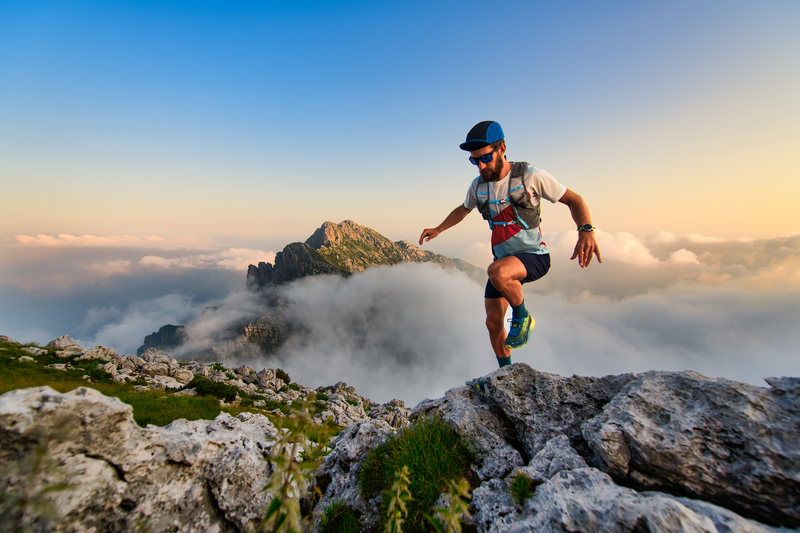
95% of researchers rate our articles as excellent or good
Learn more about the work of our research integrity team to safeguard the quality of each article we publish.
Find out more
PERSPECTIVE article
Front. Environ. Sci. , 12 October 2021
Sec. Environmental Informatics and Remote Sensing
Volume 9 - 2021 | https://doi.org/10.3389/fenvs.2021.733634
This article is part of the Research Topic Rise of Low-Cost Sensors and Citizen Science in Air Quality Studies View all 8 articles
Using low-cost air quality sensors (LCS) in citizen science projects opens many possibilities. LCS can provide an opportunity for the citizens to collect and contribute with their own air quality data. However, low data quality is often an issue when using LCS and with it a risk of unrealistic expectations of a higher degree of empowerment than what is possible. If the data quality and intended use of the data is not harmonized, conclusions may be drawn on the wrong basis and data can be rendered unusable. Ensuring high data quality is demanding in terms of labor and resources. The expertise, sensor performance assessment, post-processing, as well as the general workload required will depend strongly on the purpose and intended use of the air quality data. It is therefore a balancing act to ensure that the data quality is high enough for the specific purpose, while minimizing the validation effort. The aim of this perspective paper is to increase awareness of data quality issues and provide strategies to minimizing labor intensity and expenses while maintaining adequate QA/QC for robust applications of LCS in citizen science projects. We believe that air quality measurements performed by citizens can be better utilized with increased awareness about data quality and measurement requirements, in combination with improved metadata collection. Well-documented metadata can not only increase the value and usefulness for the actors collecting the data, but it also the foundation for assessment of potential integration of the data collected by citizens in a broader perspective.
The ongoing rapid development of smaller, cheaper sensors is radically expanding possibilities for air quality monitoring, and this new sensor technology has gained strong interest for air quality in many different fields of science as well as among the general public (Lewis et al., 2018; Lukeville, 2020; Peltier et al., 2021). LCS are often used, for example, to engage citizens, to increase spatial coverage of measurements, or to assess hyper-local pollution variability. Although this low-cost sensor (LCS) technology have great potential for new strategies in air quality control (Peltier et al., 2021), the use of LCS data has been proven difficult due to inadequate data quality, caused by, for example, technical accuracy limitations, biases, and failing of measurement procedures to meet standardized regulation (Ekman and Weilenmann, 2021).
Standardized air quality measurements are used as a tool to control legally binding limit values, and traditional monitoring is used to control that air pollution is reduced “to levels which minimize the harmful effects” (European Parliament, Council of the European Union, 2008). For European regulatory measurements, methods must strictly follow the quality assurance and quality control (QA/QC) described in the Air Quality directive (2008/50/EC). This QA/QC details selection of suitable methodology and instrumentation, representative measurement sites selection, how to correctly install and maintain instruments, and how to apply correct calibration techniques and data management. The directives also specify the metadata needed to document, for example, siting, maintenance and calibration procedures. This is to ensure high data quality and comparability of measurements (European Parliament, Council of the European Union, 2008). Due to this strict regulation, performing standardized air quality measurements are both expensive and labor intensive, which not only limits the number of measurement sites, but also the actors that can perform air quality measurements.
