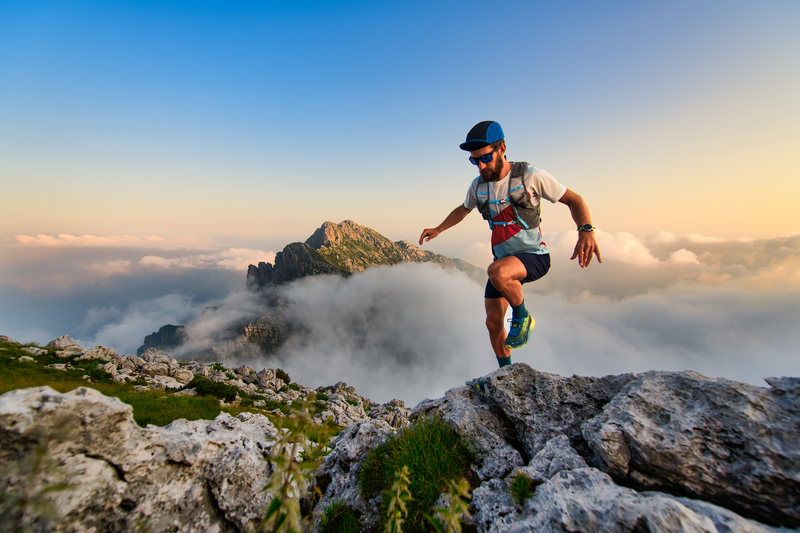
94% of researchers rate our articles as excellent or good
Learn more about the work of our research integrity team to safeguard the quality of each article we publish.
Find out more
ORIGINAL RESEARCH article
Front. Environ. Sci. , 27 September 2022
Sec. Environmental Economics and Management
Volume 10 - 2022 | https://doi.org/10.3389/fenvs.2022.1017159
A correction has been applied to this article in:
Corrigendum: Spatial correlation study on the impact of green financial development on industrial structure upgrading
Concern has been raised about how to effectively encourage industrial structure upgrading using green finance as a tool in the framework of sustainable development. This paper is based on the data from 2010 to 2019, the social network had been constructed using the gravitational model. Also, the spatial correlation of green finance development on industrial structure upgrading was studied through using the social network analysis method, as well as the detection analysis of the impact factor of green finance on industrial structure upgrading was conducted by using the geographic detector. The empirical results showed that: green finance and industrial optimization had entered a differentiated development stage in each province; green finance development would have an effect on upgrading industrial structure; and the overall network had established and maintained a benign development trend. This paper suggests that the government must improve the green finance management system as soon as possible, effectively use green finance to promote industrial optimization and introduce policies as per the characteristics of each province in order to promote the coordinated development of green finance in each province.
General Secretary Xi Jinping stated that China’s announcement to aim for carbon peaking by 2030 and carbon neutrality by 2060 is a significant strategic decision based on promoting the building of a community of human destiny and obtaining sustainable development, and the national carbon emissions trading system was officially launched on 16 July 2021. Within the context of the carbon peaking and carbon neutrality objectives, industrial upgrading and the shift in economic development from traditional resource-consuming growth to environment-friendly growth driven by technological innovation should be accelerated (Bei, Tie and Zhou, 2011b; Zhang et al., 2020; Zhao et al., 2022). Green finance could help transform traditional industrial structures into green industries, promote the transformation and upgrading of economic, energy, and industrial structures, find new economic growth areas, as well as acquire a green and low-carbon development path (Lam and Hills, 2011; Zhang and Wang, 2019a; Zhang et al., 2021; Zhou, 2022).
Through the role of capital finance, the traditional financial industry may encourage the flow of social capital to developing industries while driving out less developed ones from the market and promoting the modernization of industrial structures (Rin and Hellmann, 2002; Li and Xue, 2009; Binh et al., 2005). On the other hand, green projects have long investment cycles and poor return rates, which makes it difficult to completely resolve their financing issues by relying solely on traditional financial institutions (Andersen, 2017; He and Liu, 2018). Green finance could grasp the flow of financial capital based on the development of green financial instruments for ecological and environmental management, as well as give green enterprises preferential policies, so in order to help environmental protection industry financing, forcing high pollution, high energy consumption industries out of the market, and then acquire industrial structure upgrading, whilst green finance development may help with industrial structure optimization (Simon et al., 2012; Wang and Wang, 2021). Compared to traditional finance’s, green financing emphasizes environmental interests, so is more closely linked to the environment and has more complex geographical effects on the modernization of industrial structure (Zhou et al., 2020). Analysing spatial spill over effects and spatial correlation characteristics among regions could greatly serve each province in developing green finance as well as industrial optimization policies, which are tailored to local conditions, promoting the development of regional green finance, enhancing the efficiency of using green financial resources, and boosting the most efficient resource allocation (Wang et al., 2021; (Zhou et al., 2022). China also faces three problems that need urgent solution: Firstly, how to incorporate spatial factors into relevant studies so as to improve the efficiency of green finance in promoting industrial structure upgrading. Secondly, how to increase the role of green finance in upgrading industrial structure. Accordingly, scholars at home and abroad have focused on the impact of green finance on the upgrading of industrial structures.
Within this context, this paper is based on the data from 2009-to 2018, the social network had been constructed using the gravitational model. Also, the spatial correlation of green finance development on industrial structure upgrading was studied through using the social network analysis method, as well as the detection analysis of the impact factor of green finance on industrial structure upgrading was conducted by using the geographic detector. This paper offers three main contributions to the existing literatures. First of all, combining the current economic background and the current situation of green finance development, this paper incorporates carbon finance into the evaluation system of green finance development level, which makes the data more comprehensive and accurate. Secondly, this paper adopts a social network analysis method from the spatial characteristics of the impact of green finance on industrial structure upgrading in order to depict its overall network characteristics, individual network characteristics, and evolutionary trends, which is a useful supplement to existing studies. The findings provide new research perspectives for other researchers and help to improve the efficiency of green finance in promoting industrial structure upgrading and have practical significance for the current development in China.
