- 1School of Water Resources and Electric Power, Key Laboratory of Ecological Protection and High Quality Development in the Upper Yellow River, Key Laboratory of Water Ecological Remediation and Protection at Headwater Regions of Big Rivers, Ministry of Water Resources, Qinghai University, Xining, Qinghai, China
- 2State Key Laboratory of Plateau Ecology and Agriculture, Qinghai University, Xining, Qinghai, China
- 3State Key Laboratory of Hydroscience and Engineering, Department of Hydraulic Engineering, Tsinghua University, Beijing, China
- 4College of Environmental Sciences and Engineering, Peking University, The Key Laboratory of Water and Sediment Sciences, Ministry of Education, Beijing, China
We analyze bacterial composition, diversity, geographical distribution, and their community networks in lake water in three adjacent regions on the Qinghai-Tibet Plateau (QTP). Results show that bacterial alpha-diversity indices are much lower in the Hoh Xil (HX) than that in the Yellow River Headwater (YRH) regions and the Qaidam (QD) region. The dominant phyla in QD and YRH are Proteobacteria which account for 42.45 % and 43.64 % of all detected phyla, while Bacteroidetes is the dominant bacterial taxa in HX (46.07 %). Redundancy analysis results suggest that the most important factors in driving bacterial community composition in the three regions are altitude (QD), total nitrogen (YRH), and pH (HX), respectively. Both environmental factors and spatial factors significantly affect the bacterial community composition in QD and HX, while only environmental factors are the major drivers in YRH. Finally, network analyses reveal that the bacterial network structure in QD is more complex than those in YRH and HX, whereas the bacterial network in HX is the most stable, followed by those in QD and YRH.
Introduction
The Qinghai-Tibet Plateau (QTP), considered as “the Third Pole”, is located in the northwest of China with an average elevation of 3000 m. The climate is arid/semi-arid with a mean annual precipitation less than 400 mm and an average annual air temperature lower than 5°C (Liu et al., 2021). The lake ecosystem at the QTP is the largest and one of the most representative oligotrophic and mesotrophic lake ecosystems in the world (Wen et al., 2019). Large variations in water chemistry occur in the plateau lakes due to incomplete water mixing over multi-year intervals (Wen et al., 2019). Numerous bacteria are found in lake water bodies, which are vital parts of the aquatic ecosystem and play important functional roles in nutrient and energy cycling in the lakes (Zinger et al., 2011; Chen et al., 2019; Zhang et al., 2020b; Wang et al., 2020; Xie et al., 2020). For example, denitrifying bacteria and anammox bacteria are related to nitrogen loss in aquatic ecosystems (Wang et al., 2019). Many studies have been conducted on the bacterial community harbored in the surface water in areas of the QTP and it has been found that the bacterial communities in these lakes are highly sensitive to environmental differences such as salinity, elevation and human activities (Wu et al., 2006; Xiong et al., 2012; Yang et al., 2016a; Liu et al., 2019c; Zhong et al., 2016; Ji et al., 2019; Liu et al., 2021). For example, recent studies have reported that water salinity is a major driving force in shaping community structure of bacteria in the Tibetan lakes (Xing et al., 2009; Liu et al., 2019a; Liu et al., 2020).
The Qaidam lake and the Qinghai lake, with hypersaline (>35%) and saline water, a relatively low elevation (∼3000 m), affected by intensive human activities, are located at a gigantic inland river region on the QTP (Supplementary Figure S1), surrounded by the Kunlun, Riyue, and Qilian Mountains (Zhong et al., 2016). Several studies have reported the bacterial community structure and its influencing factors in lake water bodies in the Qaidam region (QD) (Jiang et al., 2010; Wang et al., 2011; Yang et al., 2016b; Han et al., 2017; Ren et al., 2017). A previous study reported that salinity, pH, dissolved oxygen concentration and total nitrogen concentration are the most important environmental factors that affect the prokaryotic community in these lakes (Yang 2015).
The Yellow River Headwater region (YRH, Supplementary Figure S1) has freshwater (salinity <1%), an average elevation of 4500 m (Luo et al., 2020) and less disturbance from human activities. Only two studies have reported bacterioplankton in lake waters within YRH. Previous research found that the bacterial communities in water have a significant correlation with water conductivity, total nitrogen, and total phosphorus in the thermokarst lakes from the YRH region (Ren et al., 2021a).
One study has been undertaken to investigate the response of archaea to climate change in Kusai lake at the northern Hoh Xil region (HX, Supplementary Figure S1), which has an intermediate salinity (1% < salinity <35%) and an average elevation of 4500 m (Yang 2015; Liu et al., 2019b).
These three neighboring regions, while being close geographically, are characterized by significantly different environmental conditions, providing an opportunity to study the differences of bacterial communities and their driving forces in these three typical habitats (Li et al., 2020). In this study, we systematically examine the bacterial diversity and community composition, their geographical distributions, and co-occurrence network in the three regions using 16S rRNA gene sequencing. The objectives of our investigation are: 1) to analyze the differences in bacterial diversity, community structure, and biomarkers in the lakes in the three distinct regions, 2) to identify contributions of environmental and spatial factors to variations of bacterial community composition in the three regions, and 3) to reveal bacterial co-occurrence patterns in each region. The results of this study provide insight into differences of bacterial features at the regional scale, and provide guidance for the protection and management of the plateau lake ecosystems.
Materials and methods
Sampling and processing
About 5L surface (top 0.5 m) water samples were obtained in three replicates at each sampling point of the 19 investigated lakes including six lakes in QD, seven lakes in YRH, and six lakes in HX in June 2020. For larger lakes more than two sampling points were chosen at different functional (i.e., central-C/shore-S, or inlet/outlet areas) zones (Figure 1 and Supplementary Table S1). The lakes were selected to cover most of the major lakes in each region. Water samples were saved in sterile narrow-necked glass bottles and immediately kept in a portable refrigerator. After mixing the 5L of water from each sampling point, about 3L of water sample was filtered through 0.22 μm pore polycarbonate membrane (47 mm, Millipore S-Pak, Germany) using a vacuum pump within 12 h. Filters were transferred into 50 ml sterile conical centrifuge tubs, and then stored at −20°C in a portable refrigerator until transported back to our laboratory for total DNA extraction. The residual water samples were saved at dark and cold conditions at 4°C until they were used for physicochemical parameter analyses.
Water sample analysis
At each sample site, water temperature (Temp), pH, dissolved oxygen (DO), salinity (SAL), electrical conductivity (EC), oxidation-reduction potential (ORP), turbidity (NTU), and Chlorophyll-a concentration (Chl-a) were measured in-situ using a portable multi-parameter water quality monitor (EXO™2 Sonde, SY1, United States). Additionally, total nitrogen (TN), ammonia nitrogen (NH4+-N), nitrate nitrogen (NO3−-N), nitrite nitrogen (NO2−-N), and total phosphorus (TP) of the water samples were analyzed using standard methods as described in previous studies (Zhao et al., 2017; Meng et al., 2020). After filtered through a 0.45 μm membrane, concentrations of dissolved organic carbon (DOC) in water samples were measured according to the Chinese National Standard HJ 501-2009 by a TOC elemental analyzer (TOC-L, Shimadzu, Japan). Geospatial parameters, such as longitude, latitude, and altitude were recorded with a portable global position system where water samples were collected (GPS-China), which were used to calculate the Euclidean distance between sampled sites for analysis of the spatial patterns (Mora-Ruiz et al., 2018). All these detected environmental parameters were measured three times and the average values were used for subsequent analysis.
DNA extraction, 16S rRNA genes amplification and sequence bioinformatics analysis
The total DNA information of microbial community in each water sample was extracted using the CTAB methods (Kubo et al., 2014). DNA was purified using 1% (w/v) agarose gel electrophoresis for further amplification reactions. The fragment of V4 region of bacterial 16S rRNA genes were amplified using the universal primers 515F (5′-GTGCCAGCMGCCGCGGTTA-3′) and 806R (5′-GGACTACHVGGGTWTCTAAT-3′) (Caporaso et al., 2010). All DNA PCR reactions were carried out in a 50 μl reaction volume, containing 25 μl of 2x Taq PCR mix, 3 μl Primer F (10 μM), 3 μl Primer FR (10 μM), 3 μl diluted DNA and 16 μl double distilled H2O. The cycling processes include pre-denaturation for 60 s at 98°C, followed by 34 cycles of denaturation at 94°C for 60 s, annealing at 57°C for 45 s, extension at 72°C for 60 s, and a final extension at 72°C for 10 min. After the amplification reactions, PCR products were checked in 2% (w/v) agarose gel by electrophoresis and purified using AxyPrep DNA gel extraction Kit (AxyGEN, Union City, CA, United States). Amplicons were stored at −20°C and sequenced on an Illumina HiSeq platform using the 2 bp × 250 bp paired-end read protocol at Novogene bioinformatics technology Co., Ltd. (Beijing, China). The raw sequencing data of each sample were trimmed and merged using Trimmomatic (v.0.33) and FLASH (v1.2.7, http://ccb.jhu.edu/software/FLASH/) (Wang et al., 2021). The low-quality sequencing data with average quality scores less than 20 and with a sequence length less than 75% of the tag length were removed from the obtained raw sequence. Subsequently, the corrected sequences were arranged into the same operational taxonomic units (OTUs) at a 3% threshold of sequence dissimilarity level (OTU0.03) using UPARSE (v7.0.1001, http://www.drive5.com/uparse/) and the singletons were removed. The effective sequences for each OTU were packed for annotating corresponding taxonomic information using the SILVA v138 high quality ribosomal RNA database by the ribosomal data project (RDP) classifiers (threshold 0.8) using the “cluster” command in MOTHUR (Zhao et al., 2016; Jiao et al., 2020). The raw sequencing data were submitted to the NCBI (http://www.ncbi.nlm.nih.gov) Sequence Read Archive under Bioproject number PRJNA728144.
