- 1School of Statistics and Mathematics, Shandong University of Finance and Economics, Jinan, China
- 2School of Economics and Management, Tongji University, Shanghai, China
Climate change increases the frequency of extreme climate events and impacts the economy and the society in a negative way. As typical climate events, temperature anomalies affect individual health and working conditions, particularly for industries that depend heavily on temperatures. Using a research setting of Chinese temperature-sensitive enterprises, we analyze the impact of temperature on labor productivity. The findings indicate an inverse U-shaped relationship between temperature and labor productivity, with labor productivity peaking at 24.90°C on average. Further analysis shows that labor productivity peaks in the eastern regions at a higher level (26.25°C) than in the central and western regions (20°C). Moreover, we note that technological innovation is crucial for enterprises to manage climate risks and maintain effective labor productivity. This study provides empirical evidence on the relationship between environmental risk and corporate operations, shedding light on the significance of corporate sustainable development against accelerating global climate change.
1 Introduction
Climate change, which continues to have an effect on ecosystems and socioeconomic systems, is one of the largest problems facing the globe today (Selvi et al., 2019; He et al., 2021; Hong et al., 2021; Sebestyén et al., 2021; Yu et al., 2022; Zhang et al., 2022). Global warming, environmental pollution, deforestation, soil degradation, and prolonged droughts are just a few of the significant environmental problems that have frequently surfaced along with explosive economic growth during the past few decades. These extreme events endangered human survival and caused great economic losses (Marengo et al., 2021; Sohail et al., 2022). For example, Typhoon Hagibis in 2019 brought more than 240 mm of extreme rainfall in Japan, leading to around 100 deaths and adding $4 billion to Japan’s economic losses (Wagner, 2022). According to the Global Climate Risk Index 2020, Germany’s hot weather in 2018 caused 70% of the soil to be affected by drought, resulting in a total of EUR 3 billion (US $3.54 billion) in damage.
Some scholars believe that human activities have caused greenhouse gas emissions to increase annually, making climate change often associated with temperature anomalies (Sakhel, 2017; Kouloukoui et al., 2019; Uzar, 2020; Chen et al., 2022; Shi et al., 2022). A large body of research has empirically shown that temperature anomalies can have a wide range of negative impacts, including harm to people’s physical and mental health (Deschenes, 2014; Carleton and Hsiang, 2016; Zhang et al., 2018; Fishman et al., 2019). The body temperature increases and physiological functions such as water and salt metabolism, circulation, and respiration would be disrupted in an environment with extremely high temperatures (Venugopal et al., 2020). High temperatures affect productivity by reducing human body load endurance and work efficiency while increasing operation errors and accident rates (Li et al., 2009; Somanathan et al., 2021). In an environment with extremely low temperatures, human body temperature will drop, triggering a series of protective or compensatory physiological responses, such as increased metabolic rate and accelerated heart rate and respiratory rate (McInnes et al., 2017). Long-term exposure to low temperatures can lower tactile discrimination and significantly reduce limb operating flexibility, thus reducing labor productivity (Varghese et al., 2019). In conclusion, temperature anomalies would interfere with the body’s thermal equilibrium and lower labor productivity.
According to the endogenous growth theory (Romer, 1990), labor input determines the level of industrial development since workforce productivity reflects the organization’s output capacity. Based on this, the economic concept of labor productivity-the ratio of total product output to labor input-is adopted (Hanna et al., 2005). Therefore, depending on how labor productivity is calculated, both high and low temperatures negatively impact the level of labor input and labor productivity. But Matsumoto (2019) demonstrated that labor productivity in medium-temperature zones is higher. As confirmed by Burke et al. (2015), economic productivity is non-linear in temperature. So, because people prefer moderate temperatures to extreme temperatures, there might be a non-linear link between temperature and labor productivity.
Understanding how temperature affects industrial sector, and in particular how it affects labor productivity, has become an issue of economic significance. In this paper, we examine the impact of temperature on labor productivity using construction enterprises as the research setting, as building construction tasks often take place outdoors, making labor productivity more susceptible to temperature anomalies (Blaauw et al., 2022; Feller and Vaseva, 2014; Yu et al., 2021). According to Tan and Abdul-Samad (2022), extreme temperature events would decrease labor force and hinder construction projects. The workforce reduction will hinder progress, shut down operations, reduce work efficiency, and decrease labor productivity (Gasparrini et al., 2015; Morabito et al., 2017; Dosio et al., 2018; Watts et al., 2019). So, temperature anomalies can make the construction industry more vulnerable to adverse circumstances.
We choose construction enterprises in 31 provinces in mainland China as the research setting for two reasons. On one hand, the geographic locations of provinces in mainland China span from 73°40′ east longitude of Xinjiang Uygur Autonomous Region to 135°2′30″ east longitude of Heilongjiang province, and from 3°52′ north latitude of Hainan province to 53°33′ north latitude of Heilongjiang province. So, the country has a broad temperature range, which satisfies our criteria for the variation of temperature data. On the other hand, the development of building procedures and mechanical equipment over the past few decades has greatly increased the overall productivity of the Chinese construction sector (Tian et al., 2020; Xue et al., 2022), which is still highly labor-intensive. Construction workers work outdoors and are more sensitive to temperature changes. So, we analyze the effect of temperature on labor productivity by using the data of construction enterprises from 2006 to 2019 in 31 provinces of mainland China. The results show an inverse U-shaped relationship between labor productivity and temperature. The labor productivity of construction enterprises is highest at an average temperature of 24.9°C and will decrease when temperatures are too high or too low Then, the optimum temperature for labor productivity is higher in Eastern China (26.25°C) than in Central and Western China (20°C). In addition, we conduct a test for heterogeneity in technological innovation level and find that it significantly and positively affects the relationship between temperature and labor productivity.
The primary contributions of this paper are manifested in the following two aspects. On one hand, we demonstrate an inverse U-shaped relationship between temperature and labor productivity by using a sample with a wide range of temperature spans. Scholars have conducted numerous studies on how temperature affects construction labor productivity, but the majority of them focused on the effect of high temperatures on the human body (Chinnadurai et al., 2016; Li et al., 2016). In the absence of research on the effect of low temperatures, those findings could hardly reflect the changes in construction labor productivity caused by temperature differences. On the other hand, unlike studies where temperature and labor productivity data were obtained from a small number of construction contractors (Mohamed and Srinavin, 2005; Mathee et al., 2010; Nasirzadeh and Nojedehi, 2013; Blaauw et al., 2022), we extend our study from the site level to the construction company level. This makes the findings generalizable and convincing. Therefore, this research could help businesses develop better strategies to deal with temperature fluctuations and increase labor productivity, which will improve their competitiveness and maintain sustainable growth.
