- 1Department of Biological Sciences, Virginia Tech, Blacksburg, VA, United States
- 2Department of Forest Resources and Environmental Conservation, Virginia Tech, Blacksburg, VA, United States
Near-term, ecological forecasting with iterative model refitting and uncertainty partitioning has great promise for improving our understanding of ecological processes and the predictive skill of ecological models, but to date has been infrequently applied to predict biogeochemical fluxes. Bubble fluxes of methane (CH4) from aquatic sediments to the atmosphere (ebullition) dominate freshwater greenhouse gas emissions, but it remains unknown how best to make robust near-term CH4 ebullition predictions using models. Near-term forecasting workflows have the potential to address several current challenges in predicting CH4 ebullition rates, including: development of models that can be applied across time horizons and ecosystems, identification of the timescales for which predictions can provide useful information, and quantification of uncertainty in predictions. To assess the capacity of near-term, iterative forecasting workflows to improve ebullition rate predictions, we developed and tested a near-term, iterative forecasting workflow of CH4 ebullition rates in a small eutrophic reservoir throughout one open-water period. The workflow included the repeated updating of a CH4 ebullition forecast model over time with newly-collected data via iterative model refitting. We compared the CH4 forecasts from our workflow to both alternative forecasts generated without iterative model refitting and a persistence null model. Our forecasts with iterative model refitting estimated CH4 ebullition rates up to 2 weeks into the future [RMSE at 1-week ahead = 0.53 and 0.48 loge(mg CH4 m−2 d−1) at 2-week ahead horizons]. Forecasts with iterative model refitting outperformed forecasts without refitting and the persistence null model at both 1- and 2-week forecast horizons. Driver uncertainty and model process uncertainty contributed the most to total forecast uncertainty, suggesting that future workflow improvements should focus on improved mechanistic understanding of CH4 models and drivers. Altogether, our study suggests that iterative forecasting improves week-to-week CH4 ebullition predictions, provides insight into predictability of ebullition rates into the future, and identifies which sources of uncertainty are the most important contributors to the total uncertainty in CH4 ebullition predictions.
Introduction
Near-term (day to year) ecological forecasting can improve our understanding and quantification of ecosystem processes (Dietze et al., 2018). The repeated updating of models used to generate forecasts (often called data assimilation or data-model fusion; Luo et al., 2011) is crucial for forecasting because it can iteratively improve forecast skill by updating model initial conditions or recalibrating model parameters (Luo et al., 2011; Dietze, 2017a). The iterative process of making a forecast with a model, collecting observations to compare with the forecast, and then updating the forecast models before making a new forecast embodies the scientific method and can substantially improve our representation of ecosystem processes in models (Dietze, 2017b). If the observations match the forecast, the hypothesis (as represented by the forecast model) is supported, otherwise, it provides an opportunity for revising the hypothesis by, e.g., updating model parameters, modifying the model’s driver variables, or changing the model’s equations. Thus, near-term, iterative forecasting creates a model-data feedback loop that evaluates how effectively a model predicts future ecosystem states, with the forecasts evolving as the ecosystem experiences different environmental conditions.
While near-term, iterative forecasting has been successfully applied to predict other ecological variables, to date the approach has not been broadly used in aquatic biogeochemistry. One biogeochemical process that near-term, iterative forecasts may improve predictions for is methane (CH4) ebullition, or bubble fluxes of CH4 from organic-rich sediments to the waterbody surface. Freshwater ecosystems emit large quantities of CH4 to the atmosphere (currently estimated between 117 and 212 Tg CH4 yr−1; Saunois et al., 2020). Within human-made reservoirs alone, ebullition rates can vary substantially in emissions, ranging from 1 to 1,000 mg CH4 m−2 d−1 (Deemer et al., 2016) and up to 8.9 Tg CH4 yr−1 (Johnson et al., 2021). Among the different types of freshwater CH4 emissions, ebullition can make up anywhere from 0 to 99.6% of total emissions to the atmosphere (Deemer and Holgerson, 2021) and it is considered one of the most uncertain fluxes in both inland water and global CH4 budgets (Wik et al., 2016; Saunois et al., 2020).
Ebullition’s high spatial and temporal variation makes it difficult to quantify experimentally and create appropriate models, resulting in three challenges that stand in the way of developing robust predictions of CH4 ebullition fluxes from inland waters. These include 1) model transferability across time and space (i.e., the ability to apply ebullition models across years and ecosystems); 2) identification of the forecast horizons for which future estimates provide useful information (Petchey et al., 2015); and 3) quantification of uncertainty in model predictions. First, while there are multiple different CH4 ebullition models for inland waters, e.g., process-based models that couple physical, biogeochemical, and bubble plume modules (Schmid et al., 2017), empirical models that use physical, chemical and biological drivers as predictors of CH4 ebullition fluxes (DelSontro et al., 2016; Aben et al., 2017; Grasset et al., 2021), and auto-regressive (AR) time series models (McClure et al., 2020a), it remains unknown how well they can predict CH4 ebullition dynamics over time within the same ecosystem. Moreover, given the clear interannual variability in ebullition rates across inland water systems (Burke et al., 2019; Männistö et al., 2019; Linkhorst et al., 2020), quantifying the transferability of CH4 ebullition model predictions from 1 year to another is critical for CH4 ebullition modeling. Knowing, for example, if the same model was robust for predicting CH4 ebullition across multiple years vs. if new models were needed to predict CH4 ebullition each year would greatly assist CH4 scaling efforts.
Second, the ability of current CH4 ebullition models to predict future out-of-sample observations across varying forecast horizons remains untested. An important step for improving the robustness of CH4 ebullition predictions is to use models to generate out-of-sample predictions for CH4 ebullition rates at different future horizons (e.g., days, months, or years into the future) and then compare these predictions with observations when they are available. It is possible that CH4 ebullition models may successfully simulate observed data at a given forecast horizon, but not effectively provide insight as to how far into the future the predictions provide useful information (Petchey et al., 2015). Here, we propose that exploring the transferability and effective forecast horizon of CH4 ebullition rates using near-term forecasting can serve as a robust tool for improving our understanding of future CH4 ebullition rate predictions.
Third, near-term iterative forecast workflows need to account for uncertainty in predictions, which provides a critical metric of confidence in forecasts. Moreover, partitioning the different sources of uncertainty can provide insight into model performance and measurements, which has the potential to improve aspects of the model and predictions (Dietze, 2017a; Carey et al., 2021a). In particular, forecast workflows that apply state-space Bayesian methods with iterative model refitting enables the partitioning of sources of uncertainty (e.g., parameters, initial conditions, driver data, and model processes; Dietze, 2017a; Luo et al., 2011), which is crucial for identifying strategies for improving the design for future measurements and models. For example, Thomas et al. (2018) used a state-space hierarchical Bayesian model to forecast biomass change in loblolly pine (Pinus taeda) forests in the southeastern United States and then evaluated the relative contribution of different forms of uncertainty to the total forecast uncertainty to guide improvements on future biomass predictions. However, accounting for and partitioning uncertainty still remains uncommon in ecological modeling (Harrison et al., 2017; Lewis et al., 2021), especially in forecasts of future biogeochemical processes like CH4 ebullition (Lewis et al., 2021). Thus, developing a forecast workflow that implements uncertainty partitioning at every time step will provide key insight to future model development for CH4 ebullition rates.
The goal of this work was to assess the potential of forecasting CH4 ebullition as a case study to evaluate its potential more broadly. Given the complexity of ebullition as a biogeochemical process with many different mechanisms that control its fluxes, our goal was to see if we could successfully forecast CH4, which would support its application to other aquatic biogeochemical processes. We developed an iterative forecasting workflow of weekly CH4 ebullition rates in a freshwater reservoir up to 2 weeks into the future using forecasted water temperatures, ebullition rate observations, and a state-space model fit in a Bayesian framework with iterative model refitting. We refit our model with new observations to update our ebullition model’s states, parameters, initial conditions, and driver data on each model time step and tested our ebullition rate forecast workflow against a similar forecast workflow that did not use iterative model refitting and a persistence null model. We also quantified the contributions of different sources of uncertainty to CH4 ebullition forecasts at each time step. Our objectives were: 1) develop an iterative, near-term forecasting workflow of CH4 ebullition rates and apply it to assess a CH4 ebullition model’s interannual transferability; 2) determine the effective forecast horizon of CH4 ebullition; and 3) quantify and partition the sources of uncertainty in our forecasts to inform future workflow development. Altogether, our goal was to examine the potential of near-term iterative forecasting for improving the representation of CH4 ebullition in models and providing insight into this important biogeochemical flux.
Materials and Methods
Site Description
We developed near-term, real-time, iterative CH4 ebullition rate forecasts in Falling Creek Reservoir (FCR, Figure 1) in summer 2019 and tested the efficacy of different forecasting workflows against observations of CH4 ebullition rates at different forecast horizons. FCR is a small (0.119 km2), shallow (Zmax = 9.3 m), eutrophic, drinking water reservoir located in southwestern Virginia, United States (37.30°N, 79.84°W). FCR is owned and operated by the Western Virginia Water Authority as a drinking water supply and is in a completely forested watershed (Gerling et al., 2016). FCR’s water level was managed to stay at a constant level and did not experience substantial fluctuations during this study (Carey et al., 2021a).