Statistics analysis
Non-parametric (Kruskal-Wallis) test was employed to test the difference of physicochemical parameters of water. Before performing sequence statistical analysis, the number of sequences was normalized by the sample with the smallest number (60779) of sequences (Zhong et al., 2016). Hill numbers including Richness index, Shannon index, Simpson index and Pielou evenness index were calculated to evaluate the α-diversities of bacterial communities in each geographical region (QD, YRH, and HX) using the packages of “hilldiv” (Hill 1973; Chao et al., 2010). The Mann-Whitney U test was used to examine the difference of alpha diversity between any two regions and the results of p-value < 0.05 were considered as significant (Li et al., 2019). The rarefaction curves were used to identify the sampling depth of a community, and to evaluate the reliability of sequences data of each water sample (Mania et al., 2019). The Pearson’s and Spearman’s correlations between environmental factors and alpha-diversity indices and relative abundances of top 10 phyla in the bacterial community in the three regions were calculated respectively using the “vegan” packages.
Principal coordinate analysis (PCoA) and permutational multivariate analysis of variance (PERMANOVA) were performed to reveal the regional differences in bacterial community structures based on the Bray-Curtis distance (Xia et al., 2020). We used permutation multivariate dispersion (PERMDISP) to test the dispersions of the data (Banerjee et al., 2019; Cadena et al., 2019). The taxa of the water bacterial biomarkers were identified using linear discriminant analysis (LDA) and the effect size algorithm (LEfSe) in the Novogene analysis platform. Variance inflation factors (VIFs) were used to identify the multi-collinearity among abiotic factors. Based on the results of calculated detrended correspondence analysis (DCA), the abiotic factors with VIFs values less than 10 were selected for redundancy analysis (RDA) to investigate the relationship between environmental factors and bacterial community composition (Zeng et al., 2019). Variation partitioning analysis (VPA) was used to evaluate the importance of water physicochemical and geographical factors in determining bacterial community composition with spatial factors generated using the principal coordinates of neighbor matrices (PCNM) analysis (Liu et al., 2020). Furthermore, Mantel and partial Mantel tests with 999 permutations were performed to test the contributions of water physicochemical/geospatial factors to the bacterial community composition variability in each region (Li et al., 2019).The geographic distances and the Bray-Curtis similarity of bacterial community for two pairing sampling points were calculated to reveal the distance decay patterns of bacterial community structures in the three regions and all regions using the “geosphere” and “vegan” packages (Zeng et al., 2019).
For bacterial networks, only OTUs with average of relative abundance larger than 0.01% that exist in at least 50% of all samples in each region were chosen to construct the bacterial network (Zeng et al., 2019). Furthermore, the relationships between OTUs with Spearman’s correlation coefficient r > 0.8 or r < −0.8 and Benjamini-Hochberg p < 0.01 were selected for network construction (Zhao et al., 2016; Ji et al., 2019; Ren et al., 2021b). Networks were analyzed and visualized using the “igraph” package and Gephi platform (https://gephi.org/) (Zhou et al., 2019). Mean degree, clustering coefficient, modularity, average path length, network diameter, graph density, and robustness were calculated to describe topological characteristic of the networks. The nodes in networks represent OTUs and the edge represent relationships between two OTUs. Mean degree is the average number of connected edges of each node (Jiao et al., 2020). Clustering coefficient refers to the degree of nodes which tend to cluster together in network. Modularity is the degree that a network can be divided into modules. A modularity value larger than 0.4 indicates that network have a modular structure (Jiao et al., 2020; Tu et al., 2020). Average path length and network diameter are the mean of the length of the shortest path and the largest distance between any two nodes in a network, respectively (Banerjee et al., 2019; Jiao et al., 2020). Graph density is the ratio of the edge number over the possible edge number (Jiao et al., 2020). Robustness and vulnerability are estimated to reflected network stability (representing the ability of network to resist external interference) by randomly removing 50% of nodes and the nodes with maximal vulnerability in each region (Chen and Wen 2021; Liu et al., 2022).
We used among module connectivity (Pi) and within module connectivity (Zi) plot to reveal the topological roles of network nodes (Jiao et al., 2020). According to the values of Zi and Pi, all OTUs in network could be divided into peripherals (Pi ≤ 0.62; Zi ≤ 2.5), connectors (nodes in networks connecting different modules, Pi > 0.62; Zi ≤ 2.5), module hubs (highly connected nodes in modules, Pi ≤ 0.62; Zi > 2.5), and network hubs (highly connected nodes in network, Pi > 0.62; Zi > 2.5) (Deng et al., 2012; Li et al., 2021). Network hubs, module hubs, and connectors are defined as keystones in network, so OTUs associated with these nodes are considered as the keystone species in the bacterial community (Olesen et al., 2007). All the above analyses are calculated in R (v. 4.1.3) except the LEfSe analysis.
Results
Physicochemical properties of lake water in the three regions
The values of fifteen physicochemical factors for all water samples in the three regions were summarized in Table 1. Significant differences in water physicochemical parameters were observed in the three regions. Specially, Temp, EC, ORP, and TDS were significantly higher in QD compared to those in YRH and HX (p < 0.05), while no significant differences of pH, DO, and Turb were found in the three regions (p > 0.05). Moreover, DOC content in QD varied over a wide range from 0.85 to 22.66 mg/L, but less so in YRH (1.31–7.35 mg/L) and HX (0.83–5.05 mg/L). Mean concentrations of TN and TP showed remarkable differences among the three regions (p < 0.001). In summary, environmental parameters suggested a large habitat heterogeneity across the three regions.
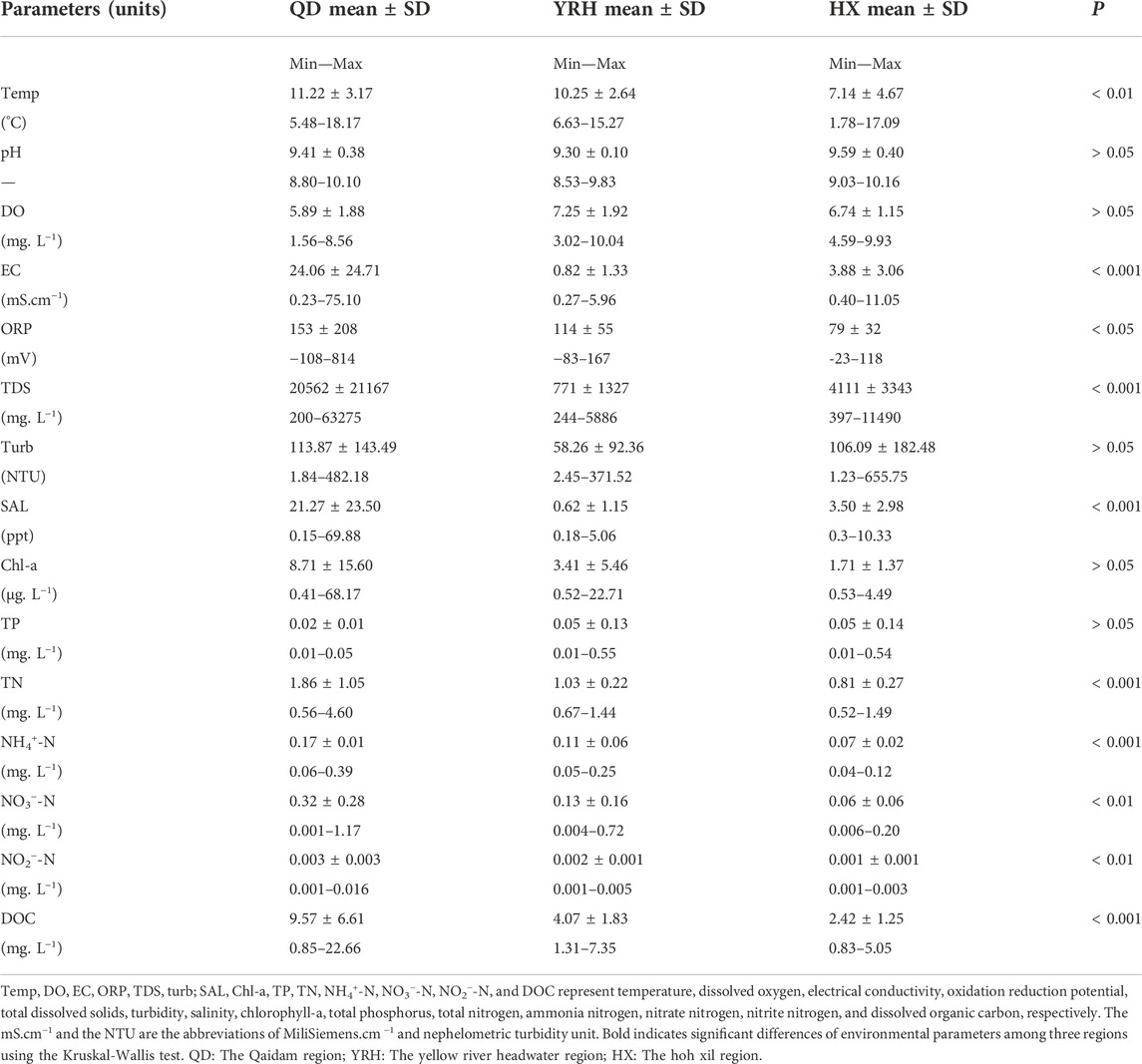
TABLE 1. Water physicochemical parameters of three regional groups on the QTP in July 2020. The data represent the mean values of samples in each regional group with standard deviation.
