- 1School of Business, East China University of Science and Technology, Shanghai, China
- 2School of Statistics and Mathematics, Shanghai Lixin University of Accounting and Finance, Shanghai, China
The process of land urbanization may result in a great change in land use structure, land use intensity, and efficiency, which could further lead to an increase in carbon dioxide (CO2) emissions. Despite rich literature on the link between urbanization and CO2 emissions, the mechanism through which land urbanization promotes CO2 emissions reductions has not been fully investigated. To address this gap, this study theoretically and empirically explores the mechanism of land urbanization’s influence on CO2 emissions by integrating land use optimization and high-quality industrial development into a unified framework. Firstly, the theoretical mechanism analysis indicates that low-level industrial development and land use management promote the increase of CO2 emissions per unit of land at the extensive land use stage; however, high-quality industrial development and land use optimization lower CO2 emissions per unit of land at the intensive land use stage. Subsequently, a STIRPAT model and a spatial adaptive semi-parametric model are employed to verify the relationship between the land urbanization rate and total CO2 emissions. The results indicate that the land urbanization rate and total CO2 emissions present an inverted U-shaped relationship. In addition, the mediating mechanism of the advanced industrial structure, CO2 emissions per unit of GDP, and CO2 emissions per unit of land, are studied using the mediating effect model. Results indicate that CO2 emissions reduction can be achieved by promoting the advanced industrial structure, reducing CO2 emissions per unit of GDP or reducing CO2 emissions per unit of land. Ultimately, this study showed that the Chinese government may reduce CO2 emissions by promoting land use structure optimization, land use intensity regulation, land use efficiency improvement, and adjusting energy consumption structure, upgrading industrial structure, and promoting emission efficiency technologies.
Introduction
Urbanization involves an important change: the conversion of large areas of cultivated land into urban land. This process is called “land urbanization” (Zhang and Xu, 2017). China has greatly promoted economic growth through land urbanization and land resource allocation. However, the rapid expansion of urban land can lead to land use/cover change (LUCC), unreasonable land allocation structures, and uncontrolled land development intensity (Yang et al., 2019). Important to note here is that LUCC is the main driving force for carbon storage in terrestrial ecosystems (Chuai et al., 2014). During the process of land urbanization, a large amount of agricultural land is converted into construction land.1 For instance, the area of urban construction land in China increased from 20,877 km2 in 1999 to 55,155.5 km2 in 2017—an increase of 164%. The proportion of secondary industrial land to construction land in China has always been maintained at approximately 20%, which greatly exceeds the international average of 5–8%. In cities with more developed manufacturing industries, such as those in the Pearl River Delta and the Yangtze River Delta, the proportion of secondary industrial land generally exceeds 40%. A high proportion of secondary industrial land leads to slow industrial structure upgrading, unclean energy consumption structures, and low energy efficiency, which cause more carbon emissions (CO2) to be released into the atmosphere (Zhou et al., 2019b). Furthermore, China’s land development intensity also exceeds a reasonable level, and the levels of economically developed cities such as Shanghai and Shenzhen are even close to 50%. Excessive land development intensity seriously impacts the ecosystem’s balance, which in turn affects carbon sequestration (Xie et al., 2018; Li et al., 2018). Since unreasonable land urbanization has increased carbon emissions, there is a need to investigate in-depth the mechanism through which land urbanization affects CO2 emissions. Research on exploring this mechanism is conducive for promoting the coordinated development of urbanization and CO2 emissions reduction through land use optimization and industrial structure adjustment.
The links between urbanization and CO2 emissions have recently been extensively investigated by existing research (Lai et al., 2016; Chuai et al., 2016). Previous studies have also addressed multiple effects of different aspects of urbanization, including economic, population, land, and social urbanization, on CO2 emissions (Zhou et al., 2019a). Generally, previous studies on the relationship between urbanization and carbon emissions focused on four lines. The first line of studies assert that rapid urbanization increases CO2 emissions both in the short and long-run (Sheng and Guo, 2016), while the second line of studies insist that urbanization can contribute to declines in the carbon emission scale, carbon emissions per capita, and carbon intensity (Yao et al., 2018). The third line of studies supposed that the urbanization exerts no significant effect in the carbon emissions (Rafiq et al., 2016; Behera and Dash, 2017). Finally, the results of the fourth line of studies show an inverted U-shaped relationship between urbanization and CO2 emissions (Martínez-Zarzoso and Maruotti, 2011; Zhang et al., 2017). Furthermore, a few mediating variables (such as technological progress, industrial structures, energy consumption structure, and foreign direct investment) have also been investigated when analyzing the relationship between urbanization and CO2 emissions (Wang et al., 2013; Wang et al., 2018b). In addition, certain studies are related to regional and industry-based heterogeneity and spatial spillovers (Zhang et al., 2016; Liu and Liu, 2019). Despite various studies explored the effect of urbanization on CO2 emissions and the mechanism to achieve the coordination of economic growth and carbon mitigation (Bekun et al., 2019; Bekun et al., 2021a), two limitations continue to restrict our understanding of sustainable development. First, the mechanism of the effect of land urbanization on CO2 emissions reduction has not yet been thoroughly explored, which limits the possibility to achieve a win-win situation of economic development and carbon mitigation through land use optimization and industrial structure adjustment. Second, previous studies employed parametric econometric models, which may have led to model setting misspecification. To fill these research gaps, this study theoretically explores the mechanism of land urbanization to promote CO2 emissions reduction, and verifies it empirically by employing a semiparametric model and mediation effect model.
In fact, the impact mechanism of land urbanization on carbon emissions is greatly different from population urbanization or economic urbanization. The urbanization in the existing literature generally refers to population urbanization or economic urbanization. The research from the perspective of population urbanization or economic urbanization is mainly to explore the impact of changes in residential consumption, industrial structure, and technical efficiency on carbon emissions after population or industry agglomeration in cities. It is worth noting that land urbanization is very different from population urbanization. The impact of land urbanization on CO2 emissions is affected by natural, economic, social, and other factors. On the one hand, in the process of land urbanization, changes in land use types will cause changes in direct carbon emissions. For example, if land is transformed from forest land, wetland, etc. to urban construction land, the carbon emission coefficient will be greatly increased. On the other hand, the land urbanization process will also cause changes in the industrial structure, technical efficiency, energy use efficiency, and carbon emission efficiency by anthropogenic activities carried on the land elements, which in turn affects the level of indirect carbon emissions. Accordingly, it is essential to explore the mechanism of land urbanization affecting CO2 emissions thoroughly regarding factors such as land use change, and changes in industrial structure and technological efficiency caused by human activities carried on the land elements.
LUCC and anthropogenic activities carried out on land are the two main drivers of terrestrial ecosystem carbon storage. In the process of land use conversion from high to low vegetation biomass, carbon is released into the atmosphere; this affects carbon emission levels (Peters et al., 2019). With the development of land urbanization, more and more cultivated lands, forest lands, and grass lands have been converted to urban construction land; put differently, such lands are converted from carbon sinks to carbon sources (Chuai et al., 2013; Chuai et al., 2014; Chuai et al., 2016). Existing studies have extensively explored the influence of LUCC on CO2 emissions (Muñoz-Rojas et al., 2011; Chuai et al., 2013; Dang et al., 2014; Chuai et al., 2016; Bossio et al., 2020). However, the impact of effective land use management and industrial structure adjustment on CO2 emissions reduction has received relatively less attention. Sustainable land use management promotes optimization of land use structure, reasonable control of land use intensity, and improvement of land use efficiency through operating mechanisms, price systems, land ownership systems, and so on (Bateman et al., 2013; Cavender-Bares et al., 2015). Notably, land use structure optimization, land use intensity regulation, and land use efficiency improvement all directly or indirectly affect the carbon emission process and carbon emission level (Cumming et al., 2014; Chen et al., 2020). However, existing research does not integrate these three perspectives and fails to fully explore the internal and mediating mechanisms of land urbanization that reduce CO2 emissions. Therefore, our study integrates factors such as land use structure optimization, reasonable control of land use intensity, and land use efficiency improvement into a complete theoretical framework to explore the mechanism of land urbanization promoting carbon mitigation. This is exactly where the most important innovation of this study lies.
