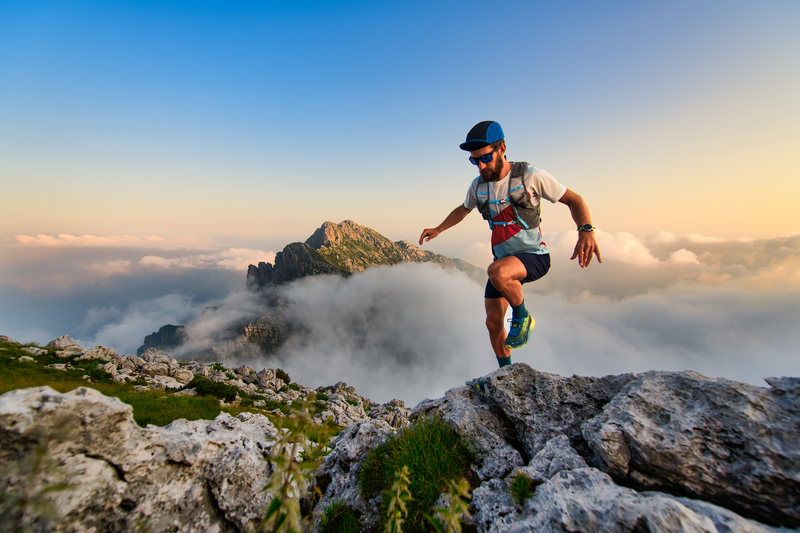
94% of researchers rate our articles as excellent or good
Learn more about the work of our research integrity team to safeguard the quality of each article we publish.
Find out more
ORIGINAL RESEARCH article
Front. Environ. Sci. , 17 October 2022
Sec. Environmental Economics and Management
Volume 10 - 2022 | https://doi.org/10.3389/fenvs.2022.1036464
This article is part of the Research Topic Ecological Intensification and Sustainable Intensification: Increasing Benefits to and Reducing Impacts on the Environment to Improve Future Agricultural and Food Systems View all 10 articles
This study explores how promoting e-commerce participation impacts the adoption of green agrotechnology by resettlers in China’s Three Gorges Reservoir area and helps rural revitalization and the realization of value from ecological produce. First, we combine induced innovation model theory with the risk perception factor of expected utility theory. A model of resettlers’ green agrotechnology adoption under different levels of e-commerce participation is constructed, and research hypotheses are proposed accordingly. Survey data gathered from resettled farmers in Zigui, the first county of the studied area, are tested empirically with an ordered probit model. The results show first, that e-commerce participation significantly and positively affects the level of green agrotechnology adoption at the 1% level; and second, that expectations of the ecological value of agricultural products and the agrotechnology support provided by e-commerce are important driving factors. The promotion effect of different modes of e-commerce participation on agrotechnology adoption differ. The risk-averse behavior of resettlers can weaken the promotion effect of e-commerce participation on agrotechnology adoption.
The increasing number of hydropower plants being built around the world to achieve clean energy has led to a large number of compulsory population movements, resulting in the emergence of resettled farmers as a group. In accordance with the World Bank’s resettled guidelines, rural resettlers in China are mostly resettled in the back-to-back mode of “returning land to land and agriculture to agriculture” for the sake of continuing their original livelihoods (Yan et al., 2018). However, due to the limited resource-carrying capacity of the Three Gorges Reservoir area, the arable land available there has become increasingly scarce. Most of the compensation land received by resettlers is fragmented and low in quality, causing not only limited output but also significant increases in the cost of crop cultivation (Zhang 2021). Chinese rural re-settlers’ per capita income was only about 76% of that of ordinary farmers in 2014. Therefore, fewer than 30% of resettlers actually cultivate the land that the government transferred to them at a high cost, and many tend to abandon the land and go out to work instead (Ni and Shao 2013). Currently, in the context of natural capital stock constraint, the only way to guarantee that resettlers can increase their income from local farming is by applying and promoting green agrotechnology.
Green agricultural technology aims to solve environmental problems and promote sustainable agricultural development. It will improve the soil by reducing pollution, leverage the ecological value of agricultural products, and raise crop yields (Zuo and Fu 2021). The waste of resources and environmental pollution caused by the unscientific nature of traditional agricultural technology hinders the green development of agriculture in resettlement areas. Achieving a green transformation of agricultural development is an important way to solve the current agricultural development challenges in resettlement areas. As resettlers’ livelihoods are highly vulnerable and they struggle to withstand the ex-post effects of risks, they mainly choose to avoid risk. Their conservative behavior in agricultural production hinders the adoption of green agrotechnology to a certain extent. Therefore, a long-term mechanism of green agrotechnology adoption is needed to solve the current problem of low level of green agrotechnology in resettlement areas and to achieve sustainable development of resettlement areas and individual income of resettlers.
For involuntary resettlers with impaired livelihood capital and broken social networks of origin, rural e-commerce, a new rural industry with convenient participation channels and an almost zero threshold for resource consumption, has become the first choice to improve the production and marketing of agricultural products (Yin and Choi 2022). For example, in the Three Gorges Reservoir area of China, the town of Xingshan is located in a mountainous area with a low land stock, but resettlers have achieved an average daily sale of 100,000 kg of citrus by using live streaming. The strong development of Internet technology has enriched agricultural e-commerce models, with traditional e-commerce, social e-commerce, and live e-commerce all expanding in scale (Chen et al., 2022). Heterogeneous participation in rural e-commerce brings innovation to the traditional agricultural products business model and concept while effectively enhancing the income of resettled households. At the same time, the online sales model requires quality standardization and ecological branding, which will certainly promote the application of green agrotechnology and bring high-quality change to resettled areas (Xiao et al., 2021).
