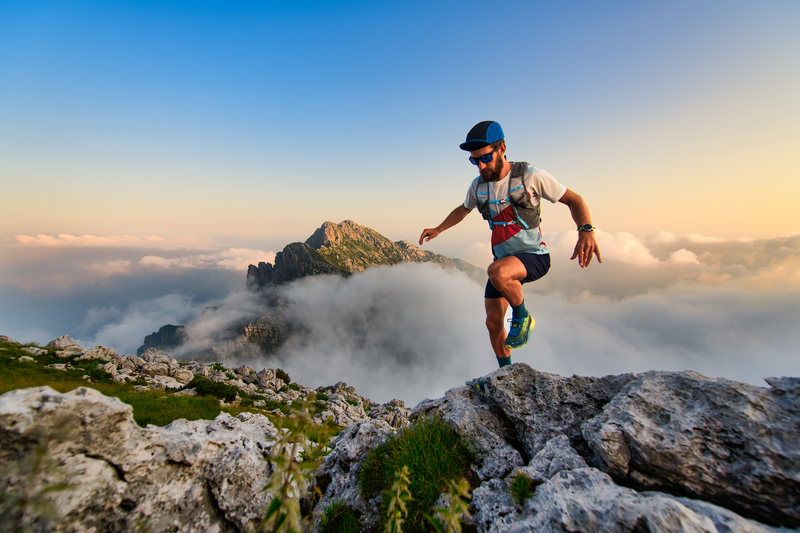
94% of researchers rate our articles as excellent or good
Learn more about the work of our research integrity team to safeguard the quality of each article we publish.
Find out more
ORIGINAL RESEARCH article
Front. Environ. Sci. , 04 January 2022
Sec. Interdisciplinary Climate Studies
Volume 9 - 2021 | https://doi.org/10.3389/fenvs.2021.783324
Climate change and land use/cover change (LUCC) have been widely recognized as the main driving forces that can affect regional hydrological processes, and quantitative assessment of their impacts is of great importance for the sustainable development of regional ecosystems, land use planning and water resources management. This study investigates the impacts of climate change and LUCC on variables such as streamflow (SF), soil moisture (SM) and evapotranspiration (ET) in the Guangdong-Hong Kong-Macao Greater Bay Area (GBA) by using Soil and Water Assessment Tools (SWAT) model under different scenarios during 1979–2018. The results show that the simulation performances were overall good, with Nash-Sutcliffe Efficiency Coefficient (NSE) and coefficient of determination (R2) greater than 0.80 for the monthly-scale SF calibration and validation. According to the results of trend and change point tests of meteorological series, the baseline period (1979–1997) and the interference period (1998–2018) were determined. Interestingly, other land use types were basically converted to urban land, leading to a rapid urbanization in the GBA. Compared with the SF values of the eight estuaries of the Pearl River Basin in the baseline period, both climate change and LUCC has led to the decrease in the SF values in the interference period, and the combined effect of climate change and LUCC was slightly greater than their individual effect. Overall, climate change and LUCC both have important impacts on regional hydrological processes in the GBA.
Climate change and land use/cover change (LUCC) are the two main driving forces affecting hydrological processes (Shi and Chen, 2018; Lamichhane and Shakya, 2019; Dosdogru et al., 2020; Guo et al., 2020; Wang Q et al., 2020). Climate change, especially changes in precipitation and temperature, will greatly affect the hydrological processes (Maghsood et al., 2019; Zhao et al., 2019; Jiang et al., 2021). Streamflow (SF) is one of the important elements in the hydrological cycle, and climate change can affect the SF mainly through changes in precipitation and evapotranspiration (ET). As one of the main sources of the SF, precipitation has a direct effect on the SF; while temperature has an indirect effect on the SF through affecting the ET (Xu et al., 2019; Yang et al., 2019). In addition, LUCC such as reservoir construction, irrigation abstraction, and soil and water conservation projects have a growing impact on the regional hydrological cycle (Iqbal et al., 2018; Xu et al., 2019; Yang et al., 2019; Zhao et al., 2021), especially under the influence of urbanization due to rapid socioeconomic development. Different land use types have different effects on precipitation interception, blocking, transpiration, and infiltration (Berg et al., 2016; Yonaba et al., 2021), so LUCC not only causes changes in surface or groundwater volume, but also changes the way regional water circulates. The expansion of urban land will reduce water retention and infiltration, increase the SF, and even increase the flood frequency (Baker and Miller, 2013; Wang Q et al., 2018). Therefore, quantitative assessment of the impacts of climate change and LUCC on regional hydrological processes is of great importance for water resources management and sustainable development (Yan et al., 2018; Liang et al., 2020).
Currently, there are three broad types of methods to quantify the contribution of climate change and LUCC to the SF, including empirical statistical methods (time series analysis), sensitivity analysis methods, and hydrological modeling methods. The empirical statistical methods are mainly based on the correlation and trend analysis of time series data (Ahn and Merwade, 2014; Hu et al., 2020), which are generally easy to implement but usually require a long-term historical hydrometeorological data (Jiang and Wang, 2016). Due to the lack of physical mechanisms, this type of methods may not be able to capture the exact nonlinear nature of the hydrological system (Wang et al., 2012). The sensitivity analysis methods are mainly based on the water balance within a basin. After the derivation of a series of equations, sensitivity analysis can be carried out to quantitatively calculate the SF attribution (Zhang K et al., 2020). This type of methods allows to estimate the sensitivity of the SF to climate variables using sensitivity indices with few data (Wang et al., 2012; Tu et al., 2015), but cannot directly quantify the effects of hydrological extremes and human activities, which limits its value (Zeng et al., 2020). The hydrological modeling methods usually divide the whole study period into a base period and an interference period, and the simulation results for these two periods are then compared to quantitatively distinguish the effects of climate change and human activities on the SF. This type of methods needs to consider a variety of parameters, and the key issue is to establish a suitable model for analysis (Tan et al., 2020). The third type of methods seems to be the most popular in recent years, and thus, will be used in this study.
In the context of global warming, previous studies mainly have focused on the SF responses (Baker and Miller, 2013; Jiang and Wang, 2016; Kundu et al., 2017; Zeng et al., 2020) rather than other important variables such as the ET and soil moisture (SM), which can also affect regional hydrological processes and need further investigation. Moreover, several studies have directly attributed LUCC to human activities (Ahn and Merwade, 2014; Zhai and Tao, 2017; Xu et al., 2020), which needs to be adequately discussed. In addition, the impacts of climate change and LUCC on regional hydrological processes are spatially distinct (Li B et al., 2020; Wang Q et al., 2020; Yonaba et al., 2021). Understanding these impacts requires an accurate assessment of the different conditions for a given region. Although several previous studies have attempted to consider both the individual and combined impacts of climate change and LUCC on regional hydrological processes, for a designated region, it is important and necessary to explore the relative contributions of climate change and LUCC to regional hydrological processes under various scenarios so that a deeper understanding of the influence mechanisms can be obtained.
For the above reasons, this study takes the Guangdong-Hong Kong-Macao Greater Bay Area (GBA), which is one of the most developed regions in China, as the study area to investigate the impacts of climate change and LUCC on regional hydrological processes. The GBA has been undergoing rapid urbanization in the past decades (Zhang J et al., 2020), and there have been more frequent extreme events recently (Wang et al., 2021), leading to an increasing flood risk in the GBA (Zhang et al., 2019a). Therefore, to identify and understand the evolution of climate change and LUCC in the GBA and their impacts on regional hydrological processes is vital to better promote the sustainable development of the GBA (Liu F et al., 2020). The main objective of this study is to quantify the individual and combined impacts of climate change and LUCC on regional hydrological processes. First, we analyzed the spatial and temporal evolution characteristics of LUCC based on land use data series. Second, we determined the baseline and interference periods based on meteorological data series. Then, we analyzed the spatial and temporal evolution characteristics of hydrological elements based on hydrological simulation results. Finally, we conducted quantitative analysis of the impacts of climate change and LUCC on several hydrological elements under different scenarios. Overall, the outcomes of this study can provide a reference for the rational allocation of regional water resources in such regions.
The GBA is located in South China (21°30′-23°40′N, 112°12′-113°48′E) and covers an area of 56,000 km2 . The GBA includes two special administrative regions [i.e., Hong Kong (HK) and Macao (MC)] and nine cities in Guangdong Province [i.e., Guangzhou (GZ), Dongguan (DG), Huizhou (HZ), Shenzhen (SZ), Foshan (FS), Zhongshan (ZS), Zhuhai (ZH), Jiangmen (JM), and Zhaoqing (ZQ)]. The GBA has a subtropical climate with a mean annual temperature of 22.3°C and a mean annual rainfall of 1832 mm. The center of the GBA is the alluvial plain of the Pearl River Delta, surrounded by hills in the west, north, and east (Li and Wen, 2018; Lyu et al., 2018; Yang et al., 2020). The GBA is a highly integrated urbanized region with the economy aggregate almost equal to that of the New York Bay Area (Wang et al., 2021); however, the development levels among cities are still uneven. Among the 11 cities, HK, SZ, and GZ are far ahead of other cities in terms of annual gross domestic product (GDP). In 2019, the GBA had a total population of over 72 million and a GDP of 11.59 trillion Yuan (Liu F et al., 2020). The rapid urbanization and high-intensity land development in the GBA has put tremendous pressure on regional hydrological and ecological systems, making this a key policy concern that required attention.
