- 1School of Mathematics and Statistics, Hanshan Normal University, Chaozhou, China
- 2Department of Mathematics and Statistics, International Islamic University Islamabad, Islamabad, Pakistan
When any amount of harmful materials (any substance or any type of energy) is introduced into the climate at a rate quicker than it very well may be scattered or securely put away, then pollution occurs. These harmful materials are known as pollutants which can be natural and can also be manmade such as trash generated by factories. These harmful materials harm the quality of land, air, and water and cause various types of pollution, which affects the environment. In this article, we analyze the effect of various types of pollution on the environment and evaluate the most harmful type of pollution through an illustrative example by employing power Bonferroni mean (BM) operators in the setting of the bipolar complex fuzzy set (BCFS), like bipolar complex fuzzy (BCF) power BM (BCFPBM), BCF weighted power BM (BCFWPBM), BCF power geometric BM (BCFPGBM), and BCF weighted power geometric BM (BCFWPGBM) operators and a decision-making (DM) procedure created on these operators in the environment of the BCFS which are introduced in this article. Furthermore, we illustrate that the introduced operators and a DM procedure in the environment of the BCFS are more effective and have a wide model and advantages than certain prevailing works.
Introduction
According to Pure Earth (an environmental organization), over 200 million people from all over the world are affected by toxic pollution. In certain parts of the world where pollution is high, women give birth to babies with defects, children have lost
Zadeh (1965) introduced the model of the fuzzy set (FS) for addressing abstruse and ambiguous information in genuine life issues. Before the model of the FS, there was a model of a crisp set in which every element has a satisfying grade (SG), either
Zhang (1994) introduced one of the modifications of the FS called the bipolar FS (BFS). In the BFS, every element has a positive satisfying grade (PSG) between
Ramot et al. (2002) developed another modification of the FS called the complex FS (CFS) because the FS cannot handle data involving a second dimension or extra fuzzy information. In the CFS, every element has a satisfying grade in
To get a more accurate alternative method, we have to consider the given assessment information and the relationship between them. The power average (PA) and power-ordered weighted average operators were first established by Yager (2001), which incorporate variable weights. Xu and Yager (2009) used power geometric operators, and Garg et al. (2021) established PA operators in the setting of the T-spherical FS. There is another apparatus called the BM operator defined by Bonferroni (1950), which considers the interrelationship of inputs. It has been utilized in numerous fields: the geometric BM (GBM) was used by Zhu et al. (2012), the BM operator was utilized in the intuitionistic FS (IFS) by Xu and Yager (2010), and picture fuzzy BM operators were investigated by Ateş and Akay (2020). Liu and Li (2017) developed PBM operators for the interval-valued IFS (IVFS). Furthermore, PA has the ability to ignore the effect of the smallest or largest information by taking various weights, and the BM operator has the ability to consider the interrelationship of each input argument. However, in DM issues and circumstances, the PA and DM operators lack the ability to tackle the data in the model of the BCFS.
The BCFS can easily portray fuzzy data or information containing positive and negative aspects, as well as extra fuzzy information (second dimension), and is very handy in dealing with complicated DM issues and genuine life issues. The model of the BCFS is wider and richer than certain prevailing conceptions like FS, BFS, and CFS, and numerous scholars have defined various AOs in the setting of the BCFS. Mahmood et al. (2021) have defined BCF HAOs, and Mahmood and Ur Rehman (2022b) have defined DAOs for the BCF set. Mahmood et al. (2022a) initiated AOs, and Rehman et al. (2022) presented Frank AOs in the environment of the BCF set. The BM operators based on BCFS were defined by Mahmood et al. (2022b). However, these defined AOs have their drawbacks, and these operations have one common drawback; it is that these operators cannot provide a fair and unbiased weight vector to the attributes because in these operators, the decision expert provides weight vectors to each attribute on his/her own choice. In the literature, there is no such structure or operator which can overcome this dilemma. Thus, this research gap created various questions such as
• How do we get fair and unbiased decisions if the data are in the structure of the BCFS?
• How do we make decisions if the weight vector is not given and the information is in the setting of the BCFS?
• How do we get the advantages of the combination of PA and BM operators in the environment of the BCFS?
