- School of Economics and Management, Northwest A&F University, Xianyang, China
As one of the key links of agricultural green sustainable development, it is an effective path to popularize green production technology and promote farmers’ adoption of green production technology by using cooperatives as an organizational vehicle. Based on the survey of 314 apple farmers in Shaanxi and Gansu provinces, the degree of adoption of green production technology was measured by the coefficient of variation method. At the same time, in view of the selection bias of farmers’ participation in cooperatives, the propensity score matching method was used to evaluate the influence of farmers’ participation in cooperatives on the adoption of green production techniques. The results showed that participation in cooperatives increases the probability of farmers adopting green production techniques from 25.29% to 30.29%, indicating that cooperatives increase the degree to which farmers adopt green production techniques. In addition, increased cognition of green production, which increases the price and net profit of products sold and reduces expectations of green production risks, is the primary channel through which cooperatives lead farmers to adopt green production technologies. Participation in cooperatives, though, increased input costs for apple production. In order to strengthen the active role of cooperatives in the popularization of green production technologies, more support should be given to cooperatives, and emphasis should be placed on strengthening their institutional development so that they can be standardized and developed at a high quality.
1 Introduction
Agricultural practices are one of the most significant contributors to global greenhouse gases and are the second largest carbon source after electricity and heat production (Solomon et al., 2007; Haller, 2022). Overuse of fertilizers and pesticides, as well as inefficient field crop management, has already resulted in a slew of environmental pollution issues, including surface and groundwater pollution, soil slab acidification, and soil erosion, all of which pose a significant threat to sustainable agricultural development (Zhang et al., 2017; Tang and Zhou, 2018). Without harmonious ecological and economic development, economic growth will not be sustainable and may even lead to the decline of ecosystems (Zhang et al., 2022).
The adoption and extent of green production technologies by smallholder farmers, who are the core participants in the agricultural production chain, will be one of the key factors affecting the sustainable development of agriculture (Liu et al., 2020). In this regard, the Chinese government has set subsidies and penalties to expand the adoption of green production technologies, for example, encouraging soil testing and fertilizer application or replacing chemical fertilizers with organic fertilizers (Zhang et al., 2016; Cui et al., 2018). However, practice shows that green and high-quality agricultural development has long faced difficulties in the widespread adoption of green production technologies among smallholder farmers (Chu et al., 2012; Luo et al., 2013; Geng et al.). There are three main reasons for this situation: first, the mechanism for promoting new technologies at the grassroots level in China is not yet sound, and publicity is insufficient (Mao et al., 2021); Second, Chinese farmers’ awareness of ecological conservation is still weak, and they have not yet shaken off their reliance on traditional production methods (Huang et al., 2018; Chèze et al., 2020); Third, due to their high technological content, green production technologies are also relatively difficult to learn and use, and their input costs are relatively high, ultimately leading to high transaction costs and business risks for farmers to adopt green production technologies (Zhao and Cai, 2012; Luo et al., 2013). Therefore, exploring the drivers of green production technology adoption among farmers has become a popular topic in the academic community. However, in exploring the drivers of the adoption of green production technologies by farmers, scholars have mainly focused on government environmental regulations and financial subsidies (Gao, 2013; Chatzimichael et al., 2014); household resource endowments such as household capital, labor, land, social networks and information networks (Zhang et al., 2013; Geng et al., 2017); market demand, market price, and market risk (Guo et al., 2007; Geng et al., 2017; Wang et al., 2018); farmers’ perceptions of ecological environment and green production (Guo and Zhao, 2017; Tong and Liu, 2017; Liu and Zhou, 2018); and farmers’ information access status (Carter et al., 2014; Genius et al., 2014; Zhang et al., 2019), among other areas.
Cooperatives offer new solutions for the development of green agriculture by promoting green production technologies and regulating green production and product supply. Although some scholars have demonstrated through experience the positive contribution of membership in cooperatives to the promotion of green production technologies such as organic fertilizer application, soil test formulation fertilization, and integrated pest management (Abebaw and Haile, 2013; Wu et al., 2016; Wossen et al., 2017; Zhang and Liu, 2020; Wan and Cai, 2021). However, mechanisms for cooperatives to promote the adoption of green production technologies by farmers have not been adequately discussed in academia. Most of the explanations given by some scholars are that when farmers join cooperatives, they have more effective access to information about new technologies, which improves their cognitive abilities and thus effectively promotes the adoption of green production technologies (Odoemenem and Obinne, 2010; Feng and Huo, 2016). It has also been argued that the channels through which cooperatives channel farmers’ passive application of soil-measuring fertilizer application technology through standardized production systems are more important than the channels through which information is transmitted through technical training (Wan and Cai, 2021).
In summary, when analyzing the mechanism, most studies focus on risk, information, and other factors perspectives, arguing that the technical information provided by cooperatives can enhance farmers’ perceptions and thus lead to their adoption of green production technologies. However, previous literature has overlooked an important issue: farmers’ adoption of green production technologies is based on their factor endowments, a combination of expected production benefits and risks. We argue that the key for cooperatives to guide farmers to adopt green production technologies is to achieve a Pareto improvement in the adoption costs and benefits. Otherwise, even if farmers have a high level of cognitive and no barriers to information access, the probability of farmers adopting new technologies will not significantly increase. Therefore, based on household surveys of 314 apple farmers in Baishui County, Shaanxi Province, and Qingcheng County, Gansu Province, we use the PSM to assess apple farmers’ participation in cooperatives and their adoption effects of green production technologies. The contributions and innovations of this paper are: First, it expands the mechanism of cooperatives to promote farmers’ adoption of green production technologies. We focus on cooperatives through information transmission channels and innovatively add a “cost-benefit” perspective. We consider how cooperatives can induce farmers to adopt green production technologies through the cognitive level, risk expectations, product premium, input cost, and net income. Second, considering the selection bias, this study uses the PSM method to explore the quantitative impact of cooperative membership on farmers’ adoption of green production technologies and uses different matching methods and sensitivity analysis to test the robustness of the PSM estimates.
2 Theoretical analysis and research hypothesis
From the perspective of a rational economic person, the ultimate goal of farmers joining cooperatives is to obtain agricultural social services to improve their livelihoods and increase their earnings rather than to contribute to environmental sustainability. Therefore, profit is their primary motivation for allocating factors of production. The more economic benefits generated by technology adoption and the fewer costs they have to pay, the higher the level of adoption of the technology by farmers (Geng et al., 2017). Based on this logic, through literature review and theoretical summary, we argue that cooperatives can increase farmers’ adoption of green production technologies by influencing their level of cognitive, risk expectations, product premiums, input costs, and net incomes (see Figure 1).
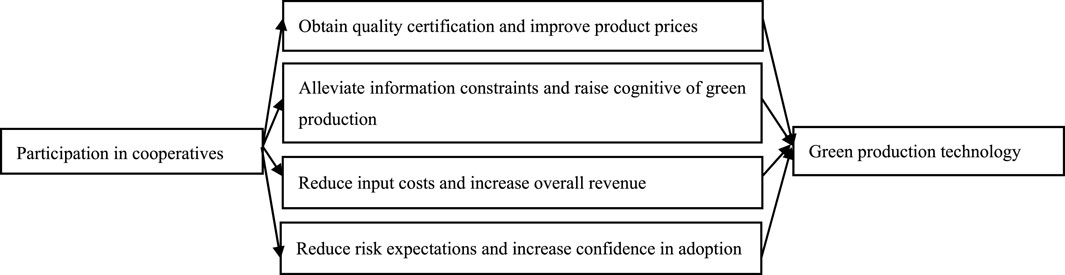
FIGURE 1. Mechanisms for participating cooperatives to influence the adoption of green production technologies by farmers.
