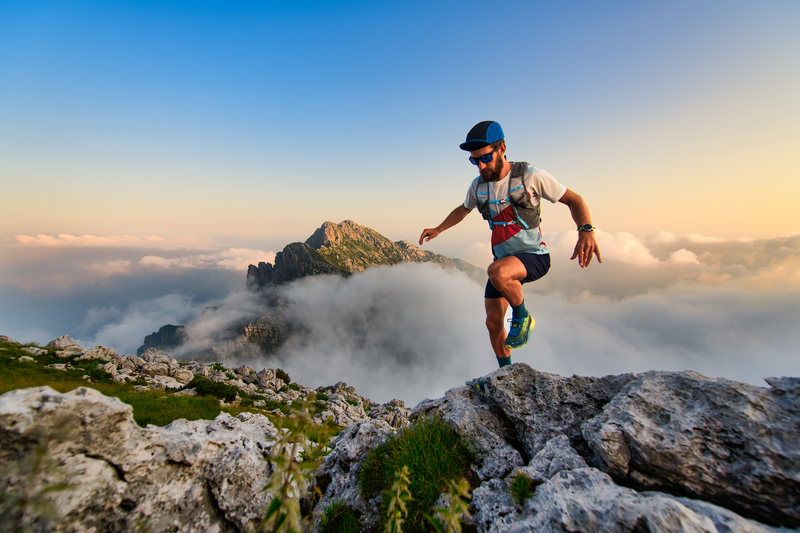
95% of researchers rate our articles as excellent or good
Learn more about the work of our research integrity team to safeguard the quality of each article we publish.
Find out more
ORIGINAL RESEARCH article
Front. Environ. Sci. , 13 December 2022
Sec. Land Use Dynamics
Volume 10 - 2022 | https://doi.org/10.3389/fenvs.2022.1001274
This article is part of the Research Topic Grassland Agriculture and Ecology Research with Multisource Remote Sensing View all 6 articles
Family farms, considered the most desirable form of Chinese agriculture, play a pivotal role in promoting rural revitalization and agricultural modernization. The purpose of this study was to summarize the spatiotemporal evolution characteristics and influencing factors of family farms to better promote the development of modern agriculture. Using provincial demonstration family farms in the urban agglomeration in the middle reaches of the Yangtze River (MYR-UA) as the research object, this study applied the nearest neighbor index, kernel density analysis, multiscale spatial clustering analysis (Ripley’s K-function), and geographically weighted regression (GWR) model to reveal the spatiotemporal dynamic evolution and influencing factors of family farms. The results indicate that: 1) from 2013 to 2021, family farms exhibited annual increases, and their development stages could be divided into rapid, stable, and slow growth periods. 2) The spatial agglomeration pattern of family farms was significant, and the intercepted points at different time periods show the distribution characteristics of the entire dispersion and local concentration. The spatial evolution characteristics of different types of family farms are nearly consistent with those of the overall family farms. 3) The overall family farms and various types of family farms show a scale effect, which first strengthens and then weakens with the change in geographical distance. 4) The spatial pattern of family farms in MYR-UA is affected by both natural and social factors, of which, social factors had the greatest influence. Finally, based on the findings of the study, policy recommendations for promoting the high-quality development of family farms are proposed.
As a new type of agricultural production and management entity, family farms undertake the vital tasks of improving agricultural production efficiency, realizing agricultural modernization, and promoting sustainable agricultural development (Wang et al., 2021). Family farms can effectively circumvent the weaknesses of traditional small-scale farming methods and have been developing for hundreds of years in developed western countries (Lowder et al., 2016). Family farms appeared in China at the end of the 20th century. Before this, there were no agricultural management models in traditional China [ (Chen et al., 2022a), (Liang et al., 2016)]. The Chinese government has focused on the cultivation and development of family farms as an agricultural management model (Gong et al., 2019).
According to statistical data from the Ministry of Agriculture and Rural Affairs of the People’s Republic of China, there were 139,000 family farms in China in 2014. In 2020, this number reached 853,000, showing a trend of continuous growth. Family farms have become a crucial force in the development of modern agriculture (Gao et al., 2017). Although family farms in various parts of the country have developed rapidly, there have been some practical problems, such as weak marketing ability, lack of labor, a single management model, and poor management [ (Wilson et al., 2010; Graeub et al., 2016; Ji et al., 2016; Gao et al., 2019)]. To solve these problems, the Chinese government issued the “High-quality Development Plan for New Agricultural Business Entities and Service Entities,” emphasizing the need to accelerate the development of family farms, actively create demonstration family farms, and ultimately develop high-quality family farms (Qian and Li, 2020a). Therefore, in this context, exploring the spatiotemporal evolution characteristics and influencing factors of family farms is of great value and practical significance to rationally plan the layout of family farms and promote the high-quality development of family farms.
Family farms first appeared in European countries and North America. The United States government defines the family farm as one in which the family owns the property rights of the farm, and family members are the main labor force and bear the primary responsibility for the operation and management of the farm (Reinhardt and Barlett, 2008). According to the Family Farm Law of Russia, family farms are independent production and operation entities with legal personal rights (Shubin, 2011). With the rapid development of family farms worldwide, measuring and improving their efficiency has become popular a research topic for many scholars. Madau used the SFA and DEA models to measure the efficiency of Italian citrus farms. It was found that the technical efficiencies estimated using the two models were essentially the same, but the scale efficiency varied significantly (Madau, 2015). Chen and Meng adopted a DEA model to measure the efficiency of Wuhan and Langxi family farms and applied the Tobit model to explore the factors affecting efficiency (Chen et al., 2022b). Qian and Li measured the efficiency of different types of family farms in Songjiang, Shanghai, China. They found significant differences in the efficiencies of different types of family farms. In recent years, scholars have gradually realized the important relationship between geographical elements and family farms and have begun to explore the spatial layout of family farms from the perspective of geography [ (Xing et al., 2021), (Ilbery and Maye, 2010)]. For example, Xiong and Zhang used Jiangjin District of Chongqing as a case study and comprehensively analyzed the spatial distribution pattern of family farms using the nearest neighbor index, kernel density analysis, and standard deviation ellipse (Xing et al., 2021). Ilbery and Maye utilized ArcGIS tools to analyze the spatial agglomeration characteristics and distribution rules of family farms in England and Wales (Ilbery and Maye, 2010). These studies have successfully introduced geographical research methods into the study of family farms.
Many studies have also considered factors that influence the formation of family farms. Based on existing research, scholars have mostly applied qualitative descriptions, spatial overlay analyses, and traditional regression models to explore the reasons for the formation of family farms (Huber et al., 2015; Dumas et al., 2016; Julia and Elena, 2016; Zhang et al., 2022). The results indicated that the formation of family farms is influenced by both natural and social factors. Natural factors include precipitation, temperature, altitude, and the soil environment, while social factors include the rural labor force, traffic accessibility, economic development level, relevant government policies, degree of marketization, and agricultural infrastructure. There is a complex network of relationships among the variables, and their interactions promote the regional formation and development of family farms.
The above analysis suggests that the research results in this field have gradually been enriched, but many issues still need to be addressed and improved. These studies mostly examined the efficiency of family farms from the perspectives of management and economics [(Burton and Walford, 2005), (Fox et al., 2021)]. Few studies have focused on the dynamic evolutionary patterns and influencing factors of family farms from the perspective of spatiotemporal heterogeneity. Second, from the perspective of influencing factors, previous studies mostly used qualitative descriptions and traditional linear regression methods to analyze the causes of the formation of family farms [(Philip et al., 2018), (Baležentis et al., 2014)]. The results obtained by these methods could not reflect the spatial heterogeneity of the influencing factors and thus could not provide specific suggestions for promoting the development of family farms. Third, from the perspective of case innovation, the existing research focused more on a single city or region [(Marchant Santiago et al., 2021), (Carte et al., 2010)] but paid less attention to the scale of urban agglomeration. There is also a lack of comparative research between the different regions.