Bacterial diversity and composition in the three investigated regions
Analysis of rarefaction curves confirmed that the samples were able to represent the species of each lake (Supplementary Figure S2A) with the observed species numbers reach plateaus. After checking and removing the low-quality sequences, a total number of 4,843,690 effective reads were obtained from all water samples in the three regions. These effective reads were clustered into a total of 20,222 OTUs distributed among 83 phyla. As shown in Supplementary Figure S2B, the total number of OTUs for QD, YRH, and HX regions were 9,594, 6,868, and 3,760, respectively. According to Supplementary Figure S2B, the proportion of shared OTUs numbers was 13.28% in the three investigated regions. The proportions of shared OTUs numbers were 26.07% between QD and YRH, 22.57% between QD and HX, and 29.71% between YRH and HX, respectively. The proportions of unique OTUs were 24.60% in QD, 10.41% in YRH, and 1.35% in HX.
Comparison analysis of four alpha diversity indices in the three regions, including the Hill Richness, Hill Shannon index, Hill Simpson index and Pielou’s evenness index, were presented in Figure 2. The highest Hill Shannon index, Hill Simpson index, and Pielou’s evenness index indicated high diversity and even distribution of the bacterial community in YRH. The highest Hill Richness reflected high abundance of bacterial in QD. The four alpha diversity indices of bacterial community were the lowest in HX (Figure 2). The indices for the bacterial community in HX were significantly lower than those in QD and YRH except the Hill Simpson index (Mann-Whitney U test p < 0.05). The four alpha diversity indices in QD and YRH did not have significant differences (Mann-Whitney U test p > 0.05).
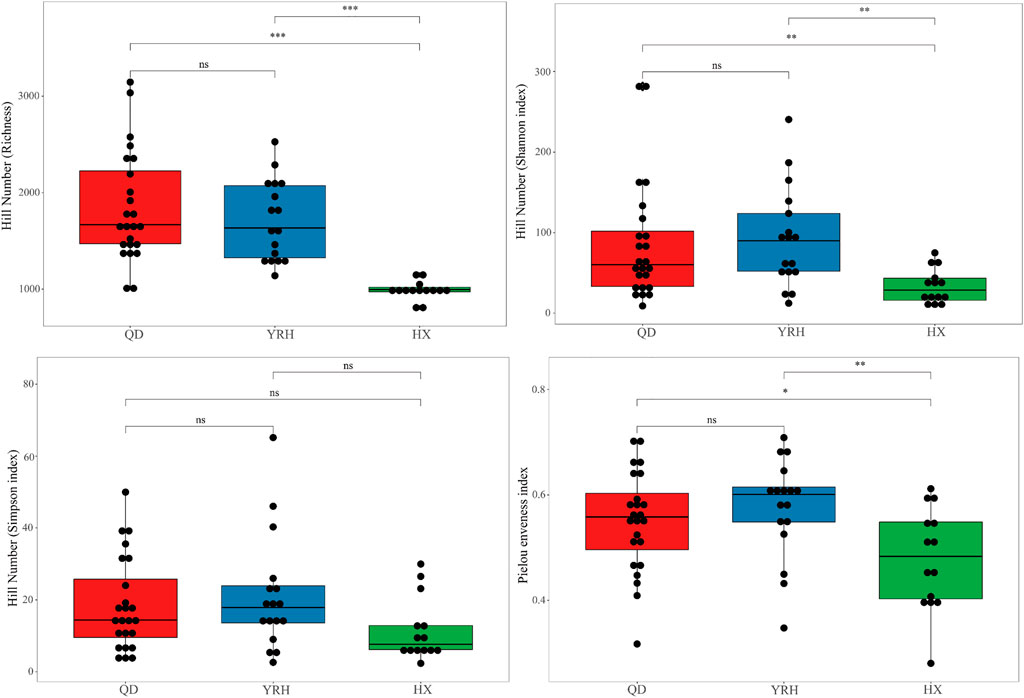
FIGURE 2. Boxplots showing the comparisons of alpha-diversity indices based on Hill numbers in the three regions. *, **, and *** represent the significant level of p < 0.05, p < 0.01, and p < 0.001 using Maun-Whitney U test., respectively. The ns indicates no significant difference in two regions.
The top 10 phyla consist of Bacteroidetes (mean relative abundance, QD = 21.12%, YRH = 19.46%, and HX = 46.06%), Proteobacteria (QD = 42.45%, YRH = 43.64%, and HX = 38.13%), Cyanobacteria (QD = 3.5%, YRH = 20.57%, and HX = 4.47%), Firmicutes (QD = 12.13%, YRH = 1.24%, and HX = 0.99%), Actinobacteria (QD = 15.07%, YRH = 8.08%, and HX = 8.69%), Verrucomicrobia (QD = 1.27%, YRH = 3.48%, and HX = 1.04%), Planctomycetes (QD = 0.59%, YRH = 1.29%, and HX = 0.16%), Acidobacteria (QD = 0.41%, YRH = 0.61%, and HX = 0.08%), Chloroflexi (QD = 0.78%, YRH = 0.57%, and HX = 0.09%), and Gemmatimonadetes (QD = 0.28%, YRH = 0.25%, and HX = 0.05%) (Supplementary Figure S3A). Proteobacteria was the dominated phylum in QD and YRH, while Bacteroidetes was the dominated phylum in HX. Meanwhile, bacterial communities also exhibited compositional differences at different sample points within the same lake. For example, the dominant phylum at QHL4 (sampling point 4 of the Qinghai lake) was Firmicutes (64.36%), but was Proteobacteria (42.62%) at QHL6 (Supplementary Figure S4), which suggested that the distributions of bacteria was heterogeneous in larger lakes.
The results of PCoA indicated that bacterial community compositions tend to cluster together in each region and the first two axes of the PCoA contributed 19.06% and 12.78% of the variance observed among three regions. PERMANOVA results also supported this observation and confirmed the significant differences of bacterial community compositions among three regions (Figure 3). Pairwise comparison with PERMDISP indicated that bacterial community compositions of lakes were significantly different between QD and YRH (F = 16.25, p = 0.001), and QD and HX (F = 11.15, p = 0.004). It is suggested that bacterial community in QD had different spatial dispersion compared with YRH and HX. However, there were insignificant difference between YRH and HX (F = 1.24, p = 0.282), indicating homogenous dispersion of bacterial community composition in these two regions.
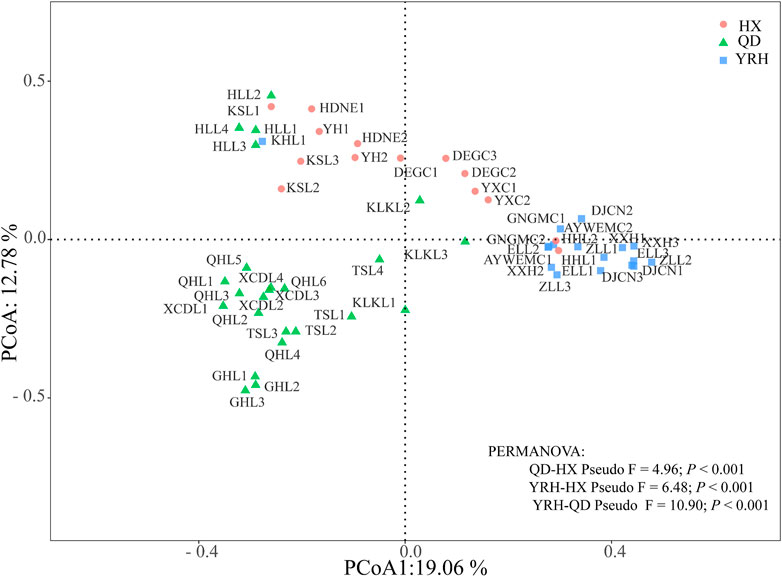
FIGURE 3. Principal coordinate analysis (PCoA) showing the compositional difference of bacteria community in the three regions based on the OTU level.
Four phyla, eleven orders and nine families were screened out across all water samples for determining the indicator and biomarkers of bacterial communities using the LEfSe algorithm (Figure 4A). At the phylum level, Firmicute was found as the indicator in QD, Cyanobacteria and Verrucomicrobia were two phyla indicators in YRH, and Bacteroidetes was the indicator in HX. There are 10 biomarkers (taxonomic) in YRH, 14 biomarkers in QD, and 19 biomarkers in HX (i.e., colored circles in Figure 4A) with significantly different abundances identified (Figure 4B).
