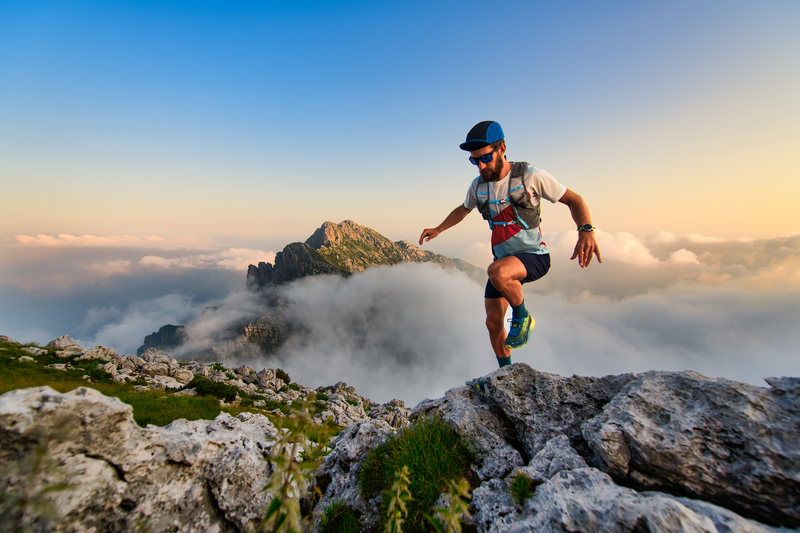
94% of researchers rate our articles as excellent or good
Learn more about the work of our research integrity team to safeguard the quality of each article we publish.
Find out more
ORIGINAL RESEARCH article
Front. Environ. Sci. , 14 January 2022
Sec. Environmental Economics and Management
Volume 9 - 2021 | https://doi.org/10.3389/fenvs.2021.783835
This article is part of the Research Topic Accentuating the Effects of Digital Circular Economy Transformations on Environmental Sustainability View all 25 articles
Knowledge sharing (KS) in the green supply chain (GSC) is jointly determined by the KS efforts of suppliers and manufacturers. This study uses the differential game method to explore the dynamic strategy of KS and the benefits of emission reduction in the process of low carbon (LC) technology in the GSC. The optimal trajectory of the knowledge stock and emission reduction benefits of suppliers and manufacturers under different strategies are obtained. The validity of the model and the results are verified by numerical simulation analysis, and the sensitivity analysis of the main parameters in the case of collaborative sharing is carried out. The results show that in the case of centralized decision-making, the KS efforts of suppliers and manufacturers are the highest, and the knowledge stock and emission reduction benefits of GSC are also the best. The cost-sharing mechanism can realize the Pareto improvement of GSC’s knowledge stock and emission reduction benefits, but the cost-sharing mechanism can only increase the supplier’s KS effort level. In addition, this study found that the price of carbon trading and the rate of knowledge decay have a significant impact on KS. The study provides a theoretical basis for promoting KS in the GSC and LC technology innovation.
With the frequent occurrence of extreme natural disasters around the world, climate change caused by excessive CO2 emissions has severely hindered the sustainable development of mankind and threatened ecosystems and biodiversity (Aldieri and Vinci, 2020; Hao and Li, 2020). In order to realize the sustainable development of mankind, the governments call for energy conservation and emission reduction, the implementation of carbon policies (carbon tax, carbon subsidies, carbon emission trading mechanism), and the development of a low carbon (LC) economy with practical actions (Wang et al., 2016). Some countries have set carbon peak and carbon neutral targets. The Chinese government firmly stated that it will reach the peak of carbon emissions by 2030 and achieve the goal of carbon neutrality by 2060. The US government has made it clear that it will achieve carbon neutrality by 2050. At present, the US federal government has not formulated a specific carbon neutral plan. However, some state governments have established relatively complete carbon emission systems and carbon market trading systems, such as the California government. But what is certain is that the US carbon neutral path mainly focuses on carbon emission reduction in energy applications, while China is more concerned about carbon emission reduction in energy supply. Regardless of the path, LC technology innovation is the fundamental driving force for achieving the goal of carbon neutrality.
The practice and exploration of LC emission reduction shows that green supply chain (GSC) and LC technology innovation are one of the important ways to develop a LC economy and reduce carbon emissions (Hoggett et al., 2014; Qu et al., 2021). Green supply chain introduces environmental protection concepts into supply chain management to improve supply chain ecology and financial performance (Khan et al., 2021c). Low-carbon technological innovations, such as Industry 4.0 and blockchain technology (Khan et al., 2021a; Khan et al., 2021d; Yu Z. et al., 2021), are essential to the sustainable development of GSC (Zhou and Ren, 2021). Knowledge is the key driving force for LC technology innovation, and acquiring knowledge can strengthen the innovation capabilities of enterprises (Rumanti et al., 2019). Collaboration refers to the process of cooperation between suppliers and manufacturers to achieve specific goals by pooling resource advantages. Collaborative innovation of LC technology is to control the carbon emissions of the GSC from an overall perspective, which can significantly reduce the carbon emissions of the GSC (Hao and Li, 2020). Under the constraints of the carbon quota system, the reduction of carbon emissions allows excess carbon allowances to be used for trading in the carbon market to obtain emissions reduction benefits. LC technology innovation relies on the sharing, capture and transformation of knowledge. Knowledge sharing (KS) is the core link of knowledge management, and it is also the key to promoting LC technology innovation (Ma et al., 2020) and improving the emission reduction benefits of the GSC. The core of knowledge management is to realize the re-creation of knowledge through the sharing, transfer, absorption, utilization and integration of knowledge between subjects, thereby completing the overall collaborative innovation process (Zhang et al., 2020). In the entire collaborative innovation process, KS is the prerequisite. This paper mainly studies the impact of KS on LC technology collaborative innovation and GSC emission reduction benefits.
Scholars have done a lot of research on LC technology innovation. Bi and Wang (2015) established an indicator system to evaluate the performance of China’s manufacturing LC technology innovation. Lyu X. et al. (2020) studied the impact of the carbon emissions trading system on low-carbon technology innovation. The results show that the carbon emissions trading system can promote the increase of low-carbon technological innovation year by year, but it will inhibit the development of low-carbon technological innovation in the short term. According to research by Zhang et al. (2021), technology priority, economic scale and research and development efficiency are the main driving factors for LC technological innovation. It can be found that the promotion of LC technology innovation from the perspective of KS does not seem to have attracted much attention. Zheng (2021) used the evolutionary game method to study the KS in the LC innovation network. However, the evolutionary game cannot reflect the dynamic changes in the amount of knowledge in the game process, and it does not consider the impact of emission reduction benefits on the KS strategy of suppliers and manufacturers. A further study is needed. As time evolves, knowledge and technology are constantly updated and revised. This has a direct impact on the KS behavior of suppliers and manufacturers. In order to improve competitiveness, suppliers and manufacturers need to constantly share and acquire knowledge (Ma et al., 2020). Therefore, the KS problem in the GSC should be studied from the perspective of the dynamic evolution of the decision-making body’s behavior. The differential game model is to study the conflict and cooperation of decision-making subjects in dynamic systems. More specifically, it is to study the optimal control problem of one or more state variables evolving over time according to the differential equation (Chen et al., 2012).