The urban agglomeration in the middle reaches of the Yangtze River (MYR-UA) has a long history of agricultural development and is a key innovation base for modern agricultural industry in China (Lv et al., 2010). In 2015, the development plan for the MYR-UA indicated that it is necessary to expand modern agricultural bases, actively cultivate new agricultural business entities, and develop efficient modern agriculture. The implementation of this plan has provided great opportunities for the development of family farms in the MYR-UA. Accordingly, the rational planning and layout of family farms is paramount for promoting agricultural modernization and rural revitalization in the MYR-UA. The purpose of this study is to explore the spatiotemporal evolution characteristics and influencing factors of family farms to provide a scientific basis for the high-quality development of family farms. Specifically, we 1) present the spatiotemporal evolution characteristics of family farms overall and those of different types, 2) identify the influencing factors and spatial heterogeneity of family farms, and 3) provide specific suggestions for the rational layout and high-quality development of family farms.
The main innovations of this study can be described as follows. First, this study combines traditional and spatial statistical methods to systematically explore the temporal evolution, spatial agglomeration, spatial density distribution, and multiscale spatial characteristics of family farms in the MYR-UA. Second, we classify the family farms in the MYR-UA and display the spatial distribution patterns of the different types of family farms. Third, we apply the geographically weighted regression (GWR) model to determine the spatial heterogeneity and distribution patterns of the influencing factors of family farms at the grid scale and refine these factors.
The MYR-UA is a critical part of the Yangtze River Economic Belt, and it mainly comprises the Wuhan Metropolitan Area (WMA), Chang–Zhu–Tan Urban Agglomeration (CZT-UA), and Poyang Lake City Group (PLCG). The MYR-UA has been a rich production area for grain crops since ancient times and has superior conditions for agricultural production. The region has the most abundant agricultural resources and the most active agricultural trade in China (Wang et al., 2020). Moreover, the MYR-UA is a flat area predominantly composed of alluvial plains. This region has a subtropical monsoon climate, indicating that it is warm and rainy. It has many rivers that provide abundant water resources to the area. Therefore, the establishment of family farms has inherent natural conditions. Furthermore, the western region of the MYR-UA is connected to the Chengdu–Chongqing economic zone, the eastern region is connected to the Wanjiang Economic Belt and the Yangtze River Delta, and the southern region is near the Pearl River Delta economic zone, which means that the region has a broad agricultural product market. Finally, the MYR-UA is located at the intersection of the vertical axis of the Beijing Harbin–Beijing Guangzhou Corridor and the horizontal axis along the Yangtze River Corridor, providing extremely convenient transport conditions. Therefore, the MYR-UA is a suitable representative area to use as a case study for practical significance. The study area is illustrated in Figure 1.
Referring to previous studies combined with the actual development of family farms in the MYR-UA, we divided the family farms into three types based on their business scope: breeding, planting, and comprehensive family farms [(Qian and Li, 2020a), (Xing et al., 2021)]. Planting family farms mostly grow food crops, vegetables, and fruits. The planting types are distinct for each farm, with some maintaining a relatively simple planting structure, whereas others have a wide variety of plants. Breeding family farms are primarily engaged in poultry farming and aquaculture. Most breeding family farms in the MYR-UA raise pigs, chickens, ducks, crayfish, fish, and pigeons. Comprehensive family farms are those that combine planting and breeding, with the business scope including accommodation, catering, picking, fishing, and recreation.
A list of 1,998 provincial demonstration family farms in the MYR-UA was obtained from the official websites of the Jiangxi Provincial People’s Government (https://jiangxi.gov.cn/, accessed on 26 February 2022), Hubei Provincial Department of Rural Agriculture (http://nyt.hubei.gov.cn/, accessed on 26 February 2022), and Hunan Provincial Department of Rural Agriculture (https://agri.hunan.gov.cn/, accessed on 26 February 2022). In 2013, the No.1 Central Document explicitly proposed to encourage and develop family farms for the first time. Therefore, 2013 was selected as the starting year for this study. Because the list of provincial model family farms in 2022 was not yet available at the time of this study, 2021 was used as the deadline year. Among the listed family farms, there were 713 planting farms, 337 breeding farms, and 948 comprehensive farms. The longitude and latitude coordinates of each family farm were queried using Baidu Maps, and the data were verified as reliable through field research and random telephone interviews. The DEM30m data of the study area was from the Data Center of Resource and Environment Science, Chinese Academy of Sciences (https://www.resdc.cn/, accessed on 10 March 2022), which was used to extract the slope values of the surrounding areas of the family farms. The land-use data were obtained from the Data Center of Resource and Environmental Sciences, Chinese Academy of Sciences (https://www.resdc.cn/, accessed on 10 March 2022), which was applied to extract the cultivated land area around the family farms. China’s 1 km grid population distribution data and GDP data were obtained from the National Earth System Science Data Center (http://www.geodata.cn/, accessed on 10 March 2022) [(Fu et al., 2014; Huang et al., 2014)]. These data were used to extract the population density and GDP data of the area around the family farms. China’s annual average temperature and precipitation data were derived from the China Meteorological Background Dataset of the Center for Resource and Environmental Science and Data, Chinese Academy of Sciences (https://www.resdc.cn/, accessed on 10 March 2022) (Xu and Zhang, 2017). Using remote sensing image data, this study digitally acquired the main traffic road network and water area of the MYR-UA. These data were used to extract the road and water network densities of the area around the family farms.
The average nearest neighbor index (ANN) indicates the mutual proximity of point elements in a geographic space. Its advantage is that it can accurately reflect the degree of spatial clustering of point data (Chen et al., 2022c). In this study, an ANN was used to determine the distribution types of family farms in the MYR-UA. The index value was calculated as follows:
where
The Z-score is calculated as follows:
Where Z-value is the standard score to determine the degree of dispersion of the family farm in space.
Kernel density analysis was used to calculate the unit density of the measured values of the point and line elements in the specified neighborhood. It is widely used in spatial clustering analyses of point data, and its advantage is that it can intuitively reflect the distribution of discrete measured values in continuous areas (Ma et al., 2022). This study used kernel density analysis to explore the spatial and temporal distribution characteristics of the family farms in the MYR-UA. The calculation is as follows (Yang et al., 2021):
where
The spatial distribution characteristics of the point features may vary with the observation scale. Point features clustered at small scales may exhibit discrete distributions at large scales. The advantage of Ripley’s K-function is that it can analyze the distribution patterns of point features at distinct spatial scales (Zhang et al., 2021). This study used Ripley’s K-function to determine the distribution patterns of the family farms at different spatial scales. The calculation is as follows:
where
Based on Eq. 5, Besag proposed the
We compared the
The grid analysis method is effective for determining and analyzing the geographical phenomena of the study area based on the spatial coordinate system. It has broad prospects for the analysis of spatial patterns (Briggs, 2018). Owing to the large scope of the study area, to accurately identify the factors influencing the spatial distribution pattern of family farms, we used the grid analysis method to divide the MYR-UA into 992 grid units. The area of the inner grids was 20 km × 20 km, while that of the boundary areas varied. Finally, based on the grid division, by extracting the number of family farms in each grid as the dependent variable and the corresponding geographic element values as independent variables, we constructed a regression model to analyze the factors influencing the spatial distribution of the farms.