Firstly, Participation in cooperatives can help farmers obtain quality certification and raise market prices. Since entering the 21st century, food safety has become the focus of social concern. People have higher nutritional, health, and safety requirements for food and are willing to pay higher prices for green products (Quan and Zeng, 2014). Excess profits from these market premiums drive farmers’ adoption of green production technologies (Li et al., 2021). Cooperatives improve the quality of green agricultural products by educating farmers on standardized production, accurate prevention, and control of agricultural risks (Ma and Huo, 2019). In addition, participation in cooperatives increases farmers’ acceptance of green production technologies by helping them to obtain certification of green product quality, increasing product recognition, and increasing the price advantage of green products produced by small farmers participating in cooperatives (Chen et al., 2021). On this basis, hypothesis H1 is proposed.
H1: If farmers participate in cooperatives to increase the market price of green products by improving product quality and increasing market recognition of their products, then they will increase their use of green production techniques.
Secondly, Participation in cooperatives reduces farmers’ information constraints and helps to increase their cognition of green production techniques. Access to and understanding green technology information are critical to farmers’ technology adoption decisions. Lack of cognition leads to the misallocation of resources and adoption risks, severely constraining farmers’ technology adoption behavior (Huang et al., 2008; Tang and Zhou, 2018). Information flows can improve farmers’ understanding and mastery of technical information and facilitate the adoption of new technologies (Huang et al., 2008). Farmers’ ability and level of access to information directly affect the distribution of resources available to them and, ultimately, the adoption of green production technologies by farmers (Tang and Zhou, 2018). Cooperatives provide a wide range of information sharing, technical training, policy explanation, and educational and training services. Participation in cooperatives can break the traditional communication patterns of networks of acquaintances, ease information constraints, improve farmers’ cognitive level and practice of green production technologies, and promote their adoption (Zhao and Cai, 2012; Du et al., 2014; Zheng et al., 2018; Ren and Hussain, 2022). Based on this, this paper proposes hypothesis H2.
H2: If participation in a cooperative, the technical training, and education provided by the cooperative can alleviate the information constraints of apple growers and increase their level of cognition of green production techniques, then the level of adoption of green production techniques by farmers will increase.
Thirdly, participation in cooperatives can reduce the cost of raising apples for apple farmers and improve overall profitability. In particular, the adoption of green production technologies requires a certain level of funding. However, high costs often act as a deterrent to farmers with weak resource endowments, small-scale operations, and fragmentation (Cai J., 2013). By joining cooperatives, the marginal costs of adopting green production technologies are greatly reduced. Cooperatives reduce procurement costs through collective action. Cooperatives can take advantage of collective procurement to increase the bargaining power of farmers, enabling them to purchase or lease needed means of production and technical equipment at lower prices and avoiding cost overflow from “seller’s markets” (Liu et al., 2021). Individual farmers are prone to additional costs due to their limited cognitive abilities and lack of judgement on the application of green technologies and the mix of factors of production; however, through the organization of technical training, cooperatives can partly compensate for farmers’ deficiency of capacity, help to improve efficiency in the use of technical equipment, regulate the use and frequency of pesticides and fertilizers, and avoid waste of resources and lower yields due to ineffective inputs. It also helps to regulate the dose and frequency of pesticide and fertilizer inputs and avoid waste of resources and reduction in yields due to ineffective inputs (Cai R., 2013; Ma and Abdulai, 2016; Mojo et al., 2017; Wan and Cai, 2021). This can effectively reduce the cost of adopting green production technology and make farmers gain considerable economic benefits, thus enhancing the adoption of green production technology by farmers. On this basis, hypothesis H3 is proposed.
H3: If participation in cooperatives, collective action by cooperatives, and technical training help to reduce the input costs of apple farmers and increase overall returns, then farmers’ adoption of green production technologies will increase.
Fourthly, participation in cooperatives helps to reduce farmers’ risk expectations of adopting green production technologies. Smallholder survival theory suggests that farmers are often “risk-averse” and that production risks are the most important factor in their decision to adopt green production technologies (Wang and Liu, 1996). The social services provided by cooperatives, such as agricultural supply, technical training and product marketing, not only reduce adoption costs and increase returns, but also, and most importantly, help farmers reduce their risk expectations green production and increase confidence in the application of new technologies (Cai, 2012). In addition, depending on the nature of the cooperative, residual distributions based on shares and transactions also provide surplus claims for cooperative members, reducing farmers’ risk expectations for adopting green production technologies. Thus, farmers’ adoption of green production technology has been improved. On this basis, hypothesis H4 is proposed.
H4: If participation in cooperatives and the social services provided help reduce farmers’ risk expectations of green production, then farmers will adopt green production technologies.
Based on the above analysis, cooperatives can improve farmers’ acceptance of green production technologies by raising product prices, raising cognitive, reducing input costs to increase net incomes, and reducing expectations of green production risks. On this basis, hypothesis H5 is presented.
H5: If apple farmers join cooperatives, their adoption of green production techniques will increase.
3 Data sources, research methods, and variable selection
3.1 Data sources
The data in this study are mainly from the apple production and cultivation study conducted by the research group in Baishui County, Shaanxi Province, and Qingcheng County, Gansu Province, from November 2020 to October 2021. Specifically, in November 2020, the team traveled to Baishui County, Shaanxi Province, to study annual data for apple growers for 2018 and 2019. The 2019 and 2020 annual data for apple growers apple surveyed in October 2021 in Qingcheng County, Gansu Province, using the same questionnaire format as the Baishui County survey. To ensure consistency and reduce annual interference with empirical results, we have aggregated 2019 data from both locations. We used 314 cross-sectional data of apple farmers from the two places in 2019 to complete the study of this paper. The team chose Shaanxi and Gansu as the study area because, according to the distribution of apple cultivation in the country, the dominant area of the Loess Plateau in Shaanxi and Gansu in 2021 was 1,220,280 ha, accounting for 58.44% of the country’s apple planting area. Among them, Shaanxi and Gansu provinces are China’s first and fifth largest apple growers. The research area of Baishui county and Qingcheng county in Shaanxi and Gansu province can objectively and genuinely reflect the production situation of apple growers in China. The team sampled using a combination of stratified and random sampling methods and random sampling. Specifically, there are seven townships in Baishui County and nine in Qingcheng County, each of which we numbered and randomly sampled using computers. By layered random sampling, the team selected three townships in Qingcheng County, Xuanma, and Yima, and three in Baishui County, namely, Leiya Shiguan and Yaohe. Villages in each commune are then numbered uniformly, and 2–3 administrative villages are randomly selected. Whether we chose two or three villages depended on how many households would participate in the second village. If the number was insufficient, a third village was selected. In the second step, we compiled a complete list of all the apple growers. Finally, 10–20 apple farmers in each of the villages.
In order to understand the current situation of apple farming households in the main production area, the research team designed a targeted questionnaire. The questionnaire consists mainly of household information (demographic structure, level of education, age structure, etc.), land production and management, household income and consumption structure, household livelihood capital, awareness and application of low-carbon technologies, participation in cooperatives, etc. 412 questionnaires were sent out in 2020 and 2021. We screen the questionnaire according to the following four criteria: 1) elimination of participants in empty-nest cooperatives and sham cooperatives; 2) elimination of samples with significant data deficits that did not reach 60% of the questionnaire questions; 3) elimination of samples with inconsistent information; and 4) elimination of samples with zero apple business area. Three hundred fourteen active samples were eventually obtained with a sample recovery rate of 76.21%. Based on the collected samples, the household characteristics and production and planting properties of sample farmers are described in this paper (Table 1).
