- School of Economics, Nanjing Audit University, Nanjing, China
The vigorous development of modern information and communication technology (ICT) has driven the digital trade featured by the ICT technique and industry as the carrier. This study empirically tests the impact of ICT-based digital trade openness on green total factor productivity (GTFP) by selecting ICT as the representative digital trade data of 30 provinces in China over the timespan 2002–2018. We employ the slack-based model and global Malmquist–Luenberger (SBM-GML) estimation method to calculate the provincial GTFP and explore the heterogeneous impact of digital trade openness on GTFP through the scale effect, technology effect, and structure effect. In terms of empirical results, the panel fixed model and panel quantile estimation model both suggest the same findings. With the continuous expansion of the scale of digital trade, its scale effect has a significant inhibitory effect on GTFP, whereas the structure effect combined with human capital and the technology effect correlated with technological research and development (R&D) have a significant promoting effect on GTFP. The panel quantile regression model reveals that the interaction intensity increases gradually from a low quantile to high quantile. Further robustness tests also verify the consistency and stability of the results. Finally, the study puts forward corresponding practical suggestions for the construction of a high-quality open pattern of digital trade and the coordinated development of GTFP. The specific policy implications include the following: (1) Emphasize on the penetration and connection effect of the new generation of ICT, and strengthen the construction of enterprise informatization. (2) Expand digital trade openness and broaden the field of industrial cooperation. (3) Optimize the industrial structure of digital trade, and accelerate the development of core industries of digital trade. (4) Gradually promote the transformation of digital trade from relying on quantity and scale to product quality.
1 Introduction
In the context of economic globalization, China has been deeply integrated into the global value chain division system. The improvement of green total factor productivity cannot be separated from the global division system with trade openness and digital trade as the main carrier. At present, China is in a critical period of economic transformation. Technological progress is the premise of realizing sustainable economic growth, which is conducive to the transformation of China’s economy to a growth model supported by total factor productivity (Shao et al., 2021). A country’s technological progress depends on the domestic R&D investment in high-tech industries and talent cultivation. Foreign innovation activities also play a direct or indirect role through knowledge spillover. Trade openness and digital trade are the main ways of technology transfer and knowledge spillover among countries, which is beneficial to the spread and diffusion of green technology in a country with the expansion of the scale of opening to the outside world. Subsequently, the improvement of GTFP and green economic development will be realized.
The enhancement of information and communication technology (ICT) has promoted the digital revolution of the industry. The digital economy with data as the core element is becoming an important part of world economic growth. As the main trade form in the development of the digital economy era, digital trade is inseparable from the development of information technology and the Internet. With the constant innovation and comprehensive application of the new generation of ICT, global digital trade has developed rapidly and become a new driving force for countries to promote economic growth (Ren et al., 2019; Cao et al., 2021). At the same time, the research on the evolvement of digital trade and its economic effects has become a hot issue concerned by global research institutions from all sectors of the society. Digital trade has become a new trend of current trade development and occupies an increasingly important position in international trade (Dong et al., 2020; Zhang et al., 2021). It stimulates new momentum for global economic growth and has received attention from all over the world. The enhancement of digital trade can effectively reduce costs, improve efficiency, optimize global resource allocation, and promote service trade, which has a far-reaching impact on the global division of labor and the construction of competitive advantage. China’s ICT trade volume and its proportion to the world are displayed in Figure 1.
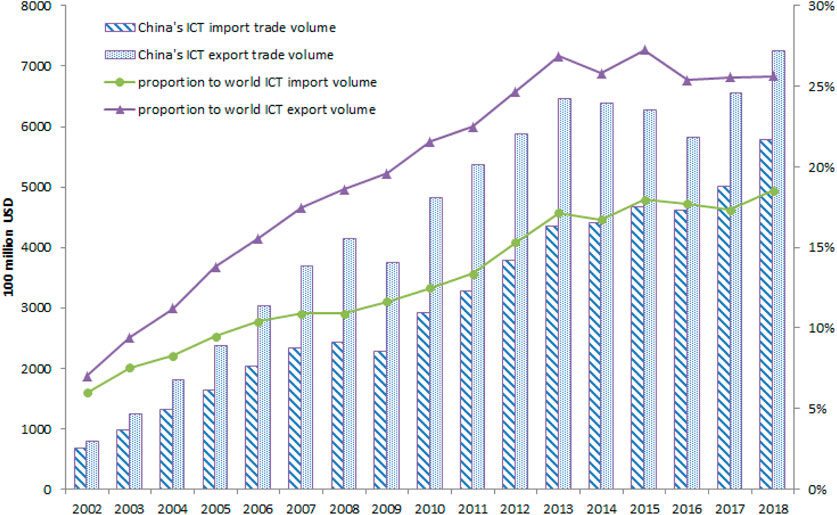
FIGURE 1. China’s total ICT import and export trade volume and its proportion to the world’s total ICT trade volume from 2002 to 2018. Note: HS Code 8517, 8525, 8526, 8527, 8528, 8529, and 8542. Data are from the WDI database of World Bank.
At present, the digital economy is entering a new era of rapid development. ICT and digital technologies such as cloud computing and big data have been integrated into all aspects of the economic field (Yan et al., 2019; Ren et al., 2021). Relying on digital economy, digital trade also shows vigorous vitality and development potential. Open economy focuses on solving the problem of internal and external linkage of development, and green growth concentrates on solving the problem of harmony between humans and nature. Then, under the comprehensive consideration of economic and environmental benefits, consideration must be given to both the trade openness and green growth. China is the global leader in digital trade after the United States. At present, three of the world’s top ten Internet giants are from China. The development of China’s digital trade depends on the rise of domestic e-commerce and the growth of Internet giants such as Alibaba and Tencent. At present, China’s e-commerce transaction volume accounts for more than 40% of the world. The breakthrough of China’s 5G technologies will continue to promote the digital transformation of domestic traditional industries (Dong X. et al., 2021). In general, China has strong development potential in digital trade whether the net effect of trade openness and digital trade on China’s GTFP is positive or negative, what the influencing path is like, and whether the interaction between digital trade and other factors is conducive to the improvement of GTFP. Therefore, this study distinguishes from the extant studies and with its main contributions in the following aspects. We make a theoretical analysis and empirical test by using the panel quantile estimation method on the mechanism of digital trade on GTFP so as to provide a new perspective for the improvement of China’s GTFP and eventually promote the construction of a high-quality opening pattern of China’s economy. The remainder of this study is organized as follows. Section 2 reviews the past relevant literature; Section 3 constructs the mathematical model and proposes the mechanism; Section 4 introduces the methodology and data source; Section 5 performs empirical research and discusses the results; and Section 6 concludes the research findings and puts forward policy suggestions correspondingly.
2 Literature Review
2.1 Studies on the Impact of Trade Openness on Green Technology Innovation
There are abundant theoretical and empirical research results on the relationship between trade openness and green technology innovation, but there is no unified conclusion on whether there is a positive or negative causal relationship between them. On the one hand, Cao and Wang (2017) proposed that trade openness can positively affect the regional green technology progress rate by changing the R&D investment cost and optimizing the labor capital ratio. Khan et al. (2018) argued that only trade liberalization in middle-income countries can play a role in energy conservation and emission reduction in the long run, while for high-income developed countries, trade liberalization will reduce the total amount of carbon dioxide emissions. Peng et al. (2020) asserted that trade openness can optimize the industrial structure to a certain extent, which is beneficial to GTFP. On the other hand, Bandyopadhyay (2006) studied the growth mechanism of GTFP in APEC (Asia Pacific Economic Cooperation) countries and found that trade openness has a negative impact on the growth of GTFP in a country. Li and Liu (2017) proposed that the improvement of trade openness will make the Yangtze River Economic Belt still promote economic development at the expense of the environment, which hinders the improvement of GTFP. Li et al. (2021) claimed that the trade mode dominated by primary products would drive many enterprises to reduce production costs by employing cheap labor, resulting in a decline in enterprises’ attention to technological innovation, which is not beneficial to the growth of GTFP. Furthermore, Hassine and Kandil (2009) proposed that trade openness can affect the change of GTFP by adjusting the industrial structure and affecting technology spillover, but it is impossible to judge whether it is positive or negative. For other nonlinear studies, Niebel, (2018) discovered that developed countries have transferred pollution-intensive industries to developing countries through national trade, and the existence of the environmental Kuznets curve can also be seen in developing countries. The reason for this phenomenon is not technological progress. Zhao et al. (2020) believed that with the constant enhancement of trade opening up, China’s economic growth promotes the increase of carbon dioxide emissions, but the growth will weaken over time, showing an inverted U-shaped curve from the beginning to a positive correlation slowly.
In terms of impact mechanism, Klevorick et al. (1995) pointed out that technological opportunities usually have obvious industry characteristics, and the difference of technological opportunities has an important impact on the difference of technological progress in various industries. Amiti and Konings (2007) found that there is an obvious “import learning” effect in import trade. By importing advanced production and emission reduction technologies, machinery and equipment, and high-tech intermediate commodities from developed countries, enterprises can quickly master advanced production technologies in a short time, improve production efficiency, reduce pollution emissions and energy consumption in the production process, and then alleviate the environmental cost burden of enterprises. Goldberg and Pavcnik, (2007) argued that the import of high-quality intermediate products enables importing countries to obtain more core technologies. Hu et al. (2020) pointed out that the import of intermediate goods in labor resource–intensive industries hinders technological innovation, while the import of intermediate goods in capital technology–intensive industries will promote technological innovation. Especially considering environmental constraints, with the increasing optimization of the import trade structure, enterprises will introduce more machinery and equipment with high content of clean technology, which will help to enrich the types of domestic intermediate inputs and promote the spillover and diffusion of green technology. Ghali et al. (2013) selected the data of Tunisia and Egypt and validated that trade innovation reduces transaction costs and leads to an increase in TFP. Trade innovation and labor resources are more important than R&D investment. Can et al. (2020) analyzed that the source of the impact of exports on total factor productivity lies in the improvement of production effect in the export sector and technology spillover to non-export sectors.
2.2 Studies on the Impact of ICT and Digital Trade on Environmental Efficiency
A large number of studies have not reached a unanimous conclusion on whether ICT promotes GTFP. Schulte et al. (2016) investigated statistical evidence on ICT by reducing the power demand of 27 industries in 13 OECD economies and believed that ICT led to higher energy efficiency. Bastida et al. (2019) found that ICT can stimulate changes in household behavior and improve power efficiency by 0–5% in EU economies. Doukas et al. (2019) also revealed the role of ICT in improving energy efficiency for GCC member states. Likewise, green technology innovation was found to be the transmission path of digital finance affecting energy and environmental performance (Cao et al., 2021; Shao et al., 2021; Yu et al., 2021). The development of ICT significantly affects economic growth and improves energy efficiency and growth efficiency by strengthening technology penetration and innovation, increasing demand, and reducing production costs (Solarin et al., 2021). Sharma et al. (2021) studied the GDP, energy consumption, gross national income, ICT, personal Internet use, electricity, and exports of emerging Asian economies and found empirical evidence that ICT accelerated economic growth. Other studies demonstrated that ICT helps to decrease carbon dioxide emissions (Hodrab et al., 2016; Park et al., 2018; Amri et al., 2019). On the contrary, Solow (1987) put forward the productivity paradox, that is, the large-scale application of computers did not bring about a corresponding increase in productivity. Collard et al. (2005) believed that the consumption of ICT commodities increases the intensity of power consumption, indicating a decline in energy efficiency in six service industries in France. Bernstein and Madlener (2010) documented similar findings in specific industries of eight European economies. Jiang and Liu (2015) assumed that informatization has not brought TFP growth, and its contribution to economic growth is mainly reflected in the deepening of ICT capital and the improvement of total factor productivity of the ICT manufacturing industry. Similarly, the conclusions by Wang and Han (2016) also supported ICT to increase the energy consumption intensity in western and central China. Differential enhanced technological progress will lead to technological progress bias. According to the technology consistency theory (Antonelli, 2016), if the technological progress bias is inconsistent with factor endowment, it will weaken the price effect and market scale effect and inhibit the promotion of GTFP. Zhou et al. (2018) discovered that ICT accounts for 4.5% of China’s industrial energy use intensity.
The construction of digital information network infrastructure is the foundation for the rise of digital economy and digital trade. Nath and Liu (2017) affirmed that the combination of informatization and industrialization can promote technological progress, industrial structure upgrading, and optimal allocation of resources. There may be many reasons for this situation, one of which is that the initial digital information network construction itself has a limited pulling effect on economic growth and may replace many other traditional industries. Only after the production of a large amount of knowledge and information has been digitized and consumers are used to the digital knowledge and information obtained through the network and applied to traditional economic activities to improve the efficiency of traditional economic activities, the promotion effect of digital economy and digital trade on GTFP will be gradually emerged. In addition, Yan et al. (2018) described the role of ICT in promoting energy productivity in 50 developed and underdeveloped economies. ICT trade could also build a bridge of technical expertise between developing economies. Goldbach et al. (2018) mentioned that energy services based on digital ICT are the key to reducing energy use intensity. National energy strategies must be synchronized with the objectives of improving ecological advantages and ensuring sustainable development. However, the key to achieving these goals is to promote technological progress and technology transfer through global trade (Liddle, 2018; Shahbaz and Sinha, 2019). In these circumstances, digital ICT trade is crucial in providing technical facilities for sustainable economic development. According to the study of Ahmed and Le (2021), ICT trade helps to improve the environmental quality by reducing carbon dioxide emissions. At present, China’s industry is in the digital transformation stage, but the depth of industrial digitization still lags behind the level of some developed countries, and the contribution of ICT-based digital trade to GTFP is not fully demonstrated. Because data elements and digital technology are different from traditional elements, when ICT capital investment, data elements, and digital technology form a new production function, the mechanism of ICT-based digital trade affecting GTFP becomes more complex. However, whether these new features driven by digital technology can really improve enterprise GTFP depends on a variety of factors. For one thing, ICT-based digital trade improves GTFP through the input and allocation of new elements of digital economy (Lange et al., 2020). In contrast, the virtual economy generated by ICT-based digital trade has a negative impact on the real economy and GTFP through the “crowding out effect” (Dong K. et al., 2021).
2.3 Current Literature Gap and Improvement and Contribution of This Study
This study relates to the past literature in terms of research scope, theoretical, and empirical methodology. Previous literature works have laid a solid foundation for this study, but the research on the impact of digital trade openness on GTFP still has some deficiencies listed below. (1) Although many scholars paid attention to the revolutionary role of digital trade on GTFP growth, they lacked systematic theoretical analysis and empirical test. (2) Digital technology is a new type of production factor. The research on the internal influencing path of it on GTFP is not thorough enough. (3) Former studies only analyzed the green development of China’s industry or the manufacturing industry. The research object is relatively single, which cannot reflect the overall green development of China, and the conclusion is not generally applicable. (4) Many literature studies have studied the impact of trade openness or digital trade on economic growth or carbon emissions, whereas the research on the impact of digital trade on GTFP is insufficient. Based on these drawbacks, this study aims to make improvements to fill the research gap and contributes to the extant studies from the following aspects. (1) GTFP is classified and calculated by the region by using the global Malmquist–Luenberger (GML) index of the non-radial and non-angle slack-based model (SBM) directional distance function, which can more truly and reliably reflect the coordinated development relationship between the environment and economic growth. (2) This study theoretically analyzes the impact mechanism of ICT-based digital trade on GTFP and empirically tests the effect of digital trade openness and interaction with other factors on GTFP based on the scale effect, structure effect, and technology effect. (3) Considering the differences in economic development among regions, this study will further analyze the impact of digital trade in different Chinese areas on GTFP. (4) Finally, from the perspective of human capital and R&D investment, this study utilizes the panel quantile model to test the impact of ICT-based digital trade on GTFP and puts forward targeted countermeasures and suggestions for the high-quality development of digital trade and promoting GTFP in combination with the regional characteristics of provinces in China.
3 Model Construction and Theoretical Mechanism
3.1. Theoretical Effect Model of Digital Trade Openness and Environmental Efficiency
Based on the general equilibrium model of environmental pollution and trade constructed by Antweiler et al., (2001), this study introduces other technical factors to analyze digital trade openness and carbon emission. On this basis, a carbon emission model considering more technical factors can be established to yield the theoretical model of the impact of digital trade on the environment. Due to pollution, production costs also depend on environmental regulations. Since the return to scale remains unchanged, the production cost of actual output other than potential output is expressed as
where
where
where
3.2 Heterogeneous Trade Model of Product Quality
We hereby construct a heterogeneous trade model of product quality to analyze the determinants of product quality and investigate the impact of digital trade on GTFP. It is assumed that the consumer utility is a functional form of constant elasticity of substitution.
where
where
where
The development of digital trade brings the integration of industrial digitization and manufacturing intelligence. More importantly, the enhancement of production efficiency and production capacity promotes the quality of trade products and eventually uplifts GTFP.
3.3 Theoretical Mechanism and Research Hypotheses of the Impact of Digital Trade Openness on GTFP
(1) The Scale Effect
The scale effect is that the opening of digital trade further promotes the rapid economic development and intensifies pollution. Due to the process of globalization and the continuous expansion of free trade area, digital trade is more frequent and popular, which promotes the production scale enterprises that could not be expanded due to resource constraints in some way. But at the same time, it will also increase the pollution to the environment. Owing to the ceaseless development of economy and expansion of the production scale, it has a scale effect on environmental pollution (Santarius et al., 2020). The expansion of the scale of digital trade will lead to the increase in domestic production and manufacturing activities, which may aggravate pollution, and even make some enterprises adopt backward production technology in order to save costs. These negative effects will inhibit the green technology innovation power of enterprises to a certain extent. Thus, the first hypothesis is as follows:
H1: The rapid expansion of the digital trade scale is not conducive to the improvement of GTFP.
(2) The Structure Effect
While digital trade empowers economic development, consumer Internet and industrial Internet are developing rapidly. The demand for human capital in relevant industries has changed fundamentally, which promotes the labor force to adapt to the transformation of the market structure. The development of digital technology and related industries provides technical means for the dissemination of educational information, helps to further optimize the educational structure, knowledge structure, and skill structure of labor force, and provides a power source for the improvement of labor productivity and GTFP growth (Antonelli, 2016). We correspondingly propose the second hypothesis.
H2: Digital trade promotes GTFP by enhancing the level of human capital.
(3) The Technology Effect
The technology effect is divided into two aspects. The first is that through digital trade, low-tech developing countries will obtain many advanced technologies and environmental pollution control experience from developed countries with high-tech industries. There are two mechanisms for the impact of technology effects on the environment. One is the openness of digital trade promotes the development and scale of enterprises, and improving the technology of enterprise production and the investment of R&D funds is conducive to improving the efficiency of resource utilization. The other is that digital trade can enable the country to obtain technological progress through the technology spillover effect after the introduction of foreign capital in order to reduce carbon dioxide emissions (Li et al., 2020). Hence, the third hypothesis is as follows:
H3: The interaction between digital trade and technology R&D can boost GTFP.
4 Methodology and Data
4.1 Econometric Techniques
Individual effects are considered in the fixed effect (FE) model and random effect (RE) model. When the individual effects are associated with the explanatory variables, the fixed effect model ought to be chosen for the coefficient estimation if the random effect model is found to be inconsistent. Otherwise, the random effect model is more suitable. The Hausman test is usually utilized to validate the applicability of these two models.
The quantile regression method was first proposed by Koenker and Bassett (1978). It fits the functional relationship of independent variables based on the conditional distribution of dependent variables. Its regression results are not sensitive to the influence of outliers, so it has the advantage of higher robustness than the traditional panel mean regression. Quantile regression can provide the overall information of dependent variables. With the help of regression of their quantiles, various segmentation points correspond to different coefficients. Therefore, varied quantile characteristics of dependent variables can be decomposed. At the same time, the quantile regression method can minimize the impact of extreme values on the estimation results; therefore, more credible and robust regression estimation results can be obtained (Yan et al., 2020; Cheng et al., 2021). The quantile linear regression model assumes that the overall
where,
It is worth noting that the main problem in the estimation model is that the traditional estimation methods are not suitable for calculating the coefficients of the panel quantile model. To cope with this issue, we adopt the panel quantile model estimation method proposed by Cheng et al. (2019), which can fully consider the parameter heterogeneity and reduce the influence of abnormal sample points on the estimation results.
4.2 Variable Selection
(1) Explained Variable
Green Total Factor productivity
The traditional total factor productivity measurement method has limitations because it fails to include environmental constraints of resource consumption and pollution emission into the input–output indicators. The global Malmquist–Luenberger (GML) index method of the SBM (slack-based model) directional distance function can make up for this deficiency. As for the measurement of GTFP, Chung et al. (1997) proposed the directional distance function and extended the Malmquist index to GML index. This method can consider both an expected output increase and unexpected output decrease, and the SBM directional distance function can solve the problem of insufficient input–output, i.e., non-slack. The variable indicators in the GTFP measurement model include factor input, expected output, and unexpected output indicators. We define the SBM directional distance function containing unexpected outputs as follows:
where
where
The variables in the GTFP measurement model include factor input, expected output, and unexpected output indicators. Factor input indicators mainly include labor input, capital input, and energy input. The labor input is measured by the number of employees per unit at the end of the year (unit: 10 thousand persons). The energy input is expressed by annual power consumption (unit: 10 thousand KWH). Capital investment is measured by capital stock (unit: 10 thousand yuan). This study uses the perpetual inventory method to calculate the capital stock of fixed assets and uses the study by Hall and Jones (1999) for reference to the treatment methods of the base period capital stock, depreciation rate, fixed asset investment price index, and total fixed asset formation to calculate China’s capital stock from 2002 to 2018. The expected output is measured by real regional GDP deflated by the 2002 price (unit: yuan). The unexpected output is expressed by the emission of industrial “three wastes” (industrial sulfur dioxide, industrial wastewater, and industrial smoke and dust) in the unit of 10 thousand tons.
It is noted that since the SBM-GML productivity index does not reflect GTFP, the change rate of GTFP is relative to the previous year, which must be transformed accordingly before it can be used for econometric regression. Taking 2002 as the base period, the GTFP level of that year is set as 1 and calculated in combination with the GML index; the GTFP indicators of 30 provinces in China from 2002 to 2018 can be obtained.
(2) Core Explanatory Variables
ICT-Based Digital Trade
Some relevant studies pointed out that digital trade is a trade mode with ICT technology as the carrier (Borga and Koncz-Bruner, 2012; Fefer, 2017). The development of the ICT industry provides software and hardware supporting facilities for the development of a country’s digital trade and its integration with various industries. It is an important guarantee and necessary element for the development of digital trade. Therefore, the scale of the ICT industry can effectively reflect the progress and potential of a country’s digital trade development. This study selects the trade volume of ICT services and ICT products to measure the scale of the ICT industry as the proxy index of a country’s digital trade development level, in which ICT services are a collection of computer and telecommunication services; ICT products encompass computers and peripheral equipment, communication equipment, consumer electronic equipment, electronic components, and other information and technology products.
(3) Other Variables
Human Capital
The level of human capital determines the quality of the labor force. A high level of human capital will effectively improve the use efficiency of relevant production factors such as material capital and technology R&D, realize the increase of returns to scale, and promote the continuous improvement of regional GTFP (Murshed et al., 2020). This study selects the proportion of the number of students in colleges and universities in the total population of each region at the end of the year as the index to measure the level of human capital. Innovative human capital has the creative characteristics of social scarcity, can realize the efficient combination of production factors and significantly improve technical efficiency, and drive economic growth with an increasing marginal return and output multiplier effect, which is the key to promoting GTFP.
Research and Development Level
R&D investment is the foundation of technological innovation and an indispensable driving factor for economic growth. The impact of technological innovation on environmental quality depends more on the progress direction of green technology (Su et al., 2021). Only when the proportion of green technology increases gradually, can China finally change the direction of technological progress and improve the environmental quality. Scholars have found that international R&D capital technology spillovers contribute to the accumulation of domestic enterprises’ knowledge capital stock, improve the environmental governance capacity, and resource the allocation efficiency and have an important impact on green technology innovation. We adopt the proportion of R&D expenditure to GDP to denote this variable.
Infrastructure
The impact of different types of transportation infrastructure on GTFP can be positive or negative. On the one hand, the transportation infrastructure is closely related to environmental pollution, which aggravates transportation energy consumption and pollution emission, and can hinder GTFP. On the other hand, the improvement of road traffic can alleviate traffic congestion to a certain extent. With the increase of urban road density, traffic congestion will be greatly improved. The increase of vehicle moving speed will reduce energy consumption and exhaust emissions (Luo, et al., 2018). This study selects the per capita road area of each region to measure the level of infrastructure construction.
Population Density
One research conclusion is that population density is positively correlated with ecological efficiency (Ohlan, 2015). Population agglomeration can bring economic agglomeration, promote professional division of labor, improve economic operation efficiency, and uplift regional ecological efficiency. Another view is that the increasing degree of agglomeration hinders the limited resources and spaces to carry the growing production capacity of enterprises. Therefore, it may produce a crowding effect and inhibit the improvement of environmental governance performance (Lin et al., 2011). Suburbanization of urban population will reduce population density, lower carbon emissions per unit area, and help improve urban environmental quality. Therefore, the effect of population density on GTFP depends on whether the agglomeration effect or crowding effect plays a leading role.
4.3 Data Source and Feature
On account of the data availability and reliability, the sample data we use from 30 Chinese provinces cover the time period 2002–2018. All the data stem from the China Statistical Yearbook and WDI database of the World Bank. The descriptive statistics and correlation matrix of the analyzed variables are shown in Table 1 and Table 2. The changing trend of GML, GEFF, and GTECH indexes and the GTFP curve of each region are visually manifested in Figure 2 and Figure 3. The policy of “the rise of central China” facilitates the quick elevation of GTFP in the central region (Zhou et al., 2018); the growth rate gradually slows down and stagnates for several years after 2009. But the gap with the eastern region has been bridging in recent years.
5 Empirical Research
5.1 Estimation of Full Sample
From the regression results of the whole sample in Table 3, the fixed effect regression results show that ICT-based digital trade has no significant inhibitory effect on GTFP. The quantile regression results also support this finding, reflecting a weak negative significant impact (H1 confirmed). This may be related to the periodicity and interval of the spillover effect of digital technology (Chen et al., 2019). Digital trade basically follows the reverse osmosis process of the “three-two-one” industry. Based on digital technology innovation with a great application value such as artificial intelligence and cloud computing, it has gradually penetrated into the value creation process of the secondary industry in recent years. Human capital has a negative significant effect in the fixed effect, while its negative impact becomes insignificant in the panel quantile regression. The level of technological R&D plays a positive role in promoting GTFP in all the models. The infrastructure and population density also have a significant positive impact on GTFP, but the impact intensity of the infrastructure decreases from a low quantile to high quantile, while the influencing intensity of the population density increases. This may be that China’s regional agglomeration economy is still enjoying the dividend of increasing returns to scale (Haftu, 2019).
5.2 Estimation of the Influence Mechanism Between IDT and HC
For a long time, China’s ICT-based digital trade usually absorbs, imitates, and re-innovates on the basis of foreign technologies. The development capacity of domestic original production processes and energy-saving technologies is relatively limited. The interaction between digital trade and human capital shows a significant positive role in promoting GTFP in Table 4. The intensity of the positive effect gradually elevates from the 25th to 90th quantile. Therefore, China has to maximize the role of digital trade in productivity improvement and resource conservation by taking human capital as the supporting condition. The labor demand induced by ICT-based digital trade is diverse. When different types of human capital on the supply side can meet the needs of industrial structure adjustment to a certain extent (O’Mahony and Vecchi, 2005), there may be a situation that digital trade and the human capital structure jointly drive the improvement of GTFP (H2 confirmed). The fixed effect and quantile regression results show that the synergy of digital trade and human capital to improve the efficiency of green development has been revealed, but this effect is still weak and needs to be further strengthened.
5.3 Estimation of the Influence Mechanism Between IDT and RD
The impact of digital trade on GTFP is significantly negative in the fixed effect model and at the 10th, 25th, 50th, 75th, and 90th quantiles, indicating that the expansion of the scale of digital trade does not lead to the improvement of environmental efficiency. The interaction term of digital trade and R&D investment has a significant positive effect on GTFP in Table 5, and the estimation results of the two models are consistent. The improvement of the technological R&D level under the guidance of ICT digital trade can strengthen the resource supply of emerging industries and the transformation and upgrading of traditional industries, and consolidate and enhance the promotion effect of digital trade on GTFP (H3 confirmed). The influence of human capital still remains insignificant. The impact of the R&D investment on GTFP is significantly positive from the 10th to 90th quantile, implying that a marginal increasing effect exists and continuous efforts should be made to improve the promotion of the R&D level on GTFP. The other variables of infrastructure and population density still maintain a significant positive impact on GTFP, and on the whole, the impact intensity is increasing from a low quantile to high quantile.
5.4 Estimation of Subgroups Categorized by Region
The interaction between digital trade and human capital and R&D shows a positive impact in the three sub-regions, and the significance is pretty high in Table 6. However, in column (4), R&D investment variables have a significant negative impact on the central region. This shows that, in fact, the R&D investment may have a dual impact on green technology progress (Ulucak and Khan, 2020). On the one hand, as the overall R&D investment increases, the green R&D investment of enterprises will increase; accordingly, the progress of green technology can thus be achieved. On the other hand, for the central region, there may be no green bias in the regional R&D investment. Too much R&D investment enters the field of non-cleaner production, which inhibits the development of the regional green industry but is not conducive to the advancement of green technology. For the other variables, the infrastructure in the western region is negative but not significant. Although the western region is sparsely populated and the per capita road area is vast, there is no evidence for the promotion of GTFP in this study. The eastern region with the most concentrated population density also has the most obvious enhancement intensity to GTFP, which decreases, in turn, in the central and western regions.
5.5 Test of Robustness
To ensure the consistency of the results, a series of robustness tests are carried out in this study. In order to separate the impact of time trend on empirical results, we divide the whole period into two roughly equal time periods, namely, 2002–2009 and 2010–2018. The analysis results are shown in columns (1) to (4) of Table 7. The coefficient sign of the core explanatory variable is still significant, and the sign remains unchanged. Then, we replace the model regression method with feasible generalized least squares (FGLS) to overcome the heteroscedasticity and autocorrelation problems that may lead to the deviation of estimation results in the data. The results are shown in columns (5) and (6). Due to the virtual characteristics of digital trade, it is difficult to find a new instrumental variable. Therefore, this study takes the one-period lagged L.IDT of IDT as the instrumental variable and adopts the two-stage least square method (2SLS). Additionally, the whole sample data are divided into coastal provinces and inland provinces by the geographic feature. The results are reported in columns (7) to (10). The interaction terms of digital trade, human capital, and technological R&D still show significant positive effects, and the results related to the other variables are also consistent with the original model, which all indicate that the regression results are robust.
5.6 Discussion
Digital trade promotes the improvement of technical efficiency, but at this stage, the weakness of key core technology links in China and the siphon effect of talents and funds brought by digital industrialization lead to the hindrance of digital trade to GTFP (Anh Tu et al., 2020). For the regional level, the development of digital trade in the eastern region is significantly ahead of that in the central and western regions. The interaction with human capital and technological R&D leads to the rapid growth of China’s GTFP (Yu et al., 2021). When the coupling between digital technology and the original production mode is insufficient, the savage growth of the digital trade volume is likely to engender an adverse effect on GTFP. This argument has also been testified by other researchers (Asongu, 2018; Jorisch et al., 2018; Noussan and Tagliapietra, 2020). Excessive informatization will indirectly inhibit the growth of GTFP through the mechanism of resource waste and labor mismatch in the similar study conducted by Acemoglu and Restrepo (2019).With the rapid development of capital deepening among regional industries, the increase of ICT investment has led to the expansion of the scale of digital trade, and some industries even have excess investment (Ishida, 2015). According to the law of diminishing marginal returns, the space for digital technology progress is bound to be compressed (Shabani and Shahnazi, 2019), which also confirms the research results of this study. When the ICT-based digital trade lacks systematic planning, it will lead to the disconnection between the cloud of the information system and the cloud of the business process, which will make it difficult to realize the advantages of the digital cloud technology in improving productivity. On the contrary, it will increase the early investment cost, resulting in a serious asymmetry between the marginal output and marginal cost of new ICT technology (Raheem et al., 2020). The research finding is consistent with the previous similar studies (Andreopoulou, 2012; Moyer and Hughes, 2012; Shehzad et al., 2021). The promotion of digital trade to GTFP depends on its effective combination with human capital and technological R&D, in order to sufficiently give full play to the positive impact of the technology effect and structure effect. In the process of energy consumption, digital products and services can promote the enhancement of the energy utilization efficiency and pollutant treatment level of enterprises and, simultaneously, fulfill the effect of digital trade on energy consumption, energy conservation, and environmental pollutant emission reduction (Lange et al., 2020).
6 Conclusions and Policy Implications
6.1. Concluding Remarks
Digital trade is an important application of digital technology in the economic field and is becoming a new engine for world economic recovery. Under the background of the rapid growth in the digital trade scale, the intelligent transformation of the manufacturing industry, and the rise of the global value chain, this study selects the panel data of China’s provincial ICT-based digital trade volume from 2002 to 2018 to comprehensively and systematically explore the logical relationship between digital trade openness and green total factor productivity. It is found that the scale effect of digital trade has a significant negative impact on GTFP, which indicates the blind expansion of the scale is not desirable, but scientific planning is required. The structure effect of digital trade combined with human capital and the technology effect associated with technological R&D significantly enhance GTFP, and the impact intensity gradually increases from a low quantile to high quantile. The conclusions of this study provide vital reference for decision-makers. On the basis of the research findings, the corresponding policy suggestions are proposed as follows.
6.2 Policy Implications
1) Emphasize on the penetration and connection effect of the new generation of ICT, and strengthen the construction of enterprise informatization. Promote the R&D and innovation of equipment technology, and integrate and utilize information and communication infrastructure. Improve the technological innovation of the manufacturing industry, and build a new modern industrial system with green and high productivity. Master the laws of human capital and technology R&D in regulating the relationship between digital trade and GTFP, and adhere to ecological priority according to the actual opening situation of digital trade in each region. Comprehensively promote the use of energy-saving and emission reduction technologies and clean productive processes, promote the greening of production technology, and improve the level of green technology in various regions. China should also promote the transformation and upgrading of the foreign digital trade structure, improve the efficiency of resource allocation, and contribute to the growth of GTFP.
2) Expand digital trade openness and broaden the field of industrial cooperation. Accelerate the transformation from traditional trade to digital trade, reduce the dependence on low value-added, high energy consumption, and high pollution industries in trade exports, and force the upgrading of the digital trade industrial structure by adopting strict environmental protection and energy consumption standards. In addition, China needs to expand the digital import trade of high and new technologies, especially increase the import of green and clean technologies, and actively guide the effective cooperation with technological R&D. Improve the ability of enterprises to digest, absorb, and use green and clean technologies; as a result, give full play to the positive and net effect of digital trade opening on promoting the improvement of GTFP.
3) Optimize the industrial structure of digital trade and accelerate the development of core industries of digital trade. At present, China is in the rapid development stage of the digital industry, which shows that China’s traditional enterprises are constantly transforming into digital enterprises. Therefore, it is necessary to further optimize the digital industrial structure and facilitate the enhancement of GTFP. Besides, China should actively promote the deep integration of digital technology and the real economy, innovate, and carry out the “digital technology plus” development model. Furthermore, utilize digital technology and the digital trade industry to drive the transformation and upgrading of traditional industries. On the other hand, China should actively promote the rapid development of the software and information industry, Internet industry, and digital communication industry which are closely related to the digital trade. Build a large-scale industrial cluster for coordinated development with the digital trade industry, and realize the intensive development of the digital trade industry.
4) Gradually promote the transformation of digital trade from relying on quantity and scale to product quality. Continuously improve the technical content and product added value of import and export goods and help regional enterprises actively implement technological innovation and independent R&D. Focus on developing and cultivating emerging technologies and high-tech industries. Jointly build a digital industry ecosystem with a regional economic driving role and international competitive advantage, and eventually promote the continuous and steady improvement of regional GTFP. From the regional perspective, the development level of digital trade in eastern, central, and western China is different. The digital infrastructure and digital industry in the eastern region are relatively developed. Human capital, technology R&D, and digital trade should be more scientifically utilized to improve GTFP. The development of digital trade in the central and western regions is comparatively backward, which cannot effectively accelerate the elevation of GTFP. The local governments should ameliorate the structure of trade in digital products and services. Furthermore, strengthen policy guidance, and emphasize the trade of high-tech and high value-added clean products. Meanwhile, expedite the formation of the inter-regional transportation network to better stimulate the flow of factors among regions.
6.3 Limitations and Recommendations to Future Studies
This study sorts out the impact mechanism of digital trade on GTFP, but there are still some limitations. First, the measurement of digital trade can build a more detailed index system, and select as many indicators as possible to measure comprehensively. However, the workload of this method is relatively large, and it is difficult to obtain data. At present, the statistical monitoring system of digital trade is not perfect, so we can only collect appropriate indicators for empirical research at this stage. Subject to the availability of data, although the data of 17 years and 30 provinces have met the requirements of panel modeling, it will undoubtedly make the research more universal if there is a longer time series and a wider cross section. With the passage of time and continuous optimization of the digital trade statistics system, the consistency and international comparability of indicators will be more improved. Second, in dealing with endogenous problems, we failed to find a better instrumental variable creatively. Third, in the empirical analysis part, this study uses small sample data at the provincial level. An obvious limitation is that the provincial level data ignore the individual heterogeneity of manufacturing enterprises.
Although this study has made a beneficial exploration on the growth path of GTFP by digital trade and obtained some conclusions and enlightenments, it still needs to perform more comprehensive research in future studies. First, further refine the analysis of the transmission mechanism of path transformation, and consolidate the theoretical basis of relevant research. Second, continue to optimize the model design and consider practical and dynamic factors to make it more scientific and effective. Third, use more consistent and long-term data to perform empirical research and enrich the quantitative conclusions, which can provide empirical support for the formulation of relevant policies. Fourth, with the continuous improvement of the database, it is necessary to conduct more in-depth investigation on enterprise data in terms of scope and time. When the data are more comprehensive, the findings will be more representative. Fifth, further refine the research on GTFP of enterprises in a certain sector of the digital trade industry to formulate more detailed, targeted, and differentiated development strategies.
Data Availability Statement
Publicly available datasets were analyzed in this study. This data can be found here: https://databank.worldbank.org/source/world-development-indicators.
Author Contributions
The author confirms being the sole contributor of this work and has approved it for publication.
Funding
The study is supported by the Priority Academic Program Development (PAPD) of Jiangsu Higher Education Institutions, the Youth Project of Social Science Foundation of Jiangsu Province (Grant No. 19EYC003), and the Teaching Reform Project of Higher Education Institute of Nanjing Audit University in 2021 (Grant No. 2021JG021). All individuals included in this section have consented to the acknowledgment.
Conflict of Interest
The author declares that the research was conducted in the absence of any commercial or financial relationships that could be construed as a potential conflict of interest.
Publisher’s Note
All claims expressed in this article are solely those of the authors and do not necessarily represent those of their affiliated organizations, or those of the publisher, the editors, and the reviewers. Any product that may be evaluated in this article, or claim that may be made by its manufacturer, is not guaranteed or endorsed by the publisher.
References
Acemoglu, D., and Restrepo, P. (2019). 8. Artificial Intelligence, Automation, and Work. Chicago: University of Chicago Press.
Ahmed, Z., and Le, H. P. (2021). Linking Information Communication Technology, Trade Globalization index, and CO2 Emissions: Evidence from Advanced Panel Techniques. Environ. Sci. Pollut. Res. 28 (7), 8770–8781. doi:10.1007/s11356-020-11205-0
Amiti, M., and Konings, J. (2007). Trade Liberalization, Intermediate Inputs, and Productivity: Evidence from Indonesia. Am. Econ. Rev. 97 (5), 1611–1638. doi:10.1257/aer.97.5.1611
Amri, F., Zaied, Y. B., and Lahouel, B. B. (2019). ICT, Total Factor Productivity, and Carbon Dioxide Emissions in Tunisia. Technol. Forecast. Soc. Change 146, 212–217. doi:10.1016/j.techfore.2019.05.028
Andreopoulou, Z. (2012). Green Informatics: ICT for green and Sustainability. Agrárinformatika/Journal Agric. Inform. 3 (2), 1–8. doi:10.17700/jai.2012.3.1.85
Anh Tu, C., Sarker, T., and Rasoulinezhad, E. (2020). Factors Influencing the green Bond Market Expansion: Evidence from a Multi-Dimensional Analysis. Jrfm 13 (6), 126. doi:10.3390/jrfm13060126
Antonelli, C. (2016). Technological Congruence and the Economic Complexity of Technological Change. Struct. Change Econ. Dyn. 38, 15–24. doi:10.1016/j.strueco.2015.11.008
Antweiler, W., Copeland, B. R., and Taylor, M. S. (2001). Is Free Trade Good for the Environment. Am. Econ. Rev. 91 (4), 877–908. doi:10.1257/aer.91.4.877
Asongu, S. A. (2018). ICT, Openness and CO2 Emissions in Africa. Environ. Sci. Pollut. Res. 25 (10), 9351–9359. doi:10.1007/s11356-018-1239-4
Bandyopadhyay, D. (2006). How Financial Development Caused Economic Growth in APEC Countries: Financial Integration with FDI or Privatization without FDI. Asia-Pacific Develop. J. 13 (1), 75–100. doi:10.18356/88e3aae6-en
Bastida, L., Cohen, J. J., Kollmann, A., Moya, A., and Reichl, J. (2019). Exploring the Role of ICT on Household Behavioural Energy Efficiency to Mitigate Global Warming. Renew. Sustain. Energ. Rev. 103, 455–462. doi:10.1016/j.rser.2019.01.004
Bernstein, R., and Madlener, R. (2010). Impact of Disaggregated ICT Capital on Electricity Intensity in European Manufacturing. Appl. Econ. Lett. 17 (17), 1691–1695. doi:10.1080/13504850903120717
Borga, M., and Koncz-Bruner, J. (2012). Trends in Digitally-Enabled Trade in Services. Washington, DC: Bureau of Economic Analysis US Department of Commerce.
Can, M., Dogan, B., and Saboori, B. (2020). Does Trade Matter for Environmental Degradation in Developing Countries? New Evidence in the Context of export Product Diversification. Environ. Sci. Pollut. Res. Int. 27, 14702–14710. doi:10.1007/s11356-020-08000-2
Cao, B., and Wang, S. (2017). Opening up, International Trade, and green Technology Progress. J. Clean. Prod. 142, 1002–1012. doi:10.1016/j.jclepro.2016.08.145
Cao, S., Nie, L., Sun, H., Sun, W., and Taghizadeh-Hesary, F. (2021). Digital Finance, green Technological Innovation and Energy-Environmental Performance: Evidence from China's Regional Economies. J. Clean. Prod. 327, 129458. doi:10.1016/j.jclepro.2021.129458
Chen, X., Gong, X., Li, D., and Zhang, J. (2019). Can Information and Communication Technology Reduce CO2 Emission? A Quantile Regression Analysis. Environ. Sci. Pollut. Res. 26 (32), 32977–32992. doi:10.1007/s11356-019-06380-8
Cheng, C., Ren, X., Dong, K., Dong, X., and Wang, Z. (2021). How Does Technological Innovation Mitigate CO2 Emissions in OECD Countries? Heterogeneous Analysis Using Panel Quantile Regression. J. Environ. Manag. 280, 111818. doi:10.1016/j.jenvman.2020.111818
Cheng, C., Ren, X., Wang, Z., and Yan, C. (2019). Heterogeneous Impacts of Renewable Energy and Environmental Patents on CO2 Emission - Evidence from the BRIICS. Sci. total Environ. 668, 1328–1338. doi:10.1016/j.scitotenv.2019.02.063
Chung, Y. H., Färe, R., and Grosskopf, S. (1997). Productivity and Undesirable Outputs: a Directional Distance Function Approach. J. Environ. Manag. 51 (3), 229–240. doi:10.1006/jema.1997.0146
Collard, F., Fève, P., and Portier, F. (2005). Electricity Consumption and ICT in the French Service Sector. Energ. Econ. 27 (3), 541–550. doi:10.1016/j.eneco.2004.12.002
Dehghan Shabani, Z., and Shahnazi, R. (2019). Energy Consumption, Carbon Dioxide Emissions, Information and Communications Technology, and Gross Domestic Product in Iranian Economic Sectors: A Panel Causality Analysis. Energy 169, 1064–1078. doi:10.1016/j.energy.2018.11.062
Dong, K., Dong, X., and Ren, X. (2020). Can Expanding Natural Gas Infrastructure Mitigate CO2 Emissions? Analysis of Heterogeneous and Mediation Effects for China. Energ. Econ. 90, 104830. doi:10.1016/j.eneco.2020.104830
Dong, K., Ren, X., and Zhao, J. (2021b). How Does Low-Carbon Energy Transition Alleviate Energy Poverty in China? A Nonparametric Panel Causality Analysis. Energ. Econ. 103, 105620. doi:10.1016/j.eneco.2021.105620
Dong, X., Jiang, Q., and Wang, J. (2021a). Assessing Embodied Carbon Emission and its Intensities in the ICT Industry: The Global Case. Front. Energ. Res. 9, 187. doi:10.3389/fenrg.2021.685021
Doukas, H., Marinakis, V., Tsapelas, J., and Sgouridis, S. (2019). Intelligent Energy Management within the Smart Cities: an EU-GCC Cooperation Opportunity. Smart Cities in the Gulf 8. 123–147. doi:10.1007/978-981-13-2011-8_8
Fefer, R. F. (2017). Digital Trade and US Trade Policy. Curr. Polit. Econ. United States Can. Mexico 19 (1), 1–52.
Ghali, S., Zitouna, H., Karray, Z., and Driss, S. (2013). Trade, Transaction Costs and TFP: Evidence from Tunisia and Egypt. Cairo: ERF Working Paper Series.
Goldbach, K., Rotaru, A. M., Reichert, S., Stiff, G., and Gölz, S. (2018). Which Digital Energy Services Improve Energy Efficiency? A Multi-Criteria Investigation with European Experts. Energy Policy 115, 239–248. doi:10.1016/j.enpol.2017.12.036
Goldberg, P. K., and Pavcnik, N. (2007). Distributional Effects of Globalization in Developing Countries. J. Econ. Lit. 45 (1), 39–82. doi:10.1257/jel.45.1.39
Haftu, G. G. (2019). Information Communications Technology and Economic Growth in Sub-saharan Africa: A Panel Data Approach. Telecommunications Policy 43 (1), 88–99. doi:10.1016/j.telpol.2018.03.010
Hall, R. E., and Jones, C. I. (1999). Why Do Some Countries Produce So Much More Output Per Worker Than Others. Q. J. Econ. 114 (1), 83–116. doi:10.1162/003355399555954
Hassine, N. B., and Kandil, M. (2009). Trade Liberalisation, Agricultural Productivity and Poverty in the Mediterranean Region. Eur. Rev. Agric. Econ. 36 (1), 1–29. doi:10.1093/erae/jbp002
Hodrab, R., Maitah, M., and Smutka, L. (2016). The Effect of Information and Communication Technology on Economic Growth: Arab World Case. Int. J. Econ. Financial Issues 6 (2), 765–775.
Hu, G., Can, M., Paramati, S. R., Doğan, B., and Fang, J. (2020). The Effect of Import Product Diversification on Carbon Emissions: New Evidence for Sustainable Economic Policies. Econ. Anal. Pol. 65, 198–210. doi:10.1016/j.eap.2020.01.004
Ishida, H. (2015). The Effect of ICT Development on Economic Growth and Energy Consumption in Japan. Telematics Inform. 32 (1), 79–88. doi:10.1016/j.tele.2014.04.003
Jiang, X., and Liu, Y. (2015). Global Value Chain, Trade and Carbon: Case of Information and Communication Technology Manufacturing Sector. Energ. Sustain. Develop. 25, 1–7. doi:10.1016/j.esd.2014.12.001
Jorisch, D., Mallin, C. A., Accurso, M., García Zaballos, A., and Iglesias Rodríguez, E. (2018). Technology for Climate Action in Latin America and the Caribbean: How ICT Mobile Solutions Contribute to a Sustainable, Low-Carbon Future. Washington: GSMA. South Pole.
Khan, N., Baloch, M. A., Saud, S., and Fatima, T. (2018). The Effect of ICT on CO2 Emissions in Emerging Economies: Does the Level of Income Matters. Environ. Sci. Pollut. Res. 25 (23), 22850–22860. doi:10.1007/s11356-017-0719-2
Klevorick, A. K., Levin, R. C., Nelson, R. R., and Winter, S. G. (1995). On the Sources and Significance of Interindustry Differences in Technological Opportunities. Res. Pol. 24 (2), 185–205. doi:10.1016/0048-7333(93)00762-i
Koenker, R., and Bassett, G. (1978). Regression Quantiles. Econometrica 46, 33–50. doi:10.2307/1913643
Lange, S., Pohl, J., and Santarius, T. (2020). Digitalization and Energy Consumption. Does ICT Reduce Energy Demand. Ecol. Econ. 176, 106760. doi:10.1016/j.ecolecon.2020.106760
Li, K.-Y., Gong, W.-C., and Choi, B.-R. (2021). The Influence of Trade and Foreign Direct Investment on Green Total Factor Productivity: Evidence from China and Korea. J. Korea Trade 25, 95–110. doi:10.35611/jkt.2021.25.2.95
Li, L., and Liu, Y. (2017). Industrial green Spatial Pattern Evolution of Yangtze River Economic Belt in China. Chin. Geogr. Sci. 27 (4), 660–672. doi:10.1007/s11769-017-0893-7
Li, Y., Dai, J., and Cui, L. (2020). The Impact of Digital Technologies on Economic and Environmental Performance in the Context of Industry 4.0: A Moderated Mediation Model. Int. J. Prod. Econ. 229, 107777. doi:10.1016/j.ijpe.2020.107777
Liddle, B. (2018). Consumption-based Accounting and the Trade-Carbon Emissions Nexus. Energ. Econ. 69, 71–78. doi:10.1016/j.eneco.2017.11.004
Lin, H.-L., Li, H.-Y., and Yang, C.-H. (2011). Agglomeration and Productivity: Firm-Level Evidence from China's Textile Industry. China Econ. Rev. 22 (3), 313–329. doi:10.1016/j.chieco.2011.03.003
Luo, Z., Wan, G., Wang, C., and Zhang, X. (2018). Urban Pollution and Road Infrastructure: A Case Study of China. China Econ. Rev. 49, 171–183. doi:10.1016/j.chieco.2017.04.008
Moyer, J. D., and Hughes, B. B. (2012). ICTs: Do They Contribute to Increased Carbon Emissions. Technol. Forecast. Soc. Change 79 (5), 919–931. doi:10.1016/j.techfore.2011.12.005
Murshed, M., Chadni, M. H., and Ferdaus, J. (2020). Does ICT Trade Facilitate Renewable Energy Transition and Environmental Sustainability? Evidence from Bangladesh, India, Pakistan, Sri Lanka, Nepal and Maldives. Energ. Ecol. Environ. 5 (6), 470–495. doi:10.1007/s40974-020-00190-2
Nath, H. K., and Liu, L. (2017). Information and Communications Technology (ICT) and Services Trade. Inf. Econ. Pol. 41, 81–87. doi:10.1016/j.infoecopol.2017.06.003
Niebel, T. (2018). ICT and Economic Growth - Comparing Developing, Emerging and Developed Countries. World Develop. 104, 197–211. doi:10.1016/j.worlddev.2017.11.024
Noussan, M., and Tagliapietra, S. (2020). The Effect of Digitalization in the Energy Consumption of Passenger Transport: An Analysis of Future Scenarios for Europe. J. Clean. Prod. 258, 120926. doi:10.1016/j.jclepro.2020.120926
O'Mahony, M., and Vecchi, M. (2005). Quantifying the Impact of ICT Capital on Output Growth: a Heterogeneous Dynamic Panel Approach. Economica 72 (288), 615–633. doi:10.1111/j.1468-0335.2005.0435.x
Ohlan, R. (2015). The Impact of Population Density, Energy Consumption, Economic Growth and Trade Openness on CO2 Emissions in India. Nat. Hazards 79 (2), 1409–1428. doi:10.1007/s11069-015-1898-0
Park, Y., Meng, F., and Baloch, M. A. (2018). The Effect of ICT, Financial Development, Growth, and Trade Openness on CO2 Emissions: an Empirical Analysis. Environ. Sci. Pollut. Res. 25 (30), 30708–30719. doi:10.1007/s11356-018-3108-6
Peng, Y., Chen, Z., Xu, J., and Lee, J. (2020). Analysis of green Total Factor Productivity Trend and its Determinants for the Countries along Silk Roads. Growth and Change 51 (4), 1711–1726. doi:10.1111/grow.12435
Raheem, I. D., Tiwari, A. K., and Balsalobre-Lorente, D. (2020). The Role of ICT and Financial Development in CO2 Emissions and Economic Growth. Environ. Sci. Pollut. Res. 27 (2), 1912–1922. doi:10.1007/s11356-019-06590-0
Ren, X., Cheng, C., Wang, Z., and Yan, C. (2021). Spillover and Dynamic Effects of Energy Transition and Economic Growth on Carbon Dioxide Emissions for the European Union: A Dynamic Spatial Panel Model. Sustain. Develop. 29 (1), 228–242. doi:10.1002/sd.2144
Ren, X., Lu, Z., Cheng, C., Shi, Y., and Shen, J. (2019). On Dynamic Linkages of the State Natural Gas Markets in the USA: Evidence from an Empirical Spatio-Temporal Network Quantile Analysis. Energ. Econ. 80, 234–252. doi:10.1016/j.eneco.2019.01.001
Santarius, T., Pohl, J., and Lange, S. (2020). Digitalization and the Decoupling Debate: Can ICT Help to Reduce Environmental Impacts while the Economy Keeps Growing. Sustainability 12 (18), 7496. doi:10.3390/su12187496
Schulte, P., Welsch, H., and Rexhäuser, S. (2016). ICT and the Demand for Energy: Evidence from OECD Countries. Environ. Resource Econ. 63 (1), 119–146. doi:10.1007/s10640-014-9844-2
Shahbaz, M., and Sinha, A. (2019). Environmental Kuznets Curve for CO2 Emissions: a Literature Survey. J. Econ. Stud. 46, 106–168. doi:10.1108/JES-09-2017-0249
Shao, W., Yin, Y., Bai, X., and Taghizadeh-Hesary, F. (2021). Analysis of the Upgrading Effect of the Industrial Structure of Environmental Regulation—Evidence from 113 Cities in China. Front. Environ. Sci. 9, 232. doi:10.3389/fenvs.2021.692478
Sharma, G. D., Rahman, M. M., Jain, M., and Chopra, R. (2021). Nexus between Energy Consumption, Information and Communications Technology, and Economic Growth: an Enquiry into Emerging Asian Countries. J. Public Aff. 21 (2), e2172. doi:10.1002/pa.2172
Shehzad, K., Xiaoxing, L., and Sarfraz, M. (2021). Envisaging the Asymmetrical Association Among FDI, ICT, and Climate Change: a Case from Developing Country. Carbon Manag. 12 (2), 123–137. doi:10.1080/17583004.2021.1890449
Solarin, S. A., Shahbaz, M., Khan, H. N., and Razali, R. B. (2021). ICT, Financial Development, Economic Growth and Electricity Consumption: New Evidence from Malaysia. Glob. Business Rev. 22 (4), 941–962. doi:10.1177/0972150918816899
Su, C.-W., Xie, Y., Shahab, S., Faisal, C. M. N., Hafeez, M., and Qamri, G. M. (2021). Towards Achieving Sustainable Development: Role of Technology Innovation, Technology Adoption and CO2 Emission for BRICS. Ijerph 18 (1), 277. doi:10.3390/ijerph18010277
Ulucak, R., and Khan, S. U. D. (2020). Does Information and Communication Technology Affect CO 2 Mitigation under the Pathway of Sustainable Development during the Mode of Globalization. Sustain. Develop. 28 (4), 857–867. doi:10.1002/sd.2041
Wang, D., and Han, B. (2016). The Impact of ICT Investment on Energy Intensity across Different Regions of China. J. Renew. Sustain. Energ. 8 (5), 055901. doi:10.1063/1.4962873
Yan, D., Kong, Y., Ren, X., Shi, Y., and Chiang, S. (2019). The Determinants of Urban Sustainability in Chinese Resource-Based Cities: A Panel Quantile Regression Approach. Sci. total Environ. 686, 1210–1219. doi:10.1016/j.scitotenv.2019.05.386
Yan, D., Ren, X., Kong, Y., Ye, B., and Liao, Z. (2020). The Heterogeneous Effects of Socioeconomic Determinants on PM2.5 Concentrations Using a Two-step Panel Quantile Regression. Appl. Energ. 272, 115246. doi:10.1016/j.apenergy.2020.115246
Yan, Z., Shi, R., and Yang, Z. (2018). ICT Development and Sustainable Energy Consumption: a Perspective of Energy Productivity. Sustainability 10 (7), 2568. doi:10.3390/su10072568
Yu, Y., Li, S., Sun, H., and Taghizadeh-Hesary, F. (2021). Energy Carbon Emission Reduction of China's Transportation Sector: An Input-Output Approach. Econ. Anal. Pol. 69, 378–393. doi:10.1016/j.eap.2020.12.014
Zhang, D., Mohsin, M., Rasheed, A. K., Chang, Y., and Taghizadeh-Hesary, F. (2021). Public Spending and green Economic Growth in BRI Region: Mediating Role of green Finance. Energy Policy 153, 112256. doi:10.1016/j.enpol.2021.112256
Zhao, T., (2020). Digital Economy, Entrepreneurship, and High-Quality Economic Development: Empirical Evidence from Urban China. Manag. World 36 (10), 65–76.
Keywords: trade openness, digital trade, green total factor productivity, China, information and communication technology
Citation: Zhang H (2021) Trade Openness and Green Total Factor Productivity in China: The Role of ICT-Based Digital Trade. Front. Environ. Sci. 9:809339. doi: 10.3389/fenvs.2021.809339
Received: 04 November 2021; Accepted: 29 November 2021;
Published: 20 December 2021.
Edited by:
Farhad Taghizadeh-Hesary, Tokai University, JapanReviewed by:
Ehsan Rasoulinezhad, University of Tehran, IranXiaohang Ren, Central South University, China
Copyright © 2021 Zhang. This is an open-access article distributed under the terms of the Creative Commons Attribution License (CC BY). The use, distribution or reproduction in other forums is permitted, provided the original author(s) and the copyright owner(s) are credited and that the original publication in this journal is cited, in accordance with accepted academic practice. No use, distribution or reproduction is permitted which does not comply with these terms.
*Correspondence: Huan Zhang, 441264219@qq.com