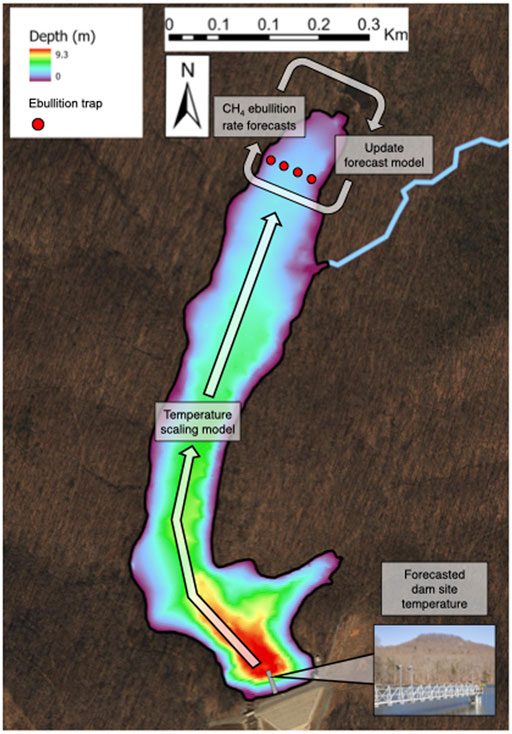
FIGURE 1. The bathymetry of Falling Creek Reservoir superimposed by a depiction of how our forecast workflow mapped spatially from the dam to the upstream transect where CH4 ebullition rates were forecasted. Our forecast workflow used water temperature forecasts from the dam site in the reservoir (following Thomas et al., 2020). The water temperature forecasts were scaled to an upstream transect (denoted by red dots on map) to generate near-term iterative CH4 ebullition rate forecasts. Simultaneously, direct observations from ebullition traps were used to iteratively update the forecast model via data assimilation.
The forecasting workflow used a model developed from a previous summer sampling season (2017) of CH4 ebullition monitoring data collected at FCR to build and calibrate our forecast models (McClure et al., 2020b). In 2017, four ebullition traps were deployed and monitored weekly from 8 May to 24 October along a shallow upstream transect (McClure et al., 2020a; Figure 1). In 2019, the year of this forecasting study, we redeployed all four traps as close as possible to their original locations in 2017 and forecasted measurements collected weekly throughout the summer. Ebullition rates were natural log-transformed (loge) + 0.1 to meet the assumptions of normality and avoid forecasts of negative ebullition rates in log-space.
Forecast Model and Model-Fitting
Our forecast model was an auto-regressive (AR) time series model with sediment-water interface (SWI) temperatures as a driver:
where
We estimated the posterior distributions of the parameters in the model using a state-space Bayesian framework. This framework includes latent states that represent the “true” ebullition rate before observation uncertainty is added. The latent state has a distribution that represents uncertainty in our capacity to model the true ebullition rate. Following Eq. 1, the state-spaced framework uses the previous time step’s latent state in the AR term:
The latent state is normally distributed with a mean equal to the prediction from Eq. 2 and standard deviation of
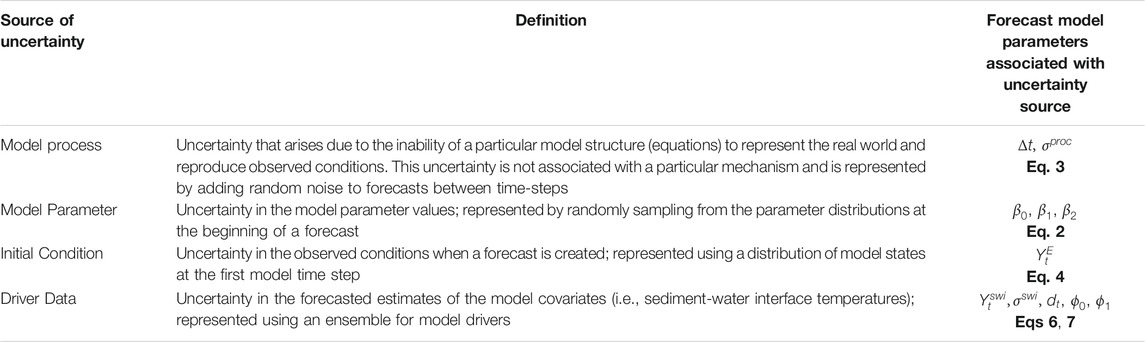
TABLE 1. Definitions of uncertainty sources that can contribute to total forecast uncertainty and what parameter and variables from the Eqs 2, 3, 4, 6, 7 correspond to the total uncertainty (derived from Dietze, 2017a).
We set the priors for the
Our model-fitting process involved four steps. First, we estimated posterior distributions of the parameters using data from summer 2017 that included weekly measurements of CH4 ebullition rate and SWI temperature using Markov chain Monte Carlo (MCMC) analyses. MCMC analyses are particularly well-suited for forecasting because they numerically estimate probability distributions for model parameter, the latent states, and process uncertainty. These distributions can subsequently be used to quantitatively assess the uncertainty associated with each aspect of the model (parameters, initial conditions, and process error; see Table 1). The MCMC analyses were carried out using the “rjags” and “R2jags” packages (Plummer, 2018) within the R statistical environment (R Core Team, 2021). We ran three MCMC chains for 70,000 iterations, including 20,000 initial iterations that were discarded as a burn-in. We assessed convergence using the potential scale reduction factor (PSRF) of the Gelman-Rubin statistic, and parameters were determined to have converged if the PSRF was between 1 and 1.05 (Gelman and Rubin, 1992).
Second, we generated prior distributions for a refitting of the state-spaced model using 2019 data that were derived from posteriors from the 2017 model-fitting. The posterior distributions from the
Third, during the summer of 2019, we refitted the model weekly as new data were collected. The refitting involved starting with the priors from the 2017 model-fitting, appending the new data to the previously collected 2019 data, and re-running the MCMC estimation of posteriors using the 2019 data. The first measurement of 2019 was used as the initial conditions for Eq. 2. As a result of this analysis design, we expected the posterior parameter distributions to reflect the prior distributions (i.e., the 2017 posteriors) early in the summer, when data from 2019 were limited, but the posterior parameter distribution would increasingly be influenced by data in 2019 as more data were collected.
Finally, the posterior estimates for the parameters (
Overview of Forecasting Workflow
Here, we outline the implementation of the forecasting workflow based on the model development and fitting described in Forecast Model and Model-Fitting, and then describe how the forecasted driver data were generated in Forecasting SWI Temperature. Approximately each week between 17 June and November 7, 2019, we generated forecasts of CH4 ebullition rates that extended approximately 2 weeks into the future (Figure 2). The forecast horizon varied between 13 and 16 days ahead depending on the exact days that the observations were ultimately collected. The forecasts presented here are technically hindcasts because they were generated after the observations were collected; however, a preliminary version of the forecasts were generated using a similar approach in real-time during the summer of 2019 (and thus those are considered true forecasts).
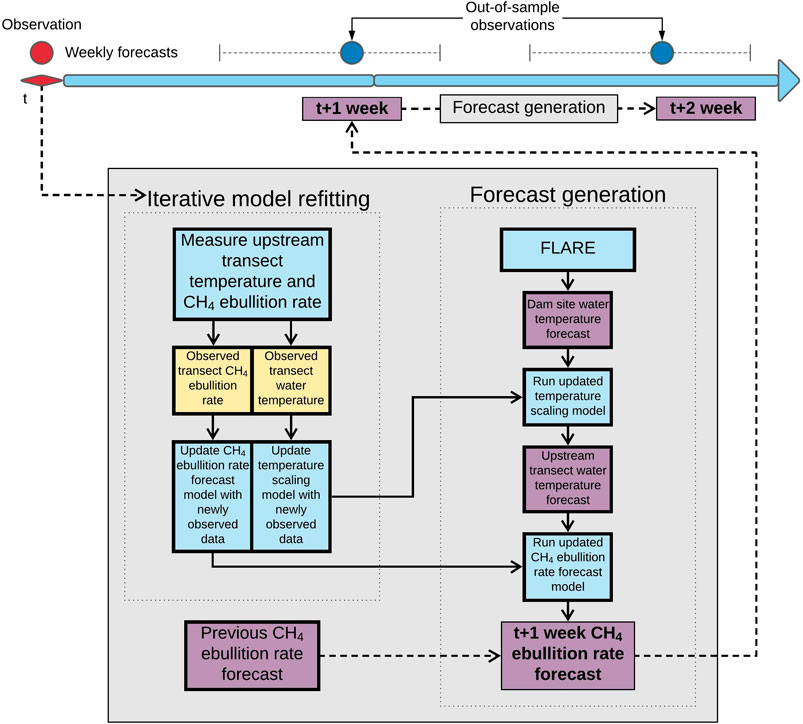
FIGURE 2. Conceptual illustration of the forecast workflow using iterative model refitting that generates near-term, iterative CH4 ebullition forecasts. The model refitting stage occurred at the time when new ebullition rates were manually collected from the reservoir. In the shaded part of the figure, the blue boxes represent processes, and the yellow and purple boxes represent the data products that resulted from each process. The yellow boxes are manually-collected data products that were used to update the forecast model and the purple boxes represent forecasted data products.
