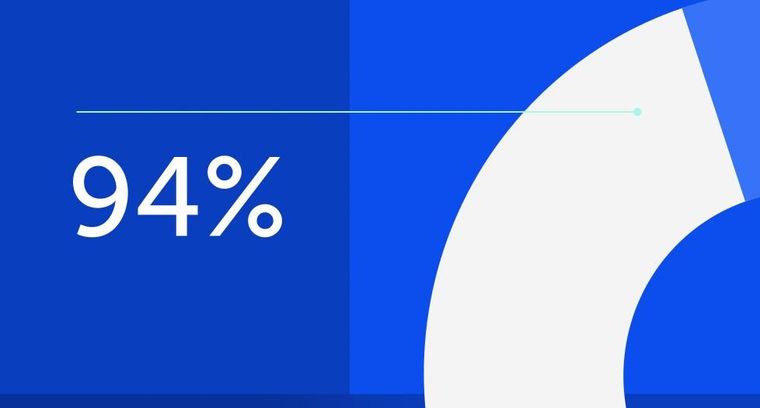
94% of researchers rate our articles as excellent or good
Learn more about the work of our research integrity team to safeguard the quality of each article we publish.
Find out more
EDITORIAL article
Front. Environ. Sci., 21 September 2022
Sec. Environmental Informatics and Remote Sensing
Volume 10 - 2022 | https://doi.org/10.3389/fenvs.2022.1028307
This article is part of the Research TopicEmerging Technologies and Techniques for Remote Sensing of Coastal and Inland WatersView all 14 articles
Editorial on the Research Topic
Emerging Technologies and Techniques for Remote Sensing of Coastal and Inland Waters
Coastal and inland waters are critical resources of immense economic and environmental value (e.g., Ledoux and Turner, 2002; Revenga and Kura, 2003). However, due to natural and anthropogenic factors, coastal and inland waters around the globe are under increasing stress. The health and biophysical status of these ecosystems need to be regularly monitored in order to ensure that they maintain their ecological functionality and services. This includes estimating concentrations of organic and inorganic constituents in water (Hernes and Benner 2003; Spencer et al., 2012), monitoring the health and distribution of submerged aquatic vegetation (Maxwell et al., 2017) and corals (Hedley et al., 2016a, and references therein), characterizing the biodiversity of phytoplankton (Mouw et al., 2017), and tracking spatio-temporal dynamics of complex biophysical and biogeochemical processes occurring in the water and adjoining wetlands (Howarth et al., 2011).
Remote sensing has become an indispensable tool for monitoring coastal and inland waters (Turpie et al., 2021, and references therein), and hyperspectral remote sensing has gained increased use in the last decade (Dierssen et al., 2015). The optical complexity typically encountered in coastal and inland waters necessitates hyperspectral sensors with a fine spectral resolution. Hyperspectral capability enables species discrimination of aquatic vegetation (Dierssen et al., 2015; Hedley J. et al., 2016) and detection of fine reflectance features of biogenic and inorganic substances in water (Mouw et al., 2016) and accessory pigments such as phycocyanin and phycoerythrin that occur in significant amounts during bloom conditions (Kudela et al., 2015). In addition to hyperspectral capability, a fine spatial resolution is needed to capture spatial heterogeneity of bio-optical features in waters where spatial variability may occur in scales as fine as a few meters (Moses et al., 2016). Sensors with hyperspectral capability and/or high spatial resolution are being used for coastal and inland water applications from a variety of platforms such as moorings, shipboard platforms, unmanned aerial vehicles (UAVs); Figure 1, and airborne and spaceborne systems.
FIGURE 1. Data acquisition using a UAV over the Choptank River, a tributary of the Chesapeake Bay, Maryland. The multispectral imagery acquired were used to estimate water quality parameters such as the concentrations of chlorophyll-a and suspended particulate matter.