Current research on empowering rural agents to promote the adoption of green agrotechnology focuses on three aspects. The first is the promotion of environmental regulations and government subsidies, which can be used to guide technology application behavior in agricultural production. Reducing the costs involved in such production will support and guarantee the sustainable application of green agrotechnology (Dong et al., 2022). Second, research has explored the contribution of regional resource endowments, where the level of development, farming history, and soil quality are direct factors determining individual adoption intentions and behaviors (Zeng et al., 2019; Wu and Zhou 2021), while population aging and the stock of agricultural technicians have obvious indirect effects (Natkhov and Vasilenok 2021). A third stream, focusing on the role of rural households and individual characteristics, has found that the degree of green agrotechnology adoption is constrained by farmers’ relational capital (Wang et al., 2020), socioeconomic status (Bidogeza et al., 2009), and learning and training opportunities (Chatzimichael et al., 2014).
In contrast, risk preferences play a dominant role in individual production decision behavior (Gao and Niu 2019), and risk perceptions, in turn, positively moderate the degree of association between risk preferences and agrotechnology adoption (Qiu et al., 2020). For example, perceived risk from agricultural production affects the use of irrigation technology (Koundouri et al., 2006), fertilizers (Adnan et al., 2019), etc. Since it is difficult for individuals to fully understand the benefits associated with the use of green agrotechnology, they tend to have doubts about the risks they need to take in technology adoption (Chavas and Nauges 2020). However, further studies have shown that farmers may use subjective risk judgements to weigh the pros and cons; that is, there is a negative relationship between the perceived risk of using green agrotechnology and the probability of adoption (Duong et al., 2019). The research outlined above is limited by the fact that the paths between variables have only been tested empirically, while specific influence mechanisms have not yet been theoretically deduced. Hence, such mechanisms are based only on the summary of phenomena and experiences and lack a scientific basis. Further exploration is required of such mechanisms and the paths whereby the interactive feedback model of e-commerce operation–agrotechnology application–income enhancement can be realized.
The Chinese government has implemented appropriate support policies for Reservoir resettlers, and the livelihood level and social integration of this group have been significantly improved. Ensuring the sustainable self-development of involuntary project resettlers has attracted research attention globally (Karimi and Taifur 2013). The current research was conducted as follows. First, we constructed a model of the benefits of green agrotechnology adoption by resettled households under different e-commerce participation scenarios, based on utility optimization theory and induced agricultural technological innovation theory. Second, we introduced risk perception as a moderating variable and combined it with expected utility theory to improve the multi-factor influence mechanism model of resettled technology adoption. Third, we proposed research hypotheses according to the theoretical derivation and empirically tested the theoretical model using the ordered probit model with survey data gathered from resettled farmers in Zigui, Hubei province, China, the first county in the Three Gorges Reservoir area. Next, we explored how the promotion of rural e-commerce participation affects the adoption of green agrotechnology by resettlers and the core elements within this process and investigated the perturbing influence of the particular risk preferences of involuntarily relocated populations in regard to promoting modern agricultural technology and achieving sustainable livelihood development. Last, we provide support for decision making in regard to compensating and supporting resettlers in water conservancy and hydropower projects.
In the theory of agricultural supply chain integration, in response to the impact of the agricultural business segment on the production segment, induced agricultural technological innovation theory was proposed at the start of the 20th century. This theory argues that the business model is closely related to agricultural technological innovation (Cowan et al., 2015) and that a proposed increase, decrease, or change of production and business factors will necessarily bring about technological innovation (Schultz 1987). Accordingly, it is clear that the promotion of Internet technologies and the development of rural e-commerce will inevitably promote changes in production by farmers, namely the use of green technologies in agriculture. On the one hand, the combination of e-commerce and the digital economy completes the construction of a digital system for the agricultural industry which is more conducive to the value-added and market demand transfer of green agricultural products and allows for more convenient mining and teaching of green agricultural technologies (Fu and Zhang 2022). On the other hand, the expansion of agricultural product sales by e-commerce and logistics platforms has led to a surge in the number of end customers with green consumption needs. The trend of standardization and branding of agricultural products has led to increasingly stringent requirements for the production process, which has also forced the improvement and standardization of the use of resettled agricultural technologies (Dong et al., 2021) as shown in Figure 1.
In the process of compulsory relocation, Reservoir resettlers lose their livelihood capital, livelihood capacity, and social network. Thus, the focus of subsequent development has been on how to achieve maximum benefits under resource constraints (Zhang 2021). It is a requirement of the principle of developmental resettled for hydropower projects in China that the post-resettled production and living standards of resettlers should not be lower than those before relocation. Therefore, whether resettled farmers can promote the application of green agrotechnology in agricultural production after participating in rural e-commerce is mainly determined by the related profit, so it can be judged according to the profit function corresponding to different participation situations of e-commerce, as shown in Eq. 1.
where
In the above equation, g is the degree of green agrotechnology adoption by resettled households, and I is other agricultural production factor inputs. The degree of households’ inputs is closely related to the level of agrotechnology they use, so the latter can be expressed in the functional form
At this point, it is necessary to find the optimal level of adoption of green agrotechnology for resettlers under the profit maximization condition, which is the derivative of profit πi to the degree of adoption of agrotechnology g as shown in Eq. 4.