Up until now, no one has answered these questions; thus, in this study, we answer these questions by combining PA, geometric BM, and BM operators in the environment of the BCFS to introduce BCFPBM, BCFWPBM, BCFPGBM, and BCFWPGBM operators. Furthermore, we describe what we are achieving from this study.
• The structure of the BCFS is one of the most advanced and widely used structures, and up until now, no one has defined these operators in the environment of the BCFS.
• Over time, the information became more complicated and tricky and almost impossible to handle with ordinary mathematical models and operators. This study will help handle complicated and tricky data and provide a fair decision. This study is the requirement of time.
• PA, GBM, and BM operators have their advantages; this study provides us with all these advantages together.
• To show the practical advantage of this study, we investigate a genuine-life numerical example related to the environment in this study.
• By taking the arbitrary weight vector, the introduced operators would be reduced to the BM operators, as defined by Mahmood et al. (2022b). Thus, the defined operators are the modification of BM operators, as defined by Mahmood et al. (2022b).
In the Background section, we review the basic concept of pollution and its types, as well as the idea of the BCFS and its properties. In the BCF power Bonferroni mean operators section, we introduce power BM operators in the setting of BCF sets to define BCFPBM, BCFWPBM, BCFPGBM, and BCFWPGBM operators. In the Application section, we introduce a DM procedure for solving genuine life issues and then present a numerical example related to pollution and its types. In the Comparison section, we show that the introduced PBM operators (BCFPBM, BCFWPBM, BCFPGBM, and BCFWPGBM) and a DM procedure in the environment of BCFS are more effective than other prevailing operators. The Conclusion section contains the concluding remarks of this article.
Background
The word pollution originates from the Latin word polluere, which essentially implies contamination. Thus, in a simple way, pollution is something that pollutes the climate. According to Pure Earth (an environmental organization), over 200 million people from all over the world are affected by toxic pollution. Various beneficial things can cause pollution, such as generating electricity from burning coal causes pollution in the air, cars discharging pollutants through exhaust pipes, the sewage and garbage produced by homes and industries cause water and land pollution, and the use of pesticides to kill insects and weeds causes water pollution , which is harmful to wildlife. These pollutants harm the quality of land, air, and water. Various kinds of pollution can be caused by natural events and also by artificial activities. The four major types of pollution are
1) Air pollution,
2) Water pollution,
3) Soil pollution, and
4) Noise pollution.
Figure 1 portrays the types of pollution.
1) Air pollution: There is an exact synthetic structure of the air that we breathe. Its vast majority comprises oxygen, dormant gases, nitrogen, and water fume. When the pollutants or things are introduced in the air, which are not normally there, they cause air pollution. A usual kind of air pollution takes place when people emit particles of burning fuels into the air. This sort of pollution seems to be excess, having a large number of minuscule particles, drifting in the air. One more typical sort of air pollution is caused by harmful gases, like nitrogen oxides, sulfur dioxide, chemical fumes, and carbon monoxide. When these gases are introduced in the air, they can be a part of the chemical reactions and produce smog and acid rain. Over two million people die every year because of air pollution. The impact of air pollution on human well-being can fluctuate generally contingent upon the contamination. Assuming the poison is exceptionally harmful, the consequences for well-being can be far and wide extreme. For instance, the leakage of methyl isocyanate gas in Bhopal, India, in 1984, killed more than two thousand people, and in north, two hundred thousand people experienced respiratory issues.
2) Water pollution: When chemicals or other harmful substances such as sewage, fertilizers, metals, mercury, and pesticides are introduced into the water, then water pollution occurs. All over the world, 44 percent of streams,
3) Soil pollution: Household and industrial garbage and waste cause soil pollution. According to the EPA, USA generated over 258 million tons of waste and garbage in 2014. More than half, i.e., 136 million tons, of the waste was assembled in landfills, and merely 34% was composted. The effects of land pollution are not that noticeable, but its implication can easily be observed. Oil spills, industrial accidents, acid rain, improper waste disposal, mining activities, etc., are a few usual reasons for soil pollution.
4) Noise pollution: despite the fact that people cannot smell or see noise pollution, it influences the climate. When various sounds reach the harmful level, for example, sound coming from industries, jets, etc., it causes noise pollution. It is obvious that there are immediate connections between well-being and noise, causing hearing loss, increase in the blood pressure, speech interference, and pressure-related ailments. For instance, according to the WHO, thousands of people die every year because of noise pollution. Ships cause underwater noise pollution. Similarly Noise pollution influence wild species to impart stronger, which can reduce their life expectancy.
