- 1State Key Laboratory of Water Resources and Hydropower Engineering Science, Wuhan University, Wuhan, China
- 2Department of Safety and Disaster Prevention Research, Seoul Institute of Technology, Seoul, South Korea
- 3Bureau of Hydrology, Changjiang Water Resource Commission, Wuhan, China
- 4Hanjiang Bureau of Hydrology and Water Resources Survey, Bureau of Hydrology, Changjiang Water Resources Commission, Xiangyang, China
The present study predicts and assesses the individual, combined, and synergistic effect of land-use change and climate change on streamflow, sediment, and total phosphorus (TP) loads under the present and future scenarios by using the Soil and Water Assessment Tool (SWAT). To predict the impacts of climate and land-use change on streamflow, sediment, and TP loads, there are 46 scenarios composed of historical climate, baseline period climate, eight climate models of Coupled Model Intercomparison Project phase 5 (CMIP5) of two representative emission pathways (RCP4.5 and RCP8.5), after downscaled and bias-corrected, two observed land-use maps (LULC 1995, LULC 2015) and the projected two future land-use maps (LU2055 and LU 2075) with the help of CA-Markov model to be fed into SWAT. The central tendency of streamflow, sediment, and TP loads under future scenarios is represented using the annual average. The intra-/inter-annual variation of streamflow, sediment, and TP loads simulated by SWAT is also analyzed using the coefficient of variation. The results show that future land-use change has a negligible impact on annual streamflow, sediment, TP loads, and intra-annual and inter-annual variation. Climate change is likely to amplify the annual streamflow and sediment and reduce the annual TP loads, which is also expected to reduce its inter-/intra-annual variation of TP loads compared with the baseline period (2000–2019). The combined impact of land-use and climate change on streamflow, sediment, and TP loads is greater than the sum of individual impacts for climate change and land-use change, especially for TP loads. Moreover, the synergistic impact caused by the interaction of climate and land use varies with variables and is more significant for TP loads. Thus, it is necessary to consider the combined climate and land-use change scenarios in future climate change studies due to the non-negligible synergistic impact, especially for TP loads. This research rare integrates the individual/combined/synergistic impact of land-use and climate change on streamflow, sediment, and TP loads and will help to understand the interaction between climate and land-use and take effective climate change mitigation policy and land-use management policy to mitigate the non-point source pollution in the future.
Introduction
Climate and land-use change critically impact water resources by directly affecting the hydrologic processes (Ervinia et al., 2019; McDonough et al., 2020). Rainfall and temperature can influence the streamflow directly (Dosdogru et al., 2020) and the physical process of nutrients, such as denitrification, mineralization, and absorption of plants (Hartmann et al., 2014; Gao et al., 2017; Ervinia et al., 2020). Land use can alter hydrological cycles, non-point source (NPS) pollution induced by fertilizer applications, and the retention capability of sediment and nutrient loading (Ye et al., 2020), resulting in water pollution (Berka et al., 2001), and urbanization will amplify the flow peak, decrease the low flow and variability of streamflow through decreasing the infiltration and increasing the surface runoff. Previous studies have shown that climate and land-use change exert considerable impact on the water quantity and quality (El-Khoury et al., 2015; Lintern et al., 2018). Furthermore, both climate and land use can modulate the nutrient turnover and greenhouse gas emissions due to the interplay of climate and land use (Peters et al., 2019). Thus, it is necessary to simultaneously consider climate and land use in future studies of variation in hydrology and non-point source pollution.
