- 1School of Environmental Science and Engineering, Southern University of Science and Technology, Shenzhen, China
- 2Power China Huadong Engineering Corporation Limited (HDEC), Hangzhou, China
- 3School of Civil Engineering, University of Leeds, Leeds, United Kingdom
In response to urban pluvial flooding and pollution, the Chinese government proposed a “sponge city” policy in 2013 that aims to improve urban stormwater management and promote sustainable urban development. However, at present, sponge city construction is still in its exploratory stage. It is still not clear which models are capable of simulating the six key processes (i.e., “retention,” “infiltration,” “storage,” “purification,” “discharge,” and “utilization”) of sponge city practices. Its various benefits (e.g., social, economic and environmental benefits) have not yet been systematically investigated in the context of the sponge city. In this study, we reviewed and compared 19 urban stormwater management models (including 13 hydrological models and 10 decision-support tools, as there are 4 overlap ones) and investigated their application in China. Firstly, we examined the mechanisms behind the hydrological models and compared the abilities of the models to simulate various processes. Secondly, we analyzed what kinds of benefits can be addressed by these decision support tools (DSTs). Finally, we discussed the applications and limitations of the models in various climate zones in China. The findings suggest that none of the models consider the impact of climate change on the sponge city practices (SCP) and none of DSTs can simulate the negative performance of SCP. Furthermore, the lack of sufficient databases in China limited the applications of many of the models. Additionally, we found that the hydrological processes corresponding to “storage” were given more attention in southern China, and “infiltration” of stormwater was of greater concern in northern China. In the context of sponge city construction, this paper provides suggestions for future model development of urban stormwater management in China, such as the development of a stormwater database and the incorporation of long-term climate change impacts into the model.
1 Introduction
Rapid urbanization increases impervious surface area and reduces opportunities for stormwater infiltration, altering the urban hydrological cycle (Oudin et al., 2018; Hou et al., 2019; Rosenberger et al., 2021; Wang and Palazzo et al., 2021). In the context of climate change, extreme rainfall events have increased in frequency, resulting in greater urban flooding potential, non-point source pollution, and more frequent discharges from combined sewer overflows (Hou et al., 2020; Mishra et al., 2021, Yang et al., 2021). Due to the inadequacy of conventional stormwater management systems, countries have begun to explore novel and more sustainable stormwater management techniques such as low impact development (LID), best management practices (BMPs), and green infrastructure (GI) in the United States, water sensitive urban design (WSUD) in Australia, sustainable drainage systems (SUDs) in the United Kingdom, low impact city design and development (LIUDD) in New Zealand, as well as the active, beautiful, clean (ABC) water program in Singapore (Chang et al., 2018; Zhang et al., 2019; Kazantsev et al., 2020; Islam et al., 2021). In 2013, the Chinese government proposed its own urban water management programme, the national “sponge city” initiative, meaning that cities can promote infiltration, retain, store, and purify stormwater like a sponge to utilize stormwater resources and alleviate the problems of flooding, water shortages, and pollution (Li H et al., 2017; Wang et al., 2017). Such SCP have significantly impacted urban hydrological processes and stormwater-resource utilization in China (Suppakittpaisarn et al., 2017; Traver and Ebrahimian, 2017; Li C et al., 2019). They can increase the permeability of urban surfaces and infiltration, alleviate the negative impacts caused by stormwater runoff (e.g., flooding and non-point source pollution), and also replenish groundwater (Fanelli et al., 2017). Moreover, through SCP, stormwater resources are collected and treated in sustainable, cost-effective ways for urban public and residential applications such as firefighting, road cleaning, and toilet flushing (Sartor et al., 2018; Grytsenko et al., 2020). “Sponge city” has been mentioned in the sixth IPCC report as one of effective practices. Chinese government also strongly promotes it in the new urban planning and defined six words to mark the functions of sponge city (i.e., “retention,” “infiltration,” “storage,” “utilization,” “purification” and “discharge”) (Xia et al., 2017). The six processes have the specific Chinese words for each of them, which are proposed in an official document issued by the Chinese State Council (i.e., Zhi for retention, Shen for infiltration, Xu for storage, Yong for utilization, Jing for purification, and Pai for discharge) (Liu H et al., 2017). They are used to describe the process from rainwater dropped from the sky to it is discharged through the surface or pipes. However, the practices lack the scientific instructions for the successful implementation.
Numerous studies have applied hydrological models to simulate processes and evaluate the cost effectiveness of various SCP configurations. For example, Li et al. (2018) used Hydrus-1D to investigate the operational effects of bioretention on tanks in Xi’an, Shanxi Province. Zhang et al. (2021) used SWMM and SUSTAIN to assess the cost effectiveness of SCP in Dali, Yunnan Province. Additionally, DSTs have supported policymakers or stakeholders in making better and more effective decisions. For example, the Spatial Suitability ANalysis TOol (SSANTO) was used in Melbourne, Australia to involve stakeholders in urban planning through integration of WSUD (Kuller et al., 2019), and STORMKIT was applied in the Australian state of Victoria to assist in the design of a stormwater system using minimum input requirements (Imteaz, 2015). A few studies have reviewed or compared model applications in multiple situations. For example, Jayasooriya and Ng (2014) selected 10 models and conducted a series of comparisons relating to data requirements, model accuracy, simulation approaches etc., and Elliott and Trowsdale (2007) compared 10 models in terms of application range, calculation methods, contaminant removal, SCP devices, and user interfaces. Haris et al. (2016) reviewed 12 models to explore their functionality, accessibility, characteristics, and components.
These studies have, however, not compared the application of stormwater models in the context of China’s sponge city policy. For example, how do models or algorithms embedded in stormwater models correspond to the six mandated functions for sponge city construction? The variables and parameters used as inputs to the models are not yet summarized for sponge city policymakers and other related stakeholders. Moreover, are current DSTs capable of supporting a cost benefit analysis of sponge city construction? These questions require further elucidation, hence our study has investigated the performance of 19 urban stormwater management models in terms of hydrological simulations and decision support in the context of the sponge city. Given that the six hydrological processes (i.e., “retention,” “infiltration,” “storage,” “utilization,” “purification” and “discharge,” Figure 1) are mandated in the construction of sponge cities (Jia et al., 2017), we have analyzed hydrological simulations based on these six processes. Additionally, sponge city practices provide environmental, economic and social benefits, an understanding of which can provide support to decision making. However, their relevant benefits in China have not been systematically summarized and evaluated (He et al., 2019; She et al., 2021). Therefore, our study also aims to evaluate DST from this perspective.
2 Materials and Methods
In this paper, we firstly reviewed the stormwater modelling and tools, which are currently assessed worldwide. Then we found the assessed their applications in China based on the published articles.
Firstly, we identified 33 stormwater models from published articles, conference papers, project websites, government technical reports and modeling guidelines. Amongst these, we selected ones simulating different SCP processes which are currently available and widely used. After manually screening, we identified 19 models, which detail information and their advantages and shortcomings are included in the Supplementary Tables. Based on their main features, we divided the models into two types: hydrological models (13 no.) and DSTs (10 no.). Amongst the 19 models, LIDRA, L-THIA LID, MUSIC, and SUSTAIN were able to model both hydrological and DST functions, hence were included in both categories. Then we compared the mechanisms and the required input variables of the hydrological models with regard to retention, infiltration, storage, utilization, purification, and discharge. We also compared the DSTs in terms of their ability to model social, economic, and environmental benefits.
Secondly, to assess the application of these 19 models in Chinese sponge city construction, we reviewed related articles in Web of Science and Scopus, excluded the review articles. Given that LID practices were firstly applied in Beijing since 2000, articles published from January 1, 2000 were investigated. The search terms in this study were “Model name + Stormwater or Rainwater”. After the manual filtering, 199 case studies were retrieved.
3 Results
3.1 Hydrological Performance of Models
The internal mechanisms and input variables for simulating the six main processes of each hydrological model are summarized in Table 1. The mathematical algorithms associated with different processes are displayed in the mechanism row. Some processes are not explicitly described in the user manual of several reviewed models, and these are shown as blanks. The comparison of the six main processes simulated by these models is described below.
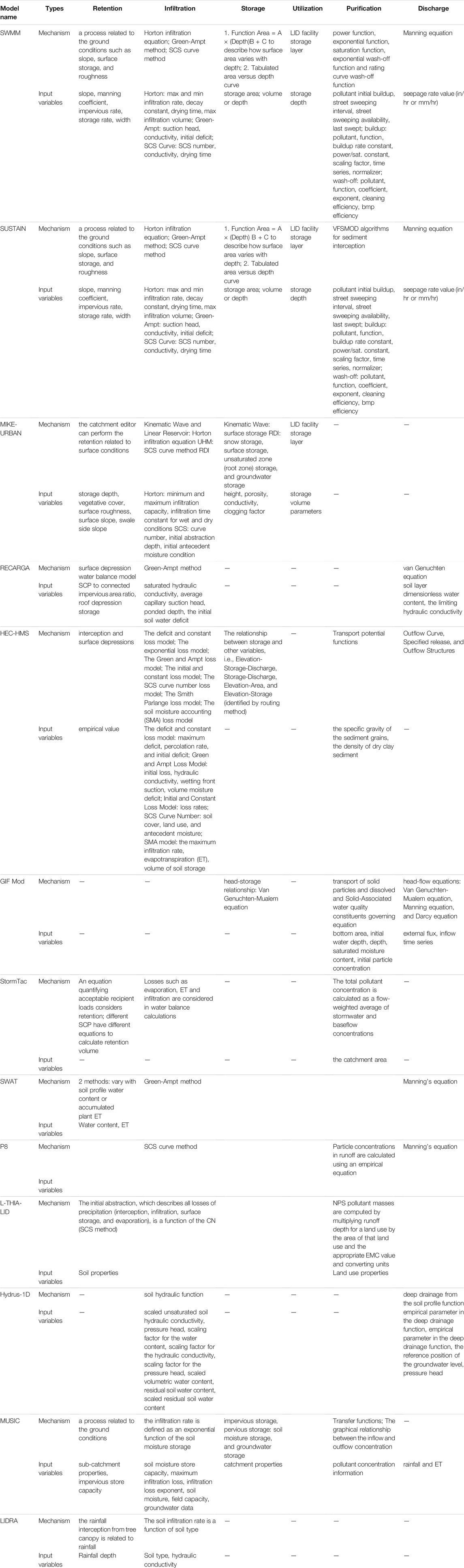
TABLE 1. Comparison of the mechanisms and input variables of hydrological models in terms of the six mandated processes in sponge city design.