As the economy and society evolve, so will the industrial structure. As the economy and society develop, upgrading is the process of shifting the focus of the country’s economy from primary to secondary and eventually tertiary industries (Kuznets, 1949; Allen and Clark, 1951). Finance performs activities such as resource allocation, risk assessment, and information transmission, all of which could enhance the efficiency of production components flowing between industries (Chen et al., 2013; Yuan and Zhou, 2020), and the impact of finance on industrial structure upgrading has gotten a great quantity of attention from academics. Patrick suggested a “demand-induced” and “supply-led” relationship between economic growth and financial development, arguing that in the less developed stage of the economy, there is a need for a supply-led approach to stimulate financial development and drive industrial progress, while industrial progress would in turn stimulate financial development (Patrick, 1966d). Meanwhile, financial development may assist in improving the industrial structure by increasing productivity and bolstering the financial environment (Aghion et al., 2005; Chen, 2011; Wang and Johansson, 2013; Pradhan et al., 2015; Sasidharan et al., 2015). On the other hand, the impact of financial development on the upgrading of industrial structure is complicated. The “financial suppression” policy, which suppresses the service sector while protecting and promoting the industrial sector’s development, leads to a low service sector share, stymies structural reform, and causes an imbalance in the domestic industrial structure (Wu and Zhang, 2016; Xu and Tan, 2020). Meanwhile, the differences in the impact of finance on the upgrading of industrial structures are not only reflected between industries, but also between regions with different levels of economic development (FQ Jin and Zhong, 2013; Wang et al., 2019).
There is currently no authoritative definition of green finance in academic circles, and there are various terms such as financial innovation, financial instruments, and the intersection of green economy and finance, but its core essence is to strive to preserve the environment by bridging the gap between finance and environmental industries, and to benefit the environment in the broader context of environmental sustainability (Gregoriou, 2015a; Wang et al., 2019). Compared with traditional finance, green finance does not pursue profits excessively, but conducts financial operations based on the principles of environmental protection and sustainable development to achieve harmonious development of resources, environment, economy and society (Ma, 2015). Green finance could hence improve the financing constraints of green sectors, while at the same time restricting “Two-high and one over-capacity” industries and energy-intensive industries (Bing et al., 2011; Liu et al., 2017). Green finance incorporates environmental protection into business operations by using green financial products to guide the development of alternative energy sources, control the promotion of environmentally polluting projects, as well as promote mergers and acquisitions and restructuring of polluting industries, which result into the dual optimization of industries and technologies as well as the long-term development of the economy (Salazar, 1998; Labatt and White, 2002; Gu, Chen and Zhang, 2021). Nevertheless, although being very effective overall in promoting industrial transformation and upgrading in China, the green financial system’s discriminatory growth, information asymmetry, and lack of enterprise supervision are all contributing to its decline (Chen and Dang, 2011; Wang and Wang, 2021). Some academics have undertaken further in-depth studies on the influence of green financing development on industrial structure upgrading based on this foundation. In each Chinese province, the effects of green credit’s effects on industrial structure upgrading as well as regional and industrial variations are examined. The influence of green credit on China’s industrial structure varies extremely by location, according to the study, as well as the “push-back” mechanism incorporated in green financial policies supports structural upgrading of secondary sectors thus preventing tertiary business development (Taghizadeh-Hesary and Yoshino, 2019; Hu, Jiang and Zhong, 2020; Li et al., 2020; Qi and Qi, 2020). Some scholars have taken into account spatial factors in their relevant studies, which have found that green finance can promote regional industrial rationality while having clearly negative spatial spill over effects on adjoining regions’ industrial rationality processes (Li and Gan, 2020; Cheng et al., 2021; Wang et al., 2021; Gao et al., 2022).
They are various issues with the existing research on how green finance affects modernizing industrial structures, including: firstly, current research focuses on differences in the contribution of green finance to industrial structure upgrading between regions, instead of its spatial correlation characteristics, also, the revealed provincial spatial spill over effects are mostly restricted to neighbouring regions, failing to depict the national geographical structure and the spatial correlation characteristics of Chinese provinces as well as the spatial structure on a national scale. Second, results of current studies are typically dependent on the economic structure of the economy. Second, existing study results are commonly explained as major elements based on current economic development and geopolitical considerations, instead of conducting a more in-depth examination of each province’s distinctive variations. This paper addresses the aforementioned flaws by Carrying out the following innovations: First of all, this paper addresses the traditional spatial econometric approach of limiting spatial linkages to adjacent and nearby areas, and takes the perspective of a region-wide study in order to depict the overall characteristics and evolutionary trends of spatially linked networks; illustrate each province’s location in the larger network and the variations in its spatial effect. Secondly, while the existing literature focuses on the ‘quantitative’ effects of green finance impacts based on the consideration of spatial factors, this study reveals the internal structure of the network, its transmission mechanism, and its evolutionary process. Thirdly, considering the interaction between the influencing factors, the detection analysis of the impact factor of green finance on industrial structure upgrading is conducted by using the geographic detector.
Accurately measuring the spatial network relationships between regions is critical to the construction of social networks. The gravity model can take into account the economic and geographical distance factors, and thus can describe the dynamic evolution of the spatial association network with panel data. Therefore, this paper is based on the gravity model to construct a social association network for the influence of green finance on industrial structure upgrading. Nevertheless, the gravity model must be amended by assigning weights according to the level of green financial development in order to account for the directionality of the inter-provincial spatial connection as well as the different contributions of the two provinces to the spatial connection (Liu et al., 2015).
The following is the modified gravity model.
