- 1College of Public Administration, Huazhong Agricultural University, Wuhan, Hubei, China
- 2School of Economics and Management, Tongji University, Shanghai, China
This study uses the introduction of the Green Credit Guidelines in 2012 as a quasi-natural experiment. We selected Chinese A-share listed enterprises from 2004 to 2020 as the sample and applied PSM-DID to examine the impact of green credit policy on the performance of construction energy-saving enterprises. The study revealed that: 1) green credit policy has a significant contribution to the performance of construction energy-saving enterprises. In addition, it still holds after the robustness tests (replacing the PSM matching method and adding or subtracting the two methods of control variables) and the placebo test. 2) A positive correlation between the performance of construction energy-saving enterprises and short-term debt. Meanwhile, short-term debt is a mediating variable between green credit policy and the performance of construction energy-saving enterprises. 3) The impact of green credit policy on the performance of non-state-owned (non-SOEs) is more pronounced compared to state-owned (SOEs). This study reveals the micro effects of green credit policy from the perspective of the performance of construction energy-saving enterprises. It not only helps to understand the economic effects of green credit policy, but also provides corresponding insights for the subsequent promotion of green credit policy and construction energy-saving enterprise development systems.
1 Introduction
Increasing global industrialization and over-exploitation of natural resources have caused environmental degradation issues (Chen L et al., 2022). In response to resource depletion and ecological disruption, 124 countries worldwide have, as of February 2021, declared their intention to become carbon neutral and achieve net-zero carbon emissions by 2050 or 2060 (Chen, 2021). In 2020, China proposed, at the 75th United Nations General Assembly, that it would adopt effective policies and measures to reach the carbon peak by 2030 and strive to achieve the national goal of carbon neutrality by 2060. The 2021 Global Status Report for Buildings and Construction showed that the construction sector accounted for 36% of global final energy consumption and 37% of energy-related CO2 emissions (Global CCS Institute, 2021). A fast-growing building boom in emerging countries makes this environmental problem even more challenging (Wang et al., 2018).
To meet future carbon emission management targets, except technical means, we also need to manage the financing and transaction costs of projects that are aligned to sustainable development goals (Kumar et al., 2022). This is known as sustainable finance, which is a finance tool that contributes to sustainability (Aizawa and Yang, 2010). As an important element of the sustainable financial system (Lian et al., 2022), China announced its green credit policy in 2007. The introduction of “green credit” has raised the threshold for loans to enterprises. Compliance with environmental testing standards, pollution control effectiveness, and ecological protection are important prerequisites for credit approval. It is to be used to curb the blind expansion of high-pollution and high-consumption industries. The China Banking Regulatory Commission issued the Green Credit Guidelines (GCGs) in 2012, calling on Chinese banks to provide credit to enterprises that emit pollutants or gobble up energy and natural resources to extend credit on preferential terms for green projects (Aizawa and Yang, 2010; Tan et al., 2022).
Debt financing from banks remains the most important source of external financing for research and development activities by enterprises in developing countries (Ayyagari et al., 2011; Liu et al., 2021). The green credit policy guides the green transformation of enterprises through capital allocation (reducing the scale of debt financing and increasing the cost of debt) for heavily polluting enterprises (Liu et al., 2021). But for environmentally friendly construction energy-saving enterprises, green credit policy is an even more superior demonstration of rapid financial performance improvement. In terms of the reallocation of credit resources, the introduction of green credit policy may increase the scale of debt financing and reduce the cost of debt, resulting in an improved capital investment structure and financial performance of construction energy-saving enterprises. In terms of policy practice, green credit policy priorities the provision of credit resources to enterprises with environmental performance, while avoiding credit support to enterprises with high environmental risks, thereby internalizing the external environmental costs of enterprises. Hence, green credit policy has to some extent facilitated the possibility of construction energy-saving enterprises financing for environmentally friendly constructions (Ghisetti et al., 2017; Wang E et al., 2019).
Previous studies on green credit have mainly focused on the manufacturing sector due to its heavy pollution (Liu et al., 2017; Sun et al., 2019), while few studies have been conducted on green credit in the construction sector. The construction sector has not yet undergone a real structural transformation, and its carbon emissions will continue to rise and contribute to climate warming. Green credit can improve the efficiency of resource allocation, continuously promote the advancement of the industrial structure, and rationalization of the industrial structure (Zhu, 2022). At the same time, the introduction of green credit policy can also encourage construction enterprises to actively improve environment protection and energy-efficiency technologies, thus efficiently solving the problem of environmental pollution (Hu et al., 2022).
In summary, only a few studies are exploring green credit policy in the construction sector. Thus, the introduction of green credit policy in the construction sector needed to be studied. Chinese enterprises always fail to take active environmental protection measures (Zhang et al., 2021). Moreover, the policy introduction is weak and the system needs to be improved in China (Kong et al., 2020). To address these issues, this study assesses the impact of green credit policy on the financial performance of construction energy-saving enterprises using secondary data on construction energy-saving enterprises in China. Furthermore, short-term debt and long-term debt may be key factors in the influencing mechanism of green credit policies on enterprises’ financial performance (Liu and Luo, 2019). Thus, the current study explores the following two research questions (RQ):
RQ1. In the context of the construction sector, what is the relationship between green credit policy and the financial performance of construction energy-saving enterprises?
RQ2. How do short-term debt and long-term debt mediate this relationship?This study explores how green credit policy influences the financial performance of construction energy-saving enterprises, while also considering the mediating effect of short-term debt and long-term debt and the moderation effect of controlling shareholders. Overall, this study makes four main contributions. Firstly, this study sampled listed construction energy-saving enterprises, contributing to the existing green credit policy-financial performance relationship literature by extending the research scope to the construction industry. Our results provide implications for the sustainable development of the construction industry. Secondly, the PSM-DID method was applied to non-random data and avoided the occurrence of sample selectivity bias and heterogeneity. It effectively makes the DID method satisfy the common trend. Thirdly, this study enriches the environmental economic research of construction enterprises by revealing how the short-term debt and long-term debt shape this green credit policy-financial performance relationship. Finally, this study can instruct the government, banks and enterprises to improve the green credit policy. The results guide policy initiatives and enterprises’ strategies targeted toward promoting the environmental efforts of construction enterprises and ultimately facilitating the achievement of the goal of carbon neutrality. The rest of this study is structured as follows. First, the study describes the theoretical foundation and research hypotheses. Then, the study reports the research methods and data analyses. The study closes with the implications and conclusions.
2 Theoretical foundation and research hypotheses
2.1 Stakeholder theory
Stakeholder theory shows that the pressure of implementing green credit may help enterprises ease the conflict between them and stakeholders, and achieve more healthy and sustainable development (Kitsikopoulos et al., 2018). The stakeholder theory dates back to 1932 and was proposed by E. Merrick Dodd. In 1963, the Stanford Research Institute (SRI) first proposed the concept of “stakeholders”. Stakeholder theory means that enterprises should consider the interests of other stakeholders in their daily operations, not only the interests of shareholders. Among them, stakeholders include employees, consumers and, above all, the community as a whole (Dodd, 1932).
As the environment deteriorates, public awareness of environmental and local ethnic issues is growing. This has led to the demand that corporations engage in socially desirable actions to establish congruence between corporate decision-making and social values (Baldini et al., 2018). Green credit policy is one of the means by which the government cooperates with financial institutions to urge enterprises to attach importance to energy conservation, environmental protection and green development through credit channels (Zhang et al., 2011). Referring to stakeholder theory, green credit’s implementation helps to alleviate the contradiction between enterprises and stakeholders, which can help them achieve more healthy and steady development (He et al., 2019b).
2.2 Signaling theory
In addition, the signaling theory shows that environmental regulation may encourage enterprises to standardize their behaviors, to transmit favorable signals to the public (Yu et al., 2017). In the financial market, due to the objective existence of information asymmetry, enterprises release the “signal” to the market by improving an enterprise’s environmental performance and social reputation, which leads to a reduction in the information asymmetry between an enterprise and its external stakeholders (Lys et al., 2015; García-Sánchez et al., 2020; Khan et al., 2021). Therefore, according to the lending targets and lending standards of green credit policy, enterprises that send a “green” signal to banks and the public can obtain more financing opportunities and reduce their financing costs. As a result, financial performance increases (Saeidi et al., 2015; Ren et al., 2020).
2.3 Green credit
Green credit is a means whereby financial institutions achieve sustainable economic and social development. It includes policies, institutional arrangements, and practices that promote environmental improvements and energy conservation through credit instruments (Lian et al., 2022). Green credit originated from the Equator Principles and is referred to as the international green credit policy (Aizawa and Yang, 2010), which is a voluntary agreement to mitigate environmental consequences and fulfill corporate social responsibility (Conley and Williams, 2011). Although not legally binding, the Equator Principles have gradually become the sector standard for financial institutions in practice, and the basic bottom line for green credit operations internationally (Lian et al., 2022).