3.1.1 Retention
Retention is an important component in calculating surface runoff, and it is usually embedded in the rainfall-runoff module (i.e., SWMM, SUSTAIN, MIKE-URBAN, L-THIA LID, and MUSIC) (Zhang D et al., 2018; Liu et al., 2019). Retention is regarded as a process related to ground conditions such as slope, surface storage and roughness (Sharior et al., 2019). The input parameters of the models are similar, including slope, surface manning coefficient, storage depth, vegetative cover and width. However, specific algorithms for retention are not separated from the runoff calculation.
The retention process in the RECARGE and HEC-HMS models include interception and surface depression. The former calculates surface depression as a function of zone thickness and storage layer thickness based on the water balance model (Tu A et al., 2020), while the latter uses an empirical equation to estimate the interception and surface depression as an empirical value may vary with different surface conditions (Roy et al., 2013; Gebre, 2015). The SWAT model can estimate retention based on soil profile water content or accumulated plant evapotranspiration (Elçi, 2017; Cheng et al., 2021). The LIDRA model considers retention as tree canopy interception, which is related to rainfall depth (Horton, 1919). Retention cannot be simulated in Hydrus-1D because the model only considers the longitudinal movement of stormwater in the soil layer and ignores its horizontal movement on the surface. GIF Mod and P8 lack specific algorithms to calculate the retention process.
3.1.2 Infiltration
With the exception of GIF Mod, all other models can simulate infiltration. LIDRA, MUSIC and Hydrus-1D use specific methods related to soil properties to calculate infiltration, whilst the others estimate infiltration based on algorithms embedded in the surface runoff modules. The most widely used methods are the Green-Ampt method and the Soil Conservation Service (SCS) curve method. SWMM, SUSTAIN, HEC-HMS and MIKE-URBAN have several methods to calculate infiltration (see below). StormTac calculates infiltration as a residual of the water balance model. RECARGA, L-THIA LID and P8 use the SCS curve method and SWAT uses the Green-Ampt method.
In SWMM and SUSTAIN, infiltration can be calculated using the Horton infiltration equation, the Green-Ampt method or the SCS curve method. Users can choose a specific method according to their research needs and data availability. For example, the Horton infiltration equation is applicable when exploring a small watershed or when soil data is inadequate; however, a limitation is that it does not consider the conditions of the saturated and unsaturated zones (Wang and Chu, 2020; Yang et al., 2020). The Green-Ampt method has strict data requirements i.e., requires comprehensive soil data (Huo et al., 2020; Salifu et al., 2021). The SCS curve method is applicable to large watersheds, but a limitation is that it does not reflect the influence of rainfall intensity and rainfall processes on runoff (Nile et al., 2018; Lian et al., 2020).
HEC-HMS calculates infiltration based on loss models, e.g., the exponential loss model, Green and Ampt loss model or the Smith Parlange loss model (Zema et al., 2017). Different models corresponded to various parameters, and users can choose the appropriate model according to research needs or data availability.
The algorithm to calculate infiltration in MIKE-URBAN is incorporated in the four surface runoff models, which are Time-Area Method, Kinematic Wave Method, Linear Reservoir Method, Unit Hydrograph Method (UHM), and one continuous hydrological model called MOUSE Rainfall Dependent Infiltration (RDI) (DHI, 2017). In the Time-Area method, infiltration is included as runoff loss, and it is not defined separately (Akram et al., 2014; Fariborzi et al., 2019). Both the Kinematic Wave and Linear Reservoir methods apply the Horton infiltration equation to calculate the infiltration (Yin et al., 2020). For UHM, the SCS Curve method is used to estimate the infiltration (Kocsis et al., 2020; Strapazan et al., 2021). In RDI, there are two methods for simulating the infiltration process: Fast Response Component (FRC) and Slow Response Component (SRC) (Zhang S et al., 2018). If the soils’ previous hydrological conditions are considered, users can choose the SRC method (Ohlin Saletti, 2021).
L-THIA LID cannot calculate infiltration based on independent methods. The infiltration coefficient in StormTac can be estimated from its relationship with runoff. LIDRA estimates soil infiltration rate from the hydraulic conductivity of different soil types (Rawls et al., 1982). In MUSIC, the infiltration rate of the soil can be calculated from an exponential function of soil moisture storage (https://wiki.ewater.org.au/display/MD6). When the soil water reserve is empty, the infiltration rate reaches its maximum value, and when the soil water reserve is full the infiltration rate gradually decreases to its minimum value. Hydrus-1D uses a soil hydraulic function which can more precisely simulate infiltration compared with the above-mentioned models, however it requires more input variables than other models (Feitosa and Wilkinson, 2016; Hilten et al., 2008; Jiang et al., 2010; Tu et al., 2021).
3.1.3 Storage
The calculation of storage is mainly based on two methods. Some models (e.g., SWMM) directly calculate storage by detecting its relationship with other parameters (e.g., catchment area and depth) (Rossman et al., 2015). Some other models (e.g., MUSIC) divide storage into different parts and calculate each part based on various corresponding methods (https://wiki.ewater.org.au/display/MD6), as shown below.
The storage curve is used in SWMM and SUSTAIN to calculate storage based on the relationship between storage and water depth (Yazdi et al., 2019; Tu et al., 2020). The former uses a linear equation to calculate storage area, while the latter applies a tabulated method (Liu et al., 2018; Deitch and Feirer, 2019); both require water depth or volume as input variables.
GIF Mod applies the Van Genuchten-Mualem equation to estimate catchment wide storage, which identifies catchment storage based on the relationship between storage and water head (Onoja et al., 2019; Weber et al., 2020). In HEC-HMS storage is computed by various equations revealing the relationship between storage and other variables such as catchment area, elevation and discharge (https://www.hec.usace.army.mil/confluence/hmsdocs/hmstrm).
In MIKE-URBAN storage is calculated using the Kinematic Wave method, which considers the surface storage as three parts: snow surface, unsaturated zone (“root zone”) and ground water in RDI (Hernes et al., 2020; Thrysøe et al., 2021). The input variables are height, porosity, conductivity and clogging factor.
In MUSIC, storage is divided into impervious storage and pervious storage. Impervious storage is a user defined constant, and pervious storage consists of soil moisture storage and groundwater storage. The specific equations are however not publicly available (https://wiki.ewater.org.au/display/MD6).
3.1.4 Purification
The number of pollutants that can be removed from stormwater by SCP is difficult to accurately simulate. Amongst the models we reviewed only MUSIC and L-THIA LID are able to simulate purification performance by different types of SCP. However, most models (such as SWMM, SUSTAIN, HEC-HMS, GIF Mod, L-THIA LID, StormTac, and P8) can calculate the concentration of sedimentation or pollutants.
For pollutant accumulation models such as SWMM and SUSTAIN, pollutant accumulation and wash-off are simulated based on the accumulation and wash-off functions (Johannessen et al., 2019). However, both functions only estimate the pollutant reduction within the runoff flow volume (Rossman et al., 2015). SUSTAIN uses the Vegetative Filter Strip Model algorithms for sediment interception, which is more accurate than SWMM (Shoemaker et al., 2009). The input variables in each process are pollutant initial buildup, street sweeping interval, street sweeping availability, and last swept. In StormTac, the total pollutant concentration is calculated as a flow-weighted average of stormwater and baseflow concentrations (Lindgren, 2019; Wu J et al., 2021). In P8, particle concentrations in runoff are calculated using an empirical equation (Walker, 1990). For pollutant transporting models, GIF Mod uses a governing equation to calculate the transport of solid particles and other water quality constituents (Massoudieh et al., 2017). HEC-HMS calculates pollutant transport with potential functions (MacAsieb et al., 2021).
In L-THIA LID, NPS pollutant concentration masses can be calculated by the runoff depth, multiplying the area and the appropriate Event Mean Concentration (EMC) value (Engel and Harbor, 2017). The treated pollutants by SCP was estimated by the treatment node in MUSIC, using the transfer functions (Gavrić et al., 2019). These transfer functions calculate the effluent concentration of stormwater based on the simple graphical relationship between inflow and outflow concentrations. Therefore, compared with other models, MUSIC can simulate stormwater quality more accurately.
Hydrus-1D and LIDRA do not include algorithms to simulate water quality.
3.1.5 Utilization
Here, it should be noted that the collection function of SCP, such as rainwater collected by rainwater harvesting tanks or cisterns, is regarded as a function of “utilization” in the context of China’s sponge cities. Therefore, only models with rainwater harvesting simulation capability (e.g., SWMM, SUSTAIN and MIKE-URBAN) were investigated in this category. These models have specific SCP modules, and users can define the collecting capacity by relevant input variables such as storage layer parameters. For example, Campisano et al. (2017) estimated rainwater harvesting and evaluated the performance of rain tanks based on SWMM. They found that SWMM overestimated this value, especially for rain tanks smaller than 2 m3. Li et al. (2021) simulated rainwater harvesting and reuse of rain barrels in Brentwood watershed (Texas, United States) and designed the optimizing parameters of rain barrels for efficient stormwater management, based on SWAT.
However, utilization functionality is still in development and there are different interpretations of it. Some researchers found stormwater utilization is associated with stormwater storage capacity, domestic water demand and current technologies (Hamdan, 2009; Nnadi et al., 2015). For example, the capability of stormwater storage is related to the size of rain tank or reservoir (Vargas et al., 2019; Kim et al., 2021). Domestic water demand can be affected the public policies and local population and industries (Takagi et al., 2018; Fernandes et al., 2020). Sustainable stormwater management can alleviate the water scarcity with some degree (Morales-Torres et al., 2016). Additionally, the development of advanced purification technologies and the new materials can also influence the stormwater utilization (Kolavani and Kolavani, 2020; Awang Ali et al., 2021).