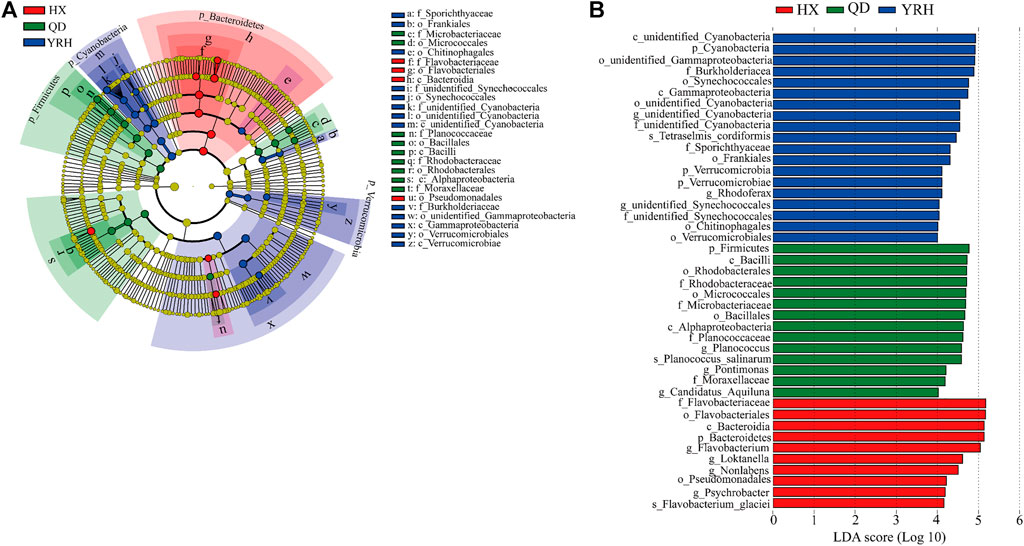
FIGURE 4. (A): Taxa cladogram comparison of bacterial communities in the three regions. (The circles from innermost to outermost represent the phylum level to the species level); (B) Linear discriminant analysis (LDA) of effective size of bacterial communities in the three regions.
Effects of environmental parameters on bacterial diversity and composition in the three regions
Pearson’s correlations between bacterial alpha diversity indices and environmental variables of sampled lakes for all three regions were calculated as shown in Supplementary Figure S5. For all three regions, bacterial diversity and richness had negative correlations with altitude and pH (p < 0.01), but positive relationships with longitude, Temp, ORP, TN, DOC, and NH4+-N (p < 0.05). The environmental variables influencing bacterial diversity indices in each region were different. Latitude, DO, and altitude had significant negative relationship with bacterial richness and diversity, whereas Temp, ORP, Chl-a, and DOC showed positive relationship with bacterial richness and diversity in QD. In YRH, longitude, altitude, EC, TDS, and SAL had significant influences on bacterial diversity and richness. DO, EC, TDS, SAL, pH, and Chl-a were the significant factors in affecting bacterial diversity and richness in HX.
Results of redundancy analysis revealed that 40.94%, 52.92%, and 73.84% of the total variability of bacterial community in QD, YRH, and HX were explained by the first two principal components (RDA1 and RDA2, Supplementary Figure S7). Among all environmental factors assessed, altitude (envfit test: r2 = 0.513, p = 0.003), TN (envfit test: r2 = 0.436, p = 0.021), and pH (envfit test: r2 = 0.702, p = 0.003) had the greatest impact on community structure of bacterial in QD, YRH, and HX, respectively (Supplementary Figure S7). Combining water samples from all of the three regions, the first two principal components in RDA explained 37.59% of the overall variability of bacterial community structure and altitude had the greatest influence on the bacterial community structure (envfit test: r2 = 0.702, p = 0.001). To decouple the effects of geographical factors and water physicochemical factors on water bacterial compositions, variance partition analysis (VPA) was performed at the OTU level. The results showed that physicochemical properties of water had larger effects on water bacterial abundances (30.20%) compared to geographical factors (14.04%, Figure 5). Additionally, Mantel and partial Mantel tests confirmed that the bacterial community composition in QD and HX regions were related to both geographical factors (QD: r = 0.221; p = 0.018 and HX: r = 0.505; p = 0.001) and water physicochemical factors (QD: r = 0.420; p = 0.001 and HX: r = 0.536; p = 0.001, Supplementary Table S2). However, the geographical factors showed insignificant impact on bacterial community structure in YRH (Supplementary Table S2), which might be due to the small geographical range of the sampled lakes in this region. It was worth noting that the contribution of uncertain factors reached 43.36%, implying that some unmeasured environmental factors might be vital as well in affecting the bacterial community composition of the lakes (Williams 1998; Chakraborty et al., 2020; Gu et al., 2020; Liu et al., 2021).
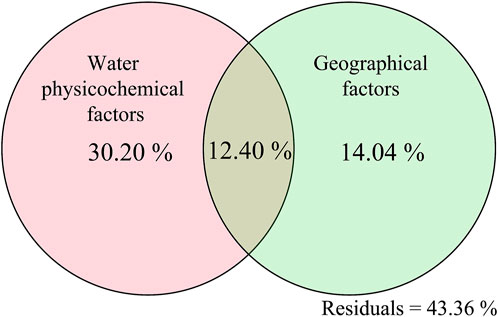
FIGURE 5. Variation partition analysis (VPA) diagram of geographical factors and water physicochemical factors in determining bacterial composition variations.
Distance decay patterns of bacterial community are the results of environmental filtering (environmental factors) and dispersal limitation (geographic factors). In general, the slope of the distance decay relationship can reflect the turnover rate of bacterial community spatially. Our results showed that the Bray-Curtis similarity of bacterial communities in each region decayed with geographic distance. This suggested that the bacterial community was not randomly dispersed in each region. The slope in HX was the steepest compared to those in QD and YRH (Figure 6), which indicated that there was the highest turnover rate of bacterial communities in HX.
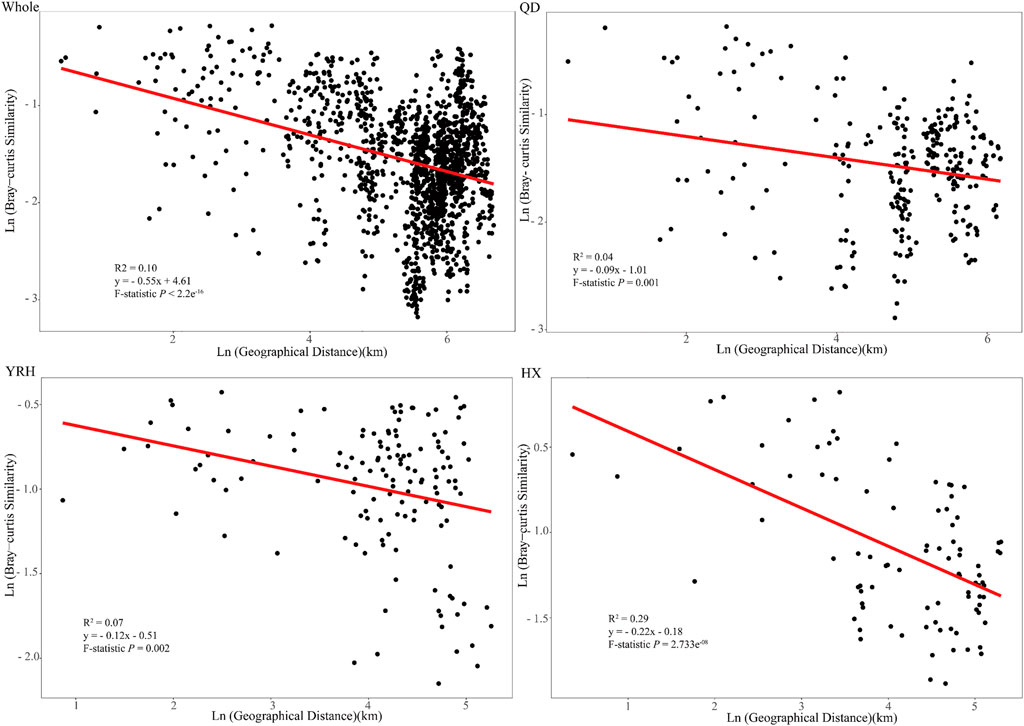
FIGURE 6. The relationship between bacterial community similarity (Bray-Curtis) and geographic distance (Euclidean distance) between any two sampling sites in each region.
Bacterial networks in the three regions
To explore the relationship among OTUs, we constructed networks in each region using species-species correlations and calculated characteristic values of network topology, as shown in Figure 7 and Supplementary Table S3. Bacterial networks in the three regions consisted of 196 nodes and 812 edges (QD), 135 nodes and 421 edges (YRH), and 102 nodes and 329 edges (HX), respectively (Figure 7). Overall, there were more positive relationships than negative ones in each region, which suggested that taxa tended to co-occur rather than exclude each other. Zi-Pi plots showed that Firmicutes and Bacteroidetes phyla were the keystone taxa (module hubs) in QD and HX, but the nodes in YRH did not reach the threshold for module hubs. There were 29, 20, and 20 connectors in QD, YRH, and HX.
