- 1State Key Joint Laboratory of Environmental Simulation and Pollution Control, School of Environment, Tsinghua University, Beijing, China
- 2Department of Microbiology and Plant Biology, Institute for Environmental Genomics, University of Oklahoma, Norman, OK, United States
- 3School of Civil Engineering and Environmental Sciences, University of Oklahoma, Norman, OK, United States
- 4Earth and Environmental Sciences, Lawrence Berkeley National Laboratory, Berkeley, CA, United States
- 5Institute of Environment and Ecology, Tsinghua Shenzhen International Graduate School, Shenzhen, China
High concentrations of antibiotics in antibiotic production wastewater can cause the widespread transmission of antibiotic resistance genes (ARGs). Here, we collected a set of time series samples from a cephalosporin production wastewater treatment plant (X-WWTP), the subsequent municipal WWTP (Y-WWTP) and the receiving stream. Using a functional gene microarray, GeoChip 5.0, which contains multiple homologous probes for 18 ARG and 13 antibiotic metabolism gene (AMG) families, we found that more than 50% of homologous probes for 20 gene families showed a relative abundance higher in X-WWTP, while only 10–20% showed lower relative abundance. The different response patterns of homologous ARG (hARGs) within the same ARG family imply environmental selection pressures are only responsible for the ARG enrichment and spread of some specific instead of all ARG-containing microorganisms, which contradicted the traditionally held belief that environmental selection pressures, especially antibiotic concentration, select for all ARG-containing microorganisms thereby selecting different hARGs in the same ARG family in an undifferentiated way. Network results imply that hARGs from three β_lactamase families enriched under the selection pressure of high cephalosporin antibiotic concentrations in X-WWTP formed positively correlated homologous ARG clusters (pohARGCs). The pohARGCs were also enhanced in the sediment of the receiving stream. The enrichment of hARGs from three β_lactamase families was likely through microorganisms belonging to the Betaproteobacteria genus.
Introduction
The biological toxicity and bacterial antibiotic resistance of antibiotic production wastewater is increasingly causing environmental issues (Arslan-Alaton and Caglayan, 2006; Guo N. et al., 2018; Hou et al., 2019; Klavarioti et al., 2009; Zhang et al., 2013). Antibiotic production wastewater contains high concentrations of organic compounds, such as heterocyclic compounds and active pharmaceutical intermediates, with substantial biological toxicity (Arslan-Alaton and Caglayan, 2006; Klavarioti et al., 2009; Wei et al., 2019). Pharmaceutically active intermediates, including antibiotic residues, and antibiotic resistant bacteria (ARB) in antibiotic containing pharmaceutical wastewater can intensify bacterial antibiotic resistance (N. Guo et al., 2018a; Hou et al., 2019; Y. Zhang et al., 2013). As a key indicator reflecting the risk of antibiotic resistance, antibiotic resistance genes (ARGs) have been frequently detected in various environmental media (Martínez, 2008; Allen et al., 2010; Che et al., 2019). The fate and removal of ARGs and factors influencing these processes have been the focus of recent research (Chen et al., 2015; Guo et al., 2017; Pallares-Vega et al., 2019).
In China, the United States, and many other countries, antibiotic production wastewater is treated at specialized antibiotic production wastewater treatment plants (WWTPs) to remove antibiotics and then at subsequent municipal WWTPs to reduce conventional biochemical indicators such as BOD, TN and TP in the wastewater before discharging the effluent to natural water bodies (SEPA, 2008; Klavarioti et al., 2009). Unlike conventional biochemical indicators, regulations have not been established for the amount of allowable ARBs or ARGs in WWTP effluent (Chokshi et al., 2019; Hobæk and Lie, 2019). Although it is known that antibiotic production WWTPs reduce the total number of ARB and ARGs in the effluent wastewater (Jäger et al., 2018; Faleye et al., 2019), how this reduction influences downstream microbial populations at subsequent WWTPs and in the receiving waters is not well researched.
Most studies of ARGs are based on the abundance of ARG subtypes or ARG families. For example, Alcock et al. used ARG families to classify ARGs in the Comprehensive Antibiotic Resistance Database (Alcock et al., 2020) and Yin et al. used ARG subtypes to classify ARGs in the Integrated Structured ARG-database (Yin et al., 2018). According to ARG classifications among the GeneBank Database (https://www.ncbi.nlm.nih.gov/genbank/), the Comprehensive Antibiotic Resistance Database (Alcock et al., 2020) and the Integrated Structured ARG-database (Yin et al., 2018), Gene families are groups of related genes that share a common ancestor. Members of gene families may be paralogs or orthologs. Paralogs are genes with similar sequences in the same species while orthologs are genes with similar sequences in different species (Ohta, 2001; Demuth and Hahn, 2009). Resistance “types” represent the class of antibiotics to which a given ARG confers resistance, and resistance “subtypes” represent individual ARGs that share a common ancestor (Yang et al., 2016). ARG subtype and ARG family are the same concept for ARGs. Sequences from the same ARG family in different hosts are called homologous ARGs (hARGs) and include orthologous ARGs and paralogous ARGs and are not always identical (Michael, 2005). The abundance of different hARGs presented different response patterns depending on their hosts (Brochier et al., 2004), necessitating the need to examine individual hARGs rather than ARG families.
In this study, we examined samples from a cephalosporin antibiotic production WWTP, the subsequent municipal WWTP, and the receiving water bodies. Statistical analysis of the microbial community composition and functional genes including hARGs were performed to find the types, pathways and environmental influence of the ARGs enriched in the antibiotic production WWTP.
Materials and Methods
Study Site Description
This study examined two WWTPs located in a pharmaceutical industrial park in Hebei Province, China. The industrial park contains more than 20 facilities that manufacture a variety of antibiotics, including amoxicillin, penicillin, ampicillin, cephalosporin, lincomycin, clindamycin, streptomycin, gentamicin, avermectin, ivermectin, doxycycline, neomycin, and minocycline, among others. The cephalosporin antibiotic production facility has a specialized WWTP (X-WWTP) to treat antibiotic production wastewater. The effluent from X-WWTP is then transported to a dedicated municipal WWTP (Y-WWTP) (approximately 1.5 km distance away) that treats effluent from all the specialized pharmaceutical production WWTPs as well as sewage within the industrial park (Figure 1). Additional information on the WWTPs is shown in Table 1.
X-WWTP mostly accepts cephalosporin antibiotic production wastewater, including 7-aminocephalosporanic acid, cefuroxime (CXM), ceftriaxone (CTR), and other cephalosporins. X-WWTP effluent comprises a majority of Y-WWTP influent (80%). Effluent from Y-WWTP is subject to the Grade A discharge standard of Pollutants for Municipal Wastewater Treatment Plant (GB18918-2002). After discharge, Y-WWTP effluent is transported to a receiving stream through a culvert. The upstream section of the receiving stream is a dry stream bed, so the main water source for the receiving stream is the effluent from Y-WWTP. Our study focused on the influent and effluent of X- and Y-WWTPs and the receiving stream.
Sample Collection and Pretreatment
Wastewater samples were collected in December 2015, January 2016, February 2016, March 2016, April 2016, July 2016, October 2016 and January 2017. Sampling from X-WWTP and Y-WWTP and the receiving stream were performed on the same day. At X-WWTP, samples were collected from the influent of the hydrolysis acidification tank (Xinf) and the effluent of the secondary sedimentation tank (Xeff). At Y-WWTP, samples were collected from the influent of the regulation tank (Yinf) and the final effluent (Yeff) of Y-WWTP. Three wastewater samples were collected from each sampling site at 8 h intervals into 2 L sterile bottles at each sampling time. The triplicate samples were combined by mixing equal volumes of each replicate into one sample. Samples were transported back to the laboratory within 2 h using an insulated case with a built-in ice pack.
River water samples were collected in December 2015, January 2016, February 2016 and March 2016. Samples were collected at 0.2 km (Ra), 1 km (Rb), 3.5 km (Rc), 4.5 km (Rd) and 6.5 km (Re) distance from the effluent culvert of Y-WWTP in the receiving stream and were labeled a, b, c, d and e, respectively. At each sampling location, 2 L of water samples were collected from three points in the stream, approximately 0.5 m apart and 0.5 m below the surface with a water collector. The three samples were then pooled to make one water sample.
