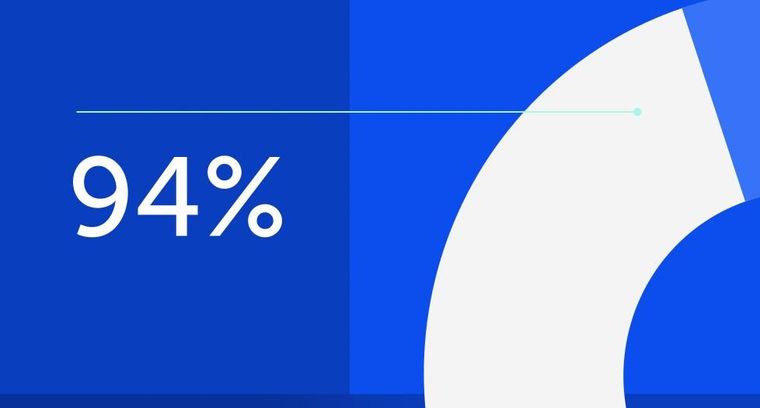
94% of researchers rate our articles as excellent or good
Learn more about the work of our research integrity team to safeguard the quality of each article we publish.
Find out more
ORIGINAL RESEARCH article
Front. Environ. Sci., 12 October 2022
Sec. Land Use Dynamics
Volume 10 - 2022 | https://doi.org/10.3389/fenvs.2022.1025646
This article is part of the Research TopicLand Use Management and Carbon Abatement in a Sustainable Development PerspectiveView all 12 articles
Nowadays, Big Data Analytics (BDA) attracted considerable interest due to its tremendous social and scientific value. The use of traditional rural education land is being replaced by the emerging network classroom. With the fast-growing data and information numbers at present, the IT world’s educational system are changing rapidly. The performance of rural school students is less satisfactory, and many rural schools have disadvantageous of having distanced from a nearby university. The schools in the countryside are located in the remote land. Rural schools are smaller and costlier to operate and likely to have a teacher shortage. These problems are all expected to be solved by the application of big data. In this paper, Big Data Analytics driven Integrated Online Learning Framework (BDAIOLF) has been proposed for rural education. The study showed that the conditions, social impact, performance, and effort expectations facilitating behavioral intentions have been essential determinants for the use of ICT for educational purposes. Data have been collected through a literature study, interviews between individuals and focus groups. Key findings indicated that rural school success is influenced by a number of factors relating to the core values of education, the teacher-learner relationship, the proficiency of English, and parental participation in teaching and learning. In rural schools, however, teaching and learning are influenced by factors such as topics, the work environment, genetic characteristics, and environmental conditions. The construction of information infrastructure is an important reason for the reduction of rural education land.
Big Data Analytics is one of the most deliberate phenomena in new information technologies and education (Arumugam and Bhargavi, 2019; Wu et al., 2022; Luo et al., 2022). Citizens benefit from quality service all over the world through advancement in computer technology and increasing digitalization of services (Kelley et al., 2018; Kumari et al., 2018). In the digitization of public services; however, large electronic data are generated with the help of Smartphones, Video records, and smartphone applications like WhatsApp, Facebook, Twitter, and much more (Yuan et al., 2018; Owadally et al., 2019). Internet connection produces an enormous amount of information. In this context, the development of large data relates to innovative methods to collect, store, and process information to increase its availability and usability (Wu et al., 2021; Ren at al., 2021). The term Big Data defines the huge quantity of structured and unstructured data that affects an organization daily (Nieto et al. ., 2019a; Nguyen et al., 2019). Big data can be analyzed to gain insights that lead to strategic organizational choices. Basic education is now known to be a major point for development in society, meaning education and learning for all (Kitchens et al., 2018). Universities, researchers, industry, and public authorities have worked together in the transformation of society and the world economy. The invention of new technology transformed educational services from traditional forms to online services (Shakeel and Baskar, 2020). The proper technology management must ensure that these services are properly offered in rural areas quickly and reliably. To enable online services, universities will concentrate on infrastructure and maximize the quality and effectiveness of educational material (Nieto et al., 2019b; Manogaran et al., 2019).
Rural development generally describes how people in relatively isolated and carefully populated areas improve their quality of life and their economic well-being (Kamau and Weda, 2019). The character of rural areas has changed, however, with the change in global production networks and increased urbanization. In rural development, there is a significant role in education, economic development, physical and social infrastructure (Zhang et al., 2015; Hao et al., 2022). The emphasis on local economic growth approaches is often typical of rural development (Jia et al., 2022; Su et al., 2021). In contrast, rural areas are highly distinctive with each other in urban regions that have many similarities (Janarthanan et al., 2020; Wu et al., 2020). Therefore, a wide variety of approaches to rural development are used worldwide. And many of the developing countries currently have very strong initiatives for rural growth. Take China as an example. In 2017, the Chinese government put forward the “rural revitalization strategy” for the first time, and subsequently issued a series of policy documents to make major strategic arrangements for it, and brought rural development to an important level (Zhang et al., 2022).