This study will select the Soil and Water Assessment Tool (SWAT) as the hydrological model, and this model needs the topographic, soil properties, land use, and meteorological data as the input data. The topographic data are from the Shuttle Radar Topography Mission at 90 m × 90 m resolution (https://www2.jpl.nasa.gov/srtm/). The soil data (scale = 1:1,000,000) are from the Harmonized World Soil Database, and there are totally 49 soil types (http://www.fao.org/home/en/). The land use data during 1992–2018 are from the European Space Agency, with a resolution of 30 m (https://www.esa.int/), and the main land use types included are Urban aeras, Cropland, Herbaceous, Tree or shrub cover, Bare areas, and Water bodies. The daily precipitation, maximum and minimum temperature, mean wind speed, solar radiation and relative humidity data during 1979–2018 are from the China Meteorological Assimilation Datasets for the SWAT model (CMADS, http://www.cmads.org/), with the resolution of 1/3°; its format is designed to match the input data format of the SWAT model, and thus, the CMADS data can be directly used without format conversion (Liu et al., 2018; Meng et al., 2019; Song et al., 2020; Wang N et al., 2020; Zhang L et al., 2020; Liu et al., 2021). The daily SF data during 2000–2013 at the Boluo hydrological station (the outlet of the East River Basin, see Figure 1) are provided by the Pearl River Water Resources Commission (Shi et al., 2018; Liu S et al., 2020).
FIGURE 1. Location of GBA, eight estuaries of the Pearl River Basin, and the Boluo hydrological station.
The SWAT model is a typical GIS-based distributed hydrological model developed by the U.S. Department of Agriculture Agricultural Research Center (Zhang et al., 2019b; Sun et al., 2020), and has been widely used to numerous river basins worldwide (Abbaspour et al., 2007; Baker and Miller, 2013). This model was originally developed to predict and evaluate the long-term impacts of human activities on water cycle, sediment, and agricultural pollutant transport in large-scale watersheds characterized by different soil types, land use types, and management conditions (Song et al., 2020; Wang N et al., 2020). The SWAT model adopts Soil Conservation Service (SCS) Curve Number (CN) method to simulate the surface SF, which is a simple conceptual approach for estimating the depth of SF based on rainfall depth. It relies on only one parameter (i.e., CN), and has been widely used (Wu et al., 2019). Regarding Hydrological Response Unit (HRU) as the basic simulation unit, the SWAT model can well simulate a variety of the land-surface hydrological processes at the sub-basin scale, e.g., the SF changes. The SWAT model has a good physical mechanism to define the model inputs, boundary conditions, spatial variability, and hydrological characteristics in a comprehensive and objective way. The spatial and temporal heterogeneities in the watershed can be reflected in the model as parameters with actual physical significances, so that the model has a good physical support from the input to the intermediate processing process to the output results, increasing the credibility and accuracy of the simulation results (Wang Q et al., 2018; Li B et al., 2020). Moreover, the SWAT model has been widely used in different watersheds in Guangdong Province and proved to be an effective tool for studying the hydrological responses to climate change and LUCC (Wu and Chen, 2013; Yin et al., 2017; Zhou YL et al., 2018; Touseef et al., 2020; Wang QF et al., 2020). Therefore, the SWAT model is selected for hydrological simulations in the study area. In this study, the East River Basin will be divided into 25 sub-basins and the GBA will be divided into 290 sub-basins. The overlapping area of the East River Basin and the GBA is 11,445 km2 (i.e., 38% of the East River Basin and 20.74% of the GBA). Therefore, the SWAT model will be calibrated in the East River Basin since it is a whole watershed, and then be applied in the GBA.
Water balance is the base of the SWAT model, and the hydrological processes mainly include precipitation, SF, infiltration, ET, baseflow, and so on. The terrestrial phase of the hydrological cycle in the SWAT model adheres to the water balance equation as follows.
where
SWAT-CUP (Calibration and Uncertainty Programs) integrates the SUFI-2 (Sequential Uncertainty Fitting version 2) algorithm, PSO (Particle Swarm Optimization) algorithm, GLUE (Generalized Likelihood Uncertainty Estimation) algorithm, ParaSOL (Parameter Solution) algorithm, and MCMC (Markov Chain Monte Carlo) algorithm. In this study, the SUFI-2 algorithm is used for automatic calibration of the SWAT model (Yang et al., 2008; Mandal et al., 2021). The SUFI-2 algorithm takes better account of the model uncertainty and is widely used in the performance evaluation of hydrological simulations because it requires fewer parameters to be calculated during the calibration process than other algorithms (Yang et al., 2008; Dosdogru et al., 2020). This algorithm can provide the optimal value and the uncertainty range of each calibrated parameter (Gao et al., 2020; Ma et al., 2020; Martínez-Retureta et al., 2020). However, the SUFI-2 algorithm does not consider the parameter relativity that affects the performance. To quantitatively evaluate the performance of the SF simulation, five objective functions, i.e., coefficient of determination (R2), Nash-Sutcliffe Efficiency Coefficient (NSE), P-factor (i.e., the percentage of observations bracketed by the 95% of the predicted uncertainty (95PPU)), R-factor, and percent bias (PBIAS), are used as the assessment criteria:
where
The Mann-Kendall method, which is a non-parametric rank-based statistical method, has been commonly used to detect the trends in the time series of hydrometeorological variables such as precipitation, SF, and temperature (Dakhlalla and Parajuli, 2015; Piniewski et al., 2017; Zhou et al., 2020a; Zhou et al., 2020b; Zou et al., 2020; Jiang et al., 2021). It has a simple calculation process, and the results are not disturbed by a few outliers in the time series. Moreover, the samples do not need to obey a certain distribution, making this method suitable for time series with non-normal distribution (Toride et al., 2018). For a given significance level
The sliding t-test method has been commonly used to detect the change points in the time series of hydrometeorological variables such as precipitation, SF, and temperature by examining whether the difference between the means of the two sample groups is significant (Wang X et al., 2018; Hu et al., 2020). If the difference exceeds a certain level of significance, it can be assumed that there is a qualitative change.
For a sequence
where
To assess the impacts of climate change and LUCC on regional hydrological processes, scenario combinations are often used when conducting the SF simulation with hydrological models (Deng et al., 2014; Tan et al., 2020; Zhou et al., 2021). According to the results in subsection 3.3, the baseline period (1979–1997) and the interference period (1998–2018) can be determined, and then, the scenarios for quantitative attribution analysis can be set as shown in Table 1.
S1 scenario is regarded as the reference scenario, which uses the land use/cover and climate data in the baseline period. By comparing the simulation results under S1 and other scenarios (S2, S3, and S4), the contributions of climate changes and LUCC to regional hydrological processes (e.g., the SF) in the GBA can be quantified. Based on S1 scenario, S2 scenario is designed to quantify the contribution of climate change, using the land use/cover data in the baseline period and climate data in the interference period, S3 scenario is designed to quantify the contribution of LUCC, using the land use/cover data in the interference period and climate data in the baseline period, and S4 scenario is designed to quantify the combined impacts of both climate change and LUCC, using the land use/cover and climate data in the interference period. In addition, the relative contribution of climate change and LUCC to the SF can be calculated as follows:
where Q1, Q2, Q3, and Q4 are the mean annual SF simulated under S1, S2, S3, and S4 scenarios, respectively.
With the SWAT model, we divided the East River Basin into 25 sub-basins and the GBA into 290 sub-basins. Combined with 49 soil types and 6 land use types, taking HRU as the basic simulation unit, the hydrological elements of each sub-basin were modeled and aggregated, and they are converged to the outlet of the whole river basin. Sensitivity analysis and automatic calibration were performed by using the SUFI-2 in the SWAT-CUP, which indicated that 10 parameters listed in Table 2 were the most sensitive.
In this study, we divided the SF data into the calibration period of 2000–2006 and the validation period of 2007–2013 to ensure that the SF data used for calibration and validation contain a certain range of hydrological events (i.e., high, normal, and low flow years) in each period (Wang et al., 2010). Figure 2A shows the comparison of the observed and simulated SF data at the Boluo hydrological station during 2000–2006 (calibration period) and 2007–2013 (validation period). The results show that NSE, R2 and PBIAS were 0.91, 0.92, 24.1% for the calibration period, while 0.89, 0.9 and 29.6% for the validation period. In general, R2 > 0.85, NSE >0.90 and PBAIS <30% indicate that the SWAT model is suitable for the study area. Meanwhile, the results show that P-factor > 0.7, R-factor < 1.5, which proves that the simulation performance is good. Figures 2B,C show the linear fittings of the simulated SF and observed SF during the calibration and validation periods, respectively. The results show that the R2 values were greater than 0.9, indicating that the simulation results of this study were reliable (Meng et al., 2019; Guo et al., 2020; Tan et al., 2020; Wang Q et al., 2020).
