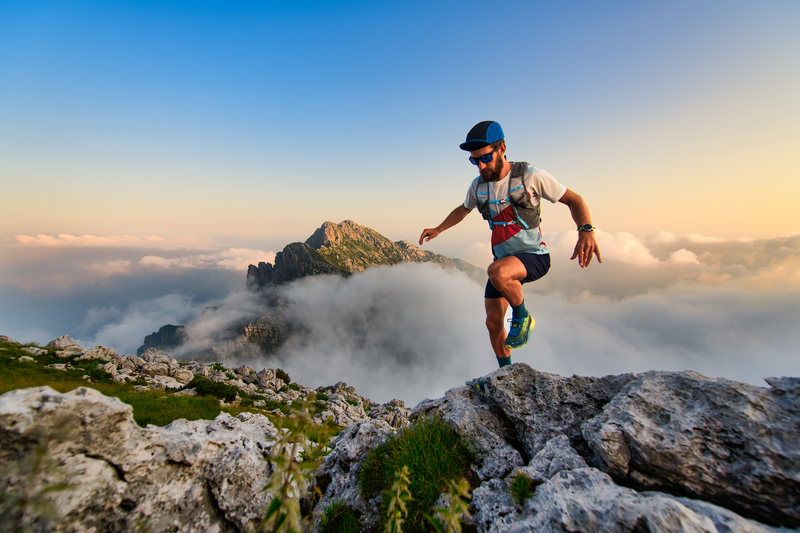
95% of researchers rate our articles as excellent or good
Learn more about the work of our research integrity team to safeguard the quality of each article we publish.
Find out more
ORIGINAL RESEARCH article
Front. Environ. Sci. , 17 October 2022
Sec. Toxicology, Pollution and the Environment
Volume 10 - 2022 | https://doi.org/10.3389/fenvs.2022.1032005
This article is part of the Research Topic Soil and Sediment Pollution, Processes and Remediation, Volume II View all 17 articles
A method with the potential for comprehensive microplastic monitoring in river sediments is presented in this study. We introduce a novel combination of electrostatic separation, density separation, and differential scanning calorimetry (DSC). Currently, microplastic analysis in sediments is limited in terms of sample masses, processing time, and analytical robustness. This work evaluated a method to process large sample masses efficiently and still obtain robust results. Four particulate matrices, including commercial sands and river sediments, were spiked with PCL, LD-PE, and PET microplastic particles (63–200 µm). Samples with a mass of 100 g and 1,000 g (sand only) contained 75 mg of each microplastic. After electrostatic separation, the mass of sand samples was reduced by 98%. Sediment samples showed a mass reduction of 70–78%. After density separation, the total mass reduction of sediment samples was above 99%. The increased concentration of total organic carbon seems to have the highest impact on mass reduction by electrostatic separation. Nevertheless, the recovery of microplastic was independent of the particulate matrix and was polymer-specific. In 100 g samples, the average recovery rates for PCL, LD-PE, and PET were 74 ± 9%, 93 ± 9%, and 120 ± 18%, respectively. The recoveries of microplastic from 1,000 g samples were 50 ± 8%, 114 ± 9%, and 82 ± 11%, respectively. In scale up experiments, high recoveries of all microplastics were observed with a decrease in standard deviation. Moreover, the biodegradable polymer PCL could be used as an internal standard to provide quality assurance of the process. This method can overcome the current limitations of routine microplastic analysis in particulate matrices. We conclude that this method can be applied for comprehensive microplastic monitoring in highly polluted sediments. More studies on electrostatic separation and polymer-specific recovery rates in complex matrices are proposed.
Polymer particles with diameters between 1 and 1,000 µm are called microplastic (Hartmann et al., 2019). Microplastic release into the environment is diverse and includes tire wear, pellet loss, surface runoff, and littering followed by degradation of larger plastic debris (Schernewski et al., 2020; Kallenbach et al., 2022; Periyasamy and Tehrani-Bagha, 2022). The main sources of microplastic are land-based (Andrady, 2011). Estimations show that microplastic input can be 73 g/(capita a) in soils and 1.8 g/(capita a) in freshwater systems in Switzerland (Kawecki and Nowack, 2019). Once microplastic particles are emitted into the environment, they enter aquatic and geological cycles (Kane and Clare, 2019; De-la-Torre et al., 2021; Weber et al., 2021) and distribute within water–sediment systems (Akdogan and Guven, 2019). Particulate matrices, such as sediments, can retain microplastic particles and act as sinks for them (Nizzetto et al., 2016; Besseling et al., 2017; Horton and Dixon, 2018). Hence, microplastic is of great interest in the environmental sciences as an emerging contaminant (Morin-Crini et al., 2022). The environmental impact of microplastics in sediments has been investigated for potential eco-toxicological effects (Bellasi et al., 2020), including starvation, oxidative stress, and potential release of adsorbed pollutants (Wright et al., 2013; Anbumani and Kakkar, 2018; Rodrigues et al., 2019; Bellasi et al., 2020).
The assessment of sinks, risks, and remediation measures requires a comprehensive microplastic monitoring strategy. Monitoring of river sediments is of particular importance due to the allocated benthic organisms and their role in the food web and energy and contaminant transfer processes (Väinölä et al., 2008; Bellasi et al., 2020). However, several challenges have to be addressed for reliable microplastic analysis in sediments. A major challenge is that the variety of methodologies for microplastic sampling, processing, and analysis can hamper the harmonization and standardization (Hanvey et al., 2017; Shim et al., 2017; Mai et al., 2018; Adomat and Grischek, 2021). Generally, microplastic analysis in sediments consists of the extraction of microplastic particles from the matrix and subsequent identification and quantification. During the extraction, a matrix such as sediment particles is removed to isolate microplastic for analysis. Hence, a mass reduction of the sample occurs.
Determination of microplastic in river sediments requires large sample masses to ensure representative results of low microplastic contents. Density separation is the most common extraction method (Dioses-Salinas et al., 2020); however, this method might not be suitable when it comes to larger sample masses due to increased processing time and equipment effort (Enders et al., 2020a; Dioses-Salinas et al., 2020). Electrostatic separation of microplastic and sediment is a promising method to reduce the total mass of sediment samples before density separation (Stock et al., 2019). This technology is particularly applied for metal–polymer waste separation and mineral refinement (Manouchehri, 2000; Köhnlechner and Sander, 2009; Das et al., 2010; Gehringer, 2021). A different chargeability of metals, minerals, and polymers are exploited to separate these components (Köhnlechner, 1999; Mirkowska et al., 2016). Particularly, electrostatic separation has gained attention for microplastic sediment separation (Felsing et al., 2018; Enders et al., 2020b). Besides cost- and time-efficiency, the benefits are high sample throughput and the reduction of large samples (Enders et al., 2020b). A lack of toxic or corrosive chemicals, the preservation of the particle surface during the process, and the potential for process automation underline the potential of this method. However, electrostatic separation is limited to a minimum particle size of 100 µm (Hoffmann, 2020). Additionally, matrix properties such as total organic carbon (TOC) and the mineral composition of sediments can hamper the separation efficiency (Enders et al., 2020b). Subsequent density separation can be applied to overcome this limitation to conduct reliable microplastic analysis.