Rural government policy is primarily concerned with the development of undeveloped villages (Li et al., 2022; Chunxiang et al., 2022). Locally-controlled, practical, implemented, problematic, and functional qualification-oriented education that contributes to rural development (Talonen et al., 2018). It diagnoses their needs, enforces their rights and takes more control of decisions affecting their lives, provides a trained workforce for rural and urban areas, connects rural and urban sectors, provides opportunities for employment and income, and increases productivity and leadership (Carfora et al., 2019). The purpose of rural development is to find ways to enhance rural life with rural people’s engagement to meet the demands of rural areas (Marano, 2019; Carlsen, 2021). The community cannot comprehend the nation’s location, culture, language, and others. As such, people, in general, must participate in their sustainable rural development themselves (Li et al., 2018; Lyubchich et al., 2019; Zhang et al., 2019). In developing countries, relevant, locally-controlled education programs are central to rural development. Education has advisable control over the development of rural people, families, communities, and society, leading to poverty reduction, income equity, and managed unemployment (Wiegard et al., 2019; Castillo et al., 2021; Gorelick and Walmsley, 2020). In rural supply, production, marketing, maintenance of personnel, education, health care, and government, education has an essential role (Pousttchi and Gleiss, 2019). Learning functions include imparting progressive reform, improving people’s social positions and living standards, encouraging rural and cultural development participatory participation, enhancing the essential skills of rural people to diagnose their needs, ensuring their rights, and increasing their control over decisions that affect their lives (Staudt and Wagner, 2018; Song et al., 2021). Figure 1 provides the process of big data analysis and innovative teaching in rural education.
In this paper, the Big Data Analytics-Driven Integrated Online Learning Framework (BDAIOLF) has been proposed for rural education. This study explores the role of big data analytics in resolving current rural education challenges. The key findings show that the success of rural school learners is affected by numerous factors linked to the basis for learning, the teacher-learner ratio, English skills, and parental participation in teaching and learning. In rural schools teaching and learning are based on factors such as subject-specificity, workplace culture, biological factors, and weather conditions. The findings revealed various factors to explain the standard of students’ performance in rural schools. It advises that the level of learning and teaching in rural schools should be better supported by these variables. Online learning consists of internet instruction, network education, or independent computer training, it essentially transmits skills and knowledge across the network (Xu et al., 2020; Scheibner et al., 2021). With the global expansion of the digital economy, some countries have also begun to consciously promote the integration of digitalization and rural education. For example, in recent years, China’s digital economy has been booming and has been firmly ranked second in the world for several years (Ren et al., 2022). Taking the development of digital economy as an opportunity, China promulgated the “Strategic Plan for Rural Revitalization (2018–2022)" in 2018, which clearly emphasized that rural education should be prioritized, the “Internet plus education” should be used to promote the construction of information infrastructure in rural schools, and the public service system of digital education resources should be optimized.
In this paper, the Big Data Analytics-Driven Integrated Online Learning Framework (BDAIOLF) has been proposed for rural education. Big data concepts and analytics are available for various applications, involving recruiting and processing admissions, donor tracking, financial planning, and student outcomes tracking, in the rural education sector. Online learning is an innovative shift in learning, allowing quick access to particular information and knowledge. Online learning allows administrations, through a coherent virtual learning environment, to overcome distances and other organizational gaps (Kaur and Pandey, 2021; Qiu and Li, 2021). Companies must educate, train, and provide cost-effective, just-in-time training to vendors, personnel, partners, and clients.
The contributions of this paper are mainly reflected in the following three aspects: First, although previous studies have noted the role of digitalization in promoting rural education, most of them discuss solutions to improve rural education based on the overall concept of digitalization. Based on the specific technology of big data analysis, this paper has designed a Big Data Analytics driven Integrated Online Learning Framework in detail, which provides a more feasible scheme for digital promotion of rural education. Secondly, previous studies rarely tested the proposed model or scheme in terms of data (Appiahene et al., 2019; Zhou, 2019), while this paper collected data through literature research, interviews between individuals and focus groups and tested the actual effect of the proposed scheme. Finally, this paper also calculates the effect difference between the previous scheme and the scheme proposed in this paper, and draws relevant charts to show the advantages and disadvantages of each scheme in the implementation effect more intuitively.
The remainder of the paper discussed as follows: Introduction Section and Literature Review Section discussed the background and existing approaches to rural education. In Big Data Analytics-Driven Integrated Online Learning Framework Section, the Big Data Analytics driven Integrated Online Learning Framework (BDAIOLF) has been proposed. In Numerical Results Section, the numerical results have been performed. Finally, Conclusion and Research Summary Section concludes the research paper.
Although digital teaching has achieved leapfrog progress in recent decades, some researchers have pointed out that in economically underdeveloped areas, some rural areas and families of disadvantaged groups, there are still problems such as imperfect information technology infrastructure and inadequate online learning preparation (Zhang et al., 2020). Other researchers also suggest that rural schools lack computer rooms for students to practice and operate, and the information equipment is single and insufficient. Schools of different sizes have unfair information equipment (Mo et al., 2014). There are insufficient teaching platforms and resources in some economically underdeveloped and remote and poverty-stricken areas, and few intelligent teaching platforms and tools based on AI + big data are available. Teaching support services are still relatively weak (Fengwei and Nan, 2020). In rural areas, there are insufficient supporting conditions for online teaching, insufficient access equipment for online education, insufficient information awareness of teachers and students, and resistance to online teaching (Wu et al., 2019).