Recommendations for standardized evaluation of LCS:s is currently under development at a European level (CEN TC 264 WG 42), and many studies have assessed possibilities to use LCS for complementing and extending the regulatory air quality measurements (e.g. 1, 2, 3, 6–8). The general conclusion is that with extensive accuracy assessment and post-processing, the quality of data from LCS:s can be increased, but considerable accuracy limitations remain thus complicating the integration of LCS data with official data. As LCS facilitate citizens to collect their own air quality data there is an increasing interest in integrating this LCS data collected by citizens with regulatory monitoring (Lukeville, 2020). However, this reduces control of, for example, measurement methods, siting and sensor validation, and insufficient metadata is often an obstacle when assessing quality of data collected by citizens (Wesseling et al., 2019). An example where citizen collected LCS data is successfully integrated with regulatory monitoring and used in open communication is the Airnow fire and smoke map (AirNow (2021). Fire and s, 2021). In these maps, the regulatory monitoring sites are complemented with numerous LCS measurements that undergo a preliminary data quality assessment, in order to increase spatial data coverage.
Initiatives integrating LCS data with regulatory measurements generally include a considerable effort in time as well as expertise regarding for example measurement methodology and QA/QC. In initiatives where neither time nor expertise is available, measurements with LCS:s can still be very useful, for example in engaging and communicating with citizens and for simpler comparative studies etc. However, regardless of the context and purpose of the data collection, awareness of basic requirements to perform representative air quality measurements is of great importance, as it would increase reliability and thus potential applications for the sensor data. In this perspective paper we address the importance of identifying the level of data quality required for the measurement purpose, establishing a suitable QA/QC approach, and assessing the level of expertise needed.
The lower cost, smaller size and easy accessibility of LCS enables citizens to perform air quality measurements outside of scientific projects. One example is the crowdsourcing initiative sensor.community, a large citizen driven initiative originally motivated by an interest in exploring LCS to measure air quality where no standardized air quality stations were available. It has engaged citizens worldwide in air quality, with over 14,500 active sensors reporting data in 71 countries. A recent study shows that the use of LCS and an active participation in this community can mediate further engagement and informal learning (Ekman, 2021). However, no organized sensor performance is applied, and very little metadata apart from the sensor location is generally collected. Performance of the sensors used is questionable with considerable risk for bias (Liu et al., 2019), and a brief assessment of possibilities to use this data for comparative purposes show that lack of metadata and data coverage are great obstacles (Lindén et al., 2020). If data quality issues are solved, this and similar initiatives could provide a considerable data resource.
Air quality measurements are a complex challenge, regardless of instrumentation used. The need for an appropriate methodology and a suitable QA/QC approach applies to all types of air quality measurements. However, while extensive effort and funding are guaranteed for these aspects to ensure successful measurements before, for example, launching satellite measurements, the issue is rarely given the same priority when using LCS. This is, of course, connected to the considerably difference in both data quality demands and room for trial and error, but it should be kept in mind when assessing the data quality and possibilities for analysis. To ensure useful data, it is necessary to apply adequate methodology, maintenance and QA/QC strategies to reach quality requirements needed for intended use of the data (Peltier et al., 2021). Likewise, it is equally important that the analysis and conclusions are adapted to the obtained data quality.
Without proper data validation it is challenging to compare LCS data with air quality limit values and unfortunate examples exist. For example, in Flåm municipality in Norway, LCS were used to assess the effects of the Covid-19 pandemic on SO2 concentrations (Svarstad, 2020). An unexpected increase SO2 was measured, but it was eventually connected to inaccuracies in data, and the study provided little insight to true changes. Another example, in an otherwise well-constructed study, is the use of LCS for assessment of childrens’ exposure at preschools recently gained a lot of publicity in Sweden (Stroh et al., 2021). However, the conclusions drawn regarding pollution sources and temporal variations partially deviated from expected patterns, and insufficient metadata makes assessment of data quality difficult. Questionable use of LSC can also be found in the scientific papers. For example, Kończak et al. (2021), included LCS for air quality measurements in a study of particle deposition on vegetation. The LCS data was one order of magnitude lower than nearby reference station measurements and presented features impossible to obtain with correct measurements. This did not however influence the main conclusions of the study. Consultation and presentation of appropriate air quality and data analysis expertise in these studies could have greatly increased potential use of the LCS data and provided additional weight to the study.