In the remainder of this paper, section 2 is the literature review on the impact of temperature on labor productivity in the construction enterprise. Section 3 introduces the methodology, including data collection and the spatial econometric model. Section 4 presents the descriptive statistical analysis and the regression results. The analysis includes baseline regression analysis, heterogeneous regression analysis in terms of geographical effects, and robustness checks. Section 5 discusses the findings and future research direction. Section 6 concludes this article.
2 Literature review
Climate change has been hastened by rapid industrialization and urbanization (Tao et al., 2022). It has raised the risk for companies operating throughout the world. (Liu et al., 2021; Wan et al., 2021; Tian et al., 2022; Wagner, 2022). For example, extreme weather events associated with flooding have a significant impact on the operation of insurance enterprises (Cremades et al., 2018), and severe drought raises a company’s cost of equity capital (Huynh et al., 2020). Understanding the impact of temperature changes on enterprises is crucial because it is predicted that global temperatures will continue to change drastically (Stern and Kaufmann, 2014; Malhi et al., 2021). Especially for enterprises whose core operations depend on natural conditions, temperature changes can have a detrimental effect on an enterprise’s financial performance, such as loss of physical assets and increased labor costs (Sun et al., 2020).
The construction industry is vulnerable to climate change risks because of its dependence on temperature and seasonal conditions. Most construction work is conducted outdoors and is labor-intensive, so workers’ health and productivity are directly affected by temperature changes (Grifoni et al., 2021). Kim and Hong’s (2020) thermal evaluation questionnaire results indicated that humans function most efficiently and comfortably at 25°C. But extreme temperatures affect workers’ health and working conditions, which then lowers labour productivity in the construction industry. These effects include high-temperature and low-temperature effects.
Based on the relationship between environmental temperature and human thermal balance, Zhu and Zhao (2006) defined high-temperature environments as either a residential environment above 35°C or a working environment above 32°C. The human body responds to thermal stress in a high-temperature environment by releasing heat to keep itself in balance. However, agricultural and construction workers frequently wear personal protective gear and clothes, especially when engaging in outside tasks. This can dramatically reduce body heat loss and increase heat stress (Grifoni et al., 2021). When air temperature approaches or exceeds skin temperature (31°C-32°C), it is almost ineffective for the human body to dissipate heat through natural air convection; instead, sweat evaporation takes over as the primary method of heat dissipation (Hargreaves, 2008). If water loss from sweat evaporation is not replenished in time, it might cause dehydration, heatstroke, heat cramps, cardiovascular disease, or even death (Venugopal et al., 2020). In addition, uncomfortable outdoor working circumstances in hot weather may affect construction workers’ willingness and capacity to work (Somanathan et al., 2021). Li et al. (2016) examined the relationship between working hours and temperature using field data from a reinforced concrete construction project in Beijing, China. The findings showed that the percentage of real working hours drops by 0.57 percent on average for every 1°C increase in temperature. Reduced nerve and muscle excitability in workers, as well as irritability and anxiety, could increase accident rates and decrease labor productivity (Li et al., 2009).
Recently, Adhvaryu et al. (2020) utilized energy-efficient LED lighting to create temperature fluctuations in the workplace and concluded that worker productivity would increase when the temperature decreases moderately. It’s noteworthy that extremely low temperatures are more harmful to health than high temperatures (Gasparrini et al., 2015; King et al., 2020). Low-temperature environment increases energy consumption, decreases tactile sensitivity, and damages the cardiovascular and cerebrovascular vessels (McInnes et al., 2017). Research by Varghese et al. (2019) has shown that low temperatures are mostly to blame for the nearly 5% of workplace accidents in Australia that are caused by ambient temperature. Especially, the outdoor work of construction enterprises is closely related to the natural environment and climatic conditions. Therefore, the reduction of ambient temperature usually directly affects the occupational sector in a negative way (Moda et al., 2019). Additionally, at low temperatures, equipment performance degrades more rapidly (Fiorese et al., 2015). All of these could reduce labor productivity and safety in the construction enterprise.
Many scholars have analyzed the impact of temperature on construction workers, in order to find the ideal temperature for boosting labor productivity. The majority of these studies focused on the study of the effects of high temperatures. For example, Li et al. (2016) measured and compared rebar workers’ performance between 2:00 pm and 3:00 pm and 7:00 pm to 9:00 pm in the summer (representing high ambient temperatures). By analyzing data from four construction sites, Mohamed and Srinavin (2005) confirmed the negative impact of high temperatures on construction labor productivity. Similarly, Chinnadurai et al. (2016) noted that working outdoors performing light and heavy construction tasks in high ambient temperatures above 28°C resulted in an 18%–35% reduction in overall worker productivity. At the same time, we find that many studies take a city or even a construction site as the research setting. For example, Mathee et al. (2010) found that sun-exposed workers in Johannesburg and Upington experience work-related discomfort and ill health in hot weather, and Nasirzadeh and Nojedehi (2013) used on-site data from a real housing construction project to develop a quantitative model of labor productivity.
Overall, researchers show that temperature conditions impose substantial economic and social burdens on enterprises. However, research on the quantitative effects of temperature remains limited and flawed. The problem with the aforementioned studies is that they only consider the high-temperature data while ignoring the low-temperature data, which can lead to biased results. Also, since the data were primarily field data for a specific construction project or city, there may have been representative errors in the choice of construction contractors, possibly leading to big measuring errors in labor productivity data. Therefore, this paper attempts to contribute by examining the effects of both high and low temperatures on labor productivity in the construction sector. To ensure the generalizability of the results for the construction industry, we select a temperature dataset with a large temperature span and collect enterprise labor productivity data from the provincial level.
3 Methodology
3.1 Data collection
We take atmospheric temperature as the key explanatory variable. Li et al. (2016) used the QUESTemp°36 thermal environment monitor to obtain on-site air temperature data. But this method of gathering field data only records the high-temperature environment for a set amount of time. By collecting data on average temperature across provinces, which includes both low and high temperatures, we could measure the effect of a wider range of temperatures. Besides, we take construction labor productivity as the dependent variable. According to the International Labour Organization, labor productivity is the gross domestic product (or value-added) per worker (Kpognon et al., 2022). This economic concept is also used to define construction labor productivity. In this paper, we use the construction labor productivity data from the National Bureau of Statistics of China (NBS), which is calculated as the ratio of the total output value of construction enterprises to the number of laborers in enterprises. Labor productivity changes at the micro level of firm and project activities make up the overall construction industry productivity (Abdel-Wahab and Vogl, 2011). Construction labor productivity data reflects the average labor productivity level of construction enterprises in a province, which can enhance the generalizability of the model’s findings.