This study makes two key contributions: First, this study explored in-depth the influential mechanism of land urbanization on CO2 emissions theoretically by integrating the land use optimization and high-quality industrial development into a unified framework. Second, this study applied a stochastic impact by regression on population, affluence and technology (STIRPAT) model and a spatially adaptive semi-parametric (SASP) model to investigate the relationship between land urbanization and CO2 emissions to accommodate stochastic factors and spatial heterogeneity. Additionally, this study employed a mediation effect model to investigate the role of advanced industrial structure, CO2 emissions per unit of GDP, and CO2 emissions per land regarding land urbanization’s effect on total CO2 emissions.
The rest of this paper is organized as follows. Section Theory provides the theoretical analysis, Section Methods describes the method, Section Data describes the data, Section Results presents the empirical results, Section Discussions discusses the results, and Section Conclusion and Policy Recommendations offers the conclusion.
Theory
In this section, we adopt the Kaemissions into fours aspects: Landya identity to decompose the driving factors of CO2 emissions into fours aspects: Land scale, land use structure, land use intensity, and carbon emission intensity (Grossman and Krueger, 1995; Wu et al., 2015). According to a country or region’s land use patterns, industrial structure, technological level, government regulation policies, and residents’ lifestyles, land urbanization can be roughly divided into two stages: extensive and intensive modes. This study explores the impact mechanism of land urbanization on CO2 from two stages: Extensive and intensive land use (Figure 1).
Kaya Decomposition to the Driving Factors of Carbon Dioxide Emissions
First, we adopt the Kaya identity to decompose the driving factors of CO2 emissions into fours aspects: Land scale, land use structure, land use intensity, and carbon emission intensity (Grossman and Krueger, 1995; Wu et al., 2015). The carbon dioxide emissions decomposition model based on land use is presented in Eq. 1. The total CO2 emissions are first decomposed into the carbon emissions of different land use types. Next, CO2 emissions on each land use type are decomposed into the product of the total land scale, land use structure, land use intensity, and carbon emission intensity. As a result, we are able to decompose carbon emissions per unit of land into the sum of the product of land use structure, land use intensity, and carbon emission intensity for different land use types, as shown in Eq. 2.
In Eqs 1, 2, Ct is the total CO2 emissions in period t, Cit represents the CO2 emissions of the i type of land use in period t, Lt is the total area of land in period t,
To reduce CO2 emissions per unit of land, it is essential to optimize the land use structure, reduce carbon emission intensity, and regulate the impact of land use intensity on CO2 emissions according to the carbon emission decomposition model. For the central government and local governments, the impact of land use on CO2 emissions can be reduced in two ways: 1) by optimizing the structure of spatial land use and 2) by innovating spatial land governance. Notably, CO2 emissions can be effectively reduced by controlling the expansion of urban construction land and the intensity of land development, compressing the scale and proportion of industrial and mining land, optimizing the structure of construction land, and optimizing the pattern of land development (Sadorsky, 2014). Simultaneously, we can promote the formation of low-carbon development by accelerating the transformation of land use types. The government should promote intensive land use in accordance with the principles of strictly controlling the total amount, revitalizing the inventory, optimizing the structure, and improving efficiency.
Extensive Land Urbanization Stage
When the economy is in the initial stage of urbanization and industrialization, the mode of land urbanization is mainly extensive (Jiang and Lin, 2012; Dong et al., 2019). At this stage, the industrial structure is dominated by high-carbon industries, and the company’s technical level and low energy efficiency have led to high carbon emission intensity. Moreover, the government’s efforts to reduce carbon emissions are relatively weak, and residents’ consumption preferences are not based on low-carbon and energy-saving products (Zhang and Da, 2015). All these factors lead to high levels of carbon emissions.
First, because the economic development stage is still in its infancy and the technical level is relatively not high, companies have mainly invested in high-carbon industries such as energy, power, chemical industry, and construction, thus making it difficult to upgrade and transform the industrial structure (Lu et al., 2019). Furthermore, the limitation of companies’ technology level leads to low energy utilization efficiency, which further leads to higher carbon emission intensity, thereby increasing companies’ carbon emission level.
Second, the government’s efforts to control carbon emission reduction are not effective enough, and reasonable carbon emission control policies have not been issued in neither industrial development nor land use (Yuyin and Jinxi, 2018). On the one hand, local government officials have to vigorously improve local economic performance based on economic performance appraisal and political promotion in the process of industrial development (Liu et al., 2020). From a rational point of view, local officials will not impose strict restrictions on the investment of companies in high-carbon industries, leading to a significant increase in carbon emissions. On the other hand, the government’s land use management methods are unreasonable. For example, a large number of low-carbon land, such as forest land and wetland, are converted into urban construction land, leading to a large increase in carbon emissions (Zhang et al., 2020). Since construction land is a net source of carbon emissions, the expansion of urban construction land has led to a surge in carbon emissions (Lai et al., 2016). Furthermore, improper land use management methods lead to a series of problems such as unreasonable land use structure, out of control of land use intensity, and low land use efficiency (Yue et al., 2017). Changes in the land allocation structure alter the carbon emission process and modify the energy consumption structure, thereby impacting the urban carbon emissions level. Excessive land development intensity will destroy the original carbon balance of the biosphere, affect the carbon emission process, and promote the increase of carbon emission levels (Li et al., 2021a). Land use efficiency affects energy use efficiency, which in turn influences the carbon emissions level.
Finally, residents’ awareness of energy saving and emission reduction is limited, which has also led to an increase in carbon emission levels to a certain extent. In the absence of publicity and guidance from the government and third-party organizations, residents will not state green, low-carbon and energy-saving products as their main consumer preference, but may instead choose high-carbon products (Rosner et al., 2021).
At the early stage of urbanization and industrialization, local governments are actively attracting investment because of the assessment pressure of economic growth and fiscal revenue. Accordingly, agricultural land or unused land is mainly converted into high-carbon industrial land. The land use structure under land resource allocation leads to the concentration of high-carbon industries, which has undesirable consequences for the carbon cycle in the biosphere system (Chen and Zhao, 2019). Meanwhile, highly energy-intensive, heavily polluting, and carbon-intensive industries form a high-carbon industrial structure dominated by low-end manufacturing industries (Liu et al., 2012; Huang et al., 2018). Moreover, local government land supply strategies and unreasonable land use management often further strengthen the rigidity of the industrial structure and suppress high-carbon to low-carbon industrial structure transformations (Xie et al., 2018; Yang et al., 2018). The unit land output is not relatively high at this stage; here, economic growth occurs at the expense of excessive energy consumption. Energy use efficiency is also low, and carbon intensity is relatively high, which causes a large amount of CO2 emissions to be released during industrial production (Zhang et al., 2016). At this stage, such highly energy-intensive, carbon-intensive and energy inefficient industrial structures, and unreasonable land use management increase CO2 emissions per unit of land. Therefore, the CO2 emissions level from the production process and land use conversion is relatively high during the extensive land use stage (Zhang and Xu, 2017).
Given below is a simple economic model to illustrate the impact of land urbanization on carbon emissions during the extensive land use stage. The input for carbon emission reduction causes a certain loss in economic output, as shown in the production function of Eq. 3. More specifically, the production function establishes that economic output Yt is the function of technology level At, capital Kt, energy consumption Et, and land resources consumption Nt. In addition, D (EMt) is the output loss function, and EMt is the CO2 emissions level.
The function of the CO2 emissions level is shown in Eq. 4. CO2 emissions are mainly caused by energy consumption in industrial production activities and land use changes (such as changes in land types, e.g., forest and grass lands into cultivated land, wet land into construction land, and agricultural land into construction land).
In Eq. 4, EMt is the CO2 emissions level; EMind denotes CO2 emissions caused by energy consumption in industrial production activities; EML and indicates CO2 emissions caused by land use change; Et and Nt respectively signify energy and land resource consumption; Bt and Ct respectively signify energy efficiency and land use efficiency; and
At the extensive land use stage, the high-carbon industry-based industrial structure, low energy efficiency, and high-carbon intensity increase CO2 emissions. Meanwhile, the limited land use management level and low land use efficiency also further raise CO2 emissions (Chuai et al., 2013; Lai et al., 2016). All these factors augment carbon emissions per unit of land during this period.