The requirement of sensor assessment and data validation will depend on the intended use of the data. To obtain the highest possible data quality, the LCS performance assessment can be used to develop post-processing correction algorithms (Castell et al., 2017; Castell et al., 2017; Zimmerman et al., 2018; Lindén et al., 2020; Peltier et al., 2021). Sahu et al. (2020) show that post-processing algorithms are diversity sensitive, where e.g. algorithms developed based on summer conditions did not perform as well when applied on autumn data. To ensure representability, the co-location LCS performance assessment period needs to be of adequate length to capture variation, and likely needs to be repeated during different seasons (Castell et al., 2017; Spinelle et al., 2017). LCS evaluation through co-location with standardized reference measurements has shown that sensor performance specified by the manufacturer often does not apply in field conditions, and site and sensor specific sensor performance assessment and correction is generally needed for relevant data analysis (e.g. 3, 6–8). Biases such as systematic offset, influence by meteorological parameters, cross sensitivity to other pollutants and drift over time are commonly occurring in LCS:s (e.g. 3, 6–8). These studies also show that although sensors of the same type often perform similarly, this cannot be guaranteed, and it is therefore recommended that a rudimentary sensor-to-sensor comparison always is performed in the intended environment. This is confirmed in an extensive review by Karagulian et al. (2019) who show that sensor evaluation may produce very different results in different studies and conclude that using previous evaluations of sensor performance assessment may result in misleading conclusions. Consequently, inadequate sensor performance assessment can lead to unusable data or incorrect analysis and conclusions.
While controlling data quality is crucial for a robust data analysis, aiming for highest possible data quality is labor intensive and demands expertise in both air quality measurements and data analysis (Peltier et al., 2021). For many projects, especially in citizen science or crowdsourcing, the demands on data quality may be considerably lower. This can potentially save both time and need for expertise. However, it is a balancing act to ensure that the level of data quality is high enough to allow a robust and relevant data analysis for the intended aim, but that an unnecessary high workload due to overworking the quality control is avoided. This balancing act can be illustrated with the QA/QC approach applied in a case study from the NordicPATH project, aimed to raise awareness and collective responsibility regarding emissions from domestic wood-burning. LCS data collected by citizens will be used in the dialogue between the citizens and municipality. Like many other LCS projects, the budget limits not only the choice and number of sensors, but also the possibilities post-processing data and validation of data. The project purchased 11 PM2.5 sensors with a specified mass concentration precision of ±10 μg/m3 in the range 0−100 μg/m3. The precision limits the possible use of the data, and the data will therefore only be used for identifying trends. A basic sensor performance assessment was made through co-located measurements for a period of a month, with representable PM concentrations ranging between 0 and 30 μg/m3 (Figure 1A). As shown in Figure 1B, the sensor-to-sensor correlation and precision of the devices is very good. Comparison with a nearby regulatory monitoring station show that although overall variation was similar, the sensor performance was not sufficient for representation of absolute values, especially in higher concentrations (Figure 1C). However, as the main aim in the project is awareness-raising and communication, as well as comparative concentrations, it was deemed sufficient accuracy for this purpose.
FIGURE 1. (A) Time series showing data from all 11 evaluated LCS (black) and nearby reference station (red), (B) inter-comparison of co-located PM2.5 measurements from three representative sensors, and (C) comparison of PM2.5 measured with the same three representative sensors, to the nearest urban background station. Reference station data was downloaded from https://luftkvalitet.nilu.no/historikk.
