- 1SILC Business School, Shanghai University, Shanghai, China
- 2School of Finance, Anhui University of Statistics and Applied Mathematics, Bengbu, Anhui, China
Green finance is an important means to promote industrial pollution reduction. This paper uses the entropy method and the TOPSIS model to calculate the green finance development index and industrial pollution index of 30 provinces in China from 2011 to 2019, and further uses the panel regression model and SDM model to test the impact of green finance on industrial pollution. The study found that there is a significant positive spatial correlation between green finance and industrial pollution; At the same time, green finance generally inhibits industrial pollution. The effect decomposition results show that the former has a significant negative direct effect and spatial spillover effect on the latter.
Introduction
The Chinese government work report for 2021 clearly proposes to advance carbon peaking and carbon neutrality in an orderly manner. Finance is an important force to achieve the goal of “dual carbon” (Niu et al., 2020a). As an important part of the modern financial system, green finance plays a key role in serving the green transition and helping to achieve carbon peaking and carbon neutrality.
During the National People’s Congress and the National People’s Congress, a number of officials from the National People’s Congress of the financial system said that green finance must make efforts in the next step in setting standards, incentive policies, product innovation and other aspects out of the “acceleration” of development run out (Fu and Peng, 2020). By the end of 2021, the balance of green foreign currency loans in China was 15.9 trillion yuan, up 33% year on year, ranking first in the world in terms of stock size. In 2021, domestic green bond issuance will exceed 600 billion yuan, up 180% year on year, and the balance will reach 1.1 trillion yuan.
How to evaluate the development performance of green finance has been a topic of in-depth research by scholars in recent years. The current literature mainly assumes that green finance represents a new type of environmental regulation that promotes technological innovation in highly polluting industries through financial means and eliminates the negative externalities of green technology (Zhang, Qian).
On the one hand, green finance lowers the threshold and risk of technological innovation and promotes the green transformation of polluting companies (Xie and Zhang, 2021); On the other hand, compared with traditional finance, green finance, by raising interest rates and strengthening supervision (Scholtens, 2002), restricts the supply of funds for highly polluting and energy-intensive industries, increases their production costs, forces industry transformation and upgrading, and promotes emission reductions from polluting companies (Lei and Wang, 2020). Overall, the academic community mainly believes that the direct impact of green finance is reflected in the reduction of industrial pollutant emissions and has launched a number of studies on the subject (Liu and Nie, 2007; Du, 2014; Li, 2017; Liu and He, 2021).
Green finance is a powerful weapon to promote technological innovation and reduce industrial pollution (Muganyi et al., 2021). With the continuous development of green finance, more and more scholars carry out relevant research. The existing literature mainly believes that green finance is a new environmental policy, which can reduce the negative externalities of green technology by stimulating technological innovation in polluting industries. On the one hand, green finance supports polluting enterprises to carry out technological innovation through innovative green financial products, reducing the threshold and risk of technological innovation; On the other hand, compared with traditional financial financing means, green finance limits the capital supply to highly polluting and energy intensive enterprises, and forces polluting enterprises to innovate, transform and reduce emissions by raising interest rates and strengthening supervision (Sun et al., 2021).
Recent research has paid less attention to the spatial context of green finance development in China. In this context, this paper takes the impact of green finance on industrial pollution as a starting point to assess China’s green finance development performance, conducts empirical research from a spatial perspective, and further examines the differences in green finance development performance in different provinces and regions.
The possible marginal contributions of this paper are: First, use the TOPSIS model modified by the entropy weight method to construct China’s green finance development index and industrial pollution emission index, it not only takes into account the limitations of single index measurement, but also comprehensively evaluates the weights of each index, avoiding the deviation caused by factors, making the index measurement more comprehensive and accurate. This paper assesses the impact of green finance development on regional industrial pollutant emissions and provides a useful reference for China’s economic green transformation, high-quality development, and energy conservation and emission reduction. Second, the development of green finance and industrial pollution has some spatial correlation. This paper further explores the correlation between green finance and industrial pollution from a space perspective. Finally, this paper further studies the heterogeneity of location conditions and pollutants between green finance and industrial pollution, providing a reference basis for exploring the realization path of China’s green finance high-quality development, and also provides decision support for effectively achieving China’s carbon peak and carbon neutrality goals.
Review of the literature
The impact of green finance on industrial pollutant emissions is multidimensional and complex (Muganyi et al., 2021). To really play the role of green finance in the economy, green finance needs to be clear about what green is (Sun et al., 2021). Only by identifying green can there be real green finance, and finance can become more effective and efficient, and better serve the real economy and green development.