The forecasts were generated using an MCMC approach to numerically represent forecast uncertainty. Because MCMC permits estimation of model parameters, latent states, and process uncertainty parameter as distributions rather than fixed values, an MCMC approach enables estimation of the uncertainty associated with each of these model components by making multiple draws from estimated distributions to build an ensemble of possible forecast outcomes. Our approach used an ensemble with 210 members. First, to represent initial condition uncertainty, the forecast was initialized using 210 random draws from a normal distribution with a mean equal to the observation at the start of the forecast (
Forecasting SWI Temperature
Because we generated forecasts of future CH4 ebullition rates, forecasts of the model covariates (i.e., SWI temperatures) were needed as part of our workflow (Figure 1, 2). To produce forecasts of SWI temperature, we used the existing Forecasting Lake And Reservoir Ecosystems (FLARE; Thomas et al., 2020) water temperature forecasting framework and infrastructure deployed in FCR to generate predictions of future water temperatures as driver data for our CH4 ebullition rate forecasts at all four traps on the transect. FLARE uses near-real time temperature observations, weather forecasts, and a hydrodynamic model (General Lake Model) to forecast water temperatures up to 16 days into the future at the “dam site” at FCR, which was located ∼700 m downstream from the ebullition traps (Figure 1). To generate SWI forecasts at the upstream ebullition transect, which had a depth of 1–3 m, we developed a temperature scaling model that converted the FLARE forecasts between 1 and 3 m at the dam site to forecasts at the transect. We estimated the posterior distributions of the parameters in the temperature scaling model using a simple (non-state space) Bayesian framework where:
and
To generate the forecast of SWI temperature, first we forecasted water temperature at the dam site using FLARE (Thomas et al., 2020) up to 16-days into the future. This produced a 210-member ensemble with uncertainty primarily derived from uncertainty in weather predictions from the NOAA Global Ensemble Forecasting System and process uncertainty associated with the hydrodynamic lake model underlying FLARE (Thomas et al., 2020). Second, we collected the mean temperature forecasts at 1- and 2-week horizons of the 1 and 3 m depth forecasts for each FLARE member ensemble and applied these values as
Field Data Collection
Approximately every week during the forecast period, we measured CH4 ebullition rates and SWI temperature manually to update the daily forecasts (Figure 2). We collected 10-min SWI temperature data with HOBO temperature loggers (HOBO Pendant Temperature/Light Data Logger, Bourne, MA, United States) deployed below each of the four ebullition traps during the 2017 training period and 2019 forecast period. Each logger was sunk using a stainless-steel weight and a nylon string to sit ∼0.1 cm above the SWI. We affixed the bottom logger ∼1 m horizontally away from each ebullition trap to prevent potential disturbance of sediments under the traps. We simultaneously downloaded the temperature logger data as ebullition bubbles which had accumulated in the traps since the last sampling date were being collected. The temperature loggers were downloaded using HOBOware version 3.7.13.
The dam site water temperature data (Figure 1) were collected using a CTD (Conductivity, Temperature, and Depth) profiler in 2017 and water temperature thermistors in 2019. In 2017, the dam site water temperature depth profiles were collected with the CTD on the same days ebullition rates were collected from the traps in the upstream transect. The CTD measured temperature every 0.25 s during the profile, resulting in temperature depth profiles at approximately 10-cm resolution through the water column. Further information and all thermistor and CTD data are available in the Environmental Data Initiative (EDI) repository following Carey et al. (2021a) and Carey et al. (2021b).
We sampled the CH4 ebullition rates at the four upstream traps following McClure et al. (2020b). The ebullition samples from each trap on the transect were extracted across a septum stopper using a needle attached to a 10-ml syringe. We injected 10 ml of ebullition gas into a 20-ml crimped top glass vial that was pre-filled with saturated salt brine solution. A secondary exit syringe extracted the salt brine solution as the sample was injected to generate 10 ml of gas headspace in the vial. Two replicates were collected from each trap on a sampling day if enough gas sample was available, resulting in up to eight possible ebullition rate samples among the four traps. The vials were stored upside down until analysis, so the remaining 10 ml of salt brine solution acted as a barrier to prevent any gas from escaping. We extracted any remaining gas from each ebullition trap using a 30-ml syringe and summed the total volume of ebullition gas collected each week.
We analyzed the manually-collected ebullition gas from the traps for its CH4 concentration using a Shimadzu Nexus-2030 Gas Chromatography—Flame Ionization Detector (GC-FID; Shimadzu Corporation; Kyoto, Japan) within 24 h of collection (following McClure et al., 2018; McClure et al., 2020b). We determined the observed CH4 ebullition rate (
where
Forecast Analysis
To evaluate the interannual transferability of the ebullition model (Objective 1), we compared the skill of the forecast workflow without iterative model refitting with the forecast workflow with iterative model refitting. If the forecast workflow with iterative model refitting performed substantially better than the workflow without iterative refitting, then model transferability from year to year is low. The alternate forecasting workflow without iterative model refitting used the same AR model (Eq. 2) and the 2019 CH4 ebullition rates as initial conditions for each 2-week forecast but sampled parameters from the posteriors that were only based on the 2017 model-fitting. As a result, the parameters did not iteratively update as new data were collected in 2019.
Next, to evaluate the effect of the forecast horizon of CH4 ebullition rates in our system (Objective 2), we compared the performance of the forecast workflow with iterative model refitting with the persistence null model. If the null model performed better than the forecast workflow with iterative model refitting, then our forecast model of CH4 ebullition rates was no better than a simple model that assumed next week’s rates would be the same as the previous week’s rates. The persistence null model was developed using a similar structure as the forecast models and assumed that CH4 ebullition rates would remain similar into the future with propagated uncertainty. The persistence null model replaced Eq. 2 with:
The persistence null model used the same MCMC methods for estimating posterior distributions as the forecasting model. Similar to the forecasting model, the null model was fit to 2017 data and the posteriors were used as priors in the weekly recalibration of the model using 2019 data.
For both of the analyses described above, we quantified the Nash-Sutcliffe efficiency (NSE) and root-mean-square-error (RMSE) of the CH4 ebullition rate forecasts from the forecast workflow with refitting, the forecast workflow without refitting, and the persistence null forecast model. The NSE is a normalized metric that evaluates a model’s performance relative to the mean of the observed time series (Nash and Sutcliffe, 1970). We calculated the NSE coefficient (Nash and Sutcliffe, 1970) as follows:
where
Forecast Uncertainty Partitioning
We quantified the total forecast uncertainty in CH4 ebullition rate forecasts among all forecast cycles as standard deviation (SD) throughout the forecasting period using the variance of the 210-member ensemble at the 1- and 2-week forecast horizons (Objective 3). Additionally, we partitioned the relative contributions of four different sources of uncertainty, model parameters (
We used a One-At-a-Time Sensitivity (OATS) analysis to determine the relative contribution of the uncertainty sources to total uncertainty (as total forecast variance) in each forecast cycle. An OATS analysis holds all sources of uncertainty at their mean except for one, and then numerically evaluates the sensitivity of the forecast to that specific source of uncertainty (Dietze, 2017b). For example, to partition parameter uncertainty, we held all other sources of uncertainty (model process, driver data, and initial condition) at their mean and only allowed the parameter values to vary among ensemble members. The relative proportion of each source of uncertainty was determined by dividing the total variance of each isolated source of uncertainty by the sum of the variance of all uncertainty sources at both the 1- and 2-week forecast horizons across the forecasting period.
Forecast Archiving and Reproducibility
All forecasting driver data (Carey et al., 2021b; Carey et al., 2021c; McClure et al., 2020b), code needed to forecast weekly CH4 ebullition rates, and the hindcasts generated during the 2019 forecast period are archived either in the Environmental Data Initiative (EDI) repository or Zenodo (McClure et al., 2021). All analyses were run in the R statistical environment version 4.1.0 (R Core Team, 2021).
Results
Observed CH4 Ebullition Rates
Throughout the forecasting period, we observed high temporal variation in mean CH4 ebullition rates at FCR’s upstream transect (Figure 3). Mean ebullition rates at the upstream transect increased from −0.48 to 1.76 loge (mg CH4 m−2 d−1) from 27 May though 24 June (Figure 3). Between 24 June and 15 July, mean CH4 ebullition rates at the transect continued to increase until the maximum CH4 ebullition rate for the forecast period was observed on 15 July [3.88 loge (mg CH4 m−2 d−1)]. Between 15 July and 16 October, the observed CH4 ebullition rates ranged between 1.56 and 3.74 loge (mg CH4 m−2 d−1). After 16 October, CH4 ebullition rates dropped to ≤1.32 loge (mg CH4 m−2 d−1) for the remainder of the forecasting period, which ended on 7 November.