In view of the above problems, some studies focus on the improvement of rural online education resources. Some scholars stressed that multi-body interaction should solve the various difficulties of online teaching in weak rural schools, build a joint construction and sharing of urban and rural integrated online education resource supply system, and promote the solution of the digital divide between urban and rural schools (Liu et al., 2020). Other scholars give more specific scheme design. Tran and Smith (2020) proposed the Convergent Parallel Mixed Methods Analysis (CPMMA) for recruiting teachers to rural hard-to-staff districts and using a multi attribute process for providing a utility analysis compared for a sample of university students from a south-east regional university, the relative importance of work characteristics. There was then a heterogeneity analysis to look at subgroup results. Finally, qualitative data were gathered and combined with quantitative findings in the survey and the interviews to establish points of convergence. The most influential considerations in the complicated-to-work district of the respondents were the various methods of analyzing, administrative support, self-confidence, and potential connections with the students. The findings of the research show that importance is granted to these areas in political discussions. Zhou (2019) suggested the Path Analysis (PA) for rural community education services on rural revitalization strategy in china. The development of rural community education is the fundamental premise of the construction of a modern countryside, and an important way of training new quality rural citizens. Rural community training is the key strategical support to the recovery of rural life and rural community education (Perry et al., 2021; Wang et al., 2022). Rural community education in China faces limitations and challenges such as low standing, the imperfection of the system, weak faculty, and weak sustainability. This paper proposes specific strategies for development based on the present rural-community education situation to promote better rural community development in China and help rural areas revitalize rural areas as soon as possible. Beach et al. (2018) introduced the Meta- Ethnographic Analysis (MEA) for educational and spatial justice in rural and urban areas. The inhabitants of remote, sparingly populated rural areas and suburbs in suburban areas often refer to their background, familial attitude to education, and sometimes to their culture, as having educational problems and lack and that this leads to educational problems that they face. In the last decades, ethnographic researchers have paid much attention to their promotion of school options and schools for the benefit, and this research focuses exactly on this topic. Appiahene et al. (2019) suggested the Ad-Hoc Networks Model (Ad-Hoc-NM), which provides education to rural communities. In their suggested system, ad-hoc wireless networks are utilized to distribute different types of information/data. In every rural area, at least one would be located in the municipal capital with a tele center equipped with computers, including multimedia to store data. Rural citizens can have access to this knowledge via wireless connections to tele center computers by linking their mobile devices. The Government aims to effectively implement this model to address the educational divide between citizens in rural and urban Ghana and to offer immersive education to large numbers of people from Ghana.
In conclusion, although previous studies have paid some attention to rural digital education, they are still relatively insufficient. On the one hand, there are few studies on the combination of big data and rural education. On the other hand, the solutions proposed in previous studies lack data illustrating the effects, which makes it difficult to test or prove the effectiveness of the solutions/models. To address the above shortcomings, this paper proposes a Big Data Analytics-Driven Integrated Online Learning Framework (BDAIOLF) for rural education, and collects data through literature research, interviews between individuals and focus groups to examine the practical effects of the proposed scheme.
In this paper, the Big Data Analytics-Driven Integrated Online Learning Framework (BDAIOLF) has been proposed for rural education. Equality of education in rural areas is still lower than in urban areas since education itself is the key to mitigate poverty and improve the conditions of life of rural people, and education quality is even worse. The government should focus on providing special education for rural students, entry into school for exceptions, support for teachers, improving the educational environment, offering free school busses, creating more scholarships, learning from native English teachers, and so on.
As shown in the Figure 2. Big Data provides a technologically creative study platform for world-class researchers and examines the complexities and opportunities to collect, analyze, and distribute large quantities of data, from computer technology, Digital Computer, and processing systems and computation. The increase in mobile and digital connectivity has rendered the world more interactive, related, and perceptible and has usually contributed to these large-scale data sets being made available. The Big Data Sets installers to progress interfaces to analyze and access certain data by anyone; for example, Google offers Google Insights freely available, while people are reluctant to give access to them. The coverage involves new technology, big-data industry standards especially advanced for big data, data procurement, distribution, cleaning, best performs privacy and policy, data protection, research into products’ business benefits, changing business intelligence roles, visualizing and designing of large-data infrastructure, physical interfaces, and robot engineering. The most common term used when referring to distance learning in distance education. It often describes how people who are locally distant can access learning. Online learning is fundamentally the allocation of expertise and information across a network. Online learning means the use of online benefits and learning processes. Web-based education, computer-oriented learning, digital cooperation, and virtual classrooms are the key applications for online learning. Online learning content is provided through the Internet, intranet/extranet, CD-ROMs video/audio, and Satellite TV. Figure 3 shows big data analytics’ essential stages.
Students and teachers come from a diversity of circumstances and have various reasons. While online learning practitioners are widely known for the use of intrinsic motivation techniques, external motivation may be utilized. A student can only be extrinsically motivated to do what is necessary to pass units without a significant deep focus on the subject. Students learning in distance style must be aware that they belong to a student’s groups and that they have access to support for the needs and technical difficulties of the unit. It is necessary to incorporate students who are intrinsically motivated by the desire to deepen their understanding of the subject contents, which promotes a deeper considerate of the topic and real and occupational environment. Big data is the data generated by learners during an online learning course or a training module in terms of the e-learning industry. Figure 4 shows big data analytics in online learning. The proposed recommendation system for courses consists of two roles: teachers and students and administrative staff. This involves the following components: online learning platform and MySQL database servers that store and maintain data produced during learner experiences like course registrations, learner behavior, etc. The architecture describes various steps in the discovery, modeling, processing, and visualization of data to identify useful rules efficiently between all courses.