Therefore, from the perspective of dynamic change, a differential game model between suppliers and manufacturers is established to explore the KS strategy and emission reduction benefits in the process of LC technology collaborative innovation in the GSC. The purpose of this study is to explore the optimal degree of KS between suppliers and manufacturers under different sharing strategies and the optimal trajectory of the evolution of knowledge stock over time. In addition, this study is dedicated to exploring the law of changes in emission reduction benefits and the main factors affecting the stock of knowledge in the GSC.
The structure of the rest of this article is as follows: The Section 2 is literature review, which reviews the relevant literature and finds out the research gaps. The Section 3 is methodology. The KS strategy model is established, and the results of the model are compared and analyzed. The Section 4 is numerical simulation, which analyzes the simulation results and studies the sensitivity of the main parameters. The Section 5 is the conclusion and implication, and puts forward the future research direction.
The green supply chain should integrate environmental factors into product design, procurement, manufacturing, sales, and distribution (Yu and Khan, 2021). Khan et al. (2021e) believed that the application of advanced technology and the establishment of contact with the external environment can break through multiple supply chain management barriers. In order to achieve more effective green innovation, companies use their social capital to generate additional competitive advantages through collaboration (Chen and Hung, 2014). Collaborative innovation provides a new direction for the development of GSCs (Zhang and He, 2018), and is an important link to gain competitive advantage and achieve sustainable development goals (Zhou et al., 2020). GSC collaborative innovation is to use the specific advantages of individual organizations to jointly solve the problem of green management through a collaborative approach (Hong et al., 2019).
Hong et al. (2019) revealed the positive impact of organization-organization collaborative innovation, organization-government collaborative innovation, and organization-institution collaborative innovation on innovation performance. Collaborative innovation partner selection is an important factor affecting the green innovation of supply chain enterprises. The technical capabilities, resource integration capabilities, and learning capabilities of partners should be focused on (Melander, 2018; Xia et al., 2020). Hao and Li (2020) explored the collaborative innovation between enterprises in a green supply chain composed of a manufacturer and a supplier. The research found that the cooperative game is the optimal scenario for collaborative innovation. In terms of the impact of consumer preferences on green innovation, He et al. (2019) found that changes in consumer preferences are a key factor in promoting green innovation by companies in the supply chain. Li Q. et al. (2020) studied the decision-making mechanism and determinants of green collaborative innovation from the perspective of transaction cost economics and social exchange theory. Yang and Lin (2020) confirmed the importance of supply chain collaboration to green innovation, and found that environmental regulations, top management commitment and social recognition are the main driving forces for the implementation of green innovation. Zhai et al. (2021) studied the positive effects of governmental incentives and punishments, the trust between enterprises on the collaborative innovation of green technology in enterprises. Song et al. (2020) analyzed the mediating role of absorptive capacity between green KS and green innovation, and suggested that companies develop their absorptive capacity to achieve green innovation.
Collaborative innovation improves the environmental performance of the GSC and is conducive to promoting the sustainable development of the GSC (Abbas and Sagsan, 2019; Shi et al., 2021). It can be found that the existing research seldom pays attention to the influence of knowledge on GSC collaborative innovation, and seldom studies the decision-making mechanism of KS behavior of suppliers and manufacturers. What is the impact mechanism between KS and LC technology innovation? It is necessary to study LC technology innovation in the green supply chain from the perspective of knowledge sharing. This is essential to improve the efficiency of LC technology innovation.
The innovation and promotion of renewable energy can curb carbon dioxide emissions, help the government develop clean industries, and achieve carbon neutrality goals (Balsalobre-Lorente et al., 2021), while having a positive impact on economic development (Khan et al., 2021b). Bekun et al. (2021) believes that sustainable economic growth can be achieved by developing clean technologies and increasing the share of renewable energy in the energy structure. Krarti and Aldubyan (2021) advocated the use of photovoltaics and wind as renewable energy technologies to achieve carbon neutrality in residential communities. Cao et al. (2021) studied the impact of cleaner production standards on the total factor productivity and resource allocation efficiency of Chinese energy companies. They found that cleaner production regulations reduce the efficiency of resource allocation in the energy industry, but increase the level of enterprise total factor productivity. Gunarathne and Sankalpani (2021) believed that clean technology should be promoted as a management technology. Gupta et al. (2021) established a framework based on the concepts of circular economy, sustainable clean production and industry 4.0 standards to evaluate the sustainable performance of manufacturing companies. Wei et al. (2022) suggested the use of photovoltaic power generation and electrolysis of hydrogen to achieve carbon emission neutrality in industrial parks, and used examples to prove that this solution would reduce emissions by 61% compared with the solution relying on the grid and natural gas. Fortes et al. (2022) analyzed the impact of climate change on the demand for renewable resources and electricity. The results showed that climate change reduces hydropower generation by 20%, which will also reduce the cost-effectiveness of solar photovoltaics.
The application and innovation of cleaner production technologies can help achieve carbon neutrality. However, it can be found that the research on knowledge management in cleaner production has not received much attention.
KS and collaborative innovation are effective models for the development of LC technologies. LC technology innovation and knowledge accumulation are mutually reinforcing (Hoette et al., 2021; Vinholis et al., 2021). Technology innovation can promote knowledge accumulation (Vinholis et al., 2021), and LC technology innovation depends on knowledge accumulation (Hoette et al., 2021). KS has a significant impact on the performance of LC technology innovation (Brandao Vinholis et al., 2021; Effendi et al., 2021). Gu et al. (2021) studied the impact of global LC technology transfer on carbon emission reduction. The study found that the sharing of LC technologies has achieved a significant increase in carbon emission reduction, while the emission reduction in developing countries is mainly limited by the stock of knowledge and research and development investment. KS in the process of LC technology transfer and innovation will be affected by organizational mechanisms (Hayashi, 2018). Abbas and Sagsan (2019) studied the effect of knowledge management on the green innovation and sustainable development of enterprises. Through structural equation models, they proved that KS has a significant impact on green innovation, and green innovation can promote the sustainable development of enterprises. The willingness of enterprises to share knowledge is related to the breadth and depth of their own knowledge. Enterprises with a wide range of knowledge are more willing to share knowledge, while enterprises with deep knowledge are unwilling to share knowledge (Lyu C. et al., 2020).
From the literature review, it can be found that the current research on LC technology innovation and KS mainly focuses on the impact of KS on LC technology innovation performance, the role of KS in promoting LC technology innovation and some obstacles to the implementation of KS. However, scholars seldom pay attention to the evolution of KS strategies in LC technology innovation and the dynamic changes of knowledge stock and emission reduction benefits under different sharing strategies. As time changes, knowledge will be updated and attenuated, and the KS strategy in LC technology innovation should also be constantly changing. Therefore, from the perspective of dynamic evolution, it is necessary to study the relationship between KS and LC technology innovation and emission reduction benefits.