Many studies have investigated green credit and environmental protection, business operations, and industrial structure (Hong et al., 2021; Hu et al., 2022; Li et al., 2022). For example, Liu et al. (2021) studied the relationship between green credit policy and enterprises’ green technology innovation performance. Lyu et al. (2022) explored the impact and mechanism of green credit policy on carbon emissions at the national and regional levels. Lai et al. (2022) discussed the impact of green credit on new energy enterprises’ value. Zhu (2022) analyzed the impact of green credit and technological innovation on industrial structure upgrading.
2.4 Green credit and enterprises’ financial performance
Many studies have shown a direct correlation between green credit and economic performance (e.g., enterprises’ financial performance and value) (Yao et al., 2021; Lai et al., 2022; Lian et al., 2022; Xi et al., 2022). Green credit policy will have a “penalty effect” on the financial performance of highly polluting enterprises and have a positive impact on the value of environmentally friendly enterprises (Yao et al., 2021; Lai et al., 2022).
The GCGs strategically set out more specific and clear requirements for financial institutions, including banks. The guidelines require banks and other financial institutions to implement green credit policy more effectively and to make every effort to promote energy conservation, emission reduction, and environmental protection (Lian et al., 2022). For example, commercial banks strictly control the threshold of credit approval by following guidelines and making the environmental performance of enterprises an important factor in credit approval. This is manifested in the form of loan support, or preferential interest rate loans, to environmentally friendly enterprises or green projects (Aizawa and Yang, 2010). In this way, construction energy-saving enterprises provide benefits and convenience, as well as achieve economic growth and environmental protection (Lai et al., 2022). This leads to the following hypothesis:
H1. Green credit policy has improved the financial performance of construction energy-saving enterprises compared to non-construction energy-saving enterprises.
2.5 Mediating effect of short-term debt and long-term debt
The process by which credit policy affects an enterprise can be described in the following way: Financial institutions influence the cash flow of enterprises through credit channels to manage their investments. This further affects the cash flow structure of enterprises, which ultimately changes the capital structure and the allocation structure of the factors of production. Consequently, the production and operation of enterprises will be greatly affected (Majumdar and Chhibber, 1999; Pisicoli and Bencivelli, 2021). In addition, enterprises with higher debt can reduce agency costs and lower value-free investments. According to static equilibrium theory, it is known that a lower corporate debt ratio leads to a lower corporate value. In turn, corporate value is closely linked to total corporate profits and return on net assets (Modigliani and Miller, 1958). When the corporate value is higher, enterprises can establish a good image of sound corporate operations as well as a greater public trust, which will lead to greater popularity of their products, which will result in increased corporate financial performance (Ioannou and Serafeim, 2012; Wang et al., 2022). Green credit policy can influence the borrowing ability and financing cost of enterprises (He et al., 2019a). The GCGs require banks to deny credit to enterprises with non-compliant environmental and social performance. From the perspective of signaling theory, financial institutions will provide preferential interest rates to eco-friendly enterprises to promote the development of environmental protection industries. Under the requirements of this policy, if commercial banks strictly control credit by policy requirements, heavily polluting enterprises with high environmental risks will be impacted by green credit policy and face debt financing dilemmas. Therefore, their debt financing scale, cost, and maturity will be constrained (Li et al., 2022). The construction energy-saving enterprises will receive preferential financing, which is propitious to enterprises’ financial performance and leads to the following hypotheses:
H2a. Green credit policy has a positive influence on the short-term debt of construction energy-saving enterprises.
H2b. Green credit policy has a positive influence on the long-term debt of construction energy-saving enterprises.
H3a. The financial performance of construction energy-saving enterprises is positively correlated with short-term debt.
H3b. The financial performance of construction energy-saving enterprises is positively correlated with long-term debt.
2.6 The heterogeneity of the effect of green credit policy on enterprises’ financial performance
Based on the type of controlling shareholders, the ownership of listed enterprises is typically classified into state-owned (SOEs) and non-state-owned (non-SOEs) (Kim et al., 2019). The proportion of the SOEs equity indicates the relationship between political factors and enterprises. SOEs have a natural political gene, and it is normal for the SOEs to have political connections and cooperate with the government (Cheng et al., 2017). Governments in China possess considerable control over the allocation of resources through their control of the SOEs. The development trend of SOEs is guided by the government’s policies and shares interests with the government. Therefore, the SOEs will more actively respond to the call of national policies, adhere to the concept of sustainable development and the scientific outlook on development, and make due contributions to environmental protection. Overall, enterprises with high political connections take a more active part in responding to the government’s environmental appeals (Li et al., 2022). In this way, SOEs tend to choose projects related to energy conservation and environmental protection because green credit policy provides favorable opportunities for them. This leads to the following hypotheses:
H4. Compared with non-SOEs, the impact of green credit policy on the financial performance of SOEs is more obvious.
3 Materials and methods
3.1 Sample and data sources
We used the A-share listed enterprises in China from 2004 to 2020 as a research sample. In 2012, the GCGs were introduced. Hence, we chose 2012 as the midpoint of this period and collected data for 8 years before and after. The reason for choosing data for the 8 years before and after is that the data of enterprises in the China Stock Market and Accounting Research (CSMAR)1 (http://cndata1.csmar.com/) database ends in March 2022. However, there is a large amount of missing annual data for 2021. Thus, we finally set the study period as 2004–2020. We used construction energy-saving enterprises as the treatment group and other enterprises as the control group. Construction energy-saving enterprises are defined by keywords (“Environmental Energy,” “Green Construction,” and “Construction Energy Efficiency”) searched through enterprise attributes in the Flush database2 (https://www.10jqka.com.cn/). We searched for a total of 166 construction energy-saving enterprises. Regarding the processing of the full sample, this study refers to the method used by Feenstra et al. (2014) to process the data. The data to be excluded are: ST listed enterprises and *ST listed enterprises; enterprises with integrated industries in the National Economic Classification; enterprises with vacant industry codes; and enterprises with selected variables less than or equal to 0. Finally, we obtain 15,093 observations from 1,022 listed enterprises for the full sample, of which the treatment group contains 10,019 and the control group contains 14,074.
3.2 Variable description
3.2.1 Dependent variable
The ROTA has been used extensively in previous studies (Binti Mohamad et al., 2017; Nguyen et al., 2021), and represents the ratio of total net profit to average total assets. It is primarily used to measure an enterprise’s ability to earn profits from its assets by reflecting the efficiency of utilizing total assets (Janicka and Sajnog, 2021). The higher this ratio is, the more profitable the total assets are (Janicka and Sajnog, 2021). In addition, the classification of the enterprise is based on fixed assets, which rely more on accounts payable that finance their total turnover. Therefore, ROTA can reflect the financial performance of an enterprise to a certain extent.
3.2.2 Independent variables
Since the GCGs were introduced in 2012, in this study the dummy variables Treated and Time are used to distinguish the four subcategories. Where Treated = 1 represents construction energy-saving enterprises, Treated = 0 represents non-construction energy-saving enterprises, Time = 1 represents the year after the introduction of green credit policy, and Time = 0 represents before the implementation of green credit policy.
3.2.3 Mediating variables
The enterprise’s debt refers to a present obligation arising from past transactions or events that are expected to result in an outflow of economic benefits. The level of debt of an enterprise is critical to its earnings and value (Yazdanfar and Öhman, 2015). Therefore, based on the availability of data, this study will examine the effect mechanism of green credit policy on the financial performance of construction energy-saving enterprises from short-term and long-term debts.
3.2.4 Control variables
To solve the bias problems that may be caused by the omission of variables in the equation of the model, as much as possible, this study controls the effects of these variables. Ten control variables are selected: stock returns (Ret) (Wang G et al., 2021), liquid ratio (Lr) (Zhang et al., 2015), enterprise total profit growth ratio (Np) (Huo and Zhang, 2017), enterprise age (Age) (Carnahan et al., 2010), enterprise liabilities to assets (L/A) ratio (Lev) (Díaz-Fernández et al., 2015), enterprise total asset turnover ratio (Tato) (Edward and Marciano, 2019), the enterprise growth rate of net profit (Profit) (Gao et al., 2021), enterprise operating growth ratio (Revenue) (Fu and Shen, 2020), equity nature (State) (Li, 2011), and Tobin’s q (Tq) (Lee et al., 2021) as control variables, and selects assets scale (Size) (Goll et al., 2008) and return on equity (Roe) (He et al., 2020) as replacement variables for the robustness test. Ret, Lr, Np, Lev, Tato, Profit, Revenue, Tq, Roe are collected from the financial annual reports of the enterprises. As important financial indicators, they are closely related to the financial performance of the enterprises (Azeez, 2015). The enterprise age is measured as the reporting period minus the number of years that the enterprise has been in the market (measured in months). Younger enterprises tend to be aggressive and innovative, while older enterprises usually experience organizational rigidity and reduced efficiency (Loderer and Waelchli, 2011; Edward and Marciano, 2019). Thus, enterprise age may affect financial performance by influencing the strategic decisions of the enterprise. The equity nature, with its unique flexibility, is the preferred way for controlling shareholders to alleviate financing constraints, and largely affects enterprises’ financial performance (Ren et al., 2022). Enterprise size is measured as the natural logarithm of the enterprise’s total assets at the end of the year. Smaller enterprises focus more on operations, while larger enterprises focus on strategy. Two different directions of development can have an impact on the financial performance of both over time (Laitinen and Kadak, 2018). The assignment rules of each variable and descriptive statistics are shown in Table 1.