FIGURE 2. (A) Comparison of the observed SF and simulated SF at the Boluo hydrological station during the calibration and validation periods; (B) linear fitting of the simulated SF and observed SF during the calibration period; and (C) linear fitting of the simulated SF and observed SF during the validation period.
Figure 3 shows the land use/cover maps in 1992 and 2018 (Figure 3A) and the area changes of land use/cover from 1992 to 2018 (Figure 3B). Compared with 1992, the area of urban areas in the GBA had a dramatic increase of 256.74% in 2018, while the areas of all other land use/cover types decreased (i.e., −13.21%, −67.84%, −2.06%, −78.49%, and −7.83% for cropland, herbaceous, tree or shrub cover, bare areas, and water bodies, respectively. It is worth noting that the main types of land use/cover in the GBA are cropland and tree or shrub cover, which account for about 70% of the GBA and are mainly distributed in ZQ, JM and HZ. In contrast, urban areas are mainly concentrated in the Pearl River Delta Plain (Figure 3A), and its area increased rapidly from 1992 to 2018 due to the rapid socioeconomic development (Figure 3B). The cities with the top five urbanization expansion areas are GZ, SZ, DG, FS, and ZS.
FIGURE 3. (A) Land use/cover maps in 1992 and 2018; (B) area changes of land use/cover from 1992 to 2018.
In addition, the conversion matrix of LUCC indicates that most herbaceous and bare areas have changed to urban areas, and only a small proportion of cropland and water bodies have changed to urban areas (Table 3). As a result, the area of urban areas has presented a substantial growth. It is worth mentioning that only the conversion rates from other land use/cover types to urban areas were relatively larger, and the interconversions of different land use/cover types would have different effects on hydrological variables, leading to impacts on regional hydrological cycle in the GBA (Tu et al., 2015).
To isolate the individual impacts of climate change and LUCC on regional hydrological processes, this study firstly determined the change points in the time series of climate variables, and then determined the baseline period and the interference period. Generally, the contribution of potential evapotranspiration is much smaller than that of precipitation, especially on rainy days; moreover, potential evapotranspiration can be closely related to temperature. Therefore, we only analyzed the precipitation and temperature changes but did not analyze the potential evaporation change in this study. In addition, Xue et al. (2021) demonstrated that radiation could be negligible when simulating streamflow with the SWAT model. Therefore, we did not consider the radiation changes in this study. Figures 4A,B show the temporal variations of precipitation and temperature in the GBA during 1979–2018 by using Mann-Kendall method, while Figures 4C,D show the results of change point test for precipitation and temperature by using sliding t-test method. Change points around 2017 and 1997 were found in the time series of precipitation (Figure 4C) and temperature (Figure 4D) from 1979 to 2018. Combining the change point tests for precipitation and temperature, the middle year 1997 was regarded as the change point from 1979 to 2018. Therefore, in this study, years before 1997 were classified as the baseline period and years after 1997 were classified as the interference period. Then, the temporal trends of precipitation and temperature during these two periods could be obtained, as shown in Figures 4A,B, respectively. Different trends could be observed for these two climatic variables since 1979, and the annual changing rates of precipitation and temperature were not quite large. Precipitation showed an increasing trend in the baseline period but a decreasing trend in the interference period, with the changing rates of 57.28 mm/10a and −39.44 mm/10a, respectively. Temperature showed increasing trends in both baseline and interference periods, and the increasing trend in the interference period (i.e., 0.33°C/10a) was slightly greater than that in the baseline period (i.e., 0.22°C/10a). In addition, the climatic variables in the GBA differed in spatial distribution characteristics during the 40-year period. Figure 5 shows the spatial distribution characteristics of precipitation and temperature in the GBA during 1979–2018. Compared with the baseline period, decreasing precipitation could be identified in the northwestern, northeastern, and central-eastern parts of the GBA, while increasing temperature could be identified in the northeastern part of the GBA.
FIGURE 4. Temporal trends of (A) precipitation and (B) temperature in the GBA during 1979–2018; Sliding t-test for (C) precipitation and (D) temperature during 1979–2018.
FIGURE 5. Spatial distribution characteristics of precipitation and temperature in the GBA during 1979–2018.
The SWAT model was used to simulate the changes of three hydrological variables (i.e., SF, SM, and ET) in the GBA during the study period. Since the SF differences in Humen and Modaomen between 1992 and 2018 were relatively large, this study will take these two estuaries as examples to analyze the variation characteristics of hydrological variables. During 1979–1997, the SF and SM in Humen increased with the changing rates of 41.97 m3/10a and 6.70 mm/10a, respectively; the SF and SM in Modaomen increased with the changing rates of 40.35 m3/10a and 9.23 mm/10a, respectively. In contrast, during 1998–2018, the SF and SM of Humen and Modaomen showed decreasing trends, with the changing rates of −47.60 m3/10a and −3.10 mm/10a for the SF and SM in Humen, and −45.18 m3/10a and −1.47 mm/10a for the SF and SM in Modaomen. However, the ET trends were significantly different from those of SF and SM. Both Humen and Modaomen showed decreasing trends from 1979 to 1997 with the changing rates of −11.17 mm/10a and −16.18 mm/10a, respectively. During 1998–2018, Humen showed an increasing trend (15.57 mm/10a), while Modaomen showed a decreasing trend (−2.29 mm/10a). Generally, the changes of the SF, SM, and ET in the GBA might be related to both climate change and LUCC. The decrease of precipitation and the increase of temperature in the GBA could lead to the increase of the ET and then affect the SF. Meanwhile, LUCC would affect not only the ET, but also the SF and SM. Different land use types have different ET rates due to different vegetation cover, leaf area index, root depth and albedo. Moreover, soil properties are one of the most important factors affecting infiltration capacity, and LUCC can indirectly affect infiltration and then the SM through changing the soil properties (Wan and Yang, 2007).
Based on the differences of hydrological variables between S1 and other three scenarios, the contributions of climate change and LUCC to hydrological cycle can be evaluated. Figure 6 shows the spatial distributions of these three hydrological variables (i.e., SF, SM, and ET) under four scenarios. These changes were not only the result of climate change, but also the direct human activities including LUCC.
Known from Figure 7, during 1979–2018, the SF in these eight estuaries all showed decreasing trends due to climate change and LUCC. Except for Hongqimen, which had the contribution of climate change to the SF slightly larger than that of LUCC, the contributions of LUCC to the SF in the other seven estuaries were all greater than those of climate change to the SF. This may be related to the changes of climatic variables in the interference period. Compared with the baseline period, precipitation in the interference period decreased by 39.44 mm/10a, and temperature in the interference period increased by 0.33°C/10a, which could synthetically lead to the decrease in the SF. However, the variation amplitudes of climatic variables were still smaller than that of LUCC, indicating that other land use/cover types were mostly converted to urban areas and the area of urban areas increased by 256.74% from 1992 to 2018 (Figure 3B). Therefore, the overall contribution of climate change to the SF was a little smaller than that of LUCC (Figure 7). For the two estuaries with the relatively large SF differences (i.e., Humen and Modaomen), the impacts of climate change and LUCC on the SF were similar. The relative contributions of climate change and LUCC to the decrease in the SF were −47% and −48.6% in Humen, and −47% and −48.3% in Maodaomen. It is worth noting that the sum of the contributions of climate change and LUCC to the SF was less than 100%, which may be due to the interaction between climate change and LUCC (Jiang et al., 2007; Li et al., 2009).
FIGURE 7. Relative contributions of climate change and LUCC to the SF decline in the eight estuaries.
Due to the absence of the observed ET and SM data, this study only quantitatively analyzed the simulated ET and SM. We calculated the relative contributions of climate change and LUCC to the simulated ET and SM by using Eqs 4, 5, and the relevant results are listed in Table 4. Known from Figure 6, the changes in the SM and ET mainly occurred in the eastern and western parts of the GBA. Limited by the length of the article, this study only showed the detailed analyses of Humen and Modaomen, and other estuaries basically had similar characteristics.
Under the impact of climate change, ET showed increasing trends in both Humen and Modaomen, with the percentage changes of 1.7 and 1.0%, respectively. In contrast, SM showed opposite trends in both Humen and Modaomen, with the percentage changes of −1.5% and −3.8%, respectively. As shown in Table 4, climate change is the main driver of SM changes, with precipitation as the main source of SM (Li, 2014; Luo et al., 2021). Generally, SM increases rapidly after the occurrence of precipitation and then gradually decreases due to plant transpiration and ET (Holsten et al., 2009). Temperature can indirectly affect SM through controlling ET. In the context of global warming, the increase in ET caused by higher temperature is universally accepted (Holsten et al., 2009; Wang et al., 2017). In general, SM can be regarded as the balance between precipitation and ET (He et al., 2012; O’Gorman, 2012). Under the impact of LUCC, ET showed decreasing trends in both Humen and Modaomen, with the percentage changes of −3.6% and −1.5%, respectively. The variation amplitudes were larger than those under the impact of climate change. SM also showed increasing trends in both Humen and Modaomen, with the percentage changes of 10.5 and 2.3%, respectively. Under the combined impacts of climate change and LUCC, ET showed decreasing trends in both Humen and Modaomen, with the percentage changes of −2.0% and −0.7%, respectively, while SM showed increasing trends in both Humen and Modaomen, with the percentage changes of 8.3 and 3.6%, respectively. The changes in ET and SM were closely related to climate change and LUCC in the study area. Influenced by urbanization, part of precipitation is retained by impervious surfaces and directly returned to the atmosphere as ET; part of precipitation infiltrates into the underground, which is absorbed by plant roots and then transpires to the atmosphere. On the one hand, urbanization may lead to a decrease in the amount of ET and infiltration by reducing permeable surfaces, plant transpiration, and canopy retention (Chen et al., 2011; Zhang and Liang, 2018). On the other hand, conversion from herbaceous to cropland may result in an increase in the SM for vegetation growth (Atchley and Maxwell, 2011; Chang et al., 2014).
