- 1School of Science, Engineering and Environment, University of Salford, Salford, United Kingdom
- 2Department of Agricultural Chemistry and Soil Science, Bidhan Chandra Krishi Viswavidyalaya, Mohanpur, India
- 3School of Environmental Science and Engineering, Indian Institute of Technology Kharagpur, Kharagpur, India
- 4Department of Geology and Geophysics, Indian Institute of Technology Kharagpur, Kharagpur, India
- 5Institute of Medical and Biomedical Education, St. George’s College, University of London, London, United Kingdom
It is now well-established that not just drinking water, but irrigation water contaminated with arsenic (As) is an important source of human As exposure through water-soil-rice transfer. While drinking water As has a permissible, or guideline value, quantification of guideline values for soil and irrigation water is limited. Using published data from 26 field studies (not pot-based experiments) from Asia, each of which reported irrigation water, soil and rice grain As concentrations from the same site, this meta-analysis quantitatively evaluated the relationship between soil and irrigation water As concentrations and the As concentration in the rice grain. A generalized linear regression model revealed As in soil to be a stronger predictor of As in rice than As in irrigation water (beta of 16.72 and 0.6, respectively, p < 0.01). Based on the better performing decision tree model, using soil and irrigation water As as independent variables we determined that Asian paddy soil As concentrations greater than 14 mg kg−1 may result in rice grains exceeding the Codex recommended maximum allowable inorganic As (i-As) concentrations of 0.2 mg kg−1 for polished rice and 0.35 mg kg−1 for husked rice. Both logistic regression and decision tree models, identified soil As as the main determining factor and irrigation water to be a non-significant factor, preventing determination of any guideline value for irrigation water. The seemingly non-significant contribution of irrigation water in predicting grain i-As concentrations below or above the Codex recommendation may be due to the complexity in the relationship between irrigation water As and rice grains. Despite modeling limitations and heterogeneity in meta-data, our findings can inform the maximum permissible As concentrations in Asian paddy soil.
1 Introduction
Arsenic (As) is a toxic, carcinogenic (Cohen et al., 2013) metalloid that occurs naturally in terrestrial and aquatic environments. Arsenic exposure, mainly through contaminated groundwater used for drinking, has widely been associated with detrimental health effects (Rahman et al., 2009). Though As exposure affects more than 200 million people worldwide (Shakoor et al., 2017), it has emerged as a major public health concern in Bangladesh and India, over the last few decades (Chakraborti et al., 2015). The World Health Organization (WHO) has established a guideline value of 10 μg L−1 for As in drinking water. Although contaminated irrigation water also contributes to As exposure by enhancing As concentrations in food crops (Mandal et al., 2019; Bhattacharyya et al., 2021), no WHO or international guideline value for irrigation water has been established to date.
Rice is a staple food for more than half of the global population, especially in Asian, African and Latin American countries (Majumder and Banik, 2019). In India and Bangladesh, daily consumption of milled rice is high (approximately 103 and 268 kg per capita year−1 respectively; FAO, 2017). In Bangladesh, approximately 73% of calorific intake comes from rice (Mwale et al., 2018) and in India it comprises 30% (IRRI, Knowledge Bank). Rice is a rich source of dietary fiber and nutrients, including carbohydrates, proteins, vitamins, and minerals (Dipti et al., 2012; Mwale et al., 2018). However, rice consumption may also be a major route of As exposure (Mondal and Polya, 2008; Mondal et al., 2010; Mondal et al., 2020). Soil serves as a significant sink for As, which is highly bioavailable to rice roots under the conditions in which rice is cultivated (Kumarathilaka et al., 2018). Rice plants are major accumulators of As compared to other cereal crops (Williams et al., 2007) and irrigation of a paddy field with As contaminated water elevates As concentrations in paddy soil (Meharg and Rahman, 2003), rice straw, and grain (Panaullah et al., 2008). In Asia, rice is the basic staple food for the majority of the population, including the region’s 560 million poor (GRiSP Global Rice Science Partnership, 2013). During 2018–19, rice consumption in China was to the extent of 146.7 million tons, followed by India at 102 million tons (ICAR-NRRI Annual Report 2020). Apart from China and India, the other major rice producing countries are Bangladesh, Indonesia, Vietnam, Thailand, and Philippines. The production together accounts for more than 80% of global rice production (ICAR-NRRI Annual Report 2020) but unfortunately some of these regions are As contaminated. For example, in Bangladesh, 2.4 million out of 4 million hectares of paddy field have been found to be As contaminated (Akinbile and Haque, 2012).
Arsenic intake from rice has become a global concern, hence different countries have set maximum tolerable concentrations of As in rice grain (Schmidt, 2015). The Joint FAO-WHO Codex Alimentarius Commission has recommended a maximum concentration of 0.2 mg kg−1 for inorganic As in polished rice and 0.35 mg kg−1 in husked rice (Codex Alimentarius Commission, 2017). However, there have been limited attempts to establish paddy soil and irrigation water As concentrations above which the maximum recommended concentrations in rice may be exceeded. The usual range of total As in uncontaminated soil is 0.1–10 mg kg−1 (Zhao et al., 2010). The European Union (EU) recommended that As in agricultural soil should not exceed 20 mg kg−1 (Rahman et al., 2007; Rahaman et al., 2013; Hussain et al., 2021). Lower and upper guideline values of 10 and 50 mg kg−1 respectively have been prescribed by Finnish regulators (Ministry of the Environment, 2007; Toth et al., 2016). However, the values recommended by the EU and the Ministry of Environment in Finland were for generic agricultural soils rather than for paddy soils. These generic agricultural values may not be appropriate for application to paddy soil conditions, which are known to enhance As bioavailability to rice roots (Meharg and Rahman, 2003). For irrigation water, a regulatory limit of 100 μg L−1 for As has been adopted (Food and Agriculture Organization FAO, 1992; Pescod, 1992). This is in line with the 100 μg L−1 maximum concentration recommended by Ayers and Westcot (1985) for trace elements in irrigation waters but is again focused on generic agricultural production rather than rice specifically.
To our knowledge, no previous studies have derived maximum tolerable concentrations of paddy soil and irrigation water As above which rice grain As may exceed the maximum allowable concentrations set by the Joint FAO-WHO Codex Alimentarius Commission (JECFA, 2017). Using a meta-analysis approach, we attempt to determine soil and irrigation water As concentrations above which rice grains cultivated in Asian paddy fields may exceed the maximum tolerable concentrations of 0.2 mg kg−1 for inorganic As in polished rice and 0.35 mg kg−1 in husked rice.
2 Materials and Methods
2.1 Data Sources
We systematically reviewed published articles reporting As concentrations in paddy soil, irrigation water, and rice grains cultivated in Asian countries. We used Boolean operators (e.g., “OR” and “AND”) to develop search terms from keywords (“arsenic,” “contamination,” “soil,” “water,” “rice,” “risk”). Searching ISI Web of Science and PubMed with these terms, we identified relevant research papers published between 1980 and 2021, since 1980 onward the severity of As contamination was recognized in Asia. Studies were only included in subsequent meta-analysis if (1) the research was carried out in the field and not as pot experiments in the laboratory; (2) it was undertaken in Asian countries; (3) the As concentration data presented included total arsenic of soil, rice grain, and irrigation water from the same study location; (4) the analysis of As was carried out using appropriate laboratory instruments rather than Field Testing Kits; and (5) details of the analytical method(s) and quality assurance procedures used for the study were provided. The PRISMA (Preferred Reporting Items for Systematic Reviews and Meta-Analysis) flowchart can be seen in Figure 1.