Rij is the strength of green finance to economic support linkage between province i and province j.
Kij is the gravitational parameter, indicating the degree of contribution of province i to Rij.
GCi is the green finance level of the ith province.
Pi represents the total resident population of the ith province. The total population influences the local ecological environment as well as industrial structure, so this paper chooses to use the total population as one of the indicators.
ICi denotes the level of industrial structure upgrading in province i.
Dij denotes the distance between province i and province j. In this paper, Dij is denoted by the distance between any two provincial capitals.
Based on the gravity model, a gravity matrix was built in this study. The mean value of the elements in the matrix’s rows served as the threshold, and if an element was greater than the threshold, it was given a value of 1, and if it was lower than the threshold, a value of 0. The elements of the gravity matrix were binarized, and then the social network of the influence of green financial development on industrial structure upgrading was built (Zhao et al., 2015).
The entropy technique to assess the level of industrial structure upgrading from the viewpoint of advanced industrial structure and industrial structure rationalisation, by using a formula based on Xu Deyun (2008) to assess advanced industrial structure (Xu, 2008).
The industrial structure is more advanced the higher the value of N, where N is the industrial structure upgrading index. The percentages of primary, secondary, and tertiary industries’ value added to the GDP are indicated by the letters y1, y2, and y3 respectively.
Drawing on Gan, Chunhui et al. (2011) utilised the Thiel index to measure the rationalization of industrial structure, the formula is as follows (Xu, 2008).
Where TL is the Thiel index, wi is the ratio of output value to GDP for industry i, and ei is the ratio of employees to total employment for industry i.
This study used the entropy method to assess the development of green finance from five dimensions: green credit, green securities, green insurance, green investment, and carbon finance. Since data on green finance at the provincial level are not yet available to the general public, this study was necessary. Table 1 shows the system of indicators for the level of development of green finance in China.
Through network density, theoretical relationship number, and actual relationship number, the network scale analysis revealed the geographical association sparsity and its evolution trend inside the network. In order to describe the overall network accessibility, rank, stability, and connection efficiency, network correlation analysis includes correlation degree, rank degree, efficiency, as well as average path.
The three ways used in this study to analyse individual network properties were centrality analysis, small-world analysis, and structural hole analysis. The Out-degree centrality and In-degree centrality had been used in the centrality analysis to characterize the impact size of each province on the overall network as well as the overall network’s influence size. In the small-world study, the clustering coefficient and the mean value of route length were used to describe the efficiency of each province’s spatial link. Effective Size, efficiency, limit system, and rank degree were used in structural hole analysis in order to define the dimension of each province’s advantage in competing for resources in the overall network.
The internal social hierarchy and the transmission mechanism of the spatial spillover effect between the core and periphery areas were divided in this study using the core-periphery analysis, and based on this, the K-core analysis has been used for dividing the internal factions of the network as per the cohesion size within the subgroups (Liu, 2009).
This paper selected green financial development, financial development, economic development, technological progress, and energy consumption indicators of each province and utilised a Geodetector to study its influence mechanism on industrial structure upgrading, since the influence mechanism of green financial development on industrial structure upgrading was complex. The unique advantage of the Geodetector was that it could detect two-factor interactions with dependent variables and avoid the issue of multicollinearity (Wang and Xu, 2012).
This included financial development assessed using the total market value of A-shares and year-end deposit balances of financial institutions in each province, economic development assessed using the provincial GDP, technological development assessed using the number of patents at the end of the year, and energy consumption assessed using by electricity consumption.
The research project for this paper was 31 provinces and regions in China (excluding Hong Kong, Macao, and Taiwan) and the study period was 2010–2019. Green credit data were taken from the China Industrial Statistical Yearbook, green securities data, green insurance data, year-end patent number and electricity consumption were taken from wind database, green investment data were taken from China Statistical Yearbook On Environment, carbon financial data from the official website of China Clean Development Mechanism Fund, industrial output value, GDP, year-end deposit balance of financial institutions from the China Statistical Yearbook, the total employment and the number of employees by industry as taken from the provincial statistics yearbooks.
In this study, Ucinet software (version 6.186) had been used for carving the overall characteristics of the social network, as illustrated in Table 2, Arcgis software was use to visualize it, see Figure 1. The rise of the spatial association of green finance on the industrial structure upgrading in the years 2010, 2013, 2016, and 2019 is examined in this article due to space constraints. Figure 1 shows the spatial links between the above 4 years of the impact of green finance on industrial structure upgrading across Chinese provinces. Table 2 shows the overall network characteristics of the impact of green finance on industrial structure upgrading in China.
TABLE 2. Overall network characteristics of the impact of green finance development on industrial structure upgrading.
FIGURE 1. Network evolution of the impact of green finance development on industrial structure upgrading.
According to Figure 1, the spatial association of green finance development on industrial structure upgrading in each Chinese province forms a social network, with direct and indirect linkage channels coexisting between provinces, and no province is isolated; nevertheless, the overall network is unstable, and the spatial linkage between provinces tends to be closer. The green finance provinces that are growing more quickly in the east and south make up the core circle of the network, while those that are growing more slowly in the west and border make up the peripheral circle. Provinces in the core circle are not only closely connected internally, but their spatial spillover effects could radiate to the peripheral circle provinces, while provinces in the peripheral circle are not only closely linked internally, but their spatial spillover effects could radiate to the peripheral circle provinces. The local proximity is the only area where there is a spatial spillover effect. As illustrated in Table 2, in regards to the number of actual relationships, the overall network density of the impact of green financial development on industrial structure upgrading between 2010 and 2019 illustrate an upward trend from 2010-to 2013, with an inverted U-shaped change in 2013–2016, as well as a rapid increase in 2016–2019, which maintains a rapid upward trend. The Green Credit Guidelines and other papers were published between 2010 and 2013, which hastened the development of green finance, and had a greater influence on modernizing China’s industrial structure. The green finance policy framework was largely built between 2010 and 2013, with the publishing of the Green Credit Guidelines and other papers, and the development of green finance accelerated, following an enhanced influence on China’s industrial structure. The development of green finance saw a setback from 2013 to 2016, and between 2016 and 2019, the idea of green and recycling development was advanced, the scale of green financial product issuance rose dramatically, and market players got more diverse. Nevertheless, the number of theoretical linkages was 930, while the actual number of relationships was 245, and network density was only 0.263, which indicates that the spatial association between provinces is still insufficient. As seen in Figure 1, when several contact channels interact to produce the overall network, and no province is isolated, the Connectedness value has always been 1, indicating that the network is extremely well linked. The overall network efficiency is diminishing, and the increasing number of redundant linking channels in the overall network intensifies the interaction impact across provinces, which led to an increased network stability. The Graph Efficiency is always zero, which indicates that the overall network has no hierarchical spatial structure and that spatial spillover effects between provinces are almost unconstrained by administrative barriers. The average path length of the network is fluctuating downward, its geographical links are getting shorter, and its inter-provincial green finance development cooperation efficiency in advancing the industrial structure is continually increasing.
Table 3 demonstrates the individual network characteristics of the influence of green finance development on industrial structure upgrading in each province with 2019 data. Figure 2 shows the centrality of the provinces and their spatial linkages in the cluster of nodes of the social network on the impact of green finance on industrial structure upgrading in China.
TABLE 3. Individual network characteristics of the impact of green financial development on industrial structure upgrading.
FIGURE 2. Network centrality graph of the impact of green financial development on industrial structure upgrading in 2019.
As illustrated in Figure 2, in 2019, the spatial association of the impact of green financial development on industrial structure upgrading in each Chinese province has built a social network with “loose inside and tight outside,” with provinces including Zhejiang and Beijing at the core level of the overall network, and with their green financial development having a significant influence on the overall network, specifically Zhejiang as the social network’s hub. Provinces at the network’s periphery, such as Jilin and Heilongjiang, primarily receive spatial influence from the core provinces, and their external spatial connection channels only exist in neighbouring provinces, resulting in a smaller spatial influence on the overall network. In contrast, Zhejiang, the social network’s centre, has a spatial spillover effect that spreads throughout the entire nation.
Zhejiang (26.000), Beijing (17.000) and Xinjiang (12.000), and Xinjiang are the three provinces with the highest out-degree centrality rankings, as indicated in Table 3. In recent years, Beijing has ranked first in the number and issuance scale of green credit and green bonds, and the pilot of green insurance has been continuously promoted. Moreover, Xinjiang and Zhejiang turned into the first pilot zones of green financial innovation and reform in 2017, as well as the sustainable and eco - friendly financial policy environment has promoted the growth of the scale. Among all provinces, Beijing (12.000), Henan (11.000), and Anhui (11.000) have the highest In-degree centrality scores (11.000). These three provinces may more easily “take advantage” of the external spatial spillover effect because they have more economic development potential, are densely populated, and are close to financially successful provinces. The variance of Out-degree centrality and In-degree centrality are 19.378 and 8.578 respectively, and the Out-degree centrality and In-degree centrality of neighbouring provinces have a closer proximity, which is a sign that the influence of green finance on industrial structure in China has altered from individual development to regional synergistic development. Also, the variance value is larger and there is an obvious difference between them, which indicates that the level of development of their own green finance and the difference of industrial structure not only determine the size of external radiation effect, but also affect their “The development advantages of each province significantly impact the former, and the development of green finance in China tends to be more “common wealth” than the “Matthew Effect".
When viewed holistically, the mean characteristic path length is 2.183, which indicates that more nodes must be connected to the network, there are more geographical limitation, network accessibility is poor, and operation efficiency is low. Zhejiang has the shortest characteristic path length (0.968), which is a sign that it is spatially connected to most of the provinces and serves as an “intermediary bridge” in order to promote cross-regional cooperation as well as enhance the efficiency of the impact of green financial development on industrial structure upgrading. 3.355, indicating that Jilin has fewer direct “channels” with other provinces and more nodes through which it must connect to the overall network. Following an overall perspective, the clustering coefficient’s mean value is 0.546, which indicates that the network’s nodes are connected both internally and externally and that the clustering coefficients of the network’s core provinces are generally low and their network connections are decentralized. In contrast, the clustering coefficients of the network’s peripheral provinces are significant. Also, their network connections are characterized by local convergence. Zhejiang (0.230), whose connections with other network nodes have not been restricted to neighbouring provinces, whose spatially connected connections are characterized by global and decentralized nature, and whose spatial spillover effect radiates the entire network, has the smallest clustering coefficient, while Jilin (0.900), whose connections with other networks are limited to neighbouring provinces, and whose spatially connected connections are characterized by global and decentralized nature, has the largest clustering coefficient.
Zhejiang has the highest Effective Size (23.611) and Efficiency (0.787), which are significantly higher than other provinces, and the lowest Constraint (0.130), which indicates that Zhejiang retains the highest degree of individual network openness, has opened most of the “channels” to connect with other provinces, has the advantage of structural holes, and has achieved the status of the most competitive network for green finance to influence industrial structure upgrading. Whereas, network periphery provinces, such as Jilin, have the lowest Effective Size of 1.333, while the Constraint is as high as 0.780. This shows that the network of the province of Jilin is almost completely closed and only associated with individual provinces, and the connection between Jilin and the overall network is almost entirely reliant on other nodes. This is an indication that Jilin is unable to effectively take part in the overall network of green finance impact on industrial structure upgrading. Therefore, spatial connectivity is severely limited, and “communication” efficiency with other nodes is extremely low, reducing the effects of its green financial development on industrial structure upgrading. The developed provinces with a Structural Hole advantage have increased “communication” efficiency, more powerful competitiveness, and faster development. In the meantime, the provinces with a structural hole disadvantage have a weaker foundation for green financial development, and their closeness will further decrease the impact on industrial structure upgrading. This would result in differences in the level of green financial development and industrial structure between provinces and regions in a given year would keep rising. Table 3 Individual network characteristics of the impact of green financial development on industrial structure upgrading.