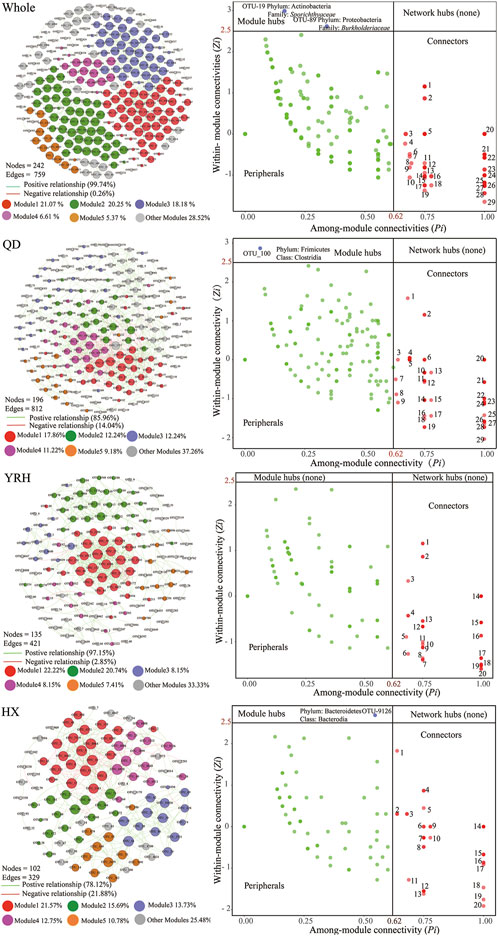
FIGURE 7. The co-occurrence networks among OTUs (left panel) and Zi-Pi plot showing the distribution of OTUs (right panel) in the whole and the three regions based on Spearman’s rank correlations (r > 0.8 or r < −0.8 p < 0.01) and their topological roles, respectively. The size of each node is proportional to the number of connections (i.e., degree), and the nodes are colored according to different types of modularity classes. Each node represents an OTU. Edges with green or red colors represent positive or negative relationship between any two OTUs.
Furthermore, the results demonstrated different topological characteristics among the three regions and the network in QD was more complex than those in YRH and HX (represented by numbers of nodes and edges) (Wang et al., 2021). The bacterial community had the highest robustness and lowest vulnerability values in HX (Supplementary Table S3). This indicated that the bacterial network in this region was more stable compared to those in the other two regions.
Discussion
Relationship between bacterial alpha diversity and environmental factors and their differences in the different regions
There are significant differences in salinity in the three regions (averagely 21.27 ppt in QD, 3.50 in HX, and 0.62 in YRH), and we suspect that salinity is the main driving force on the bacterial diversity in the three regions as also suggested in previous studies (Yang et al., 2016a; Zhong et al., 2016). Salinity had different effects on the bacterial diversity indices of lakes in QD, YRH, and HX according to our data (Supplementary Figure S5). Salinity was positively correlated with bacterial alpha diversity in QD, but negatively correlated with bacterial alpha diversity in YRH and HX regions (Supplementary Figure S5). The response of bacterial abundance and diversity to changes in salinity in YRH and HX regions follows the “general survival theory” that more extreme habitats lead to loss of microbial diversity. Generally, higher salinity in habitats increases extracellular osmosis pressure in bacteria, making the bacteria less active or even leading bacterial death. These further lead to loss of bacterial richness and diversity (Mo et al., 2021). However, the positive relationship between bacterial alpha diversity and salinity in QD is not consistent with the “general survival theory”. Possible explanations are as follows: 1) the niche availability for halotolerant bacterial in lakes in QD increased with increasing salinity (Wang et al., 2011); 2) some bacterial groups can adapt to the high salinity habitats in lakes in QD which have survived under the stress of extreme salinity for a long time (Mo et al., 2021).
Bacterial community composition and drivers
Our data showed that the top four bacteria phyla were Proteobacteria (42.43%), Bacteroidetes (21.51%), Actinobacteria (15.07%), and Firmicutes (12.13%) in QD, Proteobacteria (43.64%), Cyanobacteria (20.57%), Bacteroidetes (19.46%), and Actinobacteria (8.08%) in YRH, and Bacteroidetes (46.07%), Proteobacteria (38.13%), Actinobacteria (8.70%), and Cyanobacteria (4.48%) in HX (Supplementary Figure S3A). The relative abundance of Proteobacteria in the three regions was relatively high, which indicated that Proteobacteria were not sensitive to the extreme habitat of the plateau. This was consistent with the result of many previous studies which found Proteobacteria to be the dominant phylum in lakes (Jiang et al., 2019; Chakraborty et al., 2020). The relative abundance of Proteobacteria in HX was lower than those in QD and YRH (Supplementary Figure S3A). As shown in Supplementary Figure S8, the relative abundances of Proteobacteria in QD and YRH were significantly correlated with ORP and NO3−-N, and with TN and NO3−-N in HX. In addition, the concentrations of ORP and NO3−-N in QD and YRH were significantly different and the TN and NO3−-N concentrations in HX were lower than those in QD and YRH (Table1). Therefore, the difference in the relative abundance of Proteobacteria in the three regions might be caused by the significant differences in ORP, NO3−-N, and TN concentrations in different regions.
Bacteroidetes was the most abundant phylum in HX possibly because protorhodopsin in their cells could enhance their adaptability to the oligotrophic conditions of lakes in HX (Wang et al., 2020). The relative abundances of Actinobacteria in YRH and HX regions were similar, but were lower than that in QD. The relative abundance of Actinobacteria had significant negative relationships with DO and pH, but significant positive relationships with SAL, DOC, and TN (Supplementary Figure S8). Thus, the lower DO concentration and higher concentrations of SAL, DOC, and TN in QD may be beneficial to the survival of Actinobacteria leading to their higher relative abundance. It is worth noting that the relative abundance of Firmicutes, which can be used as an indicator of fecal pollution, is relatively high in the Qinghai lake, the Tuosu lake, and the Kelvke lake in QD (Supplementary Figure S6), implying that water quality of these lakes are threatened by livestock fecal (Zhang et al., 2020a). The concentrations of TN and NO3−-N in these three lakes are the highest when compared with all other sampling sites in QD (Supplementary Table S1).
At the class level, the dominant bacteria in the QD and YRH regions were Gamma-proteobacteria, and the dominant bacteria in HX was Bacteroidia (Supplementary Figure S3B). The class of Gamma-Proteobacteria, one member of Proteobacteria phyla, was known as the typical anti-disturbance taxa and the most abundant class present in eutrophic lakes, which contributed to nitrogen fixation, sulfur metabolism, methane metabolism, and salinity reduction of brackish lakes (Zhang et al., 2020b; Chakraborty et al., 2020; Wang et al., 2020). However, previous research reported that the dominant species was Beta-proteobacteria (37.2%) in a Tibet glacial stream and lake (Gu et al., 2020). Further analysis of the differences between the bacterial habitats in our study and in Gu et al. (2020) showed that the mean water temperature, TN, and DOC concentrations in QD and YRH regions were higher than those in Gu et al. (2020). We suspect that the inconsistency of the results may be due to the differences in habitat conditions, hydrological characteristics, and recharge water sources (Freimann et al., 2015).
Consistent with previous studies (Zhong et al., 2016; Gu et al., 2020; Tolotti et al., 2020; Yang et al., 2020), our findings showed that altitude, TN, and pH were the most important factors in determining bacterial composition variations in the three regions. In general, the effect of different altitudes on bacterial community structure is manifested in two aspects. Firstly, the changes in altitude lead to changes in the physicochemical factors of water (such as water temperature and DO concentration) (Supplementary Figure S5). Secondly, geographic isolation between habitats caused by altitude hinders the dispersal of bacteria between habitats, resulting in differences in bacterial community between lakes (Zhang et al., 2020c; Shi et al., 2020). It could be seen that altitude and the related environmental factors had significant correlations with the bacterial abundance of lakes in QD from Supplementary Figure S8. It is likely that the altitudinal effect on bacterial community structure of lakes in QD is through changing physicochemical properties of the lake water bodies. TN was the most robust driver in determining bacterial community composition in the investigated lakes in YRH (Supplementary Figure S7). Nitrogen is considered as the most important macronutrient for bacterial cellular processes (i.e., photosynthesis, respiration, energy storage and transfer, and cell division) responsible for primary production and formation of algal blooms (Wang et al., 2020; Xia et al., 2020). Variance of bacterial community structure in HX was mainly driven by pH (Supplementary Figure S7). The variations of pH value can alter the availability of nutrients and the characteristics of DOC in the habitat, and therefore affect the growth and metabolism of heterotrophic bacteria (Wang et al., 2020).