The differential game model is a combination of optimal control and game theory. It studies the problems of cooperation and conflict between game subjects in dynamic systems (Chen et al., 2012). In a continuous time frame, multiple participants play a continuous game in an effort to optimize their respective goals. Through the model solution, the strategy of the game player’s evolution over time can be obtained and the Nash equilibrium can be reached. The differential game model has certain advantages for studying the optimal problem of dynamic control, and is widely used in the research of LC technology innovation and supply chain emission reduction problems. Hao and Li (2020) used the differential game model to study the collaborative innovation mechanism between enterprises in a GSC composed of a manufacturer and a supplier. Deng et al. (2021) and Wang et al. (2019b) established a differential game model from the perspective of carbon tax and studied the optimal solutions under different decision-making modes. The research results showed that carbon tax can promote LC technology innovation. Hou et al. (2020) used the differential game model to explore the dynamic emission reduction technology investment decision-making problem in the binary supply chain composed of manufacturers and retailers. Si et al. (2020) established a time-lag differential price game model, and analyzed the equilibrium strategy of price competition between technology supply and demand companies and the local asymptotic stability of the game system at the equilibrium point. Yin and Li (2018) used stochastic differential game to analyze the LC technology sharing problem in enterprise collaborative innovation. The results showed that random interference factors have the most significant impact on the level of LC technology in cooperative games. You and Zhu (2016) used the differential game model to study the LC supply chain R&D, promotion and pricing issues. Zhang et al. (2019) explored the joint dynamic green innovation policy and pricing strategy in the hybrid manufacturing and remanufacturing system. The results showed that too high or too low a carbon emission limit will reduce the manufacturer’s revenue.
In addition, differential games are also used in the study of KS problems. Lin and Wang (2019) used differential game theory to establish a dynamic incentive model to study the dynamic KS of construction project teams. Zhu et al. (2017) considered random interference factors and established a stochastic differential game model to study the problem of KS in industry-university-research collaborative innovation. It can be found that few scholars use the differential game model to study the problem of KS and emission reduction benefits in the collaborative innovation of LC technologies in the GSC. In the GSC, the knowledge level of suppliers and manufacturers is constantly changing over time. Therefore, the differential game model is used to study the optimal shared effort level, emission reduction benefits and knowledge changes over time between suppliers and manufacturers in the GSC.
In summary, the following research gaps can be found through literature review: 1) there is a lack of research on KS strategies in the dynamic process of collaborative innovation between suppliers and manufacturers. 2) In LC technology innovation, the dynamic relationship between KS strategy and knowledge stock and emission reduction benefits has not received attention. Therefore, this paper establishes a differential game model from the perspective of KS to dynamically study the issue of LC technology collaborative innovation in the GSC. This research aims to explore the evolutionary laws of knowledge stock under different KS strategies, as well as the optimal degree of effort and optimal emission reduction benefits of KS between suppliers and manufacturers. This research is of great significance for promoting the KS of GSC, improving the efficiency of LC technology innovation and the ability of supply chain to reduce carbon emissions.
In the collaborative innovation of the GSC, the KS behavior of suppliers and manufacturers is driven by costs and benefits (Li H. et al., 2020). This study establishes a differential game model to study the KS strategy and emission reduction benefits in the collaborative innovation of LC technologies in the GSC. As time evolves, suppliers and manufacturers continue to play games to optimize their own costs and benefits and eventually reach Nash equilibrium.
Assumption 1 The amount of knowledge generated by the collaborative innovation of LC technologies in the GSC is determined by the KS effort of the supplier and the KS effort of the manufacturer. At the same time, knowledge is attenuated. As technology is iterated and updated, existing knowledge will be replaced by new knowledge. Therefore, the stock of knowledge is a dynamic variable that changes with time. Differential equations can be used to describe the law of the stock of knowledge with time (Plambeck, 2012; Ma et al., 2020):
Among them,
Assumption 2 When the degree of effort increases, the sharing cost also shows an increasing trend. Therefore, the shared cost
Among them,
Among them,
Assumption 3 In order to encourage suppliers and manufacturers to actively share knowledge and promote LC technology innovation, a government subsidy mechanism is introduced to subsidize the shared costs of suppliers and manufacturers. It is assumed that the government’s subsidy coefficients of KS costs for suppliers and manufacturers at time
Assumption 4 LC technology can promote carbon emission reduction and obtain certain economic benefits. The size of the benefits is related to the stock of knowledge in the GSC and the price of carbon allowances in the carbon trading market (Wang et al., 2019c). Therefore, the benefits of KS are ultimately manifested as emissions reduction benefits brought about by LC technology innovation. Let
Assumption 5 Suppliers and manufacturers make decisions based on complete information. And at any time
In the case of decentralized decision-making without cost sharing, suppliers and manufacturers form a Nash non-cooperative game. Both parties independently share knowledge and choose their own efforts to maximize profits. At this time, the supplier’s optimal profit function
It can be seen from the above formula that the benefits of suppliers and manufacturers at time
Proposition 1 The equilibrium results in the case of decentralized decision-making without cost sharing are as follows:
(1) The optimal trajectory of the knowledge stock
where,
(2) The optimal effort level
(3) The supplier’s emission reduction revenue function
where,
(4) The carbon emission reduction benefit function
Proof: According to the optimal control theory, from Eq. 1, the objective function of the supplier’s optimal decision-making at time
Make
The Eq. 3 can be expressed as
At this time, the supplier’s optimal decision objective function can be transformed into the following HJB equation
Expand Eq. 6
Equation 7 is about the concave function of
Similarly, according to the proof process of Eqs 3–5, the manufacturer’s optimal decision objective function
In Eq. 9,
Expand Eq. 10
Equation 11 is about the concave function of
It can be seen from Eqs 8, 12 that the degree of KS effort is positively correlated with the government subsidy coefficient and the influence coefficient of knowledge stock, while it is negatively correlated with the effort cost coefficient of KS. Substitute Eq. 8 into Eqs 7, 12 into Eq. 11, and get
Suppose the linear expressions of
Where,
In this study, the undetermined coefficient method was used to solve the equation, as shown below
Equation 18 and its first derivative
Equation 19 is substituted into
In order to obtain more information that is conducive to LC technology innovation, manufacturers encourage suppliers to actively share knowledge and share the cost of supplier sharing. The cost sharing ratio is
In order to determine the equilibrium strategy of the supplier and the manufacturer, the HJB equation is used to solve it. The solution result is shown in Proposition 2.