The online learning model offers support to self-directed learning, individuals through tools for accessing learning resources, and through the use of evaluation instruments and tools (essays, quizzes, etc.). Learning Management System includes coordinated and asynchronous (e.g., interactive chats) resources to allow it easier to make social awareness sharing effective and avoid a lack of direct interaction between students and teachers and amongst pupils. With the transition of learning into all-round learning and interactions, anytime message boards become an important part of online social learning. Interactions, involvement, social exchange, and processes of discussion and debate-led knowledge building occur mainly in course forums in formal online learning contexts. It is, therefore, only natural to describe, elucidate, and comprehend the social dynamics of online forums through an important stream of research.
Online learning is demanding in the utilization of information technologies, and educational methods have the potential to prefer social and self-directed learning. As the intricacy of the courses and the number of students registered in a course interest, it is a task for the teachers to track progress. The data saved in the databases of formal learning environments consists of valuable data to facilitate the job of the instructor. However, this data is available in raw format, and additional processing is needed for meaningful and workable information on the courses and the related social dynamics. The new discipline of learning analytics includes the collection, analyzing, and evaluation of these data to improve rural student learning outcomes. In addition, resources of any subject that are aligned with technologically adaptive skills to support the learning path result in an increase in the potential for efficient online learning through easy on-line access. Because of the initial requirements for increasing the specific performance, attempts to achieve the design of the computer interface must determine the usability of technological knowledge. To utilize potential data, to engage in the development of learning foundations and the utilization of big data can be enhanced to transform a reflective essence into an active and innovative learning environment based on the integrated resource platform.
Figure 5 shows big data analytics outcomes. Descriptive analytics is designed to describe and analyze historical student data, pedagogy, research, policy, and other administrative processes. The aim is to define model designs and report on the latest trends, such as school performance, completion levels, and graduations. Descriptive analytics often offers higher education organizations the ability to assess interactions and transactional learning and study data to detect perceivable developments and patterns which are likely to cause serious discussion. In particular, institutions with descriptive analytics can study data in LMS by examining the frequency of page views, logins, and achievement rates for a given program over time attributes for students of those who have accomplished the study and contents they often visited, etc.
Predictive analytics can allow good decisions and data-based insights for institutions. Predictive analytics purposes of estimating the probability of future events by looking at developments and recognizing associations on related problems and by detecting any future risks or possibilities. Predictive analyses might find various patterns in the data, which may not be evident with descriptive models, like population statistics and rates of completion. It can be utilized to examine students with risk behaviors, who may drop out or not follow a course early in the semester. Teachers will analyze the expected rate of completion for a given course, and teachers’ resources and curriculum are explicitly connected to their progress.
Figure 6 shows the ideal data framework that contains curriculum, administrative, department course, teaching and learning, research, and student data. Prescriptive analytics tends to help higher education institutions to evaluate their present situation and make informed selections about substitute events based on consistent and valid forecasts. It brings together analytical results from both predictive and descriptive models to evaluate and determine new ways to achieve the required results while balancing restrictions. Predictive analytics provides decision-makers to appear into their important functions for the long term and to look at (and issues) possibilities and to take the best course of action to timely manipulate the whole foresight. Big Data Analytics offers rural education to leverage existing information and to collect missing information to help them make good choices with different results.
As online education grows, universities and teachers become involved increasingly in the assumption that student learning and satisfaction in online learning environments become affected by factors. The data visualization helps to evaluate teaching, management, and research performance indicators. The research in real-time helps people to be monitored and strategies to enhance learning by reshaping and personalizing their learning experience. The education sector encountered many problems in school efficiencies, procurement of students, and retention of students, the ineffectiveness of data collection or production, and study. For this, more relevant study findings indicate high dropouts and a lower retention rate in online courses than in face-to-face lessons. It was found that rural students are satisfied with this synchronous learning employing a questionnaire and are most likely to accept online tutoring. Furthermore, students with more opportunities to communicate and discuss online learning experiences with their classmates are satisfied.
The results show that positive emotions and positive. In contrast, negative emotions like boredom will have a negative impact on the performance and self-regulated learning behavior of the students. On the other hand, an online study has shown that the nature of online learning forces students to manage and regulate their time, efforts, and motivating them to succeed and satisfy themselves. Therefore, it is important to identify the dimensions of those emotions to understand the role emotional influences in self-regulation, learning, and performance of the students to develop an effective online learning environment to foster positive emotional experiences that increase the learning and satisfaction of the students. Figure 7 shows the student’s satisfaction ratio using the proposed BDAIOLF method.
Students’ engagement, satisfaction, teaching methods, and services influenced their achievement and absorption ability. Students have to be satisfied to absorb the knowledge they receive successfully from their teachers. The aspects that have influenced and been taken into consideration, such as the total services, ways of teaching, courses, access, and convenience to facilities, the engagement of the students, and interaction with staff, would have a substantial effect on their satisfaction, success and absorption capacity. The Proposed Big Data Analytics based Online Learning Framework enhances the absorption capacity of rural students when compared to other existing approaches. Figure 8 demonstrates the student’s absorption capacity using the proposed BDAIOLF method.