Biogeography distribution patterns and their differences in the different regions
With the increases of geographical distances, the Bray-Curtis similarity of bacterial communities decreased significantly in each region and in all the three regions (Figure 6). This was consistent with a previous study which reported geographic patterns of bacterial community in 23 lakes on the western of the Tibetan Plateau (Liu et al., 2020). However, the spatial turnover rate (represented by the slope of the fitted curve: 0.55) of bacterial community in our study was much higher than that in Liu et al. (2020), which was 0.14, indicating that bacterial community of lakes in this study are subject to stronger dispersal limitation and environmental filtering (Liu et al., 2018). The salinity gradient (0.15-69.88) between lakes in our study was larger than that in Liu et al. (2020) (0.01-47.8), and range of geographical distance in our study (1.4–799 km) is smaller than that in Liu et al. (2020) (0.5–1000 km). This indicated that the effects of salinity gradient on the bacterial community geographic patterns in the plateau lakes was greater compared to geographical distance, which was further supported by VPA results (Figure 5).
Further analysis of the distance decay relationship of bacterial community in each region showed that the order of bacterial community spatial turnover rate in the three regions were as follow: HX > YRH > QD (Figure 6), indicating that the bacterial community in HX was highly sensitive to changes in geographical factors and water physicochemical factors compared to those in YRH and QD. This could be further confirmed by the Mantel and Partial results (HX: Mantel r = 0.469; YRH: Mantel r = 0.369; QD: r = 0.434, Supplementary Table S2). Furthermore, our result showed that the variation of salinity in QD was largest, followed by HX and YRH regions and only changes of Temp and Turb in HX were largest (Table1), which implied that the higher bacterial community spatial turnover rate in HX was related to the higher Temp and Turb gradients. Interestingly, the spatial turnover rate of bacterial community in QD was the smallest, while the correlation coefficient between bacterial community and environmental factors in QD was greater than that in YRH. Therefore, we speculated that it might be attributed to larger hydraulic connectivity of different sampling lakes in QD (Figure 1). Larger hydraulic connectivity created more homogenous environmental conditions for bacteria among different lakes, which led to less taxonomic differences spatially in QD (Liu et al., 2018).
Co-occurrence network of bacterial community and their differences in the different regions
Bacterial interactions play key roles in affecting bacterial community composition and its geographical distribution. We constructed bacterial networks to analyze the relationship between bacterial species as well as their ecological roles (Liu et al., 2020). The value of topological parameters reflects the complexity of network. The positive relationships represent mutual symbiosis and parasitism relationships and the negative correlations reflect predation and competition among bacterial species (Mo et al., 2021; Wang et al., 2021). We found that the proportion of positive correlations (99.74%) between bacterial species in the plateau lakes was much higher than the proportion of negative ones (0.26%), which indicated that there was niche sharing among bacterial species in the plateau lakes (Zeng et al., 2019). Compared with the bacterial network in low-altitude lakes (Jiao et al., 2020), the proportion of negative correlations between nodes in bacterial network in high-altitude lakes was smaller, indicating that there was less competition among bacterial species in high-altitude lakes. According to a previous study (Zeng et al., 2019), a higher proportion of competitive correlations among bacterial species may enhance the stability of the network under environmental perturbations. Hence, bacterial networks in high-altitude lakes may be more susceptible to environmental changes than bacterial networks in low-altitude lakes.
Additionally, we found that the complexity of bacterial network in QD was much higher than those in YRH and HX regions by comparing the bacterial networks in the three regions. We speculate that there are several possible reasons for this. Frist, the higher bacterial richness and diversity in QD can increase the interaction between bacterial species and complicate their network. Second, based on the findings of Ji et al. (2019), we suggest that the high salinity of lakes in QD increase the complexity of the bacterial network in their lakes. Complexity of bacterial network was proposed to be affected by high salinity through increasing cooperation or competition among bacterial species (Yang et al., 2020).
Key species (nodes with the most connections to other nodes in a network) in bacterial networks play an important role in maintaining the network structure (Deng et al., 2012). Relevant research results showed that removal or disappearance of key species in bacterial network could cause the bacterial network structure to change rapidly and even lead to network collapse (Zhou et al., 2020). Our results showed that the key species in QD and HX regions were Clostridia and Bacterodia, while the nodes in the bacterial network in YRH did not reach the threshold for key species. This suggests an increased possibility of disappearance of modules or decomposition of network in YRH owing to the absence of key species. The key species of the bacterial network in QD (Clostridia) can tolerate higher salinity in the habitat and decompose carbohydrates into organic acids. The protorhodopsin produced by the key species of the bacterial network in HX (Bacterodia) helps it to survive in the lakes in HX with low nutrient concentrations (Table1) and promote decomposition and fermentation of organic matter in its habitat (Wang et al., 2020).
Conclusion
We analyzed the differences in bacterial diversity, composition, geographical distribution, and their driving factors in three distinct neighboring regions on the QTP. The results showed that alpha diversity indices of bacterial community in HX were the lowest. The dominant species at the phylum and class levels in QD and YRH were the same, but with different relative abundances. The most abundant species in HX at the phylum and class levels were Bacteroidetes, Bacteroidia, and Flavobacterium, respectively. Further, community compositions of bacterial were significantly different in QD, YRH, and HX, owing to environmental heterogeneity in the three regions.
The most important factors in driving bacterial community composition at QD, YRH, and HX were altitude, TN, and pH, respectively. Both water physicochemical factors and geographical factors had significant impacts on bacterial community structure in QD and HX, but geographical factors had very small impact on the bacterial community in YRH. Correlation between geographical distance and bacterial similarity in HX was stronger than those in QD and YRH. Network analysis revealed that the bacterial community networks in the three regions were different in their structure, complexity, and stability. Bacterial communities in QD and HX had the most complex and stable networks, respectively.
Data availability statement
The datasets presented in this study can be found in online repositories. The names of the repository/repositories and accession number(s) can be found in the article/Supplementary Material.
Author contributions
DW performed investigation, visualization, and writing of the original draft. YH performed conceptualization, writing, supervision and funding acquisition. SZ performed conceptualization, writing, and supervision. SL, TW, and HY reviewed and revised the mansucript.
Funding
This study was supported by Major Basic Research Development Program of the Science and Technology Agent, Qinghai Province (2019-SF-146), The Young & Mid-aged Scientific Research Fund Project of the Qinghai university (2018-QGY-9) and the Open Research Foundation Supported by State Key Laboratory of Hydroscience and Engineering-Tsinghua University (sklhse-2019-A-04).
Conflict of interest
The authors declare that the research was conducted in the absence of any commercial or financial relationships that could be construed as a potential conflict of interest.
The reviewer SL declared a shared affiliation with the authors SL and TW to the handling editor at the time of review.
Publisher’s note
All claims expressed in this article are solely those of the authors and do not necessarily represent those of their affiliated organizations, or those of the publisher, the editors and the reviewers. Any product that may be evaluated in this article, or claim that may be made by its manufacturer, is not guaranteed or endorsed by the publisher.