Due to the length of the study, this paper uses 3 years of data, 2010, 2015 and 2019, for core-periphery analysis. Figure 3 shows the results of the core-periphery analysis. Table 4 shows the core and peripheral regions.
FIGURE 3. Core-periphery analysis of the network of the influence of green financial development on industrial structure upgrading.
As illustrated in Figure 3, the provinces in the network’s core area are mostly economically developed, which includes Beijing, or provinces with strong green financial development vitality, such as Guangdong, Zhejiang, and Xinjiang China Green Financial Reform as well as Innovation Pilot Zone. Despite having fewer provinces than the periphery region, the core area has a significantly greater density of network connections and a lot stronger spatial link for green financial growth. While provinces on the periphery are often economically backward, with slower green financial development as well as sparse spatial connections within the periphery, the network link density among the core and the periphery is high. Core area to periphery area network linkage density is higher than periphery area to core area network linkage density, and there is a clear driving impact of green finance from core area provinces to periphery area provinces on industrial structure upgrading.
The number of provinces in the core area decline, but their regional network connection density increases; the spatial influence of developed green finance provinces on industrial structure upgrading keeps rising quicker than that of lagging provinces; the network connection density of core area to peripheral area increases; the spatial spillover effect of core area provinces on peripheral area provinces increases; as well as the developed green finance provinces’ spatial influence on industrial structure upgrading keeps growing faster than that of lagging provinces; and the developed green finance provinces’ Green finance development in China is currently transitioning from individual development to regional synergistic development.
Ucinet software performs a K-core analysis of the influence network of green financial development on industrial structure upgrading using 2019 data in this study, shown in Table 5. As illustrated in Table 5, Green financial development’s impact on industrial structure upgrading could be classified into four subdivisions, with core degrees of 7, 6, 5, and 4. The following features describe the structure of the green financing development influence network on upgrading industrial structure: first and foremost, Higher-degree k-core subgroups are subgroups of lesser k-core subgroups, as well as the overall k-core network structure is pyramidal, which indicates that the overall network’s spatial connectivity is stratified and hierarchical. Secondly, the geo-location and level of green finance development have been associated to the segmentation of provinces into k-core subgroups. To some degree, the size of spatial spillover between provinces is determined by the influence of green finance on industrial structure upgrading and geographical location; for instance, Beijing and other developed green finance provinces are mostly situated in the 7-core area with their neighboring provinces and hold most of the “control” over the overall network. Third, the core degree of the nuclear partition tends to decline evenly. The degree of each nuclear zone is less different from the number of distributed provinces, and the core degree of the sub-region decreases as some of the provinces’ spatial connections are reduced. This suggests that the impact of this green financial development on the upgrading of industrial structure has entered the stage of regional synergistic development, and the development gap between regions is gradually narrowing.
TABLE 5. K-core analysis of the network of green financial development on the impact of industrial structure upgrading.
In order to further detect the impact of green finance on industrial structure upgrading, this research paper utilised the relevant data from 2010–2019 and conducts impact factor analysis using Geodetector to obtain Table 6 and interaction detection analysis between impact factors for obtaining Figure 4.
As illustrated in Table 6, financial development, economic growth, technological progress, energy consumption, as well as green finance all passed the t-test with a statistical significance of 0.01, and all of these influence factors positively helped in the upgrading of industrial structure, and their explanatory degrees were, in descending order, financial development (0.402), green finance (0.218), technological progress (0.217), and economic growth (0.113). The reason is that this t-test has a statistical significance of 0.01, financial development, economic growth, technological progress, energy consumption as well as green finance all passed the t-test with statistical significance of 0.01, and each of their influence factors positively promoted the upgrading of industrial structure, along with their explanatory degrees were, in descending order, financial development (0.402), green finance (0.218), technological progress (0.217), economic growth (0.113), and this is due to the expansion of the financial industry brought by financial development could promote the growth of the output value of the tertiary industry as well as directly contribute to the upgrading of the industrial structure. The impact of green finance on improving industrial structure, is however, much less than the impact on financial development, at 0.218. This shows that China’s green finance is still unable to deploy sufficient funds for green enterprises, and its focus is still on environmental pollution control, as well as the energy consumption is positively promoting industrial structure upgrading. 0.077, shows that China’s industrial structure has tended to be rationalized and advanced.
As illustrated from Figure 4, the influence of each element on industrial structure upgrading does not exist independently but it has been cultivated by the joint action of multiple elements, as well as the interaction between the elements is much greater as compared to the role of a single factor. However, when it interacts with financial development, economic growth, technological progress, and green finance, the degree of its influence increases significantly. With the rapid development of green finance, more capital is made to flow into the green industry and economic growth, while also promoting the development of high technology, changing China’s energy consumption structure, as well as realizing advanced industrial structure, rationalization, and ecologization.