The OLS model is a global regression model applied to evaluate the relationship between two or more elements. The advantage of the OLS model is that it minimizes the sum of squares of the residuals for all observations (Yang et al., 2022). This study initially explored the factors influencing the spatial layout of family farms using the OLS model. The calculation is as follows:
where
The OLS model can only estimate the parameters globally. Therefore, it was necessary to use the GWR model to reflect the non-stationarity of the parameters in space. Compared with the traditional regression model, GWR allows the relationships between the dependent and independent variables to vary spatially (Ma et al., 2022). This study used the GWR model to explore the factors influencing family farms and the spatial heterogeneity of these factors. The calculation is as follows:
where
The weight matrix can be described using a Gaussian function as follows:
where
To determine the best bandwidth value, we adopted the corrected Akaike information criterion (AICc) to search for the best value (Yang et al., 2022).
where
The spatial distribution patterns of family farms are influenced by several factors. Existing studies have used field research and questionnaire survey methods to explore the factors influencing the spatial distribution pattern of family farms and found that natural resources, economic development level, market demand, cultivated land resources, and traffic conditions are influencing factors (Greig, 2009; Marks-Bielska, 2013; Lowder et al., 2016; Kenny, 2017; Zhou et al., 2020; Pellegrina, 2022; Rahmani and Danesh-Yazdi, 2022; Wang et al., 2022), (Greig, 2009; Marks-Bielska, 2013; Lowder et al., 2016; Kenny, 2017; Zhou et al., 2020; Pellegrina, 2022; Rahmani and Danesh-Yazdi, 2022; Wang et al., 2022). Based on the current research results, combined with the principles of data availability and innovation, we selected topographic features, temperature, precipitation, water system characteristics, cultivated land resources, traffic conditions, economic development level, and population size as the potential influencing factors for this study from the two aspects of natural and social factors (Table 1).
The natural factors include topographic features, temperature, precipitation, water system characteristics, and cultivated-land resources. The topographic features directly affect the type and scale of the family farms (Greig, 2009) and thus have a substantial impact on the distribution. In this study, topographic features were expressed as the slope. Climatic factors and water system characteristics are the essential production conditions for family farms. Previous studies have confirmed that temperature, precipitation, and water area have an impact on the formation and development of family farms [ (Kenny, 2017; Rahmani and Danesh-Yazdi, 2022; Wang et al., 2022)]. Cultivated land resources are not only indispensable for the formation and development of family farms but are also the basis for large-scale development (Lowder et al., 2016). In this study, cultivated land resources were represented by the proportion of cultivated land area in the research unit.
Social factors include traffic conditions, economic development, and population size. Traffic is a primary factor determining the connection between a region and other areas (Zhou et al., 2020). Therefore, the geographic location of family farms is likely affected by traffic conditions. In this study, the traffic factor was represented by the density of the road network in the research unit. The level of economic development is a fundamental condition for industrial development (Pellegrina, 2022). A good economic foundation of a region plays a positive role in promoting the formation and development of family farms. This study selected the GDP level to represent the economic foundation. Population size can reflect the market consumption potential of a region, especially in the agricultural product market (Marks-Bielska, 2013). This study selected the average population density in the research unit to represent the population size.
Figure 2 shows the change in the number of family farms in the MYR-UA from 2013 to 2021. We divided the development of family farms into three stages according to the growth trends.
1) Rapid growth stage (2013–2017). After the Chinese government encouraged and supported the development of family farms in 2013, the number of family farms grew rapidly. In just 5 years, the number of family farms grew from 273 to 1,575, with an average annual increase of 325. In terms of family farms of different types, comprehensive family farms grew the fastest from 113 to 725. This was followed by planting farms that grew from 107 to 590. The slowest growth rate was observed for the breeding farms, which grew from 53 to 260.
2) Stable growth stage (2017–2019). During this stage, family farms showed a steady growth trend with an annual increase of approximately 150. In 2017, the “Guiding Opinions on Promoting the Development of Agricultural Industrial Complexes” issued by six departments, including the Ministry of Agriculture, further emphasized the basic position of family farms in agricultural industrialization. The introduction of this policy promoted the sustainable development of family farms. However, owing to the rapid growth during the early stage, the expansion of family farms in some areas faced insufficient land and market saturation, which led to a decline in the growth rate. In terms of different family farm types, the growth rate of comprehensive farms remained the fastest during this stage, increasing from 725 to 882. This was followed by planting farms that grew from 590 to 667, and the slowest growth was observed in breeding farms, which grew from 260 to 309.
3) Slow growth stage (2019–2021). In 2020, the Chinese government issued the “High-quality Development Plan for New Agricultural Business Entities and Service Entities,” emphasizing the need for the high-quality development of family farms. This stage focused on the pursuit of quality rather than the quantity of family farm development. Consequently, the growth rate slowed to an average of 70 per year. For the different types, the growth rate of planting farms was the fastest during this stage, growing from 667 to 714. This was followed by comprehensive farms, which grew from 882 to 947, and breeding farms, which grew from 309 to 337.
The ANN revealed the spatial distribution types of family farms in the MYR-UA. The Z-score and p-value results are measures of statistical significance used to determine whether to reject the null hypothesis. Table 2 shows that the ANN of overall family farms from 2013 to 2021 was less than 1, and the Z-score was less than −2.58. The results also passed the significance level test, indicating that the family farms in the MYR-UA always exhibited a distinct clustered distribution during the study period. Indicated by the trend of change in the values, the ANN and Z-score showed a significant downward trend, which meant that the degree of family farm clustering increased continuously.
Planting and comprehensive family farms were clustered in the study area, and the clustering degree increased yearly. The spatial distribution and degree of change of these two types of family farms were consistent with the overall family farms. The spatial distribution type and clustering degree of the breeding family farms changed significantly during the study period. From the perspective of spatial distribution, the breeding family farms were randomly distributed in the study area only in 2013, while the other years had a clustered distribution. The degree of spatial clustering of the breeding family farms experienced two stages of change. First, from 2013 to 2015, the ANN decreased from 0.942 to 0.719, indicating that the spatial clustering degree increased. Second, from 2015 to 2021, the ANN increased from 0.719 to 0.754, indicating that the spatial clustering degree of breeding family farms was weakening.
The kernel density analysis method was used to further analyze the spatial distribution patterns of the family farms. We used kernel density analysis in ArcGIS 10.8 spatial analysis tool, set the search radius to 20 km, and kept the default values for the other variables. We then selected 2013, 2017, and 2021 as the time points for the analysis.
1) Family farms in general. As shown in Figure 3A, the overall spatial distribution pattern of the family farms in the MYR-UA changed considerably. The application and certification of family farms in China began at the end of 2013. At this time, the number of family farms in the study area was small and exhibited a single-core distribution pattern. These farms were mainly distributed in Nanchang with clear circular structures. As the capital city of Jiangxi Province, Nanchang led the development of family farms under the guidance of national policies. From 2013 to 2017, the government introduced several measures to support the development of family farms, such as tax reductions, subsidies, and free training. Therefore, the distribution expanded rapidly, evolving from a single-core agglomeration to a multicore agglomeration. The medium- and higher-density agglomeration areas spread to the east and west sides in a band-like shape. High-density agglomeration areas expanded from Nanchang to the surrounding cities, such as Yingtan, Yichun, and Xinyu. From 2017 to 2021, the scale of the family farms expanded further, with noticeable multicenter distribution characteristics. At this time, some small-scale high-density agglomeration areas appeared in Jingdezhen, north of Changde, the central part of Hengyang, and at the junction of Yueyang and Changsha. Notably, WMA did not form a high-density or medium-high-density agglomeration area. This is because the urbanization and industrialization of WMA have developed rapidly in recent years, which has resulted in a large reduction in agricultural land. In addition, frequent flood disasters in the Jianghan Plain have seriously damaged the agricultural ecological environment and restricted the development of family farms.