Analytical methods for microplastic determination are divided into particle counting methods (i.e., Fourier-transformation infrared or Raman microscopy) and mass determining methods (i.e., pyrolysis or thermal desorption gas chromatography-mass spectrometry (pyro/ted-GC-MS; Shim et al., 2017). Both methods are complementary, but their application should be designed to match individual research goals. Regarding microplastic monitoring in sediments, mass determining methods might be sufficient (Braun, 2020). These methods provide fast data acquisition for polymer types and their quantity in environmental samples. Moreover, the risk of contamination is lower for these methods because of the reduced atmospheric exposition compared to particle counting methods. Mass determining methods are thermo-analytical techniques, such as pyro/ted-GC-MS and differential scanning calorimetry (DSC), and chemical methods including nuclear magnetic resonance (NMR) spectroscopy (Shim et al., 2017). GC-MS techniques are sensitive to organic residues in an environmental sample. Hence, sample purification with acids, bases, hydrogen peroxide, or enzymes is necessary (Kittner et al., 2022). DSC might be a promising approach for microplastic determination in environmental samples because organic residues would not influence the analysis. Polymer identification and quantification by DSC or DSC-coupled thermogravimetry also have been investigated (Majewsky et al., 2016; Rodríguez Chialanza et al., 2018; Bitter and Lackner, 2020). A recent study shows the potential of DSC for microplastic analysis in sediments (Harzdorf et al., 2022). To achieve reliable results, however, mass reduction and low microplastic losses are necessary. If these requirements are fulfilled, DSC might provide an alternative to determine semi-crystalline polymers in a range of 0.05–1.5 mg per measurement in sediment samples (Harzdorf et al., 2022).
The objective of this work was to evaluate the application of electrostatic separation and DSC for a fast and robust microplastic analysis in sediments. A combination of electrostatic separation and density separation, followed by DSC, was investigated. Electrostatic separation can enable the enrichment of large sediment samples without the use of chemicals, as required for example for the Munich Plastic Sediment Separator (Imhof et al., 2012). Additionally, the exploitation of physical properties of polymers, such as melting and crystallization, can increase analytical robustness. In comparison to pyro/ted-GC-MS methods, extensive sample purification is obsolete for DSC (Kittner et al., 2022). Moreover, an approach to establishing an internal standard was investigated. Such internal standards can ensure the quality and reliability of results (Enders et al., 2020b). The biopolymer polycaprolactone (PCL) will be applied. Its biodegradability reduces the risk of blank values in environmental samples. Furthermore, melting and crystallization signals do not interfere with the signals of common polymers (Harzdorf et al., 2022). Hence, PCL as an internal standard could provide for the recovery of microplastic particles. The scope of this study is the extraction and analysis of spiked microplastic particles from four different particulate matrices. We will discuss 1) the effects of matrix properties on the mass reduction of particulate matrices by electrostatic separation, 2) the dependence of microplastic recovery on the matrix and polymer type by DSC, and 3) the potential application of PCL as an internal standard.
Four different particulate matrices (sand 1, sand 2, sediment 1, and sediment 2) were tested. Sand 1 (Cemex AG, Dresden, Germany) and sand 2 (Saxonia Baustoffe, Dresden, Germany) were commercial sands. Sediment 1 and sediment 2 were collected at the Elbe River (GPS: 51.047371, 13.816418) and the Weisseritz River (GPS: 51.062151, 13.687450), respectively. The river sediment samples were collected with a metal shovel approximately 0.5 m from the shore at a water depth of 0.2–0.4 m. All matrices were dried at 60°C until a constant mass was reached. The air supply of the utilized dry box (Heratherm OHM400, Thermo Fisher Scientific, Schwert, Germany) was equipped with a particle filter. After drying, particles with diameters >2 mm were removed from all matrices by sieving. Finally, the sieved matrices were further characterized by sieve analysis, TOC, and x-ray diffraction measurements.
For the determination of particle size distribution, 200 g of each matrix was analyzed. The sieve analysis was conducted with a vibratory sieve shaker (AS 200, Retsch, Haan Germany) using sieves with mesh sizes from 63 μm to 2000 µm according to DIN 4220 (version 2008–11). The parameters for sieve analyses were a shaking amplitude of 1 mm and a duration of 5 min under dry conditions. After sieving, the software EasySieve 2.6 (Retsch, Haan, Germany) was used to determine the average particle size (d50-values) and fraction ratios.
Two grams of each matrix were milled to a powder with the vibratory micro mill (Pulverisette 0, Fritsch, Idar-Oberstein, Germany) equipped with a steel mortar and grinding ball (d = 50 mm). An amplitude of 1 mm for 2 min was applied to mill the matrices. TOC measurements were conducted with 200 mg of each powdered matrix (n = 3). Particulate matrices were pretreated with 200 µl 10% HCl to remove inorganic carbon before analysis. The TOC content of the matrices was determined with a multi C/N-system 1,300 (analytic Jena, Jena, Germany) at 1,200°C by combustion.
The powders as prepared for TOC measurements were used for diffraction measurements in reflection mode. A powder diffractometer XRD3003 TT from GE Inspection Technologies (Boston, MA, United States) equipped with the energy dispersive Si-drift detector Meteor 0D was used. The measurements were conducted with Cu-Kα radiation with 2θ between 10 and 80° and an increment of 0.02° with 8 seconds per increment. For a quantitative phase analysis, the programs Profex Version 4.1.0 and BGMN Version 4.2.22 and the database ICDD PDF-2 Release 2021 were applied.
Microplastic particles of low-density polyethylene (LD-PE, MKCP2615, Sigma-Aldrich, Darmstadt, Germany), recycled polyethylene terephthalate (PET, KRUPET-A IV, Kruschitz GmbH, Völkermarkt Austria), and polycaprolactone (PCL, abifor1639, Abifor AG, Zurich, Switzerland) were used. The PCL was already available as a powder. Microplastic particles of LD-PE and PET were ground using a cryo-mill (Pulverisette 0, Fritsch, Idar-Oberstein, Germany) and liquid nitrogen (Harzdorf et al., 2022). After grinding, the particles were dried at 40°C until a constant mass was reached. To obtain the targeted size range of 63–200 µm microplastic particles were sieved (see Sieve analysis Section Sieve analysis).