3.3 Model specification
In the field of policy effect evaluation, the Differences in Differences (DID) method based on “quasi-natural experiments” is the most widely used research tool in recent years (Wang H et al., 2019; Chen et al., 2021; Fu et al., 2021; Zhang and Wang, 2021). As a purely exogenous event, the GCG satisfies the basic characteristics and assumptions of a quasi-natural experiment as not occurring for experimental purposes, and not influenced by individual companies (Withers and Li, 2021). Though the presence of other unobservable factors (individual fixed effects and environmental effects) may lead to less accurate policy assessment results, DID can exclude the effect of unobservable factors by taking the difference in results before and after policy introduction (Wang Z et al., 2021; Khan et al., 2022). Specifically, by comparing the differences in the effects of events on the treatment and control groups, the effects of other interfering causal factors or omitted variables can be overcome, allowing for better identification of causal relationships.
The essence of exploring the impact of green credit policy on the financial performance of construction energy-saving enterprises is to reveal the differences in the enterprises’ financial performance before and after the trial period. However, the most important premise of using the DID model is that the treatment and control groups must satisfy the common trend, i.e., in the absence of the GCGs, the financial performance of construction energy-saving enterprises and non-construction energy-saving enterprises will move in the same direction. Inconsistencies between treatment and control groups in sample selection methods or sample classification criteria are likely to lead to different trends. The PSM-DID method, proposed and developed by Heckman et al. (1997) can effectively make the DID method satisfy the common trend. PSM method is matched to the treatment and control groups, i.e., enterprises are found in the control group that is as consistent as possible with observable variables in the treatment group. Therefore, this study used construction energy-saving enterprises as the treatment group and non-construction energy-saving enterprises as the control group.
Subsequently, this study divides the sample of listed companies into four subsamples, i.e., the treatment group before and after the introduction of green credit policy and the control group before and after accordingly. The regression model is set up as follows. To test H1 and H4, if the coefficient is significantly positive, then green credit policy is likely to increase the financial performance of construction energy-saving enterprises, and H1 and H4 are valid.
To investigate the impact of green credit policy on enterprises by controlling for their bank credit status, this study refers to Sun et al. (2019) and Wu et al. (2022) to try out the mediation effect. We explored the mechanism of the impact of green credit policy on enterprises’ financial performance in terms of both short-term and long-term debts and constructed the following mediation effect model.
Models (2) and (3) are constructed to test for H2a and H3a. If the coefficients are significantly positive, then green credit policy affects construction energy-saving enterprises’ financial performance by influencing short-term debt. Models (4) and (5) are constructed to test H2b and H3b. If the coefficients are significantly positive, then long-term debt plays a partially mediating role in influencing green credit policy for construction energy-saving enterprises.
4 Results
4.1 Empirical analysis
4.1.1 Propensity score matching results and balance test
The PSM can be used to correct for bias in the estimates of intervention effects (Grose et al., 2020; Maina et al., 2020). It allows treatment and control groups to compare the differences in their estimates under conditions that are as similar as possible (Heinze and Jüni, 2011; Dong and Lipsey, 2018). Considering that the number of observations in the control group was much larger than the treatment group, this study used a 1:4 nearest neighbor matching method and was analyzed using Stata16 through a logit model. Table 2 depicts the PSM results after sample matching. The results showed that there was a good matching effect (not unmatched in both the treatment and control groups) (Stuart, 2010; Naz et al., 2018). To ensure that propensity scores between the treatment and control groups are not significantly different and to overcome the effect of selectivity bias, a balance test needs to be performed on the matched sample data (Heo et al., 2017; Fan and Zhang, 2021). Table 3 depicts the logit regression results and the balance test results. From Rosenbaum and Runim (1983), it is known that the matching effect is achieved if the absolute value of the bias after matching is less than 20%. This study discovered that the absolute value of the bias of all variables after matching was less than 20%, and there was a significant reduction in the standard deviation of each variable after matching in the treatment and control groups. The above results indicate that the condition of the treatment and control groups are relatively close after PSM by the balance test.
4.1.2 The impact of green credit policy on enterprises’ financial performance
According to the established model (1), the regression analysis was conducted on the full sample and PSM sample, as shown in Table 4. The first and second columns are regression results for the full sample. The coefficient of Time × Treated is 0.00385, which is non-significant at the 10% level when no control variables are included. Considering that there are potential relevant omitted variables, we added control variables. The regression results showed that the coefficient of Time × Treated is 0.00477, which is significantly positive at the 5% level. Therefore, our preliminary view is that the introduction of green credit policy can have a positive effect on the financial performance of construction energy-saving enterprises to some extent. The test under the full sample fails to accurately reflect the effect of the policy because enterprises may have significantly different variables and thus require a further regression analysis after the PSM method (Qin et al., 2020; Jia et al., 2021). The coefficients of Time × Treated are significantly positive at the 5% level (both without and with the inclusion of control variables), similar to the results of the full sample. In summary, the introduction of green credit policy makes the financial performance of construction energy-saving enterprises increase remarkably, and H1 is confirmed.
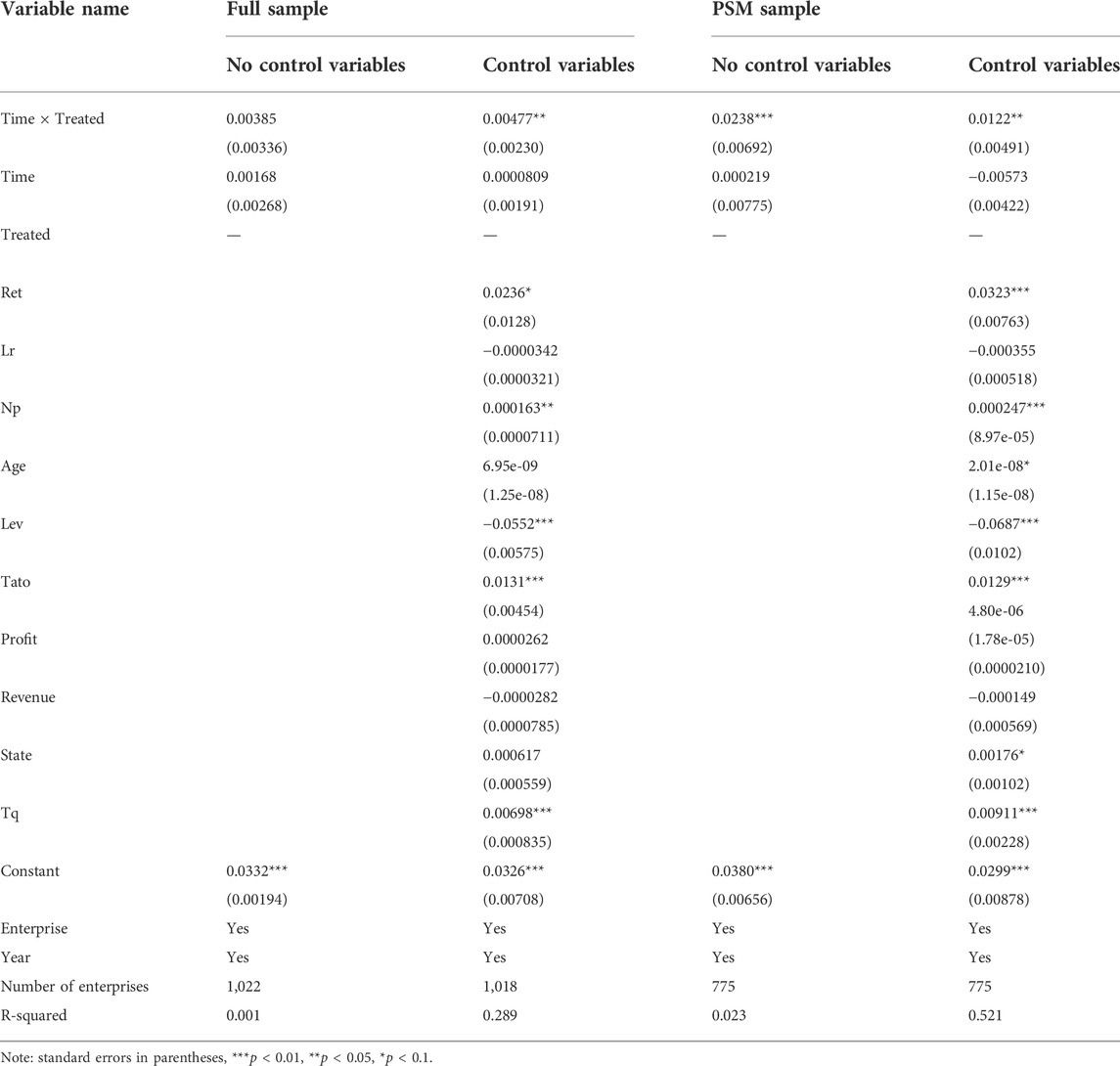
TABLE 4. A test of the impact of green credit policy on the financial performance of construction energy-saving enterprises.