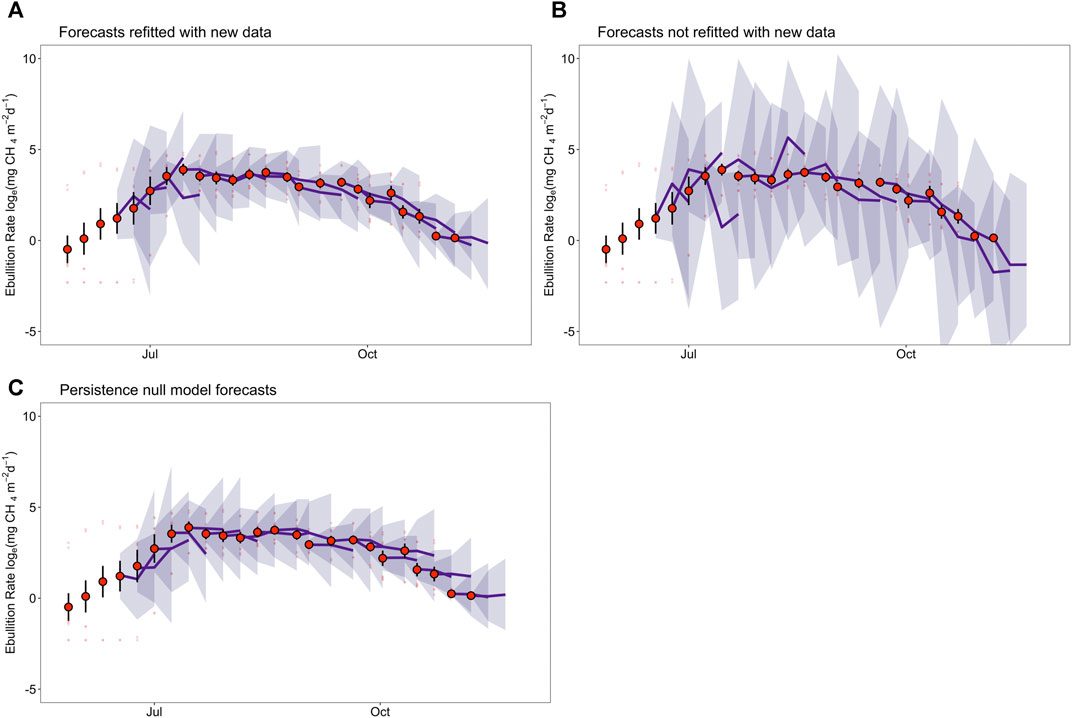
FIGURE 3. A comparison of weekly forecasted CH4 ebullition rates in loge(mg CH4 m−2 d−1) using a workflow in which forecast models were iteratively refitted with new data (A), a workflow in which forecasts were not refitted with new data (B), and a persistence null model (C) from 17 June to November 7, 2019. The large red circles plus black error bars represent the observed daily mean transect CH4 ebullition rate ±1 standard error of the mean from four ebullition traps depicted in Figure 1. The small red circles represent the measured CH4 ebullition rate from each ebullition trap on the transect. The dark purple lines represent the forecasted mean daily ebullition rate from the posterior predictions. The purple shades represent the 95% predictive intervals of the forecasts.
Forecast Evaluation and Model Transferability
All three forecast workflows - the forecast model with iterative refitting, the forecast model without refitting, and the null persistence model - generated 95% predictive intervals that encompassed out-of-sample CH4 ebullition rate observations at both 1- and 2-week forecast horizons (Figure 3). However, we found that there were differences in performance among the workflows based on our evaluation metrics (Table 2). At both 1- and 2-week forecast horizons, the forecast workflow with iterative model refitting exhibited both higher NSE and lower RMSE values than the forecast workflow without refitting (Table 2). Moreover, the forecast workflow with refitting performed better than the null model at both 1- and 2-week forecast horizons (Table 2).

TABLE 2. Forecast evaluation determined by Nash-Sutcliffe Efficiency (NSE) and root mean square error (RMSE) at 1- and 2-week forecast horizons.The best-performing workflow evaluation statistics (as indicated by the highest NSE and lowest RMSE for each forecast horizon) are highlighted in bold for each horizon.
Our result that the iterative model with refitting performed better than the iterative model without refitting indicates that model parameters estimated from 2017 ebullition rates did a relatively poor job of predicting 2019 ebullition rates, and as such, the model’s interannual transferability among years is low. Our finding of low year-to-year transferability informs methods for scaling ebullition models predicting fluxes in one waterbody at one time point to multiple waterbodies over multiple years. Specifically, this result suggests that a single prediction model of ebullition likely will not apply to other years, motivating future work to test this hypothesis in other waterbodies.
Although the forecast workflow without refitting exhibited low transferability across years, the forecast workflow with refitting successfully predicted future CH4 ebullition rates well because of the evolution of the model parameters throughout the 2019 forecasting period. We observed a substantial evolution of the intercept, AR, and SWI temperature parameters (
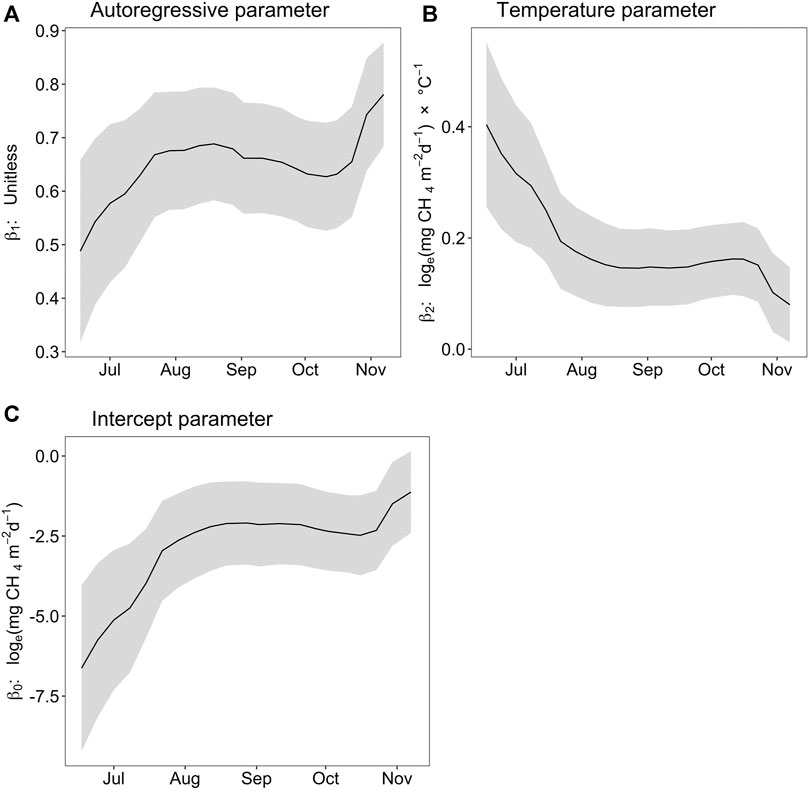
FIGURE 4. Parameter estimates of
Effective Forecast Horizons
The comparison of the workflows and the persistence null model indicated that our model’s effective forecast horizon of CH4 ebullition with iterative model refitting extended up to 2 weeks into the future (Table 2). However, at both 1- and 2-week forecast horizons the null persistence model outperformed the workflow without refitting (Table 2). To understand why the forecast workflow with iterative model refitting consistently performed better than the workflow without refitting and the persistence null model, we explored the sources of forecast uncertainty in the workflow with iterative model refitting.
Forecast Uncertainty and Uncertainty Partitioning
Over the forecasting period, the forecast uncertainty (as measured by standard deviation, SD) consistently increased from 1 to 2 weeks into the future, on average by 32% (Figure 5A). Simultaneously, total uncertainty in both forecast horizons decreased throughout the forecasting period from June to November (Figure 5B). The first forecast (starting 17 June) exhibited the largest uncertainty in both 1- and 2-week forecast horizons, with a SD of 1.67 loge (mg CH4 m−2 d−1) at 1-week and 2.46 loge (mg CH4 m−2 d−1) at the 2-week horizon (Figure 5B). By 15 July, the total forecast SD across the 1- and 2-week horizons decreased to 1.42 and 1.73 loge (mg CH4 m−2 d−1) respectively, a 15 and 30% decrease in forecast SD. The total forecast uncertainty continued to decrease though the forecasting season, and by 7 November, the final forecast of the season, the forecast uncertainty was 0.84 loge (mg CH4 m−2 d−1) at the 1-week horizon and 1.50 loge (mg CH4 m−2 d−1) at the 2-week horizon.
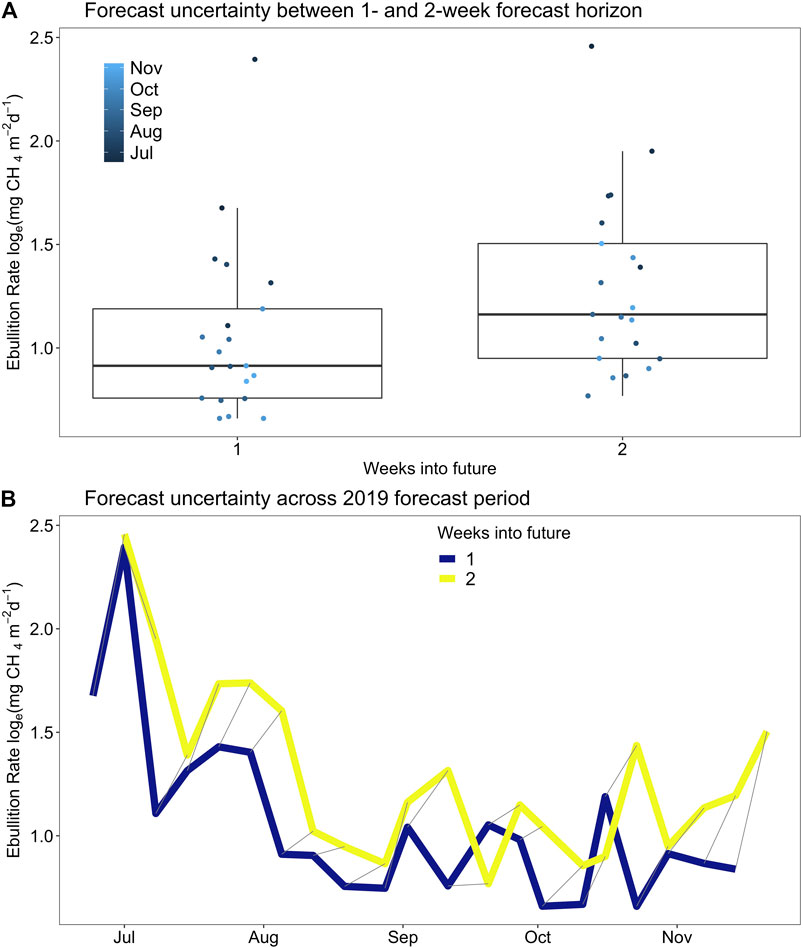
FIGURE 5. The total forecast uncertainty (as measured by standard deviation of the forecast ensembles) for each week aggregated across forecast cycles for 1 and 2 weeks into the future Panel (A). In panel (B), each different colored line represents the number of weeks into the forecast horizon. The small grey lines are included as a guide to represent each forecast and how the uncertainty of each forecast mapped across the forecasting period.