Sediment samples were collected in December 2015, January 2016, March 2016, April 2016, July 2016, October 2016 and January 2017. Sediment samples were collected at 0.2 km (SD1), 1 km (SD2), 3.5 km (SD3) and 4.5 km (SD4) away from the effluent culvert of Y-WWTP in the receiving stream. The grab-type sediment collector (HAD-QNC6-1, Hengde Instrument Co., Ltd., Beijing) was used to collect the sediment samples. At each sampling location, sediment samples were collected from three points in the stream, approximately 0.5 m apart. The sediment was collected using a quartet sampling method. An area (20 × 20 cm) was selected at each sampling location and then divided into quadrents by connecting the diagonal lines. Sediment samples were collected from each quadrent and then mixed to make one sediment sample.
After being transported to the laboratory, the 6 L wastewater and stream water samples were divided and 2 L was stored at 4°C for immediate physical and chemical indicator analysis. The remaining 4 L of each sample was suction filtered immediately using 0.22-μm-pore-size filter membranes (GSWP04700 filters, Millipore, United States). The filter membranes were stored at −80°C for DNA extraction. The sediment samples were stored at 4°C for immediate physical and chemical indicator analysis. At the same time, a portion of the sediment samples were centrifuged (15000 r/min) for 10 min and the supernatant was discarded. The sediment pellets were stored at −80°C for DNA extraction. All physical and chemical analyses were completed within 24 h.
The sampling date is designated by a four digit number indicating the year and month. The number “_1, _2, and _3” after sampling date meant parallel samples. For example, Xinf16.01 refers to the influent sample of X-WWTP, which was sampled in January 2016.
DNA Extraction, Purification, and Quantification
DNA was extracted from the filtered membrane using a HiPure Water DNA Kit (D3145-02, Magen, again, city name, province name, China) following the manufacturer’s instructions and cleaned by ethanol purification. DNA quality was assessed based on absorbance ratios [260–280 nm (>1.8); 260 to 230 nm (>1.7)] detected by a NanoDrop 2000 (ThermoFisher, America). Qualified DNA samples were stored at −80°C. Samples that did not reach the quality cutoff were not used.
PCR Amplifiation, Sequencing, and Data Processing
A two-step PCR amplification method was used for PCR product library preparation as described previously (Zhou et al., 2011). Standard primers were used to amplify the V4 region of prokaryotic 16S rRNA genes [515F (5′-GTGCCAGCMGCCGCGGTAA-3′) and 806R (5′-GGACTACHVGGGTWTCTAAT-3′)]. Sample libraries were then sequenced on an Illumina MiSeq (San Diego, CA, United States) as described previously (X. Guo et al., 2020).
16S rRNA gene sequencing data generated from MiSeq were processed by demultiplexing, triming primers, combining paired-end reads, removing sequences containing N’s, and detecting and removing chimeras using a Galaxy-based pipeline http://zhoulab5.rccc.ou.edu:8080 (Zhou et al., 2016). OTUs were generated by QIIME II with a 97% similarity threshold. The Sliva (http://www.arb-silva.de/) 16S reference database was used (Quast et al., 2012). OTUs were identified taxonomically using the QIIME II classifier (Bolyen et al., 2019). Only OTUs with greater than two reads were retained as valid data.
Functional Structure Analysis With GeoChip 5.0
The latest generation of functional gene array, GeoChip 5.0 (180K) (Zhou et al., 2015), was used to detect ARGs in the samples. GeoChip 5.0 contains 167,044 probes targeting 395,894 coding sequences from 1,593 functional gene families. A total of 15,474 probes on the GeoChip 5 are associated with antibiotic resistance, accounting for approximately 10% of the total number of gene probes. Each probe in GeoChip has its own microbial annotation which can be found at www.ou.edu/ieg. The source of the microbial annotation is the Genebank information in the NCBI database. Additional information is shown in Table 2.
In our study, 500 ng of DNA from each sample were hybridized to GeoChip at the Institute for Environmental Genomics, University of Oklahoma, as described previously (X. Guo et al., 2020). The microarray data was preprocessed using the microarray data manager pipeline on the Institute for Environmental Genomics’ website (www.ou.edu/ieg/tools/data-analysis-pipeline) as described previously (He et al., 2010; Tu et al., 2014).
Statistical Analyses
Alpha diversity analysis, beta diversity analysis, core microbiome analysis, and linear discriminant analysis effect Size (LEfSe), which is used to find the gene or functional characteristics that can best explain the differences between groups in two or more groups of samples under different biological conditions or environments and the influence of these characteristics on the differences between groups, and correlation feature pattern search analysis of 16S rRNA genes in all samples were performed on https://www.microbiomeanalyst.ca/. The alpha diversity analysis used the fragrance index. Beta diversity analysis is principal co-ordinates analysis (PCoA) based on the Bray-Curtis index. Core microbiome analysis selected OTUs that appear in more than 50% of samples and have a relative abundance greater than 0.01%. The LEfSe analysis, which compared the 16S rRNA gene abundance profiles between samples in different state, had a p-value cutoff of 0.1 and a log LDA score of 2.0. Pattern Search analysis, which searched for a pattern based on correlation analysis on defined pattern, had a p-value cutoff of 0.05 in pearson relevance.
Before GeoChip data statistical analysis, logarithmic transformation was carried out on the original data, then transferred into relative abundances. Probes detected in at least 6 of 29 samples were retained as valid data. The GeoChip data analysis of all samples was completed in R. We summarized all gene categories of each sample and analyzed the alpha, beta diversity, response ratio and abundance variation ratio analysis at the levels of ARG and AMG probes. The alpha diversity analysis used the fragrance Index. Detrended Correspondence Analysis (DCA) based on the Bray-Curtis index was used for beta diversity analysis. The response ratio of ARGs was calculated using the formula described previously (Y. Luo et al., 2006). The total abundance of all probes in every ARG family was taken for response ratio analysis. A response ratio is considered significant when p < 0.05. Network analysis of hARGs was performed using the Molecular Ecological Network Analysis platform at http://ieg4.rccc.ou.edu/mena.
Results
Microbial Community in Wastewater of WWTPs
16S rRNA gene sequencing was performed on 31 wastewater samples from X-WWTP and Y-WWTP and 18 stream water samples from the receiving stream. A total of 1,699,901 effective reads and 2,260 OTUs were obtained from wastewater and stream water samples. The maximum number of effective reads per sample was 86,766 and the minimum was 14,222. The microbial diversity of X-WWTP wastewater was lower than that in Y-WWTP (Figure 2A). The microbial diversity of X-WWTP effluent was higher than that of the influent, while there was no difference in the microbial diversity between the influent and effluent of Y-WWTP. The microbial community from the influent in X-WWTP was distinguisable from all other wastewater samples (PCoA, Figure 2B). Samples did not separate based on time, indicating that there was no variation in the wastewater microbial community over the time period examined.