3.1.6 Discharge
Discharge describes the movement of stormwater through drainage pipes, and models calculate this using various hydraulic equations (Chang et al., 2015; Cao et al., 2021). SWMM and SUSTAIN calculate the runoff routing in the pipeline based on the St. Venant flow equations for the dynamic wave and kinematic wave. Regarding the steady flow, the joint solution of Manning and continuous equation is used to calculate the flow rate (Rossman, 2015). SWAT uses the Manning equation to model stormwater movement in drainage systems, while P8 applies the curve number method to simulate runoff from pervious and indirectly connected with imperious surface (Minnesota Stormwater Manual). RECARGA and GIF Mod use the van Genuchten equation. The MIKE-URBAN module features an advanced Real-Time Control (RTC) simulation capability for urban drainage and sewer systems in the form of overflow pipes, weirs, orifices, etc. The stormwater between two nodes can be calculated with Muskingum Cunge method in MUSIC. The outflow hydrograph and pollutograph can be estimated by the continuity of mass equation (Peters, 2012). Hydrus-1D and LIDRA do not consider stormwater discharge in the urban drainage system. The processes of retention, infiltration, storage, and discharge are well simulated in SWMM, SUSTAIN, MIKE-URBAN, and MUSIC. The algorithms adopted in SWMM and SUSTAIN to simulate the above processes are similar, such that the required input parameters are the same. MIKE-URBAN can more accurately simulate the movement of stormwater in the drainage system given that it adapts five hydraulic methods depending on the process in question. The purification process, which corresponds to the improvement of water quality and the removal of pollutants, was fully simulated by MUSIC, i.e., it simulates the entire removal of various pollutants. SWMM and SUSTAIN only model the reduction in runoff mass load caused by the reduced runoff flow volume (Rossman et al., 2015; Shoemaker et al., 2009). None of these models can simulate the process of stormwater utilization and this is an area requiring further research.
3.2 The Performance of Stormwater Management Models in Decision Support and Policy Making
Amongst the 10 selected DSTs there were two free online tools, one free GIS-based tool, two commercially available software programs, two free software programs, and three free MS Excel-based tools. Specially MUSIC, WinSLAMM, UrbanBEATS and E2 STORMED DST, as stand-alone software, have more complex operating processes than the online and spreadsheet tools. The environmental, economic and social benefits evaluated by the 10 DSTs are summarized in Table 2.
3.2.1 Environmental Benefits
The environmental benefits of SCP include improved hydrological performance (Dagenais et al., 2017; Spahr et al., 2020) such as stormwater quality improvement, flood mitigation, runoff reduction and groundwater recharge, as well as indirect benefits related to ecosystem services (Andersson et al., 2015; Dietrich and Rahul Yarlagadda, 2016; Gallo et al., 2020). Improved ecosystem services include adjustment to the local microclimate, carbon sequestration and storage, biodiversity conservation and restoration, and air pollution (Gómez-Baggethun and Barton, 2013; Ando and Netusil, 2018; Kazak et al., 2018).
Most models containing DST capability only address the hydrological aspects of environmental benefits, for example the reduction of water quantity and the improvement of water quality. LIDRA, for example, evaluates the benefits related to runoff reduction in various SCP scenarios. SWMM, SUSTAIN, MUSIC, winSLAMM, WMOST, and UrbanBEATS can evaluate the benefits associated with runoff reduction and water-pollutant removal by various SCP.
The benefits associated with ecosystem services may also be assessed. For example, the E2 STORMED DST can evaluate CO2 reduction and energy benefits resulting from SCP. Additionally, the GI valuation toolkit can incorporate climate change adaptation and mitigation, as well as the biodiversity conservation related to SCP.
3.2.2 Economic Benefits
There are three main methods for evaluating the economic benefits of SCP. The first method calculates the life cycle cost of various SCP configurations. E2 STORMED DST, GI Valuation Toolkit, LIDRA and MUSIC utilize this method. The second method generates a cost-benefit curve or report to help with decision making, and is used by SUSTAIN, WinSLAMM and WMOST. SUSTAIN has an optimization module to generate the most cost-effective scenarios based on the scatter search method and the non-dominated sorting genetic algorithm II method. Regardless of the cost related to improving water quantity, WinSLAMM provides the most cost-effective solutions for urban stormwater quality requirements (Pitt et al., 2002). In WMOST, a Pareto Frontier or trade-off curve can be generated by setting control experiments. The third method is associated with net present value (NPV), which is a widely used method to assess financial viability. This method incorporates the time value of money into decision making based on discounted cash flow techniques (Ye and Tiong, 2000). In the GVC this method is adopted to evaluate economic benefits.
In terms of life cycle costs MUSIC can calculate more types of cost compared with E2 STORMED, LIDRA and L-THIA LID. More specifically, the life cycle cost of MUSIC includes the total acquisition cost, annual establishment cost, typical annual maintenance cost, renewal/adaptation cost, decommissioning cost and nominal cost. The types of cost in other models are mainly limited to construction costs and maintenance costs, for example LIDRA and L-THIA LID.
3.2.3 Social benefits
The social benefits related to SCP involve the improvement of aesthetics, public health, human welfare, public acceptability and city sustainability, noise reduction and entertainment (Keeley et al., 2013; Chini et al., 2017; BenDor et al., 2018; Bell et al., 2019). Only two tools amongst the reviewed models were found to be able to evaluate social benefits: UrbanBEATS and GI Valuation Toolkit. UrbanBEATS is a spatial planning tool with advanced visual outputs; it considers the influence of urban population density and the urban drainage system to help stakeholders make better decisions. The GI Valuation Toolkit can evaluate the improvements in community performance, health and wellbeing, tourism and recreation, and leisure related to SCP.
In conclusion, with the exception of UrbanBEATS, all other tools were found to be able to evaluate the economic benefits of SCP by calculating the NPV of the sponge system or by identifying the cost of SCP under various scenarios. Amongst the reviewed models only the GI Valuation Toolkit and UrbanBEATS can evaluate social benefits. At present, the methods used to evaluate the social benefits of SCP are mainly indicator based screening and quantification (Dagenais et al., 2017; He et al., 2019; Li H et al., 2019). Because the original purpose of SCP is to reduce urban runoff and remove pollutants, all models except GVC can evaluate environmental benefits (Steffen et al., 2013; Berland et al., 2017).
4 Case Studies in China
Among the filtered 199 studies, 146 cases with a defined municipal study area were selected. Specially, 66 of the 146 cases are in northern China, while the remainder are in southern China. Only 5 studies were published before 2010, which reflects the increased application of stormwater modeling over the last decade. Moreover, we found that most of studies were based on experiments at community or neighborhood scales. The results were mainly based on monitoring and simulations for single or multiple rainfall events, rather than long-term monitoring or simulations.
Amongst the articles we reviewed there were only seven models applied in China (Figure 2), of which SWMM was the most widely used. SWMM was capable of not only addressing the hydrological performance of SCP but, when coupled with other land use planning software, achieving real-time stormwater management and comprehensive performance evaluation (Li C et al., 2019; Zeng et al., 2021). Hydrus-1D was used to simulate infiltration-based SCP such as green roofs, permeable pavements, and bioretention (Qin et al., 2016; Fu et al., 2020; Li et al., 2020). MIKE-URBAN was useful for simulating urban stormwater drainage system performance, such as urban waterlogging (Luan et al., 2018). SUSTAIN was applied in both SCP hydrological performance assessment and assisting in the planning of SCP (Gao et al., 2015; Zhang et al., 2021). RECARGA was used to simulate the influence of bioretention on the water balance in an expressway service area in China and the appropriate parameters for bioretention was identified (Gao et al., 2018). HEC-HMS was applied to compare and analyze comprehensive regulation effects of three different storm water management scenarios (Liu et al., 2020). SWAT performed the cost-benefit analysis of SCP in Xiangxi River, China (Liu et al., 2014).
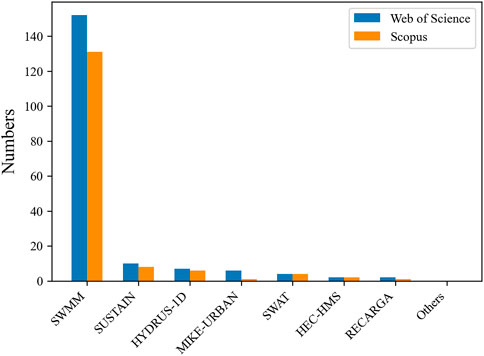
FIGURE 2. Number of publications relating to Sponge City modelling between January 1st, 2000 and July 3rd, 2021.
Early studies focused on the simulation of water quality and water quantity, as well as evaluation of the environmental benefits of SCP in terms of runoff and pollution control (Meng et al., 2014; Li et al., 2016). Outcomes identified optimal scheme choice and spatial layout amongst different configurations of SCP (Liao et al., 2015; Xu et al., 2017). Amongst the literature investigated, Guo et al. (2008) conducted the first stormwater modeling application study in Beijing with SWMM, and analyzed the different rainfall-runoff characteristics of various rainfall densities. Recently, studies related to improving the accuracy, efficiency, and robustness of hydrological models have been highlighted (Pang et al., 2020; Yin et al., 2020; Tang et al., 2021). Moreover, these stormwater management models are increasingly coupled with other platforms or models to achieve more comprehensive functions or to run simulations at larger spatial scales (Duan and Gao, 2019; Liang et al., 2020; Xu et al., 2021). Hydrological models were also integrated with remote sensing techniques, and neural networks have become more popular (Wang et al., 2021; Wu Z et al., 2021).
We further summarized the simulations of the six physical processes involved in the 199 case studies (Figure 3). Only 2 cases refer to utilization, and both of these used the SWAT model. Most studies focused on infiltration (38 no.) and discharge (32 no.). Thus, these two physical processes are well simulated in current models, whereas other processes such as storage and utilization were relatively uncommon.
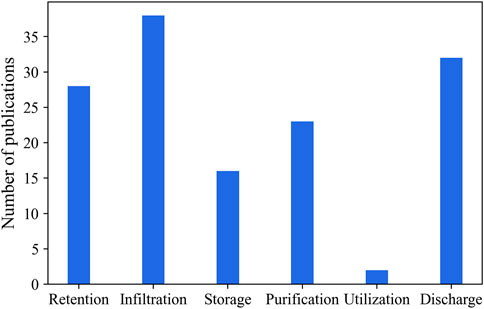
FIGURE 3. Breakdown of the six hydrological processes included in Sponge City case studies in China.
Amongst the 199 case studies, 146 cases focused on specific study areas. We extracted these and further summarized model applications to climatic zones in China. The climate classification map is based on data retrieved from the Resource and Environment Science and Data Center (Figure 4, https://www.resdc.cn/data.aspx?DATAID=243). It may be seen that these cases are mainly distributed in eastern China where precipitation is higher and sponge city pilot projects are mostly located. The cases were spread amongst 57 cities, in which Beijing has the largest portion, corresponding to stormwater-resource management demands (Zhang D et al., 2018).
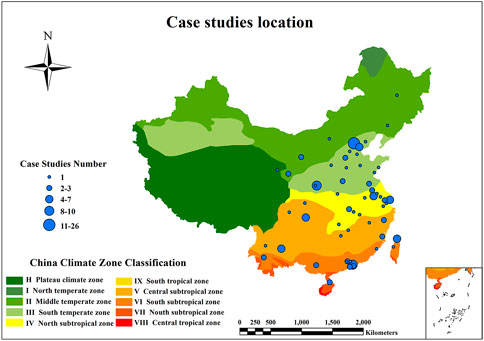
FIGURE 4. Spatial distribution of case studies, retrieved from Web of Science and Scopus between January 1st, 2000 and July 3rd, 2021.