Supplementary material
The Supplementary Material for this article can be found online at: https://www.frontiersin.org/articles/10.3389/fenvs.2022.1033160/full#supplementary-material
References
Banerjee, S., Walder, F., Buchi, L., Meyer, M., Held, A. Y., Gattinger, A., et al. (2019). Agricultural intensification reduces microbial network complexity and the abundance of keystone taxa in roots. ISME J. 13, 1722–1736. doi:10.1038/s41396-019-0383-2
Cadena, S., Aguirre-Macedo, M. L., Cerqueda-García, D., Cervantes, F. J., Herrera-Silveira, J. A., and Garcia-Maldonado, J. Q. (2019). Community structure and distribution of benthic bacteria and archaea in a stratified coastal lagoon in the southern gulf of Mexico. Estuar. Coast. Shelf Sci. 230, 106433. doi:10.1016/j.ecss.2019.106433
Caporaso, J. G., Kuczynski, J., Stombaugh, J., Bittinger, K., Bushman, F. D., Costello, E. K., et al. (2010). Qiime allows analysis of high-throughput community sequencing data. Nat. Methods 7 (5), 335–336. doi:10.1038/nmeth.f.303
Chakraborty, J., Sapkale, V., Shah, M., Rajput, V., Mehetre, G., Agawane, S., et al. (2020). Metagenome sequencing to unveil microbial community composition and prevalence of antibiotic and metal resistance genes in hypersaline and hyperalkaline lonar lake, India. Ecol. Indic. 110, 105827. doi:10.1016/j.ecolind.2019.105827
Chao, A., Chiu, C. H., and Jost, L. (2010). Phylogenetic diversity measures based on hill numbers. Phil. Trans. R. Soc. B 365, 3599–3609. doi:10.1098/rstb.2010.0272
Chen, L. M., Liu, S. T., Chen, Q., Zhu, G. B., Wu, X., Wang, J. W., et al. (2019). Anammox response to natural and anthropogenic impacts over the yangtze river. Sci. Total Environ. 665, 171–180. doi:10.1016/j.scitotenv.2019.02.096
Chen, W. D., and Wen, D. H. (2021). Archaeal and bacterial communities assembly and co-occurrence networks in subtropical mangrove sediments under spartina alterniflora invasion. Environ. Microbiome 16, 10. doi:10.1186/s40793-021-00377-y
Deng, Y., Jiang, Y. H., Yang, Y. F., He, Z. L., Luo, F., and Zhou, J. Z. (2012). Molecular ecological network analyses. BMC Bioinforma. 113, 113. doi:10.1186/1471-2105-13-113
Freimann, R., Burgmann, H., Findlay, S. E., and Robinson, C. T. (2015). Hydrologic linkages drive spatial structuring of bacterial assemblages and functioning in alpine floodplains. Front. Microbiol. 6, 1221. doi:10.3389/fmicb.2015.01221
Gu, Z. Q., Liu, K. S., Pedersen, M. W., Wang, F., Chen, Y. Y., Zeng, C., et al. (2020). Community assembly processes underlying the temporal dynamics of glacial stream and lake bacterial communities. Sci. Total Environ. 761, 143178. doi:10.1016/j.scitotenv.2020.143178
Han, R., Zhang, X., Liu, J., Long, Q. F., Chen, L. S., Liu, D. L., et al. (2017). Microbial community structure and diversity within hypersaline keke salt lake environments. Can. J. Microbiol. 63, 895–908. doi:10.1139/cjm-2016-0773
Hill, M. O. (1973). Diversity and evenness: A unifying notation and its consequences. Ecology 54, 427–432. doi:10.2307/1934352
Ji, M. K., Kong, W. D., Yue, L. Y., Wang, J. B., Deng, Y., and Zhu, L. P. (2019). Salinity reduces bacterial diversity, but increases network complexity in Tibetan plateau lakes. FEMS Microbiol. Ecol. 95, fiz190. doi:10.1093/femsec/fiz190
Jiang, H. C., Deng, S. C., Huang, Q. Y., Dong, H. L., and Yu, B. S. (2010). Response of aerobic anoxygenic phototrophic bacterial diversity to environment conditions in saline lakes and daotang river on the Tibetan plateau, nw China. Geomicrobiol. J. 27, 400–408. doi:10.1080/01490450903480269
Jiang, Y. M., Huang, H. Y., Ma, T. L., Ru, J. L, Blank, S., Kurmayer, R., et al. (2019). Temperature response of planktonic microbiota in remote alpine lakes. Front. Microbiol. 10, 1714. doi:10.3389/fmicb.2019.01714
Jiao, C. C., Zhao, D. Y., Zeng, J., Guo, L., and Yu, Z. B. (2020). Disentangling the seasonal co-occurrence patterns and ecological stochasticity of planktonic and benthic bacterial communities within multiple lakes. Sci. Total Environ. 740, 140010. doi:10.1016/j.scitotenv.2020.140010
Kubo, K., Kojima, H., and Fukui, M. (2014). Vertical distribution of major sulfate-reducing bacteria in a shallow eutrophic meromictic lake. Syst. Appl. Microbiol. 37, 510–519. doi:10.1016/j.syapm.2014.05.008
Li, J., Zhu, L. P., Li, M. H., Wang, J. B., and Ma, Q. F. (2020). Origin of modern dolomite in surface lake sediments on the central and Western Tibetan plateau. Quat. Int. 544, 65–75. doi:10.1016/j.quaint.2020.02.018
Li, L., Ning, D. L., Jeon, Y., Ryu, H., Santo Domingo, J. W., Kang, D. W., et al. (2021). Ecological insights into assembly processes and network structures of bacterial biofilms in full-scale biologically active carbon filters under ozone implementation. Sci. Total Environ. 751, 141409. doi:10.1016/j.scitotenv.2020.141409
Li, Y., Gao, Y., Zhang, W. L., Wang, C., Wang, P. F., Niu, L. H., et al. (2019). Homogeneous selection dominates the microbial community assembly in the sediment of the three gorges reservoir. Sci. Total Environ. 690, 50–60. doi:10.1016/j.scitotenv.2019.07.014
Liu, K. S., Hou, J. Z., Liu, Y. Q., Hu, A. Y., Wang, M. D., Wang, F., et al. (2019a). Biogeography of the free-living and particle-attached bacteria in Tibetan lakes. FEMS Microbiol. Ecol. 95, fiz088. doi:10.1093/femsec/fiz088
Liu, K. S., Liu, Y. Q., Han, B. P., Xu, B. Q., Zhu, L. P., Ju, T. T., et al. (2019c). Bacterial community changes in a glacial-fed Tibetan lake are correlated with glacial melting. Sci. Total Environ. 651, 2059–2067. doi:10.1016/j.scitotenv.2018.10.104
Liu, K. S., Liu, Y. Q., Hu, A. Y., Wang, F., Chen, Y. Y., Gu, Z. Q., et al. (2020). Different community assembly mechanisms underlie similar biogeography of bacteria and microeukaryotes in Tibetan lakes. FEMS Microbiol. Ecol. 96, fiaa071. doi:10.1093/femsec/fiaa071
Liu, K. S., Yao, T. D., David, P. A., Jiao, N. Z., Zeng, Y. H., Guo, B. X., et al. (2021). Bacteria in the lakes of the Tibetan plateau and polar regions. Sci. Total Environ. 754, 142248. doi:10.1016/j.scitotenv.2020.142248
Liu, S. W., Yu, H., Yu, Y. H., Huang, J., Zhou, Z. Y., Zeng, J. X., et al. (2022). Ecological stability of microbial communities in lake donghu regulated by keystone taxa. Ecol. Indic. 136, 108695. doi:10.1016/j.ecolind.2022.108695
Liu, T., Zhang, A. N., Wang, J. W., Liu, S. F., Jiang, X. T., Dang, C. Y., et al. (2018). Integrated biogeography of planktonic and sedimentary bacterial communities in the yangtze river. Microbiome 6, 16. doi:10.1186/s40168-017-0388-x
Liu, W. H., Xie, C. W., Zhao, L., Wu, T. H., Wang, W., Zhang, Y. X., et al. (2019b). Dynamic changes in lakes in the hoh xil region before and after the 2011 outburst of zonag lake. J. Mt. Sci. 16, 1098–1110. doi:10.1007/s11629-018-5085-0
Luo, D. L., Jin, H. J., Du, H. Q., Li, C., Ma, Q., Duan, S. Q., et al. (2020). Variation of alpine lakes from 1986 to 2019 in the headwater area of the yellow river, Tibetan plateau using Google Earth engine. Adv. Clim. Change Res. 11, 11–21. doi:10.1016/j.accre.2020.05.007
Mania, I., Gorra, R., Colombo, N., Freppaz, M., Martin, M., and Anesio, A. M. (2019). Prokaryotic diversity and distribution in different habitats of an alpine rock glacier-pond system. Microb. Ecol. 78, 70–84. doi:10.1007/s00248-018-1272-3
Meng, L., Zuo, R., Wang, J. S., Yang, J., Li, Q., Chen, M. H., et al. (2020). The spatial variations of correlation between microbial diversity and groundwater quality derived from a riverbank filtration site, northeast China. Sci. Total Environ. 706, 135855. doi:10.1016/j.scitotenv.2019.135855
Mo, Y. Y., Peng, F., Gao, X. F., Xiao, P., Logares, R., Jeppesen, E., et al. (2021). Low shifts in salinity determined assembly processes and network stability of microeukaryotic plankton communities in a subtropical urban reservoir. Microbiome 9, 128. doi:10.1186/s40168-021-01079-w
Mora-Ruiz, M. D. R., Cifuentes, A., Font-Verdera, F., Perez-Fernandez, C., Farias, M., Gonzalez, B., et al. (2018). Biogeographical patterns of bacterial and archaeal communities from distant hypersaline environments. Syst. Appl. Microbiol. 41, 139–150. doi:10.1016/j.syapm.2017.10.006
Olesen, J. M., Bascompte, J., Dupont, Y. L., and Jordano, P. (2007). The modularity of pollination networks. Proc. Natl. Acad. Sci. U. S. A. 104, 19891–19896. doi:10.1073/pnas.0706375104
Ren, Z., Wang, F., Qu, X. D., Elser, J. J., Liu, Y., and Chu, L. M. (2017). Taxonomic and functional differences between microbial communities in qinghai lake and its input streams. Front. Microbiol. 8, 2319. doi:10.3389/fmicb.2017.02319
Ren, Z., Zhang, C., Li, X., Ma, K, Feng, K. X, Zhang, Z., et al. (2021a). Abundant and rare bacterial taxa structuring differently in sediment and water in thermokarst lakes in the yellow river source area, qinghai-tibet plateau. bioRxiv Prepr.