A predictive model can allow teachers to lead students towards the progress of a course and to evaluate the tasks and resources most important for assessing the lesson. A predictive program enables an instructor to classify students who are under commitment immediately during a course based on the experience of this online course. When an educator receives an advisory e-mail (i.e., an e-mail requesting any difficulty), the student works hard each week and is more likely to increase his involvement. This communication is important as it measures the workload of students and discusses early school problems. The primary tasks of learning analytics in education are data collection, analysis, and feedback for students to improve their understanding. Through predictive analysis, an instructor can find out what students do with the learning material and how the assessment scores of a student are related to the level of engagement of that student. Figure 9 shows the student’s assessment score using the proposed BDAIOLF method.
The concept of big data provides opportunities to discover knowledge and to consider the value of data collection concerning innovative education, in an electronic learning environment, with vast volumes of data generated every second over the Internet. It is to encourage the educational skills of the teachers, especially in the Big Data era, in the preservation of a competitive edge to provide input on innovative teaching results. In the context of the Big Data approach, the article aims to explore the innovative teaching skills of teachers critically. By analyzing the interaction between students in a learning context and other students and teachers, students ' performance can be predicted. By analyzing the behavior of the student, it can detect the risk of students leaving the class and can take measures to retain their students at the beginning of the course. This analysis demonstrates the innovative teaching success for an informative outcome, which applies these skills to educational ideas and models in rural areas. Figure 10 shows the performance ratio using the proposed BDAIOLF method.
Big Data provides the most dynamic context through which the large data collection is successfully used, and the future of higher education is essentially developed. Big data is defined in that data volume, acquisition speed, or representation of data limits the ability to efficiently analyze using standard relation models or demands the use for effective handling of a significant horizontal scale. Big data refers to the need for data computation and storage in data-intensive applications to be distributed and paralleled. The online learning system is now able, without the intervention of a human being, to make decisions and predictions automatically through very advanced models and algorithms, an integral part of big data. An intelligent online learning network provides content sharing measurements, availability, and efficiency of the training program into consideration and at reduced costs. Figure 11 demonstrates the efficiency ratio of the suggested BDAIOLF method.
The proposed Big Data Analytics driven Integrated Online Learning Framework (BADIOLF) for rural education achieves high performance in terms of student’s satisfaction, improved assessment score and knowledge absorption capacity in the online learning environment when compared to other existing Convergent Parallel Mixed Methods Analysis (CPMMA), Path Analysis (PA), Meta-Ethnographic Analysis (MEA), Ad-Hoc networks model (Ad-Hoc-NM) methods.
This paper presents the Big Data Analytics-Driven Integrated Online Learning Framework (BDAIOLF) for rural education. It aims to support rural students to determine which courses are best suited for study by finding all interesting connections within course registrations via the database transactions. This paper clarified how big data are manipulated in the educational environment. In addition, a proposed framework has been presented for the management of big data in the online learning environment. Furthermore, soft computing intelligent techniques will speed up the functioning of the proposed model. Its performance will be improved by data mining methods. The model designed here is designed to enhance the model of big data design with a wide variety of innovative learning in the exploration of the Big Data approach. It improves the support of the learning environment for students using mobile devices to access online resources. The entire program is introduced from the huge collection of data to boost student success in the learning cycle and outcomes.
In summary, this study should contribute to providing insights into the Big Data reference model for innovative learning environment development. The findings of the experiments demonstrate the quality and effectiveness of the course recommendation program to deliver online learning and guided instruction that can direct and enable learners to the comfort of the courses.
As for the allocation of educational resources, the government should promote the co-construction and sharing of high-quality digital educational resources. On the one hand, the digital platform should be built to effectively broaden the access channels of rural educational resources, improve the quantity and quality of rural educational resources, and improve the utilization rate of educational resources. On the other hand, cross-regional education cooperation should be strengthened based on the application of high-level digital information technology, so as to ensure the co-construction and sharing of rural educational resources and the precise matching of supply and demand, and improve the misallocation and waste of rural educational resources.
In terms of educational process mode, the government should pay attention to meet the diversified and personalized education needs of rural students, and explore the localized teaching mode in line with the situation of rural students. At the same time, digital technology is used to provide teaching methods, tools and practice fields for specific subjects and courses, constantly optimize and adjust the course form and teaching mode, and explore a new teaching mode of comprehensive and deep integration of digital technology and educational technology suitable for rural learning situation.
In terms of educational methods and means, the government should strengthen the policy support, financial support and technical support for the construction of digital countryside, help the innovation of rural education methods and means, and improve the self-development ability of rural students. The deep integration of digital technology and rural education can not only change the presentation mode of rural education content, the interaction mode between teachers and students and the teaching mode, but also effectively stimulate rural students’ interest in learning, improve the participation in learning activities, and activate the autonomy, spontaneity and consciousness of rural students’ self-promotion.
The raw data supporting the conclusion of this article will be made available by the authors, without undue reservation.
NW: Conceptualization, Methodology, Funding acquisition, Supervision.