In the above equation,
The results calculated from Eq. 4 is shown in Figure 2. In the case of resettled households participating in e-commerce, when
Hypothesis 1. Participation in e-commerce has a catalytic effect on resettled growers’ green agrotechnology adoption behavior.Different e-commerce participation models will have different benefits for resettled households as the participating subjects and circulation links vary. This paper classifies resettled households’ e-commerce participation model as either platform e-commerce or social e-commerce, according to the survey data. The platform e-commerce model refers to resettled households selling through online trading platforms, such as Taobao and Jindong. The social e-commerce model refers to such households selling through a network of acquaintances to form a fixed source of online customers, such as through WeChat or QQ. The platform e-commerce model may obtain higher product revenue as a large number of merchants are participating: While the platform products are highly competitive barriers to entry, the requirements for technical investment are also higher. The social e-commerce model has price advantages, but resettled households have a limited network group of acquaintances, and the fact that e-commerce has a limited effect on increasing income leads to less willingness to adopt technical innovation and lower rates of green agrotechnology adoption. Accordingly, the following research hypothesis can be proposed.
Hypothesis 2. There are differences in the promotion of green agrotechnology adoption among resettled households according to various e-commerce participation models, with the platform e-commerce model outperforming the social e-commerce model.However, a single profit-seeking factor is not sufficient in explaining the motivation of resettled households when they adopt green agrotechnology (Adnan et al., 2021). The increased vulnerability of livelihood and the relatively scarce livelihood capital led to a weakening in the ability of involuntary resettled groups to tackle risks to their livelihoods (Gong et al., 2020). However, the application of green agrotechnology may also give rise to additional risks, including technological, natural, and market risks. For example, compared with chemical pesticides, organic pesticides are difficult to operate and highly targeted. Resettlers faced with planting new crops in resettled areas may be vulnerable to yield reduction after application of a new technology. Especially if they are not familiar with pesticide selection, application dosage, and application time (Fang et al., 2021). In addition, resettlers whose original social networks are a great distance away from the resettled area and who have poor information channels may face failure to achieve high quality and high prices for their agricultural products, and with high technology costs (Lu et al., 2018). Therefore, resettlers have a lot of uncertainty when adopting green agrotechnology, and thus perceive the existence of risks, and in view of their relatively weak risk tolerance, they are mostly risk-averse subjects. In other words, the greater levels of risk perception in the resettlers’ technology adoption behavior will directly affect the role played by e-commerce in the promotion of such behavior. From the expectation-utility theory, the utility function of resettlers’ participation in rural e-commerce is shown in Eq. 5.
Where U and E are the utility and expected utility functions of resettled households, respectively,
R in the above equation is the higher order remainder term of Eq. 6, and the process of transforming the resettled participation in the electric utility U in Eq. 5 by the equation is shown in Eq. 7.
Similarly, an equation transformation of the resettled expected utility function
Since resettlers are mostly risk-averse after experiencing loss of livelihood, R is basically negligible and has
An equation transformation of Eq. 9 can locate the willingness to resist green agrotechnology
It can be calculated that
Hypothesis 3. Individual risk perceptions will constrain the degree to which e-commerce participation promotes the adoption of green agrotechnology by resettled households.
The Three Gorges Reservoir has flooded 260 km2 of arable land, and there are 354,000 rural resettlers settled in the area. This has resulted in an extremely limited environmental capacity to produce food. Zigui County in Hubei, at the head of the Three Gorges Project Reservoir, is both a resettled area and classed as a “national poverty-stricken county,” with 25% of its total population being Reservoir resettlers. As the climate and soil environment are very suitable for the growth of Navel oranges, Zigui County has become famous as China’s “Navel Orange Township.” Therefore, in recent years, through the Three Gorges resettled support funds and other promotional projects, Zigui County has been encouraged to adopt green agriculture. At present, the Zigui navel orange production area covers 23,200 ha and has an annual output of 605,000 metric tons. Across the area’s 12 towns and 116 villages, about 198,000 people are engaged in related industries, and this is the only national citrus production area that produces fresh fruit throughout the year.
In order to solve the difficulties caused by the lack of land to compensate resettlers in the county, as well as a lack of social resources and the low premium capacity of agricultural products, Zigui County took the lead in innovating an e-commerce development model in 2014 and was selected as one of the second batch of national “e-commerce-demonstrating rural counties” in 2019. In recent years, the proportion of online sales of Navel oranges has accounted for about 55% of total sales, and the per capita net income of orange farmers increased from 1,967 USD in 2014 to 3,372 USD in 2018, becoming an important way to enhance the income of resettlers. In addition, e-commerce promotes the adoption of solar pest control lights, water and fertilizer integration, Internet of Things (IoT, through which things are connected through the Internet) management, and other green agrotechnology, using networks to strengthen the ecological brand marketed as “a river of clear water, green mountains on both sides of the river, four seasons of fresh oranges.”
The data used in this study come from the Navel orange electric business and green agrotechnical survey conducted in the resettlement area of Zigui County, Hubei Province during December 2020. The sampling points were selected from a total of 34 resettlement villages and groups in three towns and one township, namely Maoping Town, Guojiaba Town, Guizhou Town, and Shuitianba Township. At present, each of the four has an electric e-commerce logistics center, an e-commerce service center, and other infrastructure and service facilities and has several green agrotechnology demonstration orchards or planting bases, such as the Bajiaolou Green Technology Tour Park, the Flying Green Plant Protection base in Guogutai Village, Guojiaba Township, and the Alibaba Group’s Future Farm in Choumushu Village, Shuitianba Township. All these were sampled.
The specific sampling method was to randomly select eight to 10 sample villages in each township, then randomly conduct household surveys. A total of 688 resettlers were interviewed. After samples with no response or doubtful key information were excluded, 660 valid questionnaires were obtained. The number of resettled households’ adoption of various green agricultural technologies is shown in Table 1.