We use social network analysis to depict the overall characteristics and evolutionary trends of spatially linked networks based on panel data from 31 Chinese provinces from 2010 to 2019. We also describe the position of each province in the overall network as well as its spatial impact differences; reveal the internal structure of the network and its transmission mechanism and its evolutionary process, and use geographic probes for detecting the spatial heterogeneity of the impact of green financial development on industrial structure upgrading in one step. Geographic probes can be used to highlight the regional heterogeneity of the effect of green financial development on upgrading industrial structures. The main results obtained are as follows. First, the overall network has formed and maintained a benign development trend. The network density value of the influence of green finance on industrial structure upgrading is low from 2010 to 2019, but it is in a rapid upward trend after 2015, as well as the spatial connection of the influence of green finance on industrial structure upgrading between provinces is closer. The Graph Efficiency degree is always 0, and there is no transmission barrier for the spatial spillover effect of the influence of green finance on industrial structure upgrading between provinces. The network has excellent connection generally, with no province being isolated, and the network correlation degree is always 1. Additionally, green financing and industrial optimization reach a stage of growth that is unique to each province. The future development trend of each province is also quite different due to the differences in their green financial development, industrial structure and economic foundation. Provinces with developed economies and high levels of green financial development are typically in the core area and high kernel degree group and their networks have a higher point-out and In-degree centrality’s, which carries a more positive influence on the overall network, lower clustering coefficient, shorter average path, easy to produce spatial association with other provinces, and have the advantage of structural hole and “control” over the overall network, while the network The marginal provinces’ delayed green financial development and lack of direct connections to other provinces prevent them from having a competitive advantage in the overall network, which in turn hinders the modernization of industrial structure. Finally, green finance and other factors all play a positive role in promoting industrial structure upgrading. Overall, the degree of each element’s influence on industrial structure upgrading is low, and green finance and other factors cannot efficiently promote industrial structure optimization yet, and the influence of each factor does not exist independently, but has been formed by the joint action of many elements, and this interaction is much stronger than that of a single element.
We also suggest associated countermeasures in light of the data presented above. First, strengthen the system of green financial management. The government should create the green financial assessment index system as soon as possible to reduce the risk of the green financial market and increase the effectiveness of green financial project approval. Reduce the green financial market’s entry barrier, which allows more financial institutions to join, enhancing the status quo of the green financial market with banks as the single primary body, and expanding green industry financing channels. In order to promote the development of green industries as well as guide the optimization of industrial structure, introduce incentive policies for green industries, such as lowering tax burdens and providing appropriate subsidies to reduce the financing cost of green enterprises and resolve the issue of maturity mismatch.
Moreover, use green finance for supporting industrial optimization as well. Green finance could be utilised to optimise capital allocation, send green investment signals to the market, guide capital flow to new green enterprises, strictly control new high pollution and high energy consumption projects, establish new energy industries quickly, reduce coal consumption, extensively and deeply implement carbon peak action, expand the development and promotion of new green technologies, and increase enterprise environmental awareness. Improve the industrial structure, shift China’s economic growth’s pillar from industry to services, and increase the GDP proportion of the service sector. The government can use green finance to help China establish a circular economy and navigate the economic transition era more effectively.
At last, encourage the development of green finance in every province in a coordinated manner. Provinces should construct a green entry cooperation mechanism, because the influence of green finance development on industrial structure upgrading has a spatial spillover effect and a positive externality across provinces. Nevertheless, the impact of green finance on industrial structure upgrading varies depending on provincial location factors, economic foundation, green finance development level, as well as industrial structure, and the role played by each province in the overall network. Therefore, the government must implement tailored policies for each province, putting local conditions first, launching green finance projects with local features, and encouraging diversification of investment subjects in the green finance market. Green financial preferential policies can be appropriately skewed to provinces with imperfect green financial development and a backward economy, luring more green industries to “settle” in these provinces, and promoting high-quality economic development, preventing the phenomenon of delaying green financial development in some regions due to local short-term interests, and offering a long-term effect for the transformation and upgrading of industrial structure efficiency. It will also provide a long-term guarantee mechanism for the ecological transformation and upgrading of industrial structures, preventing some areas from limiting the expansion of green financing owing to local short-term interests.
At present, relevant studies mainly focus on differences in the contribution of green finance to industrial structure upgrading between regions, and few scholars have measured its spatial correlation characteristics. Therefore, this paper can be a reference for other scholars to carry out similar studies. In addition, the findings of this study provide empirical evidence on how to synergistically optimise inter-regional green finance development and its contribution to the upgrading of industrial structures. However, there are still some limitations in this study: the data has not been fully disclosed and some of the green financial products started late and are not included in the evaluation system of the level of green financial development, thus making the measurement results subject to some errors. Therefore, future measurements of the level of green financial development still need to be combined with the current condition for improvement, and relevant research will be performed on emerging green financial products.
The original contributions presented in the study are included in the article/Supplementary Material, further inquiries can be directed to the corresponding author.
WN and XD; methodology, WN; software,WN; validation, WN; formal analysis, WN; investigation, WN; resources, XD; data curation, WN; writing—original draft preparation, WN; writing—review and editing, XD; visualization, WN; supervision, XD; project administration, XD; funding acquisition, XD.
This research was funded by Zhejiang Shuren University introduced talents to start the research project, grant number 2021R024.
We thank the reviewers for their valuable comments and suggestions.
The authors declare that the research was conducted in the absence of any commercial or financial relationships that could be construed as a potential conflict of interest.
All claims expressed in this article are solely those of the authors and do not necessarily represent those of their affiliated organizations, or those of the publisher, the editors and the reviewers. Any product that may be evaluated in this article, or claim that may be made by its manufacturer, is not guaranteed or endorsed by the publisher.
The Supplementary Material for this article can be found online at: https://www.frontiersin.org/articles/10.3389/fenvs.2022.1017159/full#supplementary-material
Aghion, P., Howitt, P., and Mayer-Foulkes, D. (2005). The effect of financial development on convergence: Theory and evidence. Q. J. Econ. 120 (1), 173–222. doi:10.1162/0033553053327515
Allen, R. G. D., and Clark, C. (1951). The conditions of economic progress. Economica 18 (72), 432. doi:10.2307/2549614
Andersen, D. C. (2017). Do credit constraints favor dirty production? Theory and plant-level evidence. J. Environ. Econ. Manag. 84, 189–208. doi:10.1016/j.jeem.2017.04.002
Bei, J., Tie, L., and Zhou, D. (2011b). Transformation and upgrading of China’s industrial structure:process, issues and trends. China Ind. Econ. 2 (11). CNKI:SUN:GGYY.0.2011-02-002.