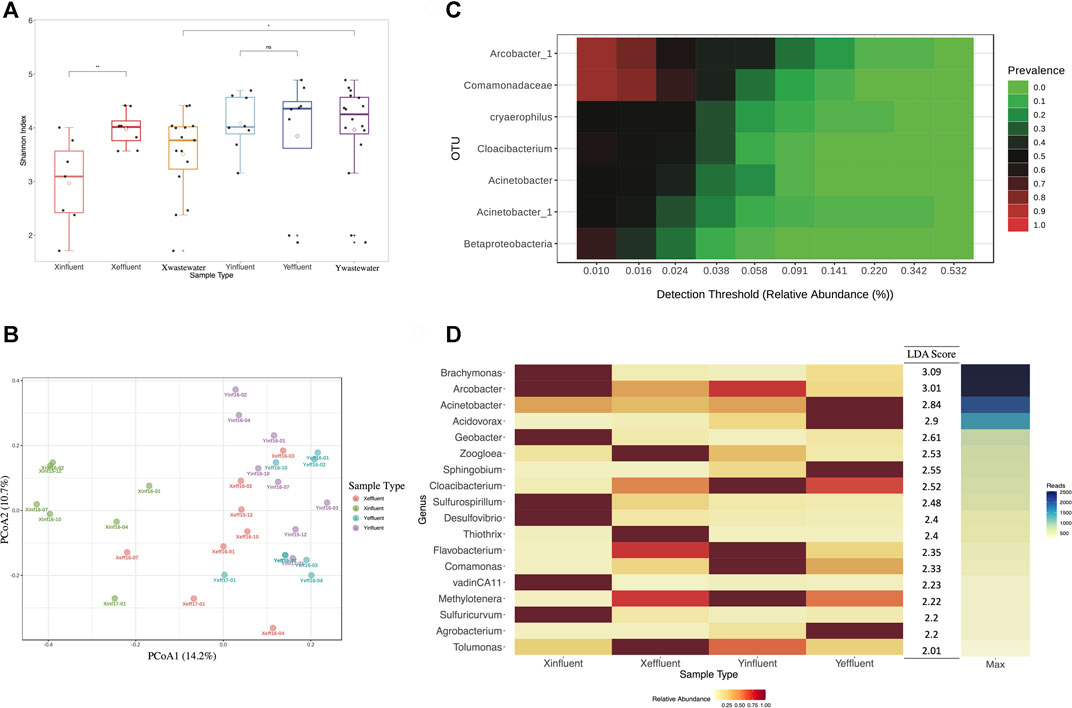
FIGURE 2. Microbial community composition analysis of wastewater samples at OTU level. (A) SHDI of wastewater samples. (B) PCoA of wastewater samples based on Bray-Curtis Index. (C) Core Microbiome analysis of wastewater samples. (D) LEfSe analysis of wastewater samples.
A total of 7 OTUs from all the wastewater samples were identified as members of the core microbiome (Figure 2C). OTUs from the Acinetobacter, Cloacibacterium and Arcobacter genera, which can cause human diarrhea, were common in wastewater from two WWTPs. LEfSe analysis of all wastewater samples selected 18 differentiated genera that best explained the differences among X-WWTP influent, X-WWTP effluent, Y-WWTP influent and Y-WWTP effluent (Figure 2D). OTUs belonging to 10 genera, including Sulfurospirillum, Desulfovibrio_1, kujiense, and Methanomethylovorans, were more abundant in X-WWTP, while OTUs belonging to 8 genera, including Acidovorax, and Comamonas_1, Sphingobium, were more abundant in Y-WWTP. In X-WWTP influent, seven of the 18 genera were in lowest abundance while 10 were in highest abundance. Most of these microorganisms were in low abundance in the effluent of Y-WWTP.
Microbial Community Composition in Stream Water and Sediment
16S rRNA gene sequencing was performed on 32 sediment samples from the receiving stream. A total of 1,545,069 effective reads and 2,095 OTUs were obtained. The maximum number of effective reads per sample was 83,531; the minimum number was 22,039. The bacterial diversity of the river water samples (Figure 3A) and river sediment samples (Figure 3C) indicated that the bacterial diversity of the river water at point a was close to the wastewater, since point a was where the WWTP effluent discharged into the stream. The bacterial diversity of the river water decreased downstream and then began to increase further downstream, while the bacterial diversity of the sediment was relatively similar from points a to c and then decreased sharply at point d. Core microbiome analysis of the stream water (Figure 3B) and sediment samples (Figure 3D) showed that only one OTU, belonging to the Betaproteobacteria class, was observed to occur frequently in each sample. PCoA of OTUs from all sediment samples indicated microbial communities from sediment at different sampling positions were different, except those between Points b and c. Pattern search analysis (Figure 3F) searched 25 differentiated OTUs based on person correlation analysis on among sediment samples from different positions. The results of Pattern Search analysis showed that the relative abundance of the top 14 genera, including Sulfurimonas, kujiense, and syntrophus_1, increased from point a to d, while 11 genera, including SB_1, Sinobacteraceae_1, and Flavobacteriaceae_1, decreased from point a to d.
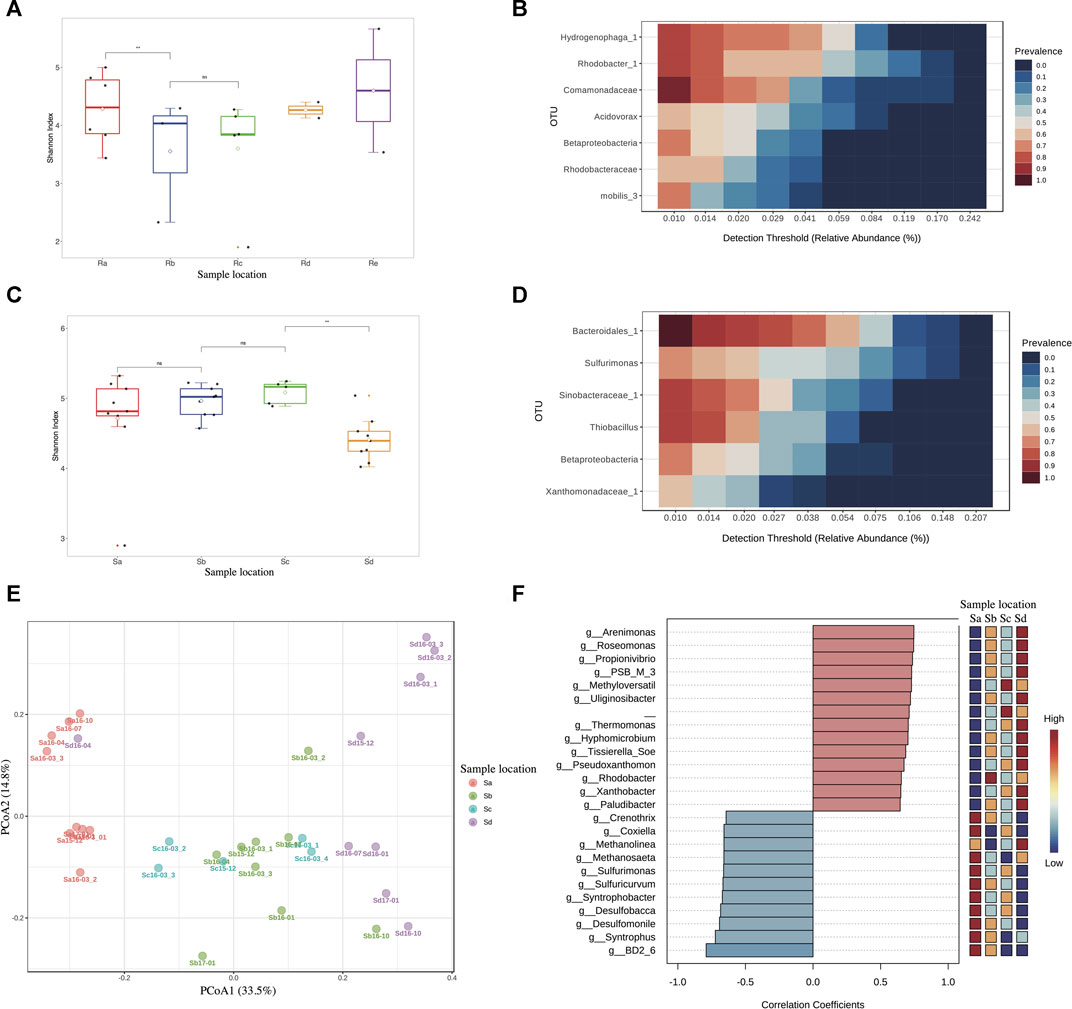
FIGURE 3. Microbial community composition of stream water and sediment samples at OTU level. (A) SHDI of stream water samples. (B) Core Microbiome analysis of stream water samples. (C) SHDI of sediment samples. (D) Core Microbiome analysis of sediment samples. (E) PCoA of sediment samples based on Bray-Curtis Index. (F) Pattern Search analysis of sediment samples.