We further analyzed these cases and found that in northern China hydrological model use tends to focus on simulation of infiltration processes of SCP (Luan et al., 2017; Li et al., 2018). Precipitation in northern China is low, and unsaturated zones can reach hundreds of meters. As a result, rainfall exhibits low infiltration and field capacity is rarely reached (Han and Banin, 1999; Li H et al., 2019). Improving soil infiltration capacity is an effective way of reducing runoff, recharging groundwater and reducing surface water flooding (Li Z et al., 2017). In the southeast coastal area, application of hydrological models focused on simulating the storage of SCP (Qin et al., 2016; Mao et al., 2017). Because rainfall is more abundant and groundwater levels are high, soil moisture content is also high in the early stages. It is vital therefore to increase stormwater storage capacity through SCP in order to reduce flood risk.
DST functionality has not yet been widely applied in China. At present, sponge city planning mainly relies on a combination of hydrological models and other decision-support methods, such as multi-objective decision making (MODM), analytic hierarchy process (AHP), and a multi-objective evolutionary algorithm (MOEA) (Jia et al., 2015; Xu et al., 2017; Zhu et al., 2021). There are no systematic social and environmental evaluation criteria for sustainable stormwater management in China.
5 Discussion and Conclusion
This research reviewed 19 stormwater models, of which 13 models can perform hydrological simulations and 10 can function as decision support tools. We primarily analyzed the hydrological models for six key processes, as mandated by the Chinese government for sponge city construction. We also compared various DSTs in evaluating the economic, social, and environmental benefits of SCP. Finally, we collected case studies of model applications in China and analyzed the spatial variability and simulation advantages in various climatic zones.
This study reveals some deficiencies in the current simulation for SCP. First, the changes in the nature of SCP over time, such as change in plant coverage and/or the volume of water storage facilities, were not longitudinally studied. In the context of climate change and more intense and frequent rainfall, greater pressure is being placed on the urban ecosystem. Hence, the related hydrological impacts have not been validated by stormwater management models. Observations for vegetation and hydrologic changes need to be recorded to enable improved performance of stormwater management models.
Second, models are known to perform well in different regions of the world, but need further refinement for more meaningful application in sponge city construction in China. For example, the construction standards of rain gardens in China are different to those in other countries, so the parameters related to the capacity of stormwater treatment are also different (Wang et al., 2009). Moreover, these hydrological models cannot fully simulate the entire circulation of stormwater in the context of a sponge city. Case studies in China have mainly focused on reducing stormwater runoff and mitigating stormwater pollution; there is no coherent analysis of how stormwater replenishes groundwater, thus requires further research. Additionally, how stormwater is utilized, such as toilet flushing and irrigation of public green spaces, is still unknown. The model algorithms for understanding how SCP affect the six processes also need to be further optimized.
Third, it should be noted that rainwater harvesting by rainwater tanks or cisterns is classified as “utilization” in the context of China’s sponge cities (Peng J et al., 2018; Peng S et al., 2018; Wei et al., 2019). How the collected water is then used (e.g., toilet flushing and urban irrigation) cannot be simulated by current stormwater management models, to our best knowledge. In the “utilization” category, we’ve clarified how to assign collected rainwater or treated stormwater (Takagi et al., 2018; Fernandes et al., 2020; Hajani and Rahman, 2014).
Finally, current DSTs only focus on evaluating the positive benefits of SCP and ignore the negative effects of SCP. Many studies have shown that SCP can also have negative effects. For example, rain gardens may accidentally increase the phosphorus content in stormwater (Hatt et al., 2009), and wetlands may cause eutrophication of water bodies (Heal et al., 2006). Reservoirs may act as a sink for pollution, endangering health in wildlife and humans (Paus et al., 2014). Data on economic costs in DSTs are lacking in China and require further study. Another limitation of DSTs is the lack of social-benefit assessment modules. Modules that evaluate the social benefits of SCP would assist in the long-term implementation of more SCP in communities. In addition, combining increased stakeholder participation with DSTs is becoming a crucial aspect of sponge city construction (Cousins, 2017; Qiao et al., 2018); DSTs are still not fully used in policy and decision-making.
Overall, for Chinese sponge cities, most models are capable of simulating the hydrological performance of SCP, however the application of DSTs is relatively poor and ineffective in China. Decision-making involves many social, economic, and political factors which may be different from the countries in which the DSTs were developed. Therefore, developing models that can adapt to China’s sponge city conditions is crucial. Our review of case studies application in China show that stormwater models use is mainly distributed to central and eastern China. In northern China, studies tend to focus on simulating infiltration, whilst in southern China studies focus on the storage functions of SCP. This finding is significant in terms of selecting an appropriate model according to climatic zone and simulation functions. In summary, in this study, we have evaluated and compared the physical mechanisms embedded in stormwater models based on water retention, infiltration, storage, utilization, purification, and discharge; these factors are important in sponge city construction in China. Additionally, our study explored the social, environmental, and economic benefits of sponge city practices, which can be simulated by current decision-support tools. Furthermore, the application of these tools was also investigated in various climatic zones in China. The study not only improves the understanding of processes involved in the construction of a sponge city, but also provides insight into the development of future stormwater models.
Author Contributions
QL wrote the manuscript, built the method, acquired data, and conducted data processing and analysis. WC conceived the study, built the method, and wrote the manuscript together. JL conceived the study, acquired the funding, and contributed to the manuscript writing. MT, YT and ZT contributed to the manuscript editing.
Funding
This work was supported by the National Key R&D Program of China (No. 2018YFE0206200). It was also supported by the High-level Special Funding of the Southern University of Science and Technology (Grant Nos. G02296302 and G02296402).
Conflict of Interest
Author Dr. YT is employed by Power China Huadong Engineering Corporation Limited.
The remaining authors declare that the research was conducted in the absence of any commercial or financial relationships that could be construed as a potential conflict of interest.
Publisher’s Note
All claims expressed in this article are solely those of the authors and do not necessarily represent those of their affiliated organizations, or those of the publisher, the editors and the reviewers. Any product that may be evaluated in this article, or claim that may be made by its manufacturer, is not guaranteed or endorsed by the publisher.
Acknowledgments
We acknowledge Dr. Wenfang Cao of the School of Environmental Science and Engineering, Southern University of Science and Technology (SUSTech) for helping edit the figures.
Supplementary Material
The Supplementary Material for this article can be found online at: https://www.frontiersin.org/articles/10.3389/fenvs.2021.816093/full#supplementary-material
References
Akram, F., Rasul, M. G., Masud, M., Khan, K., Imam, S., and Amir, I. (2014). Comparison of Different Hydrograph Routing Techniques in XPSTORM Modelling Software : A Case Study. Int. J. Environ. Ecol. Geol. Mining Eng. 8 (3), 213–223. doi:10.5281/zenodo.1093034
Andersson, E., McPhearson, T., Kremer, P., Gomez-Baggethun, E., Haase, D., Tuvendal, M., et al. (2014). Scale and Context Dependence of Ecosystem Service Providing Units. Ecosystem Services 12, 157–164. doi:10.1016/j.ecoser.2014.08.001
Ando, A., and Netusil, N. (2018). Valuing the Benefits of Green Stormwater Infrastructure. Oxford: Oxford Research Encyclopedia of Environmental Science. Available at: https://oxfordre.com/environmentalscience/view/10.1093/acrefore/9780199389414.001.0001/acrefore-9780199389414-e-605 (Accessed June 27, 2021).
Awang Ali, A. N., Bolong, N., and Abdul Taha, N. (2021). A Review on the Application of Granular Filter Media and the Utilization of Agro-Industrial Wastes for Stormwater Quality Improvement. Jurnal Teknologi. 83 (4), 75–90. doi:10.11113/jurnalteknologi.v83.15159
Bach, P. M., Kuller, M., McCarthy, D. T., and Deletic, A. (2020). A Spatial Planning-Support System for Generating Decentralised Urban Stormwater Management Schemes. Sci. Total Environ. 726, 138282. doi:10.1016/j.scitotenv.2020.138282
Bean, R., Pitt, R. E., Pitt, R. E., Voorhees, J., and Elliott, M. (2021). Urban Tree Rainfall Interception Measurement and Modeling in WinSLAMM, the Source Loading and Management Model. Jwmm. 2021, 29. doi:10.14796/jwmm.c475
Bell, C. D., Spahr, K., Grubert, E., Stokes-Draut, J., Gallo, E., McCray, J. E., et al. (2019). Decision Making on the Gray-Green Stormwater Infrastructure Continuum. J. Sustainable Water Built Environ. 5 (1), 04018016. doi:10.1061/jswbay.0000871
BenDor, T. K., Shandas, V., Miles, B., Belt, K., and Olander, L. (2018). Ecosystem Services and U.S. Stormwater Planning: An Approach for Improving Urban Stormwater Decisions. Environ. Sci. Pol. 88 (July), 92–103. doi:10.1016/j.envsci.2018.06.006
Berland, A., Shiflett, S. A., Shuster, W. D., Garmestani, A. S., Goddard, H. C., Herrmann, D. L., et al. (2017). The Role of Trees in Urban Stormwater Management. Landscape Urban Plann. 162, 167–177. doi:10.1016/j.landurbplan.2017.02.017
Campisano, A., Catania, F. V., and Modica, C. (2017). Evaluating the SWMM LID Editor Rain Barrel Option for the Estimation of Retention Potential of Rainwater Harvesting Systems. Urban Water J. 14 (8), 876–881. doi:10.1080/1573062X.2016.1254259
Cao, Y., Xu, M., Ni, P., and Mei, G. (2021). Physical and Numerical Modelling of Infiltration from Drainage Holes for Perforated Storm Sewer. Acta Geotech. 2021, 1–17. doi:10.1007/s11440-021-01247-0
Castiglia Feitosa, R., and Wilkinson, S. (2016). Modelling green Roof Stormwater Response for Different Soil Depths. Landscape Urban Plann. 153, 170–179. doi:10.1016/j.landurbplan.2016.05.007
Chang, N.-B., Lu, J.-W., Chui, T. F. M., and Hartshorn, N. (2018). Global Policy Analysis of Low Impact Development for Stormwater Management in Urban Regions. Land Use Policy. 70 (January), 368–383. doi:10.1016/j.landusepol.2017.11.024
Chang, T.-J., Wang, C.-H., and Chen, A. S. (2015). A Novel Approach to Model Dynamic Flow Interactions between Storm Sewer System and Overland Surface for Different Land Covers in Urban Areas. J. Hydrol. 524, 662–679. doi:10.1016/j.jhydrol.2015.03.014
Cheng, J., Gong, Y., Zhu, D. Z., Xiao, M., Zhang, Z., Bi, J., et al. (2021). Modeling the Sources and Retention of Phosphorus Nutrient in a Coastal River System in China Using SWAT. J. Environ. Manage. 278 (P2), 111556. doi:10.1016/j.jenvman.2020.111556
Chini, C., Canning, J., Schreiber, K., Peschel, J., and Stillwell, A. (2017). The green experiment: Cities, green Stormwater Infrastructure, and Sustainability. Sustainability. 9 (1), 105–121. doi:10.3390/su9010105
Cousins, J. J. (2017). Infrastructure and Institutions: Stakeholder Perspectives of Stormwater Governance in Chicago. Cities. 66, 44–52. doi:10.1016/j.cities.2017.03.005
Dagenais, D., Thomas, I., and Paquette, S. (2017). Siting green Stormwater Infrastructure in a Neighbourhood to Maximise Secondary Benefits: Lessons Learned from a Pilot Project. Landscape Res. 42 (2), 195–210. doi:10.1080/01426397.2016.1228861
Deitch, M. J., and Feirer, S. T. (2019). Cumulative Impacts of Residential Rainwater Harvesting on Stormwater Discharge through a Peri-Urban Drainage Network. J. Environ. Manage. 243 (May), 127–136. doi:10.1016/j.jenvman.2019.05.018
DHI (2017). MIKE Urban Collection System - Modelling of Storm Water Drainage Networks and Sewer Collection Systems (User Guide), 423. Horsholm: MIKE Powered by DHI. Available at: http://d.g.wanfangdata.com.cn/Periodical_mkhjbh201605010.aspx.