Bing, Z., Yang, Y., and Bi, J. (2011). Tracking the implementation of green credit policy in China: Top-down perspective and bottom-up reform. J. Environ. Manag. 92 (4), 1321–1327. doi:10.1016/j.jenvman.2010.12.019
Binh, K. B., Park, S. Y., and Shin, B. S. (2005). Financial structure and industrial growth: A direct evidence from oecd countries.
Chen, S. (2011). An empirical study of relationship between China’s industrial structure upgrading and finance development. China Soft Sci.
Chen, W., and Dang, H. (2011). An analysis of the functional mechanism and effect of green credit on industrial upgrading. J. Jiangxi Univ. Finance Econ.
Chen, Y., Cheng, L., and Economics, S. (2013). Research on the correlation between the finance development and the industrial structure upgrading in anhui province. J. Anhui Univ. Technol. Sci. AHUT.
Cheng, Q., Lai, X., Liu, Y., Yang, Z., and Liu, J. (2021). The influence of green credit on China’s industrial structure upgrade: Evidence from industrial sector panel data exploration. Environ. Sci. Pollut. Res. 29 (15), 22439–22453. doi:10.1007/s11356-021-17399-1
Gao, L., Tian, Q., and Meng, F. (2022). The impact of green finance on industrial reasonability in China: Empirical research based on the spatial panel durbin model. Environ. Sci. Pollut. Res. Int. doi:10.1007/s11356-022-18732-y
Gu, B., Chen, F., and Zhang, K. (2021). The policy effect of green finance in promoting industrial transformation and upgrading efficiency in China: Analysis from the perspective of government regulation and public environmental demands. Environ. Sci. Pollut. Res. 28 (34), 47474–47491. doi:10.1007/s11356-021-13944-0
He, L.-Y., and Liu, L. (2018). Emerging markets finance and trade stand by or follow? responsibility diffusion effects and green credit stand by or follow? responsibility diffusion effects and green credit. Emerg. Mark. Finance Trade 54, 1740–1760. doi:10.1080/1540496x.2018.1430566
Hu, Y., Jiang, H., and Zhong, Z. (2020). Impact of green credit on industrial structure in China: Theoretical mechanism and empirical analysis. Environ. Sci. Pollut. Res. 27 (10), 10506–10519. doi:10.1007/s11356-020-07717-4
Jin, F., and Zhong, L. (2013). An empirical study on the effect of China’s stock market financing on the regional industrial structure upgrading——based on a dynamic panel. Account. Finance (06), 24–27. doi:10.3969/j.issn.1674-3059.2013.06.005
Kuznets, S. (1949). National income and industrial structure. Econometrica 17, 205. doi:10.2307/1907310
Labatt, S., and White, R. R. (2002). Environmental finance : A guide to environmental risk assessment and financial products. Hoboken, N.J.: J. Wiley.
Lam, J. C. K., and Hills, P. (2011). Promoting technological environmental innovations. Int. J. Appl. Logist. 2 (2), 17–34. doi:10.4018/ijal.2011040102
Li, C., and Gan, Y. (2020). The spatial spillover effects of green finance on ecological environment Empirical research based on spatial econometric model. Environ. Sci. Pollut. Res. 28 (5), 5651–5665. doi:10.1007/s11356-020-10961-3
Li, P., and Xue, W. (2009). Research on upgrading of the industrial structure based on financial convergence. J. Guangdong Univ. Finance.
Li, Y., Hu, H., and Li, H. (2020). An empirical analysis of the impact of green credit on industrial structure upgrading in China-based on provincial panel data in China. Econ. Issues.
Liu, H., Liu, C., and Sun, Y. (2015). “Research on the structural characteristics of spatially linked networks of energy consumption in China and their effects,” in China industrial economy. doi:10.19581/j.cnki.ciejournal.2015.05.008
Liu, J-Y., Xia, Y., Fan, Y., Lin, S. M., and Wu, J. (2017). Assessment of a green credit policy aimed at energy-intensive industries in China based on a financial CGE model. J. Clean. Prod. 163, 293–302. doi:10.1016/j.jclepro.2015.10.111
Liu, J. (2009). Lecture notes on holistic network analysis - a practical guide to ucinet software. Shanghai: Shanghai People’s Publishing House.