Several spaceborne sensors, including CubeSats, with high resolution in the spatial and/or spectral domains that are suitable for coastal and inland water remote sensing have been launched recently or are scheduled to be launched in the near future (e.g., the Plankton, Aerosol, Cloud, ocean Ecosystem (PACE) mission; HawkEye). Current spaceborne assets have either the required spectral resolution (e.g., Hyperion; the Italian mission PRISMA - PRecursore IperSpettrale della Missione Applicativa) or spatial resolution (e.g., WorldView-3, Planet SkySat) but not both, thereby limiting their use for monitoring coastal and inland waters. This limitation is addressed through a number of software and hardware options. Advanced image processing algorithms can combine data from coarse-spatial-resolution hyperspectral data with fine-spatial-resolution multispectral data (e.g., Yokoya et al., 2017). Advanced algorithms based on radiative transfer modeling and machine learning concepts are being developed to retrieve multiple water quality parameters from airborne and spaceborne multispectral and hyperspectral data (Pahlevan et al., 2022). Hyperspectral sensors on UAVs are used to collect data at fine spatial and spectral resolutions (Joyce et al., 2018).
A special Research Topic has been dedicated to address achievements and challenges in the research, development, and application of innovative measurement technologies and water quality parameter retrieval algorithms for remote sensing of coastal and inland waters and wetlands. The following is a summary of the articles published in this Research Topic.
Submerged aquatic vegetation play a crucial ecological role primary producers and food source for marine life. Detecting their presence and estimating their biomass abundance are crucial for monitoring global biodiversity. McPherson et al. (2022) developed a rapid survey system based on UAV and diver imagery to estimate kelp canopy biomass. They successfully estimated canopy biomass in a third of their survey sites but encountered challenges due to differences in kelp patch-specific spatial characteristics across the survey sites and limitations of the survey design. They recommend optimal survey design strategies for successful retrieval of kelp canopy biomass from a UAV platform. Cavanaugh et al. (2021) developed an automated method to map kelp canopy using data from a five-channel multispectral sensor on a UAV. They reported 93% accuracy and noted that canopy cover estimates are affected by tides and currents. Wilson et al. (2020) used a combination of spectral indices and supervised image classifiers to map the presence of submerged seagrass and surface-canopy forming seaweed habitats in various water types in Atlantic Canada using data from the Multispectral Imager (MSI) onboard the Sentinel-2 satellite. The benthic habitat map had an overall accuracy of 79%.
Harmful algal blooms (HABs) in coastal and inland waters are a major environmental and health concern (e.g., Carmichael, 1997). Detecting the presence of HABs and monitoring their spread, especially in recreational areas and near drinking water intakes are critically important. Myer et al. (2020) developed a spatio-temporal model based on a hierarchical Bayesian approach to forecast the occurrence of cyanobacterial HABs in lakes across Florida. The model used data from the Ocean and Land Colour Instrument (OLCI) onboard the satellite Sentinel-3 and a number of environmental parameters. Sharp et al. (2021) investigated spatial scales of variability of the distribution of cyanobacteria in Clear Lake, California using data collected through a range of modalities including field spectrometers, autonomous underwater vehicles, UAVs, and spaceborne sensors, and discovered that the critical scale of variability is in the range of 75–175 m, consistent with previous studies.
The National Aeronautics and Space Administration (NASA) routinely conducts technology concept demonstrations to test technologies for future spaceborne missions. Guild et al. (2020) summarized results from a number of NASA-sponsored airborne experiments conducted over Monterey Bay, California and nearby inland waters, involving radiometers, sun photometers, and imaging spectrometers. The experiments demonstrated the utility and enhanced benefit from the combined use of various measurement modalities and provided valuable data for calibrating and validating algorithms for retrieving water quality parameters. Harringmeyer et al. (2021) used a partial least squares regression approach involving reflectance in the ultraviolet, blue, and near-infrared regions to detect the presence of colored dissolved organic matter (CDOM) and differentiate it from phytoplankton in water. The study was carried out in Santa Monica Bay using data from the airborne Portable Remote Imaging SpectroMeter (PRISM). The study illustrated the value of reflectance in the ultraviolet region for detecting CDOM.
Coastal wetlands are a critical part of the global ecosystem and provide valuable environmental and ecological services including storm surge protection, coastal erosion control, carbon sequestration, and habitat for a number of endangered species. Haskins et al. (2021) demonstrated the use of UAV-based techniques for monitoring restoration of a tidal marsh site in Elkhorn Slough, California by calculating the volume of soil moved, tracking whether elevation targets were achieved or not, quantifying and examining the patterns of vegetation development, and monitoring topographic change including subsidence, erosion, and creek development. Elmahdy et al. (2020) used three machine learning algorithms, namely, Random Forest, kernel logistic regression, and Naïve Bayes algorithm, to map mangrove forests in the eastern and western coastal areas of the United Arab Emirates using Landsat data over the period from 1990 to 2019. They found that the Random Forest method performed the best, and noted that there was a significant change in mangrove extent during the 2010–2019 decade compared to 1990–2000.