Dietrich, A., and Rahul Yarlagadda, C. G. (2016). Estimating the Potential Benefits of Green Stormwater Infrastructure on Developed Sites Using Hydrologic Model Simulation. Sustainable Energ. 33 (3), 676–680. doi:10.1002/ep.12428
Duan, H.-F., and Gao, X. (2019). Flooding Control and Hydro-Energy Assessment for Urban Stormwater Drainage Systems under Climate Change: Framework Development and Case Study. Water Resour. Manage. 33 (10), 3523–3545. doi:10.1007/s11269-019-02314-8
Elliott, A., and Trowsdale, S. (2007). A Review of Models for Low Impact Urban Stormwater Drainage. Environ. Model. Softw. 22 (3), 394–405. doi:10.1016/j.envsoft.2005.12.005
Elçi, A. (2017). Evaluation of Nutrient Retention in Vegetated Filter Strips Using the SWAT Model. Water Sci. Technology 76 (10), 2742–2752. doi:10.2166/wst.2017.448
Engel, B., and Harbor, J. (2017). The L-THIA LID Model Description. West Lafayette: Purdue University. Lid. Available at: https://engineering.purdue.edu/mapserve/LTHIA7/lthianew/lidIntro.php.
Fanelli, R., Prestegaard, K., and Palmer, M. (2017). Evaluation of Infiltration‐based Stormwater Management to Restore Hydrological Processes in Urban Headwater Streams. Hydrol. Process. 31 (19), 3306–3319. doi:10.1002/hyp.11266
Fariborzi, H., Sabzevari, T., Noroozpour, S., and Mohammadpour, R. (2019). Prediction of the Subsurface Flow of Hillslopes Using a Subsurface Time-Area Model. Hydrogeol J. 27 (4), 1401–1417. doi:10.1007/s10040-018-1909-9
Feitosa, R. C., and Wilkinson, S. (2016). Modelling Green Roof Stormwater Response for Different Soil Depths. Landscape Urban Plann 153, 170–179. doi:10.1016/j.landurbplan.2016.05.007
Fernandes, S., Bonfante, M. C., de Oliveira, C. T., Maldonado, M. U., and Campos, L. M. S. (2020). Decentralized Water Supply Management Model: a Case Study of Public Policies for the Utilization of Rainwater. Water Resour. Manage. 34 (9), 2771–2785. doi:10.1007/s11269-020-02575-8
Fu, X., Liu, J., Shao, W., Mei, C., Wang, D., and Yan, W. (2020). Evaluation of Permeable brick Pavement on the Reduction of Stormwater Runoff Using a Coupled Hydrological Model. Water 12 (10), 2821. doi:10.3390/w12102821
Gallo, E. M., Bell, C. D., Panos, C. L., Smith, S. M., and Hogue, T. S. (2020). Investigating Tradeoffs of green to Grey Stormwater Infrastructure Using a Planning-Level Decision Support Tool. Water. 12 (7), 2005. doi:10.3390/w12072005
Gao, J., Pan, J., Hu, N., and Xie, C. (2018). Hydrologic Performance of Bioretention in an Expressway Service Area. Water Sci. Technology 77 (7), 1829–1837. doi:10.2166/wst.2018.048
Gao, J., Wang, R., Huang, J., and Liu, M. (2015). Application of BMP to Urban Runoff Control Using SUSTAIN Model: Case Study in an Industrial Area. Ecol. Model. 318, 177–183. doi:10.1016/j.ecolmodel.2015.06.018
Gavrić, S., Leonhardt, G., Marsalek, J., and Viklander, M. (2019). Processes Improving Urban Stormwater Quality in Grass Swales and Filter Strips: A Review of Research Findings. Sci. Total Environ. 669, 431–447. doi:10.1016/j.scitotenv.2019.03.072
Gebre, S. L. (2015). Application of the HEC-HMS Model for Runoff Simulation of Upper Blue Nile River Basin. Hydrol. Curr. Res 06 (02), 1. doi:10.4172/2157-7587.1000199
Gómez-Baggethun, E., and Barton, D. N. (2013). Classifying and Valuing Ecosystem Services for Urban Planning. Ecol. Econ. 86, 235–245. doi:10.1016/j.ecolecon.2012.08.019
Grytsenko, A., Rybalova, O., Matsak, A., and Artemiev, S. (2020). Using of Production Wastes in Stormwater Drainage Purification. Msf. 1006, 194–201. doi:10.4028/www.scientific.net/MSF.1006.194
Guo, L., and Jing, S. (2008). Analysis of Rainfall in Beijing Rivers Based on SWMM. Computer Simulation 2008 (04), 282–285+329. doi:10.3969/j.issn.1006-9348.2008.04.072
Hajani, E., and Rahman, A. (2014). Rainwater Utilization From Roof Catchments in Arid Regions: A Case Study for Australia. J. Arid Environ. 111, 35–41. doi:10.1016/j.jaridenv.2014.07.007
Hamdan, S. M. (2009). A Literature Based Study of Stormwater Harvesting as a New Water Resource. Water Sci. Technology 60 (5), 1327–1339. doi:10.2166/wst.2009.396
Han, F. X., and Banin, A. (1999). Long-term Transformation and Redistribution of Potentially Toxic Heavy Metals in Arid-Zone Soils: II. Incubation at the Field Capacity Moisture Content. Water Air Soil Pollut. 114 (3–4), 221–250. doi:10.1023/A:1005006801650
Haris, H., Chow, M. F., Usman, F., Sidek, L. M., Roseli, Z. A., and Norlida, M. D. (2016). Urban Stormwater Management Model and Tools for Designing Stormwater Management of Green Infrastructure Practices. IOP Conf. Ser. Earth Environ. Sci. 32 (1), 012022. doi:10.1088/1755-1315/32/1/012022
Hatt, B. E., Fletcher, T. D., and Deletic, A. (2009). Hydrologic and Pollutant Removal Performance of Stormwater Biofiltration Systems at the Field Scale. J. Hydrol. 365 (3–4), 310–321. doi:10.1016/j.jhydrol.2008.12.001
He, B.-J., Zhu, J., Zhao, D.-X., Gou, Z.-H., Qi, J.-D., and Wang, J. (2019). Co-benefits Approach: Opportunities for Implementing Sponge City and Urban Heat Island Mitigation. Land Use Policy. 86, 147–157. doi:10.1016/j.landusepol.2019.05.003
Heal, K. V., Hepburn, D. A., and Lunn, R. J. (2006). Sediment Management in Sustainable Urban Drainage System Ponds. Water Sci. Technology. 53 (10), 219–227. doi:10.2166/wst.2006.315
Hernes, R. R., Gragne, A. S., Abdalla, E. M. H., Braskerud, B. C., Alfredsen, K., and Muthanna, T. M. (2020). Assessing the Effects of Four SUDS Scenarios on Combined Sewer Overflows in Oslo, Norway: Evaluating the Low-Impact Development Module of the Mike Urban Model. Hydrol. Res. 51 (6), 1437–1454. doi:10.2166/nh.2020.070
Hilten, R. N., Lawrence, T. M., and Tollner, E. W. (2008). Modeling Stormwater Runoff from green Roofs with HYDRUS-1D. J. Hydrol. 358 (3–4), 288–293. doi:10.1016/j.jhydrol.2008.06.010
Horton, R. E. (1919). Rainfall Interception. Mothly Waeather Rev. 12 (306), 731–732. doi:10.1126/science.12.306.731-a
Hou, J., Mao, H., Li, J., and Sun, S. (2019). Spatial Simulation of the Ecological Processes of Stormwater for Sponge Cities. J. Environ. Manage. 232 (539), 574–583. doi:10.1016/j.jenvman.2018.11.111
Hou, X., Guo, H., Wang, F., Li, M., Xue, X., Liu, X., et al. (2020). Is the Sponge City Construction Sufficiently Adaptable for the Future Stormwater Management under Climate Change? J. Hydrol. 588 (May), 125055. doi:10.1016/j.jhydrol.2020.125055
Huo, W., Li, Z., Zhang, K., Wang, J., and Yao, C. (2020). GA-PIC: An Improved Green-Ampt Rainfall-Runoff Model with a Physically Based Infiltration Distribution Curve for Semi-arid Basins. J. Hydrol. 586 (March), 124900. doi:10.1016/j.jhydrol.2020.124900