According to the list of technical systems in the “Technical Guidelines for Green Agricultural Development (2018–2030),” resettled households mainly apply six types of green agrotechnologies: physical control technology, pollution-free pesticide technology, soil formula fertilizer technology, film and water control technology, water and fertilizer integration technology, and grafting technology. Of these, 120 households (18.2%) have adopted two kinds of technologies, and 249 households (37.7%) have adopted four or more kinds of technologies, which shows that green agrotechnologies are in the emerging stage in the resettlement area.
Using Li et al. (2020) classification of degree of agrotechnology adoption, we divided resettlers’ agrotechnology adoption into five categories, from low to high, and assigned them the following values: lower adoption = 1, low adoption = 2, moderate adoption = 3, high adoption = 4, and higher adoption = 5. Since, as an explanatory variable, the degree of resettlers’ agrotechnology adoption g is a multi-valued ordered variable, we used the ordered probit model to explore the influencing factors involved, and the underlying regression model constructed is shown in Eq. 11.
In Eq. 11,
If the random disturbance term
After an ordered-probit model is constructed in Eq. 13, the regression coefficients can be estimated using the maximum likelihood estimation (MLE) method. In addition, we conducted additional analyses independent of the probit model. To analyze the extent to which e-commerce participation drives the adoption of agricultural technologies, we analyze the marginal effects of each independent variable, as shown in Eq. 14.
Where n = 1,2,......5 represents the five degrees of resettled green agrotechnology adoption,
The specific measurement has six categories of green agricultural technologies adopted by resettled households, and the value is assigned as 1 if adopted, and 0 if not. Due to variations in natural capital among resettled households, it is not suitable to use equal weighting among the categories because of limitations placed on the use of different green agricultural technologies. Therefore, the four dimensions of economic benefits, resource saving degree, ecological benefits, and operational feasibility are considered comprehensively, and the entropy value method is applied to determine their weighting coefficients. The steps are as follows: 1)Calculate the indicator weight
TABLE 2. Evaluation indicators and weights of the degree of adoption of various types of green agrotechnology by resettled households.
According to the model deduction of the previous theoretical analysis, it can be seen that the participation behavior of e-commerce. The expected sales price of agricultural products are the keys to promoting the adoption of green agrotechnology among resettlers. The improvement of agrotechnology application capacity through training is also an important factor (Liu et al., 2022).
According to the aforementioned theoretical model, it is clear that the perceived risk factors of resettled households have an impact on e-commerce’s promotion of the use of agrotechnology. These involve various aspects and varying degrees of perception, such as natural conditions, market environment, and technical capacity. According to previous studies, there are two main types of control variables. One is demographic characteristics, including gender, age, and the level of education of respondents. The other is household endowment, including the maximum years of education of members, the proportion of household labor force, annual household income, navel orange planting area, and support from local cooperative organizations.
The definition and descriptive statistics of each variable are shown in Table 3, in which the mean value of adoption of green agrotechnology is 1.763. The overall application degree still needs to be improved; however, participation in e-commerce is more than half, indicating that resettlers generally have enthusiasm to engage in e-commerce. At the same time, the current support for training in the use of agricultural technology is still insufficient with the mean value is 0.414. The risk perception of the application of agricultural technology is around the mean value, which needs to be controlled and further development of e-commerce is required to promote the popularity of green agricultural technology in resettled areas.
Before testing the role of e-commerce participation behavior in the promotion of the application of resettled farming techniques, a multiple cointegration test between the relevant independent variables was required. The resulting variance inflation factor (VIF) was far below 10, without cointegration problems. We then examined whether the original data satisfied the parallel regression hypothesis and found that the chi-square value was not significant. This meant the hypothesis was valid for analysis using the ordered-probit model. Finally, regression models were constructed when no-control variables (Model I), household head characteristics (Model II), and household head characteristics and household endowment (Model III) were added. The regression models are shown in Table 4.
TABLE 4. Regression results of the impact of green farming technology adoption among resettled orange growers.
As shown in Table 4, e-commerce participation significantly and positively influenced resettled navel orange growers’ green agrotechnology adoption behavior at the 1% level, i.e., Hypothesis 1 holds. To analyze the influence mechanism, the relationship between e-commerce participation behavior, agricultural price expectation, and technical training support was further investigated here, as shown in Table 5. The regression results show that there is a significant positive correlation between participation in e-commerce and factors that support technology adoption support regarding such as price expectation of agricultural products and technical training support.
TABLE 5. Correlation between e-commerce participation and green agrotechnology adoption support elements for resettled households.
According to the model regression results above, participation in e-commerce has a significant positive effect on resettled households’ adoption of green agrotechnology. Specifically, various government subsidies and agricultural policies increased their green agricultural product price expectations after participating in e-commerce. For example, Zigui County has successively issued documents such as “Implementation Opinions on Accelerating E-commerce Development” and “Implementation Plan of E-commerce in Rural Areas Project,” which have greatly enhanced the information used by resettlers in their agrotechnology inputs. At the same time, resettlers have been given more technical training opportunities. For example, platforms such as Suning University and Jingdong Business School dispatched lecturers to 12 townships in Zigui County to teach e-commerce production and operation.
Based on the Hypothesis 1 test, the degree of resettlers’ agro technology adoption under the role of each factor was further analyzed to explore whether the marginal effects of different degree types affected were sensitive, as shown in Table 6.
TABLE 6. Marginal effects of each influencing factor on the degree of resettlers’ adoption of agricultural technology.