Ayad et al. (2020) used data from multiple spaceborne sensors, namely RapidEye, MSI, and the Operational Land Imager (OLI) onboard Landsat-8 to detect discharges of stormwater and wastewater into the coastal ocean in Southern California and differentiate between stormwater and wastewater plumes based on optical characteristics. Kravitz et al. (2021) developed a tool based on synthetic data and machine learning techniques to retrieve multiple water quality parameters from satellite data. The tool was used to successfully retrieve concentrations of chlorophyll-a and phycocyanin and absorption coefficient of phytoplankton from MSI and OLI data acquired over Hartbeespoort Dam, South Africa.
Windle et al. (2021) investigated methods to remove the effects of surface-reflected radiance (including sun glint) on UAV-measured data. They recommend a pixel-based approach that uses absorption characteristics of water in the near-infrared region to estimate and remove surface reflectance. Dierssen et al. (2021) provide a comprehensive review and commentary on the past, present, and future state of hyperspectral aquatic remote sensing, addressing advances in sensor design, modes of deployment, algorithm development, image analysis techniques, and open-source software, which make data and techniques available and easily accessible to the public.
These articles demonstrate that aquatic remote sensing is a fast-evolving field with rapid advances in sensor technologies, measurement modalities, and innovative algorithms. These advances enable us to address critical environmental and ecological questions related to coastal and inland water ecosystems.
This Research Topic was conceived and developed by WM, AV, and SP. All three editors equally participated in editing manuscripts submitted to this Research Topic and made decisions on the manuscripts in consultation with each other and with unanimity.
The editors’ contributions to writing the editorial and editing the articles published in this Research Topic were supported by internal funds from their respective host institutes, namely, the U.S. Naval Research Laboratory, the National Oceanic and Atmospheric Administration Great Lakes Environmental Research Laboratory, and the California State University Monterey Bay.
The authors declare that the research was conducted in the absence of any commercial or financial relationships that could be construed as a potential conflict of interest.
All claims expressed in this article are solely those of the authors and do not necessarily represent those of their affiliated organizations, or those of the publisher, the editors and the reviewers. Any product that may be evaluated in this article, or claim that may be made by its manufacturer, is not guaranteed or endorsed by the publisher.
Carmichael, W. W. (1997). The cyanotoxins. Adv. Botanical Res. 2727, 211–256. doi:10.1016/s0065-2296(08)60282-7
Dierssen, H. M., Chlus, A., and Russell, B. (2015). Hyperspectral discrimination of floating mats of seagrass wrack and the macroalgae Sargassum in coastal waters of Greater Florida Bay using airborne remote sensing. Remote Sens. Environ. 167, 247–258. doi:10.1016/j.rse.2015.01.027
Hedley, J. D., Roelfsema, C. M., Chollett, I., Harborne, A. R., Heron, S. F., Weeks, J., et al. (2016a). Remote sensing of coral reefs for monitoring and management: A review. Remote Sens. 8 (2), 118. doi:10.3390/rs8020118
Hedley, J., Russell, B., Randolph, K., and Dierssen, H. (2016b). A physics-based method for the remote sensing of seagrasses. Remote Sens. Environ. 174, 134–147. doi:10.1016/j.rse.2015.12.001
Hernes, P., and Benner, R. (2003). Photochemical and microbial degradation of dissolved lignin phenols: Implications for the fate of terrigenous dissolved organic matter in marine environments. J. Geophys. Res. 108 (C9), 3291. doi:10.1029/2002JC001421
Howarth, R., Chan, F., Conley, D. J., Garnier, J., Doney, S. C., Marino, R., et al. (2011). Coupled biogeochemical cycles: Eutrophication and hypoxia in temperate estuaries and coastal marine ecosystems. Front. Ecol. Environ. 9 (1), 18–26. doi:10.1890/100008