A sample of 100 g of each matrix was spiked with 75 mg of each microplastic type. Five replicas of each matrix were prepared and used for electrostatic separation experiments. Additionally, scale-up experiments were conducted with 1,000 g samples of sand 1 and 75 mg of each microplastic type (n = 3). All samples were homogenized by stirring with a metal spoon and subsequently covered with aluminum foil.
A corona drum separator (KWS XS, Hamos, Prenzberg, Germany) was used for electrostatic separation experiments (Supplementary Material SM1). The device was selected based on previous studies (Felsing et al., 2018; Enders et al., 2020b). However, in comparison to these studies 1) the sample entry funnel was not modified (output opening: 12 cm), and 2) particle shields made of PVC were used as delivered from the manufacturer. Modifications include 3) additional weights underneath the vibrating conveyor for better control of the vibration intensity, and 4) the device had two position flaps and only the non-conductor fraction was used as a polymer-rich sample fraction. More information about the device is given in the Supplementary Material SM1.
Tests of different settings for high voltage, drum rotation, and vibration intensity were carried out to determine optimal instrument settings. Here, the mass reduction of sand 1 and recovery of LD-PE particles were used as validation criteria. The optimized instrument settings were then used for further separation experiments (Table 1).
The electrostatic separation of the prepared samples was conducted according to the following protocol: the relative humidity in the laboratory was set between 35% and 40%. Then, the device was booted and the instrument setting was entered as shown in Table 1. Next, the sample was added to the entry funnel and all residues were transferred from the storage vessel into the funnel with a brush. When the entire sample was transported from the conveyer, the vibration intensity was increased to 90% to flush the conveyer. The remaining material on the conveyer was carefully blown onto the rotating drum using pressurized air. After this procedure, all parameters were set to default and the device was turned off. The sample, which was collected on the drum scraper, was transferred into the box for the polymer-rich sample fraction. Only one separation cycle was carried out to reduce the loss of microplastic particles. Subsequently, the polymer-rich sample fraction was transferred with a brush into a storage vessel and covered with aluminum foil.
Only the polymer-rich samples from the electrostatic separation of river sediments and the samples from scale-up experiments were further enriched by density separation. For this separation, a 500-ml separation funnel and potassium iodide solution (50 wt%., density: 1.5 g/cm³, potassium iodide 99.0–100.5% Ph. Eur., VWR, Darmstadt, Germany) were used. The protocol of Enders et al. (2020) was followed to process the samples (Enders et al., 2020a). Potassium iodide solution (100 ml) was used for all samples and an extended sedimentation time of 18 h was applied. After sedimentation, the settled fraction was drained through the valve, and the remaining polymer-rich fraction was drained through the opening of the separation funnel. The sample fraction was filtrated with a paper filter. Cleaning of the funnel and the filtrated sample was conducted according to the protocol (Enders et al., 2020a). Subsequently, the samples were freeze-dried on the paper filter. Then the enriched sample was transferred into a glass vessel using a brush.
For DSC measurements (DSC 214 Polyma, Netzsch, Selb, Germany), 5–20 mg of an enriched sample was transferred in aluminum crucibles (Concavus 40 μm, Netzsch, Selb, Germany). The crucibles were sealed with a lid and subsequently pierced. Eight sub-samples (n = 8) were measured from every enriched sample. The following temperature program was used: three cycles (heating, cooling, heating) were applied between –50 and 300°C with a heating rate of 20 K/min. Additionally, there was an isothermal phase of 3 minutes between each cycle. The peak temperatures of melting and crystallization, as well as enthalpies during the cooling and the second heating phase, were determined for PCL, LD-PE, and PET using the program Proteus (version 8.0.2, Netzsch, Selb, Germany). The integration limits for the determination of melting and crystallization enthalpies (dhm and dhc) of the polymers are included in the Supplementary Materials (Supplementary Figure S2). A linear baseline was applied for the determination of enthalpies. Subsequently, the polymer mass per measurement was quantified according to the calibration data reported by Harzdorf et al. (2022) (Table 2). Equation 1 was used to calculate the polymer mass per DSC measurement:
where a1 and a2 are empirical coefficients for the melting and crystallization enthalpies (Table 2).
TABLE 2. Data reported by Harzdorf et al. (2022) for the multivariate calibration curve to quantify PCL, LD-PE, and PET by DSC measurements.
The determined polymer mass per DSC measurement mpolymer,DSC was projected to the total polymer-rich fraction (Equation 2). Consequently, the recovery rate of the polymer was calculated as the ratio of projected mass in the polymer-rich fraction mpolymer, enriched and the known mass from the sample preparation mpolymer,0 (Equation 3). Subsequently, an average recovery rate of each polymer was calculated for each particulate matrix:
where mDSC and menriched are the mass of the sample used for DSC measurement and the mass of the polymer-rich fraction, respectively.
All personal protective equipment was made of cotton. Sample preparation and the transfer were carried out with metal or glass tools and natural hairbrushes, which were pre-cleaned with plastic-free cellulose tissues and ethanol. All samples were stored in glass vessels sealed with aluminum foil. Contamination with airborne microplastic was decreased by reducing the exposure time to the atmosphere to a minimum. Further, the applied dry box was equipped with an H14 particle filter (retention 99.995%). The KWS XS was also applied in a laminar-flow box with an H14 particle filter.
Four particulate matrices were characterized regarding their particle size distribution, TOC, and quartz concentration. The particle size distribution of sand 1 had the highest d50 value whereas sand 2 had the lowest (Table 3). Similar average particle size was found for the sediment samples. In these samples, the d50 differed only by 80 µm. Moreover, the TOC concentrations for both sand samples are equal within their standard deviations (SDs). Compared to the sand samples, the sediment samples had a higher TOC. The highest TOC was found in sediment 2 and was ∼5 mg/kg above the TOC of sediment 1.
The powdered matrices were examined by XRD to determine their mineral composition (Table 3). The results show, that sand 1, sand 2, and sediment 1 contained almost equal amounts of quartz (mean: 85%). Sediment 2 had a lower quartz concentration than the other matrices. Additionally, a higher kaolinite concentration of 3.5% was found in sediment 2. Feldspar minerals, such as plagioclase and orthoclase, were identified in all matrices. Quantification of the individual feldspar minerals was not carried out.
