- 1School of Economics and Management, China University of Petroleum, Beijing, China
- 2School of Economics and Management, Beijing University of Chemical Technology, Beijing, China
- 3School of Economics, Fuyang Normal University, Fuyang, China
- 4Beijing Xuanyu Intelligent Technology Co., LTD., Beijing, China
To empirically investigate as to whether and how public infrastructure influences the global greenhouse effect, this study utilizes a panel dataset of 35 countries over the period 2003–2019 for regression estimation. Furthermore, regional heterogeneous and mediation effects between infrastructure and carbon dioxide (CO2) emissions are explored. The primary findings insist that: 1) at the global level, public infrastructure is positive associated with CO2 emissions. In other words, strengthening infrastructure at this stage is not conducive to mitigating the greenhouse effect; 2) public infrastructure construction development in Belt and Road Initiative (BRI) countries has a greater positive impact on CO2 emissions than in non-BRI countries; and 3) the mediation roles of industrial upgrading and trade openness are established; specifically, trade openness and industrial upgrading are valid transmission routes through which public infrastructure affects CO2 emissions. Based on the above three findings, a number of policy implications related to accelerate low-carbon construction of infrastructure and promote industrial upgrading are proposed.
Highlights
➢ This study examines the potential carbon effect of public infrastructure.
➢ The heterogeneous and mediation effects between variables are explored.
➢ Public infrastructure is positively related to greenhouse effect.
➢ Infrastructure in BRI countries has a greater impact on CO2 than in non-BRI.
➢ Infrastructure can affect CO2 through trade openness and industrial upgrading.
1 Introduction
Over recent years, climate change has gained significant attention following the arresting report of the Intergovernmental Panel on Climate Change (IPCC), and has prompted global policymakers to formulate policies at different administrative levels to mitigate the increasing emission of greenhouse gases, mainly carbon dioxide (CO2) emissions (Jiang and Guan, 2016; Zhao et al., 2021b; Dong et al., 2021; Dou et al., 2021). As part of the ambitious vision of the United Nations Framework Convention on Climate Change (UNFCCC), it is well-recognized that it is crucial for the public and private sectors to fulfil these goals and ensure actions on the ground (Bakhtiari, 2018; Hickmann et al., 2021). Despite the considerable efforts made by various stakeholders to implement the Paris Agreement and the Kyoto Protocol Agreement, global carbon emissions continue to show worrying trends.
While global economic activities have brought prosperity to human societies, they have also aggravated environmental degradation (Saboori and Sulaiman, 2013; Ozcan et al., 2020; Rahman, 2020). These activities include the evolution of industrial structures, urbanization expansion and international trade. In particular, dramatic increased in greenhouse gas emissions from infrastructure expansion have had a negative impact on the ecological environment on which residents depend. Infrastructure construction is the cornerstone of the evolution of human society (Cui and Sun, 2019). The infrastructure session of the United Nations 2030 Agenda for Sustainable Development stresses the need to develop quality, reliable, sustainable and disaster-resilient infrastructure by 2030 to support economic growth and enhance human well-being. In the current stage, public infrastructure construction shrinks by 2.7% globally in 2020 due to the COVID-19 pandemic. 2021 marks the beginning of a global infrastructure recovery as governments actively launch relevant stimulus policies; however, headwinds such as rising costs for materials and labour and the lingering risk of the pandemic also constrain the rapid recovery in the infrastructure sector.
As the artery and key breakthrough for economic growth, the sustainability of infrastructure plays a crucial role in accelerating social development (Liu et al., 2014). On the one hand, well-developed infrastructure provides convenient transportation conditions, production and communication conditions, it reduces trade costs, attracts capital inflows and promotes national economic growth (Sun and Cui, 2018; Toader et al., 2018; Banerjee et al., 2020). On the other hand, pubic infrastructure construction is characterized by large investment scale, long operation cycle and strong externalities, all of which have irreversible impacts on the ecological environment (Luo et al., 2018; Huang et al., 2020; Jafri et al., 2021). Therefore, the global construction of green infrastructure is imperative. To this end, by employing a sample dataset across the globe for the period 2003–2019, this study first calculates the public infrastructure composite index, and then testes the potential carbon effect of public infrastructure. Furthermore, the heterogeneous and mediating effects of infrastructure on carbon emissions are also explored.
Three contributions contribute in three ways to complement the current literature. First, the public infrastructure composite index is calculated with the improved entropy method that included four dimensions—energy, propagation, technology, and transportation. This can effectively measure public infrastructure across the globe and provide a valid reference for other scholars. Second, this study empirically examines the heterogeneous impacts of public infrastructure on CO2 emissions by dividing the full sample into two sub-samples, namely BRI and non-BRI countries; exploring the differences in the carbon emission effects of infrastructure construction can contribute to the smooth implementation of the BRI and the achievement of the global carbon neutrality target for governments in various countries. Third, whether trade openness and industrial upgrading are effective routes for infrastructure to influence greenhouse gas emissions is creatively checked. This is of great significance for exploring new transmission channels in public infrastructure-CO2 emissions nexus and formulating efficient carbon reduction strategies.
This study arranges the rest of the contents in the following steps. Section 2 reviews the related literature on the infrastructure-CO2 nexus, followed by the model and data presented in Section 3. Section 4 reports and analyzes the empirical findings on the benchmark regression, heterogeneous analysis, and mediation analysis. Section 5 summarizes the entire study and proposes the policy implications.
2 Literature review
Public infrastructure construction develops rapidly, which confers convenience to residents in their daily life and supports harmonious and fosters steady socio-economic evolution, and some scholars have focused on effects brought about by construction (Schäffler and Swilling, 2013; Karjunen et al., 2017; Parker and Zingoni de Baro, 2019). For instance, based on the panel dataset of 281 cities in China for the period 2003–2013, Xie et al. (2016) empirically explored the effect of transport infrastructure on the environment using a spatial Durbin model. The findings suggest that transport infrastructure and urban environment are negatively correlated; in other words, the vigorous construction of traffic facilities has a deteriorating effect on urban ecological environment. Notably, this finding is supported by Churchill et al. (2021), who adopted Organization for Economic Cooperation and Development (OECD) countries as their research objects. They creatively extended the carbon emission effect of infrastructure to the transport field and found that every 1% increase in transport infrastructure could contribute to a 0.4% reduction in CO2 emissions. To check whether natural gas-related infrastructure expansion contributes to a reduction in CO2 emissions, Dong et al. (2020) applied the panel dataset that spanned the period 2004–2017 to test the potential impact of natural gas infrastructure on CO2 emissions. Their findings insist that strengthening the construction of natural gas infrastructure is an effective channel and driving force for mitigating CO2 emissions. Wei and Ullah (2022) empirically investigated the potential influence of tourism and digitalization infrastructure on environmental quality in Asian economies with the quantile regression techniques. They maintained that a significant enhancement of tourism and digital infrastructure could be effective in improving environmental quality. Furthermore, Xu et al. (2022) focused their study on highway infrastructure by introducing highway mileage and CO2 emissions into the traditional production density model. Through a panel data study of 278 Chinese cities from 2003 to 2016, it was found that highway infrastructure and CO2 emissions showed a significant inverted U-shaped relationship in both local and peripheral cities. Hao and Cho (2022) checked the linkage between urban public infrastructure and CO2 emissions over the period 1990–2019 and concluded that urban public infrastructure produced a negative influential effect on CO2 emissions.
While some scholars have assessed the environmental effects of infrastructure related to various industries in the certain economies or regions, few have examined the underlying impact of infrastructure on global greenhouse effect from a global perspective. This perspective, however, provides a useful reference for governments to help achieve carbon neutrality at the level of infrastructure construction. Furthermore, as BRI is under robust promotion, it is crucial to determine whether the impact of infrastructure construction on greenhouse effect differs between BRI and non-BRI countries. It is also worthwhile to test in depth whether trade openness and industrial upgrading are effective routes for public infrastructure to contribute to CO2 emissions.
3 Model and data
3.1 Model
The environmental Kuznets curve (EKC) hypothesis suggests that a significant inverted U-shaped relationship exists between economic growth and environmental pollutants; thus, the economic growth and its square term are simultaneously added into the econometric model. Furthermore, to focus on the potential role of infrastructure in addressing the greenhouse effect, we introduce the public infrastructure into the model. The foreign investment industrial development, population density, and labor force are also added to solve the estimation bias due to omitted variables; the multidimensional estimated framework is constructed as follows:
where the subscripts i and t represent the country and year, respectively, C O 2 refers to the total amount of CO2 emissions and Infra indicates public infrastructure. Pgdp, FI, Str, Den, and Lab denote economic growth, foreign investment, industrial evolution, population density, and labor force, respectively.
On this basis, to effectively avoid estimation bias caused by data dimension differences and potential heteroscedasticity. The natural logarithm processing of Eq. 1 is treated in this study as follows:
where
Notably, Eq. 2 indicates the total effect of public infrastructure on CO2 emissions. To empirically check whether trade openness and industrial upgrading are two primary channels in affecting the nexus between public infrastructure and CO2 emissions, this study conducts another regression analysis using the mediation effect model. In this regard, trade openness and industrial upgrading are regarded as mediation variables. The specific test procedures of the mediation effect model can be seen as follows:
i) In Eq. 2, if the regression coefficient of public infrastructure is significant, a linear regression equation of infrastructure affecting trade openness and industrial upgrading is constructed in Eq. 3.
ii) After constructing of Eq. 3, another equation reflecting the impact of public infrastructure and the mediation variables (i.e., lnTra and lnInd) on CO2 emissions is subsequently constructed in Eq. 4.
In both two equations, the coefficient of public infrastructure in Eq. 4 (i.e.,
3.2 Variables and data
To address the concerns about the underlying effect of the vigorous construction of public facilities on greenhouse gas emissions across the globe, a panel dataset of 35 countries from 2003 to 2019 is applied to conduct the empirical analysis. Due to data deficiencies, other years and countries are excluded in the sample data. The specific measures and data of the variables are presented as follows:
3.2.1 Explained variable
CO2 emissions (denoted as CO2). As the main explained variable, data on CO2 emissions were collected from the former British Petroleum (BP, 2020).
3.2.2 Explanatory variable
Public infrastructure (denoted as Infra), the core explanatory variable in our study, is a multidimensional indicator including four dimensions. namely energy, propagation, transportation, and technology (for specific indicators, please refer to Supplementary Table A1 in the Supplementary Appendix). Notably, when an indicator system contains multiple dimensions, it is crucial what weights are assigned to these dimensions. In this way, the improved entropy method is a favorable solution strategy; the specific calculation procedures can be found in the work of Zhao et al. (2021a). Accordingly, a composite index of public infrastructure for each country over the sample period is obtained. The related data are collected from the World Bank (2021).
3.2.3 Mediation variables
To investigate whether trade openness and industrial upgrading are two effective channels through which public infrastructure affects greenhouse gas emissions, trade openness and industrial upgrading are adopted as mediation variables:
Trade openness (denoted as Tra). This indicator is measured by the ratio of total import and export trade to gross domestic product (GDP).
Industrial upgrading (denoted as Ind). This indicator is gauged by the tertiary sector divided by the output value of the secondary industry. Sample data related to trade openness and industrial upgrading are collected from World Bank (2021).
3.2.4 Control variables
In addition to the variables discussed above, a number of control variables are introduced in this study to improve the accuracy of estimation results, which include economic growth, foreign investment, industrial evolution, population density, and labor force. Data on these control variables are obtained from World Bank (2021). The definitions and descriptive statistics of these variables are presented in Table 1.
3.3 Correlation analysis
Before conducting the benchmark regression on the public infrastructure-CO2 nexus, this study first calculates the correlation coefficients between variables, which are shown in Table 2. In this table, it is clear that both public infrastructure and CO2 emissions show a significant positive correlation. This can be also clearly shown in Figure 1. In addition, other control variables, apart from economic growth, are also positively correlated with CO2 emissions. It is worth noting that the correlation test serves merely as a preliminary analysis of the nexus between the variables, and thus, it is necessary to select a more complex model.
4 Empirical findings
4.1 Benchmark results
Table 3 reports the regression results for both ordinary least squares (OLS) and fixed effect (FE) techniques. Among them, Models (1) and (3) do not include control variables and Models (2) and (3) add control variables. In all four models, the estimated coefficients for infrastructure (i.e., lnInfra) are significantly positive, with or without control variables, which verifies the robustness of findings. Compared with OLS estimates, the FE model effectively excludes the potential role of country and time effects on the infrastructure-CO2 nexus, and as a result, drastically improves the accuracy of the empirical regression; therefore, the FE estimation with control variables is adopted as the benchmark regression results (as indicated in the last column of Table 3). Notably, the statistics of the variance inflation factor (VIF) in Table 2 highlight the absence of multicollinearity between the variables.
Possible reasons for the positive infrastructure-CO2 nexus are that, on the one hand, the Global Energy Infrastructure Carbon Emissions and Lock-in Effect 2021 Research Report shows that thermal power, steel, cement, and land transportation are all fundamental industries that sustain global socio-economic development. In addition to the global cement industry capacity, which has been largely stable since 2015, the global thermal power and steel industries have maintained growth in recent years, and the vehicle ownership has risen sharply. However, significant increase of new infrastructure puts pressure and challenges on the future low-carbon transition (Jiang et al., 2020). Specifically, CO2 emissions from the global thermal power industry increased from 7.5 billion tons in 1990 to 13.2 billion tons in 2020, with an average annual growth rate of 2%. Emissions from the global steel industry totaled 2.72 billion tons in 2020, an increase of about 1.5 times over the past 30 years. Global carbon emissions from the cement sector increased 1.9 ties from 1990 to 2020, reaching 2.52 billion tons in 2020. Driven by the continuous growth of vehicle ownership, global carbon emissions have been rising year by year (Jiang et al., 2022; Zhao et al., 2022). CO2 emissions have increased by 75% in the last 30 years, reaching 5.7 billion tons in 2020. On the other hand, in addition to energy and transport-related infrastructure, the construction of technology and propagation infrastructure is also based on the consumption of large amounts of fossil energy, which will generate large amounts of greenhouse gases. In general, although the construction of public infrastructure has effectively filled up the shortcomings in the process of economic development at the current stage, it still faces enormous challenges on the road to low carbon emissions reduction.
To alleviate the endogeneity problem posed by omitted variables, five indicators, namely economic growth, foreign investment, industrial evolution, population density, and labor force, are added as control variables. In Table 3, the coefficients of the first and square terms of economic growth are significantly positive and negative, respectively, which emphasizes that the inverse U-shaped EKC hypothesis is satisfied between economic growth and CO2 emissions. This is in line with the findings of Gao et al. (2021) and Zhao et al. (2020). In addition, the coefficient of foreign investment is significantly negative, which supports the effective contribution of foreign investment to accelerating the process of carbon neutrality. The estimated coefficients for labor force and population density are significantly positive, and as a result, considerably contribute to the greenhouse effect. Therefore, in the process of ensuring economic growth, relevant government departments should actively optimize the investment structure to help achieve the carbon neutrality target.
4.2 Robustness checks
4.2.1 Alternative measures of explained variable
To empirically check the robustness of the positive infrastructure-CO2 nexus, Eq. 2 is re-estimated based on the OLS and FE strategies with per capita CO2 emissions (denoted as PCO2) instead of total CO2. Table 4 presents the corresponding regression results. It is clear that after replacing the explained variable, the estimated coefficients of public infrastructure are all significantly positive. This implies that the intensive construction of relevant public infrastructure will aggravate greenhouse effect, which is consistent with the conclusions of benchmark regression.
4.2.2 Alternative regression strategies
In this section, robustness tests are conducted by using the alternative regression strategies, namely feasible generalized least squares (FGLS) and instrumental variable (IV) methods constructed under the condition of the heteroscedasticity proposed by Lewbel (2012); the corresponding results are listed in Table 5. In the former technique, the null hypothesis of inter-group heteroscedasticity test (i.e., Wald test), the intra-group autocorrelation test (i.e., Wooldridge test) and the cross-sectional dependence test (i.e., BP-LM test) are significantly rejected at the 1% level. The empirical results indicate that the coefficients of public infrastructure (i.e., lnInfra) are all significant in Models (1)–(4), which validates the benchmark findings.
4.2.3 Removing the distractions of the 2008 financial crisis
The financial crisis that erupted in 2008 has an immeasurable impact on all sectors around the world, including downturns in the economy, decline in consumption, and huge shocks on infrastructure construction (Frankel and Saravelos, 2012). To this end, this study excludes the relevant data samples from 2008 to 2009 on the basis of the benchmark regression for robustness test. The first two columns of Table 6 report the corresponding results. It is evident that the findings the empirical study on the positive infrastructure-CO2 nexus are robust and reliable.
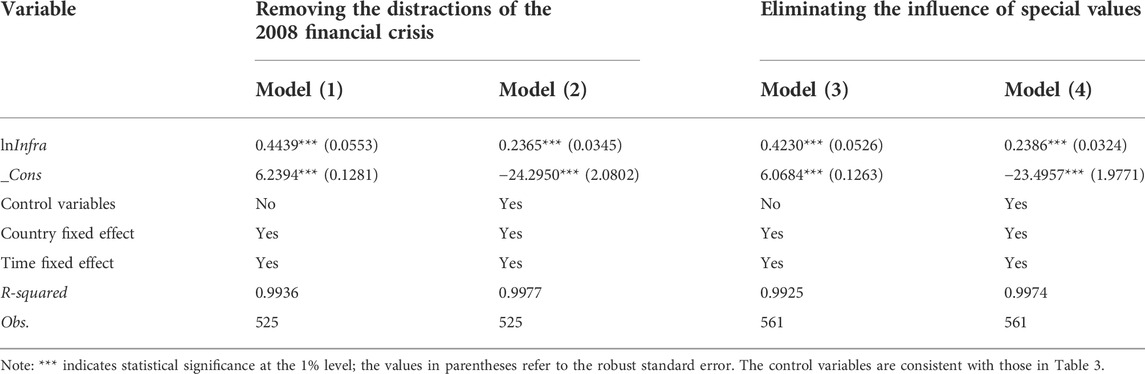
TABLE 6. Robust results of removing the distractions of the 2008 financial crisis and eliminating the influence of special values.
4.2.4 Eliminating the influence of special values
In addition to excluding the distractions of the 2008 financial crisis, the impact of special values, like the ones from the US and Japan, is further excluded from this section (Yang and Greaney, 2017). These two countries have huge gaps with other countries in terms of economic size, technological level, and infrastructure. By applying the FE technique, the last two columns of Table 6 present the corresponding results. Clearly, the positive coefficients of public infrastructure imply robustness of the benchmark findings.
4.3 Addressing the endogeneity issues
As highlighted by Ullah et al. (2018) and Yu (2013), if the regression estimation suffers from endogeneity problems caused by reverse causality or omitted variables, the estimated results will be biased. In such case, it is necessary to select appropriate instrumental variables to improve the reliability of the estimation findings. To this end, two strategies, namely, using the lag term of explanatory variables and employing the number of patent applications as instrumental variables, are applied to address endogeneity problems in the estimation model; the corresponding results are presented in Table 7, where control variables are excluded from Models (1) and (3), while control variables are added in Models (2) and (4).
In the last two columns of this table [i.e., Models (3) and (4)], the statistical values of K-P rk LM are significant at the 1% level, which rejects the null hypothesis of unidentifiable instrumental variables. The statistical values of K-P rk Wald F are significantly larger than the critical value of maximum instrumental variable (i.e., 16.38) of the Stock-Yogo test at the 10% level, which rejects the weak instrumental variable hypothesis (Kleibergen and Paap, 2006). This suggests our choice of instrumental variables is reasonable and appropriate.
From the empirical results, it can be observed that the estimated coefficients of L.lnInfra in Models (1) and (2) and lnInfra in Models (3) and (4) are significantly positive. This finding indicates that the impact of public infrastructure on CO2 emissions remains positive after endogeneity has been properly addressed. In other words, intensive construction of the public infrastructure is not an effective strategy to alleviate greenhouse effect.
4.4 Heterogeneous analysis
Studying the differential impact of public infrastructure on CO2 emissions is the concern of this study due to the distinct differences between countries in various dimensions such as facility construction, resource endowment, and greenhouse gas emissions (Zhao et al., 2021b). As a top-level national cooperation initiative, the Belt and Road Initiative depends on the existing bilateral and multilateral mechanisms between China and relevant countries, as well as effective regional cooperation platforms, to actively attract countries around the world to jointly build a community of shared interests featuring economic integration, cultural inclusiveness, and political mutual trust (Wang et al., 2021). Accordingly, the full sample is divided into two sub-samples (i.e., BRI countries and non-BRI countries) for heterogeneous analysis; the specific countries of these two sub-samples are listed in Supplementary Table A2.
By applying the FE technique, the empirical results of the heterogeneous analysis are shown in Table 8. In this table, the coefficients of public infrastructure (i.e., lnInfra) are significantly positive for BRI or non-BRI countries, which confirm that the construction of infrastructure contributes to the greenhouse effect in both BRI and non-BRI countries. However, notably, the coefficient values of public infrastructure in BRI countries are significantly larger than those in non-BRI countries; in other words, the contribution of infrastructure construction to the greenhouse effect in BRI countries is significantly larger than that in non-BRI countries. The likely reason for this is that most BRI countries have weak economies and poor infrastructure.
4.5 Mediation analysis
In addition to discussing the potential effects and heterogeneous impact of public infrastructure on greenhouse gas emissions, in this study, an effort is made to explore whether trade openness and industrial upgrading are effective channels through which public infrastructure affects CO2 emissions. In this regard, the mediation effect model is adopted and the corresponding results are listed in Table 9, where Model (1) aims to test the total effect of public infrastructure on CO2 emissions. Clearly, the coefficient of public infrastructure (i.e., lnInfra) is 0.2748 and significant at the 1% level. This implies that the construction of the fundamental infrastructure will aggravate greenhouse effect. Furthermore, the regression coefficients of public infrastructure (i.e., lnInfra) in Models (3) and (5) are significantly positive, indicating that the direct effect of public infrastructure on greenhouse effect holds true.
Furthermore, the statistics from Sobel test significantly reject the null hypothesis that trade openness and industrial upgrading are not mediation transmission pathways. The validity of the mediating effect is also verified by the fact that the confidence intervals of the statistics are excluded from zero in the Bootstrap test. To be more specific, the results of Models (2) and (3) are adopted to test whether trade openness holds as a mediating channel. The regression coefficients of public infrastructure in Model (2) and trade openness in Model (3) are both significantly positive, indicating the existence of the mediation role of trade openness; in other words, a large expansion of public infrastructure will result in significant greenhouse gases through facilitating trade openness between countries. In addition, the mediating role of industrial upgrading is examined in the results of Models (4) and (5). It is worth noting that the estimated coefficients of public infrastructure in Model (4) and industrial upgrading in Model (5) are positive and negative, respectively, suggesting that the mediating effect of industrial upgrading in affecting the infrastructure-CO2 nexus holds true. In other words, the construction of public infrastructure can reduce greenhouse gas emissions through improving industrial transition and upgrading. In conclusion, expanded public infrastructure not only directly contributes to greenhouse gas emissions, but also increases CO2 emissions through trade openness and reduce CO2 emissions through accelerated industrial upgrading and transformation. The specific transmission mechanism is presented in Figure 2.
5 Conclusion and policy implications
In this study, the entropy method is adopted to calculate the composite index of public infrastructure in 35 countries around the world, using statistics from 2003 to 2019 as a sample, and to explore the potential greenhouse effect of public infrastructure construction. In addition, the heterogeneity of BRI and non-BRI countries as well as the mediating roles of trade openness and industrial upgrading have been examined in depth. The primary findings suggest that:
1) The empirical results of the benchmark regression indicate that the coefficient of public infrastructure is 0.2160, which supports the positive relationship between public infrastructure and greenhouse gas emissions; in other words, increased construction of public infrastructure will aggravate CO2 emissions at the present stage. This conclusion is verified by a number of robustness tests.
2) The heterogeneous analysis of BRI and non-BRI countries implies that public infrastructure is positively associated with CO2 emissions in both BRI and non-BRI countries. The positive effect of public infrastructure on CO2 emissions in BRI countries is larger than that in non-BRI countries.
3) The empirical findings of mediation analysis insist that when the construction of public infrastructure directly contributes to the greenhouse effect, it will also increase CO2 emissions by accelerating frequent trade and reduce greenhouse gas emissions by enhancing the upgrading of industrial structures.
As a result of these three findings, the following policy implications are further proposed. First, due to the positive infrastructure-CO2 emissions nexus, it is imperative to strengthen the low-carbon and green construction of the public infrastructure. As it can not only help to set the stage for economic development, but also contribute to carbon neutrality. The construction of related infrastructure consumes large amount of resources, and the application of materials with high carbon content will aggravate CO2 emissions. Therefore, to reduce carbon emissions during the construction of relevant infrastructure, governments should formulate facility green access standards for infrastructure according to the spatial distribution characteristics of resources and environment, specify green requirements such as access to infrastructure site selection and regulation, form a green infrastructure evaluation system, and accelerate the promotion of new urbanization and ecological restoration.
Second, the empirical results of heterogeneity analysis show that infrastructure construction has a differential effect on carbon emissions in BRI and non-BRI countries. Therefore, it is crucial to reduce the carbon emission effect of infrastructure in countries along the Belt and Road. To this end, it is essential to promote overseas investment in line with the concepts of low-carbon and sustainable development, tap the potential of green governance and expand the fields of green cooperation.
Third, the mediating effect results indicate that industrial upgrading is an effective channel for public infrastructure to reduce CO2 emissions. In other words, accelerating industrial upgrading is an effective impetus and driving force for carbon emission reduction in the field of infrastructure construction. On the one hand, implementing the active and efficient industrial policies can help promote industrial upgrading. Local governments of all countries should introduce corresponding policies and regulations to provide effective macro support for industrial transformation. On the other hand, as a powerful weapon for industrial transformation and upgrading, technological upgrading can provide technical support for industrial transformation. Together, macro policies and advanced technologies have become an effective guarantee for promoting industrial structure upgrading.
Data availability statement
The original contributions presented in the study are included in the article/Supplementary Material, further inquiries can be directed to the corresponding author.
Author contributions
YQ: Data curation, Software, and Writing—Original Draft; RS: Conceptualization, Methodology, and Funding acquisition; JZ: Methodology, and Writing—Review and Editing; SS: Writing—Review and Editing, and Supervision; PW: Validation, Methodology, and Writing—Review and Editing.
Acknowledgments
The article is sponsored by the National Social Science Foundation of China (Grant No. 20VGQ003). The authors gratefully acknowledge the helpful reviews and comments from the editors and reviewers, which improved this manuscript considerably. Certainly, all remaining errors are our own.
Conflict of interest
PW was employed by the company Beijing Xuanyu Intelligent Technology Co., LTD.
The remaining authors declare that the research was conducted in the absence of any commercial or financial relationships that could be construed as a potential conflict of interest.
Publisher’s note
All claims expressed in this article are solely those of the authors and do not necessarily represent those of their affiliated organizations, or those of the publisher, the editors and the reviewers. Any product that may be evaluated in this article, or claim that may be made by its manufacturer, is not guaranteed or endorsed by the publisher.
Supplementary material
The Supplementary Material for this article can be found online at: https://www.frontiersin.org/articles/10.3389/fenvs.2022.1048297/full#supplementary-material
References
Bakhtiari, F. (2018). International cooperative initiatives and the United Nations framework convention on climate change. Clim. Policy 18, 655–663. doi:10.1080/14693062.2017.1321522
Banerjee, A., Duflo, E., and Qian, N. (2020). On the road: Access to transportation infrastructure and economic growth in China. J. Dev. Econ. 145, 102442. doi:10.1016/j.jdeveco.2020.102442
BP (2020). BP statistical review of world energy 2020. Available at: http://www.bp.com/en/global/corporate/energy-economics/statistical-review-of-world-energy/downloads.html.
Churchill, S. A., Inekwe, J., Ivanovski, K., and Smyth, R. (2021). Transport infrastructure and CO2 emissions in the OECD over the long run. Transp. Res. Part D Transp. Environ. 95, 102857. doi:10.1016/j.trd.2021.102857
Cui, Y., and Sun, Y. (2019). Social benefit of urban infrastructure: An empirical analysis of four Chinese autonomous municipalities. Util. Policy 58, 16–26. doi:10.1016/j.jup.2019.03.001
Dong, K., Dong, X., Jiang, Q., and Zhao, J. (2021). Assessing energy resilience and its greenhouse effect: A global perspective. Energy Econ. 104, 105659. doi:10.1016/j.eneco.2021.105659
Dong, K., Dong, X., and Ren, X. (2020). Can expanding natural gas infrastructure mitigate CO2 emissions? Analysis of heterogeneous and mediation effects for China. Energy Econ. 90, 104830. doi:10.1016/j.eneco.2020.104830
Dou, Y., Zhao, J., and Dong, J. (2021). Re-Estimating the impact of natural gas on global carbon emissions: The role of technological innovation. Front. Energy Res. 9, 651586. doi:10.3389/fenrg.2021.651586
Frankel, J., and Saravelos, G. (2012). Can leading indicators assess country vulnerability? Evidence from the 2008–09 global financial crisis. J. Int. Econ. 87, 216–231. doi:10.1016/j.jinteco.2011.12.009
Gao, J., Xu, W., and Zhang, L. (2021). Tourism, economic growth, and tourism-induced EKC hypothesis: Evidence from the mediterranean region. Empir. Econ. 60, 1507–1529. doi:10.1007/s00181-019-01787-1
Hao, Y., and Cho, H. C. (2022). Research on the relationship between urban public infrastructure, CO2 emission and economic growth in China. Environ. Dev. Sustain. 24, 7361–7376. doi:10.1007/s10668-021-01750-0
Hickmann, T., Widerberg, O., Lederer, M., and Pattberg, P. (2021). The united Nations framework convention on climate change secretariat as an orchestrator in global climate policymaking. Int. Rev. Adm. Sci. 87, 21–38. doi:10.1177/0020852319840425
Huang, G., Zhang, J., Yu, J., and Shi, X. (2020). Impact of transportation infrastructure on industrial pollution in Chinese cities: A spatial econometric analysis. Energy Econ. 92, 104973. doi:10.1016/j.eneco.2020.104973
Jafri, M. A. H., Liu, H., Majeed, M. T., Ahmad, W., Ullah, S., and Xue, R. (2021). Physical infrastructure, energy consumption, economic growth, and environmental pollution in Pakistan: An asymmetry analysis. Environ. Sci. Pollut. Res. 28, 16129–16139. doi:10.1007/s11356-020-11787-9
Jiang, H. D., Hao, W. T., Xu, Q. Y., and Liang, Q. M. (2020). Socio-economic and environmental impacts of the iron ore resource tax reform in China: A CGE-based analysis. Resour. Policy 68, 101775. doi:10.1016/j.resourpol.2020.101775
Jiang, H. D., Liu, L. J., Dong, K., and Fu, Y. W. (2022). How will sectoral coverage in the carbon trading system affect the total oil consumption in China? A CGE-based analysis. Energy Econ. 110, 105996. doi:10.1016/j.eneco.2022.105996
Jiang, X., and Guan, D. (2016). Determinants of global CO2 emissions growth. Appl. Energy 184, 1132–1141. doi:10.1016/j.apenergy.2016.06.142
Karjunen, H., Tynjälä, T., and Hyppänen, T. (2017). A method for assessing infrastructure for CO2 utilization: A case study of Finland. Appl. Energy 205, 33–43. doi:10.1016/j.apenergy.2017.07.111
Kleibergen, F., and Paap, R. (2006). Generalized reduced rank tests using the singular value decomposition. J. Econom. 133, 97–126. doi:10.1016/j.jeconom.2005.02.011
Lewbel, A. (2012). Using heteroscedasticity to identify and estimate mismeasured and endogenous regressor models. J. Bus. Econ. Stat. 30, 67–80. doi:10.1080/07350015.2012.643126
Liu, W., Holst, J., and Yu, Z. (2014). Thresholds of landscape change: A new tool to manage green infrastructure and social–economic development. Landsc. Ecol. 29, 729–743. doi:10.1007/s10980-014-0007-1
Luo, Z., Wan, G., Wang, C., and Zhang, X. (2018). Urban pollution and road infrastructure: A case study of China. China Econ. Rev. 49, 171–183. doi:10.1016/j.chieco.2017.04.008
Ozcan, B., Tzeremes, P. G., and Tzeremes, N. G. (2020). Energy consumption, economic growth and environmental degradation in OECD countries. Econ. Model. 84, 203–213. doi:10.1016/j.econmod.2019.04.010
Parker, J., and Zingoni de Baro, M. E. (2019). Green infrastructure in the urban environment: A systematic quantitative review. Sustainability 11, 3182. doi:10.3390/su11113182
Rahman, M. M. (2020). Environmental degradation: The role of electricity consumption, economic growth and globalisation. J. Environ. Manage. 253, 109742. doi:10.1016/j.jenvman.2019.109742
Saboori, B., and Sulaiman, J. (2013). Environmental degradation, economic growth and energy consumption: Evidence of the environmental Kuznets curve in Malaysia. Energy Policy 60, 892–905. doi:10.1016/j.enpol.2013.05.099
Schäffler, A., and Swilling, M. (2013). Valuing green infrastructure in an urban environment under pressure—the johannesburg case. Ecol. Econ. 86, 246–257. doi:10.1016/j.ecolecon.2012.05.008
Sun, Y., and Cui, Y. (2018). Evaluating the coordinated development of economic, social and environmental benefits of urban public transportation infrastructure: Case study of four Chinese autonomous municipalities. Transp. Policy 66, 116–126. doi:10.1016/j.tranpol.2018.02.006
Toader, E., Firtescu, B. N., Roman, A., and Anton, S. G. (2018). Impact of information and communication technology infrastructure on economic growth: An empirical assessment for the EU countries. Sustainability 10, 3750. doi:10.3390/su10103750
Ullah, S., Akhtar, P., and Zaefarian, G. (2018). Dealing with endogeneity bias: The generalized method of moments (GMM) for panel data. Ind. Mark. Manag. 71, 69–78. doi:10.1016/j.indmarman.2017.11.010
Wang, C., Miao, Z., Chen, X., and Cheng, Y. (2021). Factors affecting changes of greenhouse gas emissions in Belt and Road countries. Renew. Sustain. Energy Rev. 147, 111220. doi:10.1016/j.rser.2021.111220
Wei, L., and Ullah, S. (2022). International tourism, digital infrastructure, and CO2 emissions: Fresh evidence from panel quantile regression approach. Environ. Sci. Pollut. Res. 29, 36273–36280. doi:10.1007/s11356-021-18138-2
World Bank (2021). World development indicators. Available at: https://databank.worldbank.org/source/world-development-indicators/preview/on.
Xie, R., Fang, J., and Liu, C. (2016). Impact and spatial spillover effect of transport infrastructure on urban environment. Energy Procedia 104, 227–232. doi:10.1016/j.egypro.2016.12.039
Xu, H., Cao, S., and Xu, X. (2022). The development of highway infrastructure and CO2 emissions: The mediating role of agglomeration. J. Clean. Prod. 337, 130501. doi:10.1016/j.jclepro.2022.130501
Yang, Y., and Greaney, T. M. (2017). Economic growth and income inequality in the asia-pacific region: A comparative study of China, Japan, South Korea, and the United States. J. Asian Econ. 48, 6–22. doi:10.1016/j.asieco.2016.10.008
Yu, P. (2013). Inconsistency of 2SLS estimators in threshold regression with endogeneity. Econ. Lett. 120, 532–536. doi:10.1016/j.econlet.2013.06.023
Zhao, J., Jiang, Q., Dong, X., and Dong, K. (2021a). Assessing energy poverty and its effect on CO2 emissions: The case of China. Energy Econ. 97, 105191. doi:10.1016/j.eneco.2021.105191
Zhao, J., Jiang, Q., Dong, X., Dong, K., and Jiang, H. (2022). How does industrial structure adjustment reduce CO2 emissions? Spatial and mediation effects analysis for China. Energy Econ. 105, 105704. doi:10.1016/j.eneco.2021.105704
Zhao, J., Jiang, Q., Dong, X., and Dong, K. (2020). Would environmental regulation improve the greenhouse gas benefits of natural gas use? A Chinese case study. Energy Econ. 87, 104712. doi:10.1016/j.eneco.2020.104712
Keywords: infrastructure construction, CO2 emissions, BRI and non-BRI countries, mediation analysis, global analysis
Citation: Qian Y, Sun R, Zhao J, Song S and Wang P (2022) How does public infrastructure construction influence CO2 emissions? A global case. Front. Environ. Sci. 10:1048297. doi: 10.3389/fenvs.2022.1048297
Received: 19 September 2022; Accepted: 03 October 2022;
Published: 18 October 2022.
Edited by:
Hong-Dian Jiang, China University of Geosciences, ChinaReviewed by:
Zhang Yingying, University of International Business and Economics, ChinaLiu Guixian, Tsinghua University, China
Copyright © 2022 Qian, Sun, Zhao, Song and Wang. This is an open-access article distributed under the terms of the Creative Commons Attribution License (CC BY). The use, distribution or reproduction in other forums is permitted, provided the original author(s) and the copyright owner(s) are credited and that the original publication in this journal is cited, in accordance with accepted academic practice. No use, distribution or reproduction is permitted which does not comply with these terms.
*Correspondence: Jun Zhao, emhhb2p1bjQ3NjhAMTYzLmNvbQ==