Joyce, K. E., Duce, S., Leahy, S. M., Leon, J., and Maier, S. W. (2018). Principles and practice of acquiring drone-based image data in marine environments. Mar. Freshw. Res. 70 (7), 952–963. doi:10.1071/MF17380
Kudela, R. M., Palacios, S. L., Austerberry, D. C., Accorsi, E. K., Guild, L. S., and Torres-Perez, J. (2015). Application of hyperspectral remote sensing to cyanobacterial blooms in inland waters. Remote Sens. Environ. 167, 196–205. doi:10.1016/j.rse.2015.01.025
Ledoux, L., and Turner, R. K. (2002). Valuing Ocean and coastal resources: A review of practical examples and issues for further action. Ocean Coast. Manag. 45 (9-10), 583–616. doi:10.1016/s0964-5691(02)00088-1
Maxwell, P. S., Eklöf, J. S., van Katwijk, M. M., O'Brien, K. R., de la Torre‐Castro, M., Boström, C., et al. (2017). The fundamental role of ecological feedback mechanisms for the adaptive management of seagrass ecosystems–a review. Biol. Rev. 92 (3), 1521–1538. doi:10.1111/brv.12294
Moses, W. J., Ackleson, S. G., Hair, J. W., Hostetler, C. A., and Miller, W. D. (2016). Spatial scales of optical variability in the coastal ocean: Implications for remote sensing and in situ sampling. J. Geophys. Res. Oceans 121, 4194–4208. doi:10.1002/2016jc011767
Mouw, C. B., Greb, S., Aurin, D., DiGiacomo, P. M., Lee, Z., Twardowski, M., et al. (2016). Aquatic color radiometry remote sensing of coastal and inland waters: Challenges and recommendations for future satellite missions. Remote Sens. Environ. 160, 15–30. doi:10.1016/j.rse.2015.02.001
Mouw, C. B., Hardman-Mountford, N. J., Alvain, S., Bracher, A., Brewin, R. J., Bricaud, A., et al. (2017). A consumer's guide to satellite remote sensing of multiple phytoplankton groups in the global ocean. Front. Mar. Sci. 4, 41. doi:10.3389/fmars.2017.00041
Pahlevan, N., Smith, B., Alikas, K., Anstee, J., Barbosa, C., Binding, C., et al. (2022). Simultaneous retrieval of selected optical water quality indicators from Landsat-8, Sentinel-2, and Sentinel-3. Remote Sens. Environ. 270, 112860. doi:10.1016/j.rse.2021.112860
Revenga, C., and Kura, Y. (2003). Status and trends of biodiversity of inland water ecosystems. Montreal: Secretariat of the Convention on Biological Diversity.
Spencer, R. G., Aiken, G. R., Dornblaser, M. M., Butler, K. D., Holmes, R. M., Fiske, G., et al. (2012). Chromophoric dissolved organic matter export from U.S. rivers. Geophys. Res. Lett. 40, 1575–1579. doi:10.1002/grl.50357
Turpie, K. R., Ackleson, S. G., Byrd, K. B., and Moisan, T. A. (2021). Editorial: Science and applications of coastal remote sensing. Front. Mar. Sci. 8, 641029. doi:10.3389/fmars.2021.641029
Keywords: hyperspectral, multispectral, unmanned aerial vehicles (UAVs), CubeSats, machine learning, coastal and inland waters, wetlands
Citation: Moses WJ, Vander Woude AJ and Palacios SL (2022) Editorial: Emerging technologies and techniques for remote sensing of coastal and inland waters. Front. Environ. Sci. 10:1028307. doi: 10.3389/fenvs.2022.1028307
Received: 25 August 2022; Accepted: 29 August 2022;
Published: 21 September 2022.
Edited and reviewed by:
Alexander Kokhanovsky, Max Planck Institute for Chemistry, GermanyCopyright © 2022 Moses, Vander Woude and Palacios. This is an open-access article distributed under the terms of the Creative Commons Attribution License (CC BY). The use, distribution or reproduction in other forums is permitted, provided the original author(s) and the copyright owner(s) are credited and that the original publication in this journal is cited, in accordance with accepted academic practice. No use, distribution or reproduction is permitted which does not comply with these terms.
*Correspondence: Wesley J. Moses, d2VzbGV5Lm1vc2VzQG5ybC5uYXZ5Lm1pbA==
Disclaimer: All claims expressed in this article are solely those of the authors and do not necessarily represent those of their affiliated organizations, or those of the publisher, the editors and the reviewers. Any product that may be evaluated in this article or claim that may be made by its manufacturer is not guaranteed or endorsed by the publisher.
Research integrity at Frontiers
Learn more about the work of our research integrity team to safeguard the quality of each article we publish.