Electrostatic separation has reduced the initial mass of all matrices by at least 69% after one separation step (Figure 1). Nevertheless, clear differences between sand samples and sediment samples were found. Sand samples had higher mass reductions of 98.90 ± 0.21% (sand 1, mean ± standard deviation) and 99.1 ± 0.4% (sand 2) than sediment samples. In sediment 1 and sediment 2, the mass reduction was 69.7 ± 0.1% and 78 ± 6%, respectively. The additional density separation led to a total mass reduction above 99% for the sediment samples.
FIGURE 1. Mass reduction of the investigated particulate matrices by electrostatic separation (ES) and subsequent density separation (DS) of sediment 1 and sediment 2.
During the electrostatic separation process, a loss of sample mass was observed. For all matrices, the fine dust particles settled on the interior part of the KWS-XL and were not separated correctly. Fine particles accumulated on the metal scrapper. These particles were transferred into the sample fraction after the separation process was completed. The particles attached to the rest of the interior of the separator were discarded and counted as sample loss. This loss was 0.46 ± 0.23% (sand 1, mean ± SD), 0.31 ± 0.07% (sand 2), 0.47 ± 0.17% (sediment 1), and 0.97 ± 0.05% (sediment 2) of the initial sample mass. It was also observed, that the matrix particles whirled up when entering the electric field of the corona electrode. This interaction increased dust formation during the separation. Further, we found that control of the relative humidity in the separation chamber was an important factor to obtain reproducible results. A difference of 5% in relative humidity could lead to a difference in mass reduction for the same particulate matrix. Here a higher relative humidity in the separation chamber led to a higher mass reduction.
DSC measurements were conducted for identification and quantification of the microplastic particles in the polymer-rich sample fraction after separation. The parallel identification of the spiked microplastic types PCL, LD-PE, and PET by the peak temperatures was successful (Figure 2). The SD of the peak temperatures was below 1.6 K for all peaks except for the crystallization peak temperature of PET. Here the SD was 7 K. Moreover, no superposition of the peaks was found. Hence, the calibration curves reported by Harzdorf et al. (2022) were applied to determine the polymer mass in all enriched sample fractions. The recovery rates of PCL, LD-PE, and PET from the four investigated matrices are shown in Figure 3.
FIGURE 2. Thermogram of DSC measurements to determine peak temperatures and enthalpies of PCL, LD-PE, and PET. The three polymers were measured simultaneously.
FIGURE 3. Boxplot of recovery rates of PCL, LD-PE, and PET microplastic particles from different particulate matrices (n = 5). Recovery rates were determined by DSC measurements. The SD of the data is shown as the width of the boxes.
In general, the recovery rates of the individual microplastic types showed no distinct variation in the particulate matrices. However, in terms of SD, in some cases, there were significant differences in the recovery rates of the same microplastic type in different matrices. The recoveries for PCL and LD-PE differed significantly between sediment 1 and sediment 2. In sediment 2, the average recovery rates were 14–19% higher than in sediment 1. There were also differences between sand 2 and sediment 1 for LD-PE and between sand 1 and sediment 2 for PET. No significantly different recoveries were found for the other matrices.
Polymer-specific recovery rates were observed for the different microplastic types. PCL has shown an average recovery of 74 ± 9% (mean ± SD) over all matrices (n = 4). An average recovery of 93 ± 9% and 120 ± 18% was found for LD-PE and PET, respectively. Hence, LD-PE showed a higher recovery than PCL and lower recovery rates than PET. The recovery of 120% for PET indicates an overestimation of this polymer in our analysis and will be discussed later. Within the SD, comparable recovery rates were found for all microplastic types from sand 1 only.
The mass reduction and microplastic recovery were investigated for 1,000 g samples of sand 1. After electrostatic separation, the mass reduction was 99.241 ± 0.028%. An average sample loss of 4.1 g was observed during electrostatic separation, but a large deviation between the samples was found in the range of 0.8–8.2 g per sample. A total mass reduction of 99.98 ± 0.01% was reached after density separation. The recovery rates of all polymers were in a moderate range (Figure 4). This means the recovery rates of PCL, LD-PE and PET were 50 ± 11% (mean ± SD), 114 ± 13%, and 82 ± 14%, respectively. Compared to the 100 g samples of sand 1, the mean values of the microplastic recovery were decreased for PCL and PET and increased for LD-PE. The results for LD-PE showed an overestimation by 14%.
FIGURE 4. Boxplot of the average polymer recovery of PCL, LD-PE, and PET from 100 g (n = 5) and 1,000 g samples (n = 3) from sand 1. The SD of the data is shown as the width of the boxes.
In this study, the microplastic analysis was realized by electrostatic separation followed by density separation to reach a sufficient mass reduction. Subsequently, microplastics were examined by DSC. Matrix properties such as particle size, TOC, and mineral composition might have an impact on mass reduction (Enders et al., 2020b). This was investigated by processing four different particulate matrices and comparing their mass reduction and microplastic recovery (Figure 1). Two sand samples and two sediment samples were tested.
Both sand samples were commercial sands with different average particle sizes. According to DIN 4220, sand 1 was classified as coarse sand and sand 2 as medium sand. In commercial sands, low TOC concentrations were found because these matrices were not exposed to the environment. Hence, no organic matter, such as algae, bacteria, or plant residues, increased the TOC concentration in the sand samples. On the other hand, both sediment samples were classified as medium sand but had different TOC concentrations. The different TOC in sediment samples could be attributed to different flow velocities and catchment areas of the rivers. In the case of sediment 2, the increased concentrations of TOC and particles <63 µm could be attributed to better sedimentation conditions of the Weisseritz River.
When applying electrostatic separation to particulate matrices, particle size is an important factor. The manufacturer of the applied KWS recommends a particle size >100 µm to process minerals, such as sand and sediments (Hoffmann, 2020). However, electrostatic separation achieved a high mass reduction for sand samples independent of the particle size distribution. The mass reduction of both sediment samples was significantly different even though the average particle size was similar in sediment 1 and sediment 2. Moreover, sieve analysis revealed similar concentrations of particles <63 µm despite the distinct average particle size in all sediments (Supplementary Materials SM3). The concentration of particles <63 µm was in the range of 0.5–3.3%. In comparison, silty sands contain 10–52% of particles <63 µm (DIN 4220). Thus, the low concentration of fine particles and the average particle size did not influence the mass reduction by electrostatic separation. However, a decrease in mass reduction was reported for soil samples with higher concentrations of fine particles (Enders et al., 2020b). The removal of particles <100 µm from particulate samples led to an increase in mass reduction from an initial 1% to 15% in that study (Enders et al., 2020b). Our study shows that the investigation of particulate matrices with defined ratios of particles in different size classes is necessary to evaluate and quantify the influence of particle size on electrostatic separation.
We observed a significantly different mass reduction between sand and sediment samples (Figure 1). The mass reduction of both sediment samples was about 20–30% lower than for the sand samples. Both sediment samples showed increased TOC concentrations. Thus, TOC could have influenced the mass reduction process by electrostatic separation. Elevated TOC concentrations could originate from organic material, such as plants, leaves, and roots, as these particles have different electrostatic properties than quartz particles. On the other hand, a biofilm of organic matter originating from algae or bacteria would have increased the TOC and altered the electrostatic properties of sediment particles (Flemming and Wingender, 2010; Fang et al., 2017). Biofilms may have reduced the net conductivity of the particles and thus favor the assignment of these particles to the polymer-rich fraction. Our results showed a lower mass reduction of sediments with increased TOC (3.53 ± 0.10 to 8.19 ± 0.13 mg/kg). The variation between the mass reduction of sediment 1 and sediment 2, however, did not correlate with the TOC. Sediment 1 had a lower TOC concentration, but still a lower mass reduction than sediment 2. Hence, the total mass reduction of a particulate matrix could be dependent on both TOC and the particle size distribution. The quantification of this effect was beyond the scope of this work and requires further research.
Enders et al. (2020) discussed the role of different mineral compositions in particulate matrices to influence the efficiency of electrostatic separation (Enders et al., 2020b). According to their study, the mass reduction of sand samples was disturbed in presence of small calcite particles (<50 µm) (Enders et al., 2020b). In our study, varieties in the mineral composition of our matrices were determined (Table 4). However, XRD measurements did not identify calcite in the matrices. We found quartz to be the main mineral in all matrices. Different concentrations of feldspar were detected, as well as an increased kaolinite concentration in sediment 2. Peretti et al. (2012) reported that feldspar and quartz minerals could be separated electrostatically, with different recoveries (Peretti et al., 2012). These experiments were carried out in a free-fall separation chamber instead of a KWS. The separation of different minerals required a triboelectric charge, which occurs only to a limited extent during the separation with a KWS (Oberrauner, 2012; Peretti et al., 2012; Mirkowska et al., 2016; Gehringer, 2021). Moreover, clay particles consist typically of kaolinite and show diameters <2 µm. Such small particles cannot be removed from the metal drum by the drum scraper. Adhered clay particles on the metal drum could form an insulating layer, which would have led to a decrease in separation efficiency. Nevertheless, from our results, no correlation between mass reduction and mineral composition could be derived. Sediment 2 had a different mineral composition than the other matrices, but the obtained mass reduction showed no abnormalities despite an increased SD. Hence, the variance of mass reduction was more likely to originate from the distinct particle size distribution and TOC content in the particulate matrices.
The observed differences between the mass reduction of sand and sediment samples were contrary to the results of Felsing et al. (2018). In their study, equal mass reductions for particulate matrices were found independent of particle size and TOC after three separation steps (Felsing et al., 2018). Still, the experimental set-up between this study and the studies of Felsing et al. (2018) and Enders et al. (2020) was different in terms of high voltage, the definition of the polymer-rich sample fraction, and the number of separation steps (Felsing et al., 2018; Enders et al., 2020b). Further, the humidity of air can influence electrostatic separation (Manouchehri, 2000). Therefore, separation was carried out at a relative humidity of 35–40%. Other studies did not report the air humidity during electrostatic separation, which hampers comparisons. Nevertheless, electrostatic separation removed >70% of the initial sample mass of different particulate matrices. Subsequent density separation has been inevitable for the identification of microplastics. An efficient electrostatic separation, however, can significantly reduce the required volume of separation medium for density separation. Scale-up experiments have shown that equal mass reduction was reached independently from the sample mass. This again shows the potential of electrostatic separation. In combination with density separation, this process was efficient to extract microplastics from large particulate samples. Furthermore, lipophilic separation could be further used as an alternative to density separation to increase the greenness of microplastic analysis (Mani et al., 2019; Lechthaler et al., 2020).
DSC measurements successfully identified spiked microplastic particles after their extraction from particulate matrices (Figure 2). PCL, LD-PE, and PET particles were identified simultaneously in the same DSC measurement. Moreover, the simultaneous presence of these polymers did not influence their melting and crystallization signals. Hence, the calibration curves reported by Harzdorf et al. (2022) were applied to determine the recovery rates of microplastic.
In a few cases, the matrix could have influenced the recovery of the same microplastic type. This accounted most dominantly for sediment samples. These samples were enriched by additional density separation. A loss of microplastic could have occurred in sediment 1, which was the sample with the lowest mass reduction after electrostatic separation. The sample mass after electrostatic separation was ∼30 g. Possibly, shaking the separation funnel was not sufficient to separate microplastic and sediment particles in such large samples. This could explain the lower recoveries for sediment 1. Thus, an increase in the recovery of microplastic from such samples could be achieved by applying a spiral conveyor for mixing (Enders et al., 2020a). For the same reason, the different recovery rates of LD-PE from sand 2 and sediment 1 could have occurred. Therefore, matrix properties could have a higher impact on the enrichment of particulate matrices than on the microplastic determination by DSC.
We found that the recovery of microplastics seems to be polymer-specific (Figure 3, Figure 4). Significant differences in the recovery rate were found in 100 g samples and scale-up experiments. It was most striking that the average recovery of PET was >100% in 100 g samples. Sample heterogeneity by granular convection and contamination from environmental samples and sample handling could not lead to such overestimation. Samples for DSC measurements were randomly taken from the polymer-rich fraction. Moreover, measures for quality assurance have reduced the risk of contamination. Accordingly, polymer-specific properties of PET itself were the most likely explanation. PET has a more sterically demanding chemical structure than LD-PE and PCL. For this reason, the ratio of crystalline and amorphous phases of PET depends on its thermal processing (Demirel et al., 2011). Additionally, this change of crystallinity and hence the change of the phase transition enthalpies could have been influenced by the presence of quartz dust and organic matter during melting and crystallization. An increase of phase transition enthalpy compared to the calibration data would then result in the observed overestimation of PET.