Economic situations and weather conditions are two driving factors that could affect enterprise labor productivity (Durdyev et al., 2018; Avotra et al., 2021; Somanathan et al., 2021; Xiao and You, 2021). Therefore, we added seven economic and weather attributes, including regional gross domestic product (GDP), total output value of the construction industry, number of construction practitioners, relative humidity, wind speed, rainfall, and Sun exposure, as control variables. The sample period is from 2006 to 2019, and the data frequency is quarterly. The data are sourced from the published data of the National Bureau of Statistics of China and the China Meteorological Data Centre. The definition and measurement of variables are shown in Table 1. To ensure that the data follow a normal distribution, we took the logarithm of regional GDP and sunshine hours.
3.2 Model settings
Compared to traditional econometric models, the spatial econometric model takes into consideration the spatial dependences among neighboring regions (Anselin, 2002). The results generated by the spatial model are more precise when spatial factors play a role in the focal causal relationship. To verify the existence of spatial dependence between labor productivity and its influencing factors in the construction industry, we first conducted a spatial autocorrelation test on the residuals obtained from the ordinary least squares (OLS) regression. The results showed that the p-value of Moran’s I statistic is 0.000, rejecting the null hypothesis of spatial autocorrelation. The results indicated the existence of a spatial dependence; therefore, spatial econometric model is more suitable to implement the estimations. Following mainstream spatial econometrics literature (e.g., Anselin, 2002, 2003), we chose the binary geographical adjacent weight matrix with one representing neighboring provinces and 0 otherwise. The spatial weight matrix is defined as follows:
The spatial econometric model has two widely-used types: the spatial lag model (SLM) and the spatial error model (SEM). The model forms of SLM and SEM are:
where Y denotes the dependent variable; X represents the independent variables; W refers to the aforementioned spatial weight matrix;
4 Results
4.1 Descriptive statistics
Descriptive statistics of all variables are reported in Table 2. It indicates that the minimum quarterly Chinese construction labor productivity over the period 2006–2019 in the 31 provinces is 8,900 yuan/person, the maximum is 272,000 yuan/person, the mean value is 85,200 yuan/person, and the standard deviation is 38,400 yuan/person. And the minimum quarterly average temperature of the 31 provinces is −14.97°C, the maximum is 29.70°C, the mean value is 14.28°C, and the standard deviation is 9.84°C. The last column in Table 2 displays the coefficient of variation (Cov), which makes the variations of all variables comparable by eliminating scale difference. It is calculated by dividing standard deviation by mean. So compared to other variables, the total output value of the construction industry, the number of construction practitioners, and rainfall have larger variations in terms of the Cov. There are also large variations in average temperature and construction labor productivity. However, the variations of sunshine hours, regional GDP, relative humidity, and wind speed are relatively small.
As reported in Figure 1, the highest quarterly average temperature of the 31 provinces is in the third quarter over the period 2006–2019, followed by the second quarter. And the variations in these two-quarters are relatively small. The first quarter has the lowest average temperature and the largest variation. Construction labor productivity is also the lowest in the first quarter, but the variation is the smallest. Compared with the first quarter, the other three-quarters have higher labor productivity, but the variations are larger.
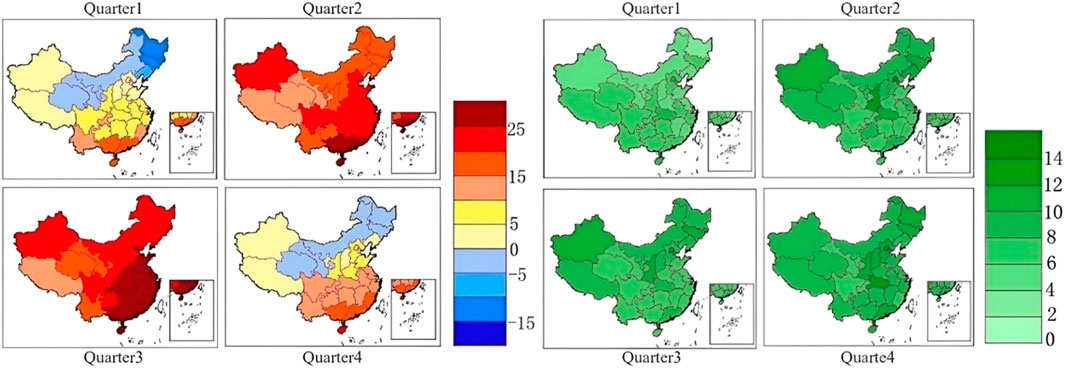
FIGURE 1. Heatmaps for average quarterly temperature (left) and construction labor productivity (right) in 31 provinces from 2006 to 2019.
4.2 Baseline regression results
We select the final regression model between SLM and SEM using results of the Lagrange Multiplier (LM) and Robust LM tests. The higher the test statistics, the better the model. The results in Table 3 indicate that SEM passed both Lagrange Multiplier and Robust LM tests (p-value <.001) and the test statistics of SEM are much greater than that of SLM. Meanwhile, according to untabulated Hausman test results and statistics from LogLikelihood Ratio, AIC, and SC, this paper chose SEM as the final model for empirical analyses.
Therefore, the spatial econometric model specification for examining the impact of temperature on construction labor productivity is:
where lpit refers to the construction labor productivity of province i in time t; atemit is the average temperature of province i in time t; tovit, lngdpit, numberit, humidityit, windit, rainfallit and lnsunshineit represent control variables introduced in Table 2, respectively. We also control for time-invariant attributes of the provinces (StateFE) and time fixed effect (TimeFE). The other parameters follow the same explanations of Eq. 2. As discussed in the theoretical analysis, labor productivity is likely to follow an inverse U-shaped relationship with temperature, i.e., the productivity raises when temperature increases from extremely cold to the optimum temperature where productivity reaches the highest and begins to decrease when temperature is higher than the optimum temperature and reaches the lowest when it is extremely hot. Therefore, we introduced its squared term (atemit2) in the regression model to test the inverse U-shaped relationship. We use Stata software to obtain the parameter estimates and the results are reported in Table 4.
The coefficients of atem and atem2 are 0.2191 (p-value < .001) and −.0044 (p-value < .001), indicating an inverse U-shaped relationship between temperature and construction labor productivity. The results also show that the spatial error correlation coefficient λ) is greater than 0 and highly significant (p-value < .001), indicating that other factors that are not included in the model have spatial spillover effects on construction labor productivity in neighboring regions. In addition, among the control variables, the total output value of the construction industry (tov) and regional GDP (lngdp) have significant and positive effects on construction labor productivity. The number of construction practitioners (number) has a significant but negative impact on construction labor productivity; that is, excessive employment in the construction industry reduces labor productivity.