Proposition 2 The equilibrium result of decentralized decision-making under cost sharing is as follows:
(1) The optimal trajectory of the knowledge stock
where,
(2) The supplier’s optimal effort level
(3) The supplier’s optimal emission reduction revenue function
where,
(4) The emission reduction revenue function of the GSC
(5) The manufacturer’s share of the supplier’s KS cost
Proof: Using the inverse induction method to solve, from Eq. 20, we can see that the supplier’s optimal profit function
where,
It can be seen that Eq. 23 is about the concave function of
In the same way, the manufacturer’s optimal profit function
where,
According to the related theory of Hessian matrix (Wang D. et al., 2019), Eq. 26 is a concave function, and the maximum value is obtained when the partial derivative is equal to zero. In order to solve
The manufacturer’s share of the supplier’s KS cost ratio
According to the characteristics of Eqs 28, 29, it is assumed that the linear expressions of
where,
Substituting
Equations 31,
Equation 32 is substituted into
Collaborative sharing is knowledge cooperation between suppliers and manufacturers aiming at the overall technology innovation of the GSC and maximizing the benefits of emission reduction. The collaborative sharing of LC technologies between suppliers and manufacturers in the GSC can improve the level of KS, realize resource integration and complement each other’s advantages. In addition, it can also reduce the information asymmetry between suppliers and manufacturers and promote LC technology innovation. In the case of collaborative sharing, it is assumed that both parties make decisions with the goal of maximizing the overall benefits of the GSC. The decision variables include the supplier’s KS effort
Using the HJB equation, the equilibrium strategy of the decision problem can be obtained, as shown in Proposition 3.
Proposition 3 The equilibrium results in centralized decision-making are as follows:
(1) The optimal trajectory of the knowledge stock
where,
(2) The supplier’s optimal effort level
(3) The optimal value function
where,
Let
At this time, the decision-making problem of collaborative KS satisfies the following HJB equation
Expand the Eq. 33 as follows
The Hessian matrix of Eq. 34 for
Since
Substituting Eq. 35 into Eq. 34 can get:
The optimal value function of Eq. 36 with respect to
Equation 37 is substituted into Eq. 36, the constraint equations about
Substituting the first derivative of Eq. 38 into Eq. 35, the equilibrium solution
Substitute Eq. 39 into
In the case of decentralized decision-making without cost sharing and decentralized decision-making with cost sharing, a comparative analysis of the degree of KS effort between suppliers and manufacturers can be obtained:
Corollary 1 When the supplier’s carbon emission reduction revenue distribution ratio is
Corollary 2 Regardless of the supplier’s carbon emission reduction revenue distribution ratio, there are
Corollary 3 When the supplier’s carbon emission reduction revenue is
Corollary 4 When the supplier’s carbon emission reduction revenue is
In order to further study the KS behavior and carbon emission reduction benefits of suppliers and manufacturers in the GSC. In this paper, Matlab is used to analyze three kinds of differential game situations. The parameter assignments are as follows:
Figure 1A depicts the improvement of the cost-sharing mechanism to the carbon emission reduction benefits of suppliers and manufacturers. The abscissa represents time
FIGURE 1. Carbon emission reduction benefits under different KS strategies. (A) Increased revenue under the cost-sharing mechanism. (B) Emission reduction benefits under different strategies.
Figure 1B describes the evolution of the overall carbon emission reduction benefits of the GSC over time under different sharing strategies.
Figure 2 depicts the trend of the knowledge stock in the GSC over time under different sharing strategies.
Figure 3 describes the KS effort level of suppliers and manufacturers in different decision-making situations. Figure 3A shows the KS effort level
The manufacturer’s share of the supplier’s KS cost is related to the supplier’s carbon emission reduction income
This paper analyzes the sensitivity of the main parameters of knowledge stock of GSC under centralized decision making. The sensitivity of supplier’s knowledge creation level
Figures 5, 6 show that with the increase of suppliers’ knowledge creation level
In Figure 6, the higher the carbon allowance price, the greater the stock of knowledge in the GSC. This shows that the carbon emissions trading mechanism will have a certain impact on the behavior of decision-making entities, and this impact can be reflected through carbon prices. When the price of carbon allowances is high, decision-making entities will actively participate in LC technology research and development activities to reduce their own carbon emissions. At the same time, excess carbon allowances can be traded in the carbon market to increase their own profits. At this time, the stock of knowledge in the GSC will gradually increase. When the price of carbon allowances is low, the decision-making body will consider whether to invest in LC technology research and development or purchase carbon allowances based on costs and benefits. At this time, the knowledge stock of the GSC is relatively small. Therefore, the price of carbon allowances plays a vital role in promoting decision-making entities to participate in LC technology research and development activities and KS. The carbon trading mechanism should be improved according to the actual situation, and the price of carbon allowances should be reasonably regulated.
Figures 7, 8 show that as the relative decay rate
Figure 9 describes the impact of the government’s cost subsidy coefficient
From the results of the sensitivity analysis, it can be seen that the cost-sharing ratio of the manufacturer to the supplier’s KS is negatively related to the supplier’s carbon emission reduction benefits. At the same time, the cost-sharing ratio will be affected by the government’s subsidy coefficient for supplier KS costs. The larger the government subsidy coefficient is, the lower the manufacturer’s cost-sharing ratio. The knowledge stock is the most sensitive to the knowledge decay rate. When the decay rate increases, the knowledge stock decreases significantly, and it shows a decreasing trend from strong to weak. In order to cope with the phenomenon of knowledge decline caused by rapid technological development, suppliers and manufacturers should collaborate in innovation to reduce the time from R&D to commercial application of LC technologies. The stock of knowledge is positively correlated with the level of knowledge creation of the supplier. The study also found that the higher the level of knowledge creation, the stronger the supplier’s ability to innovate in LC technology. Technology innovation and accumulation have increased the knowledge reserve in the GSC. The carbon price is positively correlated with the stock of knowledge. As the price of carbon increases, the stock of knowledge gradually increases. The mechanism of carbon price on knowledge stock is a feedback mechanism from emission reduction benefits to technology innovation to KS. The whole process is mainly to increase knowledge stock through LC technology innovation. The increase in carbon prices has increased suppliers’ emissions reduction benefits, and LC technology innovation is the main way to increase carbon emissions reduction benefits. The government’s subsidy policy has a certain incentive effect on the increase of knowledge stock, but the incentive effect is not significant, so it is necessary to determine a reasonable subsidy coefficient.
KS is the key to achieving green innovation (Zhou et al., 2020). The absorption and utilization of knowledge in the supply chain can achieve outstanding green innovation (Song et al., 2020). Existing studies have pointed out that the sharing strategy affects the level of KS between suppliers and manufacturers (Ma et al., 2020; Zheng, 2021). When choosing different sharing strategies, the level of KS has a certain improvement, which is related to the benefits, costs and the degree of communication between the knowledge receiver and the sharer (Li H. et al., 2020). However, this study found that the effect of different strategies on the improvement of knowledge stock is not continuously increasing. Under different strategies, the evolution trend of the knowledge stock in the GSC is that it first increases and then stabilizes over time, which indicates that the knowledge amount in the GSC can be controlled and adjusted.
This research further analyzes the change trajectory of knowledge stock and the change law of emission reduction benefits under different strategies. The study found that collaborative sharing significantly increased the supply chain’s knowledge stock and emission reduction benefits. The collaborative sharing strategy can improve the KS level of suppliers and manufacturers, and can promote LC technology innovation. This is because low-carbon supply chains can only be coordinated through revenue sharing-cost sharing (Jiang and Chen, 2016). Collaborative innovation can promote green technology research and development (Chen et al., 2017; Ardito et al., 2019; Yu X. et al., 2021). The research results of this article can explain this conclusion from another angle. Sensitivity analysis results show that the stock of knowledge has the strongest sensitivity to knowledge decay rate. However, as the decay rate increases, the timeliness of knowledge decreases rapidly over time, knowledge gradually loses its original value, and knowledge stock decreases. Collaborative innovation can reduce the research and development costs of technology innovation and shorten the research and development cycle, which can reduce the loss in the KS process and improve the efficiency of knowledge creation and green technology research and development. Cost sharing contracts can induce manufacturers to increase investment in clean technology (Shen et al., 2017), and the same cost sharing mechanism can promote the level of knowledge sharing between manufacturers and suppliers.
The carbon emission trading mechanism leads to higher operating costs for companies (Hu and Ding, 2020), because companies invest more in LC technology research and development. With the increase in carbon prices, the stock of knowledge in the GSC has increased significantly. The mechanism of carbon price on knowledge stock is a feedback mechanism from emission reduction benefits to technology innovation to KS. The whole process is mainly to realize the increase of knowledge stock through LC technology innovation. The research of Cong et al. (2020) shows that low-carbon subsidies have a positive effect on reducing emissions in the GSC. However, the research results of this article find that the knowledge sharing subsidy policy cannot significantly promote the participation of suppliers and manufacturers in the GSC in KS, and the government should reasonably set the subsidy ratio.
This paper uses a differential game model to study the KS and emission reduction benefits of suppliers and manufacturers in the process of collaborative innovation of LC technologies in the GSC. Three game models are established: decentralized decision-making without cost sharing, decentralized decision-making with cost sharing, and centralized decision-making. This study analyzes the changes in GSC’s knowledge stock and emission reduction benefits under three scenarios, as well as the optimal effort level of KS between suppliers and manufacturers. A comparative analysis of the results in various situations is carried out, and a sensitivity analysis of the main parameters is also done. The conclusions of this paper are as follows: 1) the cost-sharing mechanism can only increase the supplier’s KS efforts, and the degree of improvement is related to the supplier’s carbon emission reduction income distribution ratio. 2) In the case of the Stackelberg master-slave game, the emission reduction benefits of suppliers and manufacturers and the stock of knowledge in the GSC have increased significantly. It shows that the cost-sharing mechanism can promote the shift of the game between suppliers and manufacturers from Nash non-cooperative game to Stackelberg master-slave game, and realize the Pareto improvement of carbon emission reduction benefits. This indicates that the cost-sharing mechanism is to promote the sharing of LC technology knowledge and is an effective measure to increase GSC’s emission reduction benefits. 3) In the centralized decision-making situation, the overall benefits of the GSC and the KS efforts of suppliers and manufacturers are greater than in the decentralized decision-making situation. At the same time, the stock of knowledge in the GSC has also been improved. Explain that the collaborative sharing strategy can promote KS, acquisition and LC technology innovation. 4) The price of carbon trading and the rate of knowledge decay have a significant impact on KS in the GSC, while the impact of government subsidy policies is not significant. Therefore, the government should promote the establishment of a carbon market trading system and industry alliances to promote the sharing of GSC-LC technical knowledge, and at the same time formulate a reasonable subsidy coefficient.
This research has three contributions to GSC’s KS research. 1) This study uses the differential game method to study the KS and emission reduction benefits of suppliers and manufacturers in the process of GSC’s collaborative innovation of LC technology from the perspective of dynamic changes. This makes up for the ignorance of the game problem in continuous time in previous studies. This research is of great significance for promoting KS in GSC and LC emission reduction. 2) In terms of GSC knowledge management, this research gives the optimal choice of KS strategy for suppliers and manufacturers. The cost-sharing mechanism can significantly promote the KS of suppliers, and the collaborative sharing strategy is the most ideal choice for suppliers and manufacturers. This provides guidance for KS between suppliers and manufacturers. 3) This study reveals the internal influence mechanism of main factors on the GSC knowledge stock and the KS behavior of suppliers and manufacturers. Carbon trading price and knowledge decay rate are the main driving factors of KS in GSC. The government subsidy policy cannot significantly promote the sharing of knowledge between suppliers and manufacturers and retrograde. The research results are of great significance to promote the innovation of LC technology and improve the efficiency of KS in GSC.
In order to increase the level of KS between suppliers and manufacturers and the benefits of emission reduction, and to promote LC technology innovation, this article proposes the following suggestions:
(1) The government should encourage the establishment of LC technology innovation alliances. Innovation alliances can reduce the information asymmetry between suppliers and manufacturers, and can reduce the barriers to knowledge sharing between the two. This is helpful to promote the innovation of LC technology. The government also needs to provide some tax subsidies to alliance members to improve the KS cost and risk tolerance of suppliers and manufacturers.
(2) The GSC-KS incentive mechanism needs to be reformulated or improved. First of all, the amount of government subsidies for LC technology innovations of suppliers and manufacturers should be moderate. This study shows that an excessively high subsidy ratio will not significantly promote GSC’s KS, but will increase the government’s burden. Second, suppliers and manufacturers need to establish a cost-sharing mechanism for LC technology innovation. Suppliers and manufacturers must determine the cost-sharing ratio based on emission reduction benefits and government subsidies. This is an effective means to improve suppliers’ KS levels and GSC emission reduction benefits.
(3) The government needs to promote the establishment of a unified regional carbon emissions trading mechanism and reasonably regulate carbon trading prices. The carbon quota system needs to be established and implemented quickly. The government must strictly implement the allocation, management and assessment mechanism of carbon allowances. The price of carbon has a significant impact on the stock of GSC knowledge. Through reasonable adjustments to the carbon market allowance transaction price, the LC technology innovation and KS willingness of suppliers and manufacturers will be improved.
In the research process of this paper, only the KS between a single supplier and a single manufacturer is considered, and the retailer is not taken into consideration. At the same time, the problem of KS between multiple suppliers and multiple manufacturers is not considered. In the future, we can further study the KS of multi-agent and multi-channel GSC networks. In addition, it is important to study the influence mechanism of consumers’ green product preference on the KS behavior of suppliers and manufacturers in the GSC.
The original contributions presented in the study are included in the article/supplementary material, further inquiries can be directed to the corresponding author.
FW and HL contributed to conception and design of the study. YR organized the database. CZ performed the statistical analysis. FW wrote the first draft of the manuscript. YC and HL wrote sections of the manuscript. All authors contributed to manuscript revision, read, and approved the submitted version.
The authors acknowledge with gratitude the MOE (Ministry of Education in China) Project of Humanities and Social Sciences (No. 19YJC630078), Fund of Innovative Education Program for Graduate Students at North China University of Water Resources and Electric Power (No. YK2020-09), National Key R&D Program of China (No. 2018YFC0406905), Youth Talents Teachers Scheme of Henan Province Universities (No. 2018GGJS080), the National Natural Science Foundation of China (Nos. 71974056 and 71302191), 2021 Key R&D and Promotion Special Project of Henan Province (Tackling key science and Technology) (No. 212102310392), China Scholarship Council (No. 201908410388), 2018 Henan Province Water Conservancy Science and Technology Project (GG201828). This study would not have been possible without their financial support.
Author YR was employed by the company Henan Water Valley Innovation Technology Research Institute Co., Ltd.
The remaining authors declare that the research was conducted in the absence of any commercial or financial relationships that could be construed as a potential conflict of interest.
All claims expressed in this article are solely those of the authors and do not necessarily represent those of their affiliated organizations, or those of the publisher, the editors and the reviewers. Any product that may be evaluated in this article, or claim that may be made by its manufacturer, is not guaranteed or endorsed by the publisher.
GSC, green supply chain; GSC-KS, green supply chain knowledge sharing; KS, knowledge sharing; LC, low carbon.
Abbas, J., and Sağsan, M. (2019). Impact of Knowledge Management Practices on Green Innovation and Corporate Sustainable Development: A Structural Analysis. J. Clean. Prod. 229, 611–620. doi:10.1016/j.jclepro.2019.05.024
Aldieri, L., and Vinci, C. P. (2020). Climate Change and Knowledge Spillovers for Cleaner Production: New Insights. J. Clean. Prod. 271, 122729. doi:10.1016/j.jclepro.2020.122729
Ardito, L., Messeni Petruzzelli, A., Pascucci, F., and Peruffo, E. (2019). Inter-Firm R&D Collaborations and Green Innovation Value: The Role of Family Firms' Involvement and the Moderating Effects of Proximity Dimensions. Bus Strat Env 28 (1), 185–197. doi:10.1002/bse.2248
Balsalobre-Lorente, D., Driha, O. M., Leitão, N. C., and Murshed, M. (2021). The Carbon Dioxide Neutralizing Effect of Energy Innovation on International Tourism in EU-5 Countries under the Prism of the EKC Hypothesis. J. Environ. Manage. 298, 113513. doi:10.1016/j.jenvman.2021.113513
Bekun, F. V., Adedoyin, F. F., Lorente, D. B., and Driha, O. M. (2021). Designing Policy Framework for Sustainable Development in Next-5 Largest Economies amidst Energy Consumption and Key Macroeconomic Indicators. Environ. Sci. Pollut. Res., 1–14. doi:10.1007/s11356-021-16820-z
Bi, K., and Wang, X. (2015). Study on the Performance of Chinese Manufacturing Low-Carbon Technology Innovation in the Global Value Chain. Paris: Atlantis Press.
Brandao Vinholis, M. D. M., Macchione Saes, M. S., Carrer, M. J., and de Souza Filho, H. M. (2021). The Effect of Meso-Institutions on Adoption of Sustainable Agricultural Technology: A Case Study of the Brazilian Low Carbon Agriculture Plan. J. Clean. Prod. 280, 124334. doi:10.1016/j.jclepro.2020.124334
Breton, M., Zaccour, G., and Zahaf, M. (2005). A Differential Game of Joint Implementation of Environmental Projects. Automatica 41 (10), 1737–1749. doi:10.1016/j.automatica.2005.05.004
Cao, H., Wang, B., and Li, K. (2021). Regulatory Policy and Misallocation: A New Perspective Based on the Productivity Effect of Cleaner Production Standards in China's Energy Firms. Energy Policy 152, 112231. doi:10.1016/j.enpol.2021.112231
Chen, J., Zhang, H., and Sun, Y. (2012). Implementing Coordination Contracts in a Manufacturer Stackelberg Dual-Channel Supply Chain. Omega 40 (5), 571–583. doi:10.1016/j.omega.2011.11.005
Chen, L., Zhao, X., Tang, O., Price, L., Zhang, S., and Zhu, W. (2017). Supply Chain Collaboration for Sustainability: A Literature Review and Future Research Agenda. Int. J. Prod. Econ. 194, 73–87. doi:10.1016/j.ijpe.2017.04.005
Chen, P.-C., and Hung, S.-W. (2014). Collaborative Green Innovation in Emerging Countries: A Social Capital Perspective. Int. J. Operations Prod. Manage. 34 (3), 347–363. doi:10.1108/ijopm-06-2012-0222
Cong, J., Pang, T., and Peng, H. (2020). Optimal Strategies for Capital Constrained Low-Carbon Supply Chains under Yield Uncertainty. J. Clean. Prod. 256, 120339. doi:10.1016/j.jclepro.2020.120339
Deng, Y., You, D., and Zhang, Y. (2021). Research on Improvement Strategies for Low-Carbon Technology Innovation Based on a Differential Game: The Perspective of Tax Competition. Sustainable Prod. Consumption 26, 1046–1061. doi:10.1016/j.spc.2021.01.007
Effendi, M. I., Widjanarko, H., and Sugandini, D. (2021). Green Supply Chain Integration and Technology Innovation Performance in SMEs: A Case Study in Indonesia. The J. Asian Finance Econ. Business 8 (4), 909–916. doi:10.13106/jafeb.2021.vol8.no4.0909
Fortes, P., Simoes, S. G., Amorim, F., Siggini, G., Sessa, V., Saint-Drenan, Y.-M., et al. (2022). How Sensitive Is a Carbon-Neutral Power Sector to Climate Change? the Interplay between Hydro, Solar and Wind for Portugal. Energy 239, 122106. doi:10.1016/j.energy.2021.122106
Gu, G., Wang, Z., and Wu, L. (2021). Carbon Emission Reductions under Global Low-Carbon Technology Transfer and its Policy Mix with R&D Improvement. Energy 216, 119300. doi:10.1016/j.energy.2020.119300
Gunarathne, N., and Sankalpani, U. (2021). Diffusion of Cleaner Production in a Developing Country: The Case of Sri Lanka. J. Clean. Prod. 311, 127626. doi:10.1016/j.jclepro.2021.127626
Gupta, H., Kumar, A., and Wasan, P. (2021). Industry 4.0, Cleaner Production and Circular Economy: An Integrative Framework for Evaluating Ethical and Sustainable Business Performance of Manufacturing Organizations. J. Clean. Prod. 295, 126253. doi:10.1016/j.jclepro.2021.126253
Hao, X., and Li, B. (2020). Research on Collaborative Innovation Among Enterprises in green Supply Chain Based on Carbon Emission Trading. Sci. Prog. 103 (2), 003685042091632. doi:10.1177/0036850420916329
Hayashi, D. (2018). Knowledge Flow in Low-Carbon Technology Transfer: A Case of India's Wind Power Industry. Energy Policy 123, 104–116. doi:10.1016/j.enpol.2018.08.040
He, J., Lei, Y., and Fu, X. (2019). Do Consumer's Green Preference and the Reference Price Effect Improve Green Innovation? A Theoretical Model Using the Food Supply Chain as a Case. Int. J. Environ. Res. Public Health 16 (24), 5007. doi:10.3390/ijerph16245007
Hoggett, R., Bolton, R., Candelise, C., Kern, F., Mitchell, C., and Yan, J. (2014). Supply Chains and Energy Security in a Low Carbon Transition. Appl. Energ. 123, 292–295. doi:10.1016/j.apenergy.2014.02.008
Hong, J., Zheng, R., Deng, H., and Zhou, Y. (2019). Green Supply Chain Collaborative Innovation, Absorptive Capacity and Innovation Performance: Evidence from China. J. Clean. Prod. 241, 118377. doi:10.1016/j.jclepro.2019.118377
Hötte, K., Pichler, A., and Lafond, F. (2021). The Rise of Science in Low-Carbon Energy Technologies. Renew. Sustain. Energ. Rev. 139, 110654. doi:10.1016/j.rser.2020.110654
Hou, Q., Guan, Y., and Yu, S. (2020). Stochastic Differential Game Model Analysis of Emission-Reduction Technology under Cost-Sharing Contracts in the Carbon Trading Market. Ieee Access 8, 167328–167340. doi:10.1109/access.2020.3023391
Hu, Y., and Ding, Y. (2020). Can Carbon Emission Permit Trade Mechanism Bring Both Business Benefits and green Efficiency? China Popul. Resour. Environ. 30 (3), 56–64.
Jiang, W., and Chen, X. (2016). Optimal Strategies for Low Carbon Supply Chain with Strategic Customer Behavior and Green Technology Investment. Discrete Dyn. Nat. Soc. 2016, 1–13. doi:10.1155/2016/9645087
Khan, S. A. R., Godil, D. I., Jabbour, C. J. C., Shujaat, S., Razzaq, A., and Yu, Z. (2021a). Green Data Analytics, Blockchain Technology for Sustainable Development, and Sustainable Supply Chain Practices: Evidence from Small and Medium Enterprises. Ann. Oper. Res. doi:10.1007/s10479-021-04275-x
Khan, S. A. R., Godil, D. I., Yu, Z., Abbas, F., and Shamim, M. A. (2021b). Adoption of Renewable Energy Sources, Low‐Carbon Initiatives, and Advanced Logistical Infrastructure-An Step toward Integrated Global Progress. Sustain. Develop. doi:10.1002/sd.2243
Khan, S. A. R., Mathew, M., Dominic, P. D. D., and Umar, M. (2021c). Evaluation and Selection Strategy for green Supply Chain Using Interval-Valued Q-Rung Orthopair Fuzzy Combinative Distance-Based Assessment. Environ. Dev. Sustain. doi:10.1007/s10668-021-01876-1
Khan, S. A. R., Razzaq, A., Yu, Z., and Miller, S. (2021d). Industry 4.0 and Circular Economy Practices: A new era Business Strategies for Environmental Sustainability. Bus Strat Env 30, 4001–4014. doi:10.1002/bse.2853
Khan, S. A. R., Zkik, K., Belhadi, A., and Kamble, S. S. (2021e). Evaluating Barriers and Solutions for Social Sustainability Adoption in Multi-Tier Supply Chains. Int. J. Prod. Res. 59 (11), 3378–3397. doi:10.1080/00207543.2021.1876271
Krarti, M., and Aldubyan, M. (2021). Role of Energy Efficiency and Distributed Renewable Energy in Designing Carbon Neutral Residential Buildings and Communities: Case Study of Saudi Arabia. Energy and Buildings 250, 111309. doi:10.1016/j.enbuild.2021.111309
Li, H., Wang, F., Wang, L., Su, L., and Zhang, C. (2020a). The Stochastic Evolution Game of Knowledge Sharing in the Infrastructure PPP Supply Chain Network. Complexity 2020, 1–17. doi:10.1155/2020/8858845
Li, Q., Kang, Y., Tan, L., and Chen, B. (2020b). Modeling Formation and Operation of Collaborative Green Innovation between Manufacturer and Supplier: A Game Theory Approach. Sustainability 12 (6), 2209. doi:10.3390/su12062209
Lin, L., and Wang, H. (2019). Dynamic Incentive Model of Knowledge Sharing in Construction Project Team Based on Differential Game. J. Oper. Res. Soc. 70 (12), 2084–2096. doi:10.1080/01605682.2018.1516177
Lyu, C., Yang, J., Zhang, F., Teo, T. S. H., and Mu, T. (2020a). How Do Knowledge Characteristics Affect Firm's Knowledge Sharing Intention in Interfirm Cooperation? An Empirical Study. J. Business Res. 115, 48–60. doi:10.1016/j.jbusres.2020.04.045
Lyu, X., Shi, A., and Wang, X. (2020b). Research on the Impact of Carbon Emission Trading System on Low-Carbon Technology Innovation. Carbon Manage. 11 (2), 183–193. doi:10.1080/17583004.2020.1721977
Ma, Y., Liu, H., and Liu, Q. (2020). Differential Game Study of Industrial Cluster Synergetic Innovation Knowledge Sharing Strategy. Operations Res. Manage. Sci. 29 (9), 82–88.
Melander, L. (2018). Customer and Supplier Collaboration in Green Product Innovation: External and Internal Capabilities. Bus. Strat. Env. 27 (6), 677–693. doi:10.1002/bse.2024
Plambeck, E. L. (2012). Reducing Greenhouse Gas Emissions through Operations and Supply Chain Management. Energ. Econ. 34, S64–S74. doi:10.1016/j.eneco.2012.08.031
Qu, G., Wang, Y., Xu, L., Qu, W., Zhang, Q., and Xu, Z. (2021). Low-Carbon Supply Chain Emission Reduction Strategy Considering the Supervision of Downstream Enterprises Based on Evolutionary Game Theory. Sustainability 13 (5), 2827. doi:10.3390/su13052827
Rumanti, A. A., Wiratmadja, I. I., Muhammad, F., Rizana, A. F., and Andrawina, L. (2019). “Green Production Implementation through Perspective of Knowledge Sharing and Open Innovation: Case Study at Indonesian Handmade Batik Industries,” in 2019 Ieee International Conference on Industrial Engineering and Engineering Management, Macao, China, 15-18 Dec. 2019, 104–108.
Shen, B., Ding, X., Chen, L., and Chan, H. L. (2017). Low Carbon Supply Chain with Energy Consumption Constraints: Case Studies from China's Textile Industry and Simple Analytical Model. Supply Chain Management-an Int. J. 22 (3), 258–269. doi:10.1108/scm-05-2015-0197
Shi, R., Cui, Y., and Zhao, M. (2021). Role of Low-Carbon Technology Innovation in Environmental Performance of Manufacturing: Evidence from OECD Countries. Environ. Sci. Pollut. Res. doi:10.1007/s11356-021-15057-0
Si, F., Yan, Z., Wang, J., and Dai, D. (2020). Dynamic Analysis of Duopoly Price Game Based on Low-Carbon Technology Sharing. Math. Probl. Eng. 2020, 1–18. doi:10.1155/2020/3832571
Song, M., Yang, M. X., Zeng, K. J., and Feng, W. (2020). Green Knowledge Sharing, Stakeholder Pressure, Absorptive Capacity, and Green Innovation: Evidence from Chinese Manufacturing Firms. Bus Strat Env 29 (3), 1517–1531. doi:10.1002/bse.2450
Vinholis, M., Saes, M., Carrer, M. J., and Filho, H. (2021). The Effect of Meso-Institutions on Adoption of Sustainable Agricultural Technology: A Case Study of the Brazilian Low Carbon Agriculture Plan. J. Clean. Prod. 280 (5), 124334. doi:10.1016/j.jclepro.2020.124334
Wang, D., Wang, T., and Zhang, B. (2019a). Reduce Emissions in Cooperation and Government Subsidies Strategies in Supply Chain Based on Differential Game. Control. Decis. 34 (8), 1733–1744.