Definition 1. (Mahmood and Ur Rehman, 2022b) The theory is modeled as follows.
is known as the BCFS, where
Definition 2. (Mahmood and Ur Rehman, 2022b) For a BCFN
Definition 3. (Mahmood and Ur Rehman, 2022b) If we have two BCFNs
1)
2)
3)
4)
Definition 4. (He et al., 2014) Take a group of real numbers
Definition 5. (He et al., 2014) Take a group of real numbers
In Def (4) and (5),
1)
2)
3)
BCF power Bonferroni mean operators
In this section of this article, we introduce power BM operators in the setting of BCF sets to define BCFPBM, BCFWPBM, BCFPGBM, and BCFWPGBM operators.
Definition 6. Take a group of BCFNs
where
1)
2)
3)
Theorem 1. The aggregated outcome from Eq. 6 based on the group of BCFNs
Proof. Suppose that
First, determine
Now,
Now, determine
Next, determine
Now,
Finally, determine
Now, replace
The BCFPBM operator holds the following axioms.
1) Idempotency: Let
2) Commutativity: Let
3) Boundedness: Let a group of BCFNs
Definition 7. Take a group of BCFNs
where
Theorem 2. The aggregated outcome from Eq. 10 based on the group of BCFNs
The BCFWPBM operator holds the following axioms.
1) Idempotency: Let
2) Commutativity: Let
3) Boundedness: Let a group of BCFNs
Definition 8. Take a group of BCFNs
where
1)
2)
3)
Theorem 3. The aggregated outcome from Eq. 12 based on the group of BCFNs
Proof. Suppose that
First, determine
Now,
Now, determine
Next, determine
Now,
Finally, determine
Now, replace
The BCFPGBM operator holds the following axioms.
1) Idempotency: Let
2) Commutativity: Let
3) Boundedness: Let a group of BCFNs
Definition 9. Take a group of BCFNs
where
Theorem 4. The aggregated outcome from Eq. 16 based on the group of BCFNs
The BCFWPGBM operator holds these axioms:
1) Idempotency: Let
2) Commutativity: Let
3) Boundedness: Let a group of BCFNs
Application
In the aforementioned sections, we reviewed pollution and its types and introduced PBM operators based on the BCFS. Thus, here, we would study pollution and its types through introduced operators. As we know that pollution is a worldwide issue, big cities generally have more pollution than small cities or towns. Pollution can easily expand to those areas where no one lives. For instance, some chemicals and pesticides are observed in the Antarctic ice sheet. In the northern Pacific Ocean, a tremendous assortment of tiny plastic particle structures is called the Great Pacific Garbage Patch. Winds can carry radioactive material, inadvertently let out of an atomic reactor, and spread it over the planet. Water and air convey pollutants. Sea flows and migrating fish convey marine toxins all over. Pollution is of four major types, i.e., 1. air pollution, 2. water pollution, 3. soil pollution, and 4. noise pollution, and the causes of every type are different. Every type of pollution has a different effect on the environment. To find out the most harmful type of pollution for the environment is a DM issue. Thus, we would display how we can employ the introduced operators for the BCFS to solve this DM issue.
To employ the introduced operators for the BCFS to solve the DM issue, we define a DM procedure as follows based on the introduced operators in the environment of the BCFS.
Taking
Step 1: In genuine life issues, the information or data can be cost or benefit. Benefits do not require normalization, but costs do:
where
Step 2: After completing step 1, the aggregated values of given information or data are determined by employing any introduced operator (BCFPBM, BCFWPBM, BCFPGBM, and BCFWPGBM).
Step 3: After finding out the aggregated values, the SV of these aggregated values would be determined by employing Eq. 2, and if any two SVs are equal, then accuracy values (AVs) are determined by employing Eq. 3. Furthermore, the ranking would be made based on these SVs and AVs and would reach the best alternative.
In the following section, we solve a numerical example by employing the introduced operators and DM procedure based on the BCFS to address genuine life issues.
Numerical example
Suppose
Step 1: In this example, we do not need to perform the first step.