2) Planting family farms. Figure 3B illustrates the spatial evolution patterns of the planting family farms. In 2013, planting family farms were highly concentrated in Nanchang, forming a polar-core spatial structure. Nanchang has a vast area of arable land and a high level of agricultural modernization, providing advantages for developing planting family farms. However, the entire study area was dominated by low- and lower-density agglomerations. A few high-density agglomeration areas were scattered west of Yingtan, Nanchang, north of Shangrao, northeast of Jiujiang, and north of Jingdezhen. From 2013 to 2017, the main spatial expansion pattern of planting family farms was jump diffusion. During this stage, influenced and driven by Nanchang, planting family farms in cities around the capital city (including Yichun, Ji’an, Fuzhou, Xinyu, and Yingtan) developed rapidly, forming several high-density agglomeration areas, which also extended to the west of the study area, covering most of the cities in the CZT-UA. From 2017 to 2021, contact diffusion was the main spatial expansion pattern of planting family farms. The changes in the higher- and high-density agglomeration areas were not substantial and expanded outward from the original area. During this stage, the government focused more on the quality of the development of planting family farms.
3) Breeding family farms. Figure 3C shows the spatial evolution patterns of breeding family farms. In 2013, the number of declarations of breeding family farms was relatively small, exhibiting a spatial pattern of “one pole and multiple points.” The main gathering points were distributed in the central part of Nanchang, whereas the density in other places was more scattered. This is because Nanchang focused on training the farmers in scientific breeding and has established professional training institutions. Therefore, the development of breeding family farms in Nanchang was the fasted of the farm types. From 2013 to 2017, breeding family farms expanded rapidly to the northwest. During this stage, the higher- and high-density agglomeration areas were relatively scattered, and higher- and high-density agglomeration areas appeared in all three urban agglomerations. However, the areas with higher density values were predominantly distributed in PLCG. From 2017 to 2021, with the popularization of scientific breeding technology and experience, breeding family farms were further developed, exhibiting a dense spatial distribution pattern in the southeast and a sparse spatial distribution pattern in the northwest. The changes in the high-density agglomeration area were less substantial, and the expansion of the higher-density agglomeration area was larger. Most of the new high-density agglomeration areas appeared in the CZT-UA.
4) Comprehensive family farms. Figure 3D shows the spatial evolution patterns of the comprehensive family farms. In 2013, the spatial distribution patterns of the comprehensive and planting farms were similar. At this time, the comprehensive family farm focused primarily on planting and was supplemented by breeding. From 2013 to 2017, the government encouraged rice and shrimp farming. This move led to the rapid expansion of comprehensive family farms, forming multiple growth opportunities. The higher- and high-density agglomeration areas expanded rapidly in a point-like manner. High-density agglomeration areas were mainly distributed in the northwest of PLCG and southeast of CZT-UA, including Nanchang, west of Yingtan, east of Jiujiang, Xinyu, south of Jingdezhen, east of Shangrao, Pingxiang, Hengyang, northern Changsha, and northeast Yiyang. From 2017 to 2021, comprehensive family farms exhibited a spreading expansion trend, and the multicenter distribution pattern became more distinct. To pursue greater economic benefits, most single-family farms had begun to transform into comprehensive farms. At this time, the higher- and high-density agglomeration areas expanded significantly to the southwest, forming numerous new higher- and high-density areas in CZT-UA.
FIGURE 3. Here Kernel density analysis of family farms: (A–D) indicate family farms overall, planting family farms, breeding family farms, and comprehensive family farms, respectively.
Ripley’s function was used to analyze the spatial distribution pattern of the family farms overall and different types of family farms in the MYR-UA at multiple scales. The calculation results are presented in Figure 4. Generally, the overall family farms and different types of family farms in the MYR-UA exhibit significant spatial agglomeration distribution characteristics, which first increase and then weaken with increasing geographic distance. In 2013, 2017, and 2021 (Figures 4A–C), the trends of the
FIGURE 4. Here Ripley’s K-function analysis of family farms: (A–C) indicate family farms overall; (D–F) indicate planting family farms; (G–I) indicate breeding family farms; and (J–L) indicate comprehensive family farms.
From the distances corresponding to the peaks of the three types of family farms in 2013 (Figures 4D, 4G, 4J), the breeding and planting farms exhibited agglomeration characteristics in a large spatial range, with peaks at 104.45 and 103.78 km, respectively. This indicates that the scale range of location selection for breeding and planting family farms is large. The distance corresponding to the peak of the comprehensive family farm is the smallest (83.77 km), indicating that the scale range of its spatial location selection is small. The distances corresponding to the peaks of the three types of family farms in 2017 were not significantly different from those in 2013 (Figures 4E, 4H, 4K). From the largest to the smallest, they are breeding, planting, and comprehensive family farms, and the corresponding peak distances are 105.79, 102.7, and 85.29 km, respectively. In 2021 (Figures 4F, 4I, 4L), the distances corresponding to the peaks of the three types changed significantly. Specifically, the distance corresponding to the peaks of the breeding and planting farms decreased, and that of the comprehensive farms increased. However, the distance corresponding to the peak of breeding family farms remained the largest, which indicates that the spatial scale of location selection of the breeding family farm is the largest. The distances corresponding to the peaks of the planting and comprehensive family farms are similar, indicating that the difference in the agglomeration scale of these two types of family farms was narrowing.
Before applying the GWR model, a global OLS regression model was used to test the relationship between the explanatory and explained variables. According to the results of variable selection, we used the number of family farms in each grid as the dependent variable and eight influencing factors as the independent variables and conducted an OLS regression analysis to preliminarily test the effect degree and significance level of the explanatory variables on the explained variables. Table 3 lists the results of the OLS model.
The OLS regression results are as follows. 1) The variance inflation factor test showed that the VIF value of each influencing factor was less than 7.5, which indicated that the model variables were reasonably set and that there was no variable redundancy or multicollinearity. 2) The goodness of fit of the model was 0.26, which passed the significance test, indicating that the model was robust. 3) Among the explanatory variables, only the water system characteristics failed the significance test, while the other variables passed the statistical significance test. Temperature, precipitation, cultivated land resources, traffic conditions, economic development level, and population size had a positive impact on the spatial distribution pattern of family farms, and topographic features had a negative influence. The water system characteristics had no significant impact on the spatial distribution pattern. 4) Based on the value of the influencing factor coefficient, the traffic factor had the greatest impact on the number of family farms. When other conditions remain unchanged, the number of family farms in the unit space increased by 1.65 for every 1 unit increase in the traffic factor. The other factors were in the order of cultivated land resources, temperature, topographic features, precipitation, and population size, and the impact of the economic development level was the lowest. Overall, social factors had a greater impact than natural factors on the number of family farms. 5) The Jarque–Bera test results were significant, which indicated that the residuals did not obey a normal distribution and that the model fitting was one-sided. To improve the degree of fitting, the GWR model must be introduced.