From a realistic point of view, the current status of green development or green economy should include at least the following aspects. One is to support a green economy that uses clean and renewable energy (Zhang et al., 2009); The second is to support the structural change towards a green economy that reduces and reduces the use of fossil energy (Zeng, 2019); The third is to support a green economy that meets energy conservation and emission reduction standards and meets policy requirements (Moreno-Brid et al., 2016); Fourth, support a green economy with improved energy efficiency (Altenburg, 2006); The fifth is to support the use of big data or digital technology to implement green economy for energy saving and emission reduction (Dong and Wei, 1997); Sixth, the circular economy increases the lifespan of buildings, products and assets (Richardson, 2014).
There are four pillars to green finance: standards, disclosures, products and incentives (Yi et al., 2014). The so-called standard of green finance represents their definition standard. There must be a number of explanations as to what is green. So far there have been at least three catalogs of criteria for defining green finance: on the one hand the criteria for defining green credit, on the other hand the criteria for defining green bonds, namely the Green Bond Catalogue, and thirdly the Green Industry Catalogue. These three sets of standards are organically combined and essentially the same. In addition, there are disclosure requirements for green projects: while initially it was mainly the disclosure of environmental benefits, the disclosure content is now being successively expanded (Huang and Fan, 2010).
Green finance realizes its green concept and value mainly through green financial policies such as green credit, green securities, green insurance and carbon trading. Green credit directs cash flow to green pollution control industries and green manufacturing projects, severely restricts lending to high-energy-using, high-polluting companies, and penalizes polluting companies by suspending loans, reducing loan levels, and reclaiming previous loans (Tapio, 2005). Green bonds invest the funds raised in green industries, restrict financing levels for polluting companies, and impose severe financing penalties on polluting companies (Wang et al., 2013). Green insurance helps disperse the operational risks of green environmental protection industries and manufacturing enterprises, improve the level of environmental risk management, and achieve sustainable development (Wang and Wang, 2021). Emissions trading encourages companies to undertake independent emission reduction upgrades by formulating quantitative emission caps and rationally allocating indicators (Han et al., 2016).
Green finance promotes the reduction of industrial pollution mainly in the following three aspects: First, green finance drives capital orientation, effectively directs the transfer of funds in the financial market to green industries, increases the financing costs of enterprises and projects with high energy consumption, high pollution and overcapacity, and promotes the green transformation of the industry (Li and Jiang, 2010). Second, green finance promotes industrial integration, promotes the flow of resources into green industries, improves market competitiveness, transforms enterprises from labor-intensive to capital- and technology-intensive enterprises, optimizes resource structure, and promotes the integration and optimization of green environmental protection industries. Third, improve the level of risk prevention, green finance directs funds flowing into high-risk green high-tech industries and promotes green innovation of other traditional enterprises.
In summary, green finance increases the restrictions on polluting enterprises, effectively inhibits the development of polluting enterprises, and thereby reduces the emission of pollutants. Based on the above analysis, this paper takes the impact of green finance on industrial pollutant emissions as the entry point of performance evaluation to examine the development performance of China’s green finance.
Research design
Construction of evaluation system
How to quantify the development of green finance in different regions is a problem that needs to be solved. Although the green finance development system is still not perfect, many scholars have also done some research into formulating its rating system. Based on the definition of green finance and the importance of the policy, the existing literature is used for reference (Cao, 2004; Zou, 2017; Niu et al., 2020b), and the evaluation system is scientific, reasonable and measurable. This paper constructs green finance development level indicators from five dimensions: green credit, green securities, green investment, carbon finance and green finance attention. The specific scoring system is presented in Table 1.
In order to fully consider the impact of green finance development on industrial pollutant emissions, a comprehensive index of industrial pollutant emissions in different regions was created using a variety of pollutant input data. In order to measure the industrial pollution situation in different provinces as comprehensively and scientifically as possible, referring to the main industrial pollution data published in my country and the related literature review (Yu and Zhang, 2021), using five categories of indicators that can reflect industrial pollutant emissions are selected for a comprehensive assessment. The specific index construction system is presented in Table 2.