Step 2: The aggregated values of data in Table 1 are determined by employing introduced BCFPBM, BCFWPBM, BCFPGBM, and BCFWPGBM operators, which are explored in Table 2.
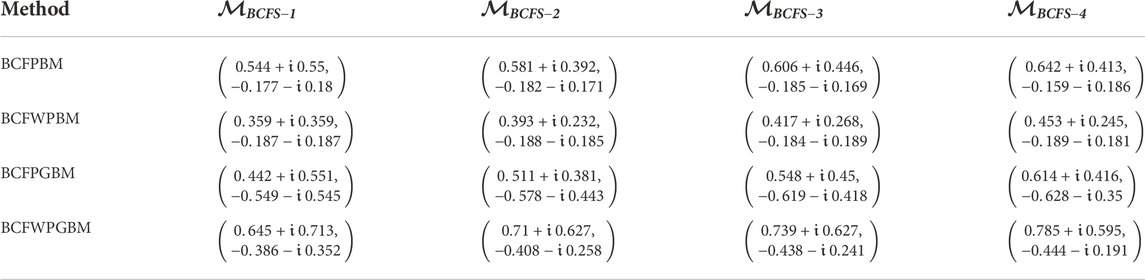
TABLE 2. Aggregated values of information are described in Table 1.
Step 3: SVs of these aggregated values determined by employing Eq. 2 are presented in Table 3.
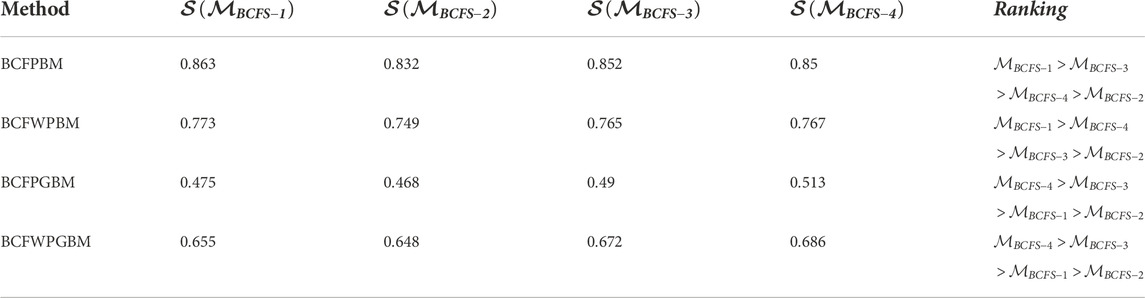
TABLE 3. SVs of the aggregated values of Table 2 and their ranking.
The rankings in Table 3 show that according to the introduced BCFPBM and BCFWPBM,
Comparison
The introduced PBM operators (BCFPBM, BCFWPBM, BCFPGBM, and BCFWPGBM) and a DM procedure in the environment of the BCFS are more effective than other prevailing operators. We now take prevailing theories, including the CF power and DM procedure defined by Hu et al. (2019), sin trigonometric AOs and the DM procedure in the environment of the BF set defined by Riaz et al. (2022), Hamacher AOs and the DM procedure in the environment of the BF set established by Wei et al. (2018), PBM AOs and the DM procedure in the setting of the interval-valued intuitionistic FS (IVIFS) introduced by Liu and Li (2017), and BM AOs and the DM procedure for the BCFS introduced by Mahmood et al. (2022b). We consider the data provided in Table 1 and aggregate it with the assistance of these prevailing theories and the operators and the DM procedure introduced in this article. The results are shown in Table 4.