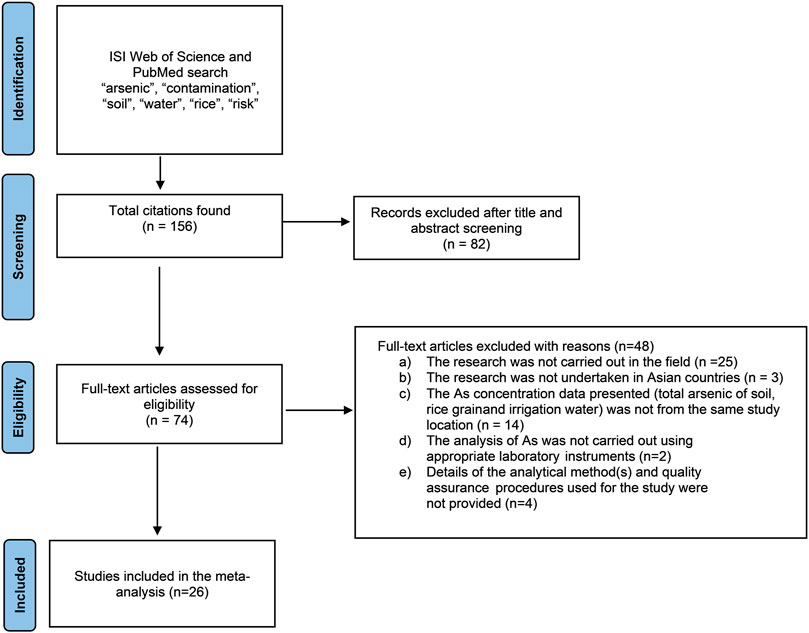
FIGURE 1. PRISMA (Preferred Reporting Items for Systematic Reviews and Meta-Analyses) flowchart showing the selection of studies eligible for a meta-analysis.
2.2 Classification of Data
The proportion of inorganic As (i-As) in rice grain was determined from published data (18 studies) from Asian countries and a weighted mean of 80 and 75% for polished rice and husked rice, respectively, was calculated (Supplementary Table S1). The total As (t-As) concentrations in rice grain in our meta-data was converted to i-As. The rice grain concentrations were divided into two groups: (1) “within the maximum tolerable concentration (≤MTC)”: As ≤ 0.35 mg kg−1 (husked rice) and ≤0.20 mg kg−1 (polished rice); (2) “above the maximum tolerable concentration (>MTC)”: >0.35 mg kg−1 (husked rice) and >0.20 mg kg−1 (polished rice) (based on the recommendation of JECFA, 2017). The whole data set was randomly split into two, 80% of the data were used as the training set and the remaining 20% formed the testing set (Mukherjee et al., 2021).
2.3 Data Analysis
2.3.1 Logistic Regression
A logistic regression model or logit model (James et al., 2013) was used to model our binary dependent variables: ≤MTC and >MTC. A probability value between 0 and 1 was allocated to each class. To identify the best fitting model, both accuracy and kappa values were considered. The residuals of the LR model were checked for normality and the distribution was further confirmed from the plot. To estimate the coefficients from the data, the model could have two (X1 and X2) or more predictors, as in this case As in soil and As in irrigation water. A linear relationship can be written in the mathematical form shown by Equation 1, where p is the probability of the event that Y = 1 and Y is the binary response variable. The quantity p (X)/(1−p(X)) is called the odds, which can take any value between 0 and ∞ and is calculated by the maximum likelihood method. β0, β1, and β2 are the coefficients.
2.3.2 Decision Tree
In this study, we used a Classification and Regression Tree (CART) which is a non-parametric supervised learning method proposed by Breiman (1984) and Ripley (1996). Decision trees are not black-box models, their outputs are easy to interpret, and the DT maps the behavior or relationship between the predictor and target variable (Dreiseitl and Ohno-Machado, 2002). The DT method has been used widely, for example, to identify heavy metals in the environment (Jouanneau et al., 2011) and to streamline the mapping of soil pollution, for example, in a study on rice cadmium concentration (Wang et al., 2020).
The algorithm divides the data set several times according to a criterion that maximizes data separation, resulting in a tree-like structure (Breiman, 1984). The most used criterion is knowledge gain, which implies that the decrease in entropy, due to each split, is maximized. The ratio of y class elements over all elements of the leaf node that contains data item x is the estimate of P (y|x) (Dreiseitl and Ohno-Machado, 2002). The best DT model was selected based on Complexity Parameter (cp) and accuracy. The cp was used to control the size of the DT and to select the optimal tree size. If the cost of adding another variable to the DT from the current node was above the value of cp, then tree building was discontinued.
In terms of model complexity, it is low in logistic regression, particularly when no or few interaction terms and variable transformations are used. The LR method uses only the statistically significant predictor variables in the model whereas DT uses the predictor variables in a hierarchical and recursive manner. DT has the flexibility of assigning the classes in one or more steps. One advantage of the LR is that it can be used to generate probabilities of class membership for each object whereas DT only generates average probabilities applicable to all the objects assigned to a particular group (Worth and Cronin, 2003).
2.3.3 Model Limitations and Assumptions
The two criteria used to assess the quality of a classification model are discrimination and calibration. Discrimination is a measure of how well the two classes in the data set are separated; calibration determines how accurate the model probability estimate is to predict the true probability (Dreiseitl and Ohno-Machado, 2002). To provide an unbiased estimate of a model’s discrimination and calibration, these values should be calculated from a data set not used in the model building process. Usually, a portion of the original data set, called the test or validation set, is put aside for this purpose, since testing on a separate data set would, in an ideal case, provide an unbiased estimation of generalization error. In small data sets as in this study, there may not be enough data for both training and testing. For this reason, the total data set was split into training set and testing set and the training data set was used as the source of information. In this case, the whole data set was divided into k pieces, k-1 pieces are used for training, and the last piece was the test set. This process of k-fold cross-validation builds k models; the numbers reported are the averages over all k test sets (Stone, 1974; Allen, 1977). The problem of over fitting both in the logistic regression and the DT analysis was controlled by k-fold cross validation (k = 10) of the training data (James et al., 2013). On the observations in the remaining fold, the number of misclassified observations was calculated. This procedure was repeated, with each validation set consisting of a different set of observations (James et al., 2013). To quantify the extent to which the predicted response value for a given observation was close to the true response value for that observation, the receiver operating characteristic (ROC) curve was used. The overall performance of a classifier, summarized over all possible thresholds, was given by the area under the curve (AUC) (James et al., 2013).
The data analysis was performed using R-Studio (version 1.3.1093 2.3.1). Splitting the data into training and test data was performed using the stats (version 4.0.3) package. The Caret package (version 6.0–86) was used to conduct logistic regression and DT analysis (Kuhn, 2008). The probability graph from the logistic regression was prepared using ggplot2 (version 3.3.3) and tidyr (version 1.1.3), and for ROC and AUC pROC (version 1.17.0.1) was used.
3 Results
3.1 Study Characteristics
Reviewing the titles and abstracts of 156 research articles that were obtained using the search terms resulted in 82 articles being identified for further consideration, of which 26 met the inclusion criteria (Section 2.1). From these 26 selected research papers (Table 1), an overall sample of 134 grain As concentrations were collated with corresponding soil and irrigation water As concentrations. Among the 26 selected studies, 15 reported grain As concentrations in husked rice and 11 reported concentrations in polished rice (Table 1). Most of the studies (n = 20) reported mean values. For the 6 studies that only reported concentration ranges (Roychowdhury et al., 2008b; Biswas et al., 2013; Srivastava et al., 2015; Sharma et al., 2017; Chowdhury et al., 2020; Reid et al., 2021), the minimum and maximum As concentrations reported for rice grain, soil and irrigation water were used. All the selected studies used credible methods for sample preparation and analysis, for example, Atomic Absorption Spectrophotometer with Flow Injection Hydride Generation (AAS-FI-HG) was used in 18 studies, Inductively Coupled Plasma Mass Spectrometry (ICP-MS) in 7 studies, and High Resolution Inductively Coupled Plasma Mass Spectrometry (HR ICP-MS) was used in 1 study (Supplementary Table S2). All have provided the results of analysis for certified reference materials, thus ensuring quality assurance except 3 studies. Rahman et al. (2010) and Rahman et al. (2014) mentioned the use of reagent blanks in duplicates and Talukder et al. (2011) followed the method as outlined and established by Loeppert and Biswas (2002). In all these studies, the irrigation water samples were filtered before instrumental analysis and the soil and rice grain samples were dried before digestion followed by instrumental analysis.
3.2 Relationship Between t-As Concentration in Rice Grain with Soil and Irrigation Water As Concentrations
Arsenic concentrations in rice grain, soil, and irrigation water based on the meta data (n = 134) are summarized in Table 2. The rice grain t-As concentration ranged from 0.0018 to 1.56 mg kg−1 with a mean value of 0.40 mg kg−1. The As concentration in soil ranged from 0.06 to 112 mg kg−1 with a mean value of 11.73 mg kg−1 and the irrigation water As content ranged from 0 to 1014 µg L−1 with a mean value of 235.49 µg L−1. The rice grain t-As content was found to be positively and significantly (p < 0.01) correlated with the soil As (Spearman rho = 0.65) and the irrigation water As (Spearman rho = 0.46) concentrations (Figures 2A,B). The soil As had a significant (p < 0.05) positive correlation with irrigation water (Spearman rho = 0.32) concentrations (Figure 2C). Of the collated meta data, 12.68% had soil As concentrations above 20 mg kg−1 and 63.43% of the data had irrigation water As above 100 μg L−1; 54% of the polished rice grain i-As meta-data exceeded the concentration of 0.20 mg kg−1 and 74% of the husked rice grain i-As meta-data exceeded 0.35 mg kg−1.
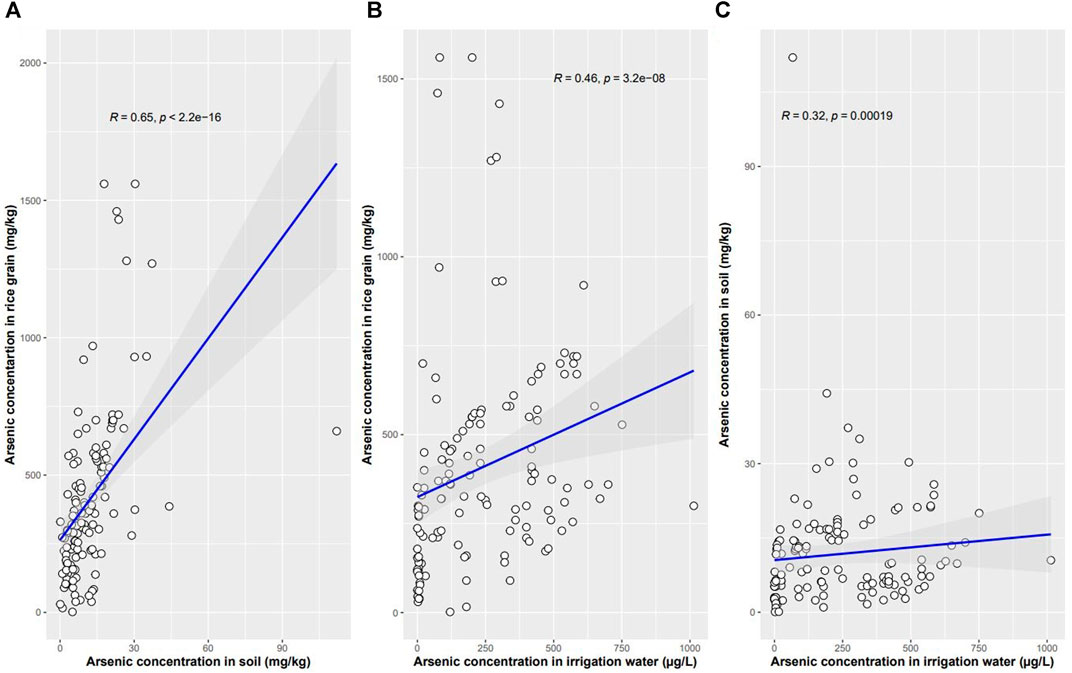
FIGURE 2. Spearman correlation between (A) rice grain t-As and soil As, (B) rice grain t-As and irrigation water As, and (C) irrigation water As and soil As (n = 134).
When a generalized linear regression model (GLM) was used on the meta data it was observed that GrainAs = 0.60 IrriAs + 16.72 SoilAs (AIC = 1900.40) when intercept was forced through zero. Both the coefficients were significant (p < 0.01). The model with intercept, GrainAs = 200.91 + 0.28 IrriAs + 11.76 SoilAs (AIC = 1877.8) had a slightly better fit, and the coefficients were significant (p < 0.05 for IrriAs and p < 0.01 for SoilAs).
3.3 Determination of the Maximum Concentration of As in Soil and Irrigation Water
The LR model predicted Probability (≤MTC |> MTC) = -1.6822 + 0.1429 SoilAs (AIC = 123.68). The soil As coefficient significantly (p < 0.01) explained the grain i-As content. When irrigation water As was added to the model, the coefficient was statistically non-significant (p > 0.05) and AIC increased to 128.17. Soil As content was 11.75 mg kg−1 when probability (≤MTC |> MTC) reached 50% (Figure 3) and hence can be considered as the soil concentration above which the i-As in grain may exceed 0.20 mg kg−1 for polished rice and 0.35 mg kg−1 for husked rice. Performance of LR model was lower than the DT, both based on the classification ability in terms of accuracy; misclassification (grain As as ≤ MTC vs. > MTC (Table 3)) and ROC plots (Figure 4). Since classifiers that perform no better than chance are expected to have an AUC below 50% (James et al., 2013), both models performed well overall, but the ROC plots confirmed that DT performed better than LR. During the training phase, DT achieved an AUC of 72.5% and LR 65.5% (Figures 4A,B) and in the testing phase (Figures 4C,D), the AUC for DT was 70.6% and for LR 65.5%.
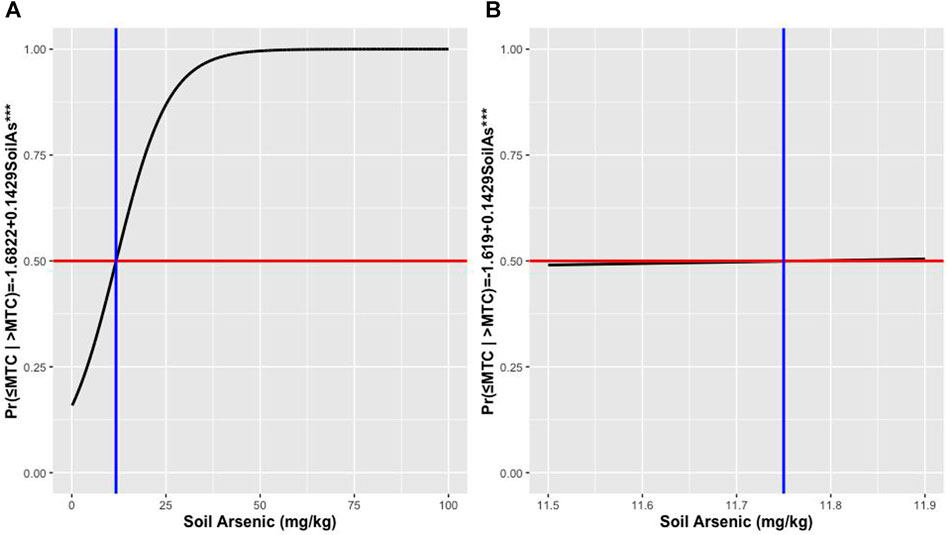
FIGURE 3. Safe limit of As in soil based on the probability plot of logistic regression with respect to soil As (A) and the cutoff point magnified (B).
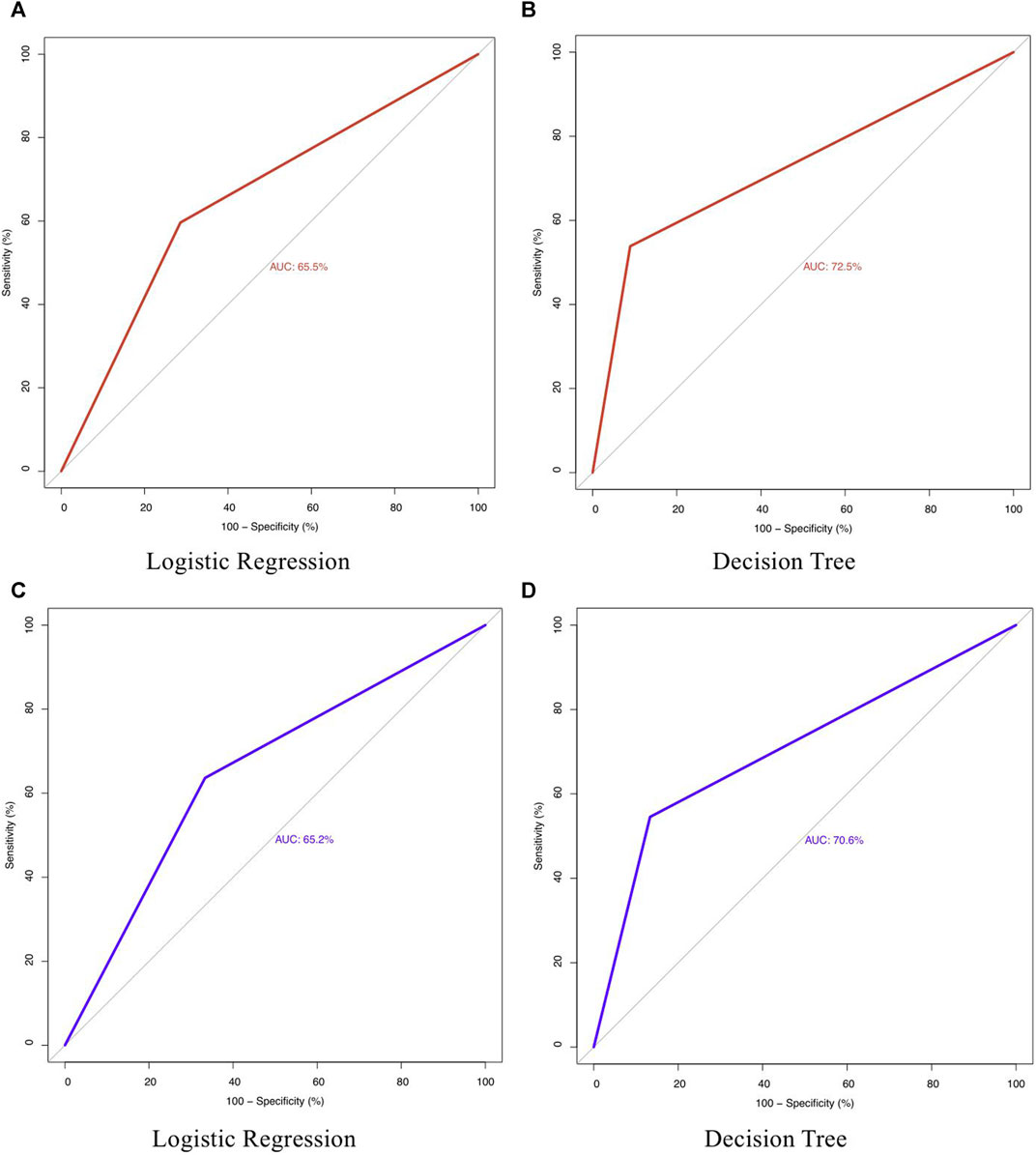
FIGURE 4. Sensitivity vs. specificity plot for decision tree and logistic regression over the training phase (A, B) and the testing phase (C, D).
The probability of grain i-As being classed ≤ MTC by the DT model was 0.52 (Figure 5). Soil As was the primary splitting variable at 14 mg kg−1. Below this soil concentration, the probability of grain i-As being classed as ≤ MTC was 0.76 (69% of the data were in this second node). When soil As was greater than 14 mg kg−1, the probability of grain As being classed > MTC was 0.85 and 31% of the data was in this node. No further splitting of the tree and inclusion of irrigation water as a successful variable was observed. An attempt was made to predict the maximum concentration in irrigation water above which the soil As exceeded 11.75 and 14 mg kg−1 using the LR and DT models, respectively. With the LR model the irrigation water As was observed to be non-significant and in the case of DT irrational splitting was observed which was also not suitable for pruning based on the complexity parameter.
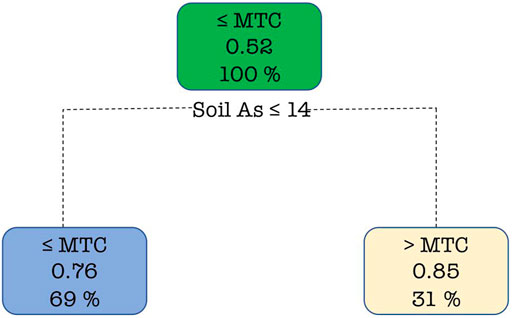
FIGURE 5. Decision tree explaining the probability of the category (≤ MTC or > MTC) of rice grain based on the As content in soil. Percentage of observations in the node and probability of class observations in the node are displayed.
3.4 Subgroup Analysis
The subgroup analysis (Supplementary Table S2) was performed using the data from the studies that also reported soil parameters like pH and organic carbon (OC) content in soil (Table 1). Several studies have reported pH (Hussain et al., 2021; Kumar et al., 2021) and organic carbon (OC) (Mandal et al., 2019; Kumari et al., 2021) content in soil as major determinants of soil As. The GLM GrainAs = 258.05 + 0.38 IrriAs−0.29 pH−0.32 OC + 19.43 SoilAs (AIC = 420.47) was only significant for the IrriAs (p < 0.05) and SoilAs (p < 0.01) coefficients. For the GLM SoilAs = 1.30 + 0.003 IrriAs + 1.03 pH−0.01 OC (AIC = 210.37) none of the coefficients were statistically significant (p > 0.05). In the logistic regression of the subgroup Pr (≤MTC |> MTC) = −1.811 + 0.003 IrriAs−0.62pH−0.01 OC + 0.28 SoilAs (AIC = 46.50), only the coefficient of SoilAs was statistically significant (p < 0.05). Using the LR model for the subgroup identified that soil As alone significantly affected the class probabilities of the rice grain As in terms of being ≤ MTC or > MTC.
4 Discussion
To our knowledge this is the first meta-analysis utilizing published data from Asian paddy fields on As in rice grain, soil, and irrigation water to determine the relationship between them and to develop a model to estimate the maximum concentration in paddy soil and irrigation water above which Codex standards for the maximum allowable i-As in rice would be exceeded (JECFA, 2017). From the 156 papers reviewed, only 26 studies (15 from India, 1 from Taiwan, 1 from Nepal, 1 from Vietnam, 1 from China and 7 from Bangladesh) met the inclusion criteria for the meta-analysis; these studies all reported t-As concentrations in rice grain, soil, and irrigation water. There was near equal split between studies that reported t-As concentrations in husked rice (15 studies) and those in polished rice (11 studies). After converting t-As to i-As, 43% for husked and 60% for polished rice concentrations exceeded the Codex standard.
Based on the GLRM, both soil and irrigation water As concentrations significantly influenced As concentrations in grain. While it is known that the bioavailability of As from soil to rice depends on various parameters including microbial activity (Kumarathilaka et al., 2019), As concentrations in soil and irrigation water are acknowledged to be the most significant factors affecting rice grain t-As concentrations (Mukherjee et al., 2017; Kumari et al., 2021). Our subgroup analysis on the impact of soil parameters (e.g., pH and OC) on rice grain As supports this, the other soil parameters being non-significant. However, in this meta-analysis, soil As was the main determining factor and this was confirmed by (1) the stronger positive correlation between paddy soil and rice As concentrations compared to irrigation water and rice grain concentrations (Figure 2); (2) the LR model having non-significant contribution of irrigation water As; and (3) the DT model predictions taking only into account the soil As classified data. This aligns with previous studies where authors reported most significant impact of soil As (Sengupta et al., 2021) and “modest if any” impact of irrigation water on t-As content of rice (Van Geen et al., 2006). On the contrary, in a recent study, mean As concentrations in groundwater used for irrigation were strongly correlated with grain t-As (Reid et al., 2021). Regardless, many studies have suggested that soil As concentrations increase with contaminated groundwater irrigation of paddy fields (Huq et al., 2006; Panaullah et al., 2008; Dittmar et al., 2010), eventually resulting in increase of grain As (Rahman et al., 2007; Lu et al., 2009; Rahman et al., 2010). The non-significant influence of irrigation water on the grain As concentrations in this study was perhaps unsurprising given the complexity in the transfer of irrigation water As to rice grain via the soil. For example, the accumulation of As in soil from the irrigation water is dependent on several factors like the temporal variation in As concentration throughout the crop-growth period, the volume of irrigation water used, and the area of the field being irrigated (Chowdhury et al., 2018; Chowdhury et al., 2020). As seen in Figure 2C, there is only a moderate increase in soil As with increase in irrigation water As concentration. In fact, some of the very high As concentrations in soil were observed when the irrigation water As concentration was less than 300 μg L−1. The accumulation of soil As also depends on long-term leaching, which is influenced by soil texture including particle size, clay content, and saturated hydraulic conductivity (Dousova et al., 2016). The complexity in the relationship between irrigation water As and grain As could be further enhanced due to irrigation practices which often include the use of both groundwater and rainwater. For example, the phase wise soil As movement and its enrichment pattern in rice due to the use of As contaminated irrigation water showed a moderate accumulation of soil As in the vegetative phase followed by a severe drop in the reproductive phase and continued buildup of As in the ripening phase (Chowdhury et al., 2018). Whereas, in rainfed rice cultivation, a moderate accumulation of As in soil in the vegetative phase followed by a rise in the reproductive phase and a decrease at the ripening stage mainly due to the dilution of the As accumulation in soil due to rainwater was noted (Chowdhury et al., 2020). Furthermore, the binding of As via ternary complexes and mobile colloids has been considered the most important but not the sole driver of As mobility in soils (Mandal et al., 2019). The other competing inorganic anions like phosphates and silicates affect the As adsorption/desorption mechanism significantly. For example, they form surface complexes with Fe and organic matter, causing an easier release of As from surface bindings at higher concentrations (Raj et al., 2021).
The maximum concentration of As in soil from the LR model was found to be 11.75 mg kg−1 whereas, based on the better performing DT model the maximum concentration was 14 mg kg−1 above which the As concentration in rice grain would exceed the Codex recommendation. This is in agreement with (1) the study from Bangladesh, investigating the accumulation and distribution of As in rice grain, and reporting that the rice grown in soils contaminated with As of 14.5 ± 0.1 mg kg−1 was not safe for human consumption (Rahman et al., 2007); (2) the recommendation of the maximum acceptable limit of As in soil of 20 mg kg−1 by the European Union (Rahman et al., 2007); and (3) the limit of 15 mg kg−1 of As in paddy soils by Ministry of Environment, Government of Japan (Punshon et al., 2017). These findings suggest that, based on the scientific data currently available for rice cultivation in Asia, an As guideline value of 14 mg kg−1 in paddy soil may be appropriate.
Being a meta-analysis one of the limitations of our study might be unavoidable heterogeneity. We have carefully limited the data based on our inclusion criteria to reduce the heterogeneity. However, both the models had a minor influence from the data of the six research papers that reported the minimum and maximum values and to overcome this, repeated cross-validation was performed (James et al., 2013). Alongside the inherent limitations of modelling, the challenge was to increase the model’s generalizability so that its applicability is not limited. It would be naive to believe that our models could be applicable to all contaminated rice growing sites worldwide, as the models have been trained with a particular set of data from a specific geographical region. However, some points should be taken into consideration. For example, the soil As data used in the meta-analysis is total As, which is obtained using an acid extraction technique and total As content in soil does not take into account how its availability is modified by important soil properties (Golui et al., 2017). However, the bioavailability of As in soil solutions is governed mainly by pH, redox potential, organic matter content, and the presence of other ions in soil (Hussain et al., 2021). The use of soil available As (extracted by NaHCO3) should be investigated as a better explanatory variable. Future work incorporating analysis of As in rhizosphere soil, soil solution, and irrigation water samples, and sequential analysis of As in soil over time would enable further improvement and validation of our model.
5 Conclusion
This meta-analysis determined that the concentrations of 14 mg kg−1 in paddy soil may be an appropriate guideline value above which rice grains cultivated in Asian paddy fields will exceed the Codex recommended maximum allowable concentrations of 0.2 mg kg−1 for i-As in polished rice and 0.35 mg kg−1 in husked rice. While the GLM predicted an overall positive relationship between As in rice grain with As in irrigation water, both LR and DT models predicted that soil As was the main determining factor. A guideline value for the irrigation water could not be derived using either the LR or DT models. The non-significant contribution of irrigation water was unsurprising, given that the As accumulation in soil due to contaminated irrigation water depends on several factors and the relationships governing transfer to rice grains are complex. Considering uncertainties and limitations of the available meta data and models, experimental studies collecting more appropriate soil and irrigation water samples along with grain rice, and analysis of soil available As, rather than total As in paddy soil, is warranted to validate these findings.
Data Availability Statement
The original contributions presented in the study are included in the article/Supplementary Material, further inquiries can be directed to the corresponding authors.
Author Contributions
JM: Data curation, methodology, formal analysis, writing - original draft preparation; SUS: Data curation, methodology; SOS: Methodology, validation; AM: Visualization, writing-review and editing; MW: Supervision, validation, writing-review and editing; SH: Supervision, writing-review and editing; DM: Conceptualization, supervision, writing- reviewing and editing.
Funding
Funding for this study was provided by Netaji Subhas -ICAR International Fellowship (F.No.18 (02)/2019-EQR/Edn) to JM.
Conflict of Interest
The authors declare that the research was conducted in the absence of any commercial or financial relationships that could be construed as a potential conflict of interest.
Publisher’s Note
All claims expressed in this article are solely those of the authors and do not necessarily represent those of their affiliated organizations, or those of the publisher, the editors, and the reviewers. Any product that may be evaluated in this article, or claim that may be made by its manufacturer, is not guaranteed or endorsed by the publisher.
Supplementary Material
The Supplementary Material for this article can be found online at: https://www.frontiersin.org/articles/10.3389/fenvs.2021.760125/full#supplementary-material
References
Ahmed, Z. U., Panaullah, G. M., Gauch, H., McCouch, S. R., Tyagi, W., Kabir, M. S., et al. (2011). Genotype and Environment Effects on rice (Oryza Sativa L.) Grain Arsenic Concentration in Bangladesh. Plant Soil 338 (1), 367–382. doi:10.1007/s11104-010-0551-7
Akinbile, C. O., and Haque, A. M. M. (2012). Arsenic Contamination in Irrigation Water for rice Production in Bangladesh: a Review. Trends Appl. Sci. Res. 7 (5), 331. doi:10.3923/tasr.2012.331.349
Allen, D. (1977). The Relationship between Variable Selection and Data Augmentation and a Method of Prediction. Technometric 16, 125–127.
Ayers, R. S., and Westcot, D. W. (1985). Water Quality for Agriculture, Vol. 29. United NationsRome, Italy: Food and Agricultural Organization, 96. 9251022631 (Accessed on 08 03, 2021).
Bhattacharya, P., Samal, A. C., Majumdar, J., and Santra, S. C. (2010a). Accumulation of Arsenic and its Distribution in rice Plant (Oryza Sativa L.) in Gangetic West Bengal, India. Paddy Water Environ. 8 (1), 63–70. doi:10.1007/s10333-009-0180-z
Bhattacharya, P., Samal, A. C., Majumdar, J., and Santra, S. C. (2010b). Uptake of Arsenic in rice Plant Varieties Cultivated with Arsenic Rich Groundwater. Environ. asia 3 (2), 34–37.
Bhattacharyya, K., Sengupta, S., Pari, A., Halder, S., Bhattacharya, P., Pandian, B. J., et al. (2021). Characterization and Risk Assessment of Arsenic Contamination in Soil-Plant (Vegetable) System and its Mitigation through Water Harvesting and Organic Amendment. Environ. Geochem. Health 43, 2819–2834. doi:10.1007/s10653-020-00796-9
Biswas, A., Biswas, S., Das, A., and Roychowdhury, T. (2018). Spatial Variability and Competing Dynamics of Arsenic, Selenium, Iron and Bioavailable Phosphate from Ground Water and Soil to Paddy Plant Parts. Groundwater Sustain. Dev. 7, 328–335. doi:10.1016/j.gsd.2018.08.001
Biswas, A., Biswas, S., and Santra, S. C. (2013). Arsenic in Irrigated Water, Soil, and rice: Perspective of the Cropping Seasons. Paddy Water Environ. 12 (4), 407–412. doi:10.1007/s10333-013-0396-9
Breiman, L., Friedman, J. H., Olshen, R. A., and Stone, C. J. (1984). Classification and Regression Trees. 1st ed. Boca Raton, FL: Routledge. doi:10.1201/9781315139470
Chakraborti, D., Rahman, M. M., Mukherjee, A., Alauddin, M., Hassan, M., Dutta, R. N., et al. (2015). Groundwater Arsenic Contamination in Bangladesh-21 Years of Research. J. Trace Elem. Med. Biol. 31, 237–248. doi:10.1016/j.jtemb.2015.01.003
Chowdhury, N. R., Das, A., Mukherjee, M., Swain, S., Joardar, M., De, A., et al. (2020). Monsoonal Paddy Cultivation with Phase-wise Arsenic Distribution in Exposed and Control Sites of West Bengal, Alongside its Assimilation in rice Grain. J. Hazard. Mater. 400, 123206. doi:10.1016/j.jhazmat.2020.123206
Chowdhury, N. R., Das, R., Joardar, M., Ghosh, S., Bhowmick, S., and Roychowdhury, T. (2018). Arsenic Accumulation in Paddy Plants at Different Phases of Pre-monsoon Cultivation. Chemosphere 210, 987–997. doi:10.1016/j.chemosphere.2018.07.041
Codex Alimentarius Commission, (2017). Joint FAO/WHO Food Standards Programme Codex Committee on Contaminants in Foods. Eleventh Session, Rio de Janeiro, Brazil. (Accessed on 07 02, 2021).
Cohen, S. M., Arnold, L. L., Beck, B. D., Lewis, A. S., Eldan, M., and Eldan, M. (2013). Evaluation of the Carcinogenicity of Inorganic Arsenic. Crit. Rev. Toxicol. 43 (9), 711–752. doi:10.3109/10408444.2013.827152
Dahal, B. M., Fuerhacker, M., Mentler, A., Karki, K. B., Shrestha, R. R., and Blum, W. E. H. (2008). Arsenic Contamination of Soils and Agricultural Plants through Irrigation Water in Nepal. Environ. Pollut. 155 (1), 157–163. doi:10.1016/j.envpol.2007.10.024
Dipti, S. S., Bergman, C., Indrasari, S. D., Herath, T., Hall, R., Lee, H., et al. (2012). The Potential of rice to Offer Solutions for Malnutrition and Chronic Diseases. Rice (N Y) 5 (16), 16–18. doi:10.1186/1939-8433-5-16
Dittmar, J., Voegelin, A., Roberts, L. C., Hug, S. J., Saha, G. C., Ali, M. A., et al. (2010). Arsenic Accumulation in a Paddy Field in Bangladesh: Seasonal Dynamics and Trends over a Three-Year Monitoring Period. Environ. Sci. Technol. 44 (8), 2925–2931. doi:10.1021/es903117r
Dousova, B., Buzek, F., Lhotka, M., Krejcova, S., and Boubinova, R. (2016). Leaching Effect on Arsenic Mobility in Agricultural Soils. J. Hazard. Mater. 307, 231–239. doi:10.1016/j.jhazmat.2015.12.030
Dreiseitl, S., and Ohno-Machado, L. (2002). Logistic Regression and Artificial Neural Network Classification Models: a Methodology Review. J. Biomed. Inform. 35, 352–359. doi:10.1016/s1532-0464(03)00034-0
FAO (2017). Rice Market Monitor. https://www.fao.org/3/I9243EN/i9243en.pdf. (Accessed on October, 2021).
Food and Agriculture Organization Fao, (1992). Wastewater Treatment and Use in Agriculture - FAO Irrigation and Drainage Paper 47. Rome, Italy: FAO.
Golui, D., Guha Mazumder, D. N., Sanyal, S. K., Datta, S. P., Ray, P., Patra, P. K., et al. (2017). Safe Limit of Arsenic in Soil in Relation to Dietary Exposure of Arsenicosis Patients from Malda District, West Bengal- A Case Study. Ecotoxicology Environ. Saf. 144, 227–235. doi:10.1016/j.ecoenv.2017.06.027
GRiSP Global Rice Science Partnership (2013). Rice Almanac. 4th edition. Los Baños (Philippines): International Rice Research Institute, 283. (Accessed on 07 25, 2021).
Hsu, W.-M., Hsi, H.-C., Huang, Y.-T., Liao, C.-S., and Hseu, Z.-Y. (2012). Partitioning of Arsenic in Soil-Crop Systems Irrigated Using Groundwater: A Case Study of rice Paddy Soils in Southwestern Taiwan. Chemosphere 86 (6), 606–613. doi:10.1016/j.chemosphere.2011.10.029
Huq, S. M., Joardar, J. C., Parvin, S., Correll, R., and Naidu, R. (2006). Arsenic Contamination in Food-Chain: Transfer of Arsenic into Food Materials through Groundwater Irrigation. J. Health Popul. Nutr. 24 (3), 305–316.
Hussain, M. M., Bibi, I., Niazi, N. K., Shahid, M., Iqbal, J., Shakoor, M. B., et al. (2021). Arsenic Biogeochemical Cycling in Paddy Soil-rice System: Interaction with Various Factors, Amendments and mineral Nutrients. Sci. Total Environ. 773, 145040. doi:10.1016/j.scitotenv.2021.145040
IRRI Knowledge Bank (2021).. http://www.knowledgebank.irri.org/ericeproduction/Importance_of_Rice.htmAccessed on October, 2021).
Islam, S., Rahman, M. M., Islam, M. R., and Naidu, R. (2017). Effect of Irrigation and Genotypes towards Reduction in Arsenic Load in rice. Sci. Total Environ. 609, 311–318. doi:10.1016/j.scitotenv.2017.07.111
James, G., Witten, D., Hastie, T., and Tibshirani, R. (2013). An Introduction to Statistical Learning with Applications in R. New York: Springer. (Accessed on 07 23, 2021).
JECFA (2017). Report of the Eleventh Session of the Codex Committee on Contaminants in Foods. Rio de Janeiro, Brazil: Joint FAO/WHO Food Standards Programme Codex Alimentarius Commission, 8. (Accessed on 07 19, 2021).
Jouanneau, S., Durand, M.-J., Courcoux, P., Blusseau, T., and Thouand, G. (2011). Improvement of the Identification of Four Heavy Metals in Environmental Samples by Using Predictive Decision Tree Models Coupled with a Set of Five Bioluminescent Bacteria. Environ. Sci. Technol., 45, 2925–2931.doi:10.1021/es1031757
Kuhn, M. (2008). Building Predictive Models in R Using the Caret Package. J. Stat. Softw. 28 (5), 1–26. doi:10.18637/jss.v028.i05
Kumar, S. N., Mishra, B. B., Kumar, S., and Mandal, J. (2021). Organo-arsenic Complexation Studies Explaining the Reduction of Uptake of Arsenic in Wheat Grown with Contaminated Irrigation Water and Organic Amendments. Water Air Soil Pollut. 232, 118. doi:10.1007/s11270-021-05065-8
Kumarathilaka, P., Seneweera, S., Meharg, A., and Bundschuh, J. (2018). Arsenic Speciation Dynamics in Paddy rice Soil-Water Environment: Sources, Physico-Chemical, and Biological Factors - a Review. Water Res. 140, 403–414. doi:10.1016/j.watres.2018.04.034
Kumarathilaka, P., Seneweera, S., Ok, Y. S., Meharg, A., and Bundschuh, J. (2019). Arsenic in Cooked rice Foods: Assessing Health Risks and Mitigation Options. Environ. Int. 127, 584–591. doi:10.1016/j.envint.2019.04.004
Kumari, P. B., Singh, Y. K., Mandal, J., Shambhavi, S., Sadhu, S. K., Kumar, R., et al. (2021). Determination of Safe Limit for Arsenic Contaminated Irrigation Water Using Solubility Free Ion Activity Model (FIAM) and Tobit Regression Model. Chemosphere 270, 128630. doi:10.1016/j.chemosphere.2020.128630
Loeppert, R. H., and Biswas, B. K. (2002). Methods of Analysis for Soil Arsenic. College Station, TX, USA: Texas A&M University.
Lu, Y., Adomako, E. E., Solaiman, A. R. M., Islam, M. R., Deacon, C., Williams, P. N., et al. (2009). Baseline Soil Variation Is a Major Factor in Arsenic Accumulation in Bengal delta Paddy rice. Environ. Sci. Technol. 43 (6), 1724–1729. doi:10.1021/es802794w
Majumder, S., and Banik, P. (2019). Geographical Variation of Arsenic Distribution in Paddy Soil, rice and rice-based Products: A Meta-Analytic Approach and Implications to Human Health. J. Environ. Manage., 233, 184, 199doi:10.1016/j.jenvman.2018.12.034
Mandal, J., Golui, D., and Datta, S. P. (2019). Assessing Equilibria of Organo-Arsenic Complexes and Predicting Uptake of Arsenic by Wheat Grain from Organic Matter Amended Soils. Chemosphere 234, 419–426. doi:10.1016/j.chemosphere.2019.06.088
Meharg, A. A., and Rahman, M. M. (2003). Arsenic Contamination of Bangladesh Paddy Field Soils: Implications for rice Contribution to Arsenic Consumption. Environ. Sci. Technol. 37 (2), 229–234. doi:10.1021/es0259842
Ministry of Environment Government of Japan, (2021). https://www.env.go.jp/en/water/soil/sp.html. (Accessed on 07 21, 2021).
Ministry of the Environment, Finland, (2007). Government Decree on the Assessment of Soil Contamination and Remediation Needs (214/2007 (Accessed on 08 01, 2021).
Mondal, D., Banerjee, M., Kundu, M., Banerjee, N., Bhattacharya, U., Giri, A. K., et al. (2010). Comparison of Drinking Water, Raw rice and Cooking of rice as Arsenic Exposure Routes in Three Contrasting Areas of West Bengal, India. Environ. Geochem. Health 32, 463–477. doi:10.1007/s10653-010-9319-5
Mondal, D., Periche, R., Tineo, B., Bermejo, L. A., Rahman, M. M., Siddique, A. B., et al. (2020). Arsenic in Peruvian rice Cultivated in the Major rice Growing Region of Tumbes River basin. Chemosphere 241, 125070. doi:10.1016/j.chemosphere.2019.125070
Mondal, D., and Polya, D. A. (2008). Rice Is a Major Exposure Route for Arsenic in Chakdaha Block, Nadia District, West Bengal, India: A Probabilistic Risk Assessment. Appl. Geochem. 23, 2987–2998. doi:10.1016/j.apgeochem.2008.06.025
Mukherjee, A., Kundu, M., Basu, B., Sinha, B., Chatterjee, M., Bairagya, M. D., et al. (2017). Arsenic Load in rice Ecosystem and its Mitigation through Deficit Irrigation. J. Environ. Manage. 197, 89–95. doi:10.1016/j.jenvman.2017.03.037
Mukherjee, A., Sarkar, S., Chakraborty, M., Duttagupta, S., Bhattacharya, A., Saha, D., et al. (2021). Occurrence, Predictors and Hazards of Elevated Groundwater Arsenic across India through Field Observations and Regional-Scale AI-Based Modeling. Sci. Total Environ. 759, 143511. doi:10.1016/j.scitotenv.2020.143511
Mwale, T., Rahman, M., and Mondal, D. (2018). Risk and Benefit of Different Cooking Methods on Essential Elements and Arsenic in rice. Ijerph 15 (6), 1056. doi:10.3390/ijerph15061056
Panaullah, G. M., Alam, T., Hossain, M. B., Loeppert, R. H., Lauren, J. G., Meisner, C. A., et al. (2008). Arsenic Toxicity to rice (Oryza Sativa L.) in Bangladesh. Plant Soil 317, 31–39. doi:10.1007/s11104-008-9786-y
Pescod, M. B. (1992). Wastewater Treatment and Use in Agriculture - FAO Irrigation and Drainage Paper 47. Rome, Italy: FAO. Accessed on 07 24, 2021).
Punshon, T., Jackson, B. P., Meharg, A. A., Warczack, T., Scheckel, K., and Guerinot, M. L. (2017). Understanding Arsenic Dynamics in Agronomic Systems to Predict and Prevent Uptake by Crop Plants. Sci. Total Environ. 581-582, 209–220. doi:10.1016/j.scitotenv.2016.12.111
Rahaman, S., Sinha, A. C., Pati, R., and Mukhopadhyay, D. (2013). Arsenic Contamination: a Potential hazard to the Affected Areas of West Bengal, India. Environ. Geochem. Health 35, 119–132. doi:10.1007/s10653-012-9460-4
Rahaman, S., and Sinha, A. C. (2013). Water Regimes: an Approach of Mitigation Arsenic in Summer rice (Oryza Sativa L.) under Different Topo Sequences on Arsenic-Contaminated Soils of Bengal delta. Paddy Water Environ. 11 (1), 397–410. doi:10.1007/s10333-012-0331-5
Rahman, M. A., Hasegawa, H., Rahman, M. M., Rahman, M. A., and Miah, M. A. M. (2007). Accumulation of Arsenic in Tissues of rice Plant (Oryza Sativa L.) and its Distribution in Fractions of rice Grain. Chemosphere 69 (6), 942–948. doi:10.1016/j.chemosphere.2007.05.044
Rahman, M. M., Ng, J. C., and Naidu, R. (2009). Chronic Exposure of Arsenic via Drinking Water and its Adverse Health Impacts on Humans. Environ. Geochem. Health 31, 189–200. doi:10.1007/s10653-008-9235-0
Rahman, M. S., Abdul Mazid Miah, M., Khaled, H. M., Islam, A., and Panaullah, G. M. (2010). Arsenic Concentrations in Groundwater, Soils, and Irrigated rice in Southwestern Bangladesh. Commun. Soil Sci. Plant Anal. 41 (16), 1889–1895. doi:10.1080/00103624.2010.495800
Rahman, M. S., Islam, M. N., Hassan, M. Z., Islam, S. A., and Zaman, S. K. (2014). Impact of Water Management on the Arsenic Content of rice Grain and Cultivated Soil in an Arsenic Contaminated Area of Bangladesh. J. Environ. Sci. Nat. Resour. 7 (2), 43–46.
Raj, A., Mandal, J., Golui, D., Sihi, D., Dari, B., Kumari, P. B., et al. (2021). Determination of Suitable Extractant for Estimating Plant Available Arsenic in Relation to Soil Properties and Predictability by Solubility‐FIAM, Water Air Soil Pollut. 232–247.
Reid, M. C., Asta, M. P., Falk, L., Maguffin, S. C., Cong Pham, V. H., Le, H. A., et al. (2021). Associations between Inorganic Arsenic in rice and Groundwater Arsenic in the Mekong Delta. Chemosphere 265, 129092. doi:10.1016/j.chemosphere.2020.129092
Ripley, B. D. (1996). Pattern Recognition and Neural Networks. Cambridge: Cambridge University Press. (Accessed on 07 23, 2021).
Roychowdhury, T. (2008a). Impact of Sedimentary Arsenic through Irrigated Groundwater on Soil, Plant, Crops and Human Continuum from Bengal delta: Special Reference to Raw and Cooked rice. Food Chem. Toxicol. 46 (8), 2856–2864. doi:10.1016/j.fct.2008.05.019
Roychowdhury, T., Uchino, T., and Tokunaga, H. (2008b). Effect of Arsenic on Soil, Plant and Foodstuffs by Using Irrigated Groundwater and Pond Water from Nadia District, West Bengal. Int. J. Environ. Pollut. 33 (2-3), 218–234. doi:10.1504/ijep.2008.019395
Sarkar, S., Basu, B., Kundu, C. K., and Patra, P. K. (2012). Deficit Irrigation: An Option to Mitigate Arsenic Load of rice Grain in West Bengal, India. Agric. Ecosyst. Environ. 146 (1), 147–152. doi:10.1016/j.agee.2011.10.008
Schmidt, C. W. (2015). In Search of “Just Right”: the challenge of Regulating Arsenic in rice. Environ. Health Perspect. 123 (1), A16–A19. doi:10.1289/ehp.123-A16
Sengupta, S., Bhattacharyya, K., Mandal, J., Bhattacharya, P., Halder, S., and Pari, A. (2021). Deficit Irrigation and Organic Amendments Can Reduce Dietary Arsenic Risk from rice: Introducing Machine Learning-Based Prediction Models from Field Data. Agric. Ecosyst. Environ. 319, 107516. doi:10.1016/j.agee.2021.107516
Shakoor, M. B., Nawaz, R., Hussain, F., Raza, M., Ali, S., Rizwan, M., et al. (2017). Human Health Implications, Risk Assessment and Remediation of As-Contaminated Water: a Critical Review. Sci. Total Environ. 601-602, 756–769. doi:10.1016/j.scitotenv.2017.05.223
Sharma, S., Kaur, I., and Nagpal, A. K. (2017). Assessment of Arsenic Content in Soil, rice Grains and Groundwater and Associated Health Risks in Human Population from Ropar Wetland, India, and its Vicinity. Environ. Sci. Pollut. Res. 24 (23), 18836–18848. doi:10.1007/s11356-017-9401-y
Sinha, B., and Bhattacharyya, K. (2014). Arsenic Toxicity in rice with Special Reference to Speciation in Indian Grain and its Implication on Human Health. J. Sci. Food Agric. 95 (7), 1435–1444. doi:10.1002/jsfa.6839
Srivastava, P. K., Singh, M., Gupta, M., Singh, N., Kharwar, R. N., Tripathi, R. D., et al. (2015). Mapping of Arsenic Pollution with Reference to Paddy Cultivation in the Middle Indo-Gangetic Plains. Environ. Monit. Assess. 187 (4), 198–214. doi:10.1007/s10661-015-4418-5
Stone, M. (1974). Cross-Validatory Choice and Assessment of Statistical Predictions. J. R. Stat. Soc. Ser. B (Methodological) 36, 111–133. doi:10.1111/j.2517-6161.1974.tb00994.x
Talukder, A. S. M. H. M., Meisner, C. A., Sarkar, M. A. R., and Islam, M. S. (2011). Effect of Water Management, Tillage Options and Phosphorus Status on Arsenic Uptake in rice. Ecotoxicology Environ. Saf. 74 (4), 834–839. doi:10.1016/j.ecoenv.2010.11.004
Tóth, G., Hermann, T., Da Silva, M. R., and Montanarella, L. (2016). Heavy Metals in Agricultural Soils of the European Union with Implications for Food Safety. Environ. Int. 88, 299–309. doi:10.1016/j.envint.2015.12.017
Van Geen, A., Zheng, Y., Cheng, Z., He, Y., Dhar, R. K., Garnier, J. M., et al. (2006). Impact of Irrigating rice Paddies with Groundwater Containing Arsenic in Bangladesh. Sci. Total Environ. 367 (2-3), 769–777. doi:10.1016/j.scitotenv.2006.01.030
Wang, L., Gao, S., Yin, X., and Yu, X. (2019). Arsenic Accumulation, Distribution and Source Analysis of rice in a Typical Growing Area in north China. Ecotoxicology Environ. Saf. 167, 429–434. doi:10.1016/j.ecoenv.2018.10.015
Wang, Y., Wu, S., Yan, D., Li, F., Chengcheng, W., Min, C., et al. (2020). Determining and Mapping the Spatial Mismatch between Soil and rice Cadmium (Cd) Pollution Based on a Decision Tree Model. Environ. Pollut. 265, 115029. doi:10.1016/j.envpol.2020.115029
Williams, P. N., Villada, A., Deacon, C., Raab, A., Figuerola, J., Green, A. J., et al. (2007). Greatly Enhanced Arsenic Shoot Assimilation in Rice Leads to Elevated Grain Levels Compared to Wheat and Barley. Environ. Sci. Technol. 41, 6854–6859. doi:10.1021/es070627i
Worth, A. P., and Cronin, M. T. D. (2003). The Use of Discriminant Analysis, Logistic Regression and Classification Tree Analysis in the Development of Classification Models for Human Health Effects. J. Mol. Struct. THEOCHEM 622, 97–111. doi:10.1016/s0166-1280(02)00622-x
Keywords: arsenic, rice, paddy soil, irrigation water, meta-analysis, decision tree, Asia
Citation: Mandal J, Sengupta S, Sarkar S, Mukherjee A, Wood MD, Hutchinson SM and Mondal D (2021) Meta-Analysis Enables Prediction of the Maximum Permissible Arsenic Concentration in Asian Paddy Soil. Front. Environ. Sci. 9:760125. doi: 10.3389/fenvs.2021.760125
Received: 17 August 2021; Accepted: 29 October 2021;
Published: 03 December 2021.
Edited by:
Raul Perez Lejano, New York University, United StatesReviewed by:
Surjit Singh, Academia Sinica, TaiwanPankaj Kumar Verma, Ben-Gurion University of the Negev, Israel
Copyright © 2021 Mandal, Sengupta, Sarkar, Mukherjee, Wood, Hutchinson and Mondal. This is an open-access article distributed under the terms of the Creative Commons Attribution License (CC BY). The use, distribution or reproduction in other forums is permitted, provided the original author(s) and the copyright owner(s) are credited and that the original publication in this journal is cited, in accordance with accepted academic practice. No use, distribution or reproduction is permitted which does not comply with these terms.
*Correspondence: Jajati Mandal, J.Mandal1@edu.salford.ac.uk; Debapriya Mondal, dmondal@sgul.ac.uk