Harzdorf et al. (2022) proposed an influence of hetero-nucleation with fine sediment particles. The high SD of the PET melting peak temperature could indicate that phase transition processes were influenced (Figure 2). However, this hypothesis requires experimental evidence, which is still to be done. Nevertheless, in scale-up experiments, no overestimation of PET occurred. There could have been a higher loss of PET due to the additional density separation in the scale-up experiments. Furthermore, additional density separation reduced the quartz dust fraction and thus decreased the chance of hetero-nucleation. Therefore, the hypothesis of hetero-nucleation favoring a change of crystallinity was supported. To what extent hetero-nucleation influences the reliability of the results should be evaluated further.
The recovery rates of LD-PE were ∼100% in both experimental setups. Yet, the scale-up experiments showed an overestimation of 14%, which cannot be explained by the SD of the data. A calculation of the uncertainty of the whole process was conducted (Supplementary Materials SM4) and estimated at ±50% for a sample mass of 1 kg. The uncertainty of DSC measurement particularly contributed to the total uncertainty. Inter-laboratory tests have shown a relative standard uncertainty of 7–13% for DSC measurements (Wampfler et al., 2022). Including these data, an overestimation of 14% could be explained by the uncertainty of DSC measurements and the calibration data.
Moreover, PCL was tested as a potential internal standard for quality assurance. We showed that the recovery of PCL was below those of LD-PE and PET. However, the recovery rates could be dependent on the polymer type. Hence, the application of PCL internal standards to represent the recovery of all polymers was limited. Nevertheless, PCL as an internal standard is still promising because of its low recovery rates (Figure 3). It could be used as a benchmark for the minimum recovery in measurements of environmental samples.
The scale-up experiments had similar recovery rates as for 100 g samples, except for PET samples (Figure 4). Hence, electrostatic separation of microplastic and particulate matrices was possible for samples with masses up to 1 kg. A further increase in sample mass could be possible. The implementation of density separation increased the mass reduction to a sample size that could be analyzed by DSC measurements. This would be possible with an even lower volume of separation solution than 100 ml (Enders et al., 2020a). However, the recovery rates of PET and PCL were significantly lower than for LD-PE. Possibly, the recovery of PET and PCL was reduced due to the smaller density difference to the applied potassium iodide solution. Potassium iodide was applied because of its reduced hazardous properties compared to zinc chloride or sodium polytungstate. The application of separation solutions with a density >1.7 g/cm3 may increase the recovery of PET and PCL. Furthermore, lipophilic separation can overcome the limitations of density separation as mentioned before.
Recovery rates were determined for a microplastic content of 75 mg/kg (ppm) in the scale-up experiments. This content was much higher than in other environmental samples (Klein et al., 2015; Adomat and Grischek, 2021). However, theoretical LOQs for semi-crystalline polymers of 2.3 mg microplastic in 1 kg sediment were calculated for this method (Supplementary Materials SM5), assuming an average mass reduction of 99.7% and recovery of 86% (Way et al., 2022).
The present study facilitates a proof of concept for the application of electrostatic separation and DSC for microplastic analysis in particulate matrices. We conclude that electrostatic separation allows a fast treatment of representative samples for microplastic analysis in sediments. DSC facilitates robust microplastic determination for particulate matrices with high microplastic contaminations. Such samples could come from harbor basins, city beaches, or river sections with low flow rates. Hence, comprehensive microplastic monitoring could be realized with this method. However, there is a certain risk of microplastic loss during enrichment and uncertainty of microplastic determination by DSC. Further adjustments to the method can help to overcome these limitations and should focus on the following aspects:
- The impact of air humidity on electrostatic separation of microplastic and sediment.
- A quantification of matrix-related effects by TOC and fine particles.
- Application of green and sustainable alternatives to density separation.
- Polymer-specific behaviors during the DSC measurements in complex matrices.
- Investigation of the recoveries for more microplastic types, including PCL.
The original contributions presented in the study are included in the article/Supplementary Material, further inquiries can be directed to the corresponding author.
LK, SS, KH, and MS designed the study, LK, MH, and YA conducted lab and field work, LK and SS analyzed the data, LK wrote the manuscript with contributions from and final approval of all authors.
This study was supported by the European Social Fund (ESF) and by the Federal State of Saxony (Project VEMIWA—Vorkommen und Verhalten von Mikroplastik in sächsischen Gewässern; Grant No. 100382142). Open Access publication was supported by the Deutsche Forschungsgemeinschaft DFG (Grant No. GO 3639/1-1) and the Forschungsinnovationsfonds (FINF) of the University of Applied Sciences Dresden.
The authors want to thank Dr. Udo Steiner from the University of Applied Sciences for XRD measurements and the support in data analysis and interpretation. Moreover, the author thank the two editors for the proofreading.
The authors declare that the research was conducted in the absence of any commercial or financial relationships that could be construed as a potential conflict of interest.
All claims expressed in this article are solely those of the authors and do not necessarily represent those of their affiliated organizations, or those of the publisher, the editors and the reviewers. Any product that may be evaluated in this article, or claim that may be made by its manufacturer, is not guaranteed or endorsed by the publisher.
The Supplementary Material for this article can be found online at: https://www.frontiersin.org/articles/10.3389/fenvs.2022.1032005/full#supplementary-material
Adomat, Y., and Grischek, T. (2021). Sampling and processing methods of microplastics in river sediments - a review. Sci. Total Environ. 758, 143691. doi:10.1016/j.scitotenv.2020.143691
Akdogan, Z., and Guven, B. (2019). Microplastics in the environment: A critical review of current understanding and identification of future research needs. Environ. Pollut. 254, 113011. doi:10.1016/j.envpol.2019.113011
Anbumani, S., and Kakkar, P. (2018). Ecotoxicological effects of microplastics on biota: A review. Environ. Sci. Pollut. Res. 25, 14373–14396. doi:10.1007/s11356-018-1999-x
Andrady, A. L. (2011). Microplastics in the marine environment. Mar. Pollut. Bull. 62, 1596–1605. doi:10.1016/j.marpolbul.2011.05.030
Bellasi, A., Binda, G., Pozzi, A., Galafassi, S., Volta, P., and Bettinetti, R. (2020). Microplastic contamination in freshwater environments: A review, focusing on interactions with sediments and benthic organisms. Environments 7, 30. doi:10.3390/environments7040030
Besseling, E., Quik, J. T. K., Sun, M., and Koelmans, A. A. (2017). Fate of nano- and microplastic in freshwater systems: A modeling study. Environ. Pollut. 220, 540–548. doi:10.1016/j.envpol.2016.10.001
Bitter, H., and Lackner, S. (2020). First quantification of semi-crystalline microplastics in industrial wastewaters. Chemosphere 258, 127388. doi:10.1016/j.chemosphere.2020.127388
Das, S., Kohnlechner, R., Aman, F., and Dascalescu, L. (2010). Corona separation of fly ash. IEEE Trans. Ind. Appl. 46, 2157–2164. doi:10.1109/TIA.2010.2071170
De-la-Torre, G. E., Dioses-Salinas, D. C., Pizarro-Ortega, C. I., and Santillán, L. (2021). New plastic formations in the Anthropocene. Sci. Total Environ. 754, 142216. doi:10.1016/j.scitotenv.2020.142216
Demirel, B., Yaras, A., and Elçiçek, H. (2011). Crystallization behavior of PET materials. BAÜ fen. Bil. Enst. Derg. Cilt 13, 26–35.