The results indicate that existing theories, for example, Hu et al. (2019), Riaz et al. (2022), Wei et al. (2018), and Liu and Li (2017), cannot solve the DM issue because the data form the structure of the BCFS. Hu et al. (2019) can merely solve the data in the model of CFS and cannot tackle the negative aspects, while Riaz et al. (2022) and Wei et al. (2018) merely resolve the information in the setting of the BFS and cannot overcome the second dimension, and Liu and Li (2017) merely resolve the information in the model of IVIFS and cannot address the second dimension and negative aspects. Furthermore, the results show that BM AO (BCF Bonferroni mean AO) (Mahmood et al., 2022b) for the BCFS and the DM approach handle the data and give us the result that
Conclusion
In this article, we studied the effect of pollution and its types on the environment by employing the introduced PBM operators and the DM procedure in the setting of the BCFS. The procedure by which the water, land, air, or other components of the natural environment are made unsafe or dirty to use is known as pollution. Pollution occurs by introducing any sort of pollutant into the environment, but the pollutant need not be visible, for example, temperature, light, and sound can be taken as pollutants if introduced into the environment artificially. For this study, we introduced the PBM operator in the setting of the BCFS, which are BCFPBM, BCFWPBM, BCFPGBM, and BCFWPGBM operators. Furthermore, we defined a DM procedure based on these PBM operators in the setting of the BCF set. After that, we described a numerical example in which we took four types of pollution and determined which type of pollution is more harmful and dangerous to the environment. We found that air pollution is more harmful than others by utilizing BCFPBM and BCFWPBM operators, and water pollution is more harmful than others by utilizing BCFPGBM and BCFWPGBM operators. Moreover, we illustrated that the introduced PBM operators and a DM procedure in the environment of the BCFS are more effective and have a wide model and advantages than certain prevailing works. We also showed that the investigated PBM AOs and the DM procedure for the BCFS gave us better and fair results than the prevailing similar AOs and DM procedures. The investigated operators and the DM procedure for the BCFS have certain limitations as well like they cannot tackle the data in the structure of BCF soft sets, complex bipolar intuitionistic fuzzy sets, BCF linguistic sets, etc.
In the future, our goal would be to review various notions like the bipolar complex fuzzy soft set (SS) (Mahmood et al., 2022c), Pythagorean FS (Li et al., 2022), picture FS (Ullah, 2021), picture fuzzy SSs (Khan et al., 2019), T-spherical FS (Javed et al., 2022), complex bipolar intuitionistic FS (Jan et al., 2022), enhancing digital innovation for the sustainable transformation of manufacturing industry (Yin et al., 2022) and try to employ the introduced work in these notions.
Data availability statement
The original contributions presented in the study are included in the article/Supplementary Material; further inquiries can be directed to the corresponding author.
Author contributions
Conceptualization, XY, TM, and UU; methodology, XY, TM, and UU; software, XY, TM, and UU; formal analysis, XY, TM, and UU; investigation, XY, TM, and UU; writing—original draft, XY, TM, and UU; writing—review and editing, XY, TM, and UU.
Funding
This work is supported by the National Natural Science Foundation of China (61877014, 12271132) and the funds provided by the Department of Education of Guangdong Province (2022A1515011460, 2021ZDJS044, PNB2103, 2021B1212040015).
Conflict of interest
The authors declare that the research was conducted in the absence of any commercial or financial relationships that could be construed as a potential conflict of interest.
Publisher’s note
All claims expressed in this article are solely those of the authors and do not necessarily represent those of their affiliated organizations, or those of the publisher, the editors, and the reviewers. Any product that may be evaluated in this article, or claim that may be made by its manufacturer, is not guaranteed or endorsed by the publisher.
References
Akram, M., and Bashir, A. (2021). Complex fuzzy ordered weighted quadratic averaging operators. Granul. Comput. 6 (3), 523–538. doi:10.1007/s41066-020-00213-7
Akram, M., Sattar, A., Karaaslan, F., and Samanta, S. (2021). Extension of competition graphs under complex fuzzy environment. Complex Intell. Syst. 7 (1), 539–558. doi:10.1007/s40747-020-00217-5
Ali, Z., Mahmood, T., Ullah, K., and Khan, Q. (2021). Einstein geometric aggregation operators using a novel complex interval-valued pythagorean fuzzy setting with application in green supplier chain management. Rep. Mech. Eng. 2 (1), 105–134. doi:10.31181/rme2001020105t
Ashraf, A., Ullah, K., Hussain, A., and Bari, M. (2022). Interval-valued picture fuzzy maclaurin symmetric mean operator with application in multiple attribute decision-making. Rep. Mech. Eng. 3 (1), 301–317. doi:10.31181/rme20020042022a
Ateş, F., and Akay, D. (2020). Some picture fuzzy Bonferroni mean operators with their application to multicriteria decision making. Int. J. Intell. Syst. 35 (4), 625–649. doi:10.1002/int.22220
Bharathi, T., and Felixia, S. (2022).Bipolar felicitous fuzzy graphs. AIP Conf. Proc. AIP Publishing LLC 2385, 130031. doi:10.1063/5.0071535
Bonferroni, C. (1950). Sulle medie multiple di potenze. Boll. dell'Unione Mat. Ital. 5 (3-4), 267–270.