As illustrated in Figure 1, there are notable temperature differences between Eastern China and Central and Western China, with the Eastern region generally having higher temperatures. Therefore, the impact of temperature on labor productivity may vary. Further, we investigate the variability of the inverse U-shaped temperature-productivity nexus in Eastern China and the rest of China (Central and Western China). The regression results for Eastern China and Central and Western China are reported in Table 4.
The results show that the inverse U-shaped relationship between temperature and construction labor productivity is observed in all parts of China. Specifically, in Eastern China, the coefficients of atem and atem2 are 0.2100 (p-value < .001) and −0.0040 (p-value < .001), respectively; while in the rest of China, the respective coefficients are 0.2080 (p-value < .001) and −0.0052 (p-value < .001). In addition, the spatial error correlation coefficient λ) of eastern provinces is not significant, but that of central and western provinces is positive (0.1660) and highly significant (p-value < .001), indicating a positive spillover effect from construction labor productivity of neighboring regions to the focal region of central and western China. In terms of control variables, the total output value of the construction industry, the number of employees in the construction industry and regional GDP all significantly impact labor productivity of the construction industry. And regional GDP in eastern regions has a much greater influence than that of central and western regions. Except for humidity, weather variables have no significant impact on construction labor productivity.
To calculate the optimum temperature that works best for labor productivity in construction industry, we take the derivative of the regression model with all control variables equal to mean values. The derivative calculation shows that when the average temperature is 24.90°C, construction labor productivity reaches the highest. Following the same derivative calculation process, we get the optimum temperature for construction labor productivity in Eastern China is 26.25°C, and 20°C in the rest of China. We thus draw the U-shaped curve between temperature and predicted construction labor productivity in Figure 2, where Figure (A) shows the inverted U-shaped curve for all regions, Figures (B) and (C) show the Eastern and Central and Western China, respectively.
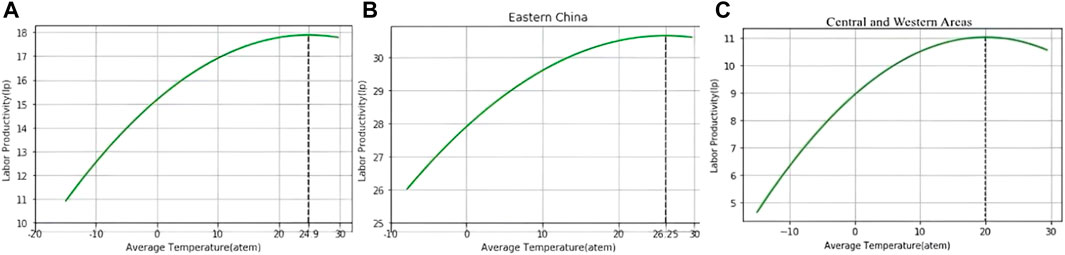
FIGURE 2. The relationship between estimated construction labor productivity and temperature of all areas (A), Eastern China (B), and Central and Western China (C).
4.3 Heterogeneity analysis of technological innovation level
Construction labor productivity relies heavily on the level of organizational technology (Ercan, 2019). Innovative technologies improve the working and living conditions of workers, which may have an important impact on the relationship between temperature and labor productivity. Therefore, we collect China’s regional innovation capability index (inno) as a proxy variable for the regional technological innovation level and test the significance of the level of technological innovation. The data are sourced from the China Regional Innovation Capability Evaluation Report, and the sample period is 2006–2019. After that, we also took logarithms of the data.
Referring to Li et al. (2008), we add the interaction term of technological innovation level (inno) with temperature (atem) and the square of temperature (atem2) to the first model in Table 4 to perform the heterogeneity analysis of regional technological innovation level. The results are reported in Table 5. The results show that the coefficient of the interaction term between atem2 and inno is 0.0022, which is significant at the 10% level. This indicates that the technological innovation level significantly affects the relationship between temperature and labor productivity. So, higher level of technological innovation leads to a flatter curve shape (Haans et al., 2016).
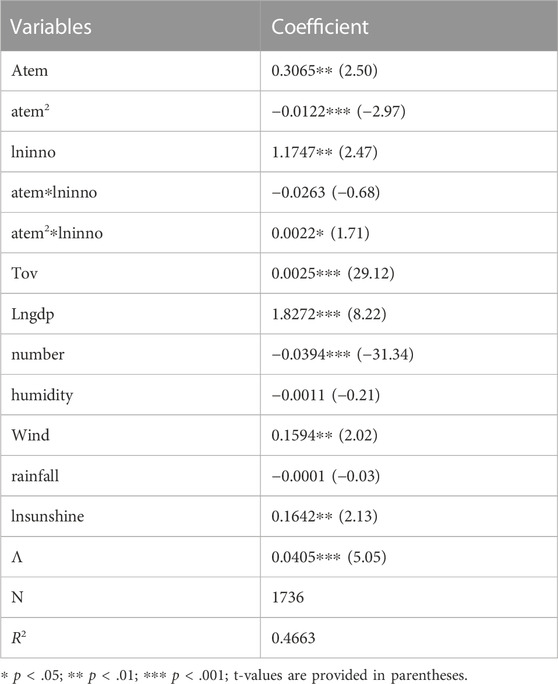
TABLE 5. Results for regression of technological innovation level, temperature and labor productivity.
4.4 Robustness checks
To check the robustness of our key findings, we use alternative measures of independent variable and add control variable to re-estimate the baseline regressions. Specifically, we use the quarterly lowest temperature (MINT) and highest temperature (MAXT), instead of average temperature, to re-examine the relationship between temperature and construction labor productivity. The results for robustness tests are displayed in Table 6. It is apparent that both results are consistent with the baseline finding, supporting the inverse U-shaped relationship between temperature and construction labor productivity. Therefore, our empirical evidence about the inverse U-shaped temperature-productivity nexus in the construction industry is robust and valid. The derivative calculation results show that when minimum temperature is 21.45°C or maximum temperature is 28.27°C, construction labor productivity reaches the highest. In addition, it has been shown that the production scale affects labor productivity by increasing the efficiency of using production tools and processing raw materials (Bárány and Siegel, 2021). So, we add a proxy variable for production size, construction completion value per capita (completion) to the regression analysis to test whether there are missing factors in the model. The results show that this variable does not affect the fit of the model or the significance of the independent variables. This suggests that the relationship we obtain between temperature and labor productivity is robust.