All long-term air quality measurements need to be subject to regular control and maintenance to ensure that a high data quality is maintained (European Parliament, Council of the European Union, 2008). Studies have shown that LCS are highly prone to technical problems requiring maintenance and repair of faulty sensors, as well as change in sensor signal with time, so called drift. This makes recurring sensor validation and maintenance crucial for maintaining a high data quality (Peltier et al., 2021). Thus, maintaining high data quality in large, long-term LCS networks risks becoming very costly and time consuming. Application of advanced machine learning and AI techniques can be useful for both post-processing algorithms as well as detection of drift (Zimmerman et al., 2018; Topalović et al., 2019). However, not all actors that can benefit for working with LCS will have the expertise nor funds to apply these advanced techniques. In citizen science project, this can be solved by limiting the measurement period, with recurring co-location measurement to minimize the risk for sensor drift (Zimmerman et al., 2018; Topalović et al., 2019).
A simpler QA/QC can be appropriate for projects where LCS are used to raise awareness and as a tool in a dialog. This can be done without access to co-location measurement at reference station, without applying any post-processing to the data. Importantly, however, this will require a clear communication from the municipality on the expectations citizens can have on the data that is collected. This highlights an important point: issues of power and empowerment are important to consider when LCS are used by municipalities in citizens science projects (Ekman and Weilenmann, 2021). It can be challenging for municipality when citizens expect a higher degree of empowerment by the collected data than what is the actual case (Ekman and Weilenmann, 2021), and often will data collected by citizens to document pollution be disregarded due to suspected bias (Garby, 2020). This issue can be avoided if the expectations on the data is harmonized with the possible use of the data. Regardless of QA/QC approach documentation of metadata is key when assessing the quality of data produced by all types measurements (European Parliament, Council of the European Union, 2008). A well-documented metadata can not only increase the value and usefulness for the actors collecting the data, but it can also open possibilities for others to use the collected data. While the documentation of metadata is strictly regulated in the air quality directive for standardized measurements, it is equally important when LCS are used (e.g.3, 9). In citizen science initiatives, there may be major deviations from the regulations described in the directives, but as long as these deviations are known, the data will likely still prove very useful. We therefore suggest that metadata is documented based on instructions in the directive (European Parliament, Council of the European Union, 2008), with all deviations from protocol noted. This will allow for a more robust assessment of data quality, increase reliability of the analysis and will greatly increase the possibilities for using the data in a broader perspective.
Based on our collected experiences of using LCS for a variety of applications with citizens, we recommend that in the initial planning of the project define a clear aim with the data collection, establishing a suitable QA/QC approach and identifying the level of expertise needed. We believe that if the required data quality is well established and a suitable QA/QC approach is applied to the measurements easier to utilize the possibilities LCS data, both for internal use in a citizen science project, but also for LCS data as a resource in big data for other actors. In projects involving multiple parts, determining responsibility early in the project for issues like data quality, maintenance, measurement methodology and metadata is crucial to ensure that the measurement aim is reached. For example, who ensures that the sensors are evaluated and maintained meet the expected performance throughout the project? Who selects a suitable measurement methodology and ensures that the measurements are performed accordingly? Who determines metadata needs and ensures that this data is collected? And finally, who ensures that the analysis and conclusions are relevant given the obtained data quality?
To utilize the potential of air quality data collected by citizens, both to complement and challenge the traditional way of air quality monitoring, the issue of data quality needs to be tackled. In Figure 2, the requirements QA/QC approaches for different applications of LCS data is illustrated. The QA/QC needs and required level of expertise will depend on the level of data quality, it is therefore a key to define a clear goal with the data collection. To ensure a robust data analysis that meets the project aim, it is important to clearly define the purpose of the data collection and level of data quality needed in an initial stage of the project. Once the need for data quality is established, a suitable QA/QC approach can be identified to ensure this is reached. As detailed in the recent WMO-report, a hierarchy of validation is presented (Peltier et al., 2021). Finally, it is crucial to assess the level of expertise needed to ensure adequate data quality from the intended measurements. A good to expert understanding of processes influencing air quality, including sensor siting and measurement methodology, is needed to determine if the measurements meet the required quality. Likewise, a good to expert understanding of statistical data analysis and sensor correction, potentially including machine learning or corresponding techniques, is needed to define a suitable QA/QC approach for the project aim. If the level of expertise to determine suitable measurement and QA/QC approaches is not available within the project, external expert advice likely needs to be sought to assess the suitability of the intended approaches. The aim of the expert input is not to complicate or disqualify the use of LCS, but rather to increase awareness of how to ensure that measurements are robust and that the analyses drawn are based on data of adequate quality. If these steps are taken, in citizen science projects, the usefulness the data will increase, since organizers will have more realistic expectations to the data. The organizers will have a responsibility to communicate the possibilities and limitation to the participants, so that the participants gain a realistic degree of empowerment.