When partitioned, driver data and model process uncertainty were consistently the largest sources of uncertainty in our forecasts at both 1- and 2-week forecast horizons among all forecasts (Figure 6). At the 1-week forecast horizon, driver data uncertainty was the largest source of uncertainty between 17 June and 11 September and then model process uncertainty was the largest source from 11 September to the last forecast on 7 November (Figure 6A). At the 2-week forecast horizon, driver data uncertainty was also the largest source of uncertainty between 17 June and 2 September and then model process and parameter uncertainty became the largest sources of uncertainty from 2 September to the last forecast on 7 November (Figure 6B). When aggregated across the 2019 period for both horizons, driver data uncertainty contributed a mean of 60% of total forecast uncertainty (Figure 6C), indicating that uncertainty in the forecasted water temperatures from FLARE and the uncertainty in the SWI temperature scaling model contributed most to uncertainty in our forecasts. Model process uncertainty was the next highest contributed source of uncertainty at 25% over the entire forecasting period. Both model parameter and initial conditions uncertainty contributed smaller proportions of total variance, with seasonal means of 11 and 4%, respectively (Figure 6C).
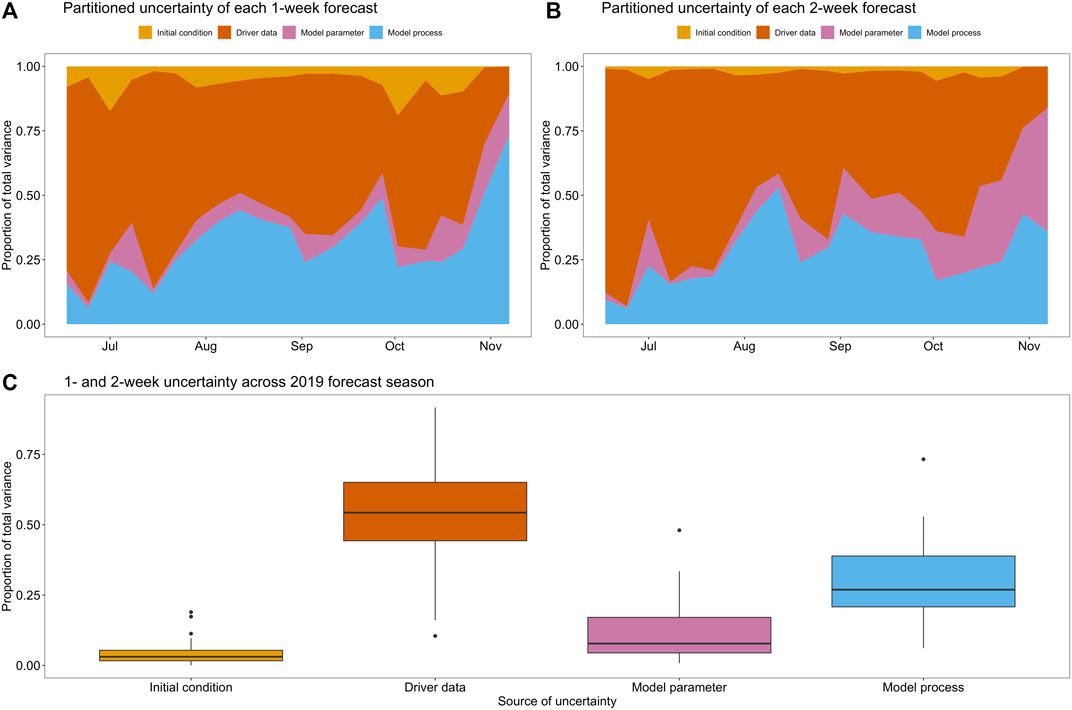
FIGURE 6. Panel (A, B) depicts how the relative contribution of different sources of forecast uncertainty (initial condition, driver, parameter, and process) from the workflow with iterative model refitting evolved over each forecasting cycle starting with the initial day of the forecast cycle 1-week (A; t+1 in Figure 2), and 2-week (B; t+2 in Figure 2) ahead forecasts. Panel (C) depicts the same sources of uncertainty aggregated across all of the forecast cycles from t+1 and t+2 that were evaluated during the 2019 forecast period.
Discussion
Our CH4 ebullition forecasting workflow demonstrated that iterative model refitting improved summer CH4 ebullition rate predictions at both 1- and 2-week forecast horizons (Table 2; Figure 3). The utility of iterative model refitting was demonstrated by the evolution of the model parameter distributions over time (Figure 4) and the improvement of the forecast workflow with refitting over the null model during the forecasting period (Table 2). In addition, the forecasts developed by iterative model refitting exhibited lower overall forecast uncertainty as the forecasting period progressed, as indicated by the reduction in the total SD (Figure 5B). The primary drivers of uncertainty in the forecasts were driver data and model process. Altogether, our CH4 ebullition rate forecasts provide insight to: the low transferability of CH4 ebullition rate prediction models among years (Objective 1), the effective forecast horizon of CH4 ebullition can extend up to 2 weeks in the future (Objective 2), and that improvements to the driver data and the CH4 ebullition models themselves would be most useful for improving CH4 ebullition rate predictions (Objective 3), as discussed below.
Model Interannual Transferability
Our comparison of the forecasting workflows with and without iterative model refitting provided useful insight on the transferability of our CH4 ebullition prediction models among years. The forecast workflow that relied on the fitted 2017 posterior distributions (without iterative refitting) performed worse than the forecasts with iterative model refitting at both 1- and 2-week horizons (Table 2). This difference is likely due to lower overall ebullition rates in 2019 than 2017, which caused the calibrated 2017 posterior distributions to consistently overestimate CH4 ebullition rates in 2019 (Figure 3). These results support previous work observing high interannual variability in CH4 ebullition rates (Burke et al., 2019; Männistö et al., 2019; Linkhorst et al., 2020), and suggest that adopting a modeling workflow that is iteratively refitted with new incoming data throughout the season will improve CH4 ebullition predictions. Given our empirical forecast model (Eq. 2), its low transferability is not surprising and points to the importance of additional covariates that may be important for driving variability in CH4 ebullition rates (e.g., Deemer and Holgerson, 2021). The addition of meaningful covariates would likely increase temporal transferability by increasing the mechanistic ability of the model to recreate observed CH4 ebullition dynamics, as well as decrease total forecast uncertainty, as discussed below.
The evolution of model parameters throughout the forecasting period (Figure 4) highlights the ability of iterative refitting to respond to the changing environmental conditions that occurred between June and November. For example, an increase in the observed ebullition rates (Figure 3) in early July was concomitant with a decrease in the SWI temperature parameter as the reservoir warmed. Between 15 July and 11 October, both the SWI temperature and AR term parameters remained largely stationary as the ebullition rates exhibited a seasonal increase and then decrease (Figure 3). After 11 October, the SWI temperature parameter decreased while the AR term parameter increased to its maximum value. The dynamic parameter values highlight how SWI temperature is likely most important for predicting CH4 ebullition in early summer, prior to the onset of stable thermal stratification. Conversely, SWI temperatures were much less important during the autumn, when there are large changes occurring week-to-week as water at the SWI undergoes dynamic fall mixing conditions.
Effective Forecast Horizons
The better performance of our forecast workflow with iterative model refitting over the persistence null model at both the 1- and 2-week forecast horizons (Table 2) provides insight on how to evaluate the predictive horizon of CH4 ebullition rates. Although CH4 ebullition is often considered a stochastic process (Bastviken et al., 2004; though see; West et al., 2016; Bezerra et al., 2020), our study suggests that CH4 ebullition may be successfully predicted up to 2 weeks ahead at the weekly time step. However, our forecasts do not provide evidence on whether CH4 ebullition rates can be accurately predicted beyond 2 weeks. Additionally, the 32% increase in the forecast ensemble uncertainty (Figure 5) between the 1 and 2-week forecast horizons in our iterative model refitting workflow (Figure 5A), indicates that the 2-week forecasts provide less meaningful predictions than the 1-week forecasts. Thus, even if the mean of the forecast ensemble closely matches the out-of-sample predictions at the 2-week horizon, our 2-week forecasts should be interpreted carefully given the substantial increase in the ensemble SD between the 1- and 2-week horizons. Altogether, we advocate for the inclusion of persistence null models and the quantification of forecast ensemble uncertainty as means for identifying the effective forecast horizon in future forecasting workflows for CH4 ebullition to inform the performance and meaningful horizon of the models being tested.