Variable description
The core explanatory variables of this paper are the green finance development index (
Level of regional economic development (
Urbanization rate (
Foreign direct investment (
Degree of openness (
Data source and processing
This paper takes 30 provinces in China (excluding Hainan, Hong Kong, Macau and Taiwan) as the research subject and takes 2011–2019 as the research period. The specific sources of relevant data are as follows: The data of the balance of green credit in each province comes from the Bank of China Social Responsibility Report, and the data of the green securities comes from the RESSET database, and the data of green investment comes from the National Bureau of Statistics, and the data of carbon finance comes from the China Clean Development Mechanism Network, and the data of the green finance attention comes from Baidu Index.
The data of relevant indicators for the discharge of industrial pollutants come from the China Environmental Yearbook; The relevant data for the four control variables are all from the official National Bureau of Statistics website.
For missing data values in individual provinces and individual years, use cubic spline interpolation to fill in the data; To objectively determine the weight between indicators, the entropy weighting method is used to weight each indicator; To accurately reflect the gap between the overall level of green finance and industrial pollution in each province, the TOPSIS method was used to solve the comprehensive assessment index.
Descriptive statistics
The descriptive statistical results of the Green Finance Development Index, Industrial Pollution Emission Index and four control variables used in this paper are presented in Table 3.
Empirical analysis
Comprehensive evaluation of complex systems
According to the comprehensive scoring system and modeling procedure set out above, the comprehensive green finance and industrial pollutant emissions score can be obtained in each region. In order to more intuitively analyze the trend of China’s total green finance and industrial pollutant emissions, the green finance and industrial pollution values from 2011 to 2019 were plotted on a trend chart, and the average value of all provinces was used to measure the overall level.
As shown in Figure 1, the development level of green finance in China generally shows an increasing trend year by year, and the level of industrial pollutant emissions generally shows a decreasing trend year by year. This is because China has paid more and more attention to sustainable development in the past 10 years, and has continuously issued relevant laws and policies to promote the development of green finance, improve the green finance development system, optimize ecological construction, and achieve simultaneous improvement in economic and environmental benefits.
Judging by the range of changes, the year-on-year increase in green finance is relatively small, but the growth trend is relatively stable; Emissions of pollutants from industry decreased significantly in the 3 years from 2015 to 2017, and the decreases in other years were also smaller. This is mainly due to the official implementation of the Environmental Protection Law of the People’s Republic of China in 2015, which promotes the continuous resolution of environmental and environmental problems. Judging by the trend changes of the two indices, green finance has an obvious restraining effect on the total industrial pollution emissions, indicating that green finance has made a significant contribution to the process of industrial pollution control in my country.
It is worth noting that after 2017, the total amount of industrial pollutant emissions fell sharply from the original level. In the current situation, where green and low-carbon technologies have not made breakthrough results, the impact of industrial environmental protection measures is not obvious.
Model settings
To prevent multicollinearity of the model, correlation coefficients between variables were calculated, and correlation coefficients between explanatory variables and control variables were both low. The VIF statistic of each variable does not exceed 10 and there is no serious multicollinearity problem. The stationarity test of the variables is performed and it is taken into account that the variables are not all stationary; The co-integration test takes into account that there is a co-integration relationship between variables and avoids false regression errors. The results of the F-test, LM-test and Hausman’s test showed that the SDM model was optimal (Zhang and Qian, 2006).
This paper assesses the development performance of China’s green finance from a spatial perspective and selects a spatial econometric model for research. In this paper, a binary adjacency space weight matrix
Using Stata software to estimate the two key variables, the Green Finance Development Index and the Industrial Pollution Index, to perform a global spatial autocorrelation analysis of the two. The test shows that there is a significant positive spatial correlation between the Industrial Pollution Emission Index and the Green Finance Development Index, including low-low correlation and high-high correlation, and also negative spatial correlation in some regions. Figure 2 shows the local correlation of the total sample in 2019.
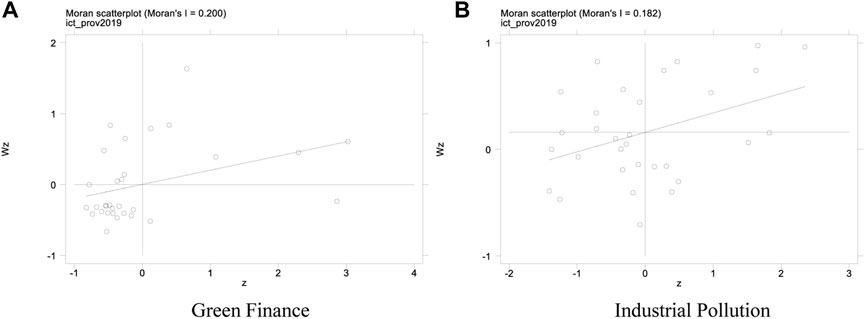
FIGURE 2. Local map of green finance and industrial pollution in 2019 (A) Green Finance (B) Industrial Pollution.