ARGs and AMGs in Two WWTPs
To determine which ARGs were present in the samples, GeoChip 5.0 analysis was performed on 25 wastewater samples from the two WWTPs, 2 sludge samples from Y-WWTP, 4 stream water samples and 27 stream sediment samples. A total of 80,166 functional genes represeting 1,110 gene families, 15 functional groups, and 129 subgroups were detected.
The relative abundances of ARGs (Figure 4A) and antibiotic synthesis genes (Figure 4B) were quantified at the gene family level in each wastewater sample. The total relative abundance of 18 types of ARGs in all samples was about 12%, and the total relative abundance of AMGs was about 0.32%. The total abundance of ARGs and AMGs in X-WWTP wastewater samples was significantly (p < 0.01) higher than that in Y-WWTP wastewater and the stream water samples. The DCA (Figure 4C) of all wastewater and stream water samples at the probe level indicated X-WWTP samples were separated from Y-WWTP and stream samples. None of the samples showed significant seasonal variation. The response ratio analysis of ARGs and AMGs from the two WWTPs (Figure 4D) indicated a greater relative abundance of Mex, MFS_antibiotic, β_lactamase_C, ABC_antibiotic_transporter, β_lactamase_A, tetx_resistance, pcbC, and lmbA genes in X-WWTP than in Y-WWTP, while the relative abundance of ABC_multidrug_fungi, β_lactamase_C, fosb, qnr, fosa, and fosx was richer in Y-WWTP.
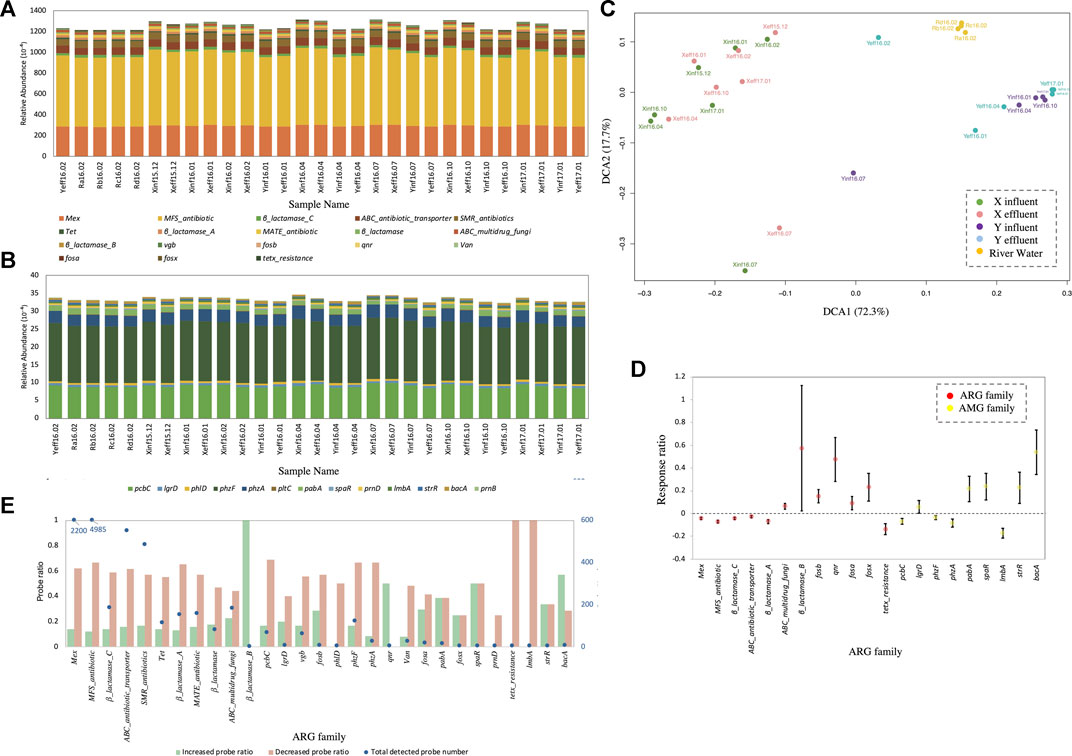
FIGURE 4. ARGs and AMGs of wastewater samples. (A) Relative abundance of ARGs at the gene family level. (B) Relative abundance of AMGs at the gene family level. (C) DCA at the Probe level. (D) Response ratio analysis on the gene family level of ARGs and AMGs in Y-WWTP compared with X-WWTP. (E) Probe quantities detected in each gene family and ratio of probes which increased or decreased in relative abundance detected in Y-WWTP compared with those detected in X-WWTP.
Most ARG families have many different probes which stand for different hARGs, and each hARG has its own host bacteria according to the Genebank imformation. Figure 4E shows the details of each ARGs family at the probe level, including the number of probes detected in each gene family and ratio of probes which increased or decreased in relative abundance detected in Y-WWTP compared with those detected in X-WWTP. More than 50% of probes from 22 ARG families, including Mex, MFS_antibiotic and β_lactamase_C, showed relative decreases, while only 10–20% of the probes showed relative increases. Five ARG families had a higher ratio of probes with relative increases, but each of these ARG families had less than 3 probes.
Network Analysis of hARGs
Network analysis of β_lactamase_A (Figure 5A), β_lactamase_C (Figure 5B) and β_lactamase (Figure 5C) hARG families from the WWTP wastewater samples showed correlations among the hARGs of these families in the β_lactamase_B was not included since it only had one hARG. Each β_lactamase ARG family had one cluster in which the hARGs were positively correlated (indicated by green linkages in Figure 5). In contrast, a proportion of hARGs that were negatively correlated (indicated by red linkages in Figure 5) with the hARGs in the positively correlated homologous ARG cluster (pohARGC). We selected seven representative hARGs from the four β-lactamase families to compare the relative abundance (Figure 5D). GB171318562, GB260648368, and GB 337746682 represented the hARGs in the pohARGCs of β_lactamase_A, β_lactamase_C, and β_lactamase ARG families and had higher abundance in X-WWTP. GB133919281, GB303325478, GB170695665, and GB315647229 represented the hARGs that were either negatively or not correlated with the pohARGCs of β_lactamase_A, β_lactamase_B, β_lactamase_C, and β_lactamase ARG families and had higher abundance in Y-WWTP.
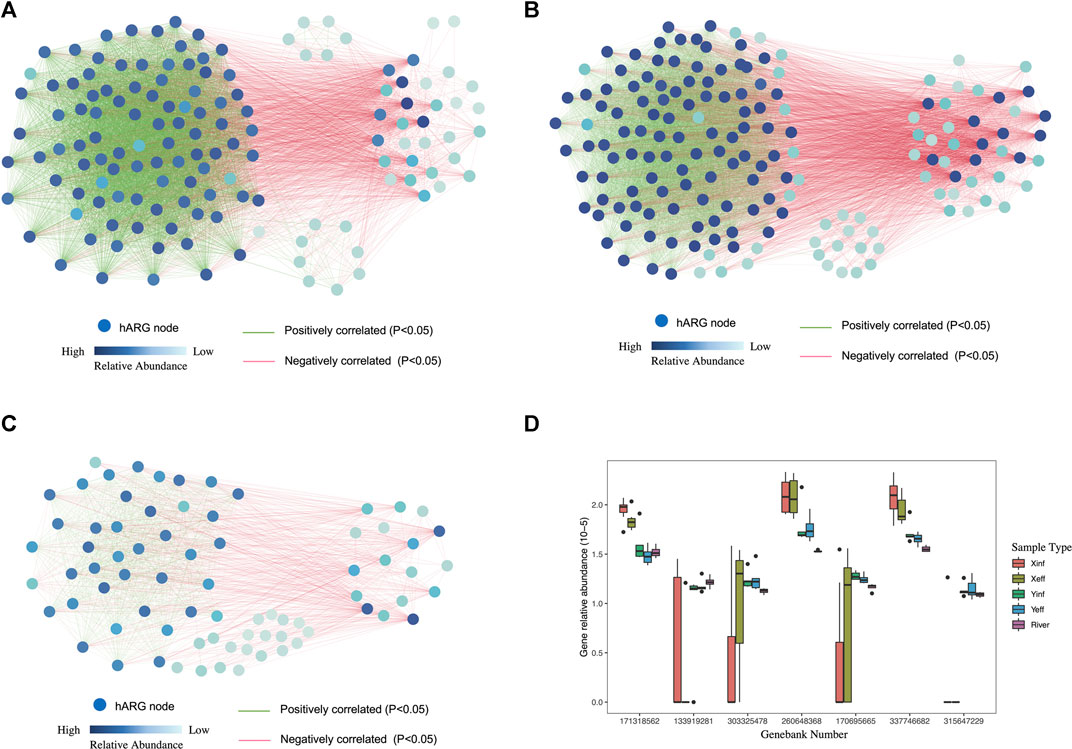
FIGURE 5. Network analysis of homologous genes of the (A) β_lactamase_A, (B) β_lactamase_C and (C) β_lactamase ARG families in wastewater samples. (D) Relative abundance of representative homologous genes of four β_lactame ARG families in wastewater and river samples.