Uncertainty Partitioning
Partitioning different sources of uncertainty (initial conditions, parameter, process, and driver uncertainty; Dietze, 2017a) in our CH4 ebullition rate forecasts allowed us to examine areas where our forecasting workflow with iterative model refitting could be improved (Figure 6). The large relative contribution of driver uncertainty in the 1- and 2-week forecasts (Figures 6A,B) during the 2019 forecasting period was likely due to uncertainty in the forecasted water temperatures from FLARE and the propagated uncertainty that arose in the SWI temperature scaling model from the downstream dam site to upstream transect site (Eqs 6, 7; Forecasting SWI Temperature). Thomas et al. (2020) found that meteorological driver data contributed the largest proportion of uncertainty in FLARE’s water temperature forecasts in FCR’s surface waters at horizons greater than 2 days into the future. Similarly, Dietze (2017a) found that meteorological driver data uncertainty contributed substantial uncertainty to net ecosystem exchange forecasts at forecast horizons greater than 1 week. Consequently, driver data uncertainty remains an important source of uncertainty that should be quantified in future forecasts of CH4 ebullition.
We also observed a larger contribution of model process uncertainty at the 1- and 2-week forecast horizons toward the end of the 2019 forecasting period, indicating that improvements to the model itself would improve CH4 ebullition rate predictions (Figure 6A). Model process uncertainty refers to the unexplained variability in the model itself, and encompasses multiple sources of variability in the model, including model structure, parameter heterogeneity, and stochastic variation (Dietze, 2017a). In the case of our forecast workflow for CH4 ebullition rates, the large contributions from model process uncertainty could have resulted from the model structure, interannual variability that occurred between 2017 and 2019, or other factors that were not accounted for in our state-space model. New forecasts of CH4 ebullition rates could be improved by specifically partitioning these potential sources of model process uncertainty using a hierarchical framework (Dietze, 2017a; Harris et al., 2018).
Forecasting System Limitations and Areas for Improvement
There are several limitations of our near-term, iterative CH4 ebullition rate forecasts that should be considered. First, there are many other potential drivers of CH4 ebullition, such as chlorophyll a, nutrients, and water pressure, among others (e.g., DelSontro et al., 2016; West et al., 2016; Harrison et al., 2017; Davidson et al., 2018). Alternative model structures (e.g., DelSontro et al., 2016; Schmid et al., 2017; Peltola et al., 2018; Grasset et al., 2021) could also improve our CH4 ebullition forecasts and improve their transferability to other freshwater ecosystems and different temporal scales. Because our forecasts relied on an AR model developed from earlier work in FCR (McClure et al., 2020a), we were unable to quantify model selection uncertainty by using an ensemble of models in our forecasting workflow (e.g., process-based ebullition models; Peltola et al., 2018; Schmid et al., 2017), which is an important step for future work. Testing different forecasting workflows with different CH4 ebullition model covariates and model types in inland water ecosystems at varying temporal and spatial scales will further improve our understanding of this important biogeochemical flux.
In addition to testing other CH4 ebullition rate models, additional improvements to our forecasting system would also include the addition of automated sensors to improve temporal coverage, incorporation of other statistical methods into the forecasting workflow (e.g., sequential data assimilation methods like particle filters and Kalman filters; Evensen, 2009; Carpenter et al., 1999; Del Moral et al., 2006; Doucet and Johansen, 2009), and other forecast model covariates which might provide more insight to mechanisms of CH4 ebullition. While our forecasting case study showed that near-term, iterative forecasts with iterative model refitting are possible with manually-collected CH4 ebullition rates, recent technological improvements in automated sensors hold great potential for advancing the future of CH4 ebullition forecasting at daily or sub-daily scales by increasing the timescale at which new data can be assimilated and at which forecasts can be made (e.g., Varadharajan et al., 2010; Delwiche and Hemond, 2017; Maher at al., 2019). Together, integrating automated CH4 ebullition rate sensors, an ensemble of predictive models, and alternate data assimilation methods have the potential to substantially advance forecasting of CH4 ebullition fluxes at sub-weekly timescales.
Scaling our CH4 Ebullition Forecasting Workflow to Other Sites
Our near-real time forecasts showed that out-of-sample CH4 ebullition rates at our littoral transect in FCR were adequately predicted at 1- and 2-week horizons using a state-space Bayesian workflow with iterative model refitting and forecasted SWI temperatures as a model covariate (Figure 3). While we acknowledge that we have only applied this forecasting workflow to one reservoir site, we envision that it has the potential to be adapted for predicting CH4 ebullition in other freshwater ecosystems. Here, we identify three critical components we think would enable others to begin making near-real time forecasts of CH4 ebullition rates from inland waters and inform ecological understanding of the processes governing ebullition rates:
1) A routine ebullition field monitoring program. The foundation of near-term, iterative forecasting is a coupled model-data feedback loop (Luo et al., 2011; Dietze, 2017b). Developing a field monitoring program that routinely samples ebullition from a waterbody of interest is necessary to develop and iteratively update ebullition forecast models. For example, this could include one automated ebullition trap with high temporal resolution or multiple passive traps that are limited in temporal resolution but are less expensive and allow for more spatial coverage. It is ideal if the monitoring data collection occurs within the maximum time horizon of the forecasted driver data to enable iterative forecast evaluation and model refitting.
2) A model to generate future predictions of ebullition that can be updated as observations become available. While we used a relatively simple state-space model fit in a Bayesian framework to account for the computational requirements to run forecasts with iterative refitting, there are many possible model types and data assimilation methods than can be applied like process-based models (Schmid et al., 2017; Peltola et al., 2018), and machine learning approaches such as neural networks (Abbasi et al., 2020) to develop CH4 ebullition forecasts. We suggest starting with something simple like a persistence null model (Eq. 9) and then adding in additional complexity when resources are available to do so.
3) Forecasted driver data. Depending on model structure, near-real time ebullition forecasts will likely rely on forecasted driver data to predict ebullition at a future time step. In lieu of having access to a water temperature forecasting system as we did for this study, meteorological forecasts (e.g., NOAA’s Global Ensemble Forecast System) can be integrated into an ebullition forecasting workflow if the CH4 ebullition model is driven by meteorological variables such as air temperature, barometric pressure, shortwave radiation, or wind speed (Joyce and Jewell 2003; Tokida et al., 2007; Wik et al., 2014; Peltola et al., 2018; McClure R. et al., 2020). For example, Tokida et al. (2007) showed how decreasing atmospheric pressure triggered substantial increases in CH4 ebullition from wetlands. Similarly, Wik et al. (2014) associated seasonal CH4 ebullitive fluxes from thermokarst lakes with shortwave radiation and Joyce and Jewell (2003) suggested that wind speed could influence bottom currents, thereby changing the shear stress at the sediments releasing ebullition bubbles.
There are several additional drivers of ebullition that have the capacity to be applied in a forecasting workflow in addition to those included in this study. However, in order to include a new driver variable in a forecasting workflow, one must produce a forecast of the driver variable, which can add additional uncertainty [e.g., our forecasting application here that translated weather forecasts into future SWI temperatures using FLARE and an empirical scaling model (Eq. 6)]. For example, changes in reservoir water level (Beaulieu et al., 2018), chemical and biological drivers such as chlorophyll a (West et al., 2016), sedimentation quantity (Wik et al., 2018), sediment quality (Wik et al., 2018), macrophyte abundance, and diel Chaoborus spp. migration (Bezerra et al., 2020), are known drivers of ebullition but rarely forecasted. This provides an opportunity for future model studies to begin generating forecasts of these drivers and testing how they perform with near-term forecasts of ebullition. Among the different predictors, chlorophyll a may be a useful one to start with as a forecast driver for CH4 ebullition as there are multiple existing workflows that have generated chlorophyll a forecasts for management (Page et al., 2018; Rousso et al., 2020). Integrating chlorophyll a forecasts into CH4 ebullition forecast workflows, similar to our example of integrating water temperature forecasts to our CH4 ebullition workflow, would likely improve forecasts of CH4 ebullition, and enable testing of how well these drivers perform at varying spatial and temporal scales.
Utility of Adopting Near-Term Iterative Forecasting Workflows
In conclusion, we successfully generated near-real time forecasts of CH4 ebullition rates, a highly variable biogeochemical flux in inland water ecosystems, using forecasted SWI temperatures and near-real time observations of ebullition rates. Further, our forecasts that iteratively updated parameters through model refitting performed better than forecasts that did not update parameters and a persistence null model at both 1- and 2-week forecast horizons, indicating that forecasting workflows with iterative model refitting have the potential to improve our estimates of this highly variable biogeochemical flux up to 2 weeks into the future. Additionally, quantifying and partitioning uncertainty provided an additional line of evidence that iterative forecasting can improve the skill of predictions with time. Uncertainty partitioning showed that our forecast model is highly sensitive to process uncertainty, highlighting the need for more resolved partitioning of variation within our predictive AR model and testing of different CH4 ebullition predictive models to improve predictions and transferability across multiple spatial and temporal scales. Altogether, we show that near-term iterative forecasting with model refitting and uncertainty partitioning has the potential to improve future CH4 ebullition rate predictions, an important step to informing our upscaling estimates of CH4 ebullition fluxes from inland waters to the global carbon cycle.
Data Availability Statement
The original contributions presented in the study are included in the article/supplementary materials, further inquiries can be directed to the corresponding author.
Author Contributions
RM and CC developed the original research idea. RM and RT developed the forecasting workflow. WW and ML contributed to code development, field data collection, and forecast model testing. RM wrote the manuscript with equal writing contributions from CC and RT. All authors provided feedback and approved the final version.