4.2 Robustness tests and placebo tests
4.2.1 Replacement PSM method
Existing studies of PSM-DID frequently use replacement PSM methods for robustness tests. The previous section used 1:4 nearest neighbor matching, so we re-matched the samples by caliper matching and kernel matching. Regression analysis results are listed in Table 5, which shows that the regression coefficient of Time × Treated was still significantly positive at the 10% level after changing the matching method, which was not significantly different from the regression result of 1:4 nearest neighbor matching, thus the model (1) is robust.
4.2.2 Increase and decrease control variables
To fully test the robustness of the model, this study was also tested by replacing control variables. The impact of green credit policy on the financial performance of construction energy-saving enterprises is analyzed by replacing Np and Lr with Size and Roe in the control variables, and the regression results are shown in Table 6. Our findings indicate that the Time × Treated coefficient remained significantly positive at the 10% level with the inclusion of control variables. There is no substantial difference between this and the regression results in the previous section, which fully confirms the robustness of the model (1).
4.2.3 Placebo tests
The above study shows that the introduction of a green credit policy leads to a remarkable improvement in the financial performance of construction energy-saving enterprises. This study used the PSM-DID model to overcome the endogeneity between green credit policy and the financial performance of construction energy-saving enterprises (Dong and Zheng, 2022; Guo et al., 2022). The most disruptive to DID’s evaluation of policy effects comes from one fact: The resulting impact may not be due to the green credit policy in this study, but is instead influenced by other policies or factors (Dong and Zheng, 2022; Guo et al., 2022). Typically, in studies using the PSM-DID model, a placebo test is utilized to test the robustness of the results (Fu et al., 2021). In Summary, to exclude the influence of random factors on the experiment and to improve the credibility of our conclusions, we used a placebo test to test the treatment results. Figure 2 reports the kernel density distribution of the estimated coefficients for the 500 randomly generated treatment groups. It shows the mean value of the regression coefficients is nearly 0, indicating that the change in the financial performance of construction energy-saving enterprises is indeed affected by the green credit policy.
4.3 Mechanism analysis
Short-term debt and long-term debt can be used to examine the mechanisms by which green credit policy affects the financial performance of construction energy-saving enterprises. Table 7 reports the results of the tests for the role of Shortdebt and Longdebt on ROTA. In particular, model (1) is the mediation effect test step one, which tests the effect of Time × Treated on ROTA. The results show that the coefficient of Time × Treated is significantly positive, indicating that green credit policy makes construction energy-saving enterprises perform better, supporting the view of H1. Models (2) and (4) are the second step, which investigates the effect of Time × Treated on the mediating variables (Shortdebt and Longdebt). The coefficient of the Time × Treated is significantly positive at the 1% level when the mediating variable is Shortdebt, indicating that green credit policy has a positive effect on the short-term debt of construction energy-saving enterprises. Whereas when the mediating variable is Longdebt, the coefficient of Time × Treated is positive but insignificant. There is not enough evidence that the introduction of green credit policy has a significant impact on the long-term debt of construction energy-saving enterprises. Models (3) and (5) are the third step, which examines the effect of ROTA on the mediating variables (Shortdebt and Longdebt). The results show that the coefficient is significantly positive when the mediating variable is Shortdebt, indicating that the financial performance of construction energy-saving enterprises is positively correlated with short-term debt. Short-term debt is a vital factor affecting the financial performance of construction energy-saving enterprises. While the coefficient is positive but insignificant when the mediating variable is Longdebt, it fails to draw the same conclusions as short-term debts. In addition, this study provided three significant tests during the mediation command test (Sobel, Doodman1, and Goodman2 tests), all of which were found to be significant. Taken together, short-term debt has a mediation effect between the introduction of green credit policy and the financial performance of construction energy-saving enterprises. We identified a mechanism for the impact of green credit policy on the construction energy-saving enterprises, which is “green credit policy-short-term debt-financial performance of construction energy-saving enterprises.” Combined with the above analysis, H2a and H3a are confirmed without supporting H2b and H3b.
4.4 Heterogeneity analysis
To further investigate whether the controlling shareholders affect the effectiveness of introducing green credit policy, this study divides the full sample and the PSM sample into SOEs and non-SOEs and then analyzes them in the subsample. Table 8 shows that the coefficients are larger (and significant) for non-SOEs in both the full sample and the PSM sample, indicating the impact of green credit policy on the financial performance of non-SOEs in construction energy-saving enterprises is more pronounced. But this is the opposite of H3. Though many studies contradict our findings (Hu et al., 2021; Chai et al., 2022; Chen Z et al., 2022), it seems to imply that the green credit policy may be beneficial to non-SOEs for construction sectors with different controlling shareholders, which needs further investigation.
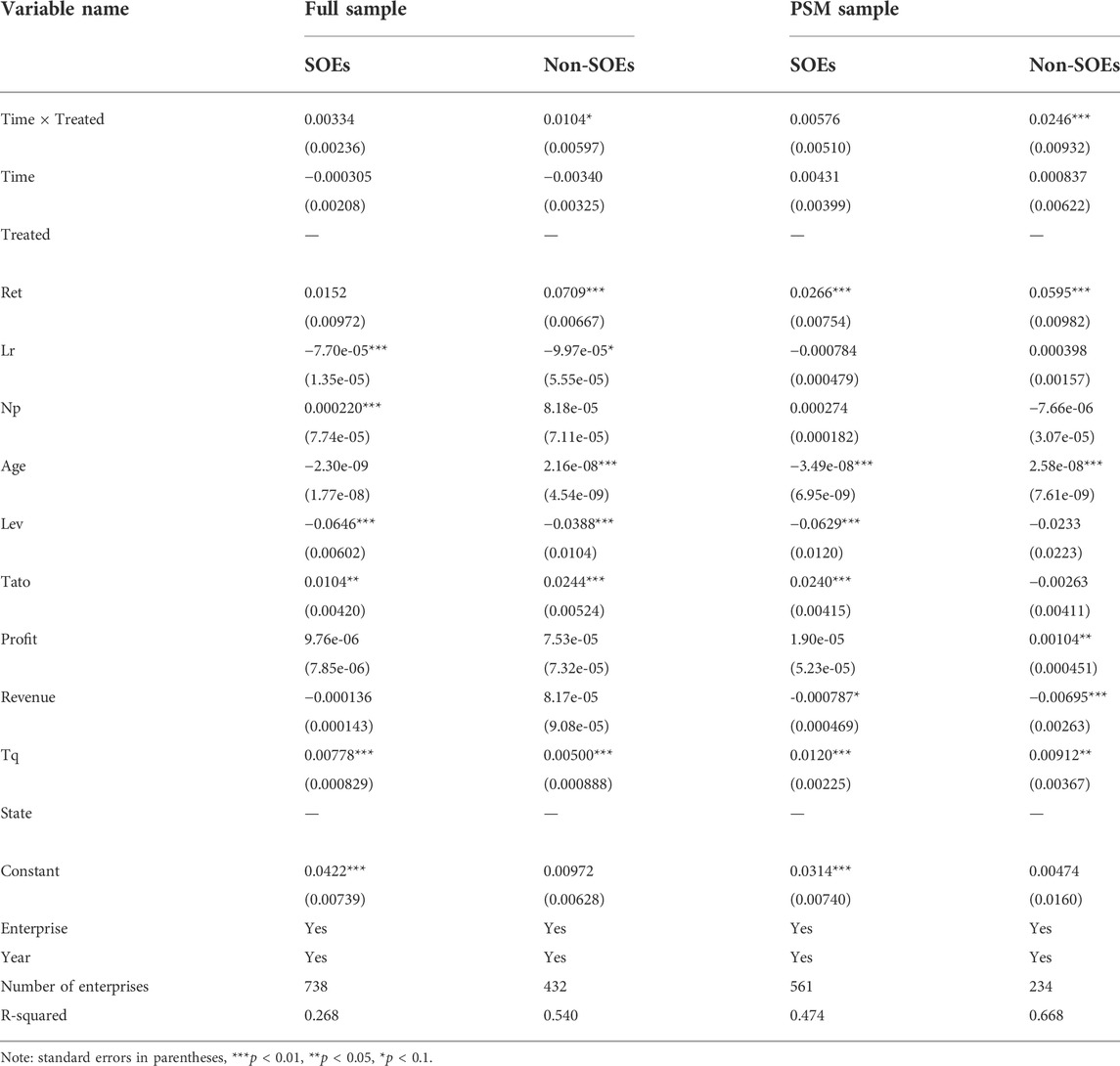
TABLE 8. A test of the impact of green credit policy on the financial performance of construction energy-saving enterprises under the difference of controlling shareholders.