Intensive Land Urbanization Stage
However, when economic development reaches the stage of high-quality land use, agricultural land is mainly converted into construction land for low-carbon industries. Here, the land-use structure has shifted from low efficiency to high efficiency (Yang et al., 2019). Optimizing the land use structure can promote a low-carbon industrial structure and a clean energy consumption structure (Xu et al., 2018). For our study, it is important to note that a low-carbon industrial structure and a clean energy consumption structure can reduce the negative impact of production processes and land use changes on CO2 emissions (Huang et al., 2018; Zhou et al., 2019b; Bekun et al., 2021b, 2021c). This effect is reflected in two aspects: 1) the structural optimization effect, that is, a reduction in highly energy-intensive and heavily polluting industries also reduces the degree of human interference in carbon emissions (Li et al., 2017; Zhou et al., 2017) and 2) the technology spillover effect, that is, the development of high-end industries promotes technological progress, which leads to an increase in energy efficiency (Liu et al., 2012; Zhou et al., 2017). As a result, carbon emissions per unit of land declines during this period.
At the stage of high-quality land use, the government implements policies regarding land use and carbon emission reduction to induce enterprises to engage in technological transformation, upgrading, or innovation. The production function for this period is shown in Eq. 5. Notably, the technological transformation, upgrading, or innovation activities of enterprises have a spillover effect on economic output.
where
At this stage, energy and land use efficiency due to the spillover effect of technological innovation increase; thus, the carbon emission level declines. Meanwhile, the sustainable land use management (caused by the policy of the land use regulation) further leads to a decline in carbon emission levels. By formulating reasonable and effective land use regulation policies, the government can promote the land use structure optimization, land use intensity control, land use efficiency improvement to reduce the negative impact of land use on carbon emissions. In addition, the government can also encourage the transformation of residents’ consumption to green, low-carbon, and energy-saving products through policy propaganda and guidance, thereby further reducing carbon emissions (Bekun et al., 2021d). The function of CO2 emissions levels during this period is shown in Eq. 6. The low-carbon industrial structure, sustainable land use management, structural optimization effect and the spillover effect of technological innovation ultimately reduce carbon emissions, which further lead to a decline in carbon emissions per unit of land during this phase (Poumanyvong and Kaneko, 2010).
An Inverted Relationship Between Land Urbanization and Carbon Emissions
From the above analysis, we hypothesize that an inverted U-shaped relationship exists between land urbanization and carbon emissions (Figure 2). The left side of the U-shaped curve (stage I) corresponds to extensive urban land use and high-carbon industrial structure. The right side of the U-shaped curve (stage III) corresponds to intensive urban land use and low-carbon industrial structure. However, there is a policy adjustment stage in the middle (stage II). The Chinese government needs to actively optimize land-use management, adjust environmental regulations, industrial policies, and fiscal and tax policies, so China can quickly enter the third stage of intensive land use and low-carbon industrial structure.
Methods
Econometric Model: STIRPAT Model
According to the Influence, Population, Affluence, and Technology (IPAT) model proposed by Ehrlich and Holdren (1971), environmental impact is determined by three factors: population (P), affluence (A), and technology (T). Specifically, the IPAT theoretical framework has been widely employed in the research field of environmental assessment (Kassouri et al., 2021). Thereafter, Dietz and Rosa (1997) extended the IPAT framework to include a stochastic component that is called the stochastic IPAT model (STIRPAT) model. According to the STIRPAT model, the effects of human activities on the environment (I) can be showed as the following equation:
where Iit denotes the respective environmental impacts, Pit is the population size, Ait is the affluence, and Tit is the technology level for prefecture-level city i and time period t.
Some studies, such as Poumanyvong and Kaneko (2010), Zhang et al. (2017), and Kassouri (2021) added the variable “urbanization” into the STIRPAT model. In this study, we added the variable “land urbanization” into the model following the log-linear version of the STIRPAT model widely used in several previous studies (Poumanyvong and Kaneko, 2010; Kassouri et al., 2021; Kassouri, 2021). The expanded STIRPAT model can be rewritten as follows.
where
Furthermore, to study the nonlinear relationship between land urbanization and CO2 emissions, a model including a quadratic term of the land urbanization rate is given as follows:
Additionally, we replace the dependent variable “the total carbon emissions (Cit)” with sectoral CO2 emissions in Eq. 10, including secondary industrial (C1it), tertiary industrial (C2it), energy (C3it), and household (C4it) sectors, to investigate the relationship between land urbanization and sectoral CO2 emissions.
Econometric Model: A Spatially Adaptive Semi-Parametric Model (SASP)
We assume that the variable “land urbanization rate” exists in the above STIRPAT model in the form of a quadratic term. In fact, there may be a misspecification in the model setting. Furthermore, the impact of land urbanization on CO2 emissions presents spatial heterogeneity and spatial spillover effects (Li et al., 2021b). To avoid model setting misspecification and the curse of dimensionality in the nonparametric model, and to accommodate spatial heterogeneity among different cities, we applied the SASP model to analyze the influential mechanism of land urbanization on CO2 emissions. The SASP model established in this study is shown below in Eq. 11 (Ruppert et al., 2003).
where
Empirical Models: Mediation Effect Model
The mediation effect model was used to examine whether
were Mit presents the mediating variables, i.e., advanced industrial structure, CO2 emissions per unit of GDP or CO2 emissions per unit of land, Zit indicates the control variables,
In Eqs 12–14, if coefficients
Data
The research sample of this study included 285 prefecture-level cities in China in 2012 and 2015. CO2 emissions data consisted of these types: Total CO2 emissions, CO2 emissions of secondary industrial, tertiary industrial, energy, and household sectors, CO2 emissions per unit of GDP, and CO2 emissions per unit of land. CO2 emissions data were taken from the 2012 and 2015 Greenhouse Gas Emission Dataset of Chinese Cities established by the China Urban GHG Working Group. The data is based on the Chinese High-Resolution Emission Gridded Database (CHRED 3.0). Notably, this dataset establishes a spatialization bottom-up method based on point emissions sources, line emission sources, and area sources to achieve 1 km of greenhouse gas emission grid data. The advanced industrial structure is calculated according to the method in Chen and Zhao (2019). Land urbanization rate was characterized by the proportion of construction land allocated for urban land area. The data were collected from the China Land and Resource Statistical Yearbook. In addition, data on population, GDP per capita and number of patents granted were also collected from the China City Statistical Yearbook. Descriptive statistics for each variable are presented in Table 1.
Results
Empirical Results Between Land Urbanization and Total CO2 Emissions
Using the STIRPAT and SASP models, the empirical results of land urbanization and total CO2 emissions are shown in Table 2, and the fitting graph of land urbanization and total CO2 emissions is shown in Figure 3. The results in column 1) of Table 2 are estimated according to the model of Eq. 9, while the results in column 2) of Table 2 are estimated according to the model of Eq. 10. The third column in Table 2 shows the parametric estimation results based on the model of Eq. 11, and the non-parametric estimation results of Eq. 11 are shown in Figure 3. The results indicate that the impact of the land urbanization rate on total CO2 emissions is positive; however, the relationship between land urbanization rate and total carbon emissions presents an inverted U-shaped curve after adding the quadratic term of the land urbanization rate. The results of Figure 3 show that as the land urbanization rate increases, total carbon emissions also rise, but the speed of increase slows down during the sample period. When the land urbanization rate is relatively low, highly energy-intensive and energy inefficient industrial structures promote the increase in total CO2 emissions. With the increase in the land urbanization rate, resource and environmental restraints and government regulations promote structural optimization and technology spillover effects. Similarly, the Chinese government could promote land use structure optimization, land use intensity regulation, land use efficiency improvement by optimizing land use regulatory policies to achieve carbon emissions reduction. These effects lead to a decrease in total CO2 emissions, which is consistent with the results of Martínez-Zarzoso and Maruotti (2011), Yao et al. (2018) and Wang et al. (2021b). The rise in China’s CO2 emissions has slowed down in the current stage. The Chinese government should implement effective policies to promote sustainable land use management, low-carbon industrial structure transformation, energy structure optimization and technological innovation, and enter the win-win phase of land urbanization rate increasement and CO2 emissions reduction as soon as possible.