The results in Table 6 show that the percentage of resettled households with low technology adoption will decrease by 25% after they participate in e-commerce, while resettled households with moderate, higher, and high adoption will increase by 6.3%, 3.7%, and 7.4%, respectively. It is confirmed that the awareness and adoption of green farming techniques among resettlers are enhanced under the e-commerce model. Resettled households with a low level of green agrotechnology application also decreased by 19.8% and 15.8%, respectively, as the price of agricultural products improved and agrotechnology training grew in popularity, but it was not as significant as the effect of e-commerce participation.
To analyze the heterogeneity in the impact of different e-commerce participation models, this paper further empirically tested the impact of e-commerce participation models on resettled households’ green farming skills. Statistics found that, among the sampled households participating in e-commerce, 153 (38.3%) used the platform e-commerce model sample and 246 (62.7%) used the social e-commerce model sample. The empirical results are shown in Table 7. According to the level of technology adoption classified by the previous section (1–5), from the overall sample, the degree of increase in agrotechnology adoption by farmers participating in e-commerce was 0.527, while the degrees of increase for the platform e-commerce and social e-commerce models were 1.286 and 0.531, respectively. The results indicate that the degree of increase for the platform e-commerce model was greater, suggesting that it could bring about a greater increase in agrotechnology adoption than the social e-commerce model.
TABLE 7. Differences in the impact of different e-commerce participation models on resettlers’ green agrotechnology adoption.
To verify the moderating role of risk perception in resettled agrotechnology adoption, Model IV was constructed by introducing the interaction term between e-commerce participation and risk perception. The results are shown in Table 8, which indicate that while e-commerce participation significantly increases the adoption of green agrotechnology, risk perception has a significant negative correlation to it. Indeed, the negative coefficient of the interaction term confirms that risk perception weakens the positive relationship between e-commerce participation and agrotechnology adoption, so Hypothesis 3 is valid.
For example, when the rainfall in Zigui and other places reached historical extremes in the summer of 2021, to ensure the taste of fresh navel oranges was maintained, the local migration management recommended the adoption of land mulching technology in the selenium-rich planting bases of Seven Princesses, Shi Wai Tian Yuan and other major e-commerce companies. However, if the residual film cannot be effectively recovered or is uncovered too late, the film will be left in the soil by weathering and decaying, and its long-term accumulation will result in serious damage and pollution to the soil. This means that the households with high soil quality requirements are cautious about employing this technology. Hypothesis 3 also illustrates that both the objective risk caused by external shocks and the subjective risk caused by incomplete information after relocation may have a significant impact on agricultural production decisions. Resettled households with weak resilience to potential risks to their livelihood, especially, are often forced to make careful trade-offs between low risk and high profit.
To test the robustness of our empirical results, one method was to construct another ordered-logit model (Model V) for the ordered variable of degree of resettlers’ green farming technology adoption to conduct a regression analysis. The other was to extract 80% of resettlers’ sample households and test them again through the ordered-probit model (Model VI). The results of both are shown in Table 8. As displayed in Table 8, there is essentially no difference in the sign (positive and negative values) of the regression coefficients for Models V and VI, or the significance level of the coefficients. This indicates the strong robustness of the hypotheses derived from the theoretical model, as well as the mechanisms of influence of the selected independent and control variables on the dependent variable.
Based on relevant theories, this study reveals the impact of resettled households’ e-commerce participation on their green technological innovations, determines the impact mechanisms, and conducts an empirical test. Our results summarize the results and experiences of modernizing involuntary among Three Gorges Reservoir area in China, which may provide a modellable case study for future rural revitalization in China. This may solve the sustainable livelihood problems of involuntary resettlers in other countries.
Due to the limited environmental capacity in the resettlement area, participation in social e-commerce among Reservoir resettlers is high, and it has gradually replaced the traditional agricultural cooperatives as the first choice to expand the distribution channels of agricultural products and generate family income. At the same time, although the adoption of green agrotechnology among resettlers is gradually expanding, adoption levels remain low to medium. Previous studies have mostly focused on the income-generating effects of e-commerce and the adoption factors and environmental effects of green agrotechnology (Takahashi and Muraoka 2019; Peng et al., 2021; Li et al., 2022), with less research on the interrelationship between the two, but the huge impact of e-commerce on agricultural production cannot be ignored. Our results show that e-commerce participation significantly increases the level of resettlers’ green agrotechnology adoption, shrinks the proportion of households with low adoption, and increases the proportion of resettled households with high adoption. This is similar to the findings of Li et al. (2021). Specifically, agricultural price expectations and related agrotechnology training support from e-commerce were the most important agrotechnology adoption drivers, and the former was more sensitive, indicating that resettlers urgently need to rely on green agricultural development to cope with an industrial hollowing-out in the Three Gorges Reservoir area due to natural capital loss and compensation resource constraints. However, green value-added agricultural products and e-commerce-assisted agrotechnical training mainly act on resettled households with low agrotechnical skills and, without the participation of e-commerce, will have limited impact effects.
Heterogeneous e-commerce participation models impact agrotechnology adoption differently. Many studies have shown that as e-commerce continues to develop, different e-commerce models, such as social e-commerce and platform e-commerce, are available (Luo 2022). Because the platform e-commerce model requires high household endowment, which is difficult for resettled households to afford, most such households choose the social e-commerce model. Chinese people value social relationships and are very good at using them (Zhang and Yang 2022). Resettled households share product information through social networks and word of mouth and can expand their product sales network more simply. Resettlers’ awareness and adoption of green farming techniques have increased under various e-commerce models. Of these, platform e-commerce participation brings the greatest degree of enhancement; the traditional e-commerce participation model has more intense market competition and consumers’ product requirements are higher (Heuer et al., 2015), which pushes resettled households to continuously improve the level of green agrotechnology adoption. In contrast, due to the limited network of resettled households’ acquaintances, the demand for green agrotechnology adoption driven by social e-commerce is lower, as is the degree of improvement it brings.