5 Discussion and implications
The results of numerous green credit policy studies show that these policies affect enterprises’ financial performance (Fried et al., 2002; Loderer and Waelchli, 2011; Brandstädter et al., 2012; Cheng and Lu, 2017; Zheng et al., 2021). However, most of the research on green credit has focused on enterprises that are heavily polluted, and less on construction energy-saving enterprises. Therefore, this study examines the impact of green credit policy on the financial performance of construction energy-saving enterprises using A-share enterprises (divided into construction energy-saving enterprises and non-construction energy-saving enterprises) from 2004 to 2020. We used PSM to match the observations and built a DID model to evaluate the effect of introducing green credit policy. Additionally, we analyzed the mechanism of the impact of green credit policy on the financial performance of construction energy-saving enterprises by a mediation effect model. Further, we categorized the sample according to the controlling shareholders (SOEs and non-SOEs) to explore the impact of green credit policy on each subsample.
5.1 Theoretical implications
This study highlights several intriguing theoretical implications.
Firstly, green credit policy has contributed significantly to the financial performance of construction energy-saving enterprises. Some previous studies find that green credit significantly improved the financial performance of eco-friendly enterprises (Yao et al., 2021; Lai et al., 2022), which is consistent with our results. We extend the research to the construction industry. With the introduction of green credit policy, financial institutions are required to comprehensively consider the environmental behavior of enterprises in their credit decisioning, according to the requirements of national policies. Meanwhile, environmental risk is the main basis for measuring credit risk. As a result, financial institutions prefer less risky enterprises to finance. Indeed, construction energy-saving enterprises are environmentally friendly and virtually immune to environmental risks. From a profitability and security perspective, the cost of credit financing for construction energy-saving enterprises is significantly lower than for non-construction energy-saving enterprises, thereby improving the financial performance of construction energy-saving enterprises. More importantly, financial institutions cannot suffer reputational damage by establishing credit relationships with enterprises involved in environmental incidents.
Secondly, the financial performance of construction energy-saving enterprises is positively related to short-term debt, and short-term debt is a mediating variable between green credit policy and the financial performance of construction energy-saving enterprises. This study enriches the green credit research of the construction industry by revealing how debt meditates this green credit policy-financial performance relationship. We identified the mechanism by which green credit policy affects construction energy-saving enterprises, namely “green credit policy-short-term debt-financial performance of construction energy-saving enterprises.” One of the core elements of the green credit policy is that for environmentally friendly enterprises and projects, banks will support them in terms of loan interest rates, loan terms, loan support amounts and loan types. Specifically, financial institutions offer loans to heavily polluting enterprises at higher interest rates than energy-saving enterprises, thereby causing heavily polluting enterprises to reduce the scale of production and reduce environmental pollution. Hence, financial institutions will provide support to construction energy-saving enterprises and promote their development.
Thirdly, the impact of green credit policy on the financial performance of non-SOEs (construction energy-saving enterprises) is more significant. Chai et al. (2022) found that SOEs are more affected by green credit policy than non-SOEs. Hu et al. (2021) confirmed that heavily polluting SOEs have greater credit constraints than non-SOEs. Chen L et al. (2022) concluded that green credit policy has a more significant contribution to low carbon technology innovation in SOEs. A unifying view that seems to emerge from all of the above studies is that as SASAC3-controlled enterprises, SOEs are subject to greater state regulation and control, ultimately leading to higher output for SOEs than non-SOEs. This view is contrary to the findings of this study, and an in-depth analysis of the data suggests that the reasons for the opposite conclusion are twofold. On the one hand, SOEs are generally thought to forgo maximum profit in the pursuit of social and political objectives. And non-SOEs are more profitable than SOEs (Dewenter and Malatesta, 2001). On the other hand, while the green credit guidelines were introduced in 2012, their application in the construction sector actually started late and developed slowly. Therefore, the impact of the construction sector on SOEs and non-SOEs may not be significantly different.
5.2 Policy implications
Green credit policy helps encourage construction energy-saving enterprises to fulfill their social responsibility to protect the environment. It can greatly facilitate the development of green enterprises in China and aid the country in achieving comprehensive, coordinated, and sustainable economic growth.
Firstly, the government, banks and enterprises need to improve the green credit policy. We find that green credit will benefit environmentally friendly enterprises. The government needs to develop actionable introduction rules based on business operations and establish an incentive mechanism, as well as a regulatory system, that matches the green credit policy. Moreover, it should strictly check the flow of green credit capital and dedicate green loans to specific purposes. Commercial banks need to carry out targeted corporate credit assessment mechanisms. Specifically, this means establishing a credit management system that meets the characteristics of green lenders and dynamically adjusting credit resources according to the specific conditions of enterprises. Meanwhile, a relevant information management platform can be established to realize information sharing between enterprises and banks to ensure that the introduction of the policy can achieve the expected effect and form a positive social impact. Enterprises should strengthen their awareness of environmental protection, pay attention to internal green governance, and consciously assume social responsibility for ecological protection. They should make full use of the development opportunities brought by the national green credit policy to change production methods, enhance green technology innovation, accelerate the green transformation and upgrade enterprise structures, and promote green and efficient development of enterprises.
Secondly, this study confirms that short-term debt positively affects the financial performance of construction energy-saving enterprises as a mediating variable. Banks can decrease the maturity of some debt relating to “sustainable finance,” and governments can provide more supportive policies for the short-term debt of construction energy-saving enterprises. These measures promote enterprises to restructure their capital and reduce the cost of financing to protect against risk.
Thirdly, the government should strengthen the external supervision and discipline of SOEs. The results of heterogeneity analysis indicate that the impact of green credit policy on the financial performance of non-SOEs was more pronounced compared to SOEs. While policy changes can lead to improvements in SOEs performance, the improvements may dissipate over time without added discipline (Yarrow et al., 1986). Non-SOEs tend to take action in light of the maximization of enterprises’ value and contribute more to economic growth (Chang et al., 2015). Policies that explicitly mix economic and political interests and incentives to enterprises should be encouraged.
6 Conclusion and future directions
Based on secondary data of A-share enterprises (divided into construction energy-saving enterprises and non-construction energy-saving enterprises) from 2004 to 2020, we use PSM to match the data and build a DID model to evaluate the effect of introducing green credit policy. First, this study proposes a set of hypotheses combined with existing green credit and financial performance literature. Second, this study defined the research variables and models. Third, this study conducted descriptive statistics, PSM test, balance test, DID test, and robustness tests to analyze the empirical results, and verified the hypotheses.
This study revealed that the introduction of the green credit policy makes the financial performance of construction energy-saving enterprises increase remarkably. Environmentally friendly enterprises benefit from green credit policy. Furthermore, short-term debt has a positive mediation effect between the introduction of green credit policy and the financial performance of construction energy-saving enterprises. In the context of green credit policy, appropriately higher levels of short-term debt can contribute to enterprises’ financial performance. This study enriches the empirical research of green credit-financial performance relationship with the mediating effect of enterprises’ debt in the construction sector. Finally, the heterogeneity analysis concludes that the impact of green credit policy on the financial performance of non-SOEs in construction energy-saving enterprises is more pronounced. This study explores the introduction effects of the green credit policy on the financial performance of Chinese construction energy-saving enterprises. The findings provide practical guidance for the government, banks and enterprises to promote the sustainable development of the construction sector.
Although this study has come to several conclusions, it also suggests further investigation. First, China launched the green credit policy in 2012, their application in the construction sector started late and developed slowly. Using the PSM-DID method to empirically test the impact mechanism of the green credit policy may require a longer period. There are many problems in the introduction of a policy during the early stages of implementation, so the effectiveness of the policy will become more accurate over time. We can do more tracking studies in the future. Second, in future studies, we can explore a more nuanced influencing mechanism of green credit policy on the financial performance of construction energy-saving enterprises. For example, the conditions of enterprises’ environmental protection (He et al., 2019b), enterprise characteristics (Mcintyre et al., 2007), regional economic development (Lai et al., 2022), and capital (Hu et al., 2020), which are hot topics of discussion in corporate governance studies. Future studies could investigate their role in the relationship between green credit policy and financial performance.
Data availability statement
The raw data supporting the conclusion of this article will be made available by the authors, without undue reservation.
Author contributions
LX and LY contributed to conception and design of the study. LX organized the database and performed the statistical analysis. LX and LY wrote the first draft of the manuscript. All authors contributed to manuscript revision, read, and approved the submitted version.
Conflict of interest
The authors declare that the research was conducted in the absence of any commercial or financial relationships that could be construed as a potential conflict of interest.
Publisher’s note
All claims expressed in this article are solely those of the authors and do not necessarily represent those of their affiliated organizations, or those of the publisher, the editors and the reviewers. Any product that may be evaluated in this article, or claim that may be made by its manufacturer, is not guaranteed or endorsed by the publisher.
Supplementary material
The Supplementary Material for this article can be found online at: https://www.frontiersin.org/articles/10.3389/fenvs.2022.1004247/full#supplementary-material
Footnotes
1CSMAR database is a research-oriented and accurate database in economic and financial field developed by Shenzhen Sigma Data Technology Co., Ltd. The data began in 1998 and has been continuously updated.
2Flush database is the largest stock trading database in China.
3State-owned Assets Supervision and Administration Commission of the State Councial (SASAC) is for the State Council directly under the ad hoc institutions.
References
Aizawa, M., and Yang, C. (2010). Green credit, green stimulus, green revolution? china’s mobilization of banks for environmental cleanup. J. Environ. Dev. 19, 119–144. doi:10.1177/1070496510371192
Ayyagari, M., Demirgüç-Kunt, A., and Maksimovic, V. (2011). Firm innovation in emerging markets: The role of finance, governance, and competition. J. Financ. Quant. Anal. 46, 1545–1580. doi:10.1017/S0022109011000378
Azeez, D. A. A. (2015). Corporate governance and firm performance: Evidence from Sri Lanka. J. Financ. Bank. Manag. 3. doi:10.15640/jfbm.v3n1a16
Binti Mohamad, N. E. A., Abd Rahman, N. R. B., and Mohd Saad, N. B. (2017). Linking working capital policy towards financial performance of small medium enterprise (SME) in Malaysia. SHS Web Conf. 36, 00021. doi:10.1051/shsconf/20173600021
Brandstädter, K., Harms, U., and Großschedl, J. (2012). Assessing system thinking through different concept-mapping practices. Int. J. Sci. Educ. 34, 2147–2170. doi:10.1080/09500693.2012.716549
Carnahan, S., Agarwal, R., and Campbell, B. (2010). The effect of firm compensation structures on the mobility and entrepreneurship of extreme performers. Business 758, 1–43. doi:10.1002/smj
Chai, S., Zhang, K., Wei, W., Ma, W., and Abedin, M. Z. (2022). The impact of green credit policy on enterprises’ financing behavior: Evidence from Chinese heavily-polluting listed companies. J. Clean. Prod. 363, 132458. doi:10.1016/j.jclepro.2022.132458
Chang, L., Li, W., and Lu, X. (2015). Government engagement, environmental policy, and environmental performance: Evidence from the most polluting chinese listed firms. Bus. Strategy Environ. 24, 1–19. doi:10.1002/bse.1802
Chen, H., Guo, W., Feng, X., Wei, W., Liu, H., Feng, Y., et al. (2021). The impact of low-carbon city pilot policy on the total factor productivity of listed enterprises in China. Resour. Conserv. Recycl. 169, 105457. doi:10.1016/j.resconrec.2021.105457
Chen, J. M. (2021). Carbon neutrality: Toward a sustainable future. Innov. (Camb). 2, 100127. doi:10.1016/j.xinn.2021.100127
Chen, L., Msigwa, G., Yang, M., Osman, A. I., Fawzy, S., Rooney, D. W., et al. (2022). Strategies to achieve a carbon neutral society: a review. Environ. Chem. Lett. 20, 2277–2310. doi:10.1007/s10311-022-01435-8
Chen, Z., Zhang, Y., Wang, H., Ouyang, X., and Xie, Y. (2022). Can green credit policy promote low-carbon technology innovation? J. Clean. Prod. 359, 132061. doi:10.1016/j.jclepro.2022.132061
Cheng, M., and Lu, Y. (2017). Investment efficiency of urban infrastructure systems: Empirical measurement and implications for China. Habitat Int. 70, 91–102. doi:10.1016/j.habitatint.2017.10.008
Cheng, Z., Wang, F., Keung, C., and Bai, Y. (2017). Will corporate political connection influence the environmental information disclosure level? Based on the panel data of A-shares from listed companies in shanghai stock market. J. Bus. Ethics 143, 209–221. doi:10.1007/s10551-015-2776-0
Conley, J. M., and Williams, C. A. (2011). Global banks as global sustainability regulators?: The equator principles. Law Policy 33, 542–575. doi:10.1111/j.1467-9930.2011.00348.x
Dewenter, K. L., and Malatesta, P. H. (2001). State-owned and privately owned firms: An empirical analysis of profitability, leverage, and labor intensity. Am. Econ. Rev. 91, 320–334. doi:10.1257/aer.91.1.320
Díaz-Fernández, M. C., González-Rodríguez, M. R., and Simonetti, B. (2015). Top management team’s intellectual capital and firm performance. Eur. Manag. J. 33, 322–331. doi:10.1016/j.emj.2015.03.004
Dong, F., and Zheng, L. (2022). The impact of market-incentive environmental regulation on the development of the new energy vehicle industry: a quasi-natural experiment based on China’s dual-credit policy. Environ. Sci. Pollut. Res. 29, 5863–5880. doi:10.1007/s11356-021-16036-1
Dong, N., and Lipsey, M. W. (2018). Can propensity score analysis approximate randomized experiments using pretest and demographic information in pre-K intervention research? Eval. Rev. 42, 34–70. doi:10.1177/0193841X17749824
Edward, J. H., and Marciano, D. (2019). “ Internationalization, firm performance, and capital structure: an empirical study in Indonesia,” in Proceedings of the 16th International Symposium on Management (INSYMA 2019), 52–56. doi:10.2991/insyma-19.2019.14
Fan, F., and Zhang, X. (2021). Transformation effect of resource-based cities based on PSM-DID model: An empirical analysis from China. Environ. Impact Assess. Rev. 91, 106648. doi:10.1016/j.eiar.2021.106648
Feenstra, R. C., Li, Z., and Yu, M. (2014). Exports and credit constraints under incomplete information: Theory and evidence from China. Rev. Econ. Stat. 96, 729–744. doi:10.1162/REST_a_00405
Fried, H. O., Lovell, C. A. K., Schmidt, S. S., and Yaisawarng, S. (2002). Accounting for environmental effects and statistical noise in Data Envelopment Analysis. J. Product. Anal. 17, 157–174. doi:10.1023/A:1013548723393
Fu, M., and Shen, H. (2020). COVID-19 and corporate performance in the energy industry. Energy Res. Lett. 1, 1–5. doi:10.46557/001c.12967
Fu, Y., He, C., and Luo, L. (2021). Does the low-carbon city policy make a difference? Empirical evidence of the pilot scheme in China with DEA and PSM-DID. Ecol. Indic. 122, 107238. doi:10.1016/j.ecolind.2020.107238
Gao, Y., Yao, Y., and Li, Y. (2021). “Analysis on the influence mechanism of corporate stock price based on Lasso-CNN neural network,” in 2021 International Conference on Computer Information Science and Artificial Intelligence (CISAI), China, Sep 17-19 (IEEE), 1048–1052. doi:10.1109/CISAI54367.2021.00210
García-Sánchez, I. M., Aibar-Guzmán, B., Aibar-Guzmán, C., and Azevedo, T. C. (2020). CEO ability and sustainability disclosures: The mediating effect of corporate social responsibility performance. Corp. Soc. Responsib. Environ. Manag. 27, 1565–1577. doi:10.1002/csr.1905
Ghisetti, C., Mancinelli, S., Mazzanti, M., and Zoli, M. (2017). Financial barriers and environmental innovations: evidence from EU manufacturing firms. Clim. Policy 17, S131–S147. doi:10.1080/14693062.2016.1242057
Goll, I., Brown Johnson, N., and Rasheed, A. A. (2008). Top management team demographic characteristics, business strategy, and firm performance in the US airline industry: The role of managerial discretion. Manag. Decis. 46, 201–222. doi:10.1108/00251740810854122
Grose, E., Wilson, S., Barkun, J., Bertens, K., Martel, G., Balaa, F., et al. (2020). Use of propensity score methodology in contemporary high-impact surgical literature. J. Am. Coll. Surg. 230, 101–112. doi:10.1016/j.jamcollsurg.2019.10.003
Guo, Q., Wang, Y., and Dong, X. (2022). Effects of smart city construction on energy saving and CO2 emission reduction: Evidence from China. Appl. Energy 313, 118879. doi:10.1016/j.apenergy.2022.118879