The default space of the OLS model was homogeneous and considered only the global characteristics of the regression coefficients, which could not reflect regional heterogeneity. Therefore, we introduced the GWR model to analyze the influence of various influencing factors on the spatial heterogeneity of the dependent variables. Before running the GWR model, we first set the parameters, took the latitude and longitude of the center of mass of each grid as the geographic coordinates, selected the fixed Gaussian function for the kernel type, used the golden section search to select the bandwidth, and considered the AICc as the bandwidth selection criterion. Based on the regression results of the GWR model, the R-squared value of the OLS model was 0.26, the AICc value was 4,251.15, while the R-squared value obtained using the GWR model was 0.41, and the value of AICc was 4,039.14. According to Fotheringham et al. (Fortheringham et al., 1996), if the AICc value of the GWR model is smaller than that of the OLS model, then the GWR model is appropriate. This implies that the GWR model in this study was more suitable than the OLS model for the regression fitting of the explanatory variables.
Table 4 presents the statistics of the mean, minimum, maximum, and upper and lower quartile values based on the regression coefficient of the GWR model. The regression coefficients of traffic conditions and cultivated land resources varied significantly, and the maximum value was high, indicating that these two factors had a significant impact on the spatial distribution pattern of the family farms. The regression coefficients of temperature, traffic conditions, economic development level, and population size were both positive and negative for different areas, indicating that these four factors had a positive impact on the spatial distribution pattern of family farms in some regions and a negative impact on the spatial distribution pattern of family farms in other regions. In all the study areas, the regression coefficients of the topographic features were negative and those of precipitation were positive.
In addition to the model parameter estimates above, the GWR model simulates the spatial differences in the degree of influence of different factors on the spatial distribution pattern of family farms. In this study, we counted the proportions of positive and negative values of the regression coefficients of the influencing factors in each grid (Figure 5). At the same time, we also used ArcGIS 10.8 software to visualize the spatial heterogeneity of the influencing factors (Figure 6).
FIGURE 5. Here Statistics of positive and negative regression coefficients for various factors: a–g indicate topographic features, temperature, precipitation, cultivated land resources, traffic conditions, economic development level, and population size, respectively.
FIGURE 6. Here Spatial distribution of the regression coefficients of various correlated variables in the geographically weighted regression (GWR) model: (A–G) indicate topographic features, temperature, precipitation, cultivated land resources, traffic conditions, economic development level, and population size, respectively.
As shown in Figure 5A, the regression coefficient values of topographic features were negative in the entire study area, indicating that topographic features had a completely negative correlation with the number of family farms. The spatial distribution of the absolute value of the regression coefficient showed a distribution trend of “high in the south and low in the north” (Figure 6A). The high-value areas of the absolute value of the regression coefficient were mainly distributed in Changsha, Xiangtan, Zhuzhou, Fuzhou, and Yingtan, indicating that topographic features had a greater impact on the spatial distribution of family farms in these areas. This may be because these areas were mainly rice- and vegetable-growing family farms and live-stock-raising family farms, which are more susceptible to terrain conditions. The low-value areas of the absolute values of the regression coefficient were distributed in Xiangyang, Jingmen, Xiaogan, Wuhan, Ezhou, and Huanggang. Most of these areas were located in low mountain areas and the Jianghan Plain, so the development of family farms was less affected by topographic conditions.
Figure 5B shows that the regression coefficient of the temperature factor was positive in most research units and negative in only 4% of research units. This means that the temperature had a positive effect on the number of family farms. The regression coefficient of the influencing factor showed a decreasing distribution trend from the center to the north and south (Figure 6B). The regions with higher regression coefficients were mainly distributed in southern Yueyang, northern Changsha, northeastern Fuzhou, Yingtan, and Shangrao, which indicated that the temperature factor in this region had a significant promoting effect on the number of family farms. The regions with low coefficients were distributed in the north of the WMA, such as Xiangyang, Jingmen, Tianmen, north of Yichang, Xiaogan, and Wuhan. This is because investment in agricultural scientific research has been strengthened in these areas in recent years, and greenhouse technology and temperature-control equipment have been used to adjust the temperature conditions required for agricultural planting and animal husbandry. Therefore, the temperature factor has little correlation with the number of family farms.
As shown in Figure 5C, the regression coefficient values of the precipitation factor were positive for the entire study area, indicating that topographic features had a complete positive correlation with the number of family farms. The high-value areas of the coefficient were mainly distributed in northwestern Yichun, southwestern Jiujiang, and eastern Xianning (Figure 6C). These areas are rich in grain and cash crops in China, and the level of precipitation is conducive to the development of family farms. The low-value areas of the coefficient, such as Fuzhou, Yingtan, and Shangrao, were distributed east of the PLCG. There are several water systems in these areas. The three river systems of Fuhe, Xinjiang, and Ganjiang pass through this area and there are abundant irrigation water sources. Therefore, precipitation was not a key factor in the development of family farms.
Figure 5D shows that the regression coefficient of cultivated land resources was positive in all the research units, which means that there was a significant positive correlation between cultivated land resources and the number of family farms. Figure 6D demonstrates that the regression coefficient of cultivated land resources varied from 0.48905 to 4.47857 and the impact varied significantly in space. The spatial distribution of the regression coefficients exhibited a decreasing trend from the southeast to the northwest. The maximum value appeared in PLCG, specifically in Xinyu, Yichun, Nanchang, Jingdezhen, Shangrao, and Yingtan. In recent years, affected by industrialization, urbanization, and natural disasters in these regions, the area of cultivated land has gradually declined. Therefore, cultivated land resources have a significant impact on the number of family farms in a region. The minimum values were observed in Yichang, Xiangyang, Huanggang, and Changde. This may be because the area has sufficient land for agriculture, animal husbandry, and aquaculture, and the development of family farms is less affected by cultivated land resources.
As shown in Figure 5E, in 74% of the study units, the number of family farms was positively correlated with traffic conditions, whereas only 26% were negatively correlated. In addition, the regression coefficient of the traffic conditions varied from −1.34760 to 7.28191, and the impact varied significantly in space (Figure 6E). The regression coefficient of the influencing factor showed distribution characteristics of high in the east and low in the west. Traffic conditions had a positive impact on the number of family farms in the entire PLCG and the central and southern areas of the CZT-UA. Traffic conditions play a pivotal role in the development of family farms in the area. In the WMA and northern areas of the CZT-UA, traffic conditions had a negative impact on the number of family farms. For these areas, convenient traffic conditions may lead to a large influx of outsiders, thus damaging the local ecological and living environments, which is not conducive to the development of family farms.
Figure 5F shows that in 72% of the study units, the number of family farms was positively correlated with the level of economic development, and 28% was negatively correlated. As shown in Figure 6F, the positive areas of the regression coefficient were mainly distributed in the WMA and central and eastern parts of the PLCG. This means that the higher the level of economic development, the greater the number of family farms in the region. The negative value area of the regression coefficient was distributed in the CZT-UA, mainly including Hengyang, Loudi, Xiangtan, Yi-yang, Changsha, Zhuzhou, southeastern Changde, and southern Yueyang. This demonstrates that the level of economic development had restricted the development of family farms in the region. The reason may be that with the improvement in the level of economic development, people’s enthusiasm to engage in agricultural production activities will decrease, and they will be more willing to engage in other industries with higher economic benefits.
As shown in Figure 5G, in 59% of the study units, the number of family farms was positively correlated with population size, and only 41% was negatively correlated. Figure 6G shows that the positive areas of the regression coefficients were mainly distributed in the CZH-UA and southwestern WMA. In these regions, an increase in population size will bring broad market prospects, which is conducive to the development of family farms. The negative value area of the regression coefficient is mainly distributed in the middle of the PLCG, such as Nanchang, Yingtan, east of Jiujiang and Shangrao, Fuzhou, Jingdezhen, and west of Yichun. In recent years, with rapid economic development and increasing population in these regions, the large population density caused tension between people and the land, which will have a negative impact on the development of family farms.
Family farms are developing rapidly in various regions (Du et al., 2020). However, the excessive expansion of family farms has resulted in a series of practical problems, which not only harm the interests of farmers but also hinder the process of agricultural modernization (Shang et al., 2021). Therefore, rationally arranging family farms and promoting the high-quality development of family farms is an urgent problem that needs to be solved. From temporal and spatial perspectives, this study comprehensively used the nearest neighbor index, kernel density analysis, Ripley’s K-function, grid analysis, and GWR model to deeply explore the spatiotemporal distribution patterns and influencing factors of family farms in the MYR-UA, which can provide a scientific reference for solving the above problems. The specific theoretical and practical significance of this study are as follows:
First, this study used a variety of geographic methods to deeply explore the spatial and temporal distribution patterns and influencing factors of family farms in the MYR-UA. In addition, this study classifies family farms and discusses the spatial distribution patterns of different types of family farms. The results of this study are of great significance in revealing the trends of modern agricultural development and enriching relevant theories of agricultural geography. Previous studies have focused primarily on the operating efficiency, operating model, and formation mechanism of certain types of family farms (Bertolozzi-Caredio et al., 2020). However, few studies have analyzed the spatial distribution characteristics and trends of different types of family farms. To fill this gap, this study applied the average nearest neighbor index, kernel density analysis, and Ripley’s K-function to comprehensively explore the spatial distribution patterns of the overall family farms and different types of family farms. As shown by the results of this study, the overall family farms and different types of family farms exhibit spatial distribution patterns of dispersion and local concentration. In addition, overall family farms and various types of family farms demonstrate a scale effect, which first strengthens and then weakens with the change in geographical distance.
Moreover, this study applied the OLS model, GWR model, and grid analysis to systematically explore the influencing factors and spatial heterogeneity of the spatial distribution pattern of family farms, which can provide a methodological reference for the same type of research. By comparing the parameter results of the two models, we found that the GWR model was more suitable than the OLS model for this study. The GWR model not only analyzed the positive and negative effects of the influencing factors but also explains the spatial heterogeneity of such influencing factors (Pravitasari et al., 2021). However, because of the large areal differences of the cities in the MYR-UA, for this analysis scale, the spatial heterogeneity of the influencing factors could not be accurately analyzed. Therefore, we introduced a grid analysis in this study, dividing the larger study area into grids of equal size. Finally, the grid unit was used as the research scale to analyze the spatial heterogeneity of the influencing factors. This method has strong applicability and scientific benefits and has been applied gradually by research institutes [(Zhu et al., 2020), (Bi et al., 2022)].
Ultimately, this study can provide scientific guidance for government departments to reasonably plan family farmland. Through the above analysis, we know that the spatial pattern of family farms is affected by many natural and social factors. The traditional view is that natural factors are the internal determinants of the distribution of family farms, while social factors are the external driving factors (Xing et al., 2021). However, the results of this study show that the absolute value of the regression coefficient of social factors, such as traffic conditions, population size, and economic development level, is higher than that of natural factors, such as terrain, temperature, precipitation, and cultivated land resources. This shows that social factors have a greater impact on the spatial layout of family farms. This is because natural factors that affect spatial distribution have gradually become controllable with the development of science and technology. For example, greenhouse planting technology and temperature-control equipment can reasonably adjust the temperature conditions required for family farm production. Automatic sprinkler irrigation equipment and artificial precipitation can improve the water resources required for family farm production. Crop grafting and seed improvement technologies can improve the yield of agricultural products, which makes cultivated land resources no longer a decisive factor for the development of family farms [(Washizu and Nakano, 2022), (Ruzzante et al., 2021)]. It can be preliminarily predicted that the spatial distribution of family farms in the future will be affected more by social factors than by natural factors.
The limitations of this study are mainly reflected in the research on the influencing factors. First, owing to the limited availability of data, influencing factors, such as the rural labor force, government policies, and land rent, were not considered in this study. In future research, we will combine management, anthropology, and other interdisciplinary research methods to improve the research on the factors affecting the spatial distribution pattern of family farms. In addition, this study did not consider the factors influencing the spatial distribution patterns of different types of family farms. In the future, we will supplement these data to explore these factors and conduct a comparative analysis.
By analyzing the spatiotemporal dynamic evolution and influencing factors of family farms in the MYR-UA, we obtained the following findings:
1) In terms of temporal changes, the overall family farms and different types of family farms in the MYR-UA exhibited an increasing annual trend and underwnt three stages: rapid, stable, and slow growth.
2) Regarding the spatial distribution patterns, family farms in the MYR-UA had significant agglomeration characteristics, and the degree of agglomeration continued to increase, showing a distribution pattern of density in the southeast and scattered in the northwest. The spatial evolution characteristics of the different types of family farms were roughly consistent with the overall family farms.
3) For multiscale spatial characteristics, the overall family farms and various types of family farms demonstrate a scale effect, which first strengthened and then weakened with the change in the geographical distance; however, there were significant differences in the scope of the location selection space.
4) The spatial pattern of family farms in the MYR-UA was affected by both natural and social factors. The influence of social factors was greater than that of natural factors. Precipitation and cultivated land resources had a positive effect on the spatial distribution of family farms, topographic features had a negative effect, and temperature, traffic conditions, economic development level, and population size had both positive and negative effects.
Based on the results of this study, the following policy implications are proposed for the construction and development of family farms in the MYR-UA. First, land for family farms should be rationally planned according to the principle of adapting measures to the local conditions. Specifically, the government should divide the land types of family farms according to topographic features and water resource conditions. For example, Nanchang, Changde, Tianmen, Yingtan, and Yichun have relatively flat terrain and abundant precipitation suitable for planting crops and small-area family farming. Therefore, these areas are conducive to the development of planting and comprehensive family farms. Xiantao, Xinyu, Yueyang, and Pingxiang have complex terrain conditions, predominantly low mountains and hills, suitable for raising poultry. In addition, many lakes in these areas provide good conditions for fishery breeding.
Second, road infrastructure construction should be strengthened to ensure the transportation of family farm products. Most products produced by family farms are not suitable for long-term storage and must be marketed in a timely manner. Therefore, the government should increase the construction of agricultural support infrastructure, especially to ensure smooth access to roads in rural and remote areas. Additionally, the government should focus on road construction in family farm cluster areas. For example, cities in the eastern part of the PLCG have numerous family farms, and a high degree of agglomeration, and are greatly affected by road facilities. The government should strengthen the construction and optimization of transportation facilities in this area.
Third, strengthening the protection of cultivated land, strictly prohibiting the occupation of cultivated land by construction projects, and avoiding the pollution and destruction of cultivated land. The government should focus on ensuring the quality of arable land, especially in areas with well-developed family farms, such as PLCG and CZT-UA. Only in this way can the output of family farms be guaranteed and their economic benefits maximized.
Finally, the construction of a market information service platform should be accelerated so that family farmers can receive comprehensive market information more rapidly and then make more effective production and operation decisions. Family farmers should pay attention not only to the local market but also to the foreign market so that they can produce and sell their products rationally. In addition, the government can build a regional cooperation and exchange platform, establish an industrial alliance, encourage farmers to share their practical experience, and finally form a family farm development model with healthy regional competition, coordinated development, and mutually beneficial cooperation.
The original contributions presented in the study are included in the article/supplementary material, further inquiries can be directed to the corresponding author.
This paper was written with the contributions of all authors as follows: conceptualization, HL and YM; methodology, JR and HL; data curation, YT; investigation, HL and JR; funding acquisition, YM and YT; project administration, YM and YT; writing—original draft, HL and YM; writing—review and editing, JR, and YT. All the authors have read and agreed to the published version of the manuscript.