Imteaz, M. A. (2015). STORMKIT: A Decision Support Tool for Stormwater System Analysis and Design. Int. J. Computer Aided Eng. Technology. 7 (3), 305–320. doi:10.1504/IJCAET.2015.071294
Islam, A., Hassini, S., and El-Dakhakhni, W. (2021). A Systematic Bibliometric Review of Optimization and Resilience within Low Impact Development Stormwater Management Practices. J. Hydrol. 599 (November 2020), 126457. doi:10.1016/j.jhydrol.2021.126457
Jayasooriya, V. M., and Ng, A. W. M. (2014). Tools for Modeling of Stormwater Management and Economics of green Infrastructure Practices: A Review. Water Air Soil Pollut. 225 (8), 1–20. doi:10.1007/s11270-014-2055-1
Jia, H., Wang, Z., Zhen, X., Clar, M., and Yu, S. L. (2017). China's Sponge City Construction: A Discussion on Technical Approaches. Front. Environ. Sci. Eng. 11 (4), 1–11. doi:10.1007/s11783-017-0984-9
Jia, H., Yao, H., Tang, Y., Yu, S. L., Field, R., and Tafuri, A. N. (2015). LID-BMPs Planning for Urban Runoff Control and the Case Study in China. J. Environ. Manage. 149, 65–76. doi:10.1016/j.jenvman.2014.10.003
Jiang, S., Pang, L., Buchan, G. D., Šimůnek, J., Noonan, M. J., and Close, M. E. (2010). Modeling Water Flow and Bacterial Transport in Undisturbed Lysimeters under Irrigations of Dairy Shed Effluent and Water Using HYDRUS-1D. Water Res. 44 (4), 1050–1061. doi:10.1016/j.watres.2009.08.039
Johannessen, B. G., Hamouz, V., Gragne, A. S., and Muthanna, T. M. (2019). The Transferability of SWMM Model Parameters between green Roofs with Similar Build-Up. J. Hydrol. 569 (January), 816–828. doi:10.1016/j.jhydrol.2019.01.004
Kazak, J., Chruściński, J., and Szewrański, S. (2018). The Development of a Novel Decision Support System for the Location of green Infrastructure for Stormwater Management. Sustainability. 10 (12), 4388. doi:10.3390/su10124388
Kazantsev, P., Marus, Y., and Smelovskaya, A. (2020). Landscape and Climate Specifics for Water Sensitive Urban Design in Vladivostok. IOP Conf. Ser. Mater. Sci. Eng. 753 (4), 042057. doi:10.1088/1757-899X/753/4/042057
Keeley, M., Koburger, A., Dolowitz, D. P., Medearis, D., Nickel, D., and Shuster, W. (2013). Perspectives on the Use of green Infrastructure for Stormwater Management in cleveland and milwaukee. Environ. Manage. 51 (6), 1093–1108. doi:10.1007/s00267-013-0032-x
Kim, J. E., Teh, E. X., Humphrey, D., and Hofman, J. (2021). Optimal Storage Sizing for Indoor arena Rainwater Harvesting: Hydraulic Simulation and Economic Assessment. J. Environ. Manage. 280, 111847. doi:10.1016/j.jenvman.2020.111847
Kocsis, I., Haidu, I., Haidu, I., and Maier, N. (2020). “Application of a Hydrological MIKE HYDRO River - UHM Model for Valea Rea River (Romania). Case Study, Flash Flood Event Occurred on August 1st, 2019,” in Air and Water – Components of f the Environment Conference Proceedings, 257–272. doi:10.24193/awc2020_24
Kolavani, N. J., and Kolavani, N. J. (2020). Technical Feasibility Analysis of Rainwater Harvesting System Implementation for Domestic Use. Sustainable Cities Soc. 62 (June), 102340. doi:10.1016/j.scs.2020.102340
Kristvik, E., Kleiven, G. H., Lohne, J., and Muthanna, T. M. (2018). Assessing the Robustness of Raingardens under Climate Change Using SDSM and Temporal Downscaling. Water Sci. Technology. 77 (6), 1640–1650. doi:10.2166/wst.2018.043
Kuller, M., Bach, P. M., Roberts, S., Browne, D., and Deletic, A. (2019). A Planning-Support Tool for Spatial Suitability Assessment of green Urban Stormwater Infrastructure. Sci. Total Environ. 686, 856–868. doi:10.1016/j.scitotenv.2019.06.051
Lee, J. G., Selvakumar, A., Alvi, K., Riverson, J., Zhen, J. X., Shoemaker, L., et al. (2012). A Watershed-Scale Design Optimization Model for Stormwater Best Management Practices. Environ. Model. Softw. 37, 6–18. doi:10.1016/j.envsoft.2012.04.011
Li, C., Peng, C., Chiang, P.-C., Cai, Y., Wang, X., and Yang, Z. (2019). Mechanisms and Applications of green Infrastructure Practices for Stormwater Control: A Review. J. Hydrol. 568 (September 2018), 626–637. doi:10.1016/j.jhydrol.2018.10.074
Li, H., Qi, Z., Qi, Z., Gui, D., and Zeng, F. (2019). Water Use Efficiency and Yield Responses of Cotton to Field Capacity-Based Deficit Irrigation in an Extremely Arid Area of China. Int. J. Agric. Biol. Eng. 12 (6), 91–101. doi:10.25165/j.ijabe.20191206.4571
Li, J., Li, Y., and Li, Y. (2016). SWMM-based Evaluation of the Effect of Rain Gardens on Urbanized Areas. Environ. Earth Sci. 75 (1), 1–14. doi:10.1007/s12665-015-4807-7
Li, J., Zhao, R., Li, Y., and Chen, L. (2018). Modeling the Effects of Parameter Optimization on Three Bioretention Tanks Using the HYDRUS-1D Model. J. Environ. Manage. 217, 38–46. doi:10.1016/j.jenvman.2018.03.078
Li, J., Zhao, R., Li, Y., and Li, H. (2020). Simulation and Optimization of Layered Bioretention Facilities by HYDRUS-1D Model and Response Surface Methodology. J. Hydrol. 586 (March), 124813. doi:10.1016/j.jhydrol.2020.124813
Li, Q., Wang, F., Yu, Y., Huang, Z., Li, M., and Guan, Y. (2019). Comprehensive Performance Evaluation of LID Practices for the Sponge City Construction: A Case Study in Guangxi, China. J. Environ. Manage. 231 (May 2018), 10–20. doi:10.1016/j.jenvman.2018.10.024
Li, S., Liu, Y., Her, Y., Chen, J., Guo, T., and Shao, G. (2021). Improvement of Simulating Sub-daily Hydrological Impacts of Rainwater Harvesting for Landscape Irrigation with Rain Barrels/cisterns in the SWAT Model. Sci. Total Environ. 798, 149336. doi:10.1016/j.scitotenv.2021.149336
Li, H., Ding, L., Ren, M., Li, C., and Wang, H. (2017). Sponge City Construction in China: A Survey of the Challenges and Opportunities. Water 9 (9), 594–617. doi:10.3390/w9090594
Li, Z., Chen, X., Liu, W., and Si, B. (2017). Determination of Groundwater Recharge Mechanism in the Deep Loessial Unsaturated Zone by Environmental Tracers. Sci. Total Environ. 586, 827–835. doi:10.1016/j.scitotenv.2017.02.061
Lian, H., Yen, H., Huang, J.-C., Feng, Q., Qin, L., Bashir, M. A., et al. (2020). CN-China: Revised Runoff Curve Number by Using Rainfall-Runoff Events Data in China. Water Res. 177, 115767. doi:10.1016/j.watres.2020.115767
Liang, C., Zhang, X., Xia, J., Xu, J., and She, D. (2020). The Effect of Sponge City Construction for Reducing Directly Connected Impervious Areas on Hydrological Responses at the Urban Catchment Scale. Water. 12 (4), 1163. doi:10.3390/W12041163
Liao, Z. L., Zhang, G. Q., Wu, Z. H., He, Y., and Chen, H. (2015). Combined Sewer Overflow Control with LID Based on SWMM: An Example in Shanghai, China. Water Sci. Technology. 71 (8), 1136–1142. doi:10.2166/wst.2015.076
Lindgren, J. (2019). Evaluation of Different Methods for Modelling Stormwater Quality. Gothenburg: Chalmers tekniska högskola/Institutionen för arkitektur och samhällsbyggnadsteknik ACE.