ARGs and AMGs in Sediment
The relative abundance of ARGs (Figure 6A) and AMGs (Figure 6B) were also calculated at the gene family level in stream water and sediment samples. The total relative abundance of each gradually increased in stream sediment over time, but no dicernible variation was observed with distance from the Y-WWTP effluent discharge. The results of the DCA (Figure 6C) indicated a seasonal variation across the secondary axis for the sediment samples, but it only explained 11.10% of the observed variation. Therefore, seasonal variation was not a major factor in the diversity variation. No changes with increasing distance were observed.
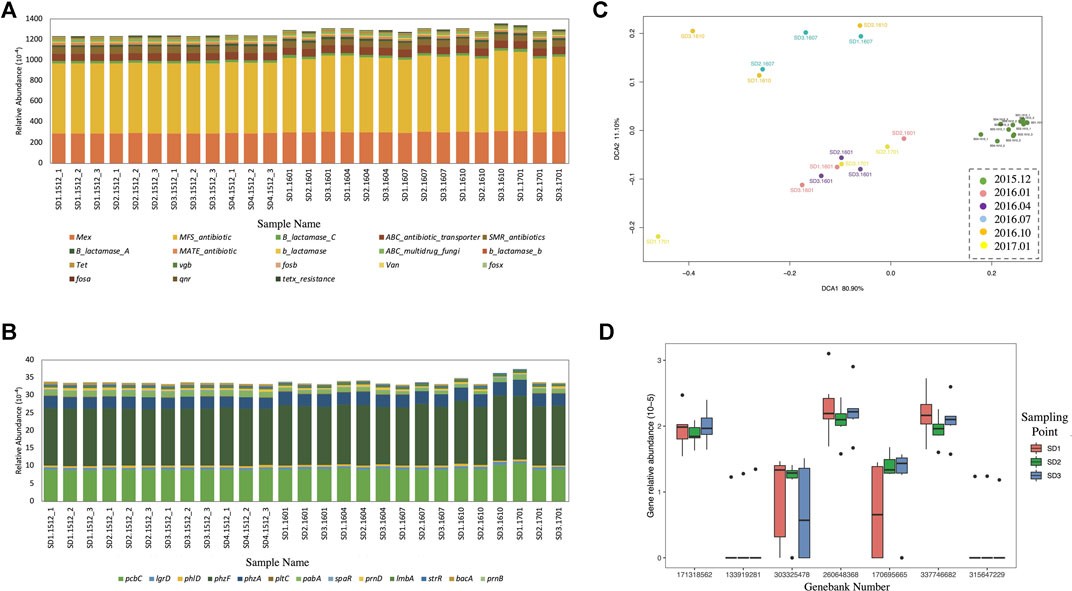
FIGURE 6. ARGs and AMGs of sediment samples. (A) Relative abundance of ARGs at the gene family level. (B) Relative abundance of AMGs at the gene family level. (C) DCA at the Probe level. (D) Relative abundance of representative homologous genes of four β_lactame ARG families.
We also selected representative hARGs from the four β-lactamase families in sediments to compare the relative abundance, with only one homologous ARG for β_lactamase_B (Figure 6D). GB171318562, GB 260648368, and GB 337746682 were abundant in all sediment samples, while GB 133919281, GB 303325478, GB 170695665, and GB 315647229 had little or no abundance in sediment samples.
Discussion
Previous studies have often examined pharmaceutical manufacturing or municipal WWTPs in isolation (X. Guo et al., 2018b; Tong et al., 2019; Wang et al., 2015). However, the continuous wastewater flow from pharmaceutical manufacturing WWTP to municipal WWTP and then into the local receiving streams has been rarely addressed in previous studies. This study revealed some essential variation in community responses and ARGs in this particular situation. Since the ARGs we detected were intracellular ARGs (Yuan et al., 2019), this study examined the variation in microbial community structure first.
In this study, we observed that the microbial diversity of X-WWTP was significantly (p < 0.01) lower than that of Y-WWTP, and that the X-WWTP influent had the lowest microbial diversity of all sampling sites. In a study of activated sludge from 12 full-scale pharmaceutical WWTPs, a low bacterial diversity was also observed for pharmaceutical production WWTPs compared to that of municipal WWTPs (F. Zhao et al., 2019a). High concentrations of organic compounds with strong biological toxicity, such as heterocyclic compounds and antibiotic active intermediates in antibiotic-containing pharmaceutical production wastewater, are the primary cause of lower microbial diversity in pharmaceutical production WWTPs (Arslan-Alaton and Caglayan, 2006; Klavarioti et al., 2009; Wei et al., 2019). β-diversity analysis (Figure 2B) showed that the X-WWTP influent microbial community was unique, while the X-WWTP effluent microbial community was more similar to that of the Y-WWTP wastewater (influent and effluent. The abundance of 21 OTUs in the X-WWTP effluent showed polarity, indicating that the treatment process in the X-WWTP had a significant remodeling effect on the microbial community. The OTUs that had extremely high abundance in X-WWTP effluent also appeared in the Y-WWTP influent, indicating that the microorganisms selected in X-WWTP were spreading to subsequent treatment process With the sewage flow.
The microbial communities in both the sediment and water of the receiving stream were initially influenced by the Y-WWTP effluent. The microbial communities from the water and sediment in the stream showed different trends in alpha diversity. Differences in the water community could be because of Y-WWTP effluent discharge with the oxidation, reduction and sunlight decomposition of pollutants and the sedimentation of pollutants and microorganisms of streams (Li et al., 2010; Price et al., 2018; Zhang et al., 2016). The Y-WWTP effluent discharged to the receiving stream had both a large number of conventional biochemical contaminants and a complex microbial community, which determined both the physiochemical and microbiological composition of the receiving streams (Price et al., 2018). The microbial diversity in the water decreased around 1 km downstream from the discharge site, indicating the microorganisms that had adapted to the environment in Y-WWTP gradually disappeared as the distance from the Y-WWTP increased because the stream environment was very different from Y-WWTP environment. Microbial diversity began to increase around 4.5 km downstream from the discharge site, reflecting a change to a more natural state in which the microorganisms had adapted to the stream environment.
Microbial communities in the sediment of receiving streams were previously found to be influenced by the effluent of WWTP discharge and stream water composition (Milaković et al., 2019). From the point of discharge to around 3.5 km downstream, the microbial diversity of the sediment remained relatively stable because most of the microorganisms in the stream water were still adapted to the Y-WWTP environment. The microbial diversity of the sediment at point d (around 4.5 km distant from the discharge point) was lowest because the microorganisms had adapted to the new environment and the phychemical properties of the stream water at this point were very different from the Y-WWTP effluent. So, the Y-WWTP effluent discharge no longer influenced the microbial community after 3.5–4.5 km downstream.
While the microbial community results of this study were similar to some previous studies, the results of the ARG and homologous ARG studies disagreed with previous findings. ARG studies have been a hot topic in the environmental field in recent years, but almost all of them focused on the abundance of ARG subtypes (J. Guo et al., 2017; F. Zhao et al., 2019a; Zhao et al., 2018). For example, qPCR provides information on the abundance of representative sequences of particular ARG subtypes (Auerbach et al., 2007; Czekalski et al., 2014), while metagenomics provides information on the abundance of all representative sequences of all ARG subtypes (Ju et al., 2019; Zhao F. et al., 2019). A limitation of these previous studies is that ARGs belonging to the same subtype (hARGs) may exhibit different responses because they are in different microorganisms (Brochier et al., 2004; Michael, 2005; Alcock et al., 2020). Here we investigated the response patterns of individual hARGs using GeoChip 5.0.
The X-WWTP and Y-WWTP wastewater were very different in terms of ARGs. We analyzed the change regularity of ARGs detected by GeoChip 5.0 at three levels: the total relative abundance of ARGs, the relative abundance of each ARG family and the relative abundance of each hARG. The total relative abundance of ARGs in X-WWTP was significantly (p < 0.01) higher than in Y-WWTP, which is consistent with previous findings (X. Guo et al., 2018b; Wang et al., 2015). Although X-WWTP contained mainly cephalosporin, a beta-lactam antibiotic, production wastewater, X-WWTP had 8 of 18 ARG families with higher relative abundance than Y-WWTP, the relative abundance of β_lactamase_B was lower than that in X-WWTP. These unexpected results indicated that the relative abundance of ARG families did not truly reflect the response pattern of ARGs so we further analyzed individual ARGs from each family.
More than 50% of probes from 18 ARG families were more abundant in X-WWTP than in Y-WWTP, which indicated hARGs’ change regularity was different from the ARG families. In order to study the change regularity of the hARGs further, hARGs from three β_lactamase families were selected for network analysis. Each family had some members that formed a positively correlated hARG cluster (pohARGC) and some that were negatively correlated with the hARGs in pohARGC (Figures 5A–C). According to the abundance analysis of representive probes, pohARGCs were composed of the hARGs that were richer in X-WWTP. Since different hARGs belonging to the same ARG family often have different microbial annotations based on GeoChip, the reason for the enrichment of pohARGCs is probably due to the enrichment of strains that carry them in X-WWTP. In other words, it is inferred that the enrichment of specific strains in X-WWTP led to the enrichment of pohARGCs. This is consistent with the specificity of the microbial community in X-WWTP in the 16S rRNA gene sequencing analysis. According to the same reason, the hARGs that were negatively correlated or not correlated with the pohARGCs may be contained by the environmental strains or from strains that were enriched in domestic sewage conditions of Y-WWTP.
The hARGs from the same ARG family have different response patterns based on their homology, indicating that changes in ARG abundance are closely related to changes within the microbial community (Klümper et al., 2019; Luo et al., 2017; Wu et al., 2017). As such, previous studies that focused on ARG families as a whole or ARGs independent of the host microorganism may not provide accurate results because some hARGs from the same ARG family exhibited different response patterns due to changes of the host microorganism or due to horizontal transfer (Oliveira et al., 2017).
It was suggested that the horizontal transfer of ARGs in two WWTPs was frequently occurring, and the horizontal transfer of ARGs in X-WWTP was more frequent than Y-WWTP (Supplementary Figure S2), which indicated that some hARGs from the same ARG family exhibited different response patterns may be due to horizontal transfer. But we can’t compare the difference in contribution between changes of the host microorganism and horizontal transfer.
Correlation networks and Variance Partitioning Analysis have often been used to look for relationships between ARG families and environmental factors. However, these methods often show spurious or indirect correlations or suggest that changes in the bacterial communities due to environmental factors are comparable to ARGs response patterns, which is hard to explain (Carr et al., 2019; Wu et al., 2019). This study indicated that environmental selection pressures are only responsible for the ARG enrichment and spread of some specific instead of all ARG-containing microorganisms, which contradicted the traditionally held belief that environmental selection pressures, especially antibiotic concentration, select for all ARG-containing microorganisms and cause the same change pattern to hARGs from the same ARG family (Durão et al., 2018; Guo X. et al., 2018; Zhao R. et al., 2019). The reason why some ARG-containing microorganisms were not enriched under environmental selection pressures may be because the hARGs in their bodies were hard to expressed.
More importantly, the ARGs in the pohARGCs that were enriched under the antibiotic selection pressure in X-WWTP were abundant in the receiving stream sediments, while background ARGs or those enriched under domestic sewage conditions did not occur in the sediments. The enrichment of ARGs in the sediments causes long-term and long-distance hazards. The enrichment of hARGs of 3 β_lactamase families was likely through Betaproteobacteria, a member of the core microbiomes of both the wastewater and sediment, which is one of the most abundant and ubiquitous ARB genera in water environment (Suvorova and Gelfand, 2019; Petrovich et al., 2020). Other bacteria and horizontal transfer of ARGs could also contribute to this enrichment, but further study is needed to confirm this.
Conclusion
This study examined the microbial community structure and ARGs (especially hARGs) in wastewater from a cephalosporin antibiotic production WWTP (X-WWTP) and the subsequent municipal WWTP (Y-WWTP), and water and sediment from the receiving stream using GeoChip 5.0. We found that the hARGs of three β_lactamase ARG families enriched under the selection pressure of high antibiotic concentration in X-WWTP formed pohARGCs. The pohARGCs were also enhanced in the sediment of the receiving stream, suggesting that these enriched ARGs likely pose a more permanent public health risk. The enrichment of pohARGCs of three β_lactamase families was likely through Betaproteobacteria genus. The different response patterns of homologous ARG (hARGs) within the same ARG family imply environmental selection pressures are only responsible for the ARG enrichment and spread of some specific instead of all ARG-containing microorganisms. This study also represents a new gradation of ARG study, the results of which indicate examining individual hARGs can reveal a more nuanced response pattern of ARGs.
Data Availability Statement
DNA sequences of 16S rRNA gene were available in NCBI Sequence Read Archive under project PRJNA767527.
Author Contributions
LC, MZ, and JZ contributed to conception and design of the study. LC, MZ, and DN organized the experiments. LC performed the statistical analysis. LC wrote the first draft of the manuscript. All authors contributed to manuscript revision, read, and approved the submitted version.
Funding
This research was supported by the National Natural Science Foundation of China (No. 51478237).
Conflict of Interest
The authors declare that the research was conducted in the absence of any commercial or financial relationships that could be construed as a potential conflict of interest.
Publisher’s Note
All claims expressed in this article are solely those of the authors and do not necessarily represent those of their affiliated organizations, or those of the publisher, the editors and the reviewers. Any product that may be evaluated in this article, or claim that may be made by its manufacturer, is not guaranteed or endorsed by the publisher.
Supplementary Material
The Supplementary Material for this article can be found online at: https://www.frontiersin.org/articles/10.3389/fenvs.2021.783676/full#supplementary-material
SUPPLEMENTARY FIGURE S1 | Microbial community composition of stream water samples at OTU level. (A) PCoA of stream water samples based on Bray-Curtis Index. (B) Pattern Search analysis of stream water samples.
SUPPLEMENTARY FIGURE S2 | The abundance of gene transfer element (Int1) in different wastewater samples.
References
Alcock, B. P., Raphenya, A. R., Lau, T. T. Y., Tsang, K. K., Bouchard, M., Edalatmand, A., et al. (2020). CARD 2020: Antibiotic Resistome Surveillance with the Comprehensive Antibiotic Resistance Database. Nucleic Acids Res. 48 (D1), D517–D525. doi:10.1093/nar/gkz935
Allen, H. K., Donato, J., Wang, H. H., Cloud-Hansen, K. A., Davies, J., and Handelsman, J. (2010). Call of the Wild: Antibiotic Resistance Genes in Natural Environments. Nat. Rev. Microbiol. 8 (4), 251–259. doi:10.1038/nrmicro2312
Arslan-Alaton, I., and Caglayan, A. E. (2006). Toxicity and Biodegradability Assessment of Raw and Ozonated Procaine Penicillin G Formulation Effluent. Ecotoxicology Environ. Saf. 63 (1), 131–140. doi:10.1016/j.ecoenv.2005.02.014
Auerbach, E. A., Seyfried, E. E., and McMahon, K. D. (2007). Tetracycline Resistance Genes in Activated Sludge Wastewater Treatment Plants. Water Res. 41 (5), 1143–1151. doi:10.1016/j.watres.2006.11.045
Bolyen, E., Rideout, J. R., Dillon, M. R., Bokulich, N. A., Abnet, C. C., Al-Ghalith, G. A., et al. (2019). Reproducible, Interactive, Scalable and Extensible Microbiome Data Science Using QIIME 2. Nat. Biotechnol. 37 (8), 852–857. doi:10.1038/s41587-019-0209-9
Brochier, C., López-Garcı́a, P., and Moreira, D. (2004). Horizontal Gene Transfer and Archaeal Origin of Deoxyhypusine Synthase Homologous Genes in Bacteria. Gene 330, 169–176. doi:10.1016/j.gene.2004.01.018
Carr, A., Diener, C., Baliga, N. S., and Gibbons, S. M. (2019). Use and Abuse of Correlation Analyses in Microbial Ecology. ISME J. 13 (11), 2647–2655. doi:10.1038/s41396-019-0459-z
Che, Y., Xia, Y., Liu, L., Li, A.-D., Yang, Y., and Zhang, T. (2019). Mobile Antibiotic Resistome in Wastewater Treatment Plants Revealed by Nanopore Metagenomic Sequencing. Microbiome 7 (1), 44. doi:10.1186/s40168-019-0663-0
Chen, B., Liang, X., Nie, X., Huang, X., Zou, S., and Li, X. (2015). The Role of Class I Integrons in the Dissemination of Sulfonamide Resistance Genes in the Pearl River and Pearl River Estuary, South China. J. Hazard. Mater. 282, 61–67. doi:10.1016/j.jhazmat.2014.06.010
Chokshi, A., Sifri, Z., Cennimo, D., and Horng, H. (2019). Global Contributors to Antibiotic Resistance. J. Glob. Infect. Dis. 11 (1), 36–42. doi:10.4103/jgid.jgid_110_18
Czekalski, N., Gascón Díez, E., and Bürgmann, H. (2014). Wastewater as a point Source of Antibiotic-Resistance Genes in the Sediment of a Freshwater lake. ISME J. 8 (7), 1381–1390. doi:10.1038/ismej.2014.8
Demuth, J. P., and Hahn, M. W. (2009). The Life and Death of Gene Families. Bioessays 31 (1), 29–39. doi:10.1002/bies.080085
Durão, P., Balbontín, R., and Gordo, I. (2018). Evolutionary Mechanisms Shaping the Maintenance of Antibiotic Resistance. Trends Microbiology 26 (8), 677–691. doi:10.1016/j.tim.2018.01.005
Faleye, A. C., Adegoke, A. A., Ramluckan, K., Fick, J., Bux, F., and Stenström, T. A. (2019). Concentration and Reduction of Antibiotic Residues in Selected Wastewater Treatment Plants and Receiving Waterbodies in Durban, South Africa. Sci. Total Environ. 678, 10–20. doi:10.1016/j.scitotenv.2019.04.410
Guo, J., Li, J., Chen, H., Bond, P. L., and Yuan, Z. (2017). Metagenomic Analysis Reveals Wastewater Treatment Plants as Hotspots of Antibiotic Resistance Genes and mobile Genetic Elements. Water Res. 123, 468–478. doi:10.1016/j.watres.2017.07.002
Guo, N., Wang, Y., Tong, T., and Wang, S. (2018a). The Fate of Antibiotic Resistance Genes and Their Potential Hosts during Bio-Electrochemical Treatment of High-Salinity Pharmaceutical Wastewater. Water Res. 133, 79–86. doi:10.1016/j.watres.2018.01.020
Guo, X., Gao, Q., Yuan, M., Wang, G., Zhou, X., Feng, J., et al. (2020). Gene-Informed Decomposition Model Predicts Lower Soil Carbon Loss Due to Persistent Microbial Adaptation to Warming. Nat. Commun. 11 (1), 4897–4912. doi:10.1038/s41467-020-18706-z
Guo, X., Yan, Z., Zhang, Y., Xu, W., Kong, D., Shan, Z., et al. (2018b). Behavior of Antibiotic Resistance Genes under Extremely High-Level Antibiotic Selection Pressures in Pharmaceutical Wastewater Treatment Plants. Sci. Total Environ. 612, 119–128. doi:10.1016/j.scitotenv.2017.08.229
Hobæk, B., and Lie, A. K. (2019). Less Is More: Norwegian Drug Regulation, Antibiotic Policy, and the “Need Clause”. Milbank Q. 97 (3), 762–795. doi:10.1111/1468-0009
Hou, J., Chen, Z., Gao, J., Xie, Y., Li, L., Qin, S., et al. (2019). Simultaneous Removal of Antibiotics and Antibiotic Resistance Genes from Pharmaceutical Wastewater Using the Combinations of Up-Flow Anaerobic Sludge Bed, Anoxic-Oxic Tank, and Advanced Oxidation Technologies. Water Res. 159, 511–520. doi:10.1016/j.watres.2019.05.034
Jäger, T., Hembach, N., Elpers, C., Wieland, A., Alexander, J., Hiller, C., et al. (2018). Reduction of Antibiotic Resistant Bacteria during Conventional and Advanced Wastewater Treatment, and the Disseminated Loads Released to the Environment. Front. Microbiol. 9, 2599. doi:10.3389/fmicb.2018.02599
Ju, F., Beck, K., Yin, X., Maccagnan, A., McArdell, C. S., Singer, H. P., et al. (2019). Wastewater Treatment Plant Resistomes Are Shaped by Bacterial Composition, Genetic Exchange, and Upregulated Expression in the Effluent Microbiomes. ISME J. 13 (2), 346–360. doi:10.1038/s41396-018-0277-8
Klavarioti, M., Mantzavinos, D., and Kassinos, D. (2009). Removal of Residual Pharmaceuticals from Aqueous Systems by Advanced Oxidation Processes. Environ. Int. 35 (2), 402–417. doi:10.1016/j.envint.2008.07.009
Klümper, U., Recker, M., Zhang, L., Yin, X., Zhang, T., Buckling, A., et al. (2019). Selection for Antimicrobial Resistance Is Reduced when Embedded in a Natural Microbial Community. ISME J. 13 (12), 2927–2937. doi:10.1038/s41396-019-0483-z
Li, D., Yu, T., Zhang, Y., Yang, M., Li, Z., Liu, M., et al. (2010). Antibiotic Resistance Characteristics of Environmental Bacteria from an Oxytetracycline Production Wastewater Treatment Plant and the Receiving River. Appl. Environ. Microbiol. 76 (11), 3444–3451. doi:10.1128/aem.02964-09
Luo, G., Li, B., Li, L.-G., Zhang, T., and Angelidaki, I. (2017). Antibiotic Resistance Genes and Correlations with Microbial Community and Metal Resistance Genes in Full-Scale Biogas Reactors as Revealed by Metagenomic Analysis. Environ. Sci. Technol. 51 (7), 4069–4080. doi:10.1021/acs.est.6b05100
Luo, Y., Hui, D., and Zhang, D. (2006). Elevated Co2Stimulates Net Accumulations of Carbon and Nitrogen in Land Ecosystems: A Meta-Analysis. Ecology 87 (1), 53–63. doi:10.1890/04-1724
Martínez, J. L. (2008). Antibiotics and Antibiotic Resistance Genes in Natural Environments. Science 321 (5887), 365–367. doi:10.1126/science.1159483
Milaković, M., Vestergaard, G., González-Plaza, J. J., Petrić, I., Šimatović, A., Senta, I., et al. (2019). Pollution from Azithromycin-Manufacturing Promotes Macrolide-Resistance Gene Propagation and Induces Spatial and Seasonal Bacterial Community Shifts in Receiving River Sediments. Environ. Int. 123, 501–511. doi:10.1016/j.envint.2018.12.050
Oliveira, P. H., Touchon, M., Cury, J., and Rocha, E. P. C. (2017). The Chromosomal Organization of Horizontal Gene Transfer in Bacteria. Nat. Commun. 8 (1), 841–911. doi:10.1038/s41467-017-00808-w
Pallares-Vega, R., Blaak, H., van der Plaats, R., de Roda Husman, A. M., Hernandez Leal, L., van Loosdrecht, M. C. M., et al. (2019). Determinants of Presence and Removal of Antibiotic Resistance Genes during WWTP Treatment: A Cross-Sectional Study. Water Res. 161, 319–328. doi:10.1016/j.watres.2019.05.100
Petrovich, M. L., Zilberman, A., Kaplan, A., Eliraz, G. R., Wang, Y., Langenfeld, K., et al. (2020). Microbial and Viral Communities and Their Antibiotic Resistance Genes throughout a Hospital Wastewater Treatment System. Front. Microbiol. 11, 153. doi:10.3389/fmicb.2020.00153
Price, J. R., Ledford, S. H., Ryan, M. O., Toran, L., and Sales, C. M. (2018). Wastewater Treatment Plant Effluent Introduces Recoverable Shifts in Microbial Community Composition in Receiving Streams. Sci. Total Environ. 613-614, 1104–1116. doi:10.1016/j.scitotenv.2017.09.162
Quast, C., Pruesse, E., Yilmaz, P., Gerken, J., Schweer, T., Yarza, P., et al. (2012). The SILVA Ribosomal RNA Gene Database Project: Improved Data Processing and Web-Based Tools. Nucleic Acids Res. 41 (D1), D590–D596. doi:10.1093/nar/gks1219
SEPA (2008). Discharge Standards of Water Pollutants for Pharmaceutical Industry Chemical Synthesis Products Category GB21904-2008. New York: Pearson.
Suvorova, I. A., and Gelfand, M. S. (2019). Comparative Genomic Analysis of the Regulation of Aromatic Metabolism in Betaproteobacteria. Front. Microbiol. 10, 642. doi:10.3389/fmicb.2019.00642
Tong, J., Tang, A., Wang, H., Liu, X., Huang, Z., Wang, Z., et al. (2019). Microbial Community Evolution and Fate of Antibiotic Resistance Genes along Six Different Full-Scale Municipal Wastewater Treatment Processes. Bioresour. Technol. 272, 489–500. doi:10.1016/j.biortech.2018.10.079
Wang, J., Mao, D., Mu, Q., and Luo, Y. (2015). Fate and Proliferation of Typical Antibiotic Resistance Genes in Five Full-Scale Pharmaceutical Wastewater Treatment Plants. Sci. Total Environ. 526, 366–373. doi:10.1016/j.scitotenv.2015.05.046
Wei, Z., Li, W., Zhao, D., Seo, Y., Spinney, R., Dionysiou, D. D., et al. (2019). Electrophilicity index as a Critical Indicator for the Biodegradation of the Pharmaceuticals in Aerobic Activated Sludge Processes. Water Res. 160, 10–17. doi:10.1016/j.watres.2019.05.057
Wu, D., Huang, X.-H., Sun, J.-Z., Graham, D. W., and Xie, B. (2017). Antibiotic Resistance Genes and Associated Microbial Community Conditions in Aging Landfill Systems. Environ. Sci. Technol. 51 (21), 12859–12867. doi:10.1021/acs.est.7b03797
Wu, D., Su, Y., Xi, H., Chen, X., and Xie, B. (2019). Urban and Agriculturally Influenced Water Contribute Differently to the Spread of Antibiotic Resistance Genes in a Mega-City River Network. Water Res. 158, 11–21. doi:10.1016/j.watres.2019.03.010
Yang, Y., Jiang, X., Chai, B., Ma, L., Li, B., Zhang, A., et al. (2016). ARGs-OAP: Online Analysis Pipeline for Antibiotic Resistance Genes Detection from Metagenomic Data Using an Integrated Structured ARG-Database. Bioinformatics 32 (15), 2346–2351. doi:10.1093/bioinformatics/btw136
Yin, X., Jiang, X.-T., Chai, B., Li, L., Yang, Y., Cole, J. R., et al. (2018). ARGs-OAP v2.0 with an Expanded SARG Database and Hidden Markov Models for Enhancement Characterization and Quantification of Antibiotic Resistance Genes in Environmental Metagenomes. Bioinformatics 34 (13), 2263–2270. doi:10.1093/bioinformatics/bty053
Yuan, Q.-B., Huang, Y.-M., Wu, W.-B., Zuo, P., Hu, N., Zhou, Y.-Z., et al. (2019). Redistribution of Intracellular and Extracellular Free & Adsorbed Antibiotic Resistance Genes through a Wastewater Treatment Plant by an Enhanced Extracellular DNA Extraction Method with Magnetic Beads. Environ. Int. 131, 104986. doi:10.1016/j.envint.2019.104986
Zhang, D., Luo, J., Lee, Z. M. P., Maspolim, Y., Gersberg, R. M., Liu, Y., et al. (2016). Characterization of Bacterial Communities in Wetland Mesocosms Receiving Pharmaceutical-Enriched Wastewater. Ecol. Eng. 90, 215–224. doi:10.1016/j.ecoleng.2015.12.043
Zhang, Y., Xie, J., Liu, M., Tian, Z., He, Z., van Nostrand, J. D., et al. (2013). Microbial Community Functional Structure in Response to Antibiotics in Pharmaceutical Wastewater Treatment Systems. Water Res. 47 (16), 6298–6308. doi:10.1016/j.watres.2013.08.003
Zhao, F., Ju, F., Huang, K., Mao, Y., Zhang, X.-X., Ren, H., et al. (2019a). Comprehensive Insights into the Key Components of Bacterial Assemblages in Pharmaceutical Wastewater Treatment Plants. Sci. Total Environ. 651, 2148–2157. doi:10.1016/j.scitotenv.2018.10.101
Zhao, R., Feng, J., Liu, J., Fu, W., Li, X., and Li, B. (2019b). Deciphering of Microbial Community and Antibiotic Resistance Genes in Activated Sludge Reactors under High Selective Pressure of Different Antibiotics. Water Res. 151, 388–402. doi:10.1016/j.watres.2018.12.034
Zhao, W., Wang, B., and Yu, G. (2018). Antibiotic Resistance Genes in China: Occurrence, Risk, and Correlation Among Different Parameters. Environ. Sci. Pollut. Res. 25 (22), 21467–21482. doi:10.1007/s11356-018-2507-z
Zhou, J., Deng, Y., Shen, L., Wen, C., Yan, Q., Ning, D., et al. (2016). Temperature Mediates continental-scale Diversity of Microbes in forest Soils. Nat. Commun. 7 (1), 12083–12110. doi:10.1038/ncomms12083
Zhou, J., He, Z., Yang, Y., Deng, Y., Tringe, S. G., and Alvarez-Cohen, L. (2015). High-Throughput Metagenomic Technologies for Complex Microbial Community Analysis: Open and Closed Formats. MBio 6 (1), e02288–14. doi:10.1128/mBio.02288-14
Keywords: pharmaceutical wastewater, antibiotic resistance genes, homologous ARG, GeoChip, receiving river
Citation: Chen L, Zhang M, Ning D, Van Nostrand JD, Yang Y, Zhou J and Zuo J (2021) Behaviors of Homologous Antibiotic Resistance Genes in a Cephalosporin WWTP, Subsequent WWTP and the Receiving River. Front. Environ. Sci. 9:783676. doi: 10.3389/fenvs.2021.783676
Received: 26 September 2021; Accepted: 22 November 2021;
Published: 17 December 2021.
Edited by:
Zhi Wang, Innovation Academy for Precision Measurement Science and Technology (CAS), ChinaReviewed by:
Xiaohui Liu, Chinese Research Academy of Environmental Sciences, ChinaYu Xia, Southern University of Science and Technology, China
Copyright © 2021 Chen, Zhang, Ning, Van Nostrand, Yang, Zhou and Zuo. This is an open-access article distributed under the terms of the Creative Commons Attribution License (CC BY). The use, distribution or reproduction in other forums is permitted, provided the original author(s) and the copyright owner(s) are credited and that the original publication in this journal is cited, in accordance with accepted academic practice. No use, distribution or reproduction is permitted which does not comply with these terms.
*Correspondence: Jiane Zuo, amlhbmUuenVvQHRzaW5naHVhLmVkdS5jbg==