: Economic Statistics Centre of Excellence. Available at: https://EconPapers.repec.org/RePEc:nsr:escoed:escoe-dp-2018-16.
Dou, Q., and Gao, X. (2022). The double-edged role of the digital economy in firm green innovation: Micro-evidence from Chinese manufacturing industry. Environ. Sci. Pollut. Res. 29, 67856–67874. doi:10.1007/s11356-022-20435-3
El-Kassar, A. N., and Singh, S. K. (2019). Green innovation and organizational performance: The influence of big data and the moderating role of management commitment and HR practices. Technol. Forecast. Soc. change 144, 483–498. doi:10.1016/j.techfore.2017.12.016
Feng, S., Zhang, R., and Li, G. (2022). Environmental decentralization, digital finance and green technology innovation. Struct. Chang. Econ. Dyn. 61, 70–83. doi:10.1016/j.strueco.2022.02.008
Flammer, Caroline (2021). Corporate green bonds. J. Financial Econ. 142 (2), 499–516. doi:10.1016/j.jfineco.2021.01.010
Goldfarb, A., and Tucker, C. (2019). Digital economics. J. Econ. Literature 57 (1), 3–43. doi:10.1257/jel.20171452
Guo, L., and Ji, S. Q. (2022). The impact of energy enterprise heterogeneity on green innovation capability – from the perspective of spatial spillover resource development and market.
Gurevitch, J., Koricheva, J., Nakagawa, S., and Stewart, G. (2018). Meta-analysis and the science of research synthesis. Nature 555, 175–182. doi:10.1038/nature25753
Hambrick, D., and Mason, P. (1984). Upper echelons: The organization as a reflection of its top managers. Acad. Manage. Rev. 9 (2), 193–206. doi:10.5465/amr.1984.4277628
He, Z., Lu, W., Hua, G., and Wang, J. (2021). Factors affecting enterprise level green innovation efficiency in the digital economy era–evidence from listed paper enterprises in China. BioResources 16 (4), 7648–7670. doi:10.15376/16.4.7648-7670
Hirshleifer, D. A., Hsu, P. H., and Li, D. (2013). Innovative efficiency and stock returns. J. Financial Econ. 107 (3), 632–654. doi:10.1016/j.jfineco.2012.09.011
Hu, A. G., and Jefferson, G. H. (2004). Returns to research and development in Chinese industry: Evidence from state-owned enterprises in beijing. China Econ. Rev. 15 (1), 86–107. doi:10.1016/s1043-951x(03)00028-2
Hubert, G., and Xuereb, J. M. (1997). Strategic orientation of the firm and new product performance. J. Mark. Res. 34, 77–90. doi:10.2307/3152066
Jahanmir, S. F., and Cavadas, J. (2018). Factors affecting late adoption of digital innovations. J. Bus. Res. 88, 337–343. doi:10.1016/j.jbusres.2018.01.058
Jensen, M. C. (2010). The modern industrial revolution exit and the failure of internal control systems. J. Appl. Corp. Finance 22 (1), 43–58.
Lee, C. C., Wang, C. W., Chiu, W. C., and Tien, T. S. (2018). Managerial ability and corporate investment opportunity. Int. Rev. Financial Analysis 57, 65–76. doi:10.1016/j.irfa.2018.02.007
Leiter, A. M., Parolini, A., and Winner, H. (2011). Environmental regulation and investment: Evidence from European industry data. Ecol. Econ. 70 (4), 759–770. doi:10.1016/j.ecolecon.2010.11.013
Li, F. (2020). The digital transformation of business models in the creative industries:A holistic framework and emerging trends. Technovation 92-93, 102012. doi:10.1016/j.technovation.2017.12.004
Li, J., Chen, L., Chen, Y., and He, J. (2022). Digital economy, technological innovation, and green economic efficiency—Empirical evidence from 277 cities in China. MDE. Manage. Decis. Econ. 43 (3), 616–629. doi:10.1002/mde.3406
Lin, R. J., Tan, K. H., and Geng, Y. (2013). Market demand, green product innovation, and firm performance: Evidence from Vietnam motorcycle industry. J. Clean. Prod. 40, 101–107. doi:10.1016/j.jclepro.2012.01.001
Lu, H., Song, C., and Lingling, L. (2021). Digital economy, innovation environment and urban innovation capabilities. Sci. Res. Manag. 42 (4), 35. doi:10.19571/j.cnki.1000-2995.2021.04.004
Ma, S., Zhang, Y., Liu, Y., Yang, H., Lv, J., and Ren, S. (2020). Data-driven sustainable intelligent manufacturing based on demand response for energy-intensive industries. J. Clean. Prod. 274, 123155. doi:10.1016/j.jclepro.2020.123155
Mandal, P., Perumal, G., Arora, H. S., Ghosh, S. K., and Grewal, H. S. (2021). Green manufacturing of nanostructured Al-Based sustainable self-cleaning metallic surfaces. J. Clean. Prod. 278, 123373. doi:10.1016/j.jclepro.2020.123373
Mao, C. X., and Zhang, C. (2018). Managerial risk-taking incentive and firm innovation: Evidence from FAS 123R. J. Financ. Quant. Anal. 53 (2), 867–898. doi:10.1017/s002210901700120x
Mishra, C. S. (2019). Does managerial ability drive firm innovativeness? IEEE Trans. Eng. Manag. 68 (4), 1139–1154. doi:10.1109/tem.2019.2923892
Nambisan, S., Lyytinen, K., Majchrzak, A., and Song, M. (2017). Digital innovation management: Reinventing innovation management research in a digital world. MIS Q. 41 (1), 223–238. doi:10.25300/misq/2017/41:1.03
Qu, F., Xu, L., and Chen, Y. (2022). Can market-based environmental regulation promote green technology innovation? Evidence from China. Front. Environ. Sci. 757, 823536. doi:10.3389/fenvs.2021.823536
Song, M., Zhao, X., and Shang, Y. (2020). The impact of low-carbon city construction on ecological efficiency: Empirical evidence from quasi-natural experiments. Resour. Conserv. Recycl. 157, 104777. doi:10.1016/j.resconrec.2020.104777
Stahl, B. C. (2021). Artificial intelligence for a better future: An ecosystem perspective on the ethics of AI and emerging digital technologies. Berlin, Germany: Springer Nature, 124.
Subramanian, A., S., and Nilakanta, S. (1996). Organizational innovativeness: Exploring the relationship between organizational determinants of innovation, types of innovations, and measures of organizational performance. Omega 24 (6), 631–647. doi:10.1016/s0305-0483(96)00031-x
Vial, G. (2019). Understanding digital transformation: A review and a research agenda. J. Strategic Inf. Syst. 28 (02), 118–144. doi:10.1016/j.jsis.2019.01.003
Wang, S. L., and Fan, Y. (2020). Study on the contribution of data production factors. Shanghai J. Econ. 10 (07), 32–39. doi:10.19626/j.cnki.cn31-1163/f.2020.07.005
Wei, Z., Yuguo, J., and Jiaping, W. (2015). Greenization of venture capital and green innovation of Chinese entity industry. Ecol. Indic. 51, 31–41. doi:10.1016/j.ecolind.2014.10.025
Wu, G., Xu, Q., Niu, X., and Li, T. (2022). How does government policy improve green technology innovation: An empirical study in China. Front. Environ. Sci. 723 (9), 794–799. doi:10.3389/fenvs.2021.799794
Xie, X. B., Wu, Y., and Yan, L. (2015). Enterprise green development path and policy suggestions under the background of digital age. Ecol. Econ. 31 (11), 88–91. doi:10.3969/j.issn.1671-4407.2015.11.020
Xu, X. C., and Zhang, M. H. (2020). Research on the scale measurement of China’s digital economy – from the perspective of international comparison China industrial economy, 23–41.
Yang, X. D., Wu, H., Ren, S., Ran, Q., and Zhang, J. (2021). Does the development of the internet contribute to air pollution control in China? Mechanism discussion and empirical test. Struct. Chang. Econ. Dyn. 56, 207–224. doi:10.1016/j.strueco.2020.12.001
Yao, X., Chen, L. S., and Zhang, Y. Z. (2022). Carbon trading mechanism and enterprise green innovation: Based on triple difference model scientific research management.
Yin, S., Dong, T., Li, B., and Gao, S. (2022b). Developing a conceptual partner selection framework: Digital green innovation management of prefabricated construction enterprises for sustainable urban development. Buildings 12, 721. doi:10.3390/buildings12060721
Yin, S., Zhang, N., Ullah, K., and Gao, S. (2022a). Enhancing digital innovation for the sustainable transformation of manufacturing industry: A pressure-state-response system framework to perceptions of digital green innovation and its performance for green and intelligent manufacturing. Systems 10, 72. doi:10.3390/systems10030072
Yu, W., Ramanathan, R., and Nath, P. (2017). Environmental pressures and performance: An analysis of the roles of environmental innovation strategy and marketing capability. Technol. Forecast. Soc. Change 117, 160–169. doi:10.1016/j.techfore.2016.12.005
Yung, K., and Chen, C. (2018). Managerial ability and firm risk-taking behavior. Rev. Quant. Finan. Acc. 51 (4), 1005–1032.
Zacher, H., R. K., and Rosing, K. (2015). Ambidextrous leadership and team innovation. Leadersh. Organ. Dev. J. 36 (1), 54–68. doi:10.1108/lodj-11-2012-0141
Zhou, H. H., Li, H. X., and Zhao, L. R. (2021). Research on the impact of digital transformation of manufacturing industry on green innovation performance – the regulatory role of digital level technology and management.
Keywords: management ability, green technological innovation, digital transformation, internal control, nature of the property right
Citation: Guang-lin X and Tao M (2023) How can management ability promote green technology innovation of manufacturing enterprises? Evidence from China. Front. Environ. Sci. 10:1051636. doi: 10.3389/fenvs.2022.1051636
Received: 23 September 2022; Accepted: 11 October 2022;
Published: 01 March 2023.
Edited by:
Shi Yin, Agricultural University of Hebei, ChinaReviewed by:
Luigi Aldieri, University of Salerno, ItalyCopyright © 2023 Guang-lin and Tao. This is an open-access article distributed under the terms of the Creative Commons Attribution License (CC BY). The use, distribution or reproduction in other forums is permitted, provided the original author(s) and the copyright owner(s) are credited and that the original publication in this journal is cited, in accordance with accepted academic practice. No use, distribution or reproduction is permitted which does not comply with these terms.
*Correspondence: Xu Guang-lin, cmV0cmV0dHRAMTYzLmNvbQ==
†These authors have contributed equally to this work
Disclaimer: All claims expressed in this article are solely those of the authors and do not necessarily represent those of their affiliated organizations, or those of the publisher, the editors and the reviewers. Any product that may be evaluated in this article or claim that may be made by its manufacturer is not guaranteed or endorsed by the publisher.
Research integrity at Frontiers
Learn more about the work of our research integrity team to safeguard the quality of each article we publish.