The SWAT model was to quantify the impacts of climate change and LUCC on regional hydrological processes under four scenarios during 1979–2018. The simulation results were calibrated and validated based on the observed SF data of the East River Basin, which were consistent with those in the previous results (Wu and Chen, 2013). As mentioned before, the overlap area between the GBA and the East River Basin accounts for 20.74% of the GBA and 38% of the East River Basin, which may affect the representativeness of the results to some extent. Moreover, although the SWAT model could well simulate the three hydrologic variables in the GBA, there were still some uncertainties in the model simulations. First, the SWAT-CUP used the SUFI-2 algorithm to evaluate the inherent uncertainties in the SWAT model, which might be due to the uncertainties in model calibration, forcing data, model structure, and model parameter estimation (Rouholahnejad et al., 2012; Ashraf Vaghefi et al., 2014; Abbaspour et al., 2017). Due to the complexity of hydrological processes, errors in hydrological data and imperfections in the model structure can bring uncertainties in the parameter calibration of the SWAT model, which may further affect the accuracy of the simulation results. Second, human activities such as reservoir operation and crop management were not considered in this study, resulting in the differences between the simulated and observed SF (Baker and Miller, 2013; Guo et al., 2016; Wu et al., 2019). Third, the simulation results were only based on two land use/cover maps in 1992 and 2018, which might cause underestimations or overestimations of LUCC in certain years and then the uncertainties in ET and SM (Li et al., 2009). Fourth, this study used the sliding t-test approach to determine the baseline period and the interference period; however, different assumptions and methods may lead to different change points, further affecting the follow-up analysis. Finally, our model was calibrated and validated based on only SF data, and thus, ET and SM simulations need further validation if the observed ET and SM data were available.
The results in this study indicated that the contribution of LUCC to regional hydrological process was greater than that of climate change. Unprecedented urban expansion has occurred in the GBA during the past decades, which may surely increase the area of impervious surface in this region. Land use/cover types in the GBA have mainly changed from cropland, herbaceous, tree or shrub cover, bare areas, water bodies to urban areas, and such results are consistent with those in the previous studies (Yang et al., 2020; Zhang J et al., 2020; Wang et al., 2021).
In addition, the conversions among different land use/cover types can affect the hydrological variables. In general, tree or shrub cover can produce less SF and SM than other land use/cover types, but more ET (Li et al., 2009). Since the biomass per unit area of tree or shrub cover and herbaceous is much lower than that of cropland, the water storage capacity, transpiration capacity, and thermal capacity of tree or shrub cover and herbaceous are all lower than that of cropland (Li Z et al., 2020). Therefore, the conversions from tree or shrub cover and herbaceous to cropland led to the changes in the SF, ET, and SM in this study. Normally, urbanization may lead to an increase in the SF. Although most of other land use/cover types in the GBA were converted to urban areas, the proportion of urbanization area in the GBA was still relatively small (i.e., about 13%). Therefore, the increase in the SF caused by urbanization may be smaller than the decrease in the SF caused by the transformation processes of other land use/cover types, leading to the overall decreasing SF in the GBA. Moreover, with the development of urbanization, a large number of natural surfaces have been transformed into impervious surfaces, which may largely affect the ecological environment of cities and generate a series of ecological and environmental problems, such as urban heat island effect and urban flooding disasters (Shi et al., 2020). Therefore, the proportion of impervious surfaces should be controlled, and green space should be increased in the future development of cities in the GBA.
This study evaluated the temporal and spatial variation characteristics of climate variables from 1979 to 2018, showing that precipitation has decreased and temperature has increased during the past 40 years. The hydrological simulation results showed the decrease in the SF, increase in the ET, and decrease in the SM due to climate change. Such results were consistent with those in the previous studies (Ji and Duan, 2019; Liu Z et al., 2020). Precipitation is an important component of hydrological cycle, which can supply water and control the ET to some extent. During precipitation, the water vapor content near the surface is close to the saturated water vapor, so the evaporation from the soil surface can be ignored. Moreover, according to the Clausius-Clapeyron equation, saturated water vapor increases along with increasing temperature, which increases the water vapor pressure difference between the surface and air, and further enhances the ET (Guo et al., 2016; Li Q et al., 2017; Shrestha et al., 2019; Yang et al., 2017). As a result, the decrease in precipitation and the increase in temperature directly led to the decrease in the SF and SM but increase in the ET (Figure 7 and Table 4). Previous studies have shown that the impacts of climate change on the regional SF mainly originates from the changes in precipitation, while precipitation is an important source of the ET and SM. With the gradually worsening trend of global warming, the effect of high temperature on the regional hydrological cycle aggravates rapidly (Wang et al., 2017; Liu et al., 2019). Such changes to the regional hydrological cycle caused by climate change have caused a redistribution of water resources in time and space, which has important implications for the rational utilization and planning management of water resources.
This study also quantified the combined impacts of climate change and LUCC on regional hydrological cycle. The results indicated that the impact of LUCC on the SF was greater than that of climate change in the GBA. Such results were inconsistent with those in the previous studies on adjacent regions, which may be due to the different change points, lengths of data, and hydrological models (Wu et al., 2017; Mendoza-Ponce et al., 2018; Wang and Stephenson, 2018). For example, reported that the earlier the change point, the smaller the relative contribution of climate change and the larger the relative contribution of human activities.
However, there is an interaction between climate change and LUCC. Climatic variables affect LUCC, and in turn LUCC affects regional climate (Li G et al., 2017). The coupled climatic variables, land use/cover types, and hydrological models are complex and complicated by the interactions and feedbacks between subsystems through physical processes that separate the individual impacts of climate change and LUCC on hydrological processes from their combined impacts. In this study, urbanization-induced LUCC has direct and indirect effects on regional climate. For other land use/cover types, in the vertical direction, tree or shrub cover can reshape the regional surface roughness, increase atmospheric dynamic turbulence, transport water vapor to a higher position, and make the atmospheric water saturation in the clouds higher. However, the reduction of tree or shrub cover will reduce the atmospheric water vapor and absorbed heat, which may further hinder cloud condensation in wet and cool atmosphere (Li Z et al., 2020).
In addition, the spatial distributions of hydrological variables under climate change and LUCC showed that, unlike the SM, there was spatial heterogeneity in the ET changes caused by climate change and LUCC in the GBA (Figure 6). There was a strong regional pattern, e.g., climate change increases the ET in northeastern, northwestern, and southwestern regions, while LUCC decreases the ET in northeastern and southwestern regions.
We need to fully aware of the following limitations, which are mainly related to three aspects. First, our assessment is only on an annual scale, which does not reflect peak SF within a year, and runoff on an interannual scale is averaged to include runoff during both abundant and dry periods. Second, due to limitations of the SWAT model and available data, other human activities (e.g., reservoir operations) were not considered in this study. Reservoirs, such as the Xinfengjiang Reservoir in the East River Basin, would most likely affect the streamflow simulation. For example, storage change in reservoirs may increase the streamflow in dry season but decrease the streamflow in rainy season. Finally, this study ignores the interactions between climate change and LUCC by separating their individual impacts from their combined impacts. Therefore, the impacts of other human activities and the interactions between climate change and LUCC remain unresolved issues. Nevertheless, aware of the above limitations, other findings can be explored for quantifying the impact of climate change and LUCC on regional hydrological processes in the GBA based on this study, which has important implications for sustainable water resources management in developed regions.
This study analyzed the temporal and spatial distribution characteristics of climatic variables (i.e., precipitation and temperature) and simulated the three selected hydrological variables (i.e., SF, ET, and SM) in the GBA during 1979–2018. Based on these results, the individual and combined impacts of climate change and LUCC were thoroughly discussed. The main findings are summarized as follows:
1) Characteristics of climate change and LUCC. The GBA has experienced rapid urbanization during the study period with most of other land use/cover types turning into urban areas. In the GBA, the dramatic human activities have changed the land use/cover types, and the interconversion of different land use/cover types can have an impact on the hydrological cycle of the study area. In addition, in the GBA, precipitation decreased, and temperature increased, with the decrease in precipitation particularly in the northwestern, northeastern, and east-central parts of the GBA, while the significant change in temperature in the northeastern part of the GBA. Therefore, in the context of complex climate change and LUCC, it is necessary to consider the coupling relationship between human activities and natural factors in the GBA, where a more effective ecological land protection mechanism should be established.