Dioses-Salinas, D. C., Pizarro-Ortega, C. I., and De-la-Torre, G. E. (2020). A methodological approach of the current literature on microplastic contamination in terrestrial environments: Current knowledge and baseline considerations. Sci. Total Environ. 730, 139164. doi:10.1016/j.scitotenv.2020.139164
Enders, K., Lenz, R., Ivar do Sul, J. A., Tagg, A. S., and Labrenz, M. (2020a). When every particle matters: A QuEChERS approach to extract microplastics from environmental samples. MethodsX 7, 100784. doi:10.1016/j.mex.2020.100784
Enders, K., Tagg, A. S., and Labrenz, M. (2020b). Evaluation of electrostatic separation of microplastics from mineral-rich environmental samples. Front. Environ. Sci. 8, 346. doi:10.3389/fenvs.2020.00112
Fang, H., Chen, Y., Huang, L., and He, G. (2017). Biofilm growth on cohesive sediment deposits: Laboratory experiment and model validation. Hydrobiologia 799, 261–274. doi:10.1007/s10750-017-3224-1
Felsing, S., Kochleus, C., Buchinger, S., Brennholt, N., Stock, F., and Reifferscheid, G. (2018). A new approach in separating microplastics from environmental samples based on their electrostatic behavior. Environ. Pollut. 234, 20–28. doi:10.1016/j.envpol.2017.11.013
Flemming, H.-C., and Wingender, J. (2010). The biofilm matrix. Nat. Rev. Microbiol. 8, 623–633. doi:10.1038/nrmicro2415
Gehringer, S. (2021). Leoben: Montanuniversität, Lehrstuhl für Aufbereitung und Veredlung. Berlin: Zu Fragen einer kontrollierten Aufladung von Mineraloberflächen für eine erfolgreiche Trennung im elektrostatischen Feld. Dissertation.
Hanvey, J. S., Lewis, P. J., Lavers, J. L., Crosbie, N. D., Pozo, K., and Clarke, B. O. (2017). A review of analytical techniques for quantifying microplastics in sediments. Anal. Methods 9, 1369–1383. doi:10.1039/C6AY02707E
Hartmann, N. B., Hüffer, T., Thompson, R. C., Hassellöv, M., Verschoor, A., Daugaard, A. E., et al. (2019). Are we speaking the same language? Recommendations for a definition and categorization framework for plastic debris. Environ. Sci. Technol. 53, 1039–1047. doi:10.1021/acs.est.8b05297
Harzdorf, J., Zeumer, R., Schirrmeister, S., Adomat, Y., Kurzweg, L., Faist, S., et al. (2022). Mikroplastik in sächsischen Gewässern. Schriftenreihe des LfULG, 1–147.
Hoffmann, K. (2020). Bedienungsanleitung (manual) - elektrostatischer korona- abscheider typ KWS-XS. Prenzberg: Hamos GmbH.
Horton, A. A., and Dixon, S. J. (2018). Microplastics: An introduction to environmental transport processes. WIREs Water 5, 419. doi:10.1002/wat2.1268
Imhof, H. K., Schmid, J., Niessner, R., Ivleva, N. P., and Laforsch, C. (2012). A novel, highly efficient method for the separation and quantification of plastic particles in sediments of aquatic environments. Limnol. Oceanogr. Methods 10, 524–537. doi:10.4319/lom.2012.10.524
Kallenbach, E. M. F., Rødland, E. S., Buenaventura, N. T., and Hurley, R. (2022). “Microplastics in terrestrial and freshwater environments,” in Microplastic in the environment: Pattern and process. Editor M. S. Bank (Cham: Springer International Publishing), 87–130. doi:10.1007/978-3-030-78627-4_4
Kane, I. A., and Clare, M. A. (2019). Dispersion, accumulation, and the ultimate fate of microplastics in deep-marine environments: A review and future directions. Front. Earth Sci. 7, 2241. doi:10.3389/feart.2019.00080
Kawecki, D., and Nowack, B. (2019). Polymer-specific modeling of the environmental emissions of seven commodity plastics as macro- and microplastics. Environ. Sci. Technol. 53, 9664–9676. doi:10.1021/acs.est.9b02900
Kittner, M., Kerndorff, A., Ricking, M., Bednarz, M., Obermaier, N., Lukas, M., et al. (2022). Microplastics in the danube river basin: A first comprehensive screening with a harmonized analytical approach. ACS Est. Water 2, 1174–1181. doi:10.1021/acsestwater.1c00439
Klein, S., Worch, E., and Knepper, T. P. (2015). Occurrence and spatial distribution of microplastics in river shore sediments of the rhine-main area in Germany. Environ. Sci. Technol. 49, 6070–6076. doi:10.1021/acs.est.5b00492
Köhnlechner, R., and Sander, S. (2009). Praktischer einsatz elektrostatischer separatoren in der Sekundärrohstoffindustrie. Berg. Huettenmaenn Monatsh 154, 136–139. doi:10.1007/s00501-009-0453-2
Köhnlechner, R. (1999). Triboelectric charging and electrostatic separation of diverse, non-conductive mixed waste, especially plastic, CA2397506A1.
Lechthaler, S., Hildebrandt, L., Stauch, G., and Schüttrumpf, H. (2020). Canola oil extraction in conjunction with a plastic free separation unit optimises microplastics monitoring in water and sediment. Anal. methods Adv. methods Appl. 12, 5128–5139. doi:10.1039/D0AY01574A
Mai, L., Bao, L.-J., Shi, L., Wong, C. S., and Zeng, E. Y. (2018). A review of methods for measuring microplastics in aquatic environments. Environ. Sci. Pollut. Res. Int. 25, 11319–11332. doi:10.1007/s11356-018-1692-0
Majewsky, M., Bitter, H., Eiche, E., and Horn, H. (2016). Determination of microplastic polyethylene (PE) and polypropylene (PP) in environmental samples using thermal analysis (TGA-DSC). Sci. total Environ. 568, 507–511. doi:10.1016/j.scitotenv.2016.06.017
Mani, T., Frehland, S., Kalberer, A., and Burkhardt-Holm, P. (2019). Using castor oil to separate microplastics from four different environmental matrices. Anal. Methods 11, 1788–1794. doi:10.1039/C8AY02559B
Manouchehri, H.-R. (2000). Triboelectric charge characteristics and electrical separation of industrial minerals. Beijing: Ulea: University of Technology, Department of Chemical and Metallurgical Engineering. Dissertation.