FIGURE 2. Schematic of the QA/QC requirements, expertise requirements and required methods for application of air quality measurement data. The typical level of sensor validation and data applications are in-line with the suggestion from Peltier et al. (2021).
We believe tackling data quality in citizens science project with an adequate QA/QC approach, can open doors for how LCS sensor data can be used as a tool not only for productive dialogues but also possible data resource for others.
The original contributions presented in the study are included in the article/supplementary materials, further inquiries can be directed to the corresponding author.
All authors contributed in the discussions for conceptualizing the work presented in this paper. ÅW and JL: Wrote the original draft. HF, JW, MG and NC: reviewed, edited and discussed the manuscript.
The work presented are with contribution from the LoV-IoT project, an IoT Sverige project financed jointly by Vinnova, FORMAS and Swedish Energy Agency; the SMURBS/ERA-NET (ERA-NET-Cofund, grant nr: 689443) and and NordForsk through funding to Nordic Participatory, healthy, and people centered cities, project number 95326.
The authors declare that the research was conducted in the absence of any commercial or financial relationships that could be construed as a potential conflict of interest.
All claims expressed in this article are solely those of the authors and do not necessarily represent those of their affiliated organizations, or those of the publisher, the editors and the reviewers. Any product that may be evaluated in this article, or claim that may be made by its manufacturer, is not guaranteed or endorsed by the publisher.
ÅW want to thank K. Ekman, B. Hagström and P. Ljungstrand are for all the interesting and challenging discussions about citizen science, data and power.
AirNow (2021). Fire and Smoke Map. Available at https://fire.airnow.gov/2021
Castell, N., Dauge, F. R., Schneider, P., Vogt, M., Lerner, U., Fishbain, B., et al. (2017). Can Commercial Low-Cost Sensor Platforms Contribute to Air Quality Monitoring and Exposure Estimates? Environ. Int. 99, 293–302. doi:10.1016/j.envint.2016.12.007
Ekman, K. (2021). Making Sense of Sensing: Scaffolding Community Knowledge in an Online Informal Scientific Engagement. Learn. Cult. Soc. Interaction 30, 100537. doi:10.1016/j.lcsi.2021.100537
Ekman, K., and Weilenmann, A. (2021). Behind the Scenes of Planning for Public Participation: Planning for Air-Quality Monitoring with Low-Cost Sensors. J. Environ. Plann. Manage. 64 (5), 865–882. doi:10.1080/09640568.2020.1787129
European Parliament, Council of the European Union (2008). Directive 2008/50/EC of the European Parliament and of the Council of 21 May 2008 on Ambient Air Quality and Cleaner Air for Europe. OJ. L152/1 Available at: https://eur-lex.europa.eu/legal-content/EN/TXT/PDF/ (Accessed June 01, 2021).
Garby, J. (2020). Planetary Health in Practice: Sensing Air Pollution and Transforming Urban Environments. Humanities Soc. Sci. Commun. 7 (35), 1–11. doi:10.1057/s41599-020-00534-7
Karagulian, F., Barbiere, M., Kotsev, A., Spinelle, L., Gerboles, M., Lagler, F., et al. (2019). Review of the Performance of Low-Cost Sensors for Air Quality Monitoring. Atmosphere 10 (9), 506. doi:10.3390/atmos10090506
Kończak, B., Cempa, M., Pierzchała, Ł., and Deska, M. (2021). Assessment of the Ability of Roadside Vegetation to Remove Particulate Matter from the Urban Air. Environ. Pollut. 268, 115465. doi:10.1016/j.envpol.2020.115465
Lewis, A., Peltier, W. R., and von Schneidemesser, E. (2018). Low-cost Sensors for the Measurement of Atmospheric Composition: Overview of Topic and Future Applications. Geneva: WMO.
Lindén, J., Watne, Å., Giebat, M., Segura-Roux, M., Ooyola, P., Vanemark, J., et al. LoV-IoT Air and Water Monitoring with Internet of Things - Air Quality Observational Platforms. Available at www.loviot.se.2020.