He, L., Wu, C., Yang, X., and Liu, J. (2019a). Corporate social responsibility, green credit, and corporate performance: an empirical analysis based on the mining, power, and steel industries of China. Nat. Hazards 95, 73–89. doi:10.1007/s11069-018-3440-7
He, L., Zhang, L., Zhong, Z., Wang, D., and Wang, F. (2019b). Green credit, renewable energy investment and green economy development: Empirical analysis based on 150 listed companies of China. J. Clean. Prod. 208, 363–372. doi:10.1016/j.jclepro.2018.10.119
He, Q., Li, X., and Zhu, W. (2020). Political connection and the walking dead: Evidence from China’s privately owned firms. Int. Rev. Econ. Finance 69, 1056–1070. doi:10.1016/j.iref.2018.12.007
Heckman, J. J., Ichimura, H., and Todd, P. E. (1997). Matching as an econometric evaluation estimator: Evidence from evaluating a job training programme. Rev. Econ. Stud. 64, 605–654. doi:10.2307/2971733
Heinze, G., and Jüni, P. (2011). An overview of the objectives of and the approaches to propensity score analyses. Eur. Heart J. 32, 1704–1708. doi:10.1093/eurheartj/ehr031
Heo, S.-H., Yoon, K.-W., Woo, S.-Y., Park, Y.-J., Kim, Y.-W., Kim, K.-H., et al. (2017). Editor’s choice – comparison of early outcomes and restenosis rate between carotid endarterectomy and carotid artery stenting using propensity score matching analysis. Eur. J. Vasc. Endovasc. Surg. 54, 573–578. doi:10.1016/j.ejvs.2017.08.006
Hong, M., Li, Z., and Drakeford, B. (2021). Do the green credit guidelines affect corporate green technology innovation? Empirical research from China. Int. J. Environ. Res. Public Health 18, 1682–1721. doi:10.3390/ijerph18041682
Hu, G., Wang, X., and Wang, Y. (2021). Can the green credit policy stimulate green innovation in heavily polluting enterprises? Evidence from a quasi-natural experiment in China. Energy Econ. 98, 105134. doi:10.1016/j.eneco.2021.105134
Hu, Q., Li, X., and Feng, Y. (2022). Do green credit affect green total factor productivity? Empirical evidence from China. Front. Energy Res. 9, 1–15. doi:10.3389/fenrg.2021.821242
Hu, Y., Jiang, H., and Zhong, Z. (2020). Impact of green credit on industrial structure in China: theoretical mechanism and empirical analysis. Environ. Sci. Pollut. Res. 27, 10506–10519. doi:10.1007/s11356-020-07717-4
Huo, X., and Zhang, L. (2017). “Research on the assessment of the risk of material misstatement of the enterprise under internet plus environment,” in 2017 3rd International Conference on Information Management (ICIM), Chengdu, China, 21st to 23rd of April (IEEE), 154–158. doi:10.1109/INFOMAN.2017.7950366
Ioannou, I., and Serafeim, G. (2012). What drives corporate social performance the role of nation-level institutions. J. Int. Bus. Stud. 43, 834–864. doi:10.1057/jibs.2012.26
Janicka, M., and Sajnog, A. (2021). The European union’s environmental policy and long-term investments of enterprises. Eur. Res. Stud. J. XXIV, 335–355. doi:10.35808/ersj/2660
Jia, R., Shao, S., and Yang, L. (2021). High-speed rail and CO2 emissions in urban China: A spatial difference-in-differences approach. Energy Econ. 99, 105271. doi:10.1016/j.eneco.2021.105271
Khan, N., Ray, R. L., Zhang, S., Osabuohien, E., and Ihtisham, M. (2022). Influence of mobile phone and internet technology on income of rural farmers: Evidence from Khyber Pakhtunkhwa Province, Pakistan. Technol. Soc. 68, 101866. doi:10.1016/j.techsoc.2022.101866
Khan, T. M., Gang, B., Fareed, Z., and Khan, A. (2021). How does CEO tenure affect corporate social and environmental disclosures in China? Moderating role of information intermediaries and independent board. Environ. Sci. Pollut. Res. 28, 9204–9220. doi:10.1007/s11356-020-11315-9
Kim, B., Pae, J., and Yoo, C. Y. (2019). Business groups and tunneling: Evidence from corporate charitable contributions by Korean companies. J. Bus. Ethics 154, 643–666. doi:10.1007/s10551-016-3415-0
Kitsikopoulos, C., Schwaibold, U., and Taylor, D. (2018). Limited progress in sustainable development: Factors influencing the environmental management and reporting of South African JSE-listed companies. Bus. Strategy Environ. 27, 1295–1301. doi:10.1002/bse.2176
Kong, X., Pan, Y., Sun, H., and Taghizadeh-Hesary, F. (2020). Can environmental corporate social responsibility reduce firms’ idiosyncratic risk? Evidence from China. Front. Environ. Sci. 8, 1–15. doi:10.3389/fenvs.2020.608115
Kumar, S., Sharma, D., Rao, S., Lim, W. M., and Mangla, S. K. (2022). Past, present, and future of sustainable finance: insights from big data analytics through machine learning of scholarly research. Ann. Oper. Res., 1–44. doi:10.1007/s10479-021-04410-8
Lai, X., Yue, S., and Chen, H. (2022). Can green credit increase firm value? Evidence from Chinese listed new energy companies. Environ. Sci. Pollut. Res. 29, 18702–18720. doi:10.1007/s11356-021-17038-9
Laitinen, E., and Kadak, T. (2018). Does the company size affect performance management system? PMSs in small, medium-sized, and large companies. Int. J. Manag. Enterp. Dev. 17, 281–305. doi:10.1504/ijmed.2018.10014496
Lee, C.-C., Lee, C.-C., and Xiao, S. (2021). Policy-related risk and corporate financing behavior: Evidence from China’s listed companies. Econ. Model. 94, 539–547. doi:10.1016/j.econmod.2020.01.022
Li, W., Cui, G., and Zheng, M. (2022). Does green credit policy affect corporate debt financing? Evidence from China. Environ. Sci. Pollut. Res. 29, 5162–5171. doi:10.1007/s11356-021-16051-2
Li, X. (2011). Expected difference, equity nature and the corporate control agreement transfer failed—the evidence from China. J. Serv. Sci. Manag. 04, 22–26. doi:10.4236/jssm.2011.41004
Lian, Y., Gao, J., and Ye, T. (2022). How does green credit affect the financial performance of commercial banks? ——evidence from China. J. Clean. Prod. 344, 131069. doi:10.1016/j.jclepro.2022.131069
Liu, J. Y., Xia, Y., Fan, Y., Lin, S. M., and Wu, J. (2017). Assessment of a green credit policy aimed at energy-intensive industries in China based on a financial CGE model. J. Clean. Prod. 163, 293–302. doi:10.1016/j.jclepro.2015.10.111
Liu, Q., and Luo, C. (2019). The impact of government integrity on investment efficiency in regional transportation infrastructure in China. Sustainability 11, 6747. doi:10.3390/su11236747
Liu, S., Xu, R., and Chen, X. (2021). Does green credit affect the green innovation performance of high-polluting and energy-intensive enterprises? Evidence from a quasi-natural experiment. Environ. Sci. Pollut. Res. 28, 65265–65277. doi:10.1007/s11356-021-15217-2
Loderer, C. F., and Waelchli, U. (2011). Firm age and performance. Working Paper 912700. doi:10.2139/ssrn.1342248
Lys, T., Naughton, J. P., and Wang, C. (2015). Signaling through corporate accountability reporting. J. Account. Econ. 60, 56–72. doi:10.1016/j.jacceco.2015.03.001
Lyu, B., Da, J., Ostic, D., and Yu, H. (2022). How does green credit promote carbon reduction ? A mediated model. Front. Environ. Sci. 10, 1–10. doi:10.3389/fenvs.2022.878060
Maina, K. W., Ritho, C. N., Lukuyu, B. A., and Rao, E. J. O. (2020). Socio-economic determinants and impact of adopting climate-smart Brachiaria grass among dairy farmers in Eastern and Western regions of Kenya. Heliyon 6, e04335. doi:10.1016/j.heliyon.2020.e04335
Majumdar, S. K., and Chhibber, P. (1999). Capital structure and performance: Evidence from a transition economy on an aspect of corporate governance. Public Choice 98, 287–305. doi:10.1023/a:1018355127454
Mcintyre, M. L., Murphy, S. A., and Mitchell, P. (2007). The top team: Examining board composition and firm performance. Corp. Gov. Int. J. Bus. Soc. 7, 547–561. doi:10.1108/14720700710827149
Modigliani, F., and Miller, M. H. (1958). The cost of capital, corporation finance and the theory of investment. Am. Econ. Rev. 48, 154–155. doi:10.4013/base.20082.07
Naz, L., Ali, A., and Fatima, A. (2018). International competitiveness and ex-ante treatment effects of CPEC on household welfare in Pakistan. Int. J. Dev. Issues 17, 168–186. doi:10.1108/IJDI-05-2017-0100
Nguyen, T. H. H., Elmagrhi, M. H., Ntim, C. G., and Wu, Y. (2021). Environmental performance, sustainability, governance and financial performance: Evidence from heavily polluting industries in China. Bus. Strategy Environ. 30, 2313–2331. doi:10.1002/bse.2748
Pisicoli, B., and Bencivelli, L. (2021). Foreign investors and target firms’ financial structure: cavalry or lucusts? Bank Italy Temi Discuss. (Working Paper) 35, 1–28.