To demonstrate the spatial spillover effect, this paper selects the SDM model for empirical analysis:
In the formula, the subscripts
Benchmark regression
Table 4 shows the regression results using common panel model and SDM. From the comparison, it can be seen that the direction and importance of the impact of green finance development on reducing industrial pollution has not changed, regardless of whether spatial correlation is taken into account or not. However, the general panel does not account for the spatial spillover effect, thus underestimating the impact of green finance on reducing industrial pollution and slightly overestimating the impact of the control variables. SDM’s estimation results show that the spatial autocorrelation coefficient is significantly positive, further confirming that there is a significant positive spatial autocorrelation between the development of green finance and industrial pollutant emissions.
Space measurement
To further explore the interactive information contained in the regression coefficients in the spatial econometric model, Table 5 provides the estimates of the mean direct effect, mean indirect effect, and mean total effect of the variables. It can be seen that the direct and indirect effects of green finance development on reducing industrial pollution are significant with coefficients of −0.571 and −0.117, respectively. That is, a 1% point increase in the development level of green finance in a given area will reduce the local industrial pollutant emission level by 0.571 percentage point and the industrial pollutant emission level of other adjacent areas by an average of 0.117 percentage points. The empirical results confirm the theoretical hypothesis.
In terms of the impact of the control variables, improving the level of economic development will significantly increase the level of industrial pollutant emissions in the region, but the impact on industrial pollutant emissions from other places is not significant; The urbanization process will significantly reduce the level of industrial pollutant emissions in the region, but will not have a significant impact on industrial pollutant emissions from other locations; Foreign direct investment will significantly reduce the level of industrial pollutant emissions in different regions, and the degree of openness to the outside world will significantly increase the level of industrial pollutant emissions in different regions.
Robustness test
Using the inverse distance matrix instead of the 0–1 matrix for the regression, most of the coefficients of the explanatory and control variables did not change significantly, so the empirical results in this paper can be considered robust (see Table 6).
Regional comparison
According to the division of administrative regions of China, the 30 provinces are divided into three regions: the eastern, central and western regions for regional comparison. The results are shown in Table 7. First, the development of green finance has a significant inhibiting effect on the level of local industrial pollution in each region, reflecting that the development of green finance has played an important role in reducing industrial pollution. Second, from strong to weak, the western, eastern and central regions should benefit from the overall benefits of green finance on industrial pollutant emissions. Third, green finance has a significant negative indirect effect on reducing industrial pollution in the eastern and central regions, while the indirect effect is not significant in the western region, possibly due to the intense industrial linkage in the western region.
Conclusion and suggestion
This paper conducts an empirical analysis of SDM based on the data from 30 provinces in China from 2011 to 2019.The empirical results confirm the theoretical hypothesis: the level of development of green finance in a region will significantly reduce the level of local industrial pollutant emissions and also have indirect effects on the outside world. The sub-regional empirical results support the conclusion of the entire sample, noting that the overall effect of industrial pollutant emissions affected by green finance ranges from strong to weak in the western, eastern and central regions. The research results of this paper provide some suggestions for the development of green finance in China and the high-quality transformation of the economy:
First, effectively integrate and unify top-level policy design, market innovation and local practice, so that the green finance industry can be comprehensively improved. As we improve policies related to the green finance industry and do a good job of shaping green finance at the highest level, we should also improve the green financial market system, encourage innovation of green financial tools, and strengthen cooperation between government and social capital. Conduct local pilot work on green finance to meet the need for green securities investment, improve market competitiveness and further promote industry development. To promote green finance effectively, local practices should also be strengthened and diversified green investors should be actively encouraged to get involved in the green finance industry.
Second, develop green finance according to local conditions. The development of green finance in different regions of China not only has similarities, but also certain spatial differences. In the development process of Green Finance, on the one hand, the green financial system that has been set up should be continuously improved and perfected. In real operation, it is also important to effectively link the experience gained from outside with the actual situation and finally to formulate a financial system that corresponds to China’s actual development. In addition, when developing green finance in the region, local governments should adapt to local conditions and choose the most suitable development path for their own positioning in order to reduce the problems existing in the development process.