Wang, M., Li, Y., Li, M., Shi, W., and Quan, S. (2019b). Will Carbon Tax Affect the Strategy and Performance of Low-Carbon Technology Sharing between Enterprises? J. Clean. Prod. 210, 724–737. doi:10.1016/j.jclepro.2018.10.321
Wang, M., Liu, Y., Shi, W., Li, M., and Zhong, C. (2019c). Research on Technology Remote Synergic Sharing Strategy of Low Carbon under the ETS in China. Syst. Engineering-Theory Pract. 39 (6), 1419–1434.
Wang, Z., Deng, X., Bai, Y., Chen, J., and Zheng, W. (2016). Land Use Structure and Emission Intensity at Regional Scale: A Case Study at the Middle Reach of the Heihe River basin. Appl. Energ. 183, 1581–1593. doi:10.1016/j.apenergy.2016.09.109
Wei, X., Qiu, R., Liang, Y., Liao, Q., Klemeš, J. J., Xue, J., et al. (2022). Roadmap to Carbon Emissions Neutral Industrial Parks: Energy, Economic and Environmental Analysis. Energy 238, 121732. doi:10.1016/j.energy.2021.121732
Xia, W., Li, B., and Yin, S. (2020). A Prescription for Urban Sustainability Transitions in China: Innovative Partner Selection Management of Green Building Materials Industry in an Integrated Supply Chain. Sustainability 12 (7), 2581. doi:10.3390/su12072581
Yang, Z., and Lin, Y. (2020). The Effects of Supply Chain Collaboration on green Innovation performance:An Interpretive Structural Modeling Analysis. Sustain. Prod. Consumption 23, 1–10. doi:10.1016/j.spc.2020.03.010
Yin, S., and Li, B. (2018). A Stochastic Differential Game of Low Carbon Technology Sharing in Collaborative Innovation System of superior Enterprises and Inferior Enterprises under Uncertain Environment. Open Maths. 16, 607–622. doi:10.1515/math-2018-0056
You, D., and Zhu, G. (2016). Differential Game Analysis of Ecological R&D, Cooperative Promotion and Pricing in the Low-Carbon Supply Chain. Control. Decis. 31 (6), 1047–1056.
Yu, X., Lan, Y., and Zhao, R. (2021a). Strategic green Technology Innovation in a Two-Stage alliance: Vertical Collaboration or Co-development? Omega-International J. Manage. Sci. 98, 102116. doi:10.1016/j.omega.2019.102116
Yu, Z., and Khan, S. A. R. (2021). Green Supply Chain Network Optimization under Random and Fuzzy Environment. Int. J. Fuzzy Syst. doi:10.1007/s40815-020-00979-7
Yu, Z., Khan, S. A. R., and Umar, M. (2021b). Circular Economy Practices and Industry 4.0 Technologies: A Strategic Move of Automobile Industry. Bus Strat Env. doi:10.1002/bse.2918
Zhai, J., Xu, X., Xu, J., and Lyu, X. (2021). Research on Green Collaborative Innovation Mechanism of Cloud Manufacturing Enterprises under Government Supervision. Math. Probl. Eng. 2021, 1–17. doi:10.1155/2021/8820791
Zhang, M., and He, W. (2018). “Research on Collaborative Innovation Mechanism of Green Construction Supply Chain Based on United Agency,” in AIP Conference Proceedings 1971, 020015. doi:10.1063/1.5041110
Zhang, S., Yu, Y., Zhu, Q., Qiu, C. M., and Tian, A. (2020). Green Innovation Mode under Carbon Tax and Innovation Subsidy: An Evolutionary Game Analysis for Portfolio Policies. Sustainability 12 (4), 1385. doi:10.3390/su12041385
Zhang, X., Geng, Y., Tong, Y. W., Kua, H. W., Tian, X., Wu, R., et al. (2021). Spatial Characteristics and its Driving Factors of Low-Carbon Energy Technology Innovation in China: A Gravity Movement and Exploratory Spatial Data Analysis. J. Clean. Prod. 295, 126481. doi:10.1016/j.jclepro.2021.126481
Zhang, Z., Gong, B., Tang, J., Liu, Z., and Zheng, X. (2019). The Joint Dynamic green Innovation and Pricing Strategies for a Hybrid System of Manufacturing and Remanufacturing with Carbon Emission Constraints. Kybernetes 48 (8), 1699–1730. doi:10.1108/k-06-2018-0339
Zhao, D., Yuan, B., and Xu, C. (2014). Dynamic Optimization about Vertical Cooperation on Carbon Emissions Reduction in Low-Carbon Supply Chain. Control. Decis. 29 (7), 1340–1344.
Zheng, C. (2021). Evolutionary Game Analysis of Knowledge Sharing in Low-Carbon Innovation Network. Complexity 2021, 1–11. doi:10.1155/2021/9995344
Zhou, K., and Ren, T. (2021). Low-carbon Technology Collaborative Innovation in Industrial Cluster with Social Exclusion: An Evolutionary Game Theory Perspective. Chaos 31 (3), 033124. doi:10.1063/5.0037956
Zhou, M., Govindan, K., and Xie, X. (2020). How Fairness Perceptions, Embeddedness, and Knowledge Sharing Drive green Innovation in Sustainable Supply Chains: An Equity Theory and Network Perspective to Achieve Sustainable Development Goals. J. Clean. Prod. 260, 120950. doi:10.1016/j.jclepro.2020.120950
Keywords: low carbon technology, knowledge sharing, collaborative innovation, differential game, emission reduction benefits
Citation: Wang F, Li H, Cao Y, Zhang C and Ran Y (2022) Knowledge Sharing Strategy and Emission Reduction Benefits of Low Carbon Technology Collaborative Innovation in the Green Supply Chain. Front. Environ. Sci. 9:783835. doi: 10.3389/fenvs.2021.783835
Received: 27 September 2021; Accepted: 27 December 2021;
Published: 14 January 2022.
Edited by:
Muhammad Mohsin, Hunan University of Humanities, Science and Technology, ChinaReviewed by:
Daniel Balsalobre-Lorente, University of Castilla-La Mancha, SpainCopyright © 2022 Wang, Li, Cao, Zhang and Ran. This is an open-access article distributed under the terms of the Creative Commons Attribution License (CC BY). The use, distribution or reproduction in other forums is permitted, provided the original author(s) and the copyright owner(s) are credited and that the original publication in this journal is cited, in accordance with accepted academic practice. No use, distribution or reproduction is permitted which does not comply with these terms.
*Correspondence: Huimin Li, bGlodWltaW4zNjQ2QDE2My5jb20=
Disclaimer: All claims expressed in this article are solely those of the authors and do not necessarily represent those of their affiliated organizations, or those of the publisher, the editors and the reviewers. Any product that may be evaluated in this article or claim that may be made by its manufacturer is not guaranteed or endorsed by the publisher.
Research integrity at Frontiers
Learn more about the work of our research integrity team to safeguard the quality of each article we publish.