Chen, H., and Tian, Z. (2022). Environmental uncertainty, resource orchestration and digital transformation: A fuzzy-set QCA approach. J. Bus. Res. 139, 184–193. doi:10.1016/j.jbusres.2021.09.048
Choudhury, B. S., Dhara, P. S., and Saha, P. (2021). An application of fuzzy logic on importing medicines. Int. J. Healthc. Manag. 14 (2), 456–461. doi:10.1080/20479700.2019.1658160
De, S. K. (2020). On degree of fuzziness and fuzzy decision making. Cybern. Syst. 51 (5), 600–614. doi:10.1080/01969722.2020.1723872
Garai, T., Biswas, G., and Santra, U. (2022). “A novel MCDM method based on possibility mean and its application to water resource management problem under bipolar fuzzy environment,” in International conference on intelligent and fuzzy systems (Cham: Springer), 405–412.
Garg, H., Ullah, K., Mahmood, T., Hassan, N., and Jan, N. (2021). T-spherical fuzzy power aggregation operators and their applications in multi-attribute decision making. J. Ambient. Intell. Humaniz. Comput. 12 (10), 9067–9080. doi:10.1007/s12652-020-02600-z
Gong, S., and Hua, G. (2022). Fuzzy edge connectivity in bipolar fuzzy networks and the applications in topology design. Int. J. Intell. Syst. 37, 5425–5442. doi:10.1002/int.22797
Gulistan, M., Khan, Z., Al-Shamiri, M. M., Azhar, M., Ali, A., Madasi, J. D., et al. (2022). A new fuzzy decision support system approach; analysis and applications. AIMS Math. 7 (8), 14785–14825. doi:10.3934/math.2022812
He, Y., He, Z., Wang, G., and Chen, H. (2014). Hesitant fuzzy power Bonferroni means and their application to multiple attribute decision making. IEEE Trans. Fuzzy Syst. 23 (5), 1655–1668. doi:10.1109/tfuzz.2014.2372074
Hu, B., Bi, L., and Dai, S. (2019). Complex fuzzy power aggregation operators. London: Mathematical Problems in Engineering. doi:10.1155/2019/9064385
Jan, N., Maqsood, R., Nasir, A., Alhilal, M. S., Alabrah, A., and Al-Aidroos, N. (2022). A new approach to model machine learning by using complex bipolar intuitionistic fuzzy information. J. Funct. Spaces 2022, 1–17. doi:10.1155/2022/3147321
Jana, C., Pal, M., and Wang, J. Q. (2019). Bipolar fuzzy Dombi aggregation operators and its application in multiple-attribute decision-making process. J. Ambient. Intell. Humaniz. Comput. 10 (9), 3533–3549. doi:10.1007/s12652-018-1076-9
Javed, M., Javeed, S., Ahmad, J., Ullah, K., and Zedam, L. (2022). Approach to multiattribute decision-making problems based on neutrality aggregation operators of picture fuzzy information. J. Funct. Spaces 2022, 1–16. doi:10.1155/2022/2762067
Jiang, Z. (2022). Improved algorithm of fuzzy set gravity centre in track system teaching field. London: Mathematical Problems in Engineering. doi:10.1155/2019/9064385
Khan, M. J., Kumam, P., Ashraf, S., and Kumam, W. (2019). Generalized picture fuzzy soft sets and their application in decision support systems. Symmetry 11 (3), 415. doi:10.3390/sym11030415
Kwon, K., Kang, M., Kim, D., and Choi, H. (2022). New tunnel risk assessment model combining ahp and fuzzy set theory. Available at SSRN 4156575.