As shown in Table 1, 55.55% of apple-growing households in the sample area had an annual income of less than 10,000 dollars, and 38.22% of households had apple income accounting for more than 60% of household income. The number of household laborers was mainly 3–4, with 61.78% of households having more than 60% agricultural laborers, indicating that the main livelihood and income of homes in the sample area came from apple farming. The degree of land fragmentation is the inverse of the total household land area ratio to the number of plots. A higher value means a higher degree of fragmentation, with the degree of land fragmentation of the sample farmers concentrated between 0 and 0.5. The present value of household production materials was generally lower than 2000 dollars. The average years of education of the sample apple growers were mainly 5–10 years, and 11.78% of the households had village cadres. The difficulty of bank borrowing refers to the difficulty for families to obtain bank credit of 7,000 dollars, which we measured on a Likert scale of 1–5, with higher values representing more incredible difficulty. 32.80% of the sample households had greater difficulty in bank borrowing than 3. The number of relatives and friends in the sample households mainly ranged from 10 to 20. The number of household living materials is mainly concentrated between 5 and 10, household gift expenses are mainly between 0 and 500 dollars, and communication expenses are mainly between 200 and 300 dollars. The proportion of the sample apple farmers who joined farmers’ professional cooperatives was 30.89%, and the proportion of households who participated in agricultural technology training 1–3 times was 55.10%.
3.2 Research methodology
In reality, however, apple farmers are not randomly assigned to the cooperative participation group (treatment group) and the non-cooperative participation group (control group). Still, they are “self-selected” by several observable factors, such as household characteristics and livelihood capital. In this case, failure to deal with the “self-selection” problem will lead to sample selection bias and, thus, biased parameter estimates. The propensity score matching (PSM) method is often used to create a control group of comparable states to address the sample selection bias caused by observable factors (Ragasa et al., 2018; Soullier and Moustier, 2018; Li et al., 2020). The specific steps are as follows:
To assess the impact of participating cooperatives on adopting green production technologies by apple growers, we first construct a general model of green production technology adoption by apple growers.
In the equation,
Then, to create such a control group, PSM calculated a propensity score or probability of apple grower participation in the cooperative based on each farmer’s pretreatment covariates and then matched participants with non-participants based on the predicted propensity score to obtain treatment and control groups that converged to a comparable balanced state (Rosenbaum and Rubin, 1983). It can be seen that the propensity matching score for apple grower participation in cooperatives is the probability of apple grower participation in cooperatives under the given conditions. Propensity matching scores are usually estimated using Logit or Probit models to match the treatment and control farmers (Li et al., 2020; Wan and Cai, 2021). In this paper, a logit model is chosen to estimate the propensity matching scores of apple growers to participate in farmers’ cooperatives.
In the equation,
After calculating the propensity matching score, the appropriate matching method must be selected to match participants and non-participants. Nearest neighbor matching (NNM), kernel matching (KM), and radius matching (RM) are commonly used to assess the impact of program participation (Ragasa et al., 2018; Soullier and Moustier, 2018). Nearest neighbor matching matches participants and non-participants using the closest propensity score. Kernel matching matches participants and non-participants using a kernel function that reflects the degree of similarity of the covariates. Radius matching matches participants only to non-participants whose propensity scores fall within the participant’s predefined neighborhood (Becker and Ichino, 2002). To ensure the robustness of the estimation results, we use all three methods in this paper.
The average treatment effect (ATE) of program participation when no matching is:
Where
The key assumption the PSM approach is that participants’ decisions depend only on observable factors. However, there may be factors swaying farmer participation that are not observed. Sensitivity analysis is needed to test the susceptibility of PSM’s estimated average therapeutic outcome (ATT) to unobserved factors. Therefore, using existing literature, we used Rosenbaum’s bounds analysis to test the sensitivity of treatment outcomes to unobservable objects (Rosenbaum and Rubin, 1983; Li et al., 2020; Ren et al., 2021).
3.3 Variable selection and descriptive statistics
3.1.1 Outcome variable
Degree to which apple growers adopting green production technologies, as defined in the “Technical Guidelines to Green Agricultural Development (2018–2030) issued by the Ministry of Agriculture and Rural China,” is:“ Green production technologies include cropland quality improvement and conservation technology, agricultural water-saving and rain-fed dry-farming technologies, fertilizer and pesticide application technologies, agricultural waste recycling technologies,...and herbivorous green and efficient production technologies.” According to the characteristics of apple production, five green production techniques were selected: organic fertilizer application technology, soil measuring formula fertilization technology, drip irrigation sprinkler irrigation technology, pest control technology, water fertilizer integration technology (Huang et al., 2018; Yang, 2018; Geng et al., 2016.). However, due to the limited the applicability of green production sub-technologies in different regions, estimates of the same weighting can lead to biased results. Based on the economic benefits, resource benefits and environmental benefits of green production technology, the weight coefficients of five sub-technologies are calculated by using the coefficient of variation method. We measure the weighted average of these five technologies to measure the extent to which apple growers adopted green production techniques (whether or not green production secondary techniques were used was a binary variable, using = 1; not = 0).
The coefficient of variation method is an objective weighting method based on statistical approaches that use the information in each hand to calculate the degree of variation of each indicator in the system and then assign weights. Samples with more significant variation are assigned more important weights; conversely, smaller weights are assigned (Li et al., 2020). Statistically, there are also objective assignment methods such as principal component analysis, factor analysis, and Amal KantiRary assignment method. We chose the coefficient of variation method because it is simple to operate and can effectively distinguish between individual indicators. Secondly, the amount of variance information of the indicators is measured by the variance, but due to the influence of the scale and order of magnitude of each indicator, the variance of each indicator is not comparable, and it is more appropriate to choose a comparable coefficient of variation (Chen, 1995).
Firstly, we measure the mean (
Then, the coefficients of variation of each indicator are normalized to obtain the secondary indicator weights of each sub-technology in the three dimensions of economic benefits, resource benefits, and environmental benefits:
Finally, we sum up the corresponding secondary index weights of economic, resource, and environmental benefits under each sub-technology to obtain the primary index weights of the five sub-technologies.
3.1.2 Treatment variable
Participation in cooperatives. According to the definition of the Law of the People’s Republic on Peasant Professional Cooperatives, peasant professional farmers’ cooperative cooperatives are collective economic organizations that are voluntarily organized and democratically managed by producers and operators of similar agricultural products or by providers and users of similar agrarian production and operation services on the basis of family contracting in rural areas. It also provides services such as the purchase of agricultural production materials, the sale, processing, transportation, storage of agricultural products, and the technology and information related to agricultural production and operation, up to online trading with members as the primary service targets. Therefore, in order to ensure the accuracy of processing variable data, farmers who joined empty and fake cooperatives are considered non-participants; farmers who joined actual operations but did not benefit from cooperative services such as production, marketing, and technical training are likewise considered non-participants. By the above criteria, 108 out of 314 apple farmers sampled in this paper belong to cooperatives or 34.39% of the total sample.
3.1.3 Matching variable
Existing research suggests that farmers’ participation and cooperatives or adoption of new technologies are rooted in the pursuit of economic gains through improved household livelihoods. Current research, therefore, shows that farmers’ decisions to participate in cooperatives and adopt new technologies are based on household livelihood capital (natural, physical, human, financial, social, and information capital) (Cai and Han, 2012; Yang, 2018, 2018; Mmbando et al., 2021; Geng et al.). Therefore, natural, physical, human, financial, social, and information capital have been selected as matching variables based on a sustainable livelihood framework (see Table 2 for more details).
Table 3 describes the essential statistical characteristics of apple farmers in the sample. Table 3 shows that cooperative farmers exhibit higher levels of adoption of green technologies, total household income, labour force, level of family education, household means of production, and agro-technical training than non-co-operative farmers.