5 Discussion
Studies in the physiology and engineering literature have discovered that temperature anomalies have a negative impact on individual health and productivity, particularly for construction workers engaged in outside projects (Kjellstrom et al., 2009). To investigate the effect of temperature on labor productivity, we analyze data on construction enterprises from 2006 to 2019 in 31 provinces across Mainland China and separately for Eastern and Central and Western China. Further, we explore the impact of technological innovation on the temperature-labor productivity relationship. The findings are robust and valid.
5.1 Discussion of baseline results
We propose and testify an inverse U-shaped link between temperature and labor productivity. The empirical analysis results show that, in the low-temperature range, as the temperature rises, labor productivity will rise; however, once the temperature reaches the “ideal level”, further temperature rises will cause a decline in labor productivity. The “ideal level” of temperature, that is, the temperature corresponding to the peak of labor productivity is 24.90°C. This is consistent with Kim and Hong (2020)’s finding that employees are most productive when the temperature is around 25°C. Considering the different climates in China, we divide the provinces into Eastern China and Central and Western China before performing the model estimation. The results show that the inverse U-shaped relationship between temperature and labor productivity still exists in different regions. But labor productivity peaks at 20°C in Central and Western China and at 26.25°C in Eastern China. The difference in optimum temperature may be due to the fact that workers in areas with hotter temperatures (Eastern China) are more accustomed to hot weather and are better adapted to heat stress than workers in other areas (Zhu et al., 2021). Therefore, workers in Eastern China are more tolerant to high temperatures and they could reach peak labor productivity at higher temperatures.
Temperature changes affect every construction worker, and a large number of affected individuals could have a substantial overall impact on construction output. An uncomfortable temperature environment may exacerbate enterprise labor productivity loss by reducing labor intensity in construction activities and the amount of labor available (Carleton and Hsiang, 2016). As climate change poses serious risks to businesses, proactive and appropriate measures are naturally required in response to these risks (Xue et al., 2021; Han et al., 2022; Liu et al., 2022; Wang, 2022). For example, by modifying work hours and work shifts, construction contractors could reduce workers’ work time and intensity on high-temperature days in summer and low-temperature days in winter. By regularly educating construction workers on how to avoid accidents in extreme temperatures, it is also beneficial in lowering the detrimental effects of extreme temperatures on labor output.
5.2 Discussion of technological innovation impact results
Considering the difference in regional levels of technological innovation in China and its impact on construction labor productivity, we further analyze whether technological innovation level affects the relationship between temperature and labor productivity. The heterogeneity test result suggests that high levels of technological innovation capability can make the inverse U-shaped curve between temperature and labor productivity smoother, reducing the volatility brought by temperature to labor productivity.
There are significant regional differences in the level of economic development and the rate of technological progress in China (Su et al., 2020), which also leads to differences in the level of technological innovation between different regions. Innovative technologies can create more advanced production machines and safer construction equipment, improving the comfort and safety aspects of the working environment (Du et al., 2022). By providing advanced production tools, innovative technologies can mitigate the negative effects of unfavorable conditions and help enterprises maintain greater levels of labor productivity when temperature changes dramatically. So, businesses should strive to strengthen technological innovation and integrate it into every aspect of engineering management.
5.3 Future research direction
Our study is limited by data availability, so future studies related to this paper could be improved in the following ways. First, neuroscience and psychology theories indicate that temperature affects labor productivity by influencing one’s physical and mental state. This implies that the collection of relevant physiological data on the human body allows the study of medically mediated effects between temperature and labor productivity. Second, the labor productivity of enterprises may also be related to other personal characteristics of construction workers, such as gender difference and education level of workers. Future research could try to obtain quarterly data on variables of construction workers’ personal characteristics in each firm to expand the control variables in this regard. Finally, considering the different climates and temperatures in China, scholars could also study the mechanisms of temperature effects on workers from a climatological perspective.
6 Conclusion
Climate change often puts businesses under a considerable amount of pressure. Nowadays, global temperatures fluctuate frequently, which destroys the working environment and physical conditions of workers and lowers labor productivity. We take Chinese construction enterprises as the research setting to examine the effect of temperature on labor productivity, and divide provinces into Eastern and Central and Western to study the temperature-productivity relationship separately. In terms of variability in the level of technological innovation, we examine the effect of technological innovation on the temperature-productivity relationship. For validity and reliability, we also implement robustness tests. The empirical results demonstrate that: 1) There is an inverse U-shaped relationship between temperature and labor productivity. The labor productivity in construction enterprises reaches its highest when the average temperature is 24.90°C, and corrodes when the temperature is either too high or too low. 2) The optimum temperature for labor productivity in Eastern China is 26.25°C, and 20°C in Central and Western China. 3) Technological innovation may mitigate the adverse effects of temperature fluctuations by providing advanced equipment, thus helping enterprises maintain greater levels of labor productivity. This paper confirms the impact of temperature on labor productivity and offers empirical support for subsequent steps to lessen the negative impact of temperature on enterprise labor productivity. It also offers a basis for policy-making related to the health issues of laborers, especially outdoor workers.
Data availability statement
The original contributions presented in the study are included in the article/Supplementary Material, further inquiries can be directed to the corresponding author.
Author contributions
WZ: methodology, validation, supervision. ND: methodology, formal analysis, writing—review and editing. YH: software, writing—review and editing. JH: data curation, writing—original draft preparation. NZ: software, formal analysis, writing—original draft preparation. All authors have read and agreed to the published version of the manuscript.
Funding
This research is supported by the National Natural Science Foundation of China (Grant Number: 72001160), the National Social Science Foundation of China (Grant number: 21BTJ064) and the Social Science Planning Foundation of Shandong Province (Grant number: 16CTJJ01).
Conflict of interest
The authors declare that the research was conducted in the absence of any commercial or financial relationships that could be construed as a potential conflict of interest.
Publisher’s note
All claims expressed in this article are solely those of the authors and do not necessarily represent those of their affiliated organizations, or those of the publisher, the editors and the reviewers. Any product that may be evaluated in this article, or claim that may be made by its manufacturer, is not guaranteed or endorsed by the publisher.