Ma, J. (2015). On building China’s green financial system [J]. Financ. forum (5), 18–27. doi:10.16529/j.cnki.11-4613/f.2015.05.002
Patrick, H. T. (1966d). Financial development and economic growth in underdeveloped countries. Econ. Dev. Cult. Change 14 (2), 174–189. doi:10.1086/450153
Pradhan, R. P., Arvin, M. B., and Norman, N. R. (2015). The dynamics of information and communications technologies infrastructure, economic growth, and financial development: Evidence from Asian countries. Technol. Soc. 42, 135–149. doi:10.1016/j.techsoc.2015.04.002
Qi, R., and Qi, L. (2020). Can synergy effect exist between green finance and industrial structure upgrade in China? Open J. Soc. Sci. 08 (08), 215–226. doi:10.4236/jss.2020.88019
Rin, M., and Hellmann, T. (2002). Banks as catalysts for industrialization. J. Financial Intermediation 11 (4), 366–397. doi:10.1006/jfin.2002.0346
Sasidharan, S., Lukose, P., and Komera, S. (2015). Financing constraints and investments in r&d: Evidence from indian manufacturing firms. Q. Rev. Econ. Finance 55 (2), 28–39. doi:10.1016/j.qref.2014.07.002
Simon, G. L., Adam, G. B., and Mann, P. (2012). Win-win scenarios at the climate–development interface: Challenges and opportunities for stove replacement programs through carbon finance. Glob. Environ. Change 22, 275–287. doi:10.1016/j.gloenvcha.2011.08.007
Taghizadeh-Hesary, F., and Yoshino, N. (2019). The way to induce private participation in green finance and investment. Finance Res. Lett. 31, 98–103. doi:10.1016/j.frl.2019.04.016
Wang, C., Zhang, X., Vilela, A. L. M., Liu, C., and Stanley, H. E. (2019). Industrial structure upgrading and the impact of the capital market from 1998 to 2015: A spatial econometric analysis in Chinese regions. Phys. A Stat. Mech. its Appl. 513, 189–201. doi:10.1016/j.physa.2018.08.168
Wang, F., Wang, R., and He, Z. (2021). The impact of environmental pollution and green finance on the high-quality development of energy based on spatial Dubin model. Resour. Policy 74, 102451. doi:10.1016/j.resourpol.2021.102451
Wang, J., and Xu, C. (2012). Geodetectors:Principles and prospects. J. Geogr. doi:10.11821/dlxb201701010
Wang, X., and Johansson, A. (2013). Financial inhibition and structural transformation of the economy. Econ. Res. 48 (2). CNKI:SUN:JJYJ0.2013-01-007.
Wang, X., and Wang, Q. (2021). Research on the impact of green finance on the upgrading of China’s regional industrial structure from the perspective of sustainable development. Resour. Policy 74, 102436. doi:10.1016/j.resourpol.2021.102436
Wu, D., and Zhang, X. (2016). Cases study on fdi’s technical spillover on China’s industry based on labor & capital intensive industries. Resour. Ind. 18 (03), 121–127. doi:10.13776/j.cnki.resourcesindustries.20160613.006
Xu, D. (2008). A theoretical explanation and verification of industrial structure upgrading pattern determination and measurement. Fiscal Res. doi:10.19337/j.cnki.34-1093/f.2018.12.003
Xu, L., and Tan, J. (2020). Financial development, industrial structure and natural resource utilization efficiency in China. Resour. Policy 66, 101642. doi:10.1016/j.resourpol.2020.101642
Yuan, Q., and Zhou, H. (2020). An analysis on bank credit and industrial structure upgrading of beijing-tianjin-hebei region-based on technological innovation mode. J. Appl. Finance Bank., 1–17. doi:10.47260/jafb/1111
Zhang, B., and Wang, Y. (2019a). The effect of green finance on energy sustainable development: A case study in China. Emerg. Mark. Finance Trade 52, 3435–3454. doi:10.1080/1540496x.2019.1695595
Zhang, J., Fan, Z., Chen, Y., Gao, J., and Liu, W. (2020). Decomposition and decoupling analysis of carbon dioxide emissions from economic growth in the context of China and the ASEAN countries. Sci. Total Environ. 714, 136649. doi:10.1016/j.scitotenv.2020.136649
Zhang, S., Wu, Z., Wang, Y., and Hao, Y. (2021). Fostering green development with green finance: An empirical study on the environmental effect of green credit policy in China. J. Environ. Manag. 296, 113159. doi:10.1016/j.jenvman.2021.113159
Zhao, C., Yang Qingqing, Q., Zhu Yuanyuan, Y., Juxin, J., and Tu Lei, L. (2015). Spatial linkages and spatial organization of Hunan’s regional economy. Econ. Geogr. doi:10.15957/j.cnki.jjdl.2015.08.008
Zhao, X., Ma, X., Chen, B., Shang, Y., and Song, M. (2022). Challenges toward carbon neutrality in China: Strategies and countermeasures. Resour. Conservation Recycl. 176, 105959. [online]. doi:10.1016/j.resconrec.2021.105959
Zhou, J. (2022). Analysis and countermeasures of green finance development under carbon peaking and carbon neutrality goals. Open J. Soc. Sci. 10 (02), 147–154. doi:10.4236/jss.2022.102009
Zhou, T., Ding, R., Du, Y., Zhang, Y., Cheng, S., and Zhang, T. (2022). Study on the coupling coordination and spatial correlation effect of green finance and high-quality economic development—evidence from China. Sustainability 14 (6), 3137. doi:10.3390/su14063137
Keywords: green finance, industrial structure upgrading, regional economy, social network analysis, Geodetector
Citation: Nian W and Dong X (2022) Spatial correlation study on the impact of green financial development on industrial structure upgrading. Front. Environ. Sci. 10:1017159. doi: 10.3389/fenvs.2022.1017159
Received: 11 August 2022; Accepted: 08 September 2022;
Published: 27 September 2022.
Edited by:
Željko Stević, University of East Sarajevo, Bosnia and HerzegovinaReviewed by:
Hamed Fazlollahtabar, Damghan University, IranCopyright © 2022 Nian and Dong. This is an open-access article distributed under the terms of the Creative Commons Attribution License (CC BY). The use, distribution or reproduction in other forums is permitted, provided the original author(s) and the copyright owner(s) are credited and that the original publication in this journal is cited, in accordance with accepted academic practice. No use, distribution or reproduction is permitted which does not comply with these terms.
*Correspondence: Xiaohong Dong, cGVyZmVjdGpvYjA0NTFAMTI2LmNvbQ==
Disclaimer: All claims expressed in this article are solely those of the authors and do not necessarily represent those of their affiliated organizations, or those of the publisher, the editors and the reviewers. Any product that may be evaluated in this article or claim that may be made by its manufacturer is not guaranteed or endorsed by the publisher.
Research integrity at Frontiers
Learn more about the work of our research integrity team to safeguard the quality of each article we publish.