Qin, X., Huang, G., Shen, H., and Fu, M. (2020). COVID-19 pandemic and firm-level cash holding—moderating effect of goodwill and goodwill impairment. Emerg. Mark. Finance Trade 56, 2243–2258. doi:10.1080/1540496X.2020.1785864
Ren, G., Mo, Y., Liu, L., Zheng, M., and Shen, L. (2022). Equity pledge of controlling shareholders, property right structure and enterprise innovation efficiency: evidence from Chinese firms. Econ. Res.-Ekon. Istra. 36, 1–21. doi:10.1080/1331677X.2022.2052331
Ren, S., Wei, W., Sun, H., Xu, Q., Hu, Y., and Chen, X. (2020). Can mandatory environmental information disclosure achieve a win-win for a firm’s environmental and economic performance? J. Clean. Prod. 250, 119530. doi:10.1016/j.jclepro.2019.119530
Rosenbaum, P. R., and Runim, D. B. (1983). The central role of the propensity score in observational studies for causal effects. Biometrika 70, 41–55. doi:10.1093/biomet/70.1.41
Saeidi, S. P., Sofian, S., Saeidi, P., Saeidi, S. P., and Saaeidi, S. A. (2015). How does corporate social responsibility contribute to firm financial performance? The mediating role of competitive advantage, reputation, and customer satisfaction. J. Bus. Res. 68, 341–350. doi:10.1016/j.jbusres.2014.06.024
Stuart, E. A. (2010). Matching methods for causal inference: A review and a look forward. Stat. Sci. 25, 1–21. doi:10.1214/09-STS313
Sun, J., Wang, F., Yin, H., and Zhang, B. (2019). Money talks: The environmental impact of China’s green credit policy. J. Policy Anal. Manage. 38, 653–680. doi:10.1002/pam.22137
Tan, X., Yan, Y., and Dong, Y. (2022). Peer effect in green credit induced green innovation: An empirical study from China’s Green Credit Guidelines. Resour. Policy 76, 102619. doi:10.1016/j.resourpol.2022.102619
Wang, E., Liu, X., Wu, J., and Cai, D. (2019). Green credit, debt maturity, and corporate investment—evidence from China. Sustainability 11, 583. doi:10.3390/su11030583
Wang, G., He, Q., Xia, B., Meng, X., and Wu, P. (2018). Impact of institutional pressures on organizational citizenship behaviors for the environment: Evidence from megaprojects. J. Manage. Eng. 34, 1–11. doi:10.1061/(ASCE)ME.1943-5479.0000628
Wang, G., Yu, G., and Shen, X. (2021). The effect of online environmental news on green industry stocks: The mediating role of investor sentiment. Phys. A Stat. Mech. its Appl. 573, 125979. doi:10.1016/j.physa.2021.125979
Wang, G., Zeng, S., Xia, B., Wu, G., and Xia, D. (2022). Influence of financial conditions on the environmental information disclosure of construction firms. J. Manage. Eng. 38, 04021078. doi:10.1061/(asce)me.1943-5479.0000982
Wang, H., Chen, Z., Wu, X., and Nie, X. (2019). Can a carbon trading system promote the transformation of a low-carbon economy under the framework of the porter hypothesis? —empirical analysis based on the PSM-DID method. Energy Policy 129, 930–938. doi:10.1016/j.enpol.2019.03.007
Wang, Z., Wu, M., Li, S., and Wang, C. (2021). The effect evaluation of China’s energy-consuming right trading policy: Empirical analysis based on PSM-DID. Sustainability 13, 11612. doi:10.3390/su132111612
Withers, M. C., and Li, C. H. (2021). “Natural experiments in business research methods,” in Oxford research encyclopedia of business and management (Oxford: Oxford University Press). doi:10.1093/acrefore/9780190224851.013.302
Wu, S., Wu, L., and Zhao, X. (2022). Impact of the green credit policy on external financing, economic growth and energy consumption of the manufacturing industry. Chin. J. Popul. Resour. Environ. 20, 59–68. doi:10.1016/j.cjpre.2022.03.007
Xi, B., Wang, Y., and Yang, M. (2022). Green credit, green reputation, and corporate financial performance: evidence from China. Environ. Sci. Pollut. Res. 29, 2401–2419. doi:10.1007/s11356-021-15646-z
Yao, S., Pan, Y., Sensoy, A., Uddin, G. S., and Cheng, F. (2021). Green credit policy and firm performance: What we learn from China. Energy Econ. 101, 105415. doi:10.1016/j.eneco.2021.105415
Yarrow, G., King, M., Mairesse, J., and Melitz, J. (1986). Privatization in theory and practice. Econ. Policy 1, 323. doi:10.2307/1344560
Yazdanfar, D., and Öhman, P. (2015). Debt financing and firm performance: an empirical study based on Swedish data. J. Risk Financ. 16, 102–118. doi:10.1108/JRF-06-2014-0085
Yu, W., Ramanathan, R., and Nath, P. (2017). Environmental pressures and performance: An analysis of the roles of environmental innovation strategy and marketing capability. Technol. Forecast. Soc. Change 117, 160–169. doi:10.1016/j.techfore.2016.12.005
Zhang, B., Yang, Y., and Bi, J. (2011). Tracking the implementation of green credit policy in China: Top-down perspective and bottom-up reform. J. Environ. Manage. 92, 1321–1327. doi:10.1016/j.jenvman.2010.12.019
Zhang, D., Mohsin, M., Rasheed, A. K., Chang, Y., and Taghizadeh-Hesary, F. (2021). Public spending and green economic growth in BRI region: Mediating role of green finance. Energy Policy 153, 112256. doi:10.1016/j.enpol.2021.112256
Zhang, L., Wu, D., and Mao, W. (2015). “Analysis of sustainable development ability for the listed SMEs in Guangdong province,” in 2015 12th International Conference on Service Systems and Service Management (ICSSSM), Guangzhou, China, 22-24 June 2015, 1. doi:10.1109/ICSSSM.2015.7170259
Zhang, Y.-J., and Wang, W. (2021). How does China’s carbon emissions trading (CET) policy affect the investment of CET-covered enterprises? Energy Econ. 98, 105224. doi:10.1016/j.eneco.2021.105224
Zheng, H., Ma, W., Wang, F., and Li, G. (2021). Does internet use improve technical efficiency of banana production in China? Evidence from a selectivity-corrected analysis. Food Policy 102, 102044. doi:10.1016/j.foodpol.2021.102044
Keywords: green credit policy, construction energy-saving enterprises, financial performance, PSM-DID, meditating effect
Citation: Li X and Lu Y (2022) How green credit policy shapes financial performance: Evidence from Chinese listed construction energy-saving enterprise. Front. Environ. Sci. 10:1004247. doi: 10.3389/fenvs.2022.1004247
Received: 27 July 2022; Accepted: 19 August 2022;
Published: 15 September 2022.
Edited by:
Hongyun Si, Shandong University of Finance and Economics, ChinaReviewed by:
Junwei Zheng, Kunming University of Science and Technology, ChinaZezhou Wu, Shenzhen University, China
Copyright © 2022 Li and Lu. This is an open-access article distributed under the terms of the Creative Commons Attribution License (CC BY). The use, distribution or reproduction in other forums is permitted, provided the original author(s) and the copyright owner(s) are credited and that the original publication in this journal is cited, in accordance with accepted academic practice. No use, distribution or reproduction is permitted which does not comply with these terms.
*Correspondence: Yiling Lu, MjIxMDA3MkB0b25namkuZWR1LmNu