Related studies have shown that uncertainty in the application and use of technologies when new technologies are introduced will also cause uncertainty in income (Chavas and Shi 2015; Hörner and Wolln 2022). Involuntary resettlers have a tendency to avoid livelihood risks and fear their own lack of resilience, thus, if they perceive risks in technology application, they are likely to weaken the green agrotechnology promotion effect brought about by e-commerce participation. Theoretical improvements and innovations are often not convincing to farmers (Bozzola and Finger 2020), especially when the technical barriers to green agrotechnology application are high or the effectiveness of use is uncertain, which will lead to higher risk perceptions in technology adoption and thus make e-commerce resettlers cautious about green agrotechnology.
Some shortcomings must be acknowledged. First, the agrotechnology selected in this paper is citrus cultivation technology, and the significance of other agricultural products still needs to be verified. Second, we tested limited factors influencing green agrotechnology. Government regulation and incentives, the role of markets, and individual capabilities still need to be further verified. Third, the rho-squared value is not at a high degree, so the model in this paper can continue to be improved. Subsequent studies can improve on the above aspects.
Based on previous technology adoption theories, this paper constructs a theoretical model of green agrotechnology adoption by resettled households in the development of e-commerce in hydropower project reservoirs, taking into account the characteristics of Chinese involuntary project resettlers, the objective of maximizing expected returns, and the perceived risk to technology application posed by resettlers’ livelihood risk resistance. Drawing on survey data from resettled households in Zigui, the first county in the Three Gorges Reservoir area, the hypotheses derived from the theoretical model were tested empirically by using the ordered probit model. The conclusions are as follows: Green agrotechnology has become more popular among resettlers, but the overall adoption level is still low; participation in e-commerce has a significant positive impact on the adoption of green agrotechnology at the 1% level. The ecological value expectation of agricultural products and the agrotechnology support provided by e-commerce are the most important driving factors, but their current effects are mostly limited to resettled households with a low level of agrotechnology. Compared with the social e-commerce participation model, platform e-commerce brings more significant improvements in technology adoption. The risk perception in resettled households’ agrotechnology application weakens the promotion effect of e-commerce participation on agrotechnology adoption, while the risk perception of e-commerce participation weakens the promotion effect of the latter on the adoption of green agrotechnology.
Based on these findings, the following policy recommendations are proposed: 1) supporting fund should be used to improve network and logistics infrastructure. Through the combination of e-commerce policy and late-stage supporting system for resettlers, we can increase the participation rate of e-commerce and promote the application of green agricultural technology. 2) On the basis of the regional brand of the products in the resettled area, we embed the spirit of resettlers and create a special brand. On the one hand, it can realize the traceability of the whole growth cycle of agricultural products, and on the other hand, it can do the whole cycle of product development and expand a variety of products. This can enhance the agronomic value expectation of resettlers by adding value to agricultural products. 3) The government-led e-commerce associations in resettled areas should promote each other with the informal business organizations of resettlers formed by e-commerce platforms. It is necessary to provide green agrotechnology training more precisely. However, it is necessary to promote close interaction between resettlers and local residents through joint participation in e-commerce. Through small-scale technology demonstration and field guidance, the dissemination and exchange of tacit knowledge in the application of agricultural technology in resettler communities should be promoted. 4) To address the risk-averse tendency of reservoir resettlers in the application of green agricultural technology, the government of resettled areas should strengthen the publicity and popularize the knowledge of technical risks to avoid excessive precautionary behavior by resettlers. Meanwhile, the government should cooperate with insurance agencies to encourage farmers to purchase agricultural insurance policies. Such insurance policies are specifically used to share the technical costs incurred by resettler groups during the medium- and long-term growth periods of agricultural products and are incorporated into late-stage systems of risk sharing. This can reduce resettlers’ hesitancy to adopt green agrotechnologies.
The raw data supporting the conclusion of this article will be made available by the authors, without undue reservation.
Ethical review and approval was not required for the study on human participants in accordance with the local legislation and institutional requirements. Written informed consent from the participants was not required to participate in this study in accordance with the national legislation and the institutional requirements.
All authors contributed to the study conception and design. The first draft of the manuscript was written by ZC, XZ, and FZ. All authors commented on previous version of the manuscript. All authors read and approved the final manuscript.
This research was financially supported by National Natural Science Foundation of China: Livelihood Elasticity Measurement and Simulation Research on Later Supporting Strategies of Involuntary Resettlers from the Perspective of Habitat Mutation (Project Number: 72271142). The Major Program of National Social Foundation of China: Study on the governance and late-stage support of inter-county relocation communities (Project Number: 21&ZD183).
The authors declare that the research was conducted in the absence of any commercial or financial relationships that could be construed as a potential conflict of interest.
All claims expressed in this article are solely those of the authors and do not necessarily represent those of their affiliated organizations, or those of the publisher, the editors and the reviewers. Any product that may be evaluated in this article, or claim that may be made by its manufacturer, is not guaranteed or endorsed by the publisher.