Li, H., Cao, Y., Su, L., and Wang, F. (2022). Selecting a project delivery system for wastewater treatment plants with related-indicators under a pythagorean fuzzy environment. Front. Environ. Sci. 560. doi:10.3389/fenvs.2022.883630
Liu, P., and Li, H. (2017). Interval-valued intuitionistic fuzzy power Bonferroni aggregation operators and their application to group decision making. Cogn. Comput. 9 (4), 494–512. doi:10.1007/s12559-017-9453-9
Mahmood, T., Rehman, U. U., Ahmmad, J., and Santos-García, G. (2021). Bipolar complex fuzzy Hamacher aggregation operators and their applications in multi-attribute decision making. Mathematics 10 (1), 23. doi:10.3390/math10010023
Mahmood, T., Rehman, U. U., Ali, Z., Aslam, M., and Chinram, R. (2022). Identification and classification of aggregation operators using bipolar complex fuzzy settings and their application in decision support systems. Mathematics 10 (10), 1726. doi:10.3390/math10101726
Mahmood, T., ur Rehman, U., Ali, Z., and Aslam, M. (2022). Bonferroni mean operators based on bipolar complex fuzzy setting and their applications in multi-attribute decision making. AIMS Math. 7 (9), 17166–17197. doi:10.3934/math.2022945
Mahmood, T., Rehman, U. U., Jaleel, A., Ahmmad, J., and Chinram, R. (2022). Bipolar complex fuzzy soft sets and their applications in decision-making. Mathematics 10 (7), 1048. doi:10.3390/math10071048
Mahmood, T., and Ur Rehman, U. (2022a). A novel approach towards bipolar complex fuzzy sets and their applications in generalized similarity measures. Int. J. Intell. Syst. 37 (1), 535–567. doi:10.1002/int.22639
Mahmood, T., and Ur Rehman., U. (2022b). A method to multi-attribute decision making technique based on Dombi aggregation operators under bipolar complex fuzzy information. Comp. Appl. Math. 41 (1), 47–23. doi:10.1007/s40314-021-01735-9
Narang, M., Joshi, M. C., Bisht, K., and Pal, A. (2022). Stock portfolio selection using a new decision-making approach based on the integration of fuzzy CoCoSo with Heronian mean operator. Decis. Mak. Appl. Manag. Eng. 5 (1), 90–112. doi:10.31181/dmame0310022022n
Pandey, S. D., Ranadive, A. S., Samanta, S., and Sarkar, B. (2022). Bipolar-valued fuzzy social network and centrality measures. Discrete Dyn. Nat. Soc. 2022, 1–13. doi:10.1155/2022/9713575
Poulik, S., and Ghorai, G. (2020). Note on “Bipolar fuzzy graphs with applications”. Knowledge-Based Syst. 192, 105315. doi:10.1016/j.knosys.2019.105315
Rajalakshmi, R., and Mary, K. J. R. (2022). Assessment for choosing the best alternative fuel under bipolar-valued fuzzy multi criteria decision making. AIP Conference Proceedings. AIP Publ. LLC 2385, 080001.
Ramot, D., Milo, R., Friedman, M., and Kandel, A. (2002). Complex fuzzy sets. IEEE Trans. Fuzzy Syst. 10 (2), 171–186. doi:10.1109/91.995119
Rehman, U. U., Mahmood, T., Albaity, M., Hayat, K., and Ali, Z. (2022). Identification and prioritization of DevOps success factors using bipolar complex fuzzy setting with Frank aggregation operators and analytical hierarchy process. IEEE Access 10, 74702–74721. doi:10.1109/access.2022.3190611
Riaz, M., Pamucar, D., Habib, A., and Jamil, N. (2022). Innovative bipolar fuzzy sine trigonometric aggregation operators and SIR method for medical tourism supply chain. London: Mathematical Problems in Engineering. doi:10.1155/2019/9064385
Rytova, E., and Gutman, S. (2019). Assessment of regional development strategy in the context of economy digitization on the basis of fuzzy set method IOP conference series: Mater. IOP Conf. Ser. Mat. Sci. Eng.IOP Publ. 497 (No. 1), 012060. doi:10.1088/1757-899x/497/1/012060
Sahu, R., Dash, S. R., and Das, S. (2021). Career selection of students using hybridized distance measure based on picture fuzzy set and rough set theory. Decis. Mak. Appl. Manag. Eng. 4 (1), 104–126. doi:10.31181/dmame2104104s