Mirkowska, M., Kratzer, M., Teichert, C., and Flachberger, H. (2016). Principal factors of contact charging of minerals for a successful triboelectrostatic separation process – A review. Berg. Huettenmaenn Monatsh 161, 359–382. doi:10.1007/s00501-016-0515-1
Morin-Crini, N., Lichtfouse, E., Liu, G., Balaram, V., Ribeiro, A. R. L., Lu, Z., et al. (2022). Worldwide cases of water pollution by emerging contaminants: A review. Environ. Chem. Lett. 18, 779. doi:10.1007/s10311-022-01447-4
Nizzetto, L., Bussi, G., Futter, M. N., Butterfield, D., and Whitehead, P. G. (2016). A theoretical assessment of microplastic transport in river catchments and their retention by soils and river sediments. Environ. Sci. Process. Impacts 18, 1050–1059. doi:10.1039/C6EM00206D
Oberrauner, A. (2012). Leoben: Montanuniversität, Lehrstuhl für Aufbereitung und Veredlung. China: Nutzung der Elektroscheidung zur trockenen Aufbereitung von fein- und feinstdispersen Körnerschwärmen. Dissertation.
Peretti, R., Serci, A., and Zucca, A. (2012). Electrostatic K-feldspar/Na-feldspar and feldspar/quartz separation: Influence of feldspar composition. Mineral Process. Extr. Metallurgy Rev. 33, 220–231. doi:10.1080/08827508.2011.563156
Periyasamy, A. P., and Tehrani-Bagha, A. (2022). A review on microplastic emission from textile materials and its reduction techniques. Polym. Degrad. Stab. 199, 109901. doi:10.1016/j.polymdegradstab.2022.109901
Rodrigues, J. P., Duarte, A. C., Santos-Echeandía, J., and Rocha-Santos, T. (2019). Significance of interactions between microplastics and POPs in the marine environment: A critical overview. TrAC Trends Anal. Chem. 111, 252–260. doi:10.1016/j.trac.2018.11.038
Rodríguez Chialanza, M., Sierra, I., Pérez Parada, A., and Fornaro, L. (2018). Identification and quantitation of semi-crystalline microplastics using image analysis and differential scanning calorimetry. Environ. Sci. Pollut. Res. Int. 25, 16767–16775. doi:10.1007/s11356-018-1846-0
Schernewski, G., Radtke, H., Hauk, R., Baresel, C., Olshammar, M., Osinski, R., et al. (2020). Transport and behavior of microplastics emissions from urban sources in the baltic sea. Front. Environ. Sci. 8, 1105. doi:10.3389/fenvs.2020.579361
Shim, W. J., Hong, S. H., and Eo, S. E. (2017). Identification methods in microplastic analysis: A review. Anal. Methods 9, 1384–1391. doi:10.1039/C6AY02558G
Stock, F., Kochleus, C., Bänsch-Baltruschat, B., Brennholt, N., and Reifferscheid, G. (2019). Sampling techniques and preparation methods for microplastic analyses in the aquatic environment – a review. TrAC Trends Anal. Chem. 113, 84–92. doi:10.1016/j.trac.2019.01.014
Väinölä, R., Witt, J. D. S., Grabowski, M., Bradbury, J. H., Jazdzewski, K., and Sket, B. (2008). Global diversity of amphipods (Amphipoda; Crustacea) in freshwater. Hydrobiologia 595, 241–255. doi:10.1007/s10750-007-9020-6
Wampfler, B., Affolter, S., Ritter, A., and Schmid, M. (2022). Measurement uncertainty in analysis of plastics: Evaluation by interlaboratory test results. Cincinnati, Ohio: Hanser Publications.
Way, C., Hudson, M. D., Williams, I. D., and Langley, G. J. (2022). Evidence of underestimation in microplastic research: A meta-analysis of recovery rate studies. Sci. Total Environ. 805, 150227. doi:10.1016/j.scitotenv.2021.150227
Weber, C. J., Opp, C., Prume, J. A., Koch, M., Andersen, T. J., and Chifflard, P. (2021). Deposition and in-situ translocation of microplastics in floodplain soils. Sci. Total Environ. 819, 152039. doi:10.1016/j.scitotenv.2021.152039
Keywords: microplastic, monitoring, sediment, electrostatic separation, DSC, recovery, differential scanning calorimetry
Citation: Kurzweg L, Schirrmeister S, Hauffe M, Adomat Y, Socher M and Harre K (2022) Application of electrostatic separation and differential scanning calorimetry for microplastic analysis in river sediments. Front. Environ. Sci. 10:1032005. doi: 10.3389/fenvs.2022.1032005
Received: 30 August 2022; Accepted: 30 September 2022;
Published: 17 October 2022.
Edited by:
Jun Zhou, University of Massachusetts Lowell, United StatesReviewed by:
Omowunmi H. Fred-Ahmadu, Covenant University, NigeriaCopyright © 2022 Kurzweg, Schirrmeister, Hauffe, Adomat, Socher and Harre. This is an open-access article distributed under the terms of the Creative Commons Attribution License (CC BY). The use, distribution or reproduction in other forums is permitted, provided the original author(s) and the copyright owner(s) are credited and that the original publication in this journal is cited, in accordance with accepted academic practice. No use, distribution or reproduction is permitted which does not comply with these terms.
*Correspondence: Lucas Kurzweg, bHVjYXMua3VyendlZ0BodHctZHJlc2Rlbi5kZQ==; Kathrin Harre, a2F0aHJpbi5oYXJyZUBodHctZHJlc2Rlbi5kZQ==
Disclaimer: All claims expressed in this article are solely those of the authors and do not necessarily represent those of their affiliated organizations, or those of the publisher, the editors and the reviewers. Any product that may be evaluated in this article or claim that may be made by its manufacturer is not guaranteed or endorsed by the publisher.
Research integrity at Frontiers
Learn more about the work of our research integrity team to safeguard the quality of each article we publish.