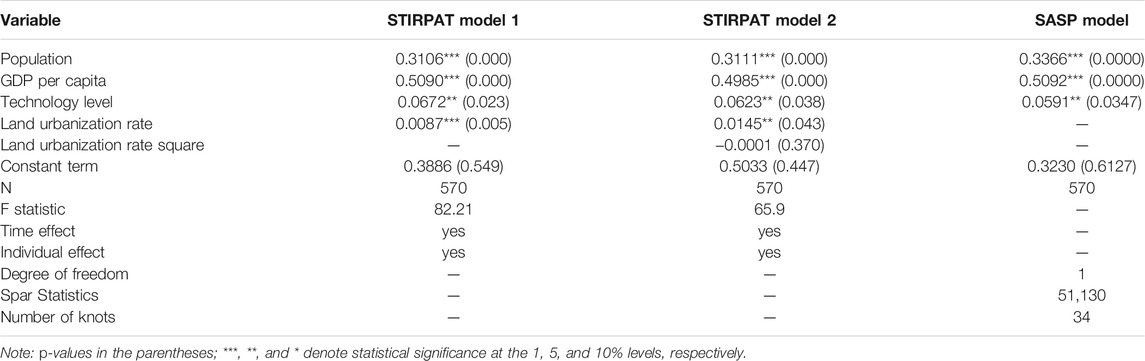
TABLE 2. Estimating results between land urbanization and total CO2 emissions based on the parametric and semiparametric model.
Reverse causality and omitted variables could lead to endogeneity between land urbanization and carbon emissions (Zhang et al., 2017; Wang et al., 2021b). To further conduct the robustness check of the benchmark regression results in Table 3 and resolve the endogeneity problem, we chose the instrumental variables of land urbanization and applied the two-stage least squares (2SLS), generalized moment method (GMM), and limited information maximum likelihood (LIML) methods to perform regression analysis (Xu et al., 2021; Nepal et al., 2021; Safiullah et al., 2021). Following the existing literature, we chose night-time light data, the number of plots of land leasing, and the area of land leasing as the instrumental variables of land urbanization, respectively. The night-time light data were derived from the Visible Infrared Imaging Radiometer Suite sensor on the Suomi NPP satellite, which provides spatially explicit observations of artificial lighting sources across human settlements at night without moonlight (Wang et al., 2018a). The data of the number of plots of land leasing, and the area of land leasing were obtained from the Chinese Land and Resources Yearbook and Chinese Land and Resources Statistical Yearbook. The night-time light data is a good instrumental variable for economic development, including land urbanization (Mellander et al., 2015; Xu et al., 2021). Land leasing scale and area are also reasonable instrumental variables for land urbanization, because they are directly related to land urbanization, but they are also exogenous variables controlled by the Chinese central government. The regression results between land urbanization and total CO2 emissions based on the instrumental variable analysis are shown in Table 3. The results indicate that the impact of land urbanization on the total CO2 emissions was robust. Land urbanization had positive and significant effects on the total CO2 emissions in the 2SLS, GMM, and LIML methods, and were consistent with the results of OLS method and Table 2.
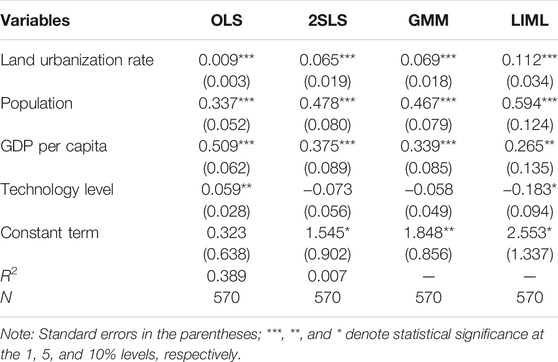
TABLE 3. Estimating results between land urbanization and total CO2 emissions based on the instrumental variable analysis.
In order to further analyze which sectors caused the increase in total carbon emissions, we empirically analyzed the relationship between land urbanization and sectoral carbon emissions. The estimated results are shown in Table 4. The results indicate that as the land urbanization rate increases, CO2 emissions of the secondary industrial, tertiary industrial, energy, and household sectors also increase. Moreover, when the land urbanization rate increases by 1%, the increase in CO2 emissions from the tertiary industrial and household sectors is higher than that in the secondary industrial and energy sectors. This may be caused by the industrial agglomeration effect and the adjustment of energy supply and consumption structure. However, the activities of the tertiary industrial and household sectors are relatively scattered, their green consumption concepts have not been firmly established, and energy-saving products are not widely used. Therefore, the Chinese government must further reduce CO2 emissions in the secondary industrial and energy sectors. It is also necessary to implement effective policies to promote CO2 emission reduction in the tertiary industrial and household sectors.
Empirical Results of the Mediating Effect of the Advanced Industrial Structure
To verify whether land urbanization can promote CO2 emissions reduction through industrial structure optimization, we employed the mediating effect model to perform an empirical analysis. The results of the mediating effect of land urbanization on reducing CO2 emissions through promoting the advanced industrial structure are shown in Table 5.
Table 5 shows that the impact of the land urbanization rate on total CO2 emissions and on the advanced industrial structure is positive and significant.1 When land urbanization and the advanced industrial structure are included in a single model, the land urbanization rate’s impact on total CO2 emissions is positive and insignificant, while the advanced industrial structure’s effect on total CO2 emissions is negative and significant. From the mediating effect model, we can conclude that the advanced industrial structure has a complete and negative mediating effect on the land urbanization rate’s influence on total CO2 emissions. As shown in Table 5, the mediation effect of the advanced industrial structure is −0.0047 [0.0088*(−0.5356)
Empirical Results of the Mediating Effect of CO2 Emissions Per Unit of GDP
To verify whether land urbanization can promote CO2 emissions reduction through lowering CO2 emissions per GDP, we employed the mediating effect model. The results are shown in Table 6.
Table 6 shows that the impact of the land urbanization rate on total carbon dioxide emissions is positive and significant, while its impact on carbon dioxide emissions per GDP is negative and significant. When the land urbanization rate and carbon dioxide emissions per GDP are put in a single model, the land urbanization rate’s impact on total carbon emissions is positive and significant, and the effect of carbon dioxide emissions per GDP on total carbon dioxide emissions is also positive and significant. From the mediating effect model, we can conclude that carbon dioxide emissions per GDP has a partial and negative mediating effect on the land urbanization rate’s influence on total carbon dioxide emissions. As shown in Table 6, the coefficient of total effect
Empirical Results of the Mediating Effect of CO2 Emissions Per Unit of Land
To verify whether land urbanization can promote CO2 emissions reduction through reducing CO2 emissions per land, we employed the mediating effect model to perform an empirical analysis. The results of the mediating effect of land urbanization on CO2 emissions reduction through reducing CO2 emissions per land are shown in Table 7.
Table 7 shows that the impact of land urbanization rate on total CO2 emissions is positive and significant, and the impact of land urbanization rate on CO2 emissions per land is positive and significant. When the land urbanization rate and CO2 emissions per land are put in a single model, the impact of the land urbanization rate on total carbon emissions is positive and significant, and the effect of CO2 emissions per land on total CO2 emissions is also positive and significant. From the mediating effect model, we can conclude that CO2 emissions per land plays a partial and positive mediating effect over the influence of land urbanization rate on total CO2 emissions. As shown in Table 7, the coefficient of total effect
Discussions
The increase in urban CO2 emissions is mainly caused by LUCC and anthropogenic activities (including both production and residential activities). Existing literature has conducted an in-depth examination of CO2 emissions caused by LUCC, which is the main driving force for carbon storage in terrestrial ecosystems (Chuai et al., 2014). First, LUCC can change vegetation’s coverage, biomass, carbon density, and carbon storage, and thus, directly affect CO2 emissions (Zhu et al., 2019). Second, LUCC also has a profound effect on soil organic carbon (Zhu et al., 2019). Among the main land use types, forest land, wetland, and unused land facilitate net carbon absorption, while cultivated land, grassland, and urban construction land facilitate net carbon emissions. The changes from forest land, wetland, and unused land to other types, and especially alterations to urban construction land, will greatly reduce vegetation biomass and release more carbon into the atmosphere (Houghton, 2003; Bailis and McCarthy, 2011). With increased development, more and more cultivated land, woodland, and grassland are transformed into urban construction land. Another main driving force for CO2 emissions is the energy consumption required for production activities on industrial and mining lands, exhaust emissions from transportation lands, and heating in residential areas. The carbon emission intensity of urban construction land is much higher than that of other land types. Among various kinds of construction land, the carbon emission intensity of industrial and mining land is the highest, transportation land is second, and that of urban and rural residential land is the lowest.