Singh, P. K. (2022). Bipolar fuzzy concepts reduction using granular-based weighted entropy. Soft Comput. 26, 9859–9871. doi:10.1007/s00500-022-07336-w
Tamir, D. E., Jin, L., and Kandel, A. (2011). A new interpretation of complex membership grade. Int. J. Intell. Syst. 26 (4), 285–312. doi:10.1002/int.20454
Ullah, K. (2021). Picture fuzzy Maclaurin symmetric mean operators and their applications in solving multiattribute decision-making problems. Mathematical problems in engineering 2021, 1098631. doi:10.1155/2021/1098631
Ur Rehman., U., and Mahmood, T. (2022). The generalized dice similarity measures for bipolar complex fuzzy set and its applications to pattern recognition and medical diagnosis. Comp. Appl. Math. 41 (6), 265–330. doi:10.1007/s40314-022-01948-6
Wan, C., Deng, F., Li, S., Omidbakhsh Amiri, S., Talebi, A. A., and Rashmanlou, H. (2022). Novel concepts in bipolar fuzzy graphs with applications. J. Math. 2022, 1–9. doi:10.1155/2022/8162474
Wei, G., Alsaadi, F. E., Hayat, T., and Alsaedi, A. (2018). Bipolar fuzzy Hamacher aggregation operators in multiple attribute decision making. Int. J. Fuzzy Syst. 20 (1), 1–12. doi:10.1007/s40815-017-0338-6
Xu, Z., and Yager, R. R. (2010). Intuitionistic fuzzy Bonferroni means. IEEE Trans. Syst. Man. Cybern. B Cybern. 41 (2), 568–578. doi:10.1109/TSMCB.2010.2072918
Xu, Z., and Yager, R. R. (2009). Power-geometric operators and their use in group decision making. IEEE Trans. Fuzzy Syst. 18 (1), 94–105.
Yager, R. R. (2001). The power average operator. IEEE Trans. Syst. Man. Cybern. A 31 (6), 724–731. doi:10.1109/3468.983429
Yamini, C., Saravanamoorthi, P., and Kailasavalli, S. (2022). A decision-making method for selecting TMT rod based on bipolar valued fuzzy sets. J. Algebraic Statistics 13 (2), 126–133. doi:10.52783/jas.v13i2.146
Yin, S., Zhang, N., Ullah, K., and Gao, S. (2022). Enhancing digital innovation for the sustainable transformation of manufacturing industry: A pressure-state-response system framework to perceptions of digital green innovation and its performance for green and intelligent manufacturing. Systems 10 (3), 72. doi:10.3390/systems10030072
Zeeshan, M., and Khan, M. (2022). 2103-6568 Complex fuzzy sets with applications in decision-making. Iran. J. Fuzzy Syst. 19 (4), 147–163. doi:10.22111/ijfs.2022.7093
Zhang, W. R. (1994). “Bipolar fuzzy sets and relations: A computational framework for cognitive modeling and multiagent decision analysis,” in NAFIPS/IFIS/NASA'94. Proceedings of the first international joint conference of the north American fuzzy information processing society biannual conference. The industrial fuzzy Control and intellige (IEEE), 305–309.
Keywords: Bonferroni mean operators, bipolar complex fuzzy set, pollution, environment, decision-making procedure
Citation: Yang X, Mahmood T and Ur Rehman U (2022) Analyzing the effect of different types of pollution with bipolar complex fuzzy power Bonferroni mean operators. Front. Environ. Sci. 10:1026316. doi: 10.3389/fenvs.2022.1026316
Received: 23 August 2022; Accepted: 04 October 2022;
Published: 23 November 2022.
Edited by:
Shi Yin, Agricultural University of Hebei, ChinaReviewed by:
Goran Cirovic, University of Novi Sad, SerbiaHamed Fazlollahtabar, Iran University of Science and Technology, Iran
Copyright © 2022 Yang, Mahmood and Ur Rehman. This is an open-access article distributed under the terms of the Creative Commons Attribution License (CC BY). The use, distribution or reproduction in other forums is permitted, provided the original author(s) and the copyright owner(s) are credited and that the original publication in this journal is cited, in accordance with accepted academic practice. No use, distribution or reproduction is permitted which does not comply with these terms.
*Correspondence: Xiaopeng Yang, aGFwcHl5YW5neHBAMTYzLmNvbQ==