This research was supported by the National Social Science Foundation of China (19BJL036; 21BJY194), Natural Science Foundation of Hainan Province (721QN219), and Hainan Graduate Innovation Research Project (Qhys 2021-1).
The authors declare that the research was conducted in the absence of any commercial or financial relationships that could be construed as a potential conflict of interest.
All claims expressed in this article are solely those of the authors and do not necessarily represent those of their affiliated organizations, or those of the publisher, the editors and the reviewers. Any product that may be evaluated in this article, or claim that may be made by its manufacturer, is not guaranteed or endorsed by the publisher.
Baležentis, T., Kriščiukaitienė, I., and Baležentis, A. (2014). A nonparametric analysis of the determinants of family farm efficiency dynamics in Lithuania. Agric. Econ. 45, 589–599. doi:10.1111/agec.12108
Bertolozzi-Caredio, D., Bardaji, I., Coopmans, I., Soriano, B., and Garrido, A. (2020). Key steps and dynamics of family farm succession in marginal extensive livestock farming. J. Rural. Stud. 76, 131–141. doi:10.1016/j.jrurstud.2020.04.030
Besag, J. (1977). Discussion on dr Ripley’s paper. J. R. Stat. Soc. B 39, 192–212. doi:10.1111/j.2517-6161.1977.tb01616.x
Bi, S., Dai, F., Chen, M., and Xu, S. (2022). A new framework for analysis of the morphological spatial patterns of urban green space to reduce PM2.5 pollution: A case study in wuhan, China. Sustain. Cities Soc. 82, 103900. doi:10.1016/j.scs.2022.103900
Briggs, R. C. (2018). Poor targeting: A gridded spatial analysis of the degree to which aid reaches the poor in africa. World Dev. 103, 133–148. doi:10.1016/j.worlddev.2017.10.020
Burton, R. J. F., and Walford, N. (2005). Multiple succession and land division on family farms in the south east of England: A counterbalance to agricultural concentration? J. Rural. Stud. 21, 335–347. doi:10.1016/j.jrurstud.2005.04.004
Carte, L., Schmook, B., Radel, C., and Johnson, R. (2010). The slow displacement of smallholder farming families: Land, hunger, and labor migration in Nicaragua and Guatemala. Land 8, 89. doi:10.3390/land8060089
Chen, J., Jiang, L., Luo, J., Tian, L., Tian, Y., and Chen, G. (2022). Characteristics and influencing factors of spatial differentiation of market service industries in rural areas around metropolises—a case study of wuhan city’s new urban districts. ISPRS Int. J. Geoinf. 11, 170. doi:10.3390/ijgi11030170
Chen, Z., Meng, Q., Yan, K., and Xu, R. (2022). The analysis of family farm efficiency and its influencing factors: Evidence from rural China. Land 11, 487. doi:10.3390/land11040487
Chen, Z., Meng, Q., Yan, K., and Xu, R. (2022). The analysis of family farm efficiency and its influencing factors: Evidence from rural China. Land 11, 487. doi:10.3390/land11040487
Du, Z., Lai, X., Long, W., and Gao, L. (2020). The short- and long-term impacts of the COVID-19 pandemic on family farms in China – evidence from a survey of 2324 farms. J. Integr. Agric. 19, 2877–2890. doi:10.1016/S2095-3119(20)63390-1
Dumas, C., Dupuis, J., Richer, F., and Louise, S. (2016). Factors that influence the next generation’s decision to take over the family farm. Fam. Bus. Rev. 8, 99–120. doi:10.1111/j.1741-6248.1995.00099.x
Fortheringham, A., Chanrlton, M., and Brunsdon, C. (1996). The geographically of parameter space: An investigation of spatial nonstationarity. Int. J. Geogr. Inf. Syst. 10, 605–627. doi:10.1080/026937996137909
Fox, G., Mooney, J., Rosati, P., and Lynn, T. (2021). AgriTech innovators: A study of initial adoption and continued use of a mobile digital platform by family-operated farming enterprises. Agriculture 11, 1283. doi:10.3390/agriculture11121283
Fu, J., Jiang, D., and Huang, Y. (2014). PopulationGrid_China. Beijing, China: Global Change Research Data Publishing & Repository.
Gao, Y., Liu, B., Yu, L., Yang, H., and Yin, S. (2019). Social capital, land tenure and the adoption of green control techniques by family farms: Evidence from Shandong and Henan Provinces of China. Land Use Policy 89, 104250. doi:10.1016/j.landusepol.2019.104250
Gao, Y., Zhang, X., Lu, J., Wu, L., and Yin, S. (2017). Adoption behavior of green control techniques by family farms in China: Evidence from 676 family farms in Huang-huai-hai Plain. Crop Prot. 99, 76–84. doi:10.1016/j.cropro.2017.05.012
Gong, T., Battese, G. E., and Villano, R. A. (2019). Family farms plus cooperatives in China: Technical efficiency in crop production. J. Asian Econ. 64, 101129. doi:10.1016/j.asieco.2019.07.002
Graeub, B. E., Chappell, M. J., Wittman, H., Ledermann, S., Kerr, R. B., and Gemmill-Herren, B. (2016). The state of family farms in the world. World Dev. 87, 1–15. doi:10.1016/j.worlddev.2015.05.012
Greig, L. (2009). An analysis of the key factors influencing Farmer’s choice of crop, Kibamba Ward, Tanzania. J. Agric. Econ. 60, 699–715. doi:10.1111/j.1477-9552.2009.00215.x
Huang, Y., Jiang, D., and Fu, J. (2014). GDPGrid_China. Beijing, China: Global Change Research Data Publishing & Repository.