Third, continue to develop innovative green financial products. The level of economic development, the degree of openness to the outside world, the rate of urbanization and foreign direct investment will also affect to some extent the intensity of the impact of green finance on reducing industrial pollution. In the empirical analysis, the performance of green finance in the western region is significant, and the effect in the more developed eastern region is slightly worse, indicating that improving China’s economic development level has not brought any environmental benefits. This may be because the current green financial products are single and cannot meet the needs of economic green transformation. Therefore, in the actual development process, innovation of green financial products should be strengthened, and green financial products should be continuously innovated and developed, focusing on supporting the research and development of green technologies and industrial ecological transformation projects.
Data availability statement
The original contributions presented in the study are included in the article/supplementary material, further inquiries can be directed to the corresponding author.
Author contributions
Conceptualization, LZ; methodology, ZM; software, LZ and ZM; validation, LZ; resources, ZM; data collection, ZM; writing—original draft preparation, LZ; writing—review and editing, LZ and ZM; supervision, LZ and ZM.
Funding
This work is funded by Anhui University of Finance and Economics Undergraduate Research and Innovation Fund Project (Grant No: XSKY22014ZD) and National Innovation and Entrepreneurship Training Program for College Student (Grant No: 202110378115).
Conflict of interest
The authors declare that the research was conducted in the absence of any commercial or financial relationships that could be construed as a potential conflict of interest.
Publisher’s note
All claims expressed in this article are solely those of the authors and do not necessarily represent those of their affiliated organizations, or those of the publisher, the editors and the reviewers. Any product that may be evaluated in this article, or claim that may be made by its manufacturer, is not guaranteed or endorsed by the publisher.
References
Altenburg, T. (2006). Governance patterns in value chains and their development impact. Eur. J. Dev. Res. 18 (4), 498–521. doi:10.1080/09578810601070795
Cao, X. (2004). Research on the relationship between economic development and environmental protection [J]. J. Soc. Sci. 77 (2), 5.
Dai, H. W., and Wang, Y. P. (2008). Analysis of the relationship between industrial transfer and regional industrial structure adjustment [J]. Contemp. Finance 16 (2), 6.
Dong, F., Tan, Q. M., Zhou, D. Q., and Li, X. H. (2010). The impact of technological progress, industrial structure and degree of opening to the outside world on China's energy consumption: Empirical evidence based on the two-step method of grey relational analysis-cointegration test [J]. China's Population. Resour. Environ. 20 (6), 6.
Dong, Y. W., and Wei, Y. J. (1997). Urbanization and environmental protection in China [J]. Environ. Her. 32 (6), 4.
Du, J. T. (2014). Financial asset structure adjustment, industrial structure upgrade and pollution emission control [J]. Econ. Geogr. 34 (11), 112–119.
Fu, Y. P., and Peng, Z. Q. (2020). Green finance development, R&D investment and regional economic growth: Empirical evidence based on the provincial panel threshold model [J]. Statistics Decision-Making 16 (21), 5.
Han, Y. H., Huang, L. X., and Wang, X. B. (2016). Has the industrial structure been optimized and upgraded to improve ecological efficiency? [J]. Res. Quantitative Econ. Technol. Econ. 33 (04), 40–59.
Huang, Q. B., and Fan, H. M. (2010). Foreign trade, economic growth and industrial structure upgrading——an empirical test based on the "four little dragons" of China, India and asia [J]. Int. Trade Issues 4 (2), 7.
Lei, H. Y., and Wang, X. X. (2020). Environmental pollution, green finance and high-quality economic development [J]. Statistics Decis. 36 (15), 18–22.
Li, H., Yuan, Y. C., and Wang, N. (2019). Evaluation of the coordinated development of regional green finance and ecological environment [J]. Statistics Decis. 35 (08), 161–164.
Li, R. L., and Jiang, Q. (2010). The pilot effect test of my country's foreign trade structure on industrial structure—based on manufacturing data analysis [J]. Int. Trade Issues 6 (8), 10.
Li, X. (2017). Analysis of industrial pollution intensity changes and influencing factors in China's provinces [J]. Statistics Decis. 54 (19), 132–135.
Liu, H. K., and He, C. (2021). The mechanism and test of green finance to promote the high-quality development of urban economy: Empirical evidence from 272 prefecture-level cities in China [J]. Invest. Res. 40 (07), 37–52.