4 Empirical results and analysis
4.1 Measurement of green production technology adoption by apple growers
Because of the variability of green production technologies, they play different roles in the production process. In order to accurately describe the attributes of green production technology, this paper uses a Likert scale to evaluate the three dimensions of economic benefit, environmental benefit, and environmental benefit of the five green production sub-technologies. Based on the subjective evaluation data of apple growers, this paper used the coefficient of variation method to calculate the weights of each indicator. The results rank according to the importance of the hands: drip and sprinkler irrigation technology, soil testing and fertilization technology, pest control technology, water and fertilizer integration technology, and organic fertilizer application technology (see Table 4).
4.2 Impact of cooperative participation on green production technology adoption by apple farmers
4.2.1 Estimation of a decision model for apple grower participation in cooperatives
Based on the Sustainable Livelihoods Framework (SLF), this paper chose the Logit model to evaluate the propensity matching scores of apple farmers’ participation in farmers’ cooperatives. Table 5 shows the results.
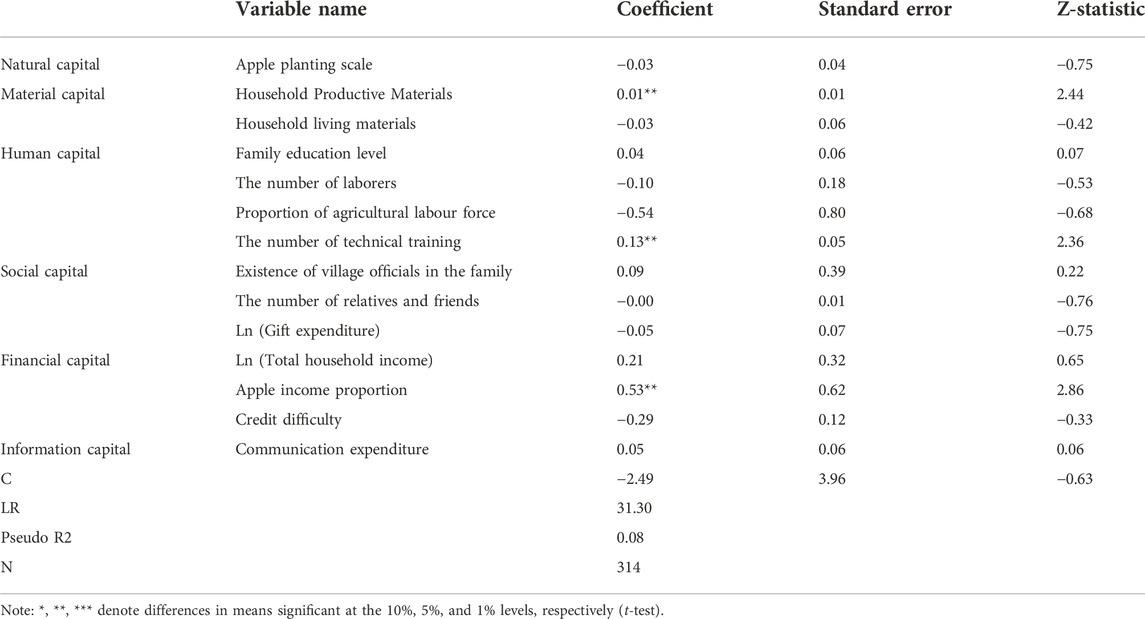
TABLE 5. Estimation results of a decision model based on logit model for apple growers’ participation in cooperatives.
4.2.2 Common support domain and balance test
The propensity score of apple growers can be calculated based on the estimation results of the apple grower participation in the cooperative model. Still, it should test the quality of the match before estimating the treatment effects. Based on the existing literature, this paper chose to assess the matching quality using the equilibrium degree of the standard support domain and pretreatment variables (explanatory variables) (Ragasa et al., 2018). When the common support domain is too narrow, it will result in samples outside the common support domain not being matched effectively.
The test results using 1-to-1 nearest neighbor matching showed (Figure 2A) that the number of samples in the treatment group outside the common support domain was two, and the number of samples after matching was 106, with a loss of 2 observations in the treatment group. As can be seen, the co-support domain retains a significant number of samples from the treatment group, and the quality of the match could be considered good. In order to ensure the robustness of the results of the common support domain tests, we performed robustness testing using nearest neighbor matching (1-to-3 match), caliper matching (0.01), and nuclear matching. The results are shown in Figures 2B–D. The results of 1-to-3 nearest neighbor matching and nuclear matching were consistent with 1-to-1 nearest neighbor matching with loss value of 2. Caliper matching loss value (0.01) is 5, which is small, indicating that the match quality passes the general support domain test.
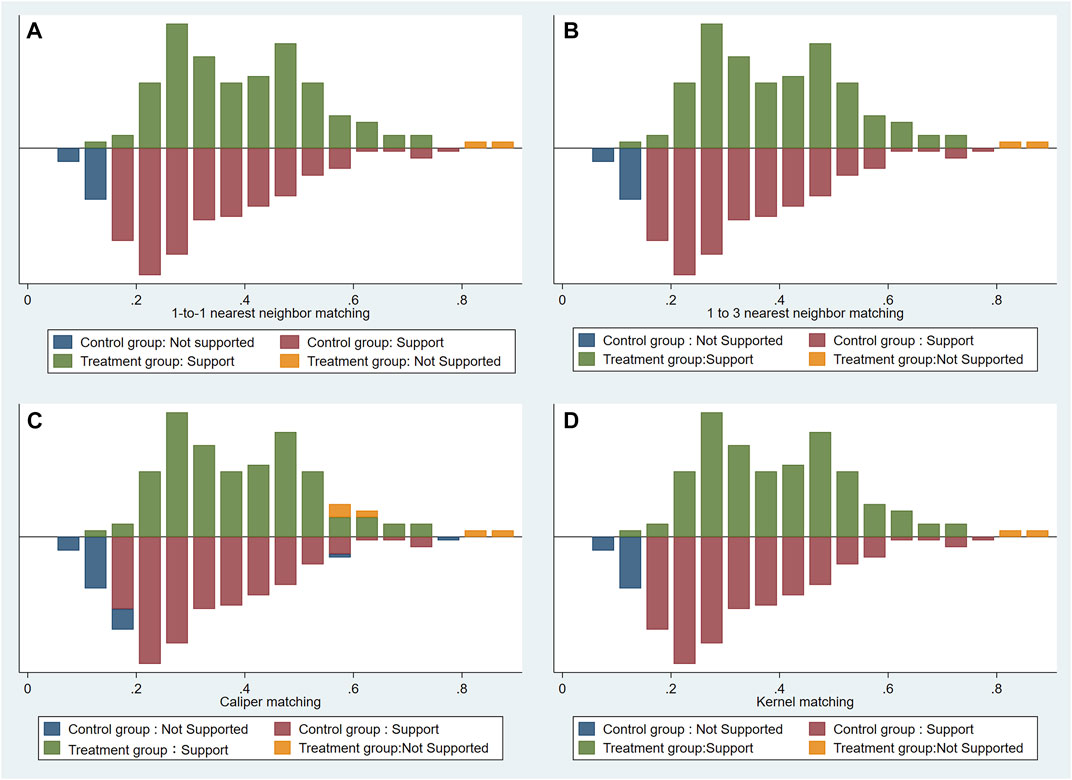
FIGURE 2. Common support domain test: (A) 1-to-1 nearest neighbor matching; (B) 1-to-3 nearest neighbor matching; (C) caliper matching (0.01); (D) kernel matching.
Table 6 shows the results of balancing tests of explanatory variables before and after matching. In the case of the 1-to-1 nearest neighbor match, the Pseudo R2 decreased from 0.079 to 0.023, the LR statistic decreased from 31.90 to 6.77, the mean bias decreased from 19.1 to 7.6, and the median bias decreased from 17.7 to 7.2. The results of the 1-to-3 nearest neighbor matching, the caliper matching (0.01), and kernel matching were tested for robustness. The bias of the total sample was significantly reduced after the propensity score matching, and the treatment group and control group had similar characteristics, i.e., passed the equilibrium test.