Liu, H., Jia, Y., and Niu, C. (2017). "Sponge City" Concept Helps Solve China's Urban Water Problems. Environ. Earth Sci. 76 (14), 1–5. doi:10.1007/s12665-017-6652-3
Liu, R., Zhang, P., Wang, X., Wang, J., Yu, W., and Shen, Z. (2014). Cost-effectiveness and Cost-Benefit Analysis of BMPs in Controlling Agricultural Nonpoint Source Pollution in China Based on the SWAT Model. Environ. Monit. Assess. 186 (12), 9011–9022. doi:10.1007/s10661-014-4061-6
Liu, W., Feng, Q., Chen, W., Wei, W., and Deo, R. C. (2019). The Influence of Structural Factors on Stormwater Runoff Retention of Extensive green Roofs: New Evidence from Scale-Based Models and Real Experiments. J. Hydrol. 569 (October), 230–238. doi:10.1016/j.jhydrol.2018.11.066
Liu, Y., Cibin, R., Bralts, V. F., Chaubey, I., Bowling, L. C., and Engel, B. A. (2016). Optimal Selection and Placement of BMPs and LID Practices with a Rainfall-Runoff Model. Environ. Model. Softw. 80, 281–296. doi:10.1016/j.envsoft.2016.03.005
Liu, Z., Lu, T., Ye, J., Wang, G., Dong, X., Withers, R., et al. (2018). Antiferroelectrics for Energy Storage Applications: a Review. Adv. Mater. Technol. 3 (9), 1800111–1800121. doi:10.1002/admt.201800111
Luan, Q., Fu, X., Song, C., Wang, H., Liu, J., and Wang, Y. (2017). Runoff Effect Evaluation of LID through SWMM in Typical Mountainous, Low-Lying Urban Areas: A Case Study in China. Water. 9 (6), 439. doi:10.3390/w9060439
Luan, Q., Zhang, K., Liu, J., Wang, D., and Ma, J. (2018). The Application of Mike Urban Model in Drainage and Waterlogging in Lincheng County, China. Proc. IAHS. 379, 381–386. doi:10.5194/piahs-379-381-2018
MacAsieb, R. Q., Orozco, C. R., and Resurreccion, A. C. (2021). Application of Coupled Hec-Hms and Us Epa Wasp for Transport Modelling of Mercury in the Mining-Impacted Ambalanga River. ASEAN Eng. J. 11 (3), 158–176. doi:10.11113/AEJ.V11.17052
Mao, X., Jia, H., and Yu, S. L. (2017). Assessing the Ecological Benefits of Aggregate LID-BMPs through Modelling. Ecol. Model. 353, 139–149. doi:10.1016/j.ecolmodel.2016.10.018
Massoudieh, A., Maghrebi, M., Kamrani, B., Nietch, C., Tryby, M., Aflaki, S., et al. (2017). A Flexible Modeling Framework for Hydraulic and Water Quality Performance Assessment of Stormwater green Infrastructure. Environ. Model. Softw. 92, 57–73. doi:10.1016/j.envsoft.2017.02.013
Meng, Y., Wang, H., Chen, J., and Zhang, S. (2014). Modelling Hydrology of a Single Bioretention System with HYDRUS-1D. Scientific World J. 2014, 1–10. doi:10.1155/2014/521047
Mishra, A., Alnahit, A., and Campbell, B. (2021). Impact of Land Uses, Drought, Flood, Wildfire, and Cascading Events on Water Quality and Microbial Communities: A Review and Analysis. J. Hydrol. 596, 125707. doi:10.1016/j.jhydrol.2020.125707
Morales-Torres, A., Escuder-Bueno, I., Andrés-Doménech, I., and Perales-Momparler, S. (2016). Decision Support Tool for Energy-Efficient, Sustainable and Integrated Urban Stormwater Management. Environ. Model. Softw. 84, 518–528. doi:10.1016/j.envsoft.2016.07.019
Yazdi, M. N., Ketabchy, M., Sample, D. J., Scott, D., and Liao, H. (2019). An Evaluation of HSPF and SWMM for Simulating Streamflow Regimes in an Urban Watershed. Environ. Model. Softw. 118 (March), 211–225. doi:10.1016/j.envsoft.2019.05.008
Nile, B. K., Hassan, W. H., and Esmaeel, B. A. (2018). An Evaluation of Flood Mitigation Using a Storm Water Management Model [SWMM] in a Residential Area in Kerbala, Iraq. IOP Conf. Ser. Mater. Sci. Eng. 433 (1), 012001. doi:10.1088/1757-899X/433/1/012001
Nnadi, E. O., Newman, A. P., Coupe, S. J., and Mbanaso, F. U. (2015). Stormwater Harvesting for Irrigation Purposes: An Investigation of Chemical Quality of Water Recycled in Pervious Pavement System. J. Environ. Manage. 147, 246–256. doi:10.1016/j.jenvman.2014.08.020
Nordman, E. E., Isely, E., Isely, P., and Denning, R. (2018). Benefit-cost Analysis of Stormwater green Infrastructure Practices for Grand Rapids, Michigan, USA. J. Clean. Prod. 200, 501–510. doi:10.1016/j.jclepro.2018.07.152
Ohlin Saletti, A. (2021). Infiltration and Inflow to Wastewater Sewer Systems - A Literature Review on Risk Management, 56. Gothenburg: Chalmers University of Technology.
Onoja, M. U., Ahmadinia, M., Shariatipour, S. M., and Wood, A. M. (2019). Characterising the Role of Parametric Functions in the Van Genuchten Empirical Model on CO2 Storage Performance. Int. J. Greenhouse Gas Control. 88 (January), 233–250. doi:10.1016/j.ijggc.2019.06.004
Oudin, L., Salavati, B., Furusho-Percot, C., Ribstein, P., and Saadi, M. (2018). Hydrological Impacts of Urbanization at the Catchment Scale. J. Hydrol. 559, 774–786. doi:10.1016/j.jhydrol.2018.02.064
Palmstrom, N., and Walker, W. W. (1990). The P8 Urban Catchment Model for Evaluating Nonpoint Source Controls at the Local Level. Enhancing States’ Lake Management Programs.
Pang, B., Shi, S., Zhao, G., Shi, R., Peng, D., and Zhu, Z. (2020). Uncertainty Assessment of Urban Hydrological Modelling from a Multiple Objective Perspective. Water. 12 (5), 1393. doi:10.3390/W12051393
Paus, K. H., Morgan, J., Gulliver, J. S., and Hozalski, R. M. (2014). Effects of Bioretention Media Compost Volume Fraction on Toxic Metals Removal, Hydraulic Conductivity, and Phosphorous Release. J. Environ. Eng. 140 (10), 04014033. doi:10.1061/(asce)ee.1943-7870.0000846
Peng, J., Zhang, X. M., and Zhang, Y. H. (2018). Study on Combining Flood Control with Rainwater Utilization of Airports in China. IOP Conf. Ser. Earth Environ. Sci. 191 (1), 012133. doi:10.1088/1755-1315/191/1/012133
Peng, S., Cui, H., and Ji, M. (2018). Sustainable Rainwater Utilization and Water Circulation Model for Green Campus Design at Tianjin University. J. Sustainable Water Built Environ. 4 (1), 04017015. doi:10.1061/jswbay.0000841
Peters, R. W. (2012). Hydrology and Floodplain Analysis, 5th Edition. Environ. Prog. Sustainable Energ. 31 (3), 332–334. doi:10.1002/ep.11677
Pitt, R., Voorhees, J., and Burger, C. (2002). “ Low Impact Development ” Calculations Using the Source Loading and Management Model (WinSLAMM). Tuscaloosa, 1–22.
Qiao, X.-J., Kristoffersson, A., and Randrup, T. B. (2018). Challenges to Implementing Urban Sustainable Stormwater Management from a Governance Perspective: A Literature Review. J. Clean. Prod. 196, 943–952. doi:10.1016/j.jclepro.2018.06.049
Qin, H.-p., Peng, Y.-n., Tang, Q.-l., and Yu, S.-L. (2016). A HYDRUS Model for Irrigation Management of green Roofs with a Water Storage Layer. Ecol. Eng. 95, 399–408. doi:10.1016/j.ecoleng.2016.06.077
Rawls, W. J., Brakensiek, C. L., and Saxtonn, K. E. (1982). Estimation of Soil Water Properties. Trans. - Am. Soc. Agric. Eng. 25 (5), 1316–1320. doi:10.13031/2013.33720
Rosenberger, L., Leandro, J., Pauleit, S., and Erlwein, S. (2021). Sustainable Stormwater Management under the Impact of Climate Change and Urban Densification. J. Hydrol. 596 (March), 126137. doi:10.1016/j.jhydrol.2021.126137
Rossman, I. A. (2015). Storm Water Management Model User’S Manuel. Version Ii. Environ. Prot. Technol. Ser. EPA. 670, 2015./2-75–017.
Roy, D., Begam, S., Ghosh, S., and Jana, S. (2013). Calibration and Validation of HEC-HMS Model for a River basin in Eastern India. ARPN J. Eng. Appl. Sci. 8 (1), 40–56.
Salifu, A., Abagale, F. K., and Kranjac-Berisavljevic, G. (2021). Estimation of Infiltration Models' Parameters Using Regression Analysis in Irrigation Fields of Northern Ghana. Ojss. 11 (03), 164–176. doi:10.4236/ojss.2021.113009
Sapdhare, H., Myers, B., Beecham, S., Brien, C., Pezzaniti, D., and Johnson, T. (2019). A Field and Laboratory Investigation of Kerb Side Inlet Pits Using Four media Types. J. Environ. Manage. 247 (June), 281–290. doi:10.1016/j.jenvman.2019.06.021
Sartor, J., Mobilia, M., and Longobardi, A. (2018). Results and Findings from 15 Years of Sustainable Urban Storm Water Management. Int. J. SAFE. 8 (4), 505–514. doi:10.2495/SAFE-V8-N4-505-514
Seo, M., Jaber, F., Srinivasan, R., and Jeong, J. (2017). Evaluating the Impact of Low Impact Development (LID) Practices on Water Quantity and Quality under Different Development Designs Using SWAT. Water. 9 (3), 193. doi:10.3390/w9030193
Shahed Behrouz, M., Zhu, Z., Matott, L. S., and Rabideau, A. J. (2020). A New Tool for Automatic Calibration of the Storm Water Management Model (SWMM). J. Hydrol. 581 (December 2019), 124436. doi:10.1016/j.jhydrol.2019.124436
Sharior, S., McDonald, W., and Parolari, A. J. (2019). Improved Reliability of Stormwater Detention basin Performance through Water Quality Data-Informed Real-Time Control. J. Hydrol. 573 (March), 422–431. doi:10.1016/j.jhydrol.2019.03.012
She, L., Wei, M., and You, X.-y. (2021). Multi-objective Layout Optimization for Sponge City by Annealing Algorithm and its Environmental Benefits Analysis. Sustainable Cities Soc. 66 (January), 102706. doi:10.1016/j.scs.2021.102706
Shoemaker, L., Riverson, J. J., Alvi, K., Zhen, J. X., Paul, S., and Rafi, T. (2009). SUSTAIN - A Framework for Placement of Best Management Practices in Urban Watersheds to Protect Water Quality. Cincinnati: Environmental Protection, 1–8. Available at: http://www.epa.gov/nrmrl/wswrd/wq/models/sustain/.
Spahr, K. M., Bell, C. D., McCray, J. E., and Hogue, T. S. (2020). Greening up Stormwater Infrastructure: Measuring Vegetation to Establish Context and Promote Cobenefits in a Diverse Set of US Cities. Urban For. Urban Green. 48 (December 2019), 126548. doi:10.1016/j.ufug.2019.126548
Stagnitta, T., Detenbeck, N., Piscopo, A., and Epa, U. S. (2018). An Overview of the U . S . EPA ’ S Watershed Management Optimization Support Tool ( WMOST ): A Case Study in Taunton , Massachusetts an Overview of the U . S . EPA ’ S Watershed Management Optimization Support Tool ( WMOST ). Singapore: A case study in Taunton.