2) Attribution analysis of climate change and LUCC based on the SWAT model and scenario combinations. The SWAT model could perform well in simulating the hydrological processes in the GBA, with all values of R2 and NSE above 0.85 during the calibration and validation periods. Then, the individual and combined impacts of climate change and LUCC on regional hydrological processes in the GBA were quantified. The results showed that both climate change and LUCC reduced the SF in all the eight estuaries, and the impact of LUCC was slightly larger than that of climate change. Therefore, the protection of major natural systems and the rational allocation of land resources in the GBA should be realized.
It is known that The GBA is one of the world’s four largest bay areas. Quantifying the regional hydrological responses to climate change and LUCC is critical for water resources management and economic development in this area. The outcomes of this study will provide useful information for stakeholders in ecological planning and management of land and water resources within the GBA. Moreover, stakeholders should focus on mitigating the negative impacts of LUCC from both hydrological and ecological perspectives, such as formulating relevant development plans or management programs. It is suggested that decision makers also need to give special consideration to the interactions between LUCC and climate change when addressing ecological conservation issues for sustainable development.
The original contributions presented in the study are included in the article/Supplementary Material, further inquiries can be directed to the corresponding authors.
SL and HS contributed to conception and design of the study. XT, YT, and JJ organized the database. XT, ZZ, and YW performed the statistical analysis. XT wrote the first draft of the manuscript. SL, ZZ, and HS wrote sections of the manuscript. All authors contributed to manuscript revision, read, and approved the submitted version.
The study was supported by the National Natural Science Foundation of China funded project (Grant No. 51909117), Natural Science Foundation of Shenzhen funded project (Grant No. JCYJ20210324105014039), Guangdong Provincial Key Laboratory of Soil and Groundwater Pollution Control, and State Environmental Protection Key Laboratory of Integrated Surface Water-Groundwater Pollution Control.
The authors declare that the research was conducted in the absence of any commercial or financial relationships that could be construed as a potential conflict of interest.
All claims expressed in this article are solely those of the authors and do not necessarily represent those of their affiliated organizations, or those of the publisher, the editors and the reviewers. Any product that may be evaluated in this article, or claim that may be made by its manufacturer, is not guaranteed or endorsed by the publisher.
Abbaspour, K. C., Yang, J., Maximov, I., Siber, R., Bogner, K., Mieleitner, J., et al. (2007). Modelling Hydrology and Water Quality in the pre-alpine/alpine Thur Watershed Using SWAT. J. Hydrol. 333, 413–430. doi:10.1016/j.jhydrol.2006.09.014
Abbaspour, K., Vaghefi, S., and Srinivasan, R. (2017). A Guideline for Successful Calibration and Uncertainty Analysis for Soil and Water Assessment: A Review of Papers from the 2016 International SWAT Conference. Water 10, 6. doi:10.3390/w10010006
Ahn, K.-H., and Merwade, V. (2014). Quantifying the Relative Impact of Climate and Human Activities on Streamflow. J. Hydrol. 515, 257–266. doi:10.1016/j.jhydrol.2014.04.062
Ashraf Vaghefi, S., Mousavi, S. J., Abbaspour, K. C., Srinivasan, R., and Yang, H. (2014). Analyses of the Impact of Climate Change on Water Resources Components, Drought and Wheat Yield in Semiarid Regions: Karkheh River Basin in Iran. Hydrol. Process. 28 (4), 2018–2032. doi:10.1002/hyp.9747
Atchley, A. L., and Maxwell, R. M. (2011). Influences of Subsurface Heterogeneity and Vegetation Cover on Soil Moisture, Surface Temperature and Evapotranspiration at Hillslope Scales. Hydrogeol. J. 19 (2), 289–305. doi:10.1007/s10040-010-0690-1
Awotwi, A., Anornu, G. K., Quaye-Ballard, J. A., Annor, T., Forkuo, E. K., Harris, E., et al. (2019). Water Balance Responses to Land-Use/land-Cover Changes in the Pra River Basin of Ghana, 1986–2025. Catena 182, 104129. doi:10.1016/j.catena.2019.104129
Baker, T. J., and Miller, S. N. (2013). Using the Soil and Water Assessment Tool (SWAT) to Assess Land Use Impact on Water Resources in an East African Watershed. J. Hydrol. 486, 100–111. doi:10.1016/j.jhydrol.2013.01.041
Berg, A., Findell, K., Lintner, B., Giannini, A., Seneviratne, S. I., van den Hurk, B., et al. (2016). Land-atmosphere Feedbacks Amplify Aridity Increase over Land under Global Warming. Nat. Clim Change 6 (9), 869–874. doi:10.1038/nclimate3029
Chang, M., Fan, S., Fan, Q., Chen, W., Zhang, Y., Wang, Y., et al. (2014). Impact of Refined Land Surface Properties on the Simulation of a Heavy Convective Rainfall Process in the Pearl River Delta Region, China. Asia-Pacific J. Atmos. Sci. 50 (S1), 645–655. doi:10.1007/s13143-014-0052-3
Chen, J., Yu, Z., Zhu, Y., and Yang, C. (2011). Relationship between Land Use and Evapotranspiration-A Case Study of the Wudaogou Area in Huaihe River basin. Procedh. Environ. Sci. 10, 491–498. doi:10.1016/j.proenv.2011.09.080
Chen, L., Wang, Y., Touati, B., Guan, H., Leng, G., Liu, W., et al. (2018). Temporal Characteristics Detection and Attribution Analysis of Hydrological Time-Series Variation in the Seagoing River of Southern China under Environmental Change. Acta Geophys. 66 (5), 1151–1170. doi:10.1007/s11600-018-0198-y
Chen, Q., Chen, H., Wang, J., Zhao, Y., Chen, J., and Xu, C. (2019). Impacts of Climate Change and Land-Use Change on Hydrological Extremes in the Jinsha River Basin. Water 11, 1398. doi:10.3390/w11071398
Cheng, G., Li, Z., Wang, X., Fan, Y., Piwowar, J., Huang, G., et al. (2018). Hydrologic Impacts of Ensemble-RCM-Projected Climate Changes in the Athabasca River Basin, Canada. J. Hydrometeorol. 19 (12), 1953–1971. doi:10.1175/JHM-D-17-0232.1
Coron, L., Andréassian, V., Perrin, C., Lerat, J., Vaze, J., Bourqui, M., et al. (2012). Crash Testing Hydrological Models in Contrasted Climate Conditions: An experiment on 216 Australian Catchments. Water Resour. Res. 48, 5552. doi:10.1029/2011wr011721
Dakhlalla, A. O., and Parajuli, P. B. (2015). Evaluation of the Best Management Practices at the Watershed Scale to Attenuate Peak Streamflow under Climate Change Scenarios. Water Resour. Manage. 30 (3), 963–982. doi:10.1007/s11269-015-1202-9
Deng, Z., Zhang, X., Li, D., and Pan, G. (2014). Simulation of Land Use/land Cover Change and its Effects on the Hydrological Characteristics of the Upper Reaches of the Hanjiang Basin. Environ. Earth Sci. 73 (3), 1119–1132. doi:10.1007/s12665-014-3465-5
Dosdogru, F., Kalin, L., Wang, R., and Yen, H. (2020). Potential Impacts of Land Use/cover and Climate Changes on Ecologically Relevant Flows. J. Hydrol. 584, 124654. doi:10.1016/j.jhydrol.2020.124654
Gao, X., Yan, C., Wang, Y., Zhao, X., Zhao, Y., Sun, M., et al. (2020). Attribution Analysis of Climatic and Multiple Anthropogenic Causes of Runoff Change in the Loess Plateau-A Case‐study of the Jing River Basin. Land Degrad. Dev. 31 (13), 1622–1640. doi:10.1002/ldr.3557
Guo, J., Su, X., Singh, V., and Jin, J. (2016). Impacts of Climate and Land Use/Cover Change on Streamflow Using SWAT and a Separation Method for the Xiying River Basin in Northwestern China. Water 8, 192. doi:10.3390/w8050192