The work presented in this paper is financed by the Hunan Academic Degree and Post graduate Education Reform Project of Department of education of Hunan Province, Project No. :2020JGYB258
The author declares that the research was conducted in the absence of any commercial or financial relationships that could be construed as a potential conflict of interest.
All claims expressed in this article are solely those of the authors and do not necessarily represent those of their affiliated organizations, or those of the publisher, the editors and the reviewers. Any product that may be evaluated in this article, or claim that may be made by its manufacturer, is not guaranteed or endorsed by the publisher.
Appiahene, P., Serwah, S., Mensah, C., and Kesse-Yaw, B. (2019). Application of wireless ad-hoc networks model to provide education to rural communities in Ghana. Int. Conf. Appl. Sci. Technol. Conf. Proc. 5 (1), 114–121.
Arumugam, S., and Bhargavi, R. (2019). A survey on driving behavior analysis in usage based insurance using big data. J. Big Data 6 (1), 86. doi:10.1186/s40537-019-0249-5
Beach, D., From, T., Johansson, M., and Öhrn, E. (2018). Educational and spatial justice in rural and urban areas in three nordic countries: A meta-ethnographic analysis. Educ. Inq. 9 (1), 4–21. doi:10.1080/20004508.2018.1430423
Carfora, M. F., Martinelli, F., Mercaldo, F., Nardone, V., Orlando, A., Santone, A., et al. (2019). A “pay-how-you-drive” car insurance approach through cluster analysis. Soft Comput. 23 (9), 2863–2875. doi:10.1007/s00500-018-3274-y
Carlsen, L. (2021). Decent work and economic growth in the European union. A partial order analysis of eurostat sdg 8 data. Green Finance 3 (4), 483–494. doi:10.3934/gf.2021022
Castillo, M. D., Anenberg, S. C., Chafe, Z. A., Huxley, R., Johnson, L. S., Kheirbek, I., et al. (2021). Quantifying the health benefits of urban climate mitigation actions: Current state of the epidemiological evidence and application in health impact assessments. Front. Sustain. Cities 3. doi:10.3389/frsc.2021.768227
Chunxiang, A., Shen, Y., and Zeng, Y. (2022). Dynamic asset-liability management problem in a continuous-time model with delay. Int. J. Control 95 (5), 1315–1336. doi:10.1080/00207179.2020.1849807
Fengwei, A., and Nan, W. (2020). Integration of urban-rural planning and human geography for online education under the impact of COVID-19. J. Intelligent Fuzzy Syst. 39 (6), 8847–8855. doi:10.3233/jifs-189282
Gorelick, J., and Walmsley, N. (2020). The greening of municipal infrastructure investments: Technical assistance, instruments, and city champions. Green Finance 2 (2), 114–134. doi:10.3934/gf.2020007
Hao, X., Wang, X., Wu, H., and Hao, Y. (2022). Path to sustainable development: Does digital economy matter in manufacturing green total factor productivity? Sustain. Dev. doi:10.1002/sd.2397
Janarthanan, R., Doss, S., and Baskar, S. (2020). Optimized unsupervised Deep learning assisted reconstructed coder in the on-nodule wearable sensor for Human Activity Recognition. Measurement.
Jia, S., Yang, C., Wang, M., and Failler, P. (2022). Heterogeneous impact of land-use on climate change: Study from a spatial perspective. Front. Environ. Sci. 510. doi:10.3389/fenvs.2022.840603
Kamau, M. M., and &Weda, C. (2019). Influence of socio-economic factors on the demand for life insurance in Kenya. J. Finance Account. 3 (4), 21–38.
Kaur, R., and Pandey, P. (2021). Air pollution, climate change, and human health in Indian cities: A brief review. Front. Sustain. Cities 3, 705131. doi:10.3389/frsc.2021.705131
Kelley, K. H., Fontanetta, L. M., Heintzman, M., and Pereira, N. (2018). Artificial intelligence: Implications for social inflation and insurance. Risk Manag. Insur. Rev. 21 (3), 373–387. doi:10.1111/rmir.12111
Kitchens, B., Dobolyi, D., Li, J., and &Abbasi, A. (2018). Advanced customer analytics: Strategic value through integration of relationship-oriented big data. J. Manag. Inf. Syst. 35 (2), 540–574. doi:10.1080/07421222.2018.1451957
Kumari, A., Tanwar, S., Tyagi, S., Kumar, N., Maasberg, M., and Choo, K. K. R. (2018). Multimedia big data computing and internet of things applications: A taxonomy and process model. J. Netw. Comput. Appl. 124, 169–195. doi:10.1016/j.jnca.2018.09.014
Li, Y., Yan, C., Liu, W., and Li, M. (2018). A principle component analysis-based random forest with the potential nearest neighbor method for automobile insurance fraud identification. Appl. Soft Comput. 70, 1000–1009. doi:10.1016/j.asoc.2017.07.027