Ren, Z., Zhang, C., Li, X., Ma, K., Zhang, Z., Feng, K. X., et al. (2021b). Bacterial communities present distinct co-occurrence networks in sediment and water of the thermokarst lakes in the yellow river source area. Front. Microbiol. 12, 716732. doi:10.3389/fmicb.2021.716732
Shi, Y., Jiang, Y. Y., Wang, S. Y., Wang, X. M., and Zhu, G. B. (2020). Biogeographic distribution of comammox bacteria in diverse terrestrial habitats. Sci. Total Environ. 717, 137257. doi:10.1016/j.scitotenv.2020.137257
Tolotti, M., Cerasino, L., Donati, C., Pindo, M., Rogora, M., Seppi, R., et al. (2020). Alpine headwaters emerging from glaciers and rock glaciers host different bacterial communities: Ecological implications for the future. Sci. Total Environ. 717, 137101. doi:10.1016/j.scitotenv.2020.137101
Tu, Q. C., Yan, Q. Y., Deng, Y., Michaletz, S. T., Buzzard, V., Weiser, M. D., et al. (2020). Biogeographic patterns of microbial co-occurrence ecological networks in six American forests. Soil Biol. Biochem. 148, 107897. doi:10.1016/j.soilbio.2020.107897
Wang, B. H., Zheng, X. F., Zhang, H. J., Xiao, F. S., Gu, H., Zhang, K. K., et al. (2020). Bacterial community responses to tourism development in the xixi national wetland park, China. Sci. Total Environ. 720, 137570. doi:10.1016/j.scitotenv.2020.137570
Wang, J. J., Yang, D. M., Zhang, Y., Shen, J., van der Gast, C., Hahn, M. W., et al. (2011). Do patterns of bacterial diversity along salinity gradients differ from those observed for macroorganisms? PLoS One 6, e27597. doi:10.1371/journal.pone.0027597
Wang, S. Y., Liu, W. Y., Zhao, S. Y., Wang, C., Zhuang, L. J., Liu, L., et al. (2019). Denitrification is the main microbial n loss pathway on the qinghai-tibet plateau above an elevation of 5000m. Sci. Total Environ. 696, 133852. doi:10.1016/j.scitotenv.2019.133852
Wang, Y. B., Hu, X. K., Sun, Y. Y., and Wang, C. X. (2021). Influence of the cold bottom water on taxonomic and functional composition and complexity of microbial communities in the southern yellow sea during the summer. Sci. Total Environ. 759, 143496. doi:10.1016/j.scitotenv.2020.143496
Wang, W. J., Yi, Y. J., Yang, Y. F., Zhou, Y., Zhang, S. H., Wang, X., et al. (2020). Impact of anthropogenic activities on the sediment microbial communities of baiyangdian shallow lake. Int. J. Sediment Res. 35, 180–192. doi:10.1016/j.ijsrc.2019.10.006
Wen, Z. D., Song, K. S., Liu, G., Shang, Y. X., Hou, J. B., Lyu, L., et al. (2019). Impact factors of dissolved organic carbon and the transport in a river-lake continuum in the tibet plateau of China. J. Hydrol. X. 579, 124202. doi:10.1016/j.jhydrol.2019.124202
Williams, W. D. (1998). Salinity as a determinant of the structure of biological communities in salt lakes. Hydrobiologia 381, 191–201. doi:10.1023/a:1003287826503
Wu, Q. L., Zwart, G., Schauer, M., Kamst-van Agterveld, M. P., and Hahn, M. W. (2006). Bacterioplankton community composition along a salinity gradient of sixteen high-mountain lakes located on the Tibetan plateau, China. Appl. Environ. Microbiol. 72, 5478–5485. doi:10.1128/AEM.00767-06
Xia, P. H., Yan, D. B., Sun, R. G., Song, X., Lin, T., and Yi, Y. (2020). Community composition and correlations between bacteria and algae within epiphytic biofilms on submerged macrophytes in a plateau lake, southwest China. Sci. Total Environ. 727, 138398. doi:10.1016/j.scitotenv.2020.138398
Xie, G. J., Tang, X. M., Shao, K. Q., Hu, Y., Liu, H., Martin, R. M., et al. (2020). Spatiotemporal patterns and environmental drivers of total and active bacterial abundances in lake taihu, China. Ecol. Indic. 114, 106335. doi:10.1016/j.ecolind.2020.106335
Xing, P., Hahn, M. W., and Wu, Q. L. (2009). Low taxon richness of bacterioplankton in high-altitude lakes of the eastern Tibetan plateau, with a predominance of bacteroidetes and synechococcus spp. Appl. Environ. Microbiol. 75, 7017–7025. doi:10.1128/AEM.01544-09
Xiong, J. B., Liu, Y. Q., Lin, X. G., Zhang, H. Y., Zeng, J., Hou, J. Z., et al. (2012). Geographic distance and ph drive bacterial distribution in alkaline lake sediments across Tibetan plateau. Environ. Microbiol. 14, 2457–2466. doi:10.1111/j.1462-2920.2012.02799.x
Yang, J., Jiang, H. C., Sun, X. X., Huang, J. R., Han, M. X., and Wang, B. C. (2020). Distinct co-occurrence patterns of prokaryotic community between the waters and sediments in lakes with different salinity. FEMS Microbiol. Ecol. 97, fiaa234. doi:10.1093/femsec/fiaa234
Yang, J., Jiang, H. C., Wu, G., Liu, W., and Zhang, G. J. (2016a). Distinct factors shape aquatic and sedimentary microbial community structures in the lakes of Western China. Front. Microbiol. 7, 1782. doi:10.3389/fmicb.2016.01782
Yang, J., Ma, L., Jiang, H. C., Wu, G., and Dong, H. L. (2016b). Salinity shapes microbial diversity and community structure in surface sediments of the qinghai-Tibetan lakes. Sci. Rep. 6, 25078. doi:10.1038/srep25078
Yang, J. (2015). Microbial response to environmental changes in qinghai-Tibetan lakes and its environmental implications. Wuhan: China university of Geosciences. (In Chinese).
Zeng, J., Jiao, C. C., Zhao, D. Y., Xu, H. M., Huang, R., Cao, X. Y., et al. (2019). Patterns and assembly processes of planktonic and sedimentary bacterial community differ along a trophic gradient in freshwater lakes. Ecol. Indic. 106, 105491. doi:10.1016/j.ecolind.2019.105491
Zeng, Q. C., An, S. S., Liu, Y., Wang, H. L., and Wang, Y. (2019). Biogeography and the driving factors affecting forest soil bacteria in an arid area. Sci. Total Environ. 680, 124–131. doi:10.1016/j.scitotenv.2019.04.184
Zhang, L., Wang, K. F., Li, X. C., Gao, G., and Jiang, J. H. (2020b). Linking bacterial community shifts with changes in the dissolved organic matter pool in a eutrophic lake. Sci. Total Environ. 719, 137387. doi:10.1016/j.scitotenv.2020.137387
Zhang, L., Zhao, F., Li, X. C., and Lu, W. J. (2020a). Contribution of influent rivers affected by different types of pollution to the changes of benthic microbial community structure in a large lake. Ecotoxicol. Environ. Saf. 198, 110657. doi:10.1016/j.ecoenv.2020.110657
Zhang, S. B., Qin, W., Xia, X. H., Xia, L. Z., Li, S. L., Zhang, L. W., et al. (2020c). Ammonia oxidizers in river sediments of the qinghai-tibet plateau and their adaptations to high-elevation conditions. Water Res. 173, 115589. doi:10.1016/j.watres.2020.115589
Zhao, D. Y., Cao, X. Y., Huang, R., Zeng, J., Shen, F., Xu, H. M., et al. (2017). The heterogeneity of composition and assembly processes of the microbial community between different nutrient loading lake zones in taihu lake. Appl. Microbiol. Biotechnol. 101, 5913–5923. doi:10.1007/s00253-017-8327-0
Zhao, D. Y., Shen, F., Zeng, J., Huang, R., Yu, Z. B., and Wu, Q. L. (2016). Network analysis reveals seasonal variation of co-occurrence correlations between cyanobacteria and other bacterioplankton. Sci. Total Environ. 573, 817–825. doi:10.1016/j.scitotenv.2016.08.150
Zhong, Z. P., Liu, Y., Miao, L. L., Wang, F., Chu, L. M., Wang, J. L., et al. (2016). Prokaryotic community structure driven by salinity and ionic concentrations in plateau lakes of the Tibetan plateau. Appl. Environ. Microbiol. 82, 1846–1858. doi:10.1128/AEM.03332-15
Zhou, H., Gao, Y., Jia, X. H., Wang, M. M., Ding, J. J., Cheng, L., et al. (2020). Network analysis reveals the strengthening of microbial interaction in biological soil crust development in the mu us sandy land, northwestern China. Soil Biol. Biochem. 144, 107782. doi:10.1016/j.soilbio.2020.107782
Zhou, L., Zhou, Y. Q., Hu, Y., Cai, J., Liu, X., Bai, C. R., et al. (2019). Microbial production and consumption of dissolved organic matter in glacial ecosystems on the Tibetan plateau. Water Res. 160, 18–28. doi:10.1016/j.watres.2019.05.048
Keywords: bacterial community, lake ecosystem, Qinghai-Tibet Plateau, biogeographic patterns, co-occurrence network
Citation: Wang D, Huang Y, Zhang S, Liu S, Wang T and Yang H (2022) Differences in bacterial diversity, composition, and community networks in lake water across three distinct regions on the Qinghai-Tibet Plateau. Front. Environ. Sci. 10:1033160. doi: 10.3389/fenvs.2022.1033160
Received: 31 August 2022; Accepted: 14 October 2022;
Published: 23 November 2022.
Edited by:
Matteo Rubinato, Coventry University, United KingdomCopyright © 2022 Wang, Huang, Zhang, Liu, Wang and Yang. This is an open-access article distributed under the terms of the Creative Commons Attribution License (CC BY). The use, distribution or reproduction in other forums is permitted, provided the original author(s) and the copyright owner(s) are credited and that the original publication in this journal is cited, in accordance with accepted academic practice. No use, distribution or reproduction is permitted which does not comply with these terms.
*Correspondence: Yuefei Huang, yuefeihuang@tsinghua.edu.cn; Shuo Zhang, zhangs2019@tsinghua.edu.cn