This study creatively explores the possibility of synergistic promotion of land urbanization and carbon reduction from the perspective of land use structure optimization, land use intensity regulation, and land use efficiency improvement. More importantly, we found strong evidence to support the environmental Kuznets curve hypothesis between land urbanization and carbon emissions based on land use optimization. In addition, this study provided new insights into the validity of the environmental Kuznets curve hypothesis and focused on how optimized land urbanization can mitigate carbon emissions. China’s land is state-owned, and the government controls the land indicators for conversion of agricultural land or unused land to urban construction land. Under this framework, the government can regulate the supply of urban construction land to influence the urban land use structure, and then affect the CO2 emissions levels through optimization of land use types. Increasing the proportion of land use types with low CO2 emission intensity is conducive to reducing carbon emission levels. Meanwhile, the government can also promote CO2 emissions reduction by regulating land use intensity. Excessive land use intensity could disrupt the balance of the atmosphere and biosphere. It could, in turn, affect the impact of ecosystems on carbon sequestration, thereby increasing CO2 emissions. In addition, the improvement of land use efficiency contributes to reducing CO2 emissions. The improvement of land use efficiency is reflected in the improvement of technical levels, energy use efficiency, and so on, resulting in the reduction of CO2 emissions.
It is noteworthy that this study integrated land use optimization and industrial development into a unified framework to analyze the impact of land urbanization on carbon emissions. High-quality land use and land urbanization contribute to declines in the scale of carbon emissions, per capita carbon emissions, and carbon intensity (Yao et al., 2018). The results of this study are consistent with those of Yao et al. (2018), Xie et al. (2018), and Wang et al. (2021a), which showed that reasonable, efficient, and sustainable land urbanization is beneficial for CO2 emissions reduction. High-quality, and sustainable land urbanization promotes low-carbon development mainly by influencing these mediating variables including industrial structure, energy intensity, energy consumption structure, and technological innovation (Li et al., 2018). More importantly, in the process of land urbanization, the government can promote land use structure optimization, rational land use intensity regulation, land use efficiency improvement by implementing innovative land use policies, which could vigorously promote carbon emissions reduction and low-carbon development.
The government should introduce land policies to promote the optimization of land use structure, land use intensity control, and land use efficiency improvement. First, the government should scientifically formulate spatial land-use planning, with a focus on low-carbon development (Zhang et al., 2018). Indicators such as carbon emissions per unit of land should be included in spatial land-use planning (Wang et al., 2021a). Local governments could control regional land use intensity, optimize land use structure by setting carbon emissions caps, or evaluating energy consumption per unit of industrial land for industrial parks. Second, the Chinese central government should strictly control the land quotas of arable land, forest land, wetland, etc. that are converted into urban construction land. This can promote the optimization of land use structure and reduce the conversion of low-carbon land to high-carbon land. Third, the government should establish a perfect development intensity control system and implement the principle of plot ratio control to restrain the damage of excessive land development intensity to the carbon balance of the biosphere. Finally, local governments should further optimize industrial policies and improve regional land use efficiency. Relevant government departments could conduct performance appraisals on enterprises in the development zone by using indicators such as land use and energy use. In addition, the government could introduce fiscal and tax preferential measures to encourage enterprises to improve their land use and energy use efficiency.
Furthermore, the government should further adjust the energy consumption structure and foster a low-carbon industrial structure, increase the efficiency of energy use, and decrease the intensity of carbon emissions. First, the government should promote energy consumption structure optimization and reducing carbon intensity (Wang et al., 2021a). It should gradually decrease the proportion of high-carbon energy such as coal and coke, and shift away from fossil fuels to renewable low-carbon energy resources such as solar energy, wave energy and wind energy. It also should greatly develop new environment-friendly energy sources to promote the optimization of its energy consumption structure (Wang et al., 2013). At this point, it is also necessary for the government to implement policy measures such as fiscal subsidies and tax incentives to encourage enterprises to optimize their energy consumption structure. Second, the government should pay great attention to upgrading the industrial structure. It will be necessary to optimize the industrial structure, make an appropriate reduction of the secondary industry, greatly develop the tertiary industry, develop the emerging low-carbon industry and boost the upgrade and cluster development of the traditional high energy consumption industry (Wang et al., 2013). Finally, the government should also actively promote technological level advancements and improve carbon emissions efficiency (Wang et al., 2021b). To this end, the government should set target cuts in carbon emissions per unit of GDP. It should also take various measures such as accelerating research and development in low-carbon technology, popularizing new energy-saving products and new technologies, and implementing incentive policies and so on to strengthen energy saving and emission reduction, thus improving energy efficiency (Wang et al., 2013; Wang et al., 2016).
In addition, the government should also make a greater effort to improve the public’s awareness of low-carbon technologies, strengthen the generalization of a low-carbon economy, foster low-carbon consumption and green consumption, and encourage households to keep to a sustainable consumption mode (Wang et al., 2013; Liu et al., 2018b). The government can promote low-carbon development by building low carbon eco-cities, encouraging residents to adopt low-carbon lifestyles, including developing low-carbon transportation habits, e.g., green commuting, shared bikes, metro system, electric and hybrid car (Li et al., 2021b). Specifically, evaluation criteria of local government by the central government should be diversified. Economic evaluation can be weakened, and environmental performance, such as green and low-carbon development indicators, can be emphasized accordingly. Eventually, this approach could lead to a decline in the level of carbon emissions per unit of land, thereby achieving a win-win situation through the synergy of land urbanization and carbon emissions reduction.
Although we only use 2 years of cross-section data to form panel data for empirical analysis, our findings will stimulate research on land use to reduce CO2 emissions and achieve low-carbon development for developing countries. In the future, more micro and high-resolution data can be used to analyze the relationship between land use and CO2 emissions.
Conclusion and Policy Recommendations
This study explored the mechanism of land urbanization’s effect on CO2 emissions in China. First, it sought to uncover this mechanism at different stages of economic development. Theoretical analysis showed that at the stage of extensive land use, the energy-intensive industrial structure, low energy use efficiency, low-level of land use management, and low land use efficiency led to an increase in CO2 emissions per unit of land. However, at the stage of high-quality land use, structural optimization effects, such as an advanced industrial structure and clean energy consumption structure, the spillover effects of technological innovation, and the high-level of land use management caused declines in the CO2 emissions scale and CO2 emissions per unit of land. Secondly, a STIRPAT model and a SASP model were used to explore the impact mechanism of land urbanization on CO2 emissions to accommodate for stochastic factors and spatial heterogeneity among different cities. Meanwhile, the mediating mechanism of the advanced industrial structure, CO2 emissions per unit of GDP and CO2 emissions per unit of land was studied using a mediating effect model. The empirical results show that high-quality land use can promote the optimization of land use, thereby reducing carbon emissions per unit of land or carbon emissions per unit of GDP and achieving CO2 emissions reduction and low-carbon development. Moreover, high-quality land use can achieve CO2 emissions reduction by promoting the advanced industrial structure. To be sure, the government would do well to work to reduce carbon emissions by optimizing spatial land use regulation and innovating spatial land governance.
There are several actions that can be taken by the government to reduce CO2 emissions. First, the government should optimize land use structure, rationally control land use intensity, and promote the effcient use of urban construction land by formulating scientific spatial land-use planning and sensible land-use policies. Indicators such as carbon emissions per unit of land could be included in spatial land-use planning. Local governments could set carbon emissions caps, energy consumption caps for enterprises to limit investment in high-carbon industries and promote low-carbon development. Meanwhile, the local governments can further use fiscal and taxation policies to encourage enterprises to improve their technological level, land use efficiency, energy use efficiency, and so on. In addition, the central government should strictly control the land indicators for the conversion of low-carbon land to high-carbon land, establish a perfect land development intensity control system, and implement floor area ratio control. Second, the government should particularly focus on cultivating low-carbon, energy-saving, and environmental protection industries and encourage the development of these industries to promote an advanced industrial structure. Efforts should be made to explore, exploit, and apply renewable, new, and green energy by developing and introducing new energy technologies and environmentally friendly technologies to achieve the targets of carbon mitigation. Finally, the government should also make an effort to increase the public’s awareness of low-carbon technologies and encourage the households to adopt a low-carbon consumption mode.