Li, Z., Yang, C., and Huang, Z. (2022). How does the fintech sector react to signals from central bank digital currencies? Finance Res. Lett. 50. 103308, doi:10.1016/j.frl.2022.103308
Liu, Y., Zang, Y., and Yang, Y. (2020). China’s rural revitalization and development: Theory, technology and management. J. Geogr. Sci. 30 (12), 1923–1942. doi:10.1007/s11442-020-1819-3
Luo, S., Yimamu, N., Li, Y., Wu, H., Irfan, M., and Hao, Y. (2022). Digitalization and sustainable development: How could digital economy development improve green innovation in China? Bus. Strategy Environ. doi:10.1002/bse.3223
Lyubchich, V., Newlands, N. K., Ghahari, A., Mahdi, T., and Gel, Y. R. (2019). Insurance risk assessment in the face of climate change: Integrating data science and statistics. WIREs Comp. Stat. 11 (4), e1462. doi:10.1002/wics.1462
Manogaran, G., Shakeel, P. M., Fouad, H., Nam, Y., Baskar, S., Chilamkurti, N., et al. (2019). Wearable IoT smart-log patch: An edge computing-based Bayesian deep learning network system for multi access physical monitoring system. Sensors 19 (13), 3030. doi:10.3390/s19133030
Marano, P. (2019). Navigating InsurTech: The digital intermediaries of insurance products and customer protection in the EU. Maastricht J. Eur. Comp. Law 26 (2), 294–315. doi:10.1177/1023263x19830345
Mo, D., Zhang, L., Luo, R., Qu, Q., Huang, W., Wang, J., et al. (2014). Integrating computer-assisted learning into a regular curriculum: Evidence from a randomised experiment in rural schools in Shaanxi. J. Dev. Eff. 6 (3), 300–323. doi:10.1080/19439342.2014.911770
Nguyen, X. N., Thaichon, P., and Nguyen Thanh, P. V. (2019). Customer-perceived value in long-term buyer–supplier relationships: The general B2B insurance sector. Serv. Mark. Q. 40 (1), 48–65. doi:10.1080/15332969.2019.1587866
Nieto, Y., Gacía-Díaz, V., Montenegro, C., González, C. C., and Crespo, R. G. (2019b). Usage of machine learning for strategic decision making at higher educational institutions. IEEE Access 7, 75007–75017. doi:10.1109/access.2019.2919343
Nieto, Y., García-Díaz, V., Montenegro, C., and Crespo, R. G. (2019c). Supporting academic decision making at higher educational institutions using machine learning-based algorithms. Soft Comput. 23 (12), 4145–4153. doi:10.1007/s00500-018-3064-6
Nieto, Y. V., García-Díaz, V., and Montenegro, C. E. (2019a). Decision-making model at higher educational institutions based on machine learning. J. UCS 25 (10), 1301–1322.
Owadally, I., Zhou, F., Otunba, R., Lin, J., and Wright, D. (2019). An agent-based system with temporal data mining for monitoring financial stability on insurance markets. Expert Syst. Appl. 123, 270–282. doi:10.1016/j.eswa.2019.01.049
Pavlović, B. R. (2019). Challenges in application of machine learning in insurance industry. Tok. osiguranja 35 (3), 7–34. doi:10.5937/tokosig1903007p
Perry, B., Diprose, K., Taylor Buck, N., and Simon, D. (2021). Localizing the SDGs in England: Challenges and value propositions for local government. Front. Sustain. Cities 3. doi:10.3389/frsc.2021.746337
Pousttchi, K., and Gleiss, A. (2019). Surrounded by middlemen-how multi-sided platforms change the insurance industry. Electron. Mark. 29 (4), 609–629. doi:10.1007/s12525-019-00363-w
Qiu, D., and Li, D. (2021). Comments on the" SSF Report" from the perspective of economic statistics. Green Finance 3 (4), 403–463. doi:10.3934/gf.2021020
Ren, S., Hao, Y., Xu, L., Wu, H., and Ba, N. (2021). Digitalization and energy: How does internet development affect China's energy consumption? Energy Econ. 98, 105220. doi:10.1016/j.eneco.2021.105220
Ren, S., Li, L., Han, Y., Hao, Y., and Wu, H. (2022). The emerging driving force of inclusive green growth: Does digital economy agglomeration work? Bus. Strategy Environ. 31 (4), 1656–1678. doi:10.1002/bse.2975
Scheibner, J., Jobin, A., and Vayena, E. (2021). Ethical issues with using internet of things devices in citizen science research: A scoping review. Front. Environ. Sci. 9, 629649. doi:10.3389/fenvs.2021.629649
Shakeel, P. M., and Baskar, S. (2020). Automatic human emotion classification in web document using fuzzy inference system (FIS): Human emotion classification. Int. J. Technol. Hum. Interact. (IJTHI) 16 (1), 94–104. doi:10.4018/ijthi.2020010107
Shelerangkon-Nejad-Esfahani, M., Eslamian, S., and &Ostad-Ali-Askari, K. (2018). Analyzing the effect of knowledge management on customer relationship management utilizing organizational factors of banking industry as mediator, case study of shahr bank. J. Adv. Civ. Eng. Manag. 1 (3).