Huber, R., Flury, C., and Finger, R. (2015). Factors affecting farm growth intentions of family farms in mountain regions: Empirical evidence for Central Switzerland. Land Use Policy 47, 188–197. doi:10.1016/j.landusepol.2015.04.006
Ilbery, B., and Maye, D. (2010). Clustering and the spatial distribution of organic farming in England and Wales. Area 43, 31–41. doi:10.1111/j.1475-4762.2010.00953.x
Ji, X., Rozelle, S., Huang, J., Zhang, L., and Zhang, T. (2016). Are China’s farms growing? China & World Econ. 24, 41–62. doi:10.1111/cwe.12143
Julia, S., and Elena, F. (2016). The future of family farming: A literature review on innovative, sustainable and succession-oriented strategies. J. Rural. Stud. 10, 117–140. doi:10.1016/j.jrurstud.2016.07.008
Kenny, D. C. (2017). Modeling of natural and social capital on farms: Toward useable integration. Ecol. Modell. 356, 1–13. doi:10.1016/j.ecolmodel.2017.04.010
Liang, L., Lin, S., and Zhang, Z. (2016). Effect of the family life cycle on the family farm scale in Southern China. Agric. Econ. 61, 429–440. doi:10.17221/68/2014-AGRICECON
Lowder, S. K., Skoet, J., and Raney, T. (2016). The number, size, and distribution of farms, smallholder farms, and family farms worldwide. World Dev. 87, 16–29. doi:10.1016/j.worlddev.2015.10.041
Lv, Y., Gu, S., and Guo, D. (2010). Valuing environmental externalities from rice-wheat farming in the lower reaches of the Yangtze River. Ecol. Econ. 69, 1436–1442. doi:10.1016/j.ecolecon.2008.12.014
Ma, Y., Li, H., and Tong, Y. (2022). Distribution differentiation and influencing factors of the high-quality development of the hotel industry from the perspective of customer satisfaction: A case study of Sanya. Sustainability 14, 6476. doi:10.3390/su14116476
Madau, F. (2015). Technical and scale efficiency in the Italian citrus farming: Comparison between SFA and DEA approaches. Agric. Econ. Rev. 16, 15–27. doi:10.22004/ag.econ.253696
Marchant Santiago, C., Rodríguez Díaz, P., Morales-Salinas, L., Paz Betancourt, L., and Ortega Fernández, L. (2021). Practices and strategies for adaptation to climate variability in family farming. An analysis of cases of rural communities in the Andes Mountains of Colombia and Chile. Agriculture 11, 1096. doi:10.3390/agriculture11111096
Marks-Bielska, R. (2013). Factors shaping the agricultural land market in Poland. Land Use Policy 30, 791–799. doi:10.1016/j.landusepol.2012.06.003
Pellegrina, H. S. (2022). Trade, productivity, and the spatial organization of agriculture: Evidence from Brazil. J. Dev. Econ.102816 156. doi:10.1016/j.jdeveco.2021.102816
Philip, K., Sophia, D., and Alistair, B. (2018). Effect of family labour on output of farms in selected eu member States: A non-parametric quantile regression approach. Eur. Rev. Agric. Econ. 3, 367–395. doi:10.1093/erae/jbx036
Pravitasari, A. E., Rustiadi, E., Priatama, R. A., Murtadho, A., Kurnia, A. A., Mulya, S. P., et al. (2021). Spatiotemporal distribution patterns and local driving factors of regional development in java. ISPRS Int. J. Geoinf. 10, 812. doi:10.3390/ijgi10120812
Qian, Z., and Li, Y. (2020). Efficiency and decision of family farms: An empirical study based on 2017 data of 943 family farms in Songjiang, Shanghai. Manag. World 36, 168–181. doi:10.19744/j.cnki.11-1235/f.2020.0060
Rahmani, J., and Danesh-Yazdi, M. (2022). Quantifying the impacts of agricultural alteration and climate change on the water cycle dynamics in a headwater catchment of Lake Urmia Basin. Agric. Water Manag. 270, 107749. doi:10.1016/j.agwat.2022.107749
Reinhardt, N., and Barlett, P. (2008). The persistence of family farms in U.S. Agriculture. Sociol. Rural. 29, 203–225. doi:10.1111/j.1467-9523.1989.tb00367.x
Ruzzante, S., Labarta, R., and Bilton, A. (2021). Adoption of agricultural technology in the developing world: A meta-analysis of the empirical literature. World Dev. 146, 105599. doi:10.1016/j.worlddev.2021.105599
Shang, H., Yi, X., Yin, C., Chen, Y., and Zhang, Z. (2021). How does the stability of land management right (SLMR) affect family farms’ cultivated land protection and quality improvement behavior (CLPQIB) in China? Land 10, 1052. doi:10.3390/land10101052
Shubin, S. (2011). Russia’s unknown agriculture. J. Rural. Stud. 27, 107–108. doi:10.1016/j.jrurstud.2010.10.003
Wang, H., Zhong, S., Guo, J., and Fu, Y. (2021). Factors affecting green agricultural production financing behavior in heilongjiang family farms: A structural equation modeling approach. Front. Psychol. 12, 692140. doi:10.3389/fpsyg.2021.692140
Wang, L., Qi, Z., Pang, Q., Xiang, Y., and Sun, Y. (2020). Analysis on the agricultural green production efficiency and driving factors of urban agglomerations in the middle reaches of the Yangtze River. Sustainability 13, 97. doi:10.3390/su13010097
Wang, M., Yuan, M., Han, P., and Wang, D. (2022). Assessing sustainable urban development based on functional spatial differentiation of urban agriculture in Wuhan, China. Land Use Policy 115, 105999. doi:10.1016/j.landusepol.2022.105999
Washizu, A., and Nakano, S. (2022). Exploring the characteristics of smart agricultural development in Japan: Analysis using a smart agricultural kaizen level technology map. Comput. Electron. Agric. 198, 107001. doi:10.1016/j.compag.2022.107001
Wilson, J., Simpson, I. H., and Landerman, R. (2010). Status variation on family farms: Effects of crop, machinery, and off-farm work. Rural. Sociol. 59, 136–153. doi:10.1111/j.1549-0831.1994.tb00526.x
Xing, X., Zhang, S., Liang, J., and Liu, J. (2021). Spatiotemporal expansion characteristics and driving forces of family farms in hilly and mountainous areas: A case study of Jiangjin District of chongqing, China. Mt. Res. 39, 71–87.
Xu, X., and Zhang, Y. (2017). China meteorological background dataset. Beijing, China: Resource and Environmental Science Data Registration and Publishing System.
Yang, L., Yu, K., Ai, J., Liu, Y., Yang, W., and Liu, J. (2022). Dominant factors and spatial heterogeneity of land surface temperatures in urban areas: A case study in Fuzhou, China. Remote Sens. (Basel). 14, 1266. doi:10.3390/rs14051266
Yang, Q., Zhang, F., An, Y., Sun, C., Wu, J., Zhang, Y., et al. (2021). Research on the spatial distribution pattern and influencing factors of China’s antipoverty (pro-poor tourism) on GIS. Discrete Dyn. Nat. Soc. 2021, 1–11. doi:10.1155/2021/6682498
Zhang, S., Wang, J., Wei, C., Xiong, X., and Liu, J. (2022). Spatiotemporal evolution and industrial response of diversified new agricultural management entities in Hilly and mountainous areas. J. Southwest Univ. 44, 118–137.
Zhang, Z., Luan, W., Tian, C., Su, M., and Li, Z. (2021). Spatial distribution equilibrium and relationship between construction land expansion and basic education schools in Shanghai based on POI data. Land 10, 1059. doi:10.3390/land10101059
Zhou, Y., Huang, H., and Liu, Y. (2020). Spatial distribution law and influencing factors of villages in China. Acta Geogr. Sin. 75, 2206–2223. doi:10.11821/dlxb202010012
Keywords: family farms, agricultural modernization, spatiotemporal heterogeneity, GIS visualization, geographical weighted regression, high-quality development
Citation: Li H, Ma Y, Ren J and Tong Y (2022) Spatiotemporal dynamic evolution and influencing factors of family farms in urban agglomerations in the middle reaches of the Yangtze River. Front. Environ. Sci. 10:1001274. doi: 10.3389/fenvs.2022.1001274
Received: 23 July 2022; Accepted: 29 November 2022;
Published: 13 December 2022.
Edited by:
Xiaocui Wu, University of Illinois at Urbana-Champaign, United StatesReviewed by:
Fengtai Zhang, Chongqing University of Technology, ChinaCopyright © 2022 Li, Ma, Ren and Tong. This is an open-access article distributed under the terms of the Creative Commons Attribution License (CC BY). The use, distribution or reproduction in other forums is permitted, provided the original author(s) and the copyright owner(s) are credited and that the original publication in this journal is cited, in accordance with accepted academic practice. No use, distribution or reproduction is permitted which does not comply with these terms.
*Correspondence: Jie Ren, b2xpdmlhcmVuakB6dWVsLmVkdS5jbg==
Disclaimer: All claims expressed in this article are solely those of the authors and do not necessarily represent those of their affiliated organizations, or those of the publisher, the editors and the reviewers. Any product that may be evaluated in this article or claim that may be made by its manufacturer is not guaranteed or endorsed by the publisher.
Research integrity at Frontiers
Learn more about the work of our research integrity team to safeguard the quality of each article we publish.