With the increasing population and the decreasing available freshwater, the water quality should be improved based on the fully identifying water pollution source. In recent years, non-point source pollution has become one of the major pollution sources as the control of point source pollution, discussed in the studies of climate and land-use changes (Teshager et al., 2016). Agricultural non-point source is the main water pollution source of nutrient load due to the intensive agricultural activities and the increased fertilizer application in agricultural zones (Zhou et al., 2020; Zhuang et al., 2020). In the future, the nutrient load will exacerbate significantly due to the change of land use, rainfall, and rising temperatures. A variety of studies (Teshager et al., 2016; Wagner et al., 2016; Zuo et al., 2016; Shrestha et al., 2018; Mukundan et al., 2020; Ridwansyah et al., 2020) have investigated the effect of climate or land-use change on hydrology, sediment, and non-point sources. Some findings have suggested that non-point source (NPS) pollution is more sensitive to land-use change (El-Khoury et al., 2015; Gao et al., 2017; Liang et al., 2020); land-use change, a dominant variable, has a considerable impact on the distribution of nutrient loads (Tu, 2009; Tang et al., 2011; Gao et al., 2017). Instead, some studies stated that temperature and rainfall are the major factors for hydrology (Kim et al., 2013; Liang et al., 2020).
Recently, there also has been an emerging trend about the studies of combined climate and land-use change to assess their individual and combined impacts on hydrology and nutrient loading, including Asia (Wu et al., 2012; Luo et al., 2020), America (Tu, 2009; Chen et al., 2017), and Africa (Chanapathi and Thatikonda, 2020). Even though some studies have suggested that land-use change plays a minor role in climate change studies, it still needs to be taken into account (Kim et al., 2013; Shrestha et al., 2018) due to the strong interplay of climate and land use which is likely to exacerbate the situation. Land-use and climate have strong interaction and exert a considerable “synergistic” effect when considering both simultaneously (Dosdogru et al., 2020). Namely, the combined impact of land-use and climate is not equal to the sum of individual effects induced by climate or land-use change (Luo et al., 2020). Therefore, it is likely to exacerbate the water resources problem if the land-use change scenario is considered in future climate change researches (Trang et al., 2017; Dosdogru et al., 2020). However, some studies suggested that the combined effect is the linear addition of individual effects (Wang et al., 2013; Shrestha et al., 2018). Therefore, a deeper understanding of the combined effects of climate and land-use change is crucial. In addition, the studies on the synergistic effects of climate change and land-use change on runoff, sediment, and nutrient load are also limited.
There are various methods to study the response of streamflow, sediment, and non-point sources pollution on climate and land-use change, such as statistical technique (Li et al., 2020; Li et al., 2020b), non-point source pollution (GBNP) model (Tang et al., 2011), and hydrological model (SLURP hydrological model, a coupled network-scale hydrological and biogeochemical model, SWAT) (Wu et al., 2012). Among these, coupling the climate model and the soil and water assessment tool (SWAT) is widely applied to investigate the hydrological, sediment, and nonpoint source pollution resulting from climate change and land-use change (CARD: SWAT literature database for peer-reviewed journal articles, 2021).
In the studies of the combined effects of climate and land-use change, very few studies simultaneously consider the combined effects on runoff, sediment, and nutrient loads. Instead, most studies consider the effects either on hydrology (Chanapathi and Thatikonda, 2020; Dosdogru et al., 2020), or on hydrology and sediment (Khoi and Suetsugi, 2014; Zuo et al., 2016; Ndulue and Mbajiorgu, 2018), or on hydrology and nutrient load (Chen et al., 2017; Molina-Navarro et al., 2018; Shrestha et al., 2018) only. However, both runoff and sediment decide the transport process of nutrient loading (Tang et al., 2018). So it is necessary to simultaneously consider runoff, sediment, and nutrient load in the studies of non-point source pollution under the climate and land-use change scenarios. In addition, this study also analyzes the synergistic effect of climate change and land-use change on streamflow, sediment, and total phosphorus (TP) loads simultaneously.