Funding
This work was supported by the U.S. National Science Foundation (CNS-1737424, DEB-1753639, DBI-1933016, DEB-1926050); the Virginia Tech Global Change Center; and the Fralin Life Sciences Institute.
Conflict of Interest
The authors declare that the research was conducted in the absence of any commercial or financial relationships that could be construed as a potential conflict of interest.
Publisher’s Note
All claims expressed in this article are solely those of the authors and do not necessarily represent those of their affiliated organizations, or those of the publisher, the editors and the reviewers. Any product that may be evaluated in this article, or claim that may be made by its manufacturer, is not guaranteed or endorsed by the publisher.
Acknowledgments
We thank the Smart Reservoir and FCR Carbon teams for their helpful feedback during this project and the Western Virginia Water Authority for their long-term support and access to the reservoir. We thank Ashley Mickens for her critical help with data collection and GC-FID analyses. Additionally, we thank Bethany Bookout, Alexandria Hounshell, Abby Lewis, Heather Wander, Rachel Corrigan, James Maze, Dexter Howard, Jacob Wynne, and Nick Hammond for their help with field data collection and Vahid Daneshmand and Renato Figueiredo for their development and maintenance of the FLARE forecast system. Paul Hanson, Erin Hotchkiss, Madeline Schreiber, and GLEON colleagues provided valuable feedback on manuscript development. Finally, as always, we thank Bobbie Niederlehner for helping with the GC-FID and laboratory analysis methods development.
References
Abbasi, T., Abbasi, T., Luithui, C., and Abbasi, S. A. (2020). A Model to Forecast Methane Emissions from Topical and Subtropical Reservoirs on the Basis of Artificial Neural Networks. Water 12 (1), 145. doi:10.3390/w12010145
Aben, R. C. H., Barros, N., Van Donk, E., Frenken, T., Hilt, S., Kazanjian, G., et al. (2017). Cross continental Increase in Methane Ebullition under Climate Change. Nat. Commun. 8 (1), 1–8. doi:10.1038/s41467-017-01535-y
Bastviken, D., Cole, J., Pace, M., and Tranvik, L. (2004). Methane Emissions from Lakes: Dependence of lake Characteristics, Two Regional Assessments, and a Global Estimate. Glob. Biogeochem. Cycles 18 (4). doi:10.1029/2004GB002238
Beaulieu, J. J., Balz, D. A., Birchfield, M. K., Harrison, J. A., Nietch, C. T., Platz, M. C., et al. (2018). Effects of an Experimental Water-Level Drawdown on Methane Emissions from a Eutrophic Reservoir. Ecosystems 21, 657–674. doi:10.1007/s10021-017-0176-2
Bezerra, M. P., McGinnis, D. F., Bezerra-Neto, J. F., and Barbosa, F. A. R. (2020). Is it Stochastic? Chaoborus Larvae Bioturbation Likely Affect the Timing of Daily Methane (CH4) Ebullitive Flux in a Tropical Reservoir. Hydrobiologia 847 (15), 3291–3308. doi:10.1007/s10750-020-04331-w
Burke, S. A., Wik, M., Lang, A., Contosta, A. R., Palace, M., Crill, P. M., et al. (2019). Long‐Term Measurements of Methane Ebullition from Thaw Ponds. J. Geophys. Res. Biogeosci. 124 (7), 2208–2221.
Carey, C. C., Hounshell, A. G., Lofton, M. E., Birgand, F., Bookout, B. J., Corrigan, R. S., et al. (2021a). Discharge Time Series for the Primary Inflow Tributary Entering Falling Creek Reservoir, Vinton, Virginia, USA 2013-2021 Ver 7. Environmental Data Initiative. doi:10.6073/pasta/8d22a432aac5560b0f45aa1b21ae4746
Carey, C. C., Lewis, A. S., McClure, R. P., Gerling, A. B., Chen, S., Das, A., et al. (2021b). Time Series of High-Frequency Profiles of Depth, Temperature, Dissolved Oxygen, Conductivity, Specific Conductivity, Chlorophyll a, Turbidity, pH, Oxidation-Reduction Potential, Photosynthetic Active Radiation, and Descent Rate for Beaverdam Reservoir, Carvins Cove Reservoir, Falling Creek Reservoir, Gatewood Reservoir, and Spring Hollow Reservoir in Southwestern Virginia, USA 2013-2020 Ver 11. Environmental Data Initiative. doi:10.6073/pasta/5448f9d415fd09e0090a46b9d4020ccc
Carey, C. C., Woelmer, W. M., Lewis, A. S., Breef-Pilz, A., Howard, D. W., and Bookout, B. J. (2021c). Time Series of High-Frequency Sensor Data Measuring Water Temperature, Dissolved Oxygen, Pressure, Conductivity, Specific Conductance, Total Dissolved Solids, Chlorophyll a, Phycocyanin, and Fluorescent Dissolved Organic Matter at Discrete Depths in Falling Creek Reservoir, Virginia, USA in 2018-2020 Ver 5. Environmental Data Initiative. doi:10.6073/pasta/88896f4a7208c9b7bddcf498258edf78
Carpenter, J., Clifford, P., and Fearnhead, P. (1999). Improved Particle Filter for Nonlinear Problems. IEE Proc. Radar Sonar Navig. 146 (1), 2–7. doi:10.1049/ip-rsn:19990255
Davidson, T. A., Audet, J., Jeppesen, E., Landkildehus, F., Lauridsen, T. L., Søndergaard, M., et al. (2018). Synergy between Nutrients and Warming Enhances Methane Ebullition from Experimental Lakes. Nat. Clim Change 8 (2), 156–160. doi:10.1038/s41558-017-0063-z
Deemer, B. R., Harrison, J. A., Li, S., Beaulieu, J. J., DelSontro, T., Barros, N., et al. (2016). Greenhouse Gas Emissions from Reservoir Water Surfaces: a New Global Synthesis. BioScience 66 (11), 949–964. doi:10.1093/biosci/biw117
Deemer, B. R., and Holgerson, M. A. (2021). Drivers of Methane Flux Differ between Lakes and Reservoirs, Complicating Global Upscaling Efforts. J. Geophys. Res. Biogeosci. 126 (4). doi:10.1029/2019JG005600
Del Moral, P., Doucet, A., and Jasra, A. (2006). Sequential Monte Carlo Samplers. J. R. Stat. Soc B. 68 (3), 411–436. doi:10.1111/j.1467-9868.2006.00553.x
Del Sontro, T., Boutet, L., St-Pierre, A., del Giorgio, P. A., and Prairie, Y. T. (2016). Methane Ebullition and Diffusion from Northern Ponds and Lakes Regulated by the Interaction between Temperature and System Productivity. Limnol. Oceanogr. 61, S62–S77. doi:10.1002/lno.10335
Delwiche, K., and Hemond, H. F. (2017). An Enhanced Bubble Size Sensor for Long‐term Ebullition Studies. Limnol. Oceanogr. Methods 15, 821–835. doi:10.1002/lom3.10201
Dietze, M. C. (2017a). Prediction in Ecology: a First-Principles Framework. Ecol. Appl. 27 (7), 2048–2060. doi:10.1002/eap.1589
Dietze, M. C. (2017b). Ecological Forecasting. Princeton, NJ: Princeton University Press, 288. ISBN 9781400885459.
Dietze, M. C., Fox, A., Beck-Johnson, L. M., Betancourt, J. L., Hooten, M. B., Jarnevich, C. S., et al. (2018). Iterative Near-Term Ecological Forecasting: Needs, Opportunities, and Challenges. Proc. Natl. Acad. Sci. USA 115 (7), 1424–1432. doi:10.1073/pnas.1710231115
Doucet, A., and Johansen, A. M. (2009). “A Tutorial on Particle Filtering and Smoothing: Fifteen Years Later,” in Handbook of Nonlinear Filtering (New York: Oxford Univ. Press). 12, 676–704.
Evensen, G. (2009). Data Assimilation: The Ensemble Kalman Filter. New York, NY: Springer Science & Business Media, 307. ISBN 978-3-642-03710-8.
Gelman, A., and Rubin, D. B. (1992). A Single Series from the Gibbs Sampler Provides a False Sense of Security. Bayesian Statis. 4, 625–631.
Gerling, A. B., Munger, Z. W., Doubek, J. P., Hamre, K. D., Gantzer, P. A., Little, J. C., et al. (2016). Whole-catchment Manipulations of Internal and External Loading Reveal the Sensitivity of a century-old Reservoir to Hypoxia. Ecosystems 19 (3), 555–571. doi:10.1007/s10021-015-9951-0
Grasset, C., Moras, S., Isidorova, A., Couture, R. M., Linkhorst, A., and Sobek, S. (2021). An Empirical Model to Predict Methane Production in Inland Water Sediment from Particular Organic Matter Supply and Reactivity. Limnol. Oceanogr. 66, 3643–3655. doi:10.1002/lno.11905
Harris, D. J., Taylor, S. D., and White, E. P. (2018). Forecasting Biodiversity in Breeding Birds Using Best Practices. Peer J 6, e4278.