Steffen, J., Jensen, M., Pomeroy, C. A., and Burian, S. J. (2013). Water Supply and Stormwater Management Benefits of Residential Rainwater Harvesting in U.S. Cities. J. Am. Water Resour. Assoc. 49 (4), 810–824. doi:10.1111/jawr.12038
Strapazan, C., Haidu, I., Haidu, I., and Irimus, I. A. (2021). A Comparative Assessment of Different Loss Methods Available in Mike Hydro River-Uhm. Carpath. J. Earth Environ. Sci. 16 (1), 261–273. doi:10.26471/cjees/2021/016/172
Suppakittpaisarn, P., Jiang, X., and Sullivan, W. C. (2017). Green Infrastructure, Green Stormwater Infrastructure, and Human Health: A Review. Curr. Landscape Ecol. Rep. 2 (4), 96–110. doi:10.1007/s40823-017-0028-y
Takagi, K., Otaki, M., and Otaki, Y. (2018). Potential of Rainwater Utilization in Households Based on the Distributions of Catchment Area and End-Use Water Demand. Water 10 (12), 1706. doi:10.3390/w10121706
Tang, S., Jiang, J., Zheng, Y., Hong, Y., Chung, E.-S., Shamseldin, A. Y., et al. (2021). Robustness Analysis of Storm Water Quality Modelling with LID Infrastructures from Natural Event-Based Field Monitoring. Sci. Total Environ. 753, 142007. doi:10.1016/j.scitotenv.2020.142007
Thrysøe, C., Balstrøm, T., Borup, M., Löwe, R., Jamali, B., and Arnbjerg-Nielsen, K. (2021). FloodStroem: A Fast Dynamic GIS-Based Urban Flood and Damage Model. J. Hydrol. 600 (May), 126521. doi:10.1016/j.jhydrol.2021.126521
Traver, R. G., and Ebrahimian, A. (2017). Dynamic Design of green Stormwater Infrastructure. Front. Environ. Sci. Eng. 11 (4), 1–6. doi:10.1007/s11783-017-0973-z
Tu A, A., Li, Y., Mo, M., and Nie, X. (2020). Hydrologial Efffects of Design Parameters Optimization of Bioretention Based on RECARGA Model. J. Soil Water Conservation 34(01), 149–153. doi:10.13870/j.cnki.stbcxb.2020.01.022
Tu, M., Wadzuk, B., and Traver, R. (2020). Methodology to Simulate Unsaturated Zone Hydrology in Storm Water Management Model (SWMM) for green Infrastructure Design and Evaluation. PLoS ONE. 15 (7), e0235528–19. doi:10.1371/journal.pone.0235528
Tu, A., Xie, S., Mo, M., Song, Y., and Li, Y. (2021). Water Budget Components Estimation for a Mature Citrus Orchard of Southern China Based on HYDRUS-1D Model. Agric. Water Management. 243 (April 2020), 106426. doi:10.1016/j.agwat.2020.106426
Vargas, D., Dominguez, I., Ward, S., and Oviedo-Ocaña, E. R. (2019). Assisting Global Rainwater Harvesting Practitioners: A Decision Support Tool for Tank Sizing Method Selection under Uncertainty. Environ. Sci. Water Res. Technol. 5 (3), 506–520. doi:10.1039/c8ew00707a
Walker, W. W. (1990). P8 Urban Catchment Model - Program Documentation Version 1.1. Prepared for IEP, Inc. and Narragansett Bay Project(October). Wisconsin.
Wang, N., and Chu, X. (2020). Revised Horton Model for Event and Continuous Simulations of Infiltration. J. Hydrol. 589 (June), 125215. doi:10.1016/j.jhydrol.2020.125215
Wang, S., and Palazzo, E. (2021). Sponge City and Social Equity: Impact Assessment of Urban Stormwater Management in Baicheng City, China. Urban Clim. 37 (February), 100829. doi:10.1016/j.uclim.2021.100829
Wang, S., Yang, L., and Bai, W. (2009). Perfect Combination of Art and Technology—Study on the Construction of Rain Garden. Chinese Landscape Architecture 25 (06), 54–57. doi:10.3969/j.issn.1000-6664.2009.06.013
Wang, X., Tian, W., and Liao, Z. (2021). Offline Optimization of Sluice Control Rules in the Urban Water System for Flooding Mitigation. Water Resour. Manage. 35 (3), 949–962. doi:10.1007/s11269-020-02760-9
Wang, Y., Sun, M., and Song, B. (2017). Public Perceptions of and Willingness to Pay for Sponge City Initiatives in China. Resour. Conservation Recycling. 122, 11–20. doi:10.1016/j.resconrec.2017.02.002
Weber, T. K. D., Finkel, M., Gonçalves, M., Vereecken, H., and Diamantopoulos, E. (2020). Pedotransfer Function for the Brunswick Soil Hydraulic Property Model and Comparison to the Van Genuchten‐Mualem Model. Water Resour. Res. 56 (9), 1–22. doi:10.1029/2019WR026820
Wei, Z., Sun, S., and Ji, X. (2019). The Inspiration of Rainwater Utilization of Foreign Sponge Campus Landscape Planning for Beijing. IOP Conf. Ser. Earth Environ. Sci. 227 (5), 052019. doi:10.1088/1755-1315/227/5/052019
Whitehouse, A. (2017). Common Economic Oversights in Green Infrastructure Valuation. Landscape Res. 42 (2), 230–234. doi:10.1080/01426397.2016.1228860
Wu J, J., Larm, T., Wahlsten, A., Marsalek, J., and Viklander, M. (2021). Uncertainty Inherent to a Conceptual Model StormTac Web Simulating Urban Runoff Quantity, Quality and Control. Urban Water J. 18 (5), 300–309. doi:10.1080/1573062X.2021.1878240
Wu Z, Z., Ma, B., Wang, H., Hu, C., Lv, H., and Zhang, X. (2021). Identification of Sensitive Parameters of Urban Flood Model Based on Artificial Neural Network. Water Resour. Manage. 35 (7), 2115–2128. doi:10.1007/s11269-021-02825-3
Xia, J., Zhang, Y., Xiong, L., He, S., Wang, L., and Yu, Z. (2017). Opportunities and Challenges of the Sponge City Construction Related to Urban Water Issues in China. Sci. China Earth Sci. 60 (4), 652–658. doi:10.1007/s11430-016-0111-8
Xu, T., Jia, H., Wang, Z., Mao, X., and Xu, C. (2017). SWMM-based Methodology for Block-Scale LID-BMPs Planning Based on Site-Scale Multi-Objective Optimization: a Case Study in Tianjin. Front. Environ. Sci. Eng. 11 (4), 1–12. doi:10.1007/s11783-017-0934-6
Xu, Z., Qu, Y., Wang, S., and Chu, W. (2021). Diagnosis of Pipe Illicit Connections and Damaged Points in Urban Stormwater System Using an Inversed Optimization Model. J. Clean. Prod. 292, 126011. doi:10.1016/j.jclepro.2021.126011
Yang, M., Zhang, Y., and Pan, X. (2020). Improving the Horton Infiltration Equation by Considering Soil Moisture Variation. J. Hydrol. 586 (January), 124864. doi:10.1016/j.jhydrol.2020.124864
Yang, Y., Li, J., Huang, Q., Xia, J., Li, J., Liu, D., et al. (2021). Performance Assessment of Sponge City Infrastructure on Stormwater Outflows Using Isochrone and SWMM Models. J. Hydrol. 597 (February), 126151. doi:10.1016/j.jhydrol.2021.126151
Ye, S., and Tiong, P. (2000). NPV-AT-Risk Method in Infrastructure Project Investment Evaluation. J. Construction Eng. Management 126 (June), 227–233. doi:10.1061/(asce)0733-9364(2000)126:3(227)
Yin, D., Evans, B., Wang, Q., Chen, Z., Jia, H., Chen, A. S., et al. (2020). Integrated 1D and 2D Model for Better Assessing Runoff Quantity Control of Low Impact Development Facilities on Community Scale. Sci. Total Environ. 720, 137630. doi:10.1016/j.scitotenv.2020.137630
Yu, Z., Montalto, F., and Behr, C. (2018). Probabilistic green Infrastructure Cost Calculations Using a Phased Life Cycle Algorithm Integrated with Uncertainties. J. Hydroinformatics. 20 (5), 1201–1214. doi:10.2166/hydro.2018.107
Zema, D. A., Labate, A., Martino, D., and Zimbone, S. M. (2017). Comparing Different Infiltration Methods of the HEC‐HMS Model: The Case Study of the Mésima Torrent (Southern Italy). Land Degrad. Develop. 28 (1), 294–308. doi:10.1002/ldr.2591
Zeng, Z., Yuan, X., Liang, J., and Li, Y. (2021). Designing and Implementing an SWMM-Based Web Service Framework to Provide Decision Support for Real-Time Urban Stormwater Management. Environ. Model. Softw. 135 (September 2020), 104887. doi:10.1016/j.envsoft.2020.104887
Zhang, C., He, M., and Zhang, Y. (2019). Urban Sustainable Development Based on the Framework of Sponge City: 71 Case Studies in China. Sustainability. 11 (6), 1544–1621. doi:10.3390/su11061544
Zhang, D., Hølland, E. S., Lindholm, G., and Ratnaweera, H. (2018). Hydraulic Modeling and Deep Learning Based Flow Forecasting for Optimizing Inter Catchment Wastewater Transfer. J. Hydrol. 567, 792–802. doi:10.1016/j.jhydrol.2017.11.029
Zhang, S., Li, Y., Ma, M., Song, T., and Song, R. (2018). Storm Water Management and Flood Control in Sponge City Construction of Beijing. Water. 10 (8), 1040–1111. doi:10.3390/w10081040
Zhang, Z., Szota, C., Fletcher, T. D., Williams, N. S. G., Werdin, J., and Farrell, C. (2018). Influence of Plant Composition and Water Use Strategies on green Roof Stormwater Retention. Sci. Total Environ. 625, 775–781. doi:10.1016/j.scitotenv.2017.12.231
Zhang, Z., Gu, J., Zhang, G., Ma, W., Zhao, L., Ning, P., et al. (2021). Design of Urban Runoff Pollution Control Based on the Sponge City Concept in a Large-Scale High-Plateau Mountainous Watershed: A Case Study in Yunnan, China. J. Water Clim. Change. 12 (1), 201–222. doi:10.2166/wcc.2019.120
Keywords: stormwater management, sponge city, green stormwater infrastructure, model comparison, stormwater utilization
Citation: Liu Q, Cui W, Tian Z, Tang Y, Tillotson M and Liu J (2022) Stormwater Management Modeling in “Sponge City” Construction: Current State and Future Directions. Front. Environ. Sci. 9:816093. doi: 10.3389/fenvs.2021.816093
Received: 16 November 2021; Accepted: 20 December 2021;
Published: 19 January 2022.
Edited by:
Pedro Neves Carvalho, Aarhus University, DenmarkCopyright © 2022 Liu, Cui, Tian, Tang, Tillotson and Liu. This is an open-access article distributed under the terms of the Creative Commons Attribution License (CC BY). The use, distribution or reproduction in other forums is permitted, provided the original author(s) and the copyright owner(s) are credited and that the original publication in this journal is cited, in accordance with accepted academic practice. No use, distribution or reproduction is permitted which does not comply with these terms.
*Correspondence: Junguo Liu, junguo.liu@gmail.com
†These authors have contributed equally to this work and share first authorship