This study takes the first step to investigate the effect of land urbanization on carbon emissions by integrating land use structure optimization, land use intensity regulation, land use efficiency improvement, industrial structural optimization, and carbon efficiency improvement. However, this study still exists some limitations. Due to data limitation, the time-frame for this study was restricted to only 2 years of high-resolution gridded data. The endogeneity in the empirical process needs to be further resolved. In the further studies, with more available high-resolution data, this study can be extended to obtain more information on the relationship between land urbanization and CO2 emissions. The treatment effect model or the method of randomized control trials could be used to resolve the endogeneity problem of this study in future. Further research regarding the nonlinear relationship between land urbanization and carbon emissions by integrating land use optimization, industrial structure upgrading, and energy use efficiency improvement remains to be done.
Data Availability Statement
The raw data supporting the conclusion of this article will be made available by the authors, without undue reservation.
Author Contributions
All authors contributed to the study conception and design. Material preparation, data collection, were performed by MT, and the first draft of the manuscript was written by MT. Data processing and analysis were performed by FH, and the manuscript was reviewed by FH. All authors commented on previous versions of the manuscript. All authors read and approved the manuscript.
Funding
This research was supported by the Major project on Humanities and Social Sciences of Shanghai Municipal Education Commission Research and Innovation Program “Research on the effective supply mechanism of urban public goods” (2017-01-07-00-02-E00008), and the Major Project of the Chinese National Social Science Fund “Research on improving the development mechanism of urban-rural integration” (21AZD036).
Conflict of Interest
The authors declare that the research was conducted in the absence of any commercial or financial relationships that could be construed as a potential conflict of interest.
Publisher’s Note
All claims expressed in this article are solely those of the authors and do not necessarily represent those of their affiliated organizations, or those of the publisher, the editors and the reviewers. Any product that may be evaluated in this article, or claim that may be made by its manufacturer, is not guaranteed or endorsed by the publisher.
Footnotes
1The land use structure is calculated according to the proportion of industrial, mining, and storage land in the total land supplied, which is a negative indicator of the land use structure optimization
References
Bailis, R., and McCarthy, H. (2011). Carbon Impacts of Direct Land Use Change in Semiarid Woodlands Converted to Biofuel Plantations in India and Brazil. GCB Bioenergy 3, 449–460. doi:10.1111/j.1757-1707.2011.01100.x
Bateman, I. J., Harwood, A. R., Mace, G. M., Watson, R. T., Abson, D. J., Andrews, B., et al. (2013). Bringing Ecosystem Services into Economic Decision-Making: Land Use in the United Kingdom. Science 341 (6141), 45–50. doi:10.1126/science.1234379
Behera, S. R., and Dash, D. P. (2017). The Effect of Urbanization, Energy Consumption, and Foreign Direct Investment on the Carbon Dioxide Emission in the SSEA (South and Southeast Asian) Region. Renew. Sustain. Energ. Rev. 70, 96–106. doi:10.1016/j.rser.2016.11.201
Bekun, F. V., Adedoyin, F. F., Lorente, D. B., and Driha, O. M. (2021b). Designing Policy Framework for Sustainable Development in Next-5 Largest Economies amidst Energy Consumption and Key Macroeconomic Indicators. Environ. Sci. Pollut. Res. 1–14. doi:10.1007/s11356-021-16820-z
Bekun, F. V., Alola, A. A., Gyamfi, B. A., and Ampomah, A. B. (2021c). The Environmental Aspects of Conventional and Clean Energy Policy in Sub-saharan Africa: Is N-Shaped Hypothesis Valid? Environ. Sci. Pollut. Res., 1–14. doi:10.1007/s11356-021-14758-w
Bekun, F. V., Alola, A. A., Gyamfi, B. A., and Yaw, S. S. (2021d). The Relevance of EKC Hypothesis in Energy Intensity Real-Output Trade-Off for Sustainable Environment in EU-27. Environ. Sci. Pollut. Res. 28, 51137–51148. doi:10.1007/s11356-021-14251-4
Bekun, F. V., Emir, F., and Sarkodie, S. A. (2019). Another Look at the Relationship between Energy Consumption, Carbon Dioxide Emissions, and Economic Growth in South Africa. Sci. Total Environ. 655, 759–765. doi:10.1016/j.scitotenv.2018.11.271
Bekun, F. V., Gyamfi, B. A., Onifade, S. T., and Agboola, M. O. (2021a). Beyond the Environmental Kuznets Curve in E7 Economies: Accounting for the Combined Impacts of Institutional Quality and Renewables. J. Clean. Prod. 314, 127924. doi:10.1016/j.jclepro.2021.127924
Bossio, D. A., Cook-Patton, S. C., Ellis, P. W., Fargione, J., Sanderman, J., Smith, P., et al. (2020). The Role of Soil Carbon in Natural Climate Solutions. Nat. Sustain. 3, 391–398. doi:10.1038/s41893-020-0491-z
Cavender-Bares, J., Polasky, S., King, E., and Balvanera, P. (2015). A Sustainability Framework for Assessing Trade-Offs in Ecosystem Services. Ecol. Soc. 20 (1), 84–138. doi:10.5751/ES-06917-200117
Chen, Y., and Zhao, L. (2019). Exploring the Relation between the Industrial Structure and the Eco-Environment Based on an Integrated Approach: A Case Study of Beijing, China. Ecol. Indicators 103, 83–93. doi:10.1016/j.ecolind.2019.04.001
Chen, Z., Song, P., and Wang, B. (2021). Carbon Emissions Trading Scheme, Energy Efficiency and Rebound Effect - Evidence from China's Provincial Data. Energy Policy 157, 112507. doi:10.1016/j.enpol.2021.112507
Chen, G., Li, X., Liu, X., Chen, Y., Liang, X., Leng, J., et al. (2020). Global Projections of Future Urban Land Expansion Under Shared Socioeconomic Pathways. Nat. Commun. 11, 537. doi:10.1038/s41467-020-14386-x
Chuai, X., Huang, X., Lai, L., Wang, W., Peng, J., and Zhao, R. (2013). Land Use Structure Optimization Based on Carbon Storage in Several Regional Terrestrial Ecosystems Across China. Environ. Sci. Pol. 25, 50–61. doi:10.1016/j.envsci.2012.05.005
Chuai, X., Huang, X., Qi, X., Li, J., Zuo, T., Lu, Q., et al. (2016). A Preliminary Study of the Carbon Emissions Reduction Effects of Land Use Control. Sci. Rep. 6, 36901. doi:10.1038/srep36901
Chuai, X., Huang, X., Wang, W., Wu, C., and Zhao, R. (2014). Spatial Simulation of Land Use Based on Terrestrial Ecosystem Carbon Storage in Coastal Jiangsu, China. Sci. Rep. 4, 5667. doi:10.1038/srep05667
Cumming, G. S., Buerkert, A., Hoffmann, E. M., Schlecht, E., von Cramon-Taubadel, S., Tscharntke, T., et al. (2014). Implications of Agricultural Transitions and Urbanization for Ecosystem Services. Nature 515, 50–57. doi:10.1038/nature13945