Guo, Y., Fang, G., Xu, Y.-P., Tian, X., and Xie, J. (2020). Identifying How Future Climate and Land Use/cover Changes Impact Streamflow in Xinanjiang Basin, East China. Sci. Total Environ. 710, 136275. doi:10.1016/j.scitotenv.2019.136275
He, Z., Zhao, W., Liu, H., and Chang, X. (2012). The Response of Soil Moisture to Rainfall Event Size in Subalpine Grassland and Meadows in a Semi-arid Mountain Range: A Case Study in Northwestern China's Qilian Mountains. J. Hydrol. 420-421, 183–190. doi:10.1016/j.jhydrol.2011.11.056
Holsten, A., Vetter, T., Vohland, K., and Krysanova, V. (2009). Impact of Climate Change on Soil Moisture Dynamics in Brandenburg with a Focus on Nature Conservation Areas. Ecol. Model. 220 (17), 2076–2087. doi:10.1016/j.ecolmodel.2009.04.038
Hu, J., Ma, J., Nie, C., Xue, L., Zhang, Y., Ni, F., et al. (2020). Attribution Analysis of Runoff Change in Min-Tuo River Basin Based on SWAT Model Simulations, China. Sci. Rep. 10 (1), 2900. doi:10.1038/s41598-020-59659-z
Iqbal, M., Dahri, Z., Querner, E., Khan, A., and Hofstra, N. (2018). Impact of Climate Change on Flood Frequency and Intensity in the Kabul River Basin. Geosciences 8, 114. doi:10.3390/geosciences8040114
Ji, L., and Duan, K. (2019). What Is the Main Driving Force of Hydrological Cycle Variations in the Semiarid and Semi-humid Weihe River Basin, China? Sci. Total Environ. 684, 254–264. doi:10.1016/j.scitotenv.2019.05.333
Jiang, C., and Wang, F. (2016). Temporal Changes of Streamflow and its Causes in the Liao River Basin over the Period of 1953-2011, Northeastern China. Catena 145, 227–238. doi:10.1016/j.catena.2016.06.015
Jiang, T., Chen, Y. D., Xu, C.-y., Chen, X., Chen, X., and Singh, P. (2007). Comparison of Hydrological Impacts of Climate Change Simulated by Six Hydrological Models in the Dongjiang Basin, South China. J. Hydrol. 336 (3-4), 316–333. doi:10.1016/j.jhydrol.2007.01.010
Jiang, Z., Shi, H., Liu, S., Zhou, Z., Wang, Y., and Cai, H. (2021). Evolution Characteristics of Potential Evapotranspiration over the Three-River Headwaters Region. Hydrol. Sci. J. 66 (10), 1552–1566. doi:10.1080/02626667.2021.1957105
Kundu, S., Khare, D., and Mondal, A. (2017). Past, Present and Future Land Use Changes and Their Impact on Water Balance. J. Environ. Manage. 197, 582–596. doi:10.1016/j.jenvman.2017.04.018
Lamichhane, S., and Shakya, N. M. (2019). Integrated Assessment of Climate Change and Land Use Change Impacts on Hydrology in the Kathmandu Valley Watershed, Central Nepal. Water 11, 2059. doi:10.3390/w11102059
Li, T., and Wen, X. (2018). Local Ecological Footprint Dynamics in the Construction of the Three Gorges Dam. Resour. Conserv. Recycling 132, 314–323. doi:10.1016/j.resconrec.2017.05.006
Li, Z., Liu, W.-z., Zhang, X.-c., and Zheng, F.-l. (2009). Impacts of Land Use Change and Climate Variability on Hydrology in an Agricultural Catchment on the Loess Plateau of China. J. Hydrol. 377 (1-2), 35–42. doi:10.1016/j.jhydrol.2009.08.007
Li G, G., Zhang, F., Jing, Y., Liu, Y., and Sun, G. (2017). Response of Evapotranspiration to Changes in Land Use and Land Cover and Climate in China during 2001-2013. Sci. Total Environ. 596-597, 256–265. doi:10.1016/j.scitotenv.2017.04.080
Li Q, Q., Sun, Y., Yuan, W., Lyu, S., and Wan, F. (2017). Streamflow Responses to Climate Change and LUCC in a Semi-arid Watershed of Chinese Loess Plateau. J. Arid Land 9 (4), 609–621. doi:10.1007/s40333-017-0095-2
Li B, B., Shi, X., Lian, L., Chen, Y., Chen, Z., and Sun, X. (2020). Quantifying the Effects of Climate Variability, Direct and Indirect Land Use Change, and Human Activities on Runoff. J. Hydrol. 584, 124684. doi:10.1016/j.jhydrol.2020.124684
Li Z, Z., Xu, Y., Sun, Y., Wu, M., and Zhao, B. (2020). Urbanization-Driven Changes in Land-Climate Dynamics: A Case Study of Haihe River Basin, China. Remote Sensing 12, 2701. doi:10.3390/rs12172701
Li, D. (2014). Assessing the Impact of Interannual Variability of Precipitation and Potential Evaporation on Evapotranspiration. Adv. Water Resour. 70, 1–11. doi:10.1016/j.advwatres.2014.04.012
Liang, J., Liu, Q., Zhang, H., Li, X., Qian, Z., Lei, M., et al. (2020). Interactive Effects of Climate Variability and Human Activities on Blue and green Water Scarcity in Rapidly Developing Watershed. J. Clean. Prod. 265, 121834. doi:10.1016/j.jclepro.2020.121834
Liu, J., Shanguan, D., Liu, S., and Ding, Y. (2018). Evaluation and Hydrological Simulation of CMADS and CFSR Reanalysis Datasets in the Qinghai-Tibet Plateau. Water 10, 513. doi:10.3390/w10040513
Liu, Y. J., Chen, J., and Pan, T. (2019). Analysis of Changes in Reference Evapotranspiration, Pan Evaporation, and Actual Evapotranspiration and Their Influencing Factors in the North China Plain during 1998-2005. Earth Space Sci. 6 (8), 1366–1377. doi:10.1029/2019ea000626
Liu, S., Yao, Y., Kuang, X., and Zheng, C. (2021). A Preliminary Investigation on the Climate-Discharge Relationship in the Upper Region of the Yarlung Zangbo River basin. J. Hydrol. 603, 127066. doi:10.1016/j.jhydrol.2021.127066
Liu F, F., Zhao, Y., Muhammad, R., Liu, X., and Chen, M. (2020). Impervious Surface Expansion: A Key Indicator for Environment and Urban Agglomeration-A Case Study of Guangdong-Hong Kong-Macao Greater Bay Area by Using Landsat Data. J. Sensors 2020, 1–21. doi:10.1155/2020/3896589
Liu S, S., Shi, H., and Sivakumar, B. (2020). Socioeconomic Drought under Growing Population and Changing Climate: A New index Considering the Resilience of a Regional Water Resources System. J. Geophys. Res. Atmos. 125 (15), e2020JD033005. doi:10.1029/2020jd033005
Liu Z, Z., Cuo, L., Li, Q., Liu, X., Ma, X., Liang, L., et al. (2020). Impacts of Climate Change and Land Use/Cover Change on Streamflow in Beichuan River Basin in Qinghai Province, China. Water 12, 1198. doi:10.3390/w12041198
Luo, Y., Gao, P., and Mu, X. (2021). Influence of Meteorological Factors on the Potential Evapotranspiration in Yanhe River Basin, China. Water 13, 1222. doi:10.3390/w13091222
Lyu, R., Zhang, J., Xu, M., and Li, J. (2018). Impacts of Urbanization on Ecosystem Services and Their Temporal Relations: A Case Study in Northern Ningxia, China. Land Use Policy 77, 163–173. doi:10.1016/j.landusepol.2018.05.022
Ma, K., Huang, X., Liang, C., Zhao, H., Zhou, X., and Wei, X. (2020). Effect of Land Use/cover Changes on Runoff in the Min River Watershed. River Res. Applic. 36 (5), 749–759. doi:10.1002/rra.3608
Maghsood, F. F., Moradi, H., Massah Bavani, A. R., Panahi, M., Berndtsson, R., and Hashemi, H. (2019). Climate Change Impact on Flood Frequency and Source Area in Northern Iran under CMIP5 Scenarios. Water 11, 273. doi:10.3390/w11020273
Mandal, U., Sena, D. R., Dhar, A., Panda, S. N., Adhikary, P. P., and Mishra, P. K. (2021). Assessment of Climate Change and its Impact on Hydrological Regimes and Biomass Yield of a Tropical River basin. Ecol. Indicators 126, 107646. doi:10.1016/j.ecolind.2021.107646
Marhaento, H., Booij, M. J., Rientjes, T. H. M., and Hoekstra, A. Y. (2019). Sensitivity of Streamflow Characteristics to Different Spatial Land-Use Configurations in Tropical Catchment. J. Water Resour. Plann. Manage. 145, 04019054. doi:10.1061/(asce)wr.1943-5452.0001122
Martínez-Retureta, R., Aguayo, M., Stehr, A., Sauvage, S., Echeverría, C., and Sánchez-Pérez, J.-M. (2020). Effect of Land Use/Cover Change on the Hydrological Response of a Southern Center Basin of Chile. Water 12, 302. doi:10.3390/w12010302
Mendoza-Ponce, A., Corona-Núñez, R., Kraxner, F., Leduc, S., and Patrizio, P. (2018). Identifying Effects of Land Use Cover Changes and Climate Change on Terrestrial Ecosystems and Carbon Stocks in Mexico. Glob. Environ. Change 53, 12–23. doi:10.1016/j.gloenvcha.2018.08.004