Adnan, N., Nordin, M. D. H., and Anwar, A. (2021). Transition pathways for Malaysian paddy farmers to sustainable agricultural practices: An integrated exhibiting tactics to adopt green fertilizer. Land Use Policy 90 (6), 104255–108129. doi:10.1016/j.landusepol.2019.104255
Adnan, N., Nordin, S. M., and Rasli, A. M. (2019). A possible resolution of Malaysian sunset industry by green fertilizer technology: Factors affecting the adoption among paddy farmers. Environ. Sci. Pollut. Res. 26 (2), 27198–27224. doi:10.1007/s11356-019-05650-9
Bidogeza, J. C., Berentsen, P. B. M., De Graaff, J. A., and Oude Lansink, G. J. M. (2009). A typology of farm households for the Umutara Province in Rwanda. Food Secur. 1 (1), 321–335. doi:10.1007/s12571-009-0029-8
Bozzola, M., and Finger, R. (2020). Stability of risk attitude, agricultural policies and production shocks: Evidence from Italy. Eur. Rev. Agric. Econ. 48 (3), 477–501. doi:10.1093/erae/jbaa021
Chatzimichael, K., Genius, M., and Tzouvelekas, V. (2014). Informational cascades and technology adoption: Evidence from Greek and German organic growers. Food Policy 49 (1), 186–195. doi:10.1016/j.foodpol.2014.08.001
Chavas, J. P., and Nauges, C. (2020). Uncertainty, learning and technology adoption in agriculture. Appl. Econ. Perspect. Policy 42 (1), 42–53. doi:10.1002/aepp.13003
Chavas, J. P., and Shi, G. M. (2015). An economic analysis of risk, management and agricultural technology. J. Agric. Resour. Econ. 40 (1), 63–79.
Chen, X., Wu, Y. L., and Zhong, R. J. (2022). Optimal marketing channel and strategy in social commerce. RAIRO-Oper. Res. 56 (3), 1203–1221. doi:10.1051/ro/2022053
Cowan, B. W., Lee, D., and Shumway, C. R. (2015). The induced innovation hypothesis and U.S. public agricultural research. Am. J. Agric. Econ. 97 (3), 727–742. doi:10.1093/ajae/aau090
Dong, H., Wang, H., and Han, J. (2022). Understanding ecological agricultural technology adoption in China using an integrated technology acceptance model—theory of planned behavior model. Front. Environ. Sci. 10, 927668. doi:10.3389/fenvs.2022.927668
Dong, S., Yang, L., Shao, X., Zhong, Y., and Qiao, P. (2021). How can channel information strategy promote sales by combining ICT and blockchain? Evidence from the agricultural sector. J. Clean. Prod. 299 (22), 126857. doi:10.1016/j.jclepro.2021.126857
Duong, T. T., Tom, B., Jo, L., and Kerstin, Z. (2019). A global review of farmers’ perceptions of agricultural risks and risk management Strategies. Agriculture 9 (1), 10. doi:10.3390/agriculture9010010
Fang, L., Hu, R., Mao, H., and Chen, S. (2021). How crop insurance influences agricultural green total factor productivity: Evidence from Chinese farmers. J. Clean. Prod. 321 (7), 128977. doi:10.1016/j.jclepro.2021.128977
Fu, W. Q., and Zhang, R. W. (2022). Can digitalization levels affect agricultural total factor productivity? Evidence from China. Front. Sustain. Food Syst. 6, 860780. doi:10.3389/fsufs.2022.860780
Gao, Y., and Niu, Z. H. (2019). Risk aversion, information acquisition ability and farmers’ adoption behavior of green control techniques. Chin. Rural. Econ. (8), 109–127.
Gong, Y. C., Zhang, R. L., Yao, K. W., Liu, B. W., and Wang, F. L. (2020). A livelihood resilience measurement framework for dam-induced displacement and resettlement. Water 12 (11), 3191. doi:10.3390/w12113191
Heuer, D., Brettel, M., and Kemper, J. (2015). Brand competition in fashion e-commerce. Electron. Commer. Res. Appl. 14 (6), 514–522. doi:10.1016/j.elerap.2015.07.007
Hörner, D., and Wolln, M. (2022). Does integrated soil fertility management increase returns to land and labor? Agric. Econ. 53 (3), 337–355. doi:10.1111/agec.12699
Karimi, S., and Taifur, W. D. (2013). Resettlement and development: A survey of two of Indonesia's koto panjang resettlement villages. Int. J. Water Resour. Dev. 29 (1), 35–49. doi:10.1080/07900627.2012.739539
Koundouri, P., Nauges, C., and Tzouvelekas, V. (2006). Technology adoption under production uncertainty: Theory and application to irrigation technology. Am. J. Agric. Econ. 88 (3), 657–670. doi:10.1111/j.1467-8276.2006.00886.x
Li, B., Xu, C., Zhu, Z., and Kong, F. (2021). Does e-commerce drive rural households engaged in non-timber forest product operations to adopt green production behaviors? J. Clean. Prod. 320, 128855. doi:10.1016/j.jclepro.2021.128855
Li, H., Tang, M., Cao, A., and Guo, L. (2022). Assessing the relationship between air pollution, agricultural insurance, and agricultural green total factor productivity: Evidence from China. Environ. Sci. Pollut. Res. Int. doi:10.1007/s11356-022-21287-7
Li, X. J., Chen, Z., Liu, F., and Xia, X. L. (2020). Does participating in E-commerce promote the adoption of green production technologies by kiwifruit growers? A counterfactual estimation based on propensity score matching method. Chin. Rural. Econ. (3), 118–135.