Therefore, identifying the individual, combined, and synergistic effects of climate and land-use change on hydrology, sediment, and diffusion source pollution is essential to predict and assess the result of global change on water resources in the future. The goal of this research is to study the individual, combined, and synergistic impact of climate change and land-use change on the streamflow, sediment, and total phosphorus (TP) loads one of the significant non-point sources pollutant based on the calibrated SWAT model and statistical analysis in the middle part of the Hanjiang River basin, China. The specified content of this study includes: 1) calibrate and validate the SWAT model; 2) assess the impact of past and future climate and land-use change on streamflow, sediment, and TP loads; 3) explore the intra-annual variation and inter-annual variation of streamflow, sediment, and TP loads under the individual and combined impacts of climate and land-use change; 4) examine the synergistic effect of climate and land-use change on streamflow, sediment, and TP loads.
Materials and Methods
Study Area and Data
The Baihe River watershed covering an area of 1.22 × 104 km2 is located in the middle of the Hanjiang River basin, China (Figure 1). The river originates from Baihe Town in Henan Province and intersects with the Tang river at Xiangyang in Hubei Province. The land use of the basin is formed of agriculture (57.3%), forest (25.4%), pasture (4.5%), developed (9.0%), water (2.5%). The basin is mainly cultivated by agricultural area, and a typical crop rotation is summer corn from June to September and winter wheat from October to June in the next year. Its average annual precipitation and temperature (1961–2019) are approximately 801 mm and 16°C, respectively. The model is based on the digital elevation model (DEM), soil map, land-use map, meteorological data, and agricultural management data. Digital elevation data are obtained from Geospatial data clouds (http://www.gscloud.cn/sources/), presenting approximately 30 m spatial resolution. Soil type information (Harmonized World Soil Database version 1.1) with 1000 m spatial resolution is from the Food and Agriculture Organization of the United Nations (FAO). Land-use data sets (LULC 1995, LULC 2005, LULC 2015) with 1000 m spatial resolution are extracted from Resources and Environment Science Data Center, Chinese Academy of Sciences (https://www.resdc.cn/Default.aspx). Land-use is regrouped into seven categories, namely, agricultural area (AGRL), forest (FRST), pasture (PAST), industrial land (UIDU), high-density residential (URHD), low-density residential (URLD), and water body. Agricultural management information, including crop planting, fertilization, and irrigation, is obtained from the field survey and the Bureau of