Harrison, J. A., Deemer, B. R., Birchfield, M. K., and O’Malley, M. T. (2017). Reservoir Water-Level Drawdowns Accelerate and Amplify Methane Emission. Environ. Sci. Technol. 51, 1267–1277. doi:10.1021/acs.est.6b03185
Johnson, M. S., Matthews, E., Bastviken, D., Deemer, B., Du, J., and Genovese, V. (2021). Spatiotemporal Methane Emission from Global Reservoirs. J. Geophys. Res. Biogeosci. 126. doi:10.1029/2021JG006305
Joyce, J., and Jewell, P. W. (2003). Physical Controls on Methane Ebullition from Reservoirs and Lakes. Environ. Eng. Geosci. 9 (2), 167–178. doi:10.2113/9.2.167
Lewis, A. S., Woelmer, W. M., Wander, H. L., Howard, D. W., Smith, J. W., McClure, R. P., et al. (2021). Increased Adoption of Best Practices in Ecological Forecasting Enables Comparisons of Forecastability Across Systems. Ecological Applications. (In press). doi:10.1002/eap.2500
Linkhorst, A., Hiller, C., DelSontro, T., Azevedo, G. M., Barros, N., Mendonça, R., et al. (2020). Comparing Methane Ebullition Variability across Space and Time in a Brazilian Reservoir. Limnol. Oceanogr. 65 (7), 1623–1634. doi:10.1002/lno.11410
Luo, Y., Ogle, K., Tucker, C., Fei, S., Gao, C., LaDeau, S., et al. (2011). Ecological Forecasting and Data Assimilation in a Data-Rich Era. Ecol. Appl. 21 (5), 1429–1442. doi:10.1890/09-1275.1
Maher, D. T., Drexl, M., Tait, D. R., Johnston, S. G., and Jeffrey, L. C. (2019). iAMES: An Inexpensive, Automated Methane Ebullition Sensor. Environ. Sci. Technol. 53, 6420–6426. doi:10.1021/acs.est.9b01881
Männistö, E., Korrensalo, A., Alekseychik, P., Mammarella, I., Peltola, O., Vesala, T., et al. (2019). Multi-year Methane Ebullition Measurements from Water and Bare Peat Surfaces of a Patterned Boreal Bog. Biogeosciences 16 (11), 2409–2421. doi:10.5194/bg-16-2409-2019
McClure, R. P., Hamre, K. D., Niederlehner, B. R., Munger, Z. W., Chen, S., Lofton, M. E., et al. (2018). Metalimnetic Oxygen Minima Alter the Vertical Profiles of Carbon Dioxide and Methane in a Managed Freshwater Reservoir. STOTEN 636, 610–620.
McClure, R. P., Lofton, M. E., Chen, S., Krueger, K. M., Little, J. C., and Carey, C. C. (2020a). The Magnitude and Drivers of Methane Ebullition and Diffusion Vary on a Longitudinal Gradient in a Small Freshwater Reservoir. J. Geophys. Res. Biogeosci. 125 (3). doi:10.1029/2019JG005205
McClure, R. P., Lofton, M. E., Chen, S., Krueger, K. M., Little, J. C., and Carey, C. C. (2020b). Methane Ebullition and Diffusion Rates, Turbulence, Water Temperature, and Water Depth Data from Falling Creek Reservoir (Virginia, USA) in the Ice-free Period during 2016-2019 Ver 2. Environmental Data Initiative. doi:10.6073/pasta/c6b1a54e356f5ab093d86dcdb89177ba
McClure, R. P., Thomas, R. Q., Lofton, M. E., Woelmer, W. M., and Carey, C. C. (2021). First Version of Reproducible Forecasts for CH4 Ebullition Rates at an Upstream Transect in a Eutrophic Reservoir in VA, USA. Available at: https://zenodo.org/badge/latestdoi/346490639.
Moriasi, D. N., Arnold, J. G., Van Liew, M. W., Bingner, R. L., Harmel, R. D., and Veith, T. L. (2007). Model Evaluation Guidelines for Systematic Quantification of Accuracy in Watershed Simulations. Trans. ASABE 50 (3), 885–900. doi:10.13031/2013.23153
Munkhammar, J., Mattsson, L., and Rydén, J. (2017). Polynomial Probability Distribution Estimation Using the Method of Moments. PloS one 12 (4), e0174573. doi:10.1371/journal.pone.0174573
Nash, J. E., and Sutcliffe, J. V. (1970). River Flow Forecasting through Conceptual Models Part I - A Discussion of Principles. J. Hydrol. 10 (3), 282–290. doi:10.1016/0022-1694(70)90255-6
Page, T., Smith, P. J., Beven, K. J., Jones, I. D., Elliott, J. A., Maberly, S. C., et al. (2018). Adaptive Forecasting of Phytoplankton Communities. Water Res. 134, 74–85. doi:10.1016/j.watres.2018.01.046
Peltola, O., Raivonen, M., Li, X., and Vesala, T. (2018). Technical Note: Comparison of Methane Ebullition Modelling Approaches Used in Terrestrial Wetland Models. Biogeosciences 15 (3), 937–951. doi:10.5194/bg-15-937-2018
Petchey, O. L., Pontarp, M., Massie, T. M., Kéfi, S., Ozgul, A., Weilenmann, M., et al. (2015). The Ecological Forecast Horizon, and Examples of its Uses and Determinants. Ecol. Lett. 18 (7), 597–611. doi:10.1111/ele.12443
R Core Team (2021). R: A Language and Environment for Statistical Computing. Vienna, Austria: R Foundation for Statistical Computing. Available at: https://www.R-project.org/.
Rousso, B. Z., Bertone, E., Stewart, R., and Hamilton, D. P. (2020). A Systematic Literature Review of Forecasting and Predictive Models for Cyanobacteria Blooms in Freshwater Lakes. Water Res. 182, 115959. doi:10.1016/j.watres.2020.115959
Saunois, M., Stavert, A. R., Poulter, B., Bousquet, P., Canadell, J. G., Jackson, R. B., et al. (2020). The Global Methane Budget 2000-2017. Earth Syst. Sci. Data 12, 1561–1623. doi:10.5194/essd-12-1561-2020
Schmid, M., Ostrovsky, I., and McGinnis, D. F. (2017). Role of Gas Ebullition in the Methane Budget of a Deep Subtropical lake: What Can We Learn from Process-Based Modeling. Limnol. Oceanogr. 62 (6), 2674–2698. doi:10.1002/lno.10598
Thomas, R. Q., Figueiredo, R. J., Daneshmand, V., Bookout, B. J., Puckett, L. K., and Carey, C. C. (2020). A Near‐Term Iterative Forecasting System Successfully Predicts Reservoir Hydrodynamics and Partitions Uncertainty in Real Time. Water Resour. Res. 56 (11). doi:10.1029/2019WR026138
Thomas, R. Q., Jersild, A. L., Brooks, E. B., Thomas, V. A., and Wynne, R. H. (2018). A Mid-century Ecological Forecast with Partitioned Uncertainty Predicts Increases in Loblolly pine forest Productivity. Ecol. Appl. 28 (6), 1503–1519. doi:10.1002/eap.1761
Tokida, T., Miyazaki, T., Mizoguchi, M., Nagata, O., Takakai, F., Kagemoto, A., et al. (2007). Falling Atmospheric Pressure as a Trigger for Methane Ebullition from Peatland. Glob. Biogeochem. Cycles 21 (2). doi:10.1029/2006GB002790
Varadharajan, C., Hermosillo, R., and Hemond, H. F. (2010). A Low‐Cost Automated Trap to Measure Bubbling Gas Fluxes. Limnol. Oceanogr. Meth. 8 (7), 363–375.
West, W. E., Creamer, K. P., and Jones, S. E. (2016). Productivity and Depth Regulate lake Contributions to Atmospheric Methane. Limnol. Oceanogr. 61 (S1), S51–S61. doi:10.1002/lno.10247
Wik, M., Johnson, J. E., Crill, P. M., DeStasio, J. P., Erickson, L., Halloran, M. J., et al. (2018). Sediment Characteristics and Methane Ebullition in Three Subarctic Lakes. J. Geophys. Res. Biogeosci. 123 (8), 2399–2411. doi:10.1029/2017JG004298
Wik, M., Thornton, B. F., Bastviken, D., MacIntyre, S., Varner, R. K., and Crill, P. M. (2014). Energy Input Is Primary Controller of Methane Bubbling in Subarctic Lakes. Geophys. Res. Lett. 41 (2), 555–560. doi:10.1002/2013GL058510
Keywords: biogeochemical fluxes, ecological forecasting, freshwater, greenhouse gases, reservoir
Citation: McClure RP, Thomas RQ, Lofton ME, Woelmer WM and Carey CC (2021) Iterative Forecasting Improves Near-Term Predictions of Methane Ebullition Rates. Front. Environ. Sci. 9:756603. doi: 10.3389/fenvs.2021.756603
Received: 10 August 2021; Accepted: 25 October 2021;
Published: 01 December 2021.
Edited by:
Daniel F. McGinnis, Université de Genève, SwitzerlandReviewed by:
Martin Schmid, Swiss Federal Institute of Aquatic Science and Technology, SwitzerlandDominic Vachon, Umeå University, Sweden
Copyright © 2021 McClure, Thomas, Lofton, Woelmer and Carey. This is an open-access article distributed under the terms of the Creative Commons Attribution License (CC BY). The use, distribution or reproduction in other forums is permitted, provided the original author(s) and the copyright owner(s) are credited and that the original publication in this journal is cited, in accordance with accepted academic practice. No use, distribution or reproduction is permitted which does not comply with these terms.
*Correspondence: Ryan P. McClure, ryan.mcclure@wsu.edu