Meng, F., Liu, T., Wang, H., Luo, M., Duan, Y., and Bao, A. (2018). An Alternative Approach to Overcome the Limitation of HRUs in Analyzing Hydrological Processes Based on Land Use/Cover Change. Water 10, 434. doi:10.3390/w10040434
Meng, X., Zhang, X., Yang, M., Wang, H., Chen, J., Pan, Z., et al. (2019). Application and Evaluation of the China Meteorological Assimilation Driving Datasets for the SWAT Model (CMADS) in Poorly Gauged Regions in Western China. Water 11, 2171. doi:10.3390/w11102171
Narsimlu, B., Gosain, A. K., Chahar, B. R., Singh, S. K., and Srivastava, P. K. (2015). SWAT Model Calibration and Uncertainty Analysis for Streamflow Prediction in the Kunwari River Basin, India, Using Sequential Uncertainty Fitting. Environ. Process. 2 (1), 79–95. doi:10.1007/s40710-015-0064-8
O’Gorman, P. A. (2012). Sensitivity of Tropical Precipitation Extremes to Climate Change. Nat. Geosci. 5 (10), 697–700. doi:10.1038/ngeo1568
Piniewski, M., Szcześniak, M., Kundzewicz, Z. W., Mezghani, A., and Hov, Ø. (2017). Changes in Low and High Flows in the Vistula and the Odra Basins: Model Projections in the European-scale Context. Hydrol. Process. 31 (12), 2210–2225. doi:10.1002/hyp.11176
Rouholahnejad, E., Abbaspour, K. C., Vejdani, M., Srinivasan, R., Schulin, R., and Lehmann, A. (2012). A Parallelization Framework for Calibration of Hydrological Models. Environ. Model. Softw. 31, 28–36. doi:10.1016/j.envsoft.2011.12.001
Shi, H., and Chen, J. (2018). Characteristics of Climate Change and its Relationship with Land Use/cover Change in Yunnan Province, China. Int. J. Climatol. 38 (5), 2520–2537. doi:10.1002/joc.5404
Shi, H., Chen, J., Wang, K., and Niu, J. (2018). A New Method and a New index for Identifying Socioeconomic Drought Events under Climate Change: a Case Study of the East River basin in China. Sci. Total Environ. 616-617, 363–375. doi:10.1016/j.scitotenv.2017.10.321
Shi, H., Chen, J., Li, T., and Wang, G. (2020). A New Method for Estimation of Spatially Distributed Rainfall through Merging Satellite Observations, Raingauge Records, and Terrain Digital Elevation Model Data. J. Hydro-Environ. Res. 28, 1–14. doi:10.1016/j.jher.2017.10.006
Shrestha, S., Sharma, S., Sharma, S., Gupta, R., and Bhattarai, R. (2019). Impact of Global Climate Change on Stream Low Flows: A Case Study of the Great Miami River Watershed, Ohio, USA. Int. J. Agric. Biol. Eng. 12 (1), 84–95. doi:10.25165/j.ijabe.20191201.4486
Song, Y., Zhang, J., Meng, X., Zhou, Y., Lai, Y., and Cao, Y. (2020). Comparison Study of Multiple Precipitation Forcing Data on Hydrological Modeling and Projection in the Qujiang River Basin. Water 12, 2626. doi:10.3390/w12092626
Sun, P., Wu, Y., Wei, X., Sivakumar, B., Qiu, L., Mu, X., et al. (2020). Quantifying the Contributions of Climate Variation, Land Use Change, and Engineering Measures for Dramatic Reduction in Streamflow and Sediment in a Typical Loess Watershed, China. Ecol. Eng. 142, 105611. doi:10.1016/j.ecoleng.2019.105611
Tan, M. L., Gassman, P. W., Yang, X., and Haywood, J. (2020). A Review of SWAT Applications, Performance and Future Needs for Simulation of Hydro-Climatic Extremes. Adv. Water Resour. 143, 103662. doi:10.1016/j.advwatres.2020.103662
Toride, K., Cawthorne, D. L., Ishida, K., Kavvas, M. L., and Anderson, M. L. (2018). Long-term Trend Analysis on Total and Extreme Precipitation over Shasta Dam Watershed. Sci. Total Environ. 626, 244–254. doi:10.1016/j.scitotenv.2018.01.004
Touseef, M., Chen, L. H., Masud, T., Khan, A., Yang, K. P., Shahzad, A., et al. (2020). Assessment of the Future Climate Change Projections on Streamflow Hydrology and Water Availability over Upper Xijiang River Basin, China. Appl. Sci. Basel 10, 3671. doi:10.3390/app10113671
Tu, X., Singh, P., Chen, X., Chen, L., Zhang, Q., and Zhao, Y. (2015). Intra-annual Distribution of Streamflow and Individual Impacts of Climate Change and Human Activities in the Dongijang River Basin, China. Water Resour. Manage. 29 (8), 2677–2695. doi:10.1007/s11269-015-0963-5
Wan, R., and Yang, G. (2007). Influence of Land Use/cover Change on Storm Runoff-A Case Study of Xitiaoxi River Basin in Upstream of Taihu Lake Watershed. Chin. Geogr. Sci. 17 (4), 349–356. doi:10.1007/s11769-007-0349-6
Wang, H., and Stephenson, S. R. (2018). Quantifying the Impacts of Climate Change and Land Use/cover Change on Runoff in the Lower Connecticut River Basin. Hydrol. Process. 32 (9), 1301–1312. doi:10.1002/hyp.11509
Wang, J., Hong, Y., Gourley, J., Adhikari, P., Li, L., and Su, F. (2010). Quantitative Assessment of Climate Change and Human Impacts on Long-Term Hydrologic Response: a Case Study in a Sub-basin of the Yellow River, China. Int. J. Climatol. 30 (14), 2130–2137. doi:10.1002/joc.2023
Wang, Y., Ding, Y., Ye, B., Liu, F., Wang, J., and Wang, J. (2012). Contributions of Climate and Human Activities to Changes in Runoff of the Yellow and Yangtze Rivers from 1950 to 2008. Sci. China Earth Sci. 56 (8), 1398–1412. doi:10.1007/s11430-012-4505-1
Wang, Z., Xie, P., Lai, C., Chen, X., Wu, X., Zeng, Z., et al. (2017). Spatiotemporal Variability of Reference Evapotranspiration and Contributing Climatic Factors in China during 1961-2013. J. Hydrol. 544, 97–108. doi:10.1016/j.jhydrol.2016.11.021
Wang, X., Cong, P., Jin, Y., Jia, X., Wang, J., and Han, Y. (2021). Assessing the Effects of Land Cover Land Use Change on Precipitation Dynamics in Guangdong–Hong Kong–Macao Greater Bay Area from 2001 to 2019. Remote Sensing 13, 1135. doi:10.3390/rs13061135
Wang N, N., Liu, W., Sun, F., Yao, Z., Wang, H., and Liu, W. (2020). Evaluating Satellite-Based and Reanalysis Precipitation Datasets with Gauge-Observed Data and Hydrological Modeling in the Xihe River Basin, China. Atmos. Res. 234, 104746. doi:10.1016/j.atmosres.2019.104746
Wang Q, Q., Xu, Y., Xu, Y., Wu, L., Wang, Y., and Han, L. (2018). Spatial Hydrological Responses to Land Use and Land Cover Changes in a Typical Catchment of the Yangtze River Delta Region. Catena 170, 305–315. doi:10.1016/j.catena.2018.06.022
Wang Q, Q., Xu, Y., Wang, Y., Zhang, Y., Xiang, J., Xu, Y., et al. (2020). Individual and Combined Impacts of Future Land-Use and Climate Conditions on Extreme Hydrological Events in a Representative basin of the Yangtze River Delta, China. Atmos. Res. 236, 104805. doi:10.1016/j.atmosres.2019.104805
Wang QF, Q. F., Qi, J. Y., Wu, H., Zeng, Y., Shui, W., Zeng, J. Y., et al. (2020). Freeze-Thaw Cycle Representation Alters Response of Watershed Hydrology to Future Climate Change. Catena 195, 104767. doi:10.1016/j.catena.2020.104767
Wang X, X., Engel, B., Yuan, X., and Yuan, P. (2018). Variation Analysis of Streamflows from 1956 to 2016 along the Yellow River, China. Water 10, 1231. doi:10.3390/w10091231
Wu, Y., and Chen, J. (2013). Analyzing the Water Budget and Hydrological Characteristics and Responses to Land Use in a Monsoonal Climate River basin in South China. Environ. Manage. 51 (6), 1174–1186. doi:10.1007/s00267-013-0045-5
Wu, J., Miao, C., Zhang, X., Yang, T., and Duan, Q. (2017). Detecting the Quantitative Hydrological Response to Changes in Climate and Human Activities. Sci. Total Environ. 586, 328–337. doi:10.1016/j.scitotenv.2017.02.010
Wu, J., Chen, X., Yu, Z., Yao, H., Li, W., and Zhang, D. (2019). Assessing the Impact of Human Regulations on Hydrological Drought Development and Recovery Based on a ‘simulated-Observed’ Comparison of the SWAT Model. J. Hydrol. 577, 123990. doi:10.1016/j.jhydrol.2019.123990
Xu, X., Wang, Y.-C., Kalcic, M., Muenich, R. L., Yang, Y. C. E., and Scavia, D. (2019). Evaluating the Impact of Climate Change on Fluvial Flood Risk in a Mixed-Use Watershed. Environ. Model. Softw. 122, 104031. doi:10.1016/j.envsoft.2017.07.013