References
Abdel-Wahab, M., and Vogl, B. (2011). Trends of productivity growth in the construction industry across Europe, US and Japan. Constr. Manag. Econ. 29 (6), 635–644. doi:10.1080/01446193.2011.573568
Adhvaryu, A., Kala, N., and Nyshadham, A. (2020). The light and the heat: Productivity co-benefits of energy-saving technology. Rev. Econ. Stat. 102 (4), 779–792. doi:10.1162/rest_a_00886
Anselin, L. (2003). Spatial externalities, spatial multipliers, and spatial econometrics. Int. Reg. Sci. Rev. 26 (2), 153–166. doi:10.1177/0160017602250972
Anselin, L. (2002). Under the hood issues in the specification and interpretation of spatial regression models. Agr. Econ. 17 (3), 247–267. doi:10.1111/j.1574-0862.2002.tb00120.x
Avotra, A. A. R. N., Chenyun, Y., Yongmin, W., Lijuan, Z., and Nawaz, A. (2021). Conceptualizing the state of the art of corporate social responsibility (CSR) in green construction and its nexus to sustainable development. Front. Environ. Sci. 9, 774822. doi:10.3389/fenvs.2021.774822
Bárány, Z. L., and Siegel, C. (2021). Engines of sectoral labor productivity growth. Rev. Econ. Dyn. 39, 304–343. doi:10.1016/j.red.2020.07.007
Blaauw, S. A., Maina, J. W., and O'Connell, J. (2022). Exposure of construction workers to hazardous emissions in highway rehabilitation projects measured with low-cost sensors. Environ. Pollut. 313, 119872. doi:10.1016/j.envpol.2022.119872
Burke, M., Hsiang, S. M., and Miguel, E. (2015). Global non-linear effect of temperature on economic production. Nature 527 (7577), 235–239. doi:10.1038/nature15725
Carleton, T. A., and Hsiang, S. M. (2016). Social and economic impacts of climate. Science 353 (6304), aad9837. doi:10.1126/science.aad9837
Chen, J., Li, Q., and Wang, X. (2022). Does the government’s environmental attention improve enterprise green innovation?—evidence from China. Front. Environ. Sci. 10, 999492. doi:10.3389/fenvs.2022.999492
Chinnadurai, J., Venugopal, V., Kumaravel, P., and Paramesh, R. (2016). Influence of occupational heat stress on labour productivity–a case study from Chennai, India. Int. J. Product. Perform. Manag. 65 (2), 245–255. doi:10.1108/IJPPM-08-2014-0121
Cremades, R., Surminski, S., Máñez Costa, M., Hudson, P., Shrivastava, P., and Gascoigne, J. (2018). Using the adaptive cycle in climate-risk insurance to design resilient futures. Nat. Clim. Change 8 (1), 4–7. doi:10.1038/s41558-017-0044-2
Deschenes, O. (2014). Temperature, human health, and adaptation: A review of the empirical literature. Energy Econ. 46, 606–619. doi:10.1016/j.eneco.2013.10.013
Dosio, A., Mentaschi, L., Fischer, E. M., and Wyser, K. (2018). Extreme heat waves under 1.5 °C and 2°C global warming. Environ. Res. Lett. 13 (5), 054006. doi:10.1088/1748-9326/aab827
Du, Q., Deng, Y., Zhou, J., Wu, J., and Pang, Q. (2022). Spatial spillover effect of carbon emission efficiency in the construction industry of China. Environ. Sci. Pollut. Res. 29 (2), 2466–2479. doi:10.1007/s11356-021-15747-9
Durdyev, S., Ismail, S., and Kandymov, N. (2018). Structural equation model of the factors affecting construction labor productivity. J. Constr. Eng. Manage. 144 (4), 04018007. doi:10.1061/(ASCE)CO.1943-7862.0001452
Ercan, T. (2019). New three-part model of innovation activity in construction companies. J. Constr. Eng. Manage. 145 (5), 04019022. doi:10.1061/(ASCE)CO.1943-7862.0001645
Feller, U., and Vaseva, I. I. (2014). Extreme climatic events: Impacts of drought and high temperature on physiological processes in agronomically important plants. Front. Environ. Sci. 2, 39. doi:10.3389/fenvs.2014.00039
Fiorese, E., Bonollo, F., Timelli, G., Arnberg, L., and Gariboldi, E. (2015). New classification of defects and imperfections for aluminum alloy castings. Int. J. Mater. 9 (1), 55–66. doi:10.1007/BF03355602
Fishman, R., Carrillo, P., and Russ, J. (2019). Long-term impacts of exposure to high temperatures on human capital and economic productivity. J. Environ. Econ. Manage. 93, 221–238. doi:10.1016/j.jeem.2018.10.001
Gasparrini, A., Guo, Y., Hashizume, M., Lavigne, E., Zanobetti, A., Schwartz, J., et al. (2015). Mortality risk attributable to high and low ambient temperature: A multicountry observational study. Lancet 386 (9991), 369–375. doi:10.1016/S0140-6736(14)62114-0
Grifoni, D., Messeri, A., Crisci, A., Bonafede, M., Pasi, F., Gozzini, B., et al. WORKLIMATE Collaborative Group (2021). Performances of limited area models for the WORKLIMATE heat–health warning system to protect worker’s health and productivity in Italy. Int. J. Environ. Res. Public Health 18 (18), 9940. doi:10.3390/ijerph18189940
Haans, R. F., Pieters, C., and He, Z. L. (2016). Thinking about U: Theorizing and testing U-and inverted U-shaped relationships in strategy research. Strateg. Manag. J. 37 (7), 1177–1195. doi:10.1002/smj.2399
Han, Z., Wang, Y., and Pang, J. (2022). Does environmental regulation promote green merger and acquisition? Evidence from the implementation of China’s newly revised environmental protection law. Front. Environ. Sci. 10, 1042260. doi:10.3389/fenvs.2022.1042260
Hanna, A. S., Taylor, C. S., and Sullivan, K. T. (2005). Impact of extended overtime on construction labor productivity. J. Constr. Eng. Manage. 131 (6), 734–739. doi:10.1061/(asce)0733-9364(2005)131:6(734)
Hargreaves, M. (2008). Physiological limits to exercise performance in the heat. J. Sci. Med. Sport. 11 (1), 66–71. doi:10.1016/j.jsams.2007.07.002
He, K., Ramzan, M., Awosusi, A. A., Ahmed, Z., Ahmad, M., and Altuntaş, M. (2021). Does globalization moderate the effect of economic complexity on CO2 emissions? Evidence from the top 10 energy transition economies. Front. Environ. Sci. 9, 778088. doi:10.3389/fenvs.2021.778088
Hong, M., Chen, S., and Zhang, K. (2021). Impact of the “low-carbon city pilot” policy on energy intensity based on the empirical evidence of Chinese cities. Front. Environ. Sci. 9, 717737. doi:10.3389/fenvs.2021.717737
Huynh, T. D., Nguyen, T. H., and Truong, C. (2020). Climate risk: The price of drought. J. Corp. Finance 65, 101750. doi:10.1016/j.jcorpfin.2020.101750
Kim, H., and Hong, T. (2020). Determining the optimal set-point temperature considering both labor productivity and energy saving in an office building. Appl. Energy 276, 115429. doi:10.1016/j.apenergy.2020.115429
King, M., Ray, M., Mulligan, D., and Carnahan, H. (2020). Does training in the cold improve cold performance? Int. J. Ind. Ergon. 76, 102926. doi:10.1016/j.ergon.2020.102926
Kjellstrom, T., Holmer, I., and Lemke, B. (2009). Workplace heat stress, health and productivity–an increasing challenge for low and middle-income countries during climate change. Glob. Health Action 2 (1), 2047. doi:10.3402/gha.v2i0.2047
Kouloukoui, D., de Oliveira Marinho, M. M., da Silva Gomes, S. M., Kiperstok, A., and Torres, E. A. (2019). Corporate climate risk management and the implementation of climate projects by the world's largest emitters. J. Clean. Prod. 238, 117935. doi:10.1016/j.jclepro.2019.117935
Kpognon, K. D., Atangana Ondoa, H., Bah, M., and Asare-Nuamah, P. (2022). Fostering labour productivity growth for productive and decent job creation in sub-saharan african countries: The role of institutional quality. J. Knowl. Econ. 13 (3), 1962–1992. doi:10.1007/s13132-021-00794-x
Li, H., Meng, L., Wang, Q., and Zhou, L. A. (2008). Political connections, financing and firm performance: Evidence from Chinese private firms. J. Dev. Econ. 87 (2), 283–299. doi:10.1016/j.jdeveco.2007.03.001
Li, K. W., Yu, R. F., Gao, Y., Maikala, R. V., and Tsai, H. H. (2009). Physiological and perceptual responses in male Chinese workers performing combined manual materials handling tasks. Int. J. Ind. Ergon. 39 (2), 422–427. doi:10.1016/j.ergon.2008.08.004
Li, X., Chow, K. H., Zhu, Y., and Lin, Y. (2016). Evaluating the impacts of high-temperature outdoor working environments on construction labor productivity in China: A case study of rebar workers. Build. Environ. 95, 42–52. doi:10.1016/j.buildenv.2015.09.005
Liu, H., Jiang, J., Xue, R., Meng, X., and Hu, S. (2022). Corporate environmental governance scheme and investment efficiency over the course of COVID-19. Finance Res. Lett. 47, 102726. doi:10.1016/j.frl.2022.102726
Liu, H., Wang, Y., Xue, R., Linnenluecke, M., and Cai, C. W. (2021). Green commitment and stock price crash risk. Finance Res. Lett. 47, 102646. doi:10.1016/j.frl.2021.102646
Malhi, G. S., Kaur, M., and Kaushik, P. (2021). Impact of climate change on agriculture and its mitigation strategies: A review. Sustainability 13 (3), 1318. doi:10.3390/su13031318
Marengo, J. A., Camarinha, P. I., Alves, L. M., Diniz, F., and Betts, R. A. (2021). Extreme rainfall and hydro-geo-meteorological disaster risk in 1.5, 2.0, and 4.0° C global warming scenarios: An analysis for Brazil. Front. Clim. 3, 610433. doi:10.3389/fclim.2021.610433
Mathee, A., Oba, J., and Rose, A. (2010). Climate change impacts on working people (the HOTHAPS initiative): Findings of the South African pilot study. Glob. Health Action 3 (1), 5612. doi:10.3402/gha.v3i0.5612
Matsumoto, K. I. (2019). Climate change impacts on socioeconomic activities through labor productivity changes considering interactions between socioeconomic and climate systems. J. Clean. Prod. 216, 528–541. doi:10.1016/j.jclepro.2018.12.127
McInnes, J. A., Akram, M., MacFarlane, E. M., Keegel, T., Sim, M. R., and Smith, P. (2017). Association between high ambient temperature and acute work-related injury: A case-crossover analysis using workers' compensation claims data. Scand. J. Work Environ. Health 43 (1), 86–94. doi:10.5271/sjweh.3602
Moda, H. M., Filho, W. L., and Minhas, A. (2019). Impacts of climate change on outdoor workers and their safety: Some research priorities. Int. J. Environ. Res. Public Health 16 (18), 3458. doi:10.3390/ijerph16183458
Mohamed, S., and Srinavin, K. (2005). Forecasting labor productivity changes in construction using the PMV index. Int. J. Ind. Ergon. 35 (4), 345–351. doi:10.1016/j.ergon.2004.09.008
Morabito, M., Crisci, A., Messeri, A., Messeri, G., Betti, G., Orlandini, S., et al. (2017). Increasing heatwave hazards in the southeastern European Union capitals. Atmosphere 8 (7), 115. doi:10.3390/atmos8070115
Nasirzadeh, F., and Nojedehi, P. (2013). Dynamic modeling of labor productivity in construction projects. Int. J. Proj. Manag. 31 (6), 903–911. doi:10.1016/j.ijproman.2012.11.003
Romer, P. M. (1990). Endogenous technological change. J. Polit. Econ. 98 (5), 71–102. doi:10.3386/w3210
Sakhel, A. (2017). Corporate climate risk management: Are European companies prepared? J. Clean. Prod. 165, 103–118. doi:10.1016/j.jclepro.2017.07.056
Sebestyén, V., Czvetkó, T., and Abonyi, J. (2021). The applicability of big data in climate change research: The importance of system of systems thinking. Front. Environ. Sci. 9, 619092. doi:10.3389/fenvs.2021.619092
Selvi, A., Rajasekar, A., Theerthagiri, J., Ananthaselvam, A., Sathishkumar, K., Madhavan, J., et al. (2019). Integrated remediation processes toward heavy metal removal/recovery from various environments-a review. Front. Environ. Sci. 7, 66. doi:10.3389/fenvs.2019.00066
Shi, Q., Shan, Y., Zhong, C., Cao, Y., and Xue, R. (2022). How would GVCs participation affect carbon intensity in the “Belt and Road Initiative” countries? Energy Econ. 111, 106075. doi:10.1016/j.eneco.2022.106075
Sohail, M. T., Mustafa, S., Ma, M., and Riaz, S. (2022). Agricultural communities’ risk assessment and the effects of climate change: A pathway toward green productivity and sustainable development. Front. Environ. Sci. 10, 948016. doi:10.3389/fenvs.2022.948016
Somanathan, E., Somanathan, R., Sudarshan, A., and Tewari, M. (2021). The impact of temperature on productivity and labor supply: Evidence from Indian manufacturing. J. Polit. Econ. 129 (6), 1797–1827. doi:10.1086/713733