4.2.3 Analysis of the effect of participation in cooperatives on the adoption of green production technologies by apple growers
The estimated results of the effect of participating cooperatives on adopting green production technologies by apple growers show in Table 7. Taking the estimation results of 1-to-1 nearest neighbor matching, the average treatment effect (ATT) of participation in cooperatives on green production technology adoption among apple growers was 5% and passed the test at the 1% significance level. Participation in cooperatives can significantly contribute to the adoption of green production technology among apple growers. From the mean, the probability of adopting green production technology among farmers who did not participate in cooperatives was 25.29%. The likelihood of using green production technology among farmers who participated in cooperatives raised to 30.29%, which was a 5% increase. Moreover, the ATT estimates of 1-to-3 nearest neighbor matching, caliper matching (0.01), and kernel matching were 7.02%, 7.75%, and 7.14%, respectively, and the positive effect of participation in cooperatives on the adoption of green production technologies by apple growers can be considered robust.

TABLE 7. Estimated results of the overall effect of participation in cooperatives on the adoption of green production technologies by apple growers.
4.2.4 Sensitivity analysis
The key assumption of the PSM approach is that the decision of apple farmers to participate in cooperatives depends only on observable factors. However, a number of some unobserved factors may affect farmers’ participation in cooperatives. Sensitivity analysis is therefore needed to test the susceptibility of PSM’s estimated average therapeutic outcome (ATT) to unobserved factors. In this study, Rosenbaum’s bounds analysis was used to detect sensitivity to unobserved factors in therapeutic efficacy (ATT). Table 8 shows the results of Rosenbaum’s bounds sensitivity analysis. The parameter γ (≥1) is a measure of freedom from hidden bias, and the baseline scenario is the absence of hidden bias (γ = 1) (Rosenbaum and Rubin, 1983). The higher the value of γ, the higher the incidence of hidden bias (the higher the likelihood of the presence of unobservables). Based on the literature, conduct a sensitivity analysis in this paper for γ ranging from 1 to 2 (Dillon, 2011). Report the upper and lower significance levels (Sig+ and Sig−) for each value of γ. Suppose an upper (or lower) limit of significance can observe. In that case, it assumes that the average treatment effect (ATT) of the PSM estimates remains significant even for a given degree of hidden bias. As can be seen from the results in Table 8 (with 1-to-1 nearest neighbor matching), farmers’ degree of adoption of green production technologies is insensitive to a given degree of hidden bias. As the hidden bias is double, Sig− decreases from 0.023 to below 0.001, indicating that the estimates are primarily robust and significant at the 1% significance level (from γ = 1 to γ = 2). The results are more robust whether using 1-to-3 nearest neighbor matching, caliper matching (0.01), or kernel matching. In other words, the positive impact of participating cooperatives on apple growers’ green production technology adoption behavior is unlikely to change significantly, even in some unobservable factors.
4.3 Mechanistic analysis of the impact of participation in cooperatives on the adoption of green production technologies by apple growers
These studies show that participation in cooperatives can significantly promote the adoption of green production technologies by apple growers, so how does the participation of cooperatives influence apple-growing farmers’ green production decisions? According to previous theoretical analysis, farmer participation in cooperatives influences farmers’ green production behavior through a combination of the selling price, perception level, input cost, net benefit, and risk expectation. This section provides an empirical examination of this influence mechanism; a descriptive statistical analysis of the variables used is presented in Table 2, and the validation results are presented in Table 9.
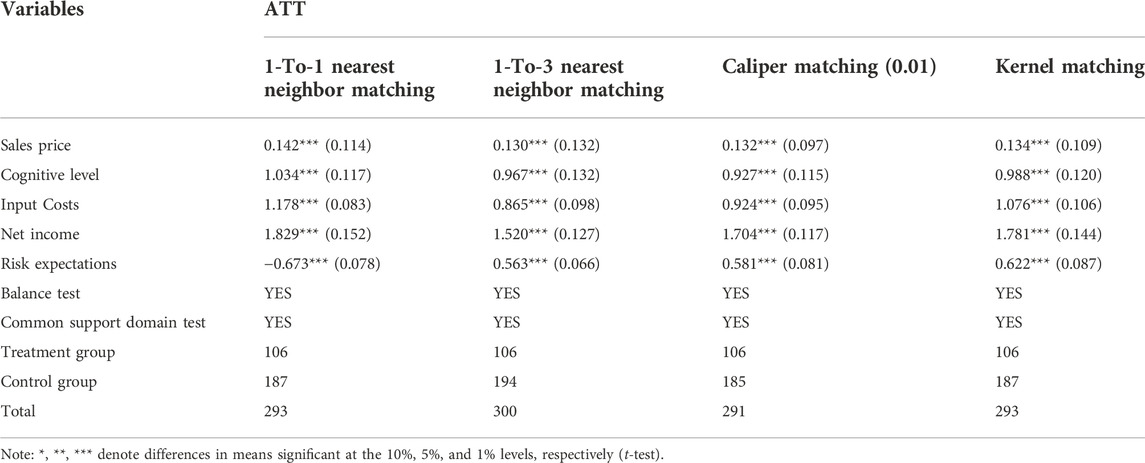
TABLE 9. Regression results of the mechanism of the effect of participation in cooperatives on the adoption of green production technologies by apple growers.
Table 9 estimates the impact of participation in cooperatives on the potential channels through which participation in cooperatives can influence apple farmers to adopt green production technologies. The estimation results of the four matching methods are the same. Estimates show that participation in cooperatives can significantly improve farmers’ economic benefits (sales prices and net income) and perceptions and reduce risk expectations for green production. Specifically, ATT data showed that farmers participating in cooperatives saw an increase in apple sales, with net apple earnings of 0.142USD/kg and 1829USD/ha, respectively. Compared to non-participants, their cognitive of green production increased by 1.034, followed by a 0.673 reduction in risk expectations for green output. However, the paper also found that while participation in cooperatives increased farmers’ economic net income, the average input cost per ha of apple production increased by USD 1178. Since farmers often require to standardize their production after joining cooperatives to meet the quality requirements of organic and green products, they must invest more in agricultural facilities and adopt green production techniques. We conclude that raising cognition, increasing selling prices and net incomes, and reducing risk expectations of green production are potential channels through which participation in cooperatives can significantly influence the adoption of green production technologies.
5 Discussion
As new agricultural business entities, cooperatives’ advantages of intensification, scale, and organization help reduce the search cost and application risk of green production technologies for fruit farmers and promote the diffusion and spread of green production technologies, and cooperatives are supposed to become key subjects of agricultural technology diffusion systems such as green production technologies (Zhang and Liu, 2020). However, few studies have revealed the underlying mechanisms by which cooperatives promote the adoption of green production techniques by farmers. This study aims to evaluate the promotion effect of cooperatives on the application of farmers’ green production technology adoption and to clarify how cooperatives influence farmers’ green production technology adoption decisions. We conducted a systematic review of existing research and quantitative analysis using the PSM method and a sample of 314 apple farmers in Shaanxi and Gansu, China.
In assessing the impact, the paper finds that joining cooperatives helped increase farmers’ adoption of green production techniques, much as many scholars have done (Cai and Han, 2012; Abebaw and Haile, 2013; Wossen et al., 2017; Ma and Abdulai, 2019; Wan and Cai, 2021). We found that participation in cooperatives increased the probability of apple farmers adopting green production techniques from 25.29% to 30.29%, verifying that participation in cooperatives contributed significantly to fruit farmers’ adoption of green production techniques. It is an important measure to promote the transformation of agriculture to green production to guide farmers to join cooperatives and take the road of large-scale and standardized management.