Song, X., Cao, M., Zhai, K., Gao, X., Wu, M., and Yang, T. (2021). The effects of spatial planning, well-being, and behavioural changes during and after the COVID-19 pandemic. Front. Sustain. Cities 3. doi:10.3389/frsc.2021.686706
Staudt, Y., and Wagner, J. (2018). What customer, policy and distribution characteristics drive the development of insurance customer relationships?–A case study analysis. Int. J. Bank Mark. 36 (6), 1098–1124. doi:10.1108/ijbm-11-2016-0175
Su, Y., Li, Z., and Yang, C. (2021). Spatial interaction spillover effects between digital financial technology and urban ecological efficiency in China: An empirical study based on spatial simultaneous equations. Int. J. Environ. Res. Public Health 18 (16), 8535. doi:10.3390/ijerph18168535
Talonen, A., Holmlund-Rytkönen, M., and &Strandvik, T. (2018). Mental models of customer ownership in the executive board: A case study in the pension insurance sector. J. Co-op. Organ. Manag. 6 (1), 1–10. doi:10.1016/j.jcom.2018.02.001
Tran, H., and Smith, D. A. (2020). What matters most for recruiting teachers to rural hard-to-staff districts: A mixed methods analysis of employment-related conditions. Am. J. Educ. 126 (3), 447–481. doi:10.1086/708252
Wang, W., Muravey, D., Shen, Y., and Zeng, Y. (2022). Optimal investment and reinsurance strategies under 4/2 stochastic volatility model. Scand. Actuar. J., 1–37. doi:10.1080/03461238.2022.2108335
Wiegard, R., Guhr, N., Krylow, S., and &Breitner, M. H. (2019). Analysis of wearable technologies’ usage for pay-as-you-live tariffs: Recommendations for insurance companies. ZVersWiss. 108 (1), 63–88. doi:10.1007/s12297-019-00431-2
Wu, D., Li, C. C., Zhou, W. T., Tsai, C. C., and Lu, C. (2019). Relationship between ICT supporting conditions and ICT application in Chinese urban and rural basic education. Asia Pac. Educ. Rev. 20 (1), 147–157. doi:10.1007/s12564-018-9568-z
Wu, H., Ba, N., Ren, S., Xu, L., Chai, J., Irfan, M., et al. (2022). The impact of internet development on the health of Chinese residents: Transmission mechanisms and empirical tests. Socio-Economic Plan. Sci. 81, 101178. doi:10.1016/j.seps.2021.101178
Wu, H., Hao, Y., and Ren, S. (2020). How do environmental regulation and environmental decentralization affect green total factor energy efficiency: Evidence from China. Energy Econ. 91, 104880. doi:10.1016/j.eneco.2020.104880
Wu, H., Xue, Y., Hao, Y., and Ren, S. (2021). How does internet development affect energy-saving and emission reduction? Evidence from China. Energy Econ. 103, 105577. doi:10.1016/j.eneco.2021.105577
Xu, M., Albitar, K., and Li, Z. (2020). Does corporate financialization affect EVA? Early evidence from China. Green Finance 2 (4), 392–408. doi:10.3934/gf.2020021
Yuan, X., Li, D., Mohapatra, D., and Elhoseny, M. (2018). Automatic removal of complex shadows from indoor videos using transfer learning and dynamic thresholding. Comput. Electr. Eng. 70, 813–825. doi:10.1016/j.compeleceng.2017.12.026
Zhang, R., Yuan, Y., Li, H., and Hu, X. (2022). Improving the framework for analyzing community resilience to understand rural revitalization pathways in China. J. Rural Stud. 94, 287–294. doi:10.1016/j.jrurstud.2022.06.012
Zhang, W., Wang, Y., Yang, L., and Wang, C. (2020). Suspending classes without stopping learning: China’s education emergency management policy in the COVID-19 outbreak. J. Risk Financ. Manag. 13 (3), 55. doi:10.3390/jrfm13030055
Zhang, X., Ming, X., Liu, Z., Zheng, M., and &Qu, Y. (2019). A new customization model for enterprises based on improved framework of customer to business: A case study in automobile industry. Adv. Mech. Eng. 11 (3), 168781401983388. doi:10.1177/1687814019833882
Zhang, Z., Qi, Q., Kumar, N., Chilamkurti, N., and Jeong, H. Y. (2015). A secure authentication scheme with anonymity for session initiation protocol using elliptic curve cryptography. Multimed. Tools Appl. 74 (10), 3477–3488. doi:10.1007/s11042-014-1885-6
Keywords: land use, big data analytics, rural education, online learning framework, China
Citation: Wei N (2022) Decreasing land use and increasing information infrastructure: Big data analytics driven integrated online learning framework in rural education. Front. Environ. Sci. 10:1025646. doi: 10.3389/fenvs.2022.1025646
Received: 23 August 2022; Accepted: 28 September 2022;
Published: 12 October 2022.
Edited by:
Xiaodong Yang, Xinjiang University, ChinaReviewed by:
Yong Ye, Chongqing Jiaotong University, ChinaCopyright © 2022 Wei. This is an open-access article distributed under the terms of the Creative Commons Attribution License (CC BY). The use, distribution or reproduction in other forums is permitted, provided the original author(s) and the copyright owner(s) are credited and that the original publication in this journal is cited, in accordance with accepted academic practice. No use, distribution or reproduction is permitted which does not comply with these terms.
*Correspondence: Na Wei, Mjc2Njc3NzYxQHFxLmNvbQ==
Disclaimer: All claims expressed in this article are solely those of the authors and do not necessarily represent those of their affiliated organizations, or those of the publisher, the editors and the reviewers. Any product that may be evaluated in this article or claim that may be made by its manufacturer is not guaranteed or endorsed by the publisher.
Research integrity at Frontiers
Learn more about the work of our research integrity team to safeguard the quality of each article we publish.