Stern, D. I., and Kaufmann, R. K. (2014). Anthropogenic and natural causes of climate change. Clim. Change 122 (1), 257–269. doi:10.1007/s10584-013-1007-x
Su, X., Zhou, S., Xue, R., and Tian, J. (2020). Does economic policy uncertainty raise corporate precautionary cash holdings? Evidence from China. Acc. Finance 60 (5), 4567–4592. doi:10.1111/acfi.12674
Sun, Y., Yang, Y., Huang, N., and Zou, X. (2020). The impacts of climate change risks on financial performance of mining industry: Evidence from listed companies in China. Resour. Policy 69, 101828. doi:10.1016/j.resourpol.2020.101828
Tan, C. K. L., and Abdul-Samad, Z. (2022). A study of the impact of COVID-19 on construction workforce productivity in Malaysia. Int. J. Product. Perform. Manag. (ahead-of-print). doi:10.1108/IJPPM-07-2021-0421
Tao, H., Zhuang, S., Xue, R., Cao, W., Tian, J., and Shan, Y. (2022). Environmental finance: An interdisciplinary review. Technol. Forecast. Soc. Change 179, 121639. doi:10.1016/j.techfore.2022.121639
Tian, J. F., Pan, C., Xue, R., Yang, X. T., Wang, C., Ji, X. Z., et al. (2020). Corporate innovation and environmental investment: The moderating role of institutional environment. Adv. Clim. Chang. Res. 11 (2), 85–91. doi:10.1016/j.accre.2020.05.003
Tian, J., Yu, L., Xue, R., Zhuang, S., and Shan, Y. (2022). Global low-carbon energy transition in the post-COVID-19 era. Appl. Energy 307, 118205. doi:10.1016/j.apenergy.2021.118205
Uzar, U. (2020). Political economy of renewable energy: Does institutional quality make a difference in renewable energy consumption? Renew. Energy 155, 591–603. doi:10.1016/j.renene.2020.03.172
Varghese, B. M., Barnett, A. G., Hansen, A. L., Bi, P., Hanson-Easey, S., Heyworth, J. S., et al. (2019). The effects of ambient temperatures on the risk of work-related injuries and illnesses: Evidence from Adelaide, Australia 2003–2013. Environ. Res. 170, 101–109. doi:10.1016/j.envres.2018.12.024
Venugopal, V., Latha, P. K., Shanmugam, R., Krishnamoorthy, M., and Johnson, P. (2020). Occupational heat stress induced health impacts: A cross-sectional study from south Indian working population. Adv. Clim. Change Res. 11 (1), 31–39. doi:10.1016/j.accre.2020.05.009
Wagner, G. (2022). Climate risk is financial risk. Science 376 (6598), 1139. doi:10.1126/science.add2160
Wan, D., Xue, R., Linnenluecke, M., Tian, J., and Shan, Y. (2021). The impact of investor attention during COVID-19 on investment in clean energy versus fossil fuel firms. Finance Res. Lett. 43, 101955. doi:10.1016/j.frl.2021.101955
Wang, W. (2022). Tax equity, green innovation and corporate sustainable development. Front. Environ. Sci. 10, 1062179. doi:10.3389/fenvs.2022.1062179
Watts, N., Amann, M., Arnell, N., Ayeb-Karlsson, S., Belesova, K., Boykoff, M., et al. (2019). The 2019 report of the lancet countdown on health and climate change: Ensuring that the health of a child born today is not defined by a changing climate. Lancet 394 (10211), 1836–1878. doi:10.1016/S0140-6736(19)32596-6
Xiao, H., and You, J. (2021). The heterogeneous impacts of human capital on green total factor productivity: Regional diversity perspective. Front. Environ. Sci. 9, 713562. doi:10.3389/fenvs.2021.713562
Xue, R., Qian, G., Qian, Z., and Li, L. (2021). Entrepreneurs’ implicit and explicit achievement motives and their early international commitment. Manag. Int. Rev. 61 (1), 91–121. doi:10.1007/s11575-020-00436-5
Xue, R., Qian, G., Qian, Z., and Li, L. (2022). How do foreign customers' perceptions of product-harm crises affect their transfer of capability-and character-based stigma? Int. Mark. Rev. 39 (1), 120–141. doi:10.1108/IMR-09-2020-0197
Yu, S., Cai, C., Zhang, S., and You, P. (2022). Does climate change affect enterprises’ ability to sell their products? Front. Ecol. Evol. 10, 944964. doi:10.3389/fevo.2022.944964
Yu, Y., Umer, W., Yang, X., and Antwi-Afari, M. F. (2021). Posture-related data collection methods for construction workers: A review. Autom. Constr. 124, 103538. doi:10.1016/j.autcon.2020.103538
Zhang, P., Deschenes, O., Meng, K., and Zhang, J. (2018). Temperature effects on productivity and factor reallocation: Evidence from a half million Chinese manufacturing plants. J. Environ. Econ. Manage. 88, 1–17. doi:10.1016/j.jeem.2017.11.001
Zhang, X., Zhang, R., Zhao, M., Wang, Y., and Chen, X. (2022). Policy orientation, technological innovation and energy-carbon performance: An empirical study based on China’s new energy demonstration cities. Front. Environ. Sci. 10, 846742. doi:10.3389/fenvs.2022.846742
Zhu, J., Wang, S., Zhang, B., and Wang, D. (2021). Adapting to changing labor productivity as a result of intensified heat stress in a changing climate. GeoHealth 5, e2020GH000313. doi:10.1029/2020GH000313
Keywords: climate change risk, extreme weather, temperature anomalies, labor productivity, technological innovation
Citation: Zhang W, Ding N, Han Y, He J and Zhang N (2023) The impact of temperature on labor productivity——evidence from temperature-sensitive enterprises. Front. Environ. Sci. 10:1039668. doi: 10.3389/fenvs.2022.1039668
Received: 08 September 2022; Accepted: 12 December 2022;
Published: 04 January 2023.
Edited by:
Shiyang Hu, Chongqing University, ChinaCopyright © 2023 Zhang, Ding, Han, He and Zhang. This is an open-access article distributed under the terms of the Creative Commons Attribution License (CC BY). The use, distribution or reproduction in other forums is permitted, provided the original author(s) and the copyright owner(s) are credited and that the original publication in this journal is cited, in accordance with accepted academic practice. No use, distribution or reproduction is permitted which does not comply with these terms.
*Correspondence: Yilong Han, eWlsb25nLmhhbkB0b25namkuZWR1LmNu