Liu, Y. Y., Shi, R. L., Peng, Y. T., Wang, W., and Fu, X. H. (2022). Impacts of technology training provided by agricultural cooperatives on farmers’ adoption of biopesticides in China. Agriculture 12 (3), 316. doi:10.3390/agriculture12030316
Lu, Y. H., Chen, S. J., and Li, X. M. (2018). Relationship poverty: The fracture and reconstruction of immigrant social relations network: Take S village of resettled in danjiangkou reservoir for example. China Agric. Univ. J. Soc. Sci. Ed. 35 (2), 114–122. doi:10.13240/j.cnki.caujsse.2018.02.025
Luo, N. (2022). Innovation of E-commerce development model under the background of artificial intelligence and wireless communication. Wirel. Commun. Mob. Comput., 2022, 8572911–8572917. doi:10.1155/2022/8572911
Natkhov, T., and Vasilenok, N. (2021). Skilled immigrants and technology adoption: Evidence from the German settlements in the Russian empire. Explor. Econ. Hist. 81, 101399. doi:10.1016/j.eeh.2021.101399
Ni, J., and Shao, J. (2013). The drivers of land use change in the migration area, Three Gorges Project, China: Advances and prospects. J. Earth Sci. 24 (1), 136–144. doi:10.1007/s12583-013-0306-5
Peng, C., Biao, M. A., and Zhang, C. (2021). Poverty alleviation through e-commerce: Village involvement and demonstration policies in rural China. J. Integr. Agric. 20 (4), 998–1011. doi:10.1016/S2095-3119(20)63422-0
Qiu, H. G., Su, L. F., Zhang, Y. T., and Tang, J. J. (2020). Risk preference, risk perception and farmers’ adoption of conservation tillage. Chin. Rural. Econ. (7), 59–79.
Takahashi, K., Muraoka, R., and Otsuka, K. (2019). Technology adoption, impact, and extension in developing countries' agriculture: A review of the recent literature. Agric. Econ. 51 (1), 31–45. doi:10.1111/agec.12539
Tian, T., Zhang, Y., and Mei, Y. (2022). Intelligent analysis of precision marketing of green agricultural products based on big data and GIS. Earth Sci. Inf. 15, 1395–1406. doi:10.1007/s12145-022-00789-5
Wang, W. K., Wang, J., Liu, K. B., and Wu, Y. J. (2020). Overcoming barriers to agriculture green technology diffusion through stakeholders in China: A social network analysis. Int. J. Environ. Res. Public Health 17 (19), 6976–76. doi:10.3390/ijerph17196976
Wu, Q., and Zhou, J. (2021). Need for cognitive closure, information acquisition and adoption of green prevention and control technology. Ecol. Chem. Eng. S 28 (1), 129–143. doi:10.2478/eces-2021-0011
Xiao, J., Wang, W., and Tsai, S. (2021). Coupling of agricultural product marketing and agricultural economic development based on big data analysis and “Internet+”. Mob. Inf. Syst., 2021, 1–10. doi:10.1155/2021/3702064
Yan, D. C., Wang, M., Wang, H. B., and Shi, G. Q. (2018). Policy and implementation of land-based resettlement in China (1949–2014). Int. J. Water Resour. Dev. 34 (3), 453–471. doi:10.1080/07900627.2017.1417824
Yin, Z. H., and Choi, C. H. (2022). Does e-commerce narrow the urban–rural income gap? Evidence from Chinese provinces. Internet Res. 32 (4), 1427–1452. doi:10.1108/INTR-04-2021-0227
Zeng, Y. M., Zhang, J. B., He, K., and Cheng, L. L. (2019). Who cares what parents think or do? Observational learning and experience-based learning through communication in rice farmers’ willingness to adopt sustainable agricultural technologies in Hubei province, China. Environ. Sci. Pollut. Res. 26 (12), 12522–12536. doi:10.1007/s11356-019-04609-0
Zhang, Q., and Yang, J. (2022). Model construction of hierarchical polarization characteristics combined with social E-commerce consumer behavior. Secur. Commun. Netw. 2022, 9666677. doi:10.1155/2022/9666677
Zhang, Y. (2021). Understanding China's dam-induced resettlement under the institutionalised governance process of policy coevolution. J. Environ. Manag. 283 (7), 111968. doi:10.1016/j.jenvman.2021.111968
Keywords: e-commerce participation, green agrotechnology adoption, risk perception, reservoir resettlers, ordered-probit model
Citation: Zhao X, Cui Z and Zhao F (2022) Does e-commerce participation affect green agrotechnology adoption among reservoir resettlers? The case of China’s Three Gorges Reservoir area. Front. Environ. Sci. 10:1036464. doi: 10.3389/fenvs.2022.1036464
Received: 04 September 2022; Accepted: 05 October 2022;
Published: 17 October 2022.
Edited by:
Aaron Kinyu Hoshide, University of Maine, United StatesReviewed by:
Muhammad Asad Ur Rehman Naseer, Bahauddin Zakariya University, PakistanCopyright © 2022 Zhao, Cui and Zhao. This is an open-access article distributed under the terms of the Creative Commons Attribution License (CC BY). The use, distribution or reproduction in other forums is permitted, provided the original author(s) and the copyright owner(s) are credited and that the original publication in this journal is cited, in accordance with accepted academic practice. No use, distribution or reproduction is permitted which does not comply with these terms.
*Correspondence: Xu Zhao, emhhb3h1QGN0Z3UuZWR1LmNu
Disclaimer: All claims expressed in this article are solely those of the authors and do not necessarily represent those of their affiliated organizations, or those of the publisher, the editors and the reviewers. Any product that may be evaluated in this article or claim that may be made by its manufacturer is not guaranteed or endorsed by the publisher.
Research integrity at Frontiers
Learn more about the work of our research integrity team to safeguard the quality of each article we publish.