Dang, Y., Ren, W., Tao, B., Chen, G., Lu, C., Yang, J., et al. (2014). Climate and Land Use Controls on Soil Organic Carbon in the Loess Plateau Region of China. Plos One 9, e95548–11. doi:10.1371/journal.pone.0095548
Dietz, T., and Rosa, E. A. (1997). Effects of Population and Affluence on CO2 Emissions. Proc. Natl. Acad. Sci. 94, 175–179. doi:10.1073/pnas.94.1.175
Dong, F., Bian, Z., Yu, B., Wang, Y., Zhang, S., Li, J., et al. (2018). Can Land Urbanization Help to Achieve Co2 Intensity Reduction Target or Hinder it? Evidence from China. Resour. Conservation Recycling 134, 206–215. doi:10.1016/j.resconrec.2018.02.009
Dong, F., Wang, Y., Su, B., Hua, Y., and Zhang, Y. (2019). The Process of Peak CO2 Emissions in Developed Economies: A Perspective of Industrialization and Urbanization. Resour. Conservation Recycling 141, 61–75. doi:10.1016/j.resconrec.2018.10.010
Ehrlich, P. R., and Holdren, J. P. (1971). Impact of Population Growth. Science 171, 1212–1217. doi:10.1126/science.171.3977.1212
Gonzalez, O., and Mackinnon, D. P. (2016). A Bifactor Approach to Model Multifaceted Constructs in Statistical Mediation Analysis. Educ. Psychol. Meas. 78 (1), 5–31. doi:10.1177/0013164416673689
Grossman, G. M., and Krueger, A. B. (1995). Economic Growth and the Environment. Q. J. Econ. 110 (2), 353–377. doi:10.2307/2118443
Guo, R., Zhao, Y., Shi, Y., Li, F., Hu, J., and Yang, H. (2017). Low Carbon Development and Local Sustainability from a Carbon Balance Perspective. Resour. Conservation Recycling 122, 270–279. doi:10.1016/j.resconrec.2017.02.019
Houghton, R. A. (2003). Revised Estimates of the Annual Net Flux of Carbon to the Atmosphere from Changes in Land Use and Land Management 1850-2000. Tellus B: Chem. Phys. Meteorology 55, 378–390. doi:10.3402/tellusb.v55i2.16764
Huang, Y., Li, L., and Yu, Y. (2018). Does Urban Cluster Promote the Increase of Urban Eco-Efficiency? Evidence from Chinese Cities. J. Clean. Prod. 197, 957–971. doi:10.1016/j.jclepro.2018.06.251
Jiang, Z., and Lin, B. (2012). China's Energy Demand and its Characteristics in the Industrialization and Urbanization Process. Energy Policy 49, 608–615. doi:10.1016/j.enpol.2012.07.002
Kassouri, Y., Alola, A. A., and Savaş, S. (2021). The Dynamics of Material Consumption in Phases of the Economic Cycle for Selected Emerging Countries. Resour. Pol. 70, 101918. doi:10.1016/j.resourpol.2020.101918
Kassouri, Y. (2021). Monitoring the Spatial Spillover Effects of Urbanization on Water, Built-Up Land and Ecological Footprints in Sub-Saharan Africa. J. Environ. Manage. 300, 113690. doi:10.1016/j.jenvman.2021.113690
Lai, L., Huang, X., Yang, H., Chuai, X., Zhang, M., Zhong, T., et al. (2016). Carbon Emissions from Land-Use Change and Management in China between 1990 and 2010. Sci. Adv. 2, e1601063. doi:10.1126/sciadv.1601063
Li, J., Guo, X., Chuai, X., Xie, F., Yang, F., Gao, R., et al. (2021a). Reexamine China's Terrestrial Ecosystem Carbon Balance under Land Use-Type and Climate Change. Land Use Policy 102, 105275. doi:10.1016/j.landusepol.2020.105275
Li, J., Huang, X., Chuai, X., and Yang, H. (2021b). The Impact of Land Urbanization on Carbon Dioxide Emissions in the Yangtze River Delta, China: A Multiscale Perspective. Cities 116, 103275. doi:10.1016/j.cities.2021.103275
Li, S., Zhou, C., Wang, S., and Hu, J. (2018). Dose Urban Landscape Pattern Affect CO2 Emission Efficiency? Empirical Evidence from Megacities in China. J. Clean. Prod. 203, 164–178. doi:10.1016/j.jclepro.2018.08.194
Li, Z., Sun, L., Geng, Y., Dong, H., Ren, J., Liu, Z., et al. (2017). Examining Industrial Structure Changes and Corresponding Carbon Emission Reduction Effect by Combining Input-Output Analysis and Social Network Analysis: a Comparison Study of China and Japan. J. Clean. Prod. 162, 61–70. doi:10.1016/j.jclepro.2017.05.200
Liu, B., Tian, C., Li, Y., Song, H., and Ma, Z. (2018b). Research on the Effects of Urbanization on Carbon Emissions Efficiency of Urban Agglomerations in China. J. Clean. Prod. 197, 1374–1381. doi:10.1016/j.jclepro.2018.06.295
Liu, D., Xu, C., Yu, Y., Rong, K., and Zhang, J. (2020). Economic Growth Target, Distortion of Public Expenditure and Business Cycle in China. China Econ. Rev. 63, 101373. doi:10.1016/j.chieco.2019.101373
Liu, F., and Liu, C. (2019). Regional Disparity, Spatial Spillover Effects of Urbanisation and Carbon Emissions in China. J. Clean. Prod. 241, 118226. doi:10.1016/j.jclepro.2019.118226
Liu, Y., Li, J., and Yang, Y. (2018a). Strategic Adjustment of Land Use Policy under the Economic Transformation. Land Use Policy 74, 5–14. doi:10.1016/j.landusepol.2017.07.005
Liu, Y., Song, Y., and Arp, H. P. (2012). Examination of the Relationship between Urban Form and Urban Eco-Efficiency in China. Habitat Int. 36, 171–177. doi:10.1016/j.habitatint.2011.08.001
Lu, H., Guo, L., and Zhang, Y. (2019). Oil and Gas Companies' Low-Carbon Emission Transition to Integrated Energy Companies. Sci. Total Environ. 686, 1202–1209. doi:10.1016/j.scitotenv.2019.06.014
Mackinnon, D. P., Warsi, G., and Dwyer, J. H. (1995). A Simulation Study of Mediated Effect Measures. Multivariate Behav. Res. 30 (1), 41–62. doi:10.1207/s15327906mbr3001_3
Martínez-Zarzoso, I., and Maruotti, A. (2011). The Impact of Urbanization on CO2 Emissions: Evidence from Developing Countries. Ecol. Econ. 70 (7), 1344–1353. doi:10.1016/j.ecolecon.2011.02.009
Mellander, C., Lobo, J., Stolarick, K., and Matheson, Z. (2015). Night-Time Light Data: A Good Proxy Measure for Economic Activity? PLoS ONE 10 (10), e0139779. doi:10.1371/journal.pone.0139779
Muñoz-Rojas, M., De la Rosa, D., Zavala, L. M., Jordán, A., and Anaya-Romero, M. (2011). Changes in Land Cover and Vegetation Carbon Stocks in Andalusia, Southern Spain (1956-2007). Sci. Total Environ. 409 (14), 2796–2806. doi:10.1016/j.scitotenv.2011.04.009
Nepal, R., Musibau, H. O., and Jamasb, T. (2021). Energy Consumption as an Indicator of Energy Efficiency and Emissions in the European Union: A GMM Based Quantile Regression Approach. Energy Policy 158, 112572. doi:10.1016/j.enpol.2021.112572
Peters, M. K., Hemp, A., Appelhans, T., Becker, J. N., Behler, C., Classen, A., et al. (2019). Climate-Land-Use Interactions Shape Tropical Mountain Biodiversity and Ecosystem Functions. Nature 568, 88–92. doi:10.1038/s41586-019-1048-z
Poumanyvong, P., and Kaneko, S. (2010). Does Urbanization lead to Less Energy Use and Lower CO2 Emissions? A Cross-Country Analysis. Ecol. Econ. 70, 434–444. doi:10.1016/j.ecolecon.2010.09.029
Rafiq, S., Salim, R., and Nielsen, I. (2016). Urbanization, Openness, Emissions, and Energy Intensity: A Study of Increasingly Urbanized Emerging Economies. Energ. Econ. 56, 20–28. doi:10.1016/j.eneco.2016.02.007
Rosner, Y., Amitay, Z., and Perlman, A. (2021). Consumer's Attitude, Socio-Demographic Variables and Willingness to purchase green Housing in Israel. Environ. Dev. Sustain., 1–22. doi:10.1007/s10668-021-01659-8
Ruppert, D., Wand, M. P., and Caroll, R. J. (2003). Semi-Parametric Regression. Cambridge: Cambridge University Press.