In a mechanistic analysis, this paper proves that collective action, such as technical training, standardized production, and unified marketing, can reduce farmers’ adoption risks. This group behavior and internal communication mechanisms among cooperative members enhance farmers’ perceptions of green production, which is more consistent with existing research (Odoemenem and Obinne, 2010; Feng and Huo, 2016; Geng et al., 2017; Wan and Cai, 2021). However, the marginal contribution of this paper lies in finding and validating other possible implementation approaches. Specifically, we find that even when preferential services were provided to apple farmers participating in cooperatives, green products still cost 1178 USD/ha more than traditional production methods. However, the participants’ product prices and net profits were 0.142USD/kg and 1829USD/ha, respectively, higher than traditional producers. Therefore, we believe that the key to incentivizing participants to adopt green production technologies may lie in ensuring that the ultimate economic benefits of farmers’ green production are higher than traditional agricultural production methods. Cooperatives should therefore aim to increase the price and net profit of their products by, for example, broadening distribution channels through e-commerce sales, and increasing the market recognition of their products through mechanisms such as product quality certification, traceability of origin and product demonstration. At the same time, procurement costs have been reduced through collective procurement of production materials and technical equipment, or by actively applying for additional policy subsidies and incentives.
It is worth noting that this depends to a large extent on the quality of cooperative development because the laws and regulations, and systems governing the normative development of cooperatives in China are not yet sound, leading to the emergence of a large number of “fake cooperatives.” The lack of a properly regulated internal governance system severely reduces the effectiveness of cooperative technology promotion, technology demonstration, and technology radiation (Pu et al., 2014). Therefore, efforts should be made to establish and improve cooperative governance mechanisms and service functions, improve the applicability, convenience, and economy of green production technologies, and provide organizational support and product security for the popularization and application of green production technologies.
6 Research conclusion
Green production is an important means to solve environmental problems in agricultural production. In China, where pesticides and fertilizers are overused, green production has been paid more and more attention by academia. Based on a household survey of 314 apple growers in Baishui County, Shaanxi Province, and Qingcheng County Gansu Province, from November 2020 to October 2021, this paper constructs a counterfactual framework using propensity score matching to empirically examine the impact of farmer participation in cooperatives on green production technology adoption.
We found that participation in cooperatives had a significant positive impact on farmers’ adoption of green production technologies. More precisely, if farmers participate in cooperatives, the probability of adopting green production technologies increases by 5%. It is worth noting that while farmers’ participation in cooperatives will lead to more significant investment, improved product quality will increase farmers’ economic efficiency in terms of sales prices and net income. In addition, the social services provided by cooperatives, such as technical training, agricultural supply, product sales, and surplus product distribution, can effectively raise farmers’ cognition of green production and reduce the risk expectations of farmers adopting green production technologies. In other words, despite the increase in inputs from farmers, farmers’ participation in cooperatives, higher product prices, higher levels of cognition, higher net income, and lower risk expectations all contribute to the choice of green production technologies.
The study also has two significant limitations. First, we use data from Shaanxi and Gansu Province, two major apple-producing regions in China, which may lead to regional particularities and may not fully reflect the participation of the China apple farmers’ Cooperative. More detailed survey data are required to describe China’s situation. Nevertheless, we believe the outcome will not change, as most apple production is concentrated in northwestern China and Shandong Province. This is despite high consumer approval of the quality of apples grown in Shandong Province.
On the other hand, the structure of agricultural production in Northwest China is similar. The most representative provinces are Shaanxi and Gansu, with diverse topography and topography. Secondly, our estimate of ATT may be underestimated if there are positive spillover effects from participation. For example, these non-cooperative farmers can observe and learn from Cooperative participants and change their production practices to be more environmentally sustainable. In future studies, it may be worth exploring whether apple farmers participating in cooperatives have spillover effects.
7 Research implications
In response to the current problem of the limited size of cooperatives, governments at all levels should strengthen their support for cooperatives in terms of capital, technology, human resources and projects, in particular subsidies and preferential policies for cooperatives to purchase green production technologies and equipment and provide services. In view of the current situation of unregulated development within cooperatives, which is loosely governed and focuses only on economic benefits, we will speed up the formulation of laws and regulations to promote the normative and high-quality development of cooperatives, strengthen the construction of internal governance systems of cooperatives and strengthen supervision of their operation. Thirdly, we should integrate the service functions of various types of cooperatives, focus on supporting cooperatives with comprehensive production management and standardized production, and give better play to the leading role of cooperatives in green production. In response to the above research findings, this paper puts forward the following policy revelations: Firstly, it attaches importance to the critical role of farmers’ professional cooperatives in the promotion of green production technology and encourages regions to establish a joint agricultural technology promotion service system with agricultural technology centers (stations and institutes) as the core, new agricultural business entities such as agricultural cooperatives as the backbone, and multiple entities such as research institutes and leading agricultural enterprises to participate jointly, and carry out a The system is based on new agricultural business entities such as agricultural cooperatives, with the participation of multiple entities such as research institutes and leading agricultural enterprises. Secondly, we will strengthen farmers’ professional cooperatives’ support, guidance, and supervision and promote their normative development. In response to the current problem of the limited size of cooperatives, governments at all levels should strengthen their support for cooperatives in terms of capital, technology, human resources, and projects, in particular subsidies and preferential policies for cooperatives to purchase green production technologies and equipment and provide services. In view of the current situation of unregulated development within cooperatives, which is loosely governed and focuses only on economic benefits, we will speed up the formulation of laws and regulations to promote the normative and high-quality development of cooperatives, strengthen the construction of internal governance systems of cooperatives and strengthen supervision of their operation. Thirdly, we should integrate the service functions of various cooperatives, focus on supporting cooperatives with comprehensive production management and standardized production, and give better play to the leading role of cooperatives in green production.
Data availability statement
The raw data supporting the conclusion of this article will be made available by the authors, without undue reservation.
Author contributions
DJL contributed to design of the study. DJL and JY finished the field experiments. DJL performed the data analysis. DJL wrote the first draft of the manuscript. DJL wrote sections of the manuscript. DJL and HMD revised the grammar, spelling and word presentation of the article. DJL, HMD, and JY completed the revision of the reviewers’ opinions. All authors contributed to manuscript revision, read, and approved the submitted version.
Funding
This research was funded by the National Key R&D Program of China (grant numbers 2017YFE0181100), the Northwest Agriculture and Forestry University Program (Grant Numbers TGZX 2021–44), and the College of Economics and Management in Northwest Agriculture and Forestry University Postgraduate Scientific Research Innovation Project (Grant Numbers JGYJSCXXM202205).
Conflict of interest
The authors declare that the research was conducted in the absence of any commercial or financial relationships that could be construed as a potential conflict of interest.
The reviewer SH declared a shared affiliation with the authors to the handling editor at the time of review.
Publisher’s note
All claims expressed in this article are solely those of the authors and do not necessarily represent those of their affiliated organizations, or those of the publisher, the editors and the reviewers. Any product that may be evaluated in this article, or claim that may be made by its manufacturer, is not guaranteed or endorsed by the publisher.
Supplementary material
The Supplementary Material for this article can be found online at: https://www.frontiersin.org/articles/10.3389/fenvs.2022.1026273/full#supplementary-material
References
Abebaw, D., and Haile, M. G. (2013). The impact of cooperatives on agricultural technology adoption: Empirical evidence from Ethiopia. Food Policy 38, 82–91. doi:10.1016/j.foodpol.2012.10.003
Becker, S. O., and Ichino, A. (2002). Estimation of average treatment effects based on propensity scores. Stata J. 2, 358–377. doi:10.1177/1536867X0200200403
Cai, J. (2013a). Analysis of the impact of financial lending on agricultural technology adoption under different capital endowments. Forum Sci. Technol. China 93, 98–104. doi:10.13580/j.cnki.fstc.2013.10.016
Cai, R. (2013b). Contractual arrangements in agricultural cooperatives: Allocation of production decision rights :an empirical analysis based on farmers’ perspective. Chin. Rural. Econ. 60, 70–78.
Cai, R., and Han, H. (2012). Analysis of farmers’ behavioral decision to participate in cooperatives and its influencing factors-Apple farmers in Shandong Province as an example. China Rural. Surv. 32, 40–95.
Cai, S. (2012). Exploring the determinants of adoption behaviour of clean technologies in agriculture: A case of integrated pest management. Analysis farmers’ IPM Technol. Adopt. Behav. its Eff. 20, 67–82. doi:10.1080/19761597.2012.681435
Carter, M., Laajaj, R., and Yang, D. (2014). Subsidies and the persistence of technology adoption: Field experimental evidence from Mozambique. Cambridge, MA: National Bureau of Economic Research.
Chatzimichael, K., Genius, M., and Tzouvelekas, V. (2014). Informational cascades and technology adoption: Evidence from Greek and German organic growers. Food Policy 49, 186–195. doi:10.1016/j.foodpol.2014.08.001
Chen, C., Kong, Y., and Xu, L. (2021). The impact of regional public branding and standardized production on fruit farmers’ business income : An empirical test based on 771 fruit farmers in ji, su and Gan provinces. J. Agro-Forestry Econ. Manag. 20, 569–577. doi:10.16195/j.cnki.cn36-1328/f.2021.05.59
Chen, S. (1995). Objective weighting methods for indicators in integrated evaluation. Shanghai Stat. 16, 18.
Chèze, B., David, M., and Martinet, V. (2020). Understanding farmers’ reluctance to reduce pesticide use: A choice experiment. Ecol. Econ. 167, 106349. doi:10.1016/j.ecolecon.2019.06.004
Chu, C., Feng, S., and Zhang, W. (2012). An empirical analysis of farmers’ behavior in adopting environmentally friendly agricultural technologies: An example of organic fertilizer and soil testing and fertilization technologies. Chin. Rural. Econ. 28, 68–77.
Cui, Z., Zhang, H., Chen, X., Zhang, C., Ma, W., Huang, C., et al. (2018). Pursuing sustainable productivity with millions of smallholder farmers. Nature 555, 363–366. doi:10.1038/nature25785
Dillon, A. (2011). The effect of irrigation on poverty reduction, asset accumulation, and informal insurance: Evidence from northern Mali. World Dev. 39, 2165–2175. doi:10.1016/j.worlddev.2011.04.006
Du, B., Kang, J., and Li, S. (2014). Analysis of factors influencing farmers’ willingness to produce safely. J. Northwest A&F Univ. Sci. Ed. 10, 71–75. doi:10.13968/j.cnki.1009-9107.2014.03.014
Feng, x., and Huo, x. (2016). A study of social networks’ incentives for farmers to adopt environmentally friendly technologies. J. Chongqing Univ. Sci. Ed. 22, 72–81. doi:10.11835/j.issn.1008-5831.2016.03.010
Gao, X. (2013). A study on the behavior of large rice farmers applying low-carbon agricultural technology. Sci. Technol. Manag. Res. 33, 113–116. doi:10.3969/j.issn.1000-7695.2013.14.025
Geng, y., Zheng, s., and Lu, q. (2017). The influence of economic incentives and social networks on farmers’ green control technology adoption behaviour - evidence from a major kiwifruit producing area in Shaanxi. J. Huazhong Agric. Univ. Sci. Ed. 37, 59–69+150. doi:10.13300/j.cnki.hnwkxb.2017.06.008
Geng, Y., Zheng, S., and Wang, J. (2016). The influence of government extension and supply chain organizations on farmers’ adoption behavior of biological control technologies. J. Northwest A&F Univ. Sci. Ed. 17, 116–122. doi:10.13968/j.cnki.1009-9107.2017.01.16
Genius, M., Koundouri, P., Nauges, C., and Tzouvelekas, V. (2014). Information transmission in irrigation technology adoption and diffusion: Social learning, extension services, and spatial effects. Am. J. Agric. Econ. 96, 328–344. doi:10.1093/ajae/aat054
Guo, H., Jolly, R. W., and Zhu, J. (2007). Contract farming in China: Perspectives of farm households and agribusiness firms. Comp. Econ. Stud. 49, 285–312. doi:10.1057/palgrave.ces.8100202
Guo, l., and Zhao, j. (2017). A study on farmers’ willingness to apply biopesticides from the perspective of cognitive conflict: Empirical evidence based on 639 rice farmers in jiangsu. J. Nanjing Agric. Univ. Sci. Ed. 17, 123–133.
Haller, A. (2022). Influence of agricultural chains on the carbon footprint in the context of European green pact and crises. Agriculture 12, 751. doi:10.3390/agriculture12060751
Huang, J., Qi, L., and Chen, R. (2008). Technical information knowledge, risk preferences, and farmers’ pesticide application. J. Manag. World 24, 71–76.
Huang, Y., Luo, X., Li, R., and Zhang, J. (2018). Farmers’ perceptions, external environment, and willingness to produce green agriculture based on 632 farmers’ research data in Hubei Province. Resour. Environ. Yangtze Basin 27, 680–687.
Li, D., Zhou, H., and Zhou, L. (2021). Brand premiums and quality and safety of agricultural products : An example from rice cultivation in jiangsu. J. Finance Econ. 47, 34–48. doi:10.16538/j.cnki.jfe.20200918.402
Li, X., Chen, Z., Liu, F., and Xia, X. (2020). Does participation in e-commerce promote green production technology adoption among kiwifruit growers? -Counterfactual estimation based on propensity score matching. Chin. Rural. Econ., 118–135.
Liu, H., Liu, Y., and Fu, X. (2021). Can standardised production services by cooperatives boost farmers’ incomes? Rural. Econ. 39, 55–62.
Liu, Y., Sun, D., Wang, H., Wang, X., Yu, G., and Zhao, X. (2020). An evaluation of China’s agricultural green production: 1978–2017. J. Clean. Prod. 243, 118483. doi:10.1016/j.jclepro.2019.118483
Liu, z., and Zhou, j. (2018). Information capability, environmental risk perception and adoption of pro-environmental behaviour by farmers - an empirical test based on broiler farmers in Liaoning Province. J. Agrotechnical Econ. 37, 135–144. doi:10.13246/j.cnki.jae.20181009.003
Luo, X., Feng, S., and Shi, X. (2013). Evaluation of farmers’ environmental-friendly technology adoption behavior and its environmental and economic effects in Taihu Lake Basin: An example of soil testing and fertilizer application technology. J. Nat. Resour. 28, 1891–1902. doi:10.11849/zrzyxb.2013.11.006
Ma, W., and Abdulai, A. (2016). Does cooperative membership improve household welfare? Evidence from apple farmers in China. Food Policy 58, 94–102. doi:10.1016/j.foodpol.2015.12.002
Ma, W., and Abdulai, A. (2019). IPM adoption, cooperative membership and farm economic performance: Insight from apple farmers in China. CAER 11, 218–236. doi:10.1108/CAER-12-2017-0251
Ma, X., and Huo, X. (2019). Standardized apple production, regulation effects and suggestions for improvement : A survey and analysis based on 960 apple farmers in 11 counties in Shandong, Shaanxi and Gansu provinces. Issues Agric. Econ. 40, 37–48. doi:10.13246/j.cnki.iae.2019.03.004
Mao, H., Luo, X., Tang, J., and Huang, Y. (2021). Adoption decisions for multiple green production technologies: Influencing factors and correlation analysis. J. China Agric. Univ. 26, 231–244. doi:10.11841/j.issn.1007-4333.2021.06.23
Mmbando, F., Mbeyagala, E., Binagwa, P., Karimi, R., Opie, H., Ochieng, J., et al. (2021). Adoption of improved mungbean production technologies in selected east african countries. Agriculture 11, 528. doi:10.3390/agriculture11060528
Mojo, D., Fischer, C., and Degefa, T. (2017). The determinants and economic impacts of membership in coffee farmer cooperatives: Recent evidence from rural Ethiopia. J. Rural Stud. 50, 84–94. doi:10.1016/j.jrurstud.2016.12.010
Odoemenem, I., and Obinne, C. (2010). Assessing the factors influencing the utilization of improved cereal crop production technologies by small-scale farmers in Nigeria. Indian J. Sci. Technol. 3, 180–183. doi:10.17485/ijst/2010/v3i2.23
Pu, x., Fan, w., and Lu, l. (2014). Evolutionary analysis of equity preferences, strong reciprocity tendencies, and production norms of farmers’ cooperatives. J. China Agric. Univ. Sci. 31, 51–62. doi:10.13240/j.cnki.caujsse.2014.01.004
Quan, S., and Zeng, Y. (2014). Food safety: Consumers’ labeling choices and self-protective behaviors. China Popul. Resour. Environ. 24, 77–85. doi:10.3969/j.issn.1002-2104.2014.04.011
Ragasa, C., Lambrecht, I., and Kufoalor, D. S. (2018). Limitations of contract farming as a pro-poor strategy: The case of maize outgrower schemes in upper west Ghana. World Dev. 102, 30–56. doi:10.1016/j.worlddev.2017.09.008
Ren, Y., Peng, Y., Castro Campos, B., and Li, H. (2021). The effect of contract farming on the environmentally sustainable production of rice in China. Sustain. Prod. Consum. 28, 1381–1395. doi:10.1016/j.spc.2021.08.011
Ren, Z., and Hussain, R. Y. (2022). A mediated–moderated model for green human resource management: An employee perspective. Front. Environ. Sci. 10, 973692. doi:10.3389/fenvs.2022.973692
Rosenbaum, P. R., and Rubin, D. B. (1983). The central role of the propensity score in observational studies for causal effects. Biometrika 70, 41–55. doi:10.1093/biomet/70.1.41
Solomon, S. (2007). Climate change 2007: The physical science basis: Contribution of working group I to the fourth assessment report of the intergovernmental panel on climate change. Cambridge ; New York: Cambridge University Press. Intergovernmental Panel on Climate Change, and Intergovernmental Panel on Climate Change.
Soullier, G., and Moustier, P. (2018). Impacts of contract farming in domestic grain chains on farmer income and food insecurity. Contrasted evidence from Senegal. Food Policy 79, 179–198. doi:10.1016/j.foodpol.2018.07.004
Tang, L., and Zhou, J. (2018). Social capital, information acquisition and Farmers’Behavior of electronic commerce. J. South China Agric. Univ. Sci. Ed. 17, 73–82. doi:10.7671/j.issn.1672-0202.2018.03.007
Tong, h., and Liu, w. (2017). An empirical study on the factors influencing farmers’ adoption of straw regranting technology - based on survey data from 311 households. Rural. Econ. 35, 108–114.
Wan, L., and Cai, H. (2021). Study on the impact of cooperative participation on farmers’ adoption of soil testing and fertilizer application technology-based on the perspective of standardized production. J. Agrotechnical Econ. 40, 63–77. doi:10.13246/j.cnki.jae.2021.03.005
Wang, S., and Liu, X. (1996). Analysis of poor farmers’ behavior in accepting new technologies under incomplete information. Issues Agric. Econ. 17, 31–36. doi:10.1007/BF02943147
Wang, Y., Zhu, Y., Zhang, S., and Wang, Y. (2018). What could promote farmers to replace chemical fertilizers with organic fertilizers? J. Clean. Prod. 199, 882–890. doi:10.1016/j.jclepro.2018.07.222
Wossen, T., Abdoulaye, T., Alene, A., Haile, M. G., Feleke, S., Olanrewaju, A., et al. (2017). Impacts of extension access and cooperative membership on technology adoption and household welfare. J. Rural Stud. 54, 223–233. doi:10.1016/j.jrurstud.2017.06.022
Wu, B., Liu, J., Xu, X., and Zhang, Z. (2016). A study on the impact of farm household organization on farmers’ technology adoption. J. Agrotechnical Econ., 25–33. doi:10.13246/j.cnki.jae.2016.08.003
Yang, Z. (2018). Aging, social networks, and farmers’ green production technology adoption behavior-validation from data of farmers in six provinces in the Yangtze River basin. China Rural. Surv. 40, 44–58.
Zhang, C., Liu, Y., Lu, Z., Feng, J., Xu, W., and Yang, Z. (2020). Global research status and trends of UKA for knee osteoarthritis: A bibliometric analysis. Arthroplasty 42, 20–26+92. doi:10.1186/s42836-020-00039-3
Zhang, Q., Zhou, H., Lv, S., and Hu, N. (2013). Analysis of factors influencing farmers’ willingness to participate in cooperatives: A case study in acheng city, heilongjiang province. J. Agrotechnical Econ., 98–104. doi:10.13246/j.cnki.jae.2013.03.009
Zhang, T., Yan, T., He, K., and Zhang, J. (2017). The influence of capital endowment on farmers’ willingness to invest in green production: The case of straw returning to farmland. China Popul. Resour. Environ. 27, 78–89. doi:10.12062/cpre.20170422
Zhang, T., Zho, J., Hussain, R. Y., Wang, M., and Ren, K. (2022). Research on the cultivation of green competitiveness among Chinese heavily polluting enterprises under country/district environmental regulations. Front. Environ. Sci. 10, 955744. doi:10.3389/fenvs.2022.955744
Zhang, W., Cao, G., Li, X., Zhang, H., Wang, C., Liu, Q., et al. (2016). Closing yield gaps in China by empowering smallholder farmers. Nature 537, 671–674. doi:10.1038/nature19368
Zhang, Y., Sun, X., and Han, Y. (2019). Influence of social networks and awareness of water conservation on the adoption of water-saving technologies in wheat production - based on farm household survey data in Jilu and Henan. J. Agrotechnical Econ. 38, 127–136. doi:10.13246/j.cnki.jae.2019.11.011
Zhao, L., and Cai, S. (2012). Analysis of factors influencing farmers’ IPM technology adoption behavior: Empirical evidence based on wuhu city, anhui province. Issues Agric. Econ. 33, 50–57+111.
Keywords: cooperatives, green production technology, technology adoption, propensity score matching, sustainable development, China
Citation: Li D, Dang H and Yu J (2022) Can participation in cooperatives promote the adoption of green production techniques by Chinese apple growers: Counterfactual estimation based on propensity score matching. Front. Environ. Sci. 10:1026273. doi: 10.3389/fenvs.2022.1026273
Received: 23 August 2022; Accepted: 02 November 2022;
Published: 16 November 2022.
Edited by:
Zeeshan Fareed, Huzhou University, ChinaReviewed by:
Md. Shakhawat Hossain, Northwest A&F University, ChinaApurbo Sarkar, The University of Queensland, Australia
Ilyas Ahmad, University of Education Lahore, Pakistan
Copyright © 2022 Li, Dang and Yu. This is an open-access article distributed under the terms of the Creative Commons Attribution License (CC BY). The use, distribution or reproduction in other forums is permitted, provided the original author(s) and the copyright owner(s) are credited and that the original publication in this journal is cited, in accordance with accepted academic practice. No use, distribution or reproduction is permitted which does not comply with these terms.
*Correspondence: Jin Yu, eXVqaW5AbndhZnUuZWR1LmNu