Agriculture and Rural Affairs (http://nyj.nanyang.gov.cn/tzgg/311745.htm). The point sources pollution of domestic discharge for each county is calculated based on the local population from Nanyang statistical information networks (http://www.nytjj.gov.cn/index.php?a=show&c=index&catid=6&id=1775&m=content) and the pollution yield per capital from Manual of Pollution Discharge Coefficient of Domestic Sources (The Second National Survey of Pollution Sources).
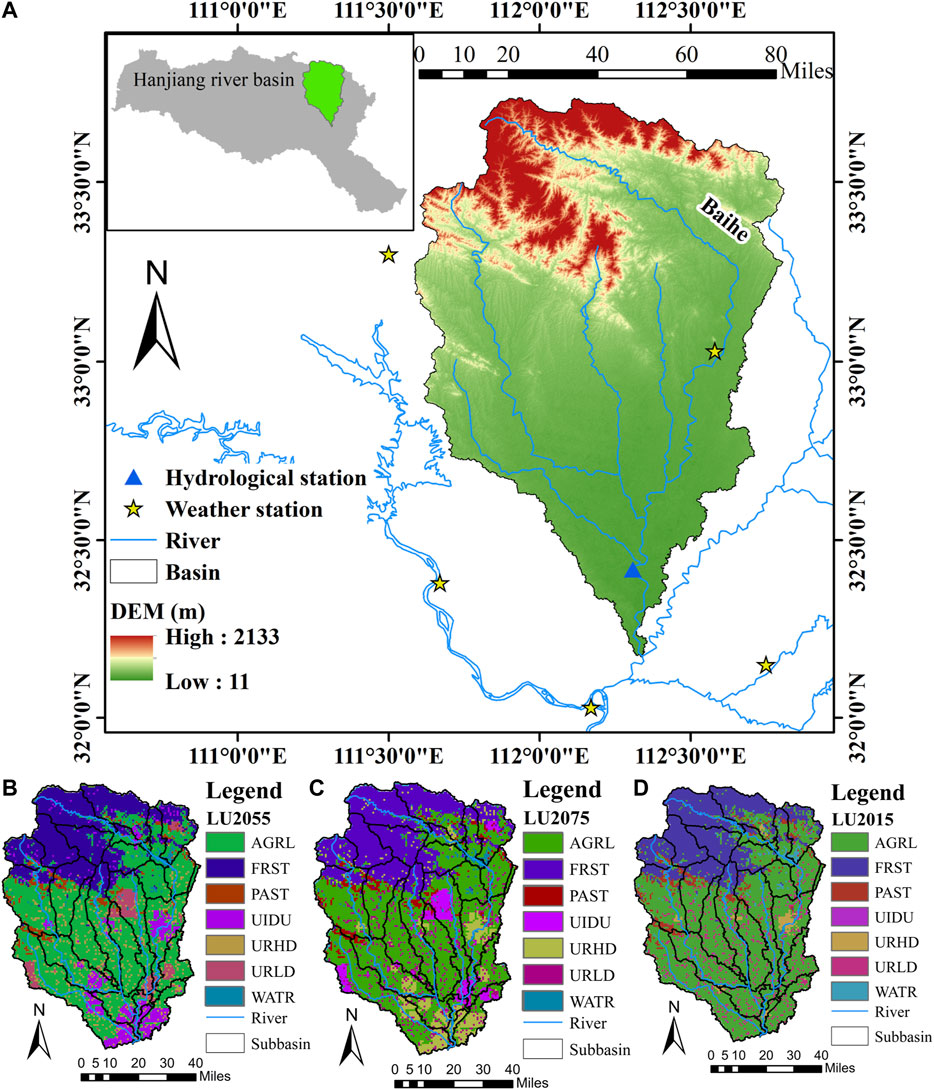
FIGURE 1. Study area along with hydrologic station and climate stations and the land-use map for LU 2015, LU 2055, and LU 2075.
The SWAT model needs meteorological data including daily rainfall, maximum/minimum temperature, wind speed, relative humidity, and solar radiation to drive running. There are four meteorological stations in the study area, and the required historical meteorological data can be downloaded from the China Meteorological Data Service Center. In addition, we can obtain another daily rainfall data in the hydrological station from the Hanjiang Bureau of Hydrology and Water Resources Survey, and the Inverse Distance Weight (IDW) is used to interpolate the corresponding data of four meteorological stations to obtain the other meteorological data except for daily rainfall. Therefore, a total of eight GCMs from Coupled Model Intercomparison Project phase 5 (CMIP5) are employed to predict future daily rainfall and maximum/minimum temperature in 2041–2080. The eight GCMs (Table 1) outputs of rainfall and temperature in five meteorological stations under RCP4.5 and RCP8.5 (including one hydrological station) in Baihe River watershed are extracted, and the error correction is carried out using Daily Bias Correction (DBC) method.
The monthly streamflow, sediment loads, and TP (Total Phosphorus) loads in the hydrological station are obtained from the Hanjiang Bureau of Hydrology and Water Resources Survey to calibrate and validate the constructed SWAT model. The data sets used in this study are detailed in Table 2.
SWAT
The SWAT developed by the United States Department of Agriculture (USDA) (Arnold et al., 1998) is used to simulate water quantity and quality based on climate and land-use change. It divides a watershed into numerous sub-basins and HRU subsequently defined by unique land use, soil types, and slope classes. The SCS curve number method is employed to compute surface runoff, and the variable storage coefficient method is used to calculate channel routing. The Penman/Monteith equation is used to compute the potential ET. Phosphorus in the soil is mainly added by applying fertilizers, manure, or residues, and is lost from the soil through plant absorption and leaching. Six different pools of phosphorus in the soil are monitored by SWAT. Three pools are inorