- 1Nelson Institute for Environmental Studies, University of Wisconsin-Madison, Madison, WI, United States
- 2U.S. Geological Survey, National Land Imaging Program and Social and Economic Analysis Branch, Fort Collins Science Center, Fort Collins, CO, United States
- 3College of University Libraries and Learning Sciences, University of New Mexico, Albuquerque, NM, United States
It is well-known that Earth observation (EO) data plays a critical role in scientific understanding about the global environment. There is also growing support for the use of EO data to provide context-specific insights, with significant implications for their use in decision support systems. Technological development over recent years, including cloud computing infrastructure, machine learning techniques, and rapid expansion of the velocity, volume, and variety of space-borne data sources, offer huge potential to provide solutions to the myriad environmental problems facing society and the planet. The USGS/NASA Landsat Program, the longest continuously gathered source of land surface data, has played a central role in our understanding of environmental change, particularly for its contribution of longitudinal products that offer greater context for present research and decision support activities. The challenge facing the Landsat and EO data community, however, now lies in moving beyond context-specific knowledge generation to translating such knowledge into tangible value for society. Drawing from an open data ecosystem framework and qualitative social science methods, we map the Landsat data ecosystem (LDE) and the relationships linking multiple actors responsible for processing, indexing, analyzing, synthesizing, and translating raw Landsat data into information that is useful, useable, and used by end users in particular social-environmental contexts. Both the role of Big Data and associated technologies are discussed as they relate to the ultimate use of Landsat-derived information products to guide decision-making, and key data ecosystem characteristics that shape the likelihood of these products’ use are highlighted.
Introduction
The Landsat Program is a series of Earth-observing satellite missions jointly managed by the United States Geological Survey (USGS) and the National Aeronautics and Space Administration (NASA). NASA oversees satellite construction and launch. The USGS directs Landsat flight operations; coordinates the ground receiving station network; and manages the acquisition, production, calibration, validation, and distribution of Landsat data and derived products for users worldwide. USGS Earth Resources Observation and Science Center (EROS) is home to the world’s largest collection of remotely sensed images of the Earth’s land surface and the primary source of Landsat satellite images and data products. The Landsat Program’s continuous archive (1972-present) provides a landscape-level view of Earth that enables users to better understand the scope, nature, and speed of change to the natural and built environment. Landsat represents the world’s longest continuously acquired collection of space-based moderate-resolution land remote sensing data. Business, government, nonprofit, and academic users place a high value on Landsat data for various applications. Landsat data contribute to decisions about land, water, and resource use that protect life, property, and the environment; advance science, technology, and education; and grow economies. Understanding the value of Landsat data also takes many forms.
The topic of value broadly defined has been considered across academic disciplines and through time (Brown, 1984). Working in the context of the telecommunications industry, Li and Whalley (2002) expanded the idea of a single linear value chain. They posited the idea of “value networks,” describing them as “composed of all the different actors drawn from a range of industries that collectively provide goods and service to the end users,” (p.456) and noting that this conceptualization better captured the growing number of “entry and exit points” (p.453) to the system. When applied in the context of Earth observation (EO) data users, the “value network” framework captures the growing number and types of actors and the connections between them. The multidirectional flow of information between system actors may thus be traced along various and overlapping value chains. A “data ecosystem” refers to this network of interacting system actors that directly or indirectly consume, produce, or provide data and related resources (Oliveira et al., 2019).
As discussed in the 2019 National Plan for Civil Earth Observations, measuring and reporting on the value of EO data is difficult, even though its use increases knowledge and quality of life in myriad ways. Complicating the task of valuing public-domain EO data (such as Landsat) are its characteristics as a public good: 1) it is non-exclusionary and therefore open and usable to all, and 2) it is non-competitive in use meaning it can be used without limiting others’ ability to use it (U.S. Group on Earth Observations, 2019). Indeed, the ability of many actors to employ Landsat data because of its free and open data policy contributes to its value (National Geospatial Advisory Committee Landsat Advisory Group, 2019), but also complicates the measurement of that value.
Nevertheless, past and present efforts have attempted to classify the monetary and non-monetary value of EO data, including those by governmental agencies such as NASA, USGS, and the National Oceanic and Atmospheric Administration (NOAA), as well as by communities of practice (e.g., GEOValue), academic researchers, and others. A range of approaches have been used to measure the value of EO data including the Value of Information framework (e.g., NASA’s VALUABLES (Resources for the Future, 2021)), market valuation (e.g., Copernicus Market Report (The European Commission and PwC Advisory France, 2019)), and benefit chain (e.g., Fritz et al., 2008). Landsat data is widely viewed as a strong investment from an economic value perspective (Campbell, 2015; Loomis et al., 2015). However, “economic measures of value are a small subset of what is encompassed by value” (Brown, 1984, pg. 239).
Growing numbers of users of EO data increase the value of the data itself (Virapongse et al., 2020). Studies employing “use-driven” or “end-user” approaches are one tool to facilitate this by understanding the needs of EO data users. For example, the GEOEssential project began by looking at the end goal for EO data (to inform policy, such as the United Nations’ Sustainable Development Goals), and working backwards to identify the indicators needed to inform policy, the “Essential Variables” (EVs) (variables that significantly affect the “Earth system”) that contribute to those indicators, and finally the EO data sources needed to create the EVs (Lehmann et al., 2020).
Aggregate user needs studies have also contributed to understanding the EO data most important to users and have spurred the development of value-added EO data and service providers that serve as intermediaries between EO originators such as USGS and EO data users such as researchers or policy makers. One such study integrated the idea of following a “chain of users—from researchers to intermediate users to end users,” to help “characterize this flow of data among users” (Zell et al., 2012, pg. 1600). Another, the GEO-Bene Project, recognized that the value of EO data cannot always best be captured in exact economic terms. The data and decisions made with it often contribute to things not easily valued in monetary terms, including public goods, information for which recipients could not pay its market price, and general societal benefit (Fritz et al., 2008). These studies make important contributions to knowledge of user needs but do not explore created value from the user perspective when these needs are met.
This data provider and data user centered approach complements the more vertically integrated technical perspective that is embedded in services oriented architectural (SOA) models and processing workflows for geospatial data broadly, and EO data specifically such as those described by Yang et al. (2010); Vescoukis et al. (2012); Li et al. (2015); Li et al. (2020). These models emphasize the technical components (e.g., data capture, storage, processing, analysis, encoding, visualization) and the interaction between these component systems through both standards-based web services (e.g., the Open Geospatial Consortium’s web map, web feature, and web coverage services (OGC Standards, 2021)) and custom service interfaces. While critical for meeting the technical requirements for efficient exchange of data between systems, a systematic understanding of the actors and their roles within these technical architectures, the ecosystem focus of this paper, is critical for meeting the needs of those users and providers as the landscape of EO data and services continues to evolve.
One component of describing the value of EO data is an understanding of the benefits or value it has for the user and their work (be that decision-making, creating a product, advocacy work, etc.) (Pearlman et al., 2019, pg. 4). In this paper we define value from the actor perspective as the qualitative benefits of decision support for end users of Landsat data and information products. This study aims to describe the value of Landsat data from actors’ perspectives. We trace some of the many paths data follows through an ecosystem of interlinked data sources, value-added data and service providers, and data users, where value accrues at each node of the data ecosystem. Additionally, we characterize technical and social factors of value in the growing ecosystem of EO data and service providers.
Methods
A qualitative approach was chosen for this initial, exploratory research into mapping the data ecosystem formed around Landsat data, or the Landsat data ecosystem (LDE). Qualitative research provides a deeper understanding of the LDE from the actors’ viewpoint. There are few studies that go beyond economic modelling of EO and investigate value from the perspective of the actors. Qualitative findings are based on themes, patterns, and relationships. Results in qualitative studies are grounded in the perspectives and observations of the specific interviewees in the analysis.
This study used a semi-structured interview format with open-ended questions. The interview questions covered four major topics related to the actors’ roles in the LDE: 1) where/how actors access Landsat data, 2) what actors do with the data, 3) what happens to the products created, and 4) what are the value, benefits, and challenges with using Landsat data. Data collected were rich, in-depth, and unique to each interviewee. Interviews were conducted via video call or phone call at a time coordinated by the interviewer and interviewee and lasted 30–60 min. If the interviewee consented, the interviewers recorded the audio from the interview for transcription into a text file for later analysis. For any interviews for which the interviewee did not consent to audio recording, the notes taken during and immediately after the interview by the interviewer were included for analysis instead of a transcript.
We targeted participation from various sectors throughout the data ecosystem to capture the depth and variation of specific use cases and understand the ecosystem components that support value-generation as Landsat data and derived products move from actor to actor. We searched via the internet for private companies and non-profit organizations who use Landsat data for Big Data applications and identified those that are most commonly referenced in geospatial industry news sites and professional organizations. We also used existing connections within the Landsat community to identify subject matter experts (SMEs) from the public and academic sectors who use Landsat for Big Data applications or are knowledgeable of these applications. Snowball sampling was used to identify additional interviewees. We asked interviewees to distribute the study recruitment email on our behalf. If additional actors wished to participate in the study, we moved forward with those interviews. Organizations or SMEs were included if they use Landsat for Big Data applications or produce platforms or analytics tools that allow users or customers to do so. Organizations or SMEs were excluded if they did not use Landsat data at all or did not use Landsat data for Big Data applications.
Twenty-six interviews were conducted. Interviewees represented a wide array of actor roles within the LDE. Cloud Service Providers (CSP) (n = 2), Data as a Service (DaaS) providers (n = 4), Platform as a Service (PaaS) providers (n = 5), Software as a Service (SaaS) providers (n = 2), Information Product as a Service (IPaaS) providers (n = 5), and End Users (n = 8) were the actor roles identified and interviewed within this study. These actor roles are defined and described below.
Each digital-recorded interview was transcribed verbatim. Data analysis was an iterative process that focused on identifying relevant themes, patterns, and relationships concerning how actors access Landsat data, what they do with Landsat data, how they deliver their products and services, and what the value, benefits, and challenges are with using Landsat data. Major themes were identified through the open coding of each individual interview transcript. We concluded by aggregating common themes that we found within the ecosystem. The actors are confidential, and the themes are presented in aggregate form. In addition to the interviews, the PIs used discourse analysis to review publicly available Earth observation user community case studies to find additional information about the value of Landsat. Relevant themes were incorporated into the qualitative analysis. This study has been verified as exempt from Institutional Review Board requirements according to 45CFR46.104(d)(2).
Results and Discussion
Mapping the Landsat Data Ecosystem
The LDE is composed of value chains that begin with strategic planning to develop and launch an Earth observing satellite, development of the infrastructure needed to process the data provided by the satellite, and the generation of initial products based on those data. The economic, scientific, technical, and political resources required to complete such a mission are immense. The topic of satellite hardware value chains has been studied elsewhere (The European Commission and PwC Advisory France, 2019), as have the technical aspects of services oriented architectures that enable geoscience analysis at scale (Yang et al., 2010; Vescoukis et al., 2012; Li et al., 2015; Li et al., 2020). In this paper we examine the LDE downstream of EROS by following the processing of raw reflectance data from the Landsat sensors into standardized Landsat data collections, Analysis Ready Data (ARD) products, and information products which are used in decision-making, with a focus on the organizational actors that participate in the addition of value to those products along the value chain, all from the actors’ perspective.
Landsat Data Ecosystem Actor Roles
The data ecosystem is complex. Entities in the LDE (referred to as “actors” in this analysis) store, process, analyze, and deliver data, platforms, software, services, and information products which travel through ecosystem nodes to downstream actors. In contrast with other analyses which focus on the technical elements that enable these activities (Yang et al., 2010; Vescoukis et al., 2012; Li et al., 2015; Li et al., 2020), the focus here is on the roles that the different actors play in the LDE. Discrete roles or categories of system actors are not clear-cut, leading to variation in conceptual classifications depending on the case study focus. Here, we focus on the societal value generated by Landsat data as it travels through the ecosystem and is applied to decision-making by end users. From three broad categories of data ecosystem actors–Data Originators, Data Intermediaries, and End Users–we further classified Data Intermediaries by their roles in facilitating data and value-added product transmission to downstream actors. These five categories are Cloud Service Providers (CSP), Data as a Service (DaaS) providers, Platform as a Service (PaaS) providers, Software as a Service (SaaS) providers, and Information Product as a Service (IPaaS) providers–aligning these with the more broadly used industry standard terms (Table 1). We developed our typology based on examples from our interview data of individual actors’ use of Landsat or Landsat-derived products rather than their organizations’ operations as a whole.
Overlap exists between actors in the tools they use and the value-added products they develop. For this study, we define an actor’s role in the LDE by the products or services they provide to downstream actors (Table 1). CSPs’ primary role is to provide generalized data storage and computational resources. DaaS providers host EO data products and provide the capability to build analytics infrastructure that uses web service access methods to interact with the hosted data products. PaaS providers frequently leverage these DaaS capabilities to develop and maintain user-accessible application programming interfaces (APIs) that facilitate Big EO data processing. SaaS providers perform many of the same tasks as PaaS providers, but they provide customized services for client requests rather than the more generic PaaS services through which users can build their own analytic and visualization workflows. DaaS, PaaS, and SaaS providers often develop similar infrastructure and analytical tools, and in some cases perform similar tasks, particularly in developing analytics architecture using CSP-provided resources. However, DaaS providers create value-added spatial data products rather than analytics platforms (like PaaS providers) or software and processing infrastructure (like SaaS providers). Finally, IPaaS providers may also develop value-added spatial data products through a PaaS platform or with custom software infrastructure to leverage CSP services but work directly with end users to provide data and information products such as maps, statistical summaries, and reports for specific decision support applications (unlike DaaS providers). Figure 1 shows the relationships between actors that have different roles in the LDE.
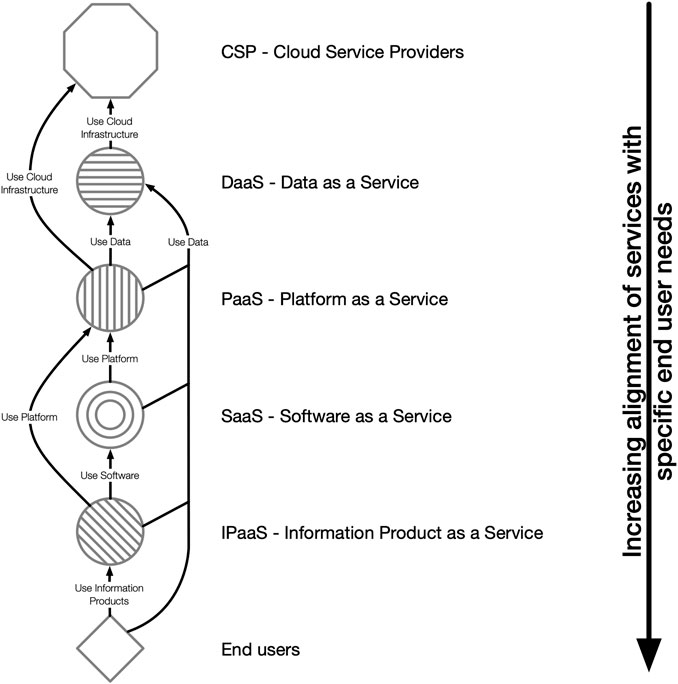
FIGURE 1. Illustration of the relationships between actors that have different roles in the Landsat Data Ecosystem (LDE). Arrows point from users to providers of products and services. The actor roles are arranged with the upstream actors at the top and downstream actors and end users at the bottom. There is increasing alignment with specific end user needs going down the value chain, as indicated by the arrow on the right.
Each of these terms has also been defined within the industry and academia except for IPaaS. Other data ecosystem actor roles that we chose not to include in our analysis are IaaS and InaaS, respectively Infrastructure as a Service and Information as a Service (Manvi and Shyam, 2014; Esch et al., 2016; Gartner, 2021). IaaS is a broader term that includes CSP and covers the technical capacity underlying DaaS, PaaS, and SaaS. While we acknowledge the foundational importance of IaaS, in this paper we focus on specific actor roles enabled by IaaS rather than IaaS itself. We interpreted InaaS as overlapping with both DaaS and IPaaS, so to clarify and distinguish our meaning when referring to these services we use only DaaS and IPaaS. We created the term IPaaS to highlight the concrete products such as maps and reports that add value to Landsat data by interpreting it for end users.
Movement of Landsat Data in the Data Ecosystem
In this section we trace the flow of Landsat data as it is transformed into value-added products and delivered to downstream actors. We present the movement of these data in terms of how the actors access and/or download the data, the value-added processing or analysis that they perform on the data, and how they deliver or make their value-added products available to downstream actors. Table 2 summarizes the data inputs, data actions, and data outputs that characterize actor interactions with Landsat data and derived products as they move downstream toward end users. Figure 2 maps the relationships between actors we interviewed and other actors they interact with while showing their role(s) within the ecosystem and distance from EROS along their respective value chains. Figure 3 illustrates the distribution of actor roles by distance from EROS, highlighting that the different roles tend to preferentially occupy up-, mid-, or downstream positions in their value chains.
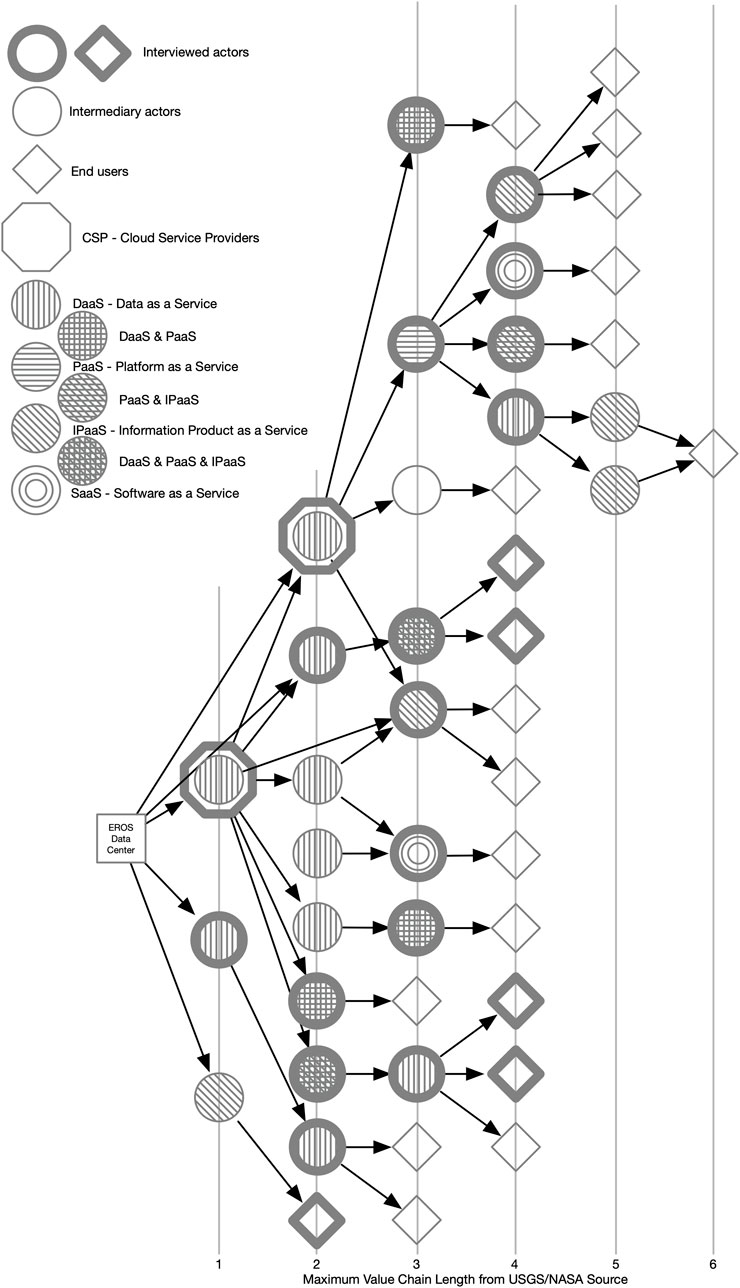
FIGURE 2. Illustration of value chain connections and actor types for the interviewed Landsat ecosystem actors. Value chain length increases from left to right and represents the maximum value chain length to each actor when there exist multiple upstream connections. The symbology for each actor represents the one or more roles that the actor plays within the examined value chains. EROS, U.S. Geological Survey Earth Resources Observation and Science Center; USGS, U.S. Geological Survey; NASA, National Aeronautics and Space Administration.
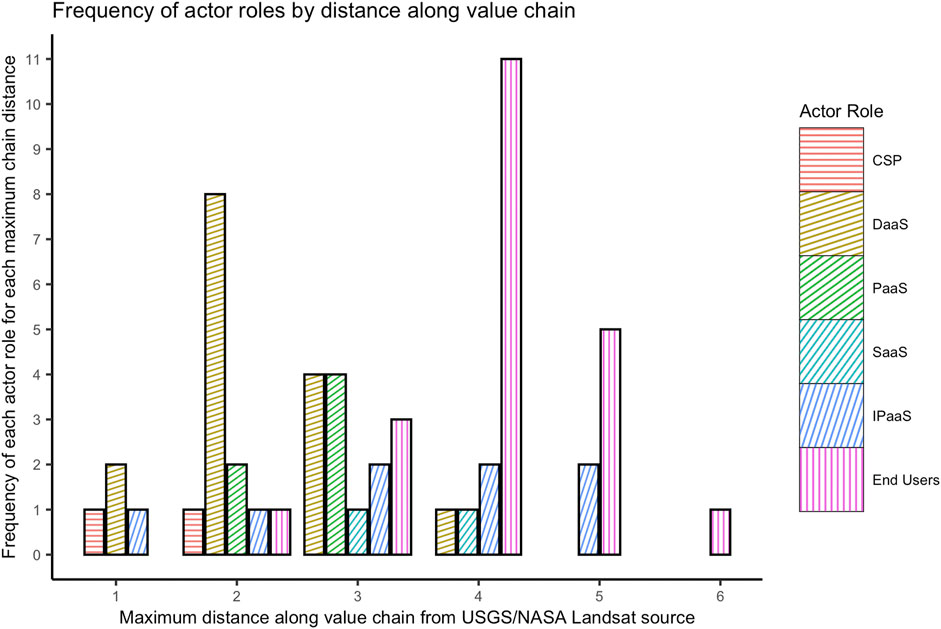
FIGURE 3. Illustration of the distribution of actor roles by distance from the EROS Landsat data source. Actors that play multiple roles in the ecosystem are counted once for each role that they play. USGS, U.S. Geological Survey; NASA, National Aeronautics and Space Administration; CSP, Cloud Service Providers; DaaS, Data as a Servicer providers; PaaS, Platform as a Service providers; SaaS, Software as a Service providers; IPaaS, Information Product as a Service providers.
Cloud Service Providers
CSPs provide the storage capacity and computational resources needed by actors in other roles to ingest, store, and process data. Other actors in the LDE leverage CSP capabilities to build and/or deliver products and services to their downstream users without having to maintain this capacity for themselves. The distinction between CSP, DaaS, and PaaS is that CSPs provide cloud infrastructure that is used in EO analytic processes among many other uses, while DaaS and PaaS providers frequently store their data and develop their platforms within the cloud infrastructure built by CSPs and have a specific focus on EO data, analytics, products, and services. Although this is the general case, there is some blurring of the roles in reality, as CSPs also do some work to ensure security, reliability, timeliness, and wide availability of data stored in the cloud. They also “identify the intended use” of data and communicate with downstream actors to help them access the right data, according to an interview. CSPs store data from multiple sensors besides Landsat and include metadata, which enables integration of data from multiple sources. Notably, there is nothing that needs to be done to maintain the cloud itself, so in CSPs’ day-to-day work they support DaaS activities at a foundational level.
Data as a Service Providers
DaaS actors in our study similarly downloaded or accessed Landsat data through automated pipelines to a CSP or EROS or processed the data using PaaS provider’s platforms. In each of these cases, DaaS providers worked closely with PaaS providers, CSPs, or EROS throughout the production of their data products and reported that such relationships enabled quicker processing and troubleshooting when challenges arose. Some actors performed roles as both CSPs and DaaS providers. One actor described their organization’s long relationship with an upstream data provider and their mutual exchange of information about processing methods:
“What we’ve found since we started this work back in the early 2000s, mapping methodologies improved. The imagery itself is improved, and so what both we and [the upstream provider] have found is that there were things that needed to be fixed once we saw some of these issues cropping up.”
As DaaS actors process Landsat data to generate mainly national- or global-scale products, exchange of such information saves time and increases accuracy of results. Oliveira et al. (2019) observe that a data ecosystem is characterized by collaboration and feedback in multiple directions–upstream to data providers, downstream to data users, and horizontally between users at the same level.
DaaS providers produced Landsat-based spatial data products representing land cover change or classification, often utilizing the entire Landsat archive. Most employed processing to clean or calibrate data prior to analysis, particularly for products that incorporated data from multiple Landsat missions or in regions where cloud cover is common. Providers also incorporated other EO data sources into their products or explored ways to incorporate them but expressed challenges in ensuring comparability of their data products when incorporating newer sources of data. For more literature about integrating data from Landsat and other sensors, see Gao et al. (2006); Lehmann et al. (2014); Walker et al. (2014); Gomo et al. (2018); Zhu et al. (2019).
In general, the DaaS providers emphasized the importance of ensuring the accuracy of their data products and following scientific best practices throughout their analyses. One actor attributed the accuracy of their product to their team’s collaborative approach to methodology, workflow, and documentation development, while others emphasized validation against ground truth points or high-resolution imagery. DaaS providers were more removed from their downstream users than other actor types, with less reported interaction with and understanding of their downstream users and the applications of their datasets.
“Like I said, I probably feel like there’s at least two users, if not more, of the data out there for every one that we might be aware of. Probably a lot more.”
Each DaaS provider interviewed reported large numbers of users, measured mostly by data download counts from their web platforms, but interacted with downstream actors only when contacted by them directly. DaaS providers seemed to view their role in the LDE as generating stable, objective, or science-quality data products agnostic of downstream use in value-added analyses or by end users in a decision-making capacity. The specific purposes for which these products are used did not seem to influence the products they developed or methodologies they employed to produce them.
Platform as a Service Providers
PaaS actors reported accessing Landsat data through direct ingest pipelines to EROS or a DaaS provider that hosted the data. Most reported automating the ingest process with scripts that compare the platform’s and the provider’s data holdings, index newly available scenes, and process the data to make it useable on their platform. This requires the PaaS provider to download large volumes of data to local or cloud-based storage, but from the perspective of platform users the data are locally stored and readily available. “We bring the data into ‘hot storage’ where, along with the other data sets that we’re pulling in, we can basically bring up the catalogue products in our platform that reference the Landsat data.” The PaaS services are built on CSP infrastructure and actors frequently reported leveraging that provider’s holdings of the Landsat archive where possible because of the efficiency and performance of collocated data ingest and analysis within the shared data and computational CSP environment. However, several PaaS actors also reported ingesting Landsat and EO products from multiple providers due to bandwidth constraints or the availability of particular data products that outweighed the costs associated with establishing and maintaining multiple ingest procedures.
All interviewed PaaS providers emphasized that the role of their platform is to provide easy access to data, analytics, and computing resources. One PaaS provider described their platform as allowing users to “bring multiple remote sensing libraries together into a central platform and provide the ability to process that data without having to download and manually do that on your own infrastructure.” PaaS providers detailed the technical requirements for building and maintaining such a central platform, including multiple pre-processing and indexing tasks that allow data to be quickly incorporated into geospatial workflows. These tasks include calibrating, correcting, and georeferencing imagery from different sources or building cloud-free mosaic base maps. Several expressed their organization’s efforts to automate these processes to simplify the user’s experience: “We apply everything to each scene as it comes in ... so that you can literally just treat it like a Google map in some ways,” and “We do it once so that not everybody has to do it over and over.”
Most PaaS providers reported developing machine learning models, analytical products, and other applications for use on top of their data library, and this is also shown in the literature (Tamiminia et al., 2020; Wu et al., 2020). Algorithms mentioned by PaaS providers include those for water extent mapping, land cover classification, and change detection. These algorithms are made available for users to build analytical workflows on the platform or generate information products like change alerts or time series data cubes. One provider characterized their platform as a “marketplace” in which users may purchase machine learning models from different providers to run on the platform’s library of EO data or upload their own models to leverage the platform’s computing power. While providers recognized the value they provided by indexing and processing Landsat data for analysis (the “grungy IT part of doing science with this data,” as one provider called it), they characterized their analytical tools as the most exciting feature of their platform for users: “People are very excited ... they don’t have to try to compute that stuff. They can, hopefully, just tap into a reliable, trustworthy, already computed version.” Developing these analytics was also more appealing to PaaS providers who, at least in one case, would dedicate less time to pre-processing if they could.
The primary role PaaS providers in our study play in the LDE is providing customers the capability to conduct their own Big EO data analyses without managing file structures, large-scale data storage, variable data characteristics, and other challenges that complicate interoperability. Once these barriers are removed, however, use of most platforms’ full capability still requires some level of geospatial programming. PaaS providers acknowledged their users’ varying expertise in this regard and offer several ways to access analytical services including platform functionality through an interactive web interface that does not require coding ability and support services like workshops or training on advanced API use. Some offer their analytical expertise as a service and use their API to generate datasets or reports requested by customers.
Software as a Service Providers
SaaS providers reported accessing data, both Landsat and other spatial datasets, from several providers and through a variety of means. One SaaS provider focused primarily on back-end development and reported using DaaS-hosted data almost exclusively except where clients had existing relationships with other CSPs or DaaS providers. They accessed the DaaS-hosted data using the SpatioTemporal Asset Catalogs (STAC) indexing standard for its functionality across a variety of data providers and its ease of use in filtering archived imagery by spectral range and cloud cover without needing to learn imagery-specific metadata formats (SpatioTemporal Asset Catalogs, 2021).
One SaaS provider primarily developed decision support applications for clients to address specific, localized, environmental issues. Most of their recent applications have been developed using publicly accessible PaaS providers where they access and process Landsat data. The end users of these applications are often unfamiliar with EO data. This SaaS provider described efforts to build products on a PaaS provider’s infrastructure that make Landsat-derived information both useful and accessible:
“It bridges the gap for those local decision makers, policymakers … It makes this tool available to them to monitor water quality over a wide temporal and physical scale. It’s pretty impressive. [PaaS provider] not only made it possible for [our] team to create this tool in just a short time span … but it also makes this tool available for the decision makers, the policymakers, the partners and just make it very accessible.”
While SaaS providers varied in the products and services they developed for downstream actors, they worked directly with clients to understand the challenges they faced and design custom software solutions to address them. While much of the work these actors described echoes the data management, calibration, and software architecture labor reported by PaaS providers for developing and maintaining EO analytics platforms, SaaS providers differentiate themselves from PaaS providers by delivering solutions built from the tools developed by PaaS. One SaaS provider summarized their work as a combination of software development–building geospatial data pipelines in particular–and consulting:
“We get data from satellites, drones, wherever, then put it in a different format whether it’s a graph, an Excel spreadsheet, even a map. We facilitate that for companies or nonprofits and help guide their process about how to exploit and best use geospatial data ... Customers will come to us with problems, and we will create solutions which they build themselves or we have a team that can build them and ship them out to customers.”
The role of SaaS providers in the LDE was to fulfill the needs of downstream actors by developing software for processing or cleaning EO data for specific applications, and incorporating Landsat to calibrate, validate, or co-register other data sources. They also used Landsat more broadly as a “go-to” EO data source for Big Data analyses, particularly those focused on long term change detection. They expressed the importance of Landsat as a teaching tool for software developers new to spatial data processing as most were familiar with the Landsat program, and the archive’s large volume of freely available data lowered the barrier to processing EO data at scale.
Information Product as a Service Providers
IPaaS providers generally reported ingesting Landsat and Landsat-derived products from DaaS providers or accessing and processing it through a PaaS provider. Those that accessed Landsat through a PaaS provider also utilized DaaS-hosted data or downloaded Landsat directly from USGS Earth Explorer for applications that required more timely data access or greater control over processing than was possible through their PaaS provider. One IPaaS provider described their organization’s connection with an upstream data provider who uploads value-added products directly to the IPaaS provider’s cloud storage as they are produced. Most IPaaS providers also reported using other sources of EO data–particularly where they are readily accessible through their PaaS provider–including Sentinel-1 and 2, MODIS, and a variety of high-resolution multispectral data.
The value-added processing reported by IPaaS providers was similar to that described by DaaS providers. Both employed methods like machine learning, spectral indexing, and computational or spatial modeling to develop land cover and change products, and both providers’ products covered large spatial scales. Many IPaaS providers also discussed the accuracy assessment and validation procedures that went into their products before publication. IPaaS and DaaS providers differed in that the former developed value-added products such as reports, statistical summaries, and maps for specific user groups and/or application areas while the latter created user-agnostic data products and had little understanding of their end users. IPaaS providers reported regularly interacting with their users, building specific products to meet users’ needs, and incorporating feedback into future product iterations or producing localized versions of a product to fulfill specific user requirements:
“We have one person who [their] job only is just following up, finding out what do users want, what they didn’t like to use, and so constantly playing it back to us and say, ‘Oh, but users want something else.’”
“We were just aiming to create specific products for use by specific groups in specific situations. Basically a consultant working for free, that’s essentially what it came down to.”
In much the same way as SaaS providers, IPaaS providers often played a consultant role where EO data could aid in end users’ decision-making. Many of the IPaaS providers described their products relative to existing sources of information used by decision makers, indicating their focus on developing products for use in specific applications. For example, one IPaaS provider cited local government use of in situ sensors for monitoring hazardous storm events and the sensors’ unreliability during flooding or political conflict. Use of Landsat and other EO data allowed decision makers to receive the same insights more quickly, safely, and reliably. Another IPaaS provider emphasized the importance of field survey data collected by their end users as an input to their vegetation models, while one such end user reported that the IPaaS provider’s value-added products improved on their monitoring operations by providing added context and filling gaps in survey data resulting from difficult terrain or distant field sites.
The role of IPaaS providers in the LDE was to transform data products into value-added information products that end users with limited technical knowledge could use for their decision-making processes. The ways in which IPaaS providers delivered their value-added products downstream varied, with some working closely with end users to provide training and interpretation of information products and others simply uploading data files to their organization website. In all cases, though, IPaaS providers described close relationships with end users who benefited from their value-added products and providers’ efforts to deliver products in accessible and useful formats for their end users’ applications. For one provider, this meant minimal processing and analysis was done to their input data but several weeks were spent developing data visualizations and supporting interpretive material. Others reported developing interactive web maps and customizable statistical reports tailored to different environments and localities, see also (Lehmann et al., 2014; Wehn and Evers, 2015). In some cases, IPaaS providers reported printing out paper maps and bringing them to end users who requested them.
End Users
End users interviewed largely accessed their data or information products through IPaaS providers’ web platforms or direct contact with an IPaaS organization. In one case, an end user accessed their value-added product as an image service through ArcGIS Hub where they produced a map of their area of interest. Another end user of the same product reported viewing the data through the platform before requesting the raw data files from the DaaS provider to use them in research. Others similarly accessed information products like maps and graphs and downloaded the spatial data files themselves through an IPaaS provider’s online dashboard. One actor lacked reliable internet access to download entire raster data files. They reported using the dashboard’s basic analytical capabilities to generate maps for use in their conservation advocacy work. Other end users received printed or digital maps and statistical reports directly from the IPaaS provider. Notably, the distinction between upstream producers and downstream users was blurred in some cases where end users provided in situ or field survey data for use in an IPaaS provider’s spatial data products.
While end users as LDE actors do not produce value-added spatial data or information products, several reported how they use remote sensing products and the role they hoped they would play in society. One discussed their collaboration with a disease-vector control board to understand the relationship between heat and vector-borne disease risk and hoped their findings would shape the board’s ongoing management policies. Others use the information they access on IPaaS platforms to lobby decision makers for specific policies or incorporate the information directly into strategic resource management plans. One regional public lands manager reported their office provides relevant information to their field personnel in “exactly [the format] our end users are familiar with.”
Benefits, Challenges, and Suggested Improvements of Landsat
We asked about the benefits of Landsat data and the Landsat-derived products relevant to data ecosystem actors’ operations. Some benefits of Landsat included sensor characteristics such as spatial resolution, temporal resolution, and spectral band coverage. Actors tended to describe the sensor characteristics as suiting their needs for their organizations’ operations. They praised Landsat’s data calibration, quality, consistency over time, free public access, historical archive, familiarity, spatial resolution, spatial coverage, interoperability with other data sources, and the efficiency it provides for field work. “Just being able to access the same data, whether it’s from 1985 to 2019, in the same way with the same fields, and processed the same way, that’s huge.” Combining Landsat data with other remote sensing (e.g., Sentinel-2) and in situ field data afforded actors higher temporal resolution and novel analytical approaches relative to their use of Landsat data alone, see also (Wulder et al., 2008; Petrou et al., 2015; Lawford, 2019). Consistent with the literature, free public access and the historical archive were the most often mentioned benefits of the Landsat archive by actors in this study (Wulder et al., 2008; Banskota et al., 2014; Petrou et al., 2015; Zhu et al., 2019). Several actors reported that much of their organization’s operations depended upon Landsat data (“We definitely couldn’t have done this project if it weren’t for Landsat.”) or that some of their most important applications would not be possible without free access to it, consistent with Loomis et al. (2015).
Although actors were largely satisfied with Landsat, they provided suggestions for improvements when prompted. Some requests were to maintain the beneficial aspects of Landsat, including consistency in the data from one mission to the next for ease of continuing long term analyses, and “keeping it free, keeping it available, keeping it updated.” Upstream actors said more about challenges with the data than downstream actors, most of whom trusted the upstream actors to deliver quality products. A few challenges for upstream actors included cloud cover, changes to data products, and balancing improving data accuracy with maintaining consistency. Most actors reported they would like to see improvements in Landsat’s spatial resolution, and many recognized that desiring higher spatial resolution was a common request within the data ecosystem, even when it was not considered essential. Several acknowledged the challenges in processing more pixels that come with higher spatial resolution data but did not anticipate this posing a significant hurdle to their organization, in part because of access to cloud computing resources for storing and analyzing EO data within the data ecosystem. Wulder et al. (2008) reiterate the higher processing needs of high resolution imagery (less than 10 m) and add that very high resolution imagery is impractical for land cover change monitoring because it creates the need to reaggregate individual landscape elements into land cover types. As one our interviewees remarked, “We call that kind of thinking [asking for ever-higher resolution] ‘precisionism.’ ... For a lot of the large management decisions we’re making on rangelands, you don’t need to know all that. In fact, it’s just noise.” Additional actor-suggested Landsat improvements included increased temporal resolution, additional spectral bands, easier access, more Analysis Ready Data, and better cloud-masking algorithms.
Translating Knowledge Into Societal Value
While upstream actors play a critical role in the LDE by processing and analyzing Landsat data to produce novel data products, generation of societal value from Landsat data that goes beyond purely scientific understanding requires effective translation of this understanding into policy or management outcomes in particular social-ecological contexts. Such translation requires not only quality data-driven insights but information drawn from these insights that is useful, useable, and used by end users–this often requires that upstream actors engage with downstream actors to understand their needs (Bamzai-Dodson et al., 2021). Keil et al. (1995) define “perceived usefulness” and “ease of use” with respect to user acceptance and use of decision-support systems. Perceived usefulness, the degree to which a person believes that using a particular system would enhance their job performance, and ease of use, the degree to which a person believes that using a particular system would require minimal effort, guide our examination of the factors shaping decision-making by end users within the LDE. We also briefly discuss a variety of social and political factors not directly related to technical design characteristics, or the validity of scientific knowledge used therein, that variably shaped use outcomes sought by end users. These factors relate to science-policy debates more broadly (Jasanoff, 2004; Wesselink et al., 2013) and, while adequate attention to the topic is beyond the scope of this paper, illustrate an urgent area for future research aimed at understanding how to better translate EO-derived knowledge into beneficial outcomes for society and the environment.
Computational, Analytical, and Technological Bridges and Barriers of Cloud Infrastructure
Cloud infrastructure enables data processing at speeds and scales previously impossible, as documented in the literature (Zhu et al., 2019; Wu et al., 2020). Although actors still expressed issues with time delays between image capture and when cloud providers make the imagery available, decision makers see more timely delivery of data and information products as a result of the cloud infrastructure. “Instead of taking 3 weeks or a month to produce the [dataset] for the 100 biggest cities, we’re now able to produce the [dataset] for every city in the U.S., big and small, 14,000 of ’em in about 4 h.” Processing technologies and standards like Cloud-Optimized GeoTIFFs, Analysis Ready Data, and STAC make cloud computing easier, reduce processing time, increase efficiency, and minimize error, see also (Dwyer et al., 2018; Fergason et al., 2021). However, upstream actors observed that when downstream actors unfamiliar with EO data and data science methodology have access to powerful cloud computing resources and large datasets, they sometimes fail to conduct accuracy assessments or misunderstand the substantial effort required to produce a valid product, leading to creation of inaccurate data and/or information products, “we complain a little bit to some extent that it’s become very easy to produce a dataset of questionable quality. A lotta folks don’t really understand the ins and the outs, the errors, but they can click those buttons, and get an output.” Many upstream product and service providers in our study expressed recognition of the representational nuances in EO data and the tradeoffs between global and local accuracy. Downstream actors largely did not express concern over accuracy of data products, with some expressing their trust in the data provider, emphasizing greater importance on the timeliness of data access, or that “precisionism” is unnecessary in the context of large land management decisions, as quoted above.
Free and Open Data Policy, Landsat’s Versatility, and the Landsat Knowledge Base
As mentioned above, Landsat’s historical archive and free and open data policy were commonly reported benefits for actors throughout our study’s snapshot of the LDE. Landsat’s historical archive offers the oldest and longest baseline of any land-observing multi-spectral data archive, providing actors greater flexibility for assessing landscape changes throughout history, seen in our interviews (“It’s the ability to peer back into the past to understand what management practices were. We can measure progress and adoption against what things were like 5, 10, 15 years ago”) and in the literature (Wulder et al., 2008; Roy et al., 2014; Fu et al., 2015; Zhu et al., 2019). Actors reported that the free and open policy made data more accessible, particularly benefiting those without the ability to pay (“A lot of these areas we work in don’t have the budget to purchase daily imagery from a high-resolution commercial provider ... Having a suite of publicly accessible data has been huge”), also see Loomis et al. (2015).
Many actors hinted at a more subtle outcome of Landsat’s history. The processing methodologies and research practices built around Landsat–and subsequently shared within and among research universities–have shaped the community’s approach to EO data-driven science over time (“It’s kind of the benchmark in remote sensing, right?”; “It’s a known quantity by this point, so it’s not rocket science to work with”; “The idea that Landsat is this global piece of public infrastructure is great. It’s like the GPS system”). Many of the indices and algorithms commonly used for processing and classifying EO data, for example, were refined on Landsat imagery, as further described in (Hardy and Anderson, 1973; Rogers et al., 1975). Actors also recognized the importance of the Landsat community’s knowledge base for learning new analytical approaches (“There is a lot of white papers being published, a lot of data sets”; “There are a ton of tutorials and blog posts about how to set it up”). Further, Landsat was a pioneer in providing policy makers and land managers a novel perspective of the planet for managing resources and monitoring change, see also (Anderson et al., 1975; Baker, 1975; Metz and Wiepking, 1980). These characteristics have arguably cemented Landsat’s legacy at least as much as the tangible scientific outcomes its data have enabled–even as data from high-resolution, hyperspectral, and near-real time platforms increasingly disrupt “conventional” remote sensing science. Indeed, Landsat’s moderate spatial resolution and large spectral range made it a widely applicable sensor and contributed to its success as a science mission from its beginning. However, several important factors mediated the translation of scientific knowledge into informed use in decision-making.
Communication and the Role of User-Facing Data Intermediaries
IPaaS and SaaS providers in our study experienced higher frequency and depth of interaction with downstream actors using their outputs compared to most DaaS and PaaS providers. Communication between different actor levels in the ecosystem, such as between IPaaS or SaaS and end users, increases benefits to society through adjustments to products and delivery to better align with end user needs: actors that had a clear understanding of their users’ needs addressed specific problems and provided products that were more relevant and useful to those actors. IPaaS and SaaS providers also discussed making adjustments or improvements to their products following downstream feedback. In most cases these adjustments were not to the underlying data itself but to the ways it was delivered or made available to downstream actors. One actor made their data available for download through an interactive online dashboard, but also employed a team to connect with data users, understand their information needs, and to deliver relevant products to them directly. This outreach allowed the provider to understand the diversity of end users’ needs more fully: some expressed the need for more timely data, while others required a certain threshold of accuracy even if it meant the data were delayed. Even within a single application type such as flooding there is the need for both immediate (e.g., downstream warning response) and less immediate (e.g., evaluation of insurance risk/claims) information (Blyth, 1997). Guided by nuanced understanding of downstream actors, providers were better able to weigh the costs and benefits of otherwise unquestioned components of their operation like the format of data they made available, or how frequently they improved the algorithms underlying their products.
Most upstream DaaS providers reported some interaction with downstream actors or expressed interest in furthering their understanding of other actors, but did not maintain downstream actor outreach, training, or technical transfer as a formal component of their operations in comparison with IPaaS and SaaS providers. As such, the interactions they did describe with downstream actors were largely transactional in nature (e.g., troubleshooting data access) or responsive to either actor projects or a broader pattern of actor feedback (“A lot of the products that we produce are used in response to a project”; “That was an online application we built because we got so many requests from people to give them a summary of the change that could be derived from our data”). The outcomes of these interactions benefited actors who contacted the DaaS provider or otherwise provided feedback on their data products but were largely unidirectional and did not shape substantial changes to particular data products or stir development of new products aimed at particular end users or applications. This was due in part to the larger number of downstream actors using DaaS data products and the accompanying difficulty in fully understanding all of their use cases, particularly where data and services are free to access. These providers acknowledged there are many downstream users of their data, and in some cases maintained public collections of use cases, but they commonly lacked understanding about the diversity of applications in which their data are employed:
“Collecting downstream data on Landsat users is really hard because probably 99 percent of the time, you only hear about it when people have a problem. It’s like people leaving restaurant reviews. They only leave bad ones.”
Within the LDE, work sector may play a significant role in the degree to which data providers interact with actors to improve the utility of their data or services (Gasco-Hernandez et al., 2017). However, examples of greater and lesser downstream interaction were found across actor work sectors in our study. Public sector DaaS and IPaaS providers seemed to understand downstream actors to a lesser degree than did non-profit DaaS or IPaaS providers, while private sector PaaS providers reported varying degrees of understanding of their downstream users and applications. Both the private sector and the public sector SaaS providers we interviewed worked directly with clients to deliver services; they understood their downstream users quite well and referred to their products and services as custom-made “solutions” to their clients’ problems. PaaS providers who also offered consulting and analytics as a service worked directly with customers to understand requirements and provided the most relevant solution they had available but reported less interaction with or knowledge about their platform users due in part to the platform’s self-service model:
“A lot of the Landsat usage tends to be self-service, right? Many times, we’re not engaged in the actual commercial or customer conversations with those [users] because [the data] are free and publicly available to access through our platform, so there’s not necessarily a lot of conversations that are happening between our sales team or business development team and some of those users.”
The exception was where the provider’s organization included an outreach or applications team as part of its business model. These organizations built user outreach into their operations and sought feedback about user needs and difficulties:
“The fun part about my job is I get to learn all of this stuff that all these people are trying to do. It’s twice as hard because not only do I have to learn what they’re trying to do, I have to learn what they’re doing wrong so that I can go fix what they’re trying to do.”
The downstream actors we interviewed for this study were all users of PaaS providers with outreach or applications-research teams; other PaaS providers either did not respond to requests for connection with platform users or could not do so due to non-disclosure agreements. All platform users we did interview were from the non-profit or academic sector, or provided data or information products to these sectors, reflecting different priorities between private and non-profit or academic sectors in the application of EO data for addressing particular problems.
Trust in and Familiarity of Landsat
Actor trust in Landsat products as accurate or otherwise useful representations of the environment also shaped likelihood of product use by end users we interviewed. We differentiate trust as a sentiment toward actors in the LDE and their products from authoritative sources and authoritative data which are official designations. Reported trust in data products was identified in explicit statements about actor experiences when ground-truthing data against sources already trusted by them, and implicitly in descriptions of actor interactions with upstream providers or downstream users of data or services. There was a notable asymmetry between upstream and downstream actors in their trust toward data products and proper use in downstream analysis and decision-making. As noted, downstream actors generally trusted the validity of data products and services from upstream providers because they trusted upstream actor technical expertise. This type of trust may stem from positive past experiences with the same upstream provider or the provider’s positive reputation, as suggested by social psychology and marketing literature (Johnson and Grayson, 2005). Trust in service providers is mediated by the actor’s level of industry knowledge, length of relationship with the service provider, and perception of the service provider’s expertise, performance, and warmth (Coulter and Coulter, 2002, 2003; Johnson and Grayson, 2005). Another explanation is that there is an expectation, formal or informal, within the industry that any service provider reaches a threshold of excellence, or that there is a culture of trust (Sztompka, 1998). From our literature search, it seemed that there was a surge of interest and literature at the turn of the millennium and not many updates relevant to our study afterward. One exception to downstream actors’ trust in upstream products was when a DaaS provider’s algorithm change introduced uncertainty into a downstream IPaaS provider’s land change model, making it difficult to accurately attribute changes to landscape transformation or model error. In contrast, upstream actors we interviewed worried about inappropriate use of their products. One end user echoes these concerns, noting that the ease of using machine learning algorithms can be misleading at times and lead to misuse which creates problems downstream. Maps can greatly influence decision makers who perceive them as objective and trustworthy without recognizing potential inaccuracies or considering the ways they may distort reality (Monmonier, 1991). These actor differences may stem from differing goals around the use of Landsat data and lack of intercommunication.
Trust in the LDE is also connected to transparency. This study revealed that lack of transparency can lead to frustration from downstream actors who would like to know the process of how products are generated. On the other hand, transparency of data and metadata engenders a higher level of confidence in the final products.
“Having that metadata is really important, because it gives accountability in terms of the data set that’s being used. If somebody wanted to track down what that data set was–what are the error statistics? how was it produced? who produced it? how can I contact the person who produced it?–then it’s fully documented.”
Sztompka (1998) also reports a link between trust and transparency in national cultures. Broadly in the United States, distrust in public institutions is increasing (Gauchat, 2012; McGrath, 2017). Confidence in science has declined among conservatives (especially highly educated conservatives) in the United States since the 1970s, while moderates have had consistently low confidence in science (Gauchat, 2012). Although we did not see evidence of these trends extending to distrust of Landsat within the group of actors we interviewed, future studies could examine whether trust in Landsat data is declining among the general population and whether transparency mitigates the trend. Within the LDE, actors need differing levels of expertise, depending on what products they use in the ecosystem, and this may influence their trust throughout the ecosystem. Future studies on this along with other factors that influence decision-making, such as alignment with existing opinion, degree of scientific consensus, and political positions, may benefit the literature.
Similar to trust, many actors mentioned their comfort or familiarity with Landsat data and the ways these factors benefited their operations. The ubiquity of Landsat use in the Earth sciences, particularly in public research universities, lowered the barrier to entry for new developers working with Big EO data. Actors indicated that Landsat is the sensor that most remote sensing scientists used when they received training, so they preferred to work with Landsat over other sensors. This indicates that the LDE will continue to grow as long as Landsat is the primary dataset used to train remote sensing scientists and users. Metadata readability and data calibration also contributed to actors’ sense of comfort associated with the data. Even if the data quality from other data sources is comparable, Landsat is the one that sees the most use due to this familiarity and comfort. “Generally, just using Landsat, mainly because of comfort. It’s what I know, and it’s there, and it’s pretty good.” The comfort and familiarity with Landsat lead to more people using Landsat data, growing the LDE. These factors potentially increase the societal value produced within the data ecosystem if the expanded upstream use also leads to broader downstream and end user applications. We would argue that not only do the aspects of comfort and familiarity increase use of the data, they also increase trust and acceptance of the outputs such that their value in decision support rises. Trust, comfort, and familiarity with Landsat data upstream thus create a wealth of societal value through consequences downstream.
Application-Focused Products and Accessible Information
The main value of Landsat to society is enhanced decision-making for application areas such as those represented in our study: agriculture, deforestation, forest carbon and other ecosystem services, humanitarian and health, mining, oil and gas, water quality, wetlands, and wildfire. This exemplifies Landsat’s versatility in creating value for many different areas. The value of upstream activities is often a composite of the value their products generate for actors downstream. Some of the benefits for decision-making in these areas have already been mentioned in this paper, such as efficiency and spatial and temporal context compared to ground surveys and in situ sensors, interoperability, and trust. Landsat helps end users monitor and communicate what is happening on landscapes. It contributes to conservation and restoration, planning and policy, projections and modelling, assessment of decisions, and allocating funds. Here we elaborate upon products created with particular applications in mind.
“These tools, I think, generally are just-are best to use within some sort of decision-making framework. If you’re not gonna use them in a way that will affect how you’re doing things, then you’re just messing around with it. It’s a thought exercise. Ultimately, the reason that we create these tools and try to get them into people’s hands is to be able to help them to think through whatever decisions they’re having to make.”
A number of Landsat-derived products mentioned in the interviews were developed by other actors in the ecosystem in response to specific information needs identified by end users. These actors understood the uses and limitations of existing ground surveys or in situ sensors and saw an opportunity for satellite data to play the same role with greater accuracy, efficiency, and reliability, see also (Blyth, 1997). This was a bottom-up, user-centric approach in that products were developed to solve a problem (Virapongse et al., 2020), in contrast to more top-down approach where useful applications for a new product are sought after it has been released. The latter characterizes the approach described by some of the DaaS providers who emphasize the scientific applications of their data products and invest significant time into accuracy assessment and validation but express difficulty in understanding end user applications. There are many end uses in decision-making for these data products and their derivatives, but user-agnostic products seemed less likely to fulfill end user requirements than products created in response to a specific problem.
Understanding end users’ needs, how EO products can fulfill them, and how to provide these products in a way that is most relevant and useful to them was challenging for many actors. One SaaS provider cited the difficulties in bridging the gap between research and real-world application:
“I think that’s one barrier for entry that comes up ... we’re producing a lot of good data, but it’s just hard for the [users] to apply that to their work long term. A big aim of ours is to make those final products, the final data, as accessible and as useable as possible for them ... There’s quite a bit of research and reconnection down the line to make sure that if the project turned out to be useful, how accessible the data is, that kind of thing. Definitely always on our minds.”
The SaaS provider works closely with clients to create custom tools, but without extending the relationships beyond delivery the tools often do not get long term use.
The types of applications for which data products are used also shape the importance of understanding actor requirements, according to interviewees. We argue that applications where the relationship is clear between improved information and improved decision outcomes, and where successful outcomes are measurable, benefit from a more user-centric data product. For example, land managers we talked to who work closely with data and information products benefit greatly from information products that they work on themselves. The end users in this study used data and information products for a range of uses including landscape and resource management and planning, academic research, and policy or environmental advocacy. Resource managers and planners reported that EO information products provided greater spatial and historical context about the areas they managed and saved significant time in conducting field surveys to gather data, see also (Giuliani et al., 2017). Spatial products are a valuable tool for their applications, but do not replace existing methods and are used within existing end user decision-making frameworks along with other relevant information sources.
“You don’t just throw out your operation overnight because of our product, not that anyone would do that, but because our product says there’s this trend on your landscape, you wanna be thoughtful, as a user, about how you are using these maps, what they can tell you and what they can’t tell you.”
Advocacy-focused end users reported similar benefits of spatial data products for enhancing understanding of environmental issues like deforestation or mining, but were less enthusiastic about the process of translating these insights to achieve desired policy outcomes.
“I’m not very sure how the government is getting those data into use, but that’s the objective.” One actor who recognized the attention of policy makers was spread across many different issues nonetheless expressed frustration at their organization’s difficulty attracting attention to their own cause. “It’s hard to get, I guess, the government’s attention because of everything else that’s going on.” While generating novel and often highly accurate scientific insights about the environment is facilitated through cloud computing and Big Data technologies, providing this information to policy makers did not necessarily drive significant change or lead to desired outcomes for this actor and others.
In contrast to land and resource management applications (e.g., allocating funds to stop spread of invasive species), advocacy (e.g., lobbying city planners to block industry expansion) is focused on decisions tied to politics and subject to influence by competing interests. In these cases, the challenge was not a lack of information that is solved by relevant EO data products but that the desired outcome itself is not universally agreed upon by all interested parties:
“Our problems that we’re trying to solve on the ground for climate change mitigation, they’re all governance and politics. That’s what’s really weak ... We are giving a lot of good facts to really describe the severity of the problem ... if there’s no response, at least we’ll have a really precise record of the Earth’s demise.”
We argue that derivation of the benefits that Landsat-derived products can provide (e.g., improved spatial context, timelier insights, greater efficiency) is contingent upon outcomes that are clearly defined and agreed upon, and this is rarely the case for contentious human-environment issues.
This tension highlights the tradeoffs between user-agnostic and user-centric products. According to interviews, user-centric products will be more relevant for fewer (or only one) applications, while user-agnostic products may be less relevant but useable to a greater number of actors. For relatively simple value-added products like cloud-free mosaics or land cover classifications, downstream product developers may develop more user-centric data products without having to produce the initial user-agnostic value-added product. There is less funding in smaller organizations focused on specific sites or applications, yet these actors have a more nuanced and specific understanding of data requirements and how a particular product will be used to solve problems. The value that upstream providers create in developing generalized, value-added datasets across large areas or time frames is that downstream value-added developers who focus on specific applications may leverage these generalized products and refine them for their own niche use cases, like a cloud-free base map produced by a PaaS provider being used to study deforestation.
Conclusion
The Landsat Data Ecosystem (LDE) is complex and evolving, and the actors and relationships examined in this paper represent a fraction of those comprising the broader Landsat user community. There is great potential for further research on the movement of EO data through the data ecosystem. Qualitative research methods like those used in this study offer a powerful approach to understanding the benefits, challenges, and value actors experience using EO data. Such an approach can also illuminate the role of social factors like communication, trust, and applications of EO data science. In this paper, we highlighted LDE characteristics, both technical and social, that shape the movement of Landsat data downstream to add value for downstream actors. Overall, the observation from one of the study participants that “it’s not rocket science to work with” when referring to Landsat data best illustrates the accrued value along the value chains examined here. Though every Landsat value chain literally starts with “rocket science”, as intermediate actors process, reformat, analyze, and share Landsat derived products their complexity is reduced and value for use is increased. Actors interviewed in this study reported Landsat’s historical archive and availability as a free and open data source as beneficial or essential for their applications. Those who incorporated machine learning algorithms and cloud computing infrastructure, particularly where available on a user-friendly analytics platform, reported that these tools saved significant time and facilitated analyses at greater scale compared to previous approaches. Similarly, downstream users of data products created using cloud computing and machine learning/artificial intelligence generally reported the time- and cost-saving benefits of these products.
Through interviewing LDE actors to understand how societal value is generated, we identified several challenges. First, there is a need for more granular analysis of the differences between actor roles within each organization. Actor roles in this study often overlapped in terms of what providers delivered to downstream users which complicated efforts to assign distinct actor roles to each organization. The qualitative interview approach employed in mapping the LDE offers potential for uncovering nuances that are not readily visible from the outside. Second, even where products were well-fit to an actor’s capability and delivered information that reduced uncertainty in decision-making, there were often other factors that shaped the likelihood of a product’s use in practice. Institutional momentum of existing operations was, in some cases, a difficult hurdle to overcome in adopting Landsat-derived decision support tools. This was linked to end users’ familiarity and frequency of communication with product providers, such that end users who worked more closely with data providers reported more openness to adopting novel decision-making processes in their day-to-day operations than did end users with less frequent contact. This highlights the importance of studying the human dimensions of data-driven decision-making (Forsyth, 2002; Dryzek, 2013; Bennett et al., 2017). Finally, actual incorporation of decision support tools in practice does not guarantee achievement of desired outcomes for decision makers or translation of benefits to society more broadly as information availability is only one factor that ultimately impacts decision-making. More understanding is needed of the political context in which decision-making occurs and the extent of decision makers’ power to influence change in order to understand how EO-derived information can support these efforts.
As this paper focused on the generation of value using Landsat, it is also important for future efforts that translate EO data into societal value to consider the often-significant differences in how value is conceptualized between private, public, non-profit, or academic intermediaries, as well as social values held by members of the public not involved in decision-making. Value is generated when end users use data for decision-making (Virapongse et al., 2020), and value is thus connected to the ability to measure outcomes related to data use. Outcome success for private sector end users is quantifiable in economic terms, driving the commercialization of value-added products that offer competitive market advantage. Private sector intermediaries in this study were bound by non-disclosure agreements from sharing customer information, and value-added products were rarely available free of charge. Value of spatial information for resource management or environmental advocacy, on the other hand, is more difficult to measure (Ostendorf, 2011), and success is primarily framed in non-quantifiable societal benefits rather than monetary measures. As such, sharing of data products by public, non-profit, and academic actors in this study was not shaped by efforts to acquire a return on investment and were made publicly available for downstream use. The value of these data and that generated by their use, however, is not easily measured. Non-monetary valuation approaches and efforts to understand social values formation will be especially relevant to further research examining how EO data generates societal value (Ravenscroft, 2019; Stålhammar, 2021).
The value of EO data and their technical characteristics have been the focus of numerous studies using quantitative analytical methods (Bouma et al., 2011; Miller et al., 2013; Loomis et al., 2015; Straub et al., 2019). There is also a rich and growing body of literature on open data ecosystems in a variety of contexts (Lindman et al., 2005; Zuiderwijk et al., 2014; Styrin et al., 2017). In this study we used a qualitative approach to understand the societal value produced through the Landsat data ecosystem. We focused on the interactions between actors and the ways in which they transformed Landsat data and derived products to deliver value downstream. By examining value as perceived by end users, we identified a variety of non-technical dimensions of the data ecosystem that shaped product usefulness and useability in decision support applications. Actors positioned directly upstream of end users, particularly those familiar with EO data-driven decision-making, played a critical role in delivering products that fit end user information needs. User trust in data products and communication between actors also played an outstanding role shaping the value of derived products. As the volume and variety of EO data continue to grow, so does the complexity in measuring societal value generated by their use. Qualitative approaches like that employed herein offer a promising methodology for mapping this increasingly complex Big Data landscape and understanding, rather than simply quantifying, the value these data provide for society.
Data Availability Statement
The datasets presented in this article are not readily available because this is a Human Subjects study that doesn’t allow for a public dataset. Requests to access the datasets should be directed to cstraub@usgs.gov.
Ethics Statement
The studies involving human participants were reviewed and approved by Solutions IRB (https://www.solutionsirb.com/).
Author Contributions
Conceptualization, EM, CS, and KB; methodology, EM, CS, and SS; software, EM, CS and SS; validation, EM, CS and SS; formal analysis, EM and SS; investigation, EM, SS, and CS; resources, CS and KB; data curation, EM and SS; literature review, EM, SS, and AM; writing, reviewing, and editing, EM, SS, AM, KB, and CS; visualization, KB; supervision, CS; project administration, CS.
Conflict of Interest
The authors declare that the research was conducted in the absence of any commercial or financial relationships that could be construed as a potential conflict of interest.
Publisher’s Note
All claims expressed in this article are solely those of the authors and do not necessarily represent those of their affiliated organizations, or those of the publisher, the editors, and the reviewers. Any product that may be evaluated in this article, or claim that may be made by its manufacturer, is not guaranteed or endorsed by the publisher.
Acknowledgments
We gratefully acknowledge the helpful inputs from the editor and reviewers.
References
Anderson, A. T., Schultz, D. T., and Buchman, N. (1975). “Landsat Inventory of Surface-Mined Areas Using Extendible Digital Techniques,” in Lyndon B. Johnson Space Center NASA Earth Resources Survey Symp. Available: https://core.ac.uk/reader/42884860.
Baker, R. N. (1975). LANDSAT Data: a New Perspective for Geology. Photogrammetric Eng. Remote Sensing 41 (10), 1233–1239.
Bamzai-Dodson, A., Cravens, A. E., Wade, A., and McPherson, R. A. (2021). Engaging with Stakeholders to Produce Actionable Science: a Framework and Guidance. Weather, Clim. Soc. doi:10.1175/WCAS-D-21-0046.1
Banskota, A., Kayastha, N., Falkowski, M. J., Wulder, M. A., Froese, R. E., and White, J. C. (2014). Forest Monitoring Using Landsat Time Series Data: A Review. Can. J. Remote Sensing 40 (5), 362–384. doi:10.1080/07038992.2014.987376
Bennett, N. J., Roth, R., Klain, S. C., Chan, K., Christie, P., and Clark, D. A. (2017). Conservation Social Science: Understanding and Integrating Human Dimensions to Improve Conservation. Biol. Conservation 205, 93–108. doi:10.1016/j.biocon.2016.10.006
Blyth, K. (1997). Floodnet: a Telenetwork for Acquisition, Processing and Dissemination of Earth Observation Data for Monitoring and Emergency Management of Floods. Hydrological Process. 11 (10), 1359–1375. doi:10.1002/(SICI)1099-1085(199708)11:10<1359:AID-HYP529>3.0.CO;2-6
Bouma, J. A., Kuik, O., and Dekker, A. G. (2011). Assessing the Value of Earth Observation for Managing Coral Reefs: An Example from the Great Barrier Reef. Sci. total Environ. 409 (21), 4497–4503. doi:10.1016/j.scitotenv.2011.07.023
Brown, M. E., and Escobar, V. M. (2019). NASA's Early Adopter Program Links Satellite Data to Decision Making. Remote Sensing 11 (4), 11. doi:10.3390/rs11040406
Brown, T. C. (1984). The Concept of Value in Resource Allocation. Land Econ. 60 (3), 231–246. doi:10.2307/3146184
Campbell, J. (2015). Landsat Seen as Stunning Return on Public Investment. Available: https://landsat.gsfc.nasa.gov/article/landsat-seen-stunning-return-public-investment.
Coulter, K. S., and Coulter, R. A. (2002). Determinants of Trust in a Service Provider: the Moderating Role of Length of Relationship. J. Serv. Marketing 16 (1), 35–50. doi:10.1108/08876040210419406
Coulter, K. S., and Coulter, R. A. (2003). The Effects of Industry Knowledge on the Development of Trust in Service Relationships. Int. J. Res. Marketing 20 (1), 31–43. doi:10.1016/S0167-8116(02)00120-9
Dryzek, J. S. (2013). The Politics of the Earth: Environmental Discourses. Oxford, England: OUP Oxford.
Dwyer, J. L., Roy, D. P., Sauer, B., Jenkerson, C. B., Zhang, H. K., and Lymburner, L. (2018). Analysis Ready Data: Enabling Analysis of the Landsat Archive. Remote Sensing 10 (1363). doi:10.3390/rs10091363
Esch, T., Asamer, H., Boettcher, M., Brito, F., Hirner, A., Marconcini, M., et al. (2016). Earth Observation-Based Service Platforms - a New Instrument to Provide Geo-Information for Urban and Regional Planning. In 2016 IEEE International Geoscience and Remote Sensing Symposium. Beijing, China: IEEE. doi:10.1109/IGARSS.2016.7729455
Fergason, R. L., Hunter, M. A., Laura, J. R., and Hare, T. M. (2021). “Analysis Ready Data Available through the SpatioTemporal Asset Catalog (STAC) Specification: Investigating the Application to Planetary Data,” in 5th Planetary Data Workshop & Planetary Science Informatics & Analytics. Available at: https://www.hou.usra.edu/meetings/planetdata2021/pdf/7023.pdf.
Forsyth, T. (2002). Critical Political Ecology: The Politics of Environmental Science. London: Routledge.
Fritz, S., Scholes, R. J., Obersteiner, M., Bouma, J., and Reyers, B. (2008). A Conceptual Framework for Assessing the Benefits of a Global Earth Observation System of Systems. IEEE Syst. J. 2 (3), 338–348. doi:10.1109/jsyst.2008.926688
Fu, A., Li, J., and Pirasteh, S. (2015). “Long-Term Change Dynamics Using Landsat Archive for the Region of Waterloo in Ontario, Canada,” in Monitoring and Modeling of Global Changes: A Geomatics Perspective. Springer Remote Sensing/Photogrammetry. Editors J. Li, and X. Yang (Dordrecht: Springer), 63–86. doi:10.1007/978-94-017-9813-6_4
Gao, F., Masek, J., Schwaller, M., and Hall, F. (2006). On the Blending of the Landsat and MODIS Surface Reflectance: Predicting Daily Landsat Surface Reflectance. IEEE Trans. Geosci. Remote Sensing 44 (8), 2207–2218. doi:10.1109/TGRS.2006.872081
Gartner (2021). Gartner Glossary. [Online]. Available: https://www.gartner.com/en/information-technology/glossary (Accessed September 22, 2021).
Gasco-Hernandez, M., Sandoval-Almazan, R., and Gil-Garcia, J. R. (2017). “Open Innovation and Co-creation in the Public Sector: Understanding the Role of Intermediaries,” in International Conference on Electronic Participation (Cham: Springer International Publishing), 140–148. doi:10.1007/978-3-319-64322-9_12
Gauchat, G. (2012). Politicization of Science in the Public Sphere: A Study of Public Trust in the United States, 1974 to 2010. Am. Sociological Rev. 77 (2), 167–187. doi:10.1177/0003122412438225
Giuliani, G., Dao, H., De Bono, A., Chatenoux, B., Allenbach, K., De Laborie, P., et al. (2017). Live Monitoring of Earth Surface (LiMES): A Framework for Monitoring Environmental Changes from Earth Observations. Remote Sensing Environ. 202, 222–233. doi:10.1016/j.rse.2017.05.040
Gomo, F. F., Macleod, C., Rowan, J., Yeluripati, J., and Topp, K. (2018). Supporting Better Decisions across the Nexus of Water, Energy and Food through Earth Observation Data: Case of the Zambezi basin. Proc. IAHS 376, 15–23. doi:10.5194/piahs-376-15-2018
Hardy, E. E., and Anderson, J. R. (1973). “A Land Use Classification System for Use with Remote-Sensor Data,” in LARS Symposia (West Lafayette, Indiana: Purdue Research Foundation). Available: http://docs.lib.purdue.edu/lars_symp/2.
Jasanoff, S. (Editor) (2004). States of Knowledge: The Co-production of Science and the Social Order. London: Routledge Taylor & Francis Group.
Johnson, D., and Grayson, K. (2005). Cognitive and Affective Trust in Service Relationships. J. Business Res. 58 (4), 500–507. doi:10.1016/S0148-2963(03)00140-1
Keil, M., Beranek, P. M., and Konsynski, R. B. (1995). Usefulness and Ease of Use: Field Study Evidence Regarding Task Considerations. Decis. Support Syst. 13 (1), 75–91. doi:10.1016/0167-9236(94)E0032-M
Lawford, R. G. (2019). A Design for a Data and Information Service to Address the Knowledge Needs of the Water-Energy-Food (W-E-F) Nexus and Strategies to Facilitate its Implementation. Front. Environ. Sci. 7 (56), 1–11. doi:10.3389/fenvs.2019.00056
Lehmann, A., Giuliani, G., Ray, N., Rahman, K., Abbaspour, K. C., Nativi, S., et al. (2014). Reviewing Innovative Earth Observation Solutions for Filling Science-Policy Gaps in Hydrology. J. Hydrol. 518, 267–277. doi:10.1016/j.jhydrol.2014.05.059
Lehmann, A., Nativi, S., Mazzetti, P., Maso, J., Serral, I., Spengler, D., et al. (2020). GEOEssential - Mainstreaming Workflows from Data Sources to Environment Policy Indicators with Essential Variables. Int. J. Digital Earth 13 (2), 322–338. doi:10.1080/17538947.2019.1585977
Li, F., and Whalley, J. (2002). Deconstruction of the Telecommunications Industry: From Value Chains to Value Networks. Telecommunications Policy 26 (9), 451–472. doi:10.1016/S0308-5961(02)00056-3
Li, Z., Yang, C., Jin, B., Yu, M., Liu, K., Sun, M., et al. (2015). Enabling Big Geoscience Data Analytics with a Cloud-Based, MapReduce-Enabled and Service-Oriented Workflow Framework. Plos One 10 (3), e0116781. doi:10.1371/journal.pone.0116781
Li, Z., Tang, W., Huang, Q., Shook, E., and Guan, Q. (Eds) (2020). Big Data Computing for Geospatial Applications. MDPI AG. Available at: https://www.mdpi.com/books/pdfview/book/3121.
Lindman, J., Kinnari, T., and Rossi, M. (2005). Business Roles in the Emerging Open-Data Ecosystem. IEEE Softw. 33 (5), 54–59. doi:10.1109/MS.2015.25
Loomis, J., Koontz, S., Miller, H., and Richardson, L. (2015). Valuing Geospatial Information: Using the Contingent Valuation Method to Estimate the Economic Benefits of Landsat Satellite Imagery. Photogrammetric Eng. Remote Sensing 81 (8), 647–656. doi:10.14358/PERS.81.8.647
Manvi, S. S., and Shyam, G. K. (2014). Resource Management for Infrastructure as a Service (IaaS) in Cloud Computing: A Survey. J. Netw. Comp. Appl. 41, 424–440. doi:10.1016/j.jnca.2013.10.004
McGrath, M. (2017). Beyond Distrust: When the Public Loses Faith in American Institutions. Natl. Civic Rev. 106 (2), 46–51. doi:10.1002/ncr.21321
Metz, G. G., and Wiepking, P. J. (1980). EROS: A Space Program for Earth Resources. Washington, DC: U.S. Department of the Interior/Geological Survey.
Miller, H. M., Richardson, L., Koontz, S. R., Loomis, J., and Koontz, L. (2013). Users, Uses, and Value of Landsat Satellite Imagery—Results from the 2012 Survey of Users", Open-File Report 2013–1269. U.S. Geol. Surv. doi:10.3133/ofr/20131269
National Geospatial Advisory Committee Landsat Advisory Group (2019). Evaluation of a Range of Landsat Data Cost Sharing Models.
OGC Standards (2021). OGC Standards. [Online]. OGC. Available: https://www.ogc.org/docs/is. (Accessed October 21, 2021).
Oliveira, M. I. S., de Fátima Barros Lima, G., and Farias Lóscio, B. (2019). Investigations into Data Ecosystems: A Systematic Mapping Study. Knowledge Inf. Syst. 61 (2), 589–630. doi:10.1007/s10115-018-1323-6
Ostendorf, B. (2011). Overview: Spatial Information and Indicators for Sustainable Management of Natural Resources. Ecol. Indicators 11 (1), 97–102. doi:10.1016/j.ecolind.2010.10.003
Pearlman, F., Lawrence, C., Pindilli, E., Geppi, D., Shapiro, C., Grasso, M., et al. (2019). Demonstrating the Value of Earth Observations—Methods, Practical Applications, and Solutions—Group on Earth Observations Side Event Proceedings. U.S. Geol. Surv. Open-File Report 2019–1033. doi:10.3133/ofr20191033
Petrou, Z. I., Manakos, I., and Stathaki, T. (2015). Remote Sensing for Biodiversity Monitoring: a Review of Methods for Biodiversity Indicator Extraction and Assessment of Progress towards International Targets. Biodiversity and Conservation 24, 2333–2363. doi:10.1007/s10531-015-0947-z
Ravenscroft, N. (2019). A New Normative Economics for the Formation of Shared Social Values. Sustainability Sci. 14 (5), 1297–1307. doi:10.1007/s11625-018-0652-4
Resources for the Future (2021). Impact Assessments. [Online]. Available: https://www.rff.org/valuables/impact-assessments/(Accessed June 2021).
Rogers, R. H., Wilson, C. L., Reed, L. E., Shah, N. J., Akeley, R., Mara, T. G., et al. (1975). “Environmental Monitoring from Spacecraft Data,” in LARS Symposia (West Lafayette, Indiana: Purdue Research Foundation). Available: http://docs.lib.purdue.edu/lars_symp/2.
Roy, D. P., Wulder, M. A., Loveland, T. R., Woodcock, C. E., Allen, R. G., Anderson, M. C., et al. (2014). Landsat-8: Science and Product Vision for Terrestrial Global Change Research. Remote Sensing Environ. 145, 154–172. doi:10.1016/j.rse.2014.02.001
SpatioTemporal Asset Catalogs (2021). SpatioTemporal Asset Catalogs: Enabling Online Search and Discovery of Geospatial Assets. [Online]. Available: https://stacspec.org/(Accessed October 28, 2021).
Stålhammar, S. (2021). Assessing People’s Values of Nature: Where Is the Link to Sustainability Transformations? Front. Ecol. Evol. 9 (145), 1–7. doi:10.3389/fevo.2021.624084
Straub, C. L., Koontz, S. R., and Loomis, J. B. (2019). Economic Valuation of Landsat Imagery. Reston: U.S. Geological Survey. Open-File Report 2019-1112. doi:10.3133/ofr20191112
Styrin, E., Luna-Reyes, L. F., and Harrison, T. M. (2017). Open Data Ecosystems: an International Comparison. Transforming Government: People, Process and Policy 11 (1), 132–156. doi:10.1108/TG-01-2017-0006
Sztompka, P. (1998). Trust, Distrust and the Paradox of Democracy. Eur. J. Soc. Theor. 1 (1), 19–32. doi:10.1177/136843198001001003
Tamiminia, H., Salehi, B., Mahdianpari, M., Quackenbush, L., Adeli, S., and Brisco, B. (2020). Google Earth Engine for Geo-Big Data Applications: A Meta-Analysis and Systematic Review. ISPRS J. Photogrammetry Remote Sensing 124, 152–170. doi:10.1016/j.isprsjprs.2020.04.001
The European Commission, and PwC Advisory France (2019). Copernicus Market Report. Available: https://www.copernicus.eu/sites/default/files/2019-02/PwC_Copernicus_Market_Report_2019_PDF_version.pdf (Accessed July 28, 2021).
Vescoukis, V., Doulamis, N., and Karagiorgou, S. (2012). A Service Oriented Architecture for Decision Support Systems in Environmental Crisis Management. Future Generation Comp. Syst. 28 (3), 593–604. doi:10.1016/j.future.2011.03.010
Virapongse, A., Pearlman, F., Pearlman, J., Murambadoro, M. D., Kuwayama, Y., and Glasscoe, M. T. (2020). Ten Rules to Increase the Societal Value of Earth Observations. Earth Sci. Inform. 13 (2), 233–247. doi:10.1007/s12145-020-00453-w
Walker, J. J., de Beurs, K. M., and Wynne, R. H. (2014). Dryland Vegetation Phenology across an Elevation Gradient in Arizona, USA, Investigated with Fused MODIS and Landsat Data. Remote Sensing Environ. 144, 85–97. doi:10.1016/j.rse.2014.01.007
Wehn, U., and Evers, J. (2015). The Social Innovation Potential of ICT-Enabled Citizen Observatories to Increase eParticipation in Local Flood Risk Management. Tech. Soc. 42, 187–198. doi:10.1016/j.techsoc.2015.05.002
Wesselink, A., Buchanan, K. S., Georgiadou, Y., and Turnhout, E. (2013). Technical Knowledge, Discursive Spaces and Politics at the Science–Policy Interface. Environ. Sci. Pol. 30, 1–9. doi:10.1016/j.envsci.2012.12.008
Wu, B., Tian, F., Zhang, M., Zeng, H., and Zeng, Y. (2020). Cloud Services with Big Data Provide a Solution for Monitoring and Tracking Sustainable Development Goals. Geogr. Sustainability 1 (1), 25–32. doi:10.1016/j.geosus.2020.03.006
Wulder, M. A., White, J. C., Goward, S. N., Masek, J. G., Irons, J. R., Herold, M., et al. (2008). Landsat Continuity: Issues and Opportunities for Land Cover Monitoring. Remote Sensing Environ. 112, 955–969. doi:10.1016/j.rse.2007.07.004
Yang, C., Raskin, R., Goodchild, M., and Gahegan, M. (2010). Geospatial Cyberinfrastructure: Past, Present and Future. Comput. Environ. Urban Syst. 34 (4), 264–277. doi:10.1016/j.compenvurbsys.2010.04.001
Zell, E., Huff, A. K., Carpenter, A. T., and Friedl, L. A. (2012). A User-Driven Approach to Determining Critical Earth Observation Priorities for Societal Benefit. IEEE J. Selected Top. Appl. Earth Observations Remote Sensing 5 (6), 1594–1602. doi:10.1109/jstars.2012.2199467
Zhu, Z., Wulder, M. A., Roy, D. P., Woodcock, C. E., Hansen, M. C., Radeloff, V. C., et al. (2019). Benefits of the Free and Open Landsat Data Policy. Remote Sensing Environ. 224, 382–385. doi:10.1016/j.rse.2019.02.016
Keywords: Landsat, data ecosystem, values, decision support, societal benefits, qualitative, remote sensing, Earth observation
Citation: Molder EB, Schenkein SF, McConnell AE, Benedict KK and Straub CL (2022) Landsat Data Ecosystem Case Study: Actor Perceptions of the Use and Value of Landsat. Front. Environ. Sci. 9:805174. doi: 10.3389/fenvs.2021.805174
Received: 29 October 2021; Accepted: 20 December 2021;
Published: 04 February 2022.
Edited by:
Dmitry Efremenko, German Aerospace Center (DLR), GermanyReviewed by:
Polina Lemenkova, Université libre de Bruxelles, BelgiumLeo Lymburner, Geoscience Australia, Australia
Copyright © 2022 Molder, Schenkein, McConnell, Benedict and Straub. This is an open-access article distributed under the terms of the Creative Commons Attribution License (CC BY). The use, distribution or reproduction in other forums is permitted, provided the original author(s) and the copyright owner(s) are credited and that the original publication in this journal is cited, in accordance with accepted academic practice. No use, distribution or reproduction is permitted which does not comply with these terms.
*Correspondence: Crista L. Straub, cstraub@usgs.gov
†These authors have contributed equally to this work and share first authorship