Liu, X., and Nie, C. G. (2007). China's industrial economic development and changes in industrial pollution emissions [J]. Statistics Decis. 24 (04), 65–67.
Moreno-Brid, J. C., Garry, S., and Krozer, A. (2016). All. Minimum wages and inequality in Mexico: A Latin AMericanPerspective [J]. Rev. De. Econ. 43, 113–129.
Muganyi, T., Linnan, Y., and Sun, H. P. (2021). Green finance, fintech and environmental protection: Evidence from China. Environ. Sci. Ecotechnology 7, 100107. doi:10.1016/j.ese.2021.100107
Niu, H. P., Zhang, X. Y., and Zhang, P. D. (2020). Institutional changes and effect evaluation of my country's green financial policies: Taking the empirical study of green credit as an example [J]. Manag. Rev. 32 (8), 10.
Niu, H. P., Zhang, X. Y., et al. (2020). Institutional changes and effect evaluation of my country's green financial policies: Taking the empirical study of green credit as an example [J]. Manag. Rev. 32 (08), 3–12.
Pan, Y., and Liu, H. H. (2010). An empirical study on the relationship between China's foreign direct investment and industrial structure upgrading [J]. Statistics Decis. 25 (2), 3.
Richardson, R. (2014). Environmental finance: Environmental compliance can Be profitable. Nat. Gas. Elec. 31 (3), 9–12. doi:10.1002/gas.21787
Sun, H., Edziah, B. K., Sun, C., and Kporsu, A. K. (2021). Institutional quality and its spatial spillover effects on energy efficiency. Socio-Economic Plan. Sci. 23, 101023. doi:10.1016/j.seps.2021.101023
Tapio, P. (2005). Towards a theory of decoupling: Degrees of decoupling in the EU and the case of road traffic in Finland between 1970 and 2001. Transp. Policy 12 (2), 137–151. doi:10.1016/j.tranpol.2005.01.001
Wang, W., Liu, R., Zhang, M., and Li, H. (2013). Decomposing the decoupling of energy-related CO2 emissions and economic growth in Jiangsu Province. Energy Sustain. Dev. 17 (1), 62–71. doi:10.1016/j.esd.2012.11.007
Wang, X., and Wang, Y. (2021). Research on green credit policy to promote green innovation [J]. Manag. World 37 (06), 173–188+11.
Xie, Q. X., and Zhang, Y. (2021). Green credit policy, supporting hand and enterprise innovation and transformation [J]. Sci. Res. Manag. 42 (01), 124–134.
Xu, Y. G. (2018). The market logic of green finance development [J]. China Sci. Technol. Forum 264 (04), 9–10.
Yi, J. P., Jiang, C., and Peng, Y. (2014). Research on the current situation and countermeasures of green finance development in my country [J]. Spec. Econ. Zone 18 (5), 3.
Yu, P., and Zhang, J. P. (2021). Coupling and coordination evaluation of regional green finance and high-quality development [J. ]. Statistics Decision-Making 37 (24), 142–146.
Zeng, W. (2019). Analysis of green finance helping my country's high-quality economic development. J. Mark. 11 (38).
Zhang, J., Chen, S. Y., and Gary, H. Y. (2009). Structural reform and China's industrial growth [J]. Econ. Res. 34 (7), 17.
Zhang, M. (2006). A preliminary study on foreign investment and environmental protection in my country [J]. Ecol. Econ. 19 (3), 5.
Zhang, Y., and Qian, S. T. (2006). Green finance, environmental technology progress bias and industrial structure cleaning [J]. Vienna, Austria: Scientific research management, 1–18.
Keywords: green finance, industrial pollution, entropy method, space measurement, spatial spillover effect
Citation: Zhang L and Mei Z (2022) Green finance and industrial pollution: Empirical research based on spatial perspective. Front. Environ. Sci. 10:1003327. doi: 10.3389/fenvs.2022.1003327
Received: 26 July 2022; Accepted: 26 October 2022;
Published: 08 December 2022.
Edited by:
Asif Razzaq, Dalian University of Technology, ChinaCopyright © 2022 Zhang and Mei. This is an open-access article distributed under the terms of the Creative Commons Attribution License (CC BY). The use, distribution or reproduction in other forums is permitted, provided the original author(s) and the copyright owner(s) are credited and that the original publication in this journal is cited, in accordance with accepted academic practice. No use, distribution or reproduction is permitted which does not comply with these terms.
*Correspondence: Lechao Zhang, WmhhbmdsY2gyMDAzQDE2My5jb20=