Sadorsky, P. (2014). The Effect of Urbanization on CO2 Emissions in Emerging Economies. Energ. Econ. 41, 147–153. doi:10.1016/j.eneco.2013.11.007
Safiullah, M., Kabir, M. N., and Miah, M. D. (2021). Carbon Emissions and Credit Ratings. Energ. Econ. 100, 105330. doi:10.1016/j.eneco.2021.105330
Sheng, P., and Guo, X. (2016). The Long-Run and Short-Run Impacts of Urbanization on Carbon Dioxide Emissions. Econ. Model. 53, 208–215. doi:10.1016/j.econmod.2015.12.006
Wang, H., Lu, S., Lu, B., and Nie, X. (2021a). Overt and Covert: The Relationship between the Transfer of Land Development Rights and Carbon Emissions. Land Use Policy 108, 105665. doi:10.1016/j.landusepol.2021.105665
Wang, L., Fan, H., and Wang, Y. (2018a). Estimation of Consumption Potentiality Using VIIRS Night-Time Light Data. PLoS ONE 13 (10), e0206230. doi:10.1371/journal.pone.0206230
Wang, P., Wu, W., Zhu, B., and Wei, Y. (2013). Examining the Impact Factors of Energy-Related CO2 Emissions Using the STIRPAT Model in Guangdong Province, China. Appl. Energ. 106, 65–71. doi:10.1016/j.apenergy.2013.01.036
Wang, S., Zeng, J., Huang, Y., Shi, C., and Zhan, P. (2018b). The Effects of Urbanization on CO2 Emissions in the Pearl River Delta: A Comprehensive Assessment and Panel Data Analysis. Appl. Energ. 228, 1693–1706. doi:10.1016/j.apenergy.2018.06.155
Wang, W.-Z., Liu, L.-C., Liao, H., and Wei, Y.-M. (2021b). Impacts of Urbanization on Carbon Emissions: An Empirical Analysis from OECD Countries. Energy Policy 151, 112171. doi:10.1016/j.enpol.2021.112171
Wang, Y., Li, L., Kubota, J., Han, R., Zhu, X., and Lu, G. (2016). Does Urbanization lead to More Carbon Emission? Evidence from a Panel of BRICS Countries. Appl. Energ. 168, 375–380. doi:10.1016/j.apenergy.2016.01.105
Wang, Y., Li, X., Kang, Y., Chen, W., Zhao, M., and Li, W. (2019). Analyzing the Impact of Urbanization Quality on CO2 Emissions: What Can Geographically Weighted Regression Tell Us? Renew. Sustain. Energ. Rev. 104, 127–136. doi:10.1016/j.rser.2019.01.028
Wang, Z., and Feng, C. (2015). Sources of Production Inefficiency and Productivity Growth in China: A Global Data Envelopment Analysis. Energ. Econ. 49, 380–389. doi:10.1016/j.eneco.2015.03.009
Wu, C., Li, G., Yue, W., Lu, R., Lu, Z., and You, H. (2015). Effects of Endogenous Factors on Regional Land-Use Carbon Emissions Based on the Grossman Decomposition Model: A Case Study of Zhejiang Province, China. Environ. Manage. 55, 467–478. doi:10.1007/s00267-014-0406-8
Xie, H., Zhai, Q., Wang, W., Yu, J., Lu, F., and Chen, Q. (2018). Does Intensive Land Use Promote a Reduction in Carbon Emissions? Evidence from the Chinese Industrial Sector. Resour. Conservation Recycling 137, 167–176. doi:10.1016/j.resconrec.2018.06.009
Xu, H., Brown, D. G., Moore, M. R., and Currie, W. S. (2018). Optimizing Spatial Land Management to Balance Water Quality and Economic Returns in a Lake Erie Watershed. Ecol. Econ. 145, 104–114. doi:10.1016/j.ecolecon.2017.08.015
Xu, L., Fan, M., Yang, L., and Shao, S. (2021). Heterogeneous green Innovations and Carbon Emission Performance: Evidence at China's City Level. Energ. Econ. 99, 105269. doi:10.1016/j.eneco.2021.105269
Yao, X., Kou, D., Shao, S., Li, X., Wang, W., and Zhang, C. (2018). Can Urbanization Process and Carbon Emission Abatement Be Harmonious? New Evidence from China. Environ. Impact Assess. Rev. 71, 70–83. doi:10.1016/j.eiar.2018.04.005
Yang, L., Yang, Y., Zhang, X., and Tang, K. (2018). Whether China’s Industrial Sectors Make Efforts to Reduce CO2 Emissions From Production? A Decomposed Decoupling Analysis. Energy 160, 796–809. doi:10.1016/j.energy.2018.06.186
Yang, Y., Jiang, G., Zheng, Q., Zhou, D., and Li, Y. (2019). Does the Land Use Structure Change Conform to the Evolution Law of Industrial Structure? An Empirical Study of Anhui Province, China. Land Use Policy 81, 657–667. doi:10.1016/j.landusepol.2018.11.016
Yue, Y., Ye, X., Zou, X., Wang, J.-a., and Gao, L. (2017). Research on Land Use Optimization for Reducing Wind Erosion in sandy Desertified Area: A Case Study of Yuyang County in Mu Us Desert, China. Stoch. Environ. Res. Risk Assess. 31, 1371–1387. doi:10.1007/s00477-016-1223-9
Yuyin, Y., and Jinxi, L. (2018). The Effect of Governmental Policies of Carbon Taxes and Energy-Saving Subsidies on enterprise Decisions in a Two-Echelon Supply Chain. J. Clean. Prod. 181, 675–691. doi:10.1016/j.jclepro.2018.01.188
Zhang, G., Zhang, N., and Liao, W. (2018). How Do Population and Land Urbanization Affect CO2 Emissions under Gravity center Change? A Spatial Econometric Analysis. J. Clean. Prod. 202, 510–523. doi:10.1016/j.jclepro.2018.08.146
Zhang, N., Wang, B., and Chen, Z. (2016). Carbon Emissions Reductions and Technology Gaps in the World's Factory, 1990-2012. Energy Policy 91, 28–37. doi:10.1016/j.enpol.2015.12.042
Zhang, N., Yu, K., and Chen, Z. (2017). How Does Urbanization Affect Carbon Dioxide Emissions? A Cross-Country Panel Data Analysis. Energy Policy 107, 678–687. doi:10.1016/j.enpol.2017.03.072
Zhang, W., and Xu, H. (2017). Effects of Land Urbanization and Land Finance on Carbon Emissions: A Panel Data Analysis for Chinese Provinces. Land Use Policy 63, 493–500. doi:10.1016/j.landusepol.2017.02.006
Zhang, Y.-J., and Da, Y.-B. (2015). The Decomposition of Energy-Related Carbon Emission and its Decoupling with Economic Growth in China. Renew. Sustain. Energ. Rev. 41, 1255–1266. doi:10.1016/j.rser.2014.09.021
Zhang, Y., Guo, S., Shi, X., Qian, X., and Nie, R. (2021). A Market Instrument to Achieve Carbon Neutrality: Is China's Energy-Consumption Permit Trading Scheme Effective? Appl. Energ. 299, 117338. doi:10.1016/j.apenergy.2021.117338
Zhang, Y., Liu, Y., Wang, Y., Liu, D., Xia, C., Wang, Z., et al. (2020). Urban Expansion Simulation towards Low-Carbon Development: A Case Study of Wuhan, China. Sustain. Cities Soc. 63, 102455. doi:10.1016/j.scs.2020.102455
Zhou, C., Wang, S., and Wang, J. (2019a). Examining the Influences of Urbanization on Carbon Dioxide Emissions in the Yangtze River Delta, China: Kuznets Curve Relationship. Sci. Total Environ. 675, 472–482. doi:10.1016/j.scitotenv.2019.04.269
Zhou, X.-Y., Lei, K., Meng, W., and Khu, S.-T. (2017). Industrial Structural Upgrading and Spatial Optimization Based on Water Environment Carrying Capacity. J. Clean. Prod. 165, 1462–1472. doi:10.1016/j.jclepro.2017.07.246
Zhou, Y., Kong, Y., Sha, J., and Wang, H. (2019b). The Role of Industrial Structure Upgrades in Eco-Efficiency Evolution: Spatial Correlation and Spillover Effects. Sci. Total Environ. 687, 1327–1336. doi:10.1016/j.scitotenv.2019.06.182
Keywords: land urbanization, carbon mitigation, land use optimization, industrial structure adjustment, China
Citation: Tang M and Hu F (2021) How Does Land Urbanization Promote CO2 Emissions Reduction? Evidence From Chinese Prefectural-Level Cities. Front. Environ. Sci. 9:766839. doi: 10.3389/fenvs.2021.766839
Received: 30 August 2021; Accepted: 19 November 2021;
Published: 14 December 2021.
Edited by:
Xiaolei Sun, Chinese Academy of Sciences (CAS), ChinaReviewed by:
Yacouba Kassouri, Leipzig University, GermanyFestus Victor Bekun, Gelişim Üniversitesi, Turkey
Copyright © 2021 Tang and Hu. This is an open-access article distributed under the terms of the Creative Commons Attribution License (CC BY). The use, distribution or reproduction in other forums is permitted, provided the original author(s) and the copyright owner(s) are credited and that the original publication in this journal is cited, in accordance with accepted academic practice. No use, distribution or reproduction is permitted which does not comply with these terms.
*Correspondence: Fengxia Hu, ZnhodTAyMjhAMTI2LmNvbQ==