Liu, H.-Y., Schneider, P., Haugen, R., and Vogt, M. (2019). Performance Assessment of a Low-Cost PM2.5 Sensor for a Near Four-Month Period in Oslo, Norway. Atmosphere 10 (2), 41. doi:10.3390/atmos10020041
Lukeville, A. (2020). Assessing Air Quality through Citizen Science. Copenhagen: European Environment Agency.
N. Castell, F. R. Dauge, R. Dongol, M. Vogt, and P. Schneider (Editors) (2017). Uncertainty in Air Quality Observations Using Low-Cost Sensors (EGU General Assembly Conference Abstracts).
Peltier, R. E., Castell, N., Clements, A. L., Dye, T., Hüglin, C., Kroll, J. H., et al. (2021). An Update on Low-Cost Sensors for the Measurement of Atmospheric Composition.
Sahu, R., Dixit, K. K., Mishra, S., Kumar, P., Shukla, A. K., Sutaria, R., et al. (2020). Validation of Low-Cost Sensors in Measuring Real-Time PM10 Concentrations at Two Sites in Delhi National Capital Region. Sensors 20 (5), 1347. doi:10.3390/s20051347
Spinelle, L., Gerboles, M., Villani, M. G., Aleixandre, M., and Bonavitacola, F. (2017). Field Calibration of a Cluster of Low-Cost Commercially Available Sensors for Air Quality Monitoring. Part B: NO, CO and CO2. Sensors Actuators B: Chem. 238, 706–715. doi:10.1016/j.snb.2016.07.036
Stroh, E., Isaxson, C., Oudin, A., and Malmqvist, E. (2021). Luftföroreningshalter På Förskolegårdar.
Svarstad, S. (2020). Trur Ikkje På at Forureininga I Flåm Gjekk opp Då Cruiseskipa Forsvann. NRKno 09, 12.
Topalović, D. B., Davidović, M. D., Jovanović, M., Bartonova, A., Ristovski, Z., and Jovašević-Stojanović, M. (2019). In Search of an Optimal In-Field Calibration Method of Low-Cost Gas Sensors for Ambient Air Pollutants: Comparison of Linear, Multilinear and Artificial Neural Network Approaches. Atmos. Environ. 213, 640–658. doi:10.1016/j.atmosenv.2019.06.028
Wesseling, J., Ruiter, H., Blokhuis, C., Drukker, D., Weijers, E., Volten, H., et al. (2019). Development and Implementation of a Platform for Public Information on Air Quality, Sensor Measurements, and Citizen Science. Atmosphere 10 (8), 445. doi:10.3390/atmos10080445
Keywords: QA/QC - quality assurance/quality control, co-location, data post-processing, sensor performance assessment, measurement requirements, crowd source
Citation: Watne ÅK, Linden J, Willhelmsson J, Fridén H, Gustafsson M and Castell N (2021) Tackling Data Quality When Using Low-Cost Air Quality Sensors in Citizen Science Projects. Front. Environ. Sci. 9:733634. doi: 10.3389/fenvs.2021.733634
Received: 30 June 2021; Accepted: 28 September 2021;
Published: 12 October 2021.
Edited by:
Jennifer Ann Salmond, The University of Auckland, New ZealandReviewed by:
Lena Weissert, Aeroqual Ltd., New ZealandCopyright © 2021 Watne, Linden, Willhelmsson, Fridén, Gustafsson and Castell. This is an open-access article distributed under the terms of the Creative Commons Attribution License (CC BY). The use, distribution or reproduction in other forums is permitted, provided the original author(s) and the copyright owner(s) are credited and that the original publication in this journal is cited, in accordance with accepted academic practice. No use, distribution or reproduction is permitted which does not comply with these terms.
*Correspondence: Ågot K. Watne, YWdvdC53YXRuZUBpdmwuc2U=
†These authors have contributed equally to this work and share first authorship
Disclaimer: All claims expressed in this article are solely those of the authors and do not necessarily represent those of their affiliated organizations, or those of the publisher, the editors and the reviewers. Any product that may be evaluated in this article or claim that may be made by its manufacturer is not guaranteed or endorsed by the publisher.
Research integrity at Frontiers
Learn more about the work of our research integrity team to safeguard the quality of each article we publish.