Xu, H., Ren, Y., Zheng, H., Ouyang, Z., and Jiang, B. (2020). Analysis of Runoff Trends and Drivers in the Haihe River Basin, China. Int. J. Environ. Res. Public Health 17, 1577. doi:10.3390/ijerph17051577
Xue, B., Zhang, H., Wang, Y., Tan, Z., Zhu, Y., and Shrestha, S. (2021). Modeling Water Quantity and Quality for a Typical Agricultural plain basin of Northern China by a Coupled Model. Sci. Total Environ. 790, 148139. doi:10.1016/j.scitotenv.2021.148139
Yan, R., Zhang, X., Yan, S., Zhang, J., and Chen, H. (2018). Spatial Patterns of Hydrological Responses to Land Use/cover Change in a Catchment on the Loess Plateau, China. Ecol. Indicators 92, 151–160. doi:10.1016/j.ecolind.2017.04.013
Yang, J., Reichert, P., Abbaspour, K. C., Xia, J., and Yang, H. (2008). Comparing Uncertainty Analysis Techniques for a SWAT Application to the Chaohe Basin in China. J. Hydrol. 358 (1-2), 1–23. doi:10.1016/j.jhydrol.2008.05.012
Yang, L., Feng, Q., Yin, Z., Wen, X., Si, J., Li, C., et al. (2017). Identifying Separate Impacts of Climate and Land Use/cover Change on Hydrological Processes in Upper Stream of Heihe River, Northwest China. Hydrol. Process. 31 (5), 1100–1112. doi:10.1002/hyp.11098
Yang, W., Long, D., and Bai, P. (2019). Impacts of Future Land Cover and Climate Changes on Runoff in the Mostly Afforested River basin in North China. J. Hydrol. 570, 201–219. doi:10.1016/j.jhydrol.2018.12.055
Yang, G., Zhao, Y., Xing, H., Fu, Y., Liu, G., Kang, X., et al. (2020). Understanding the Changes in Spatial Fairness of Urban Greenery Using Time-Series Remote Sensing Images: A Case Study of Guangdong-Hong Kong-Macao Greater Bay. Sci. Total Environ. 715, 136763. doi:10.1016/j.scitotenv.2020.136763
Yin, J., He, F., Xiong, Y. J., and Qiu, G. Y. (2017). Effects of Land Use/land Cover and Climate Changes on Surface Runoff in a Semi-humid and Semi-arid Transition Zone in Northwest China. Hydrol. Earth Syst. Sci. 21 (1), 183–196. doi:10.5194/hess-21-183-2017
Yonaba, R., Biaou, A. C., Koïta, M., Tazen, F., Mounirou, L. A., Zouré, C. O., et al. (2021). A Dynamic Land Use/land Cover Input Helps in Picturing the Sahelian Paradox: Assessing Variability and Attribution of Changes in Surface Runoff in a Sahelian Watershed. Sci. Total Environ. 757, 143792. doi:10.1016/j.scitotenv.2020.143792
Zeng, F., Ma, M.-G., Di, D.-R., and Shi, W.-Y. (2020). Separating the Impacts of Climate Change and Human Activities on Runoff: A Review of Method and Application. Water 12, 2201. doi:10.3390/w12082201
Zhai, R., and Tao, F. (2017). Contributions of Climate Change and Human Activities to Runoff Change in Seven Typical Catchments across China. Sci. Total Environ. 605-606, 219–229. doi:10.1016/j.scitotenv.2017.06.210
Zhang, Y., and Liang, S. (2018). Impacts of Land Cover Transitions on Surface Temperature in China Based on Satellite Observations. Environ. Res. Lett. 13, 024010. doi:10.1088/1748-9326/aa9e93
Zhang, L., Nan, Z., Yu, W., Zhao, Y., and Xu, Y. (2018). Comparison of Baseline Period Choices for Separating Climate and Land Use/land Cover Change Impacts on Watershed Hydrology Using Distributed Hydrological Models. Sci. Total Environ. 622-623, 1016–1028. doi:10.1016/j.scitotenv.2017.12.055
Zhang, S., Fang, C., Kuang, W., and Sun, F. (2019a). Comparison of Changes in Urban Land Use/Cover and Efficiency of Megaregions in China from 1980 to 2015. Remote Sensing 11, 1834. doi:10.3390/rs11151834
Zhang, S., Wu, Y., Sivakumar, B., Mu, X., Zhao, F., Sun, P., et al. (2019b). Climate Change-Induced Drought Evolution over the Past 50 Years in the Southern Chinese Loess Plateau. Environ. Model. Softw. 122, 104519. doi:10.1016/j.envsoft.2019.104519
Zhang J, J., Yu, L., Li, X., Zhang, C., Shi, T., Wu, X., et al. (2020). Exploring Annual Urban Expansions in the Guangdong-Hong Kong-Macau Greater Bay Area: Spatiotemporal Features and Driving Factors in 1986–2017. Remote Sensing 12, 2615. doi:10.3390/rs12162615
Zhang K, K., Ruben, G. B., Li, X., Li, Z., Yu, Z., Xia, J., et al. (2020). A Comprehensive Assessment Framework for Quantifying Climatic and Anthropogenic Contributions to Streamflow Changes: A Case Study in a Typical Semi-arid North China basin. Environ. Model. Softw. 128, 104704. doi:10.1016/j.envsoft.2020.104704
Zhang L, L., Meng, X., Wang, H., Yang, M., and Cai, S. (2020). Investigate the Applicability of CMADS and CFSR Reanalysis in Northeast China. Water 12, 996. doi:10.3390/w12040996
Zhao, P., Lü, H., Yang, H., Wang, W., and Fu, G. (2019). Impacts of Climate Change on Hydrological Droughts at basin Scale: A Case Study of the Weihe River Basin, China. Quat. Int. 513, 37–46. doi:10.1016/j.quaint.2019.02.022
Zhao, Y., Liu, S., and Shi, H. (2021). Impacts of Dams and Reservoirs on Local Climate Change: a Global Perspective. Environ. Res. Lett. 16, 104043. doi:10.1088/1748-9326/ac263c
Zhou, Z., Ding, Y., Shi, H., Cai, H., Fu, Q., Liu, S., et al. (2020a). Analysis and Prediction of Vegetation Dynamic Changes in China: Past, Present and Future. Ecol. Indicators 117, 106642. doi:10.1016/j.ecolind.2020.106642
Zhou, Z., Shi, H., Fu, Q., Li, T., Gan, T. Y., Liu, S., et al. (2020b). Is the Cold Region in Northeast China Still Getting Warmer under Climate Change Impact? Atmos. Res. 237, 104864. doi:10.1016/j.atmosres.2020.104864
Zhou, X., Huang, G., Li, Y., Lin, Q., Yan, D., and He, X. (2021). Dynamical Downscaling of Temperature Variations over the Canadian Prairie Provinces under Climate Change. Remote Sensing 13, 4350. doi:10.3390/rs13214350
Zhou X, X., Huang, G., Piwowar, J., Fan, Y., Wang, X., Li, X., et al. (2018). Hydrologic Impacts of Ensemble-RCM-Projected Climate Changes in the Athabasca River Basin, Canada. J. Hydrometeorol. 19, 1953. doi:10.1175/jhm-d-17-0232.1
Zhou YL, Y. L., Lai, C. G., Wang, Z. L., Chen, X. H., Zeng, Z. Y., Chen, J. C., et al. (2018). Quantitative Evaluation of the Impact of Climate Change and Human Activity on Runoff Change in the Dongjiang River Basin, China. Water 10, 571. doi:10.3390/w10050571
Keywords: climate change, land use/cover change, regional hydrological processes, greater bay area, SWAT
Citation: Tan X, Liu S, Tian Y, Zhou Z, Wang Y, Jiang J and Shi H (2022) Impacts of Climate Change and Land Use/Cover Change on Regional Hydrological Processes: Case of the Guangdong-Hong Kong-Macao Greater Bay Area. Front. Environ. Sci. 9:783324. doi: 10.3389/fenvs.2021.783324
Received: 26 September 2021; Accepted: 13 December 2021;
Published: 04 January 2022.
Edited by:
Huimin Lei, Tsinghua University, ChinaCopyright © 2022 Tan, Liu, Tian, Zhou, Wang, Jiang and Shi. This is an open-access article distributed under the terms of the Creative Commons Attribution License (CC BY). The use, distribution or reproduction in other forums is permitted, provided the original author(s) and the copyright owner(s) are credited and that the original publication in this journal is cited, in accordance with accepted academic practice. No use, distribution or reproduction is permitted which does not comply with these terms.
*Correspondence: Suning Liu, dTMwMDI5MDZAY29ubmVjdC5oa3UuaGs=; Haiyun Shi, c2hpaHlAc3VzdGVjaC5lZHUuY24=
Disclaimer: All claims expressed in this article are solely those of the authors and do not necessarily represent those of their affiliated organizations, or those of the publisher, the editors and the reviewers. Any product that may be evaluated in this article or claim that may be made by its manufacturer is not guaranteed or endorsed by the publisher.
Research integrity at Frontiers
Learn more about the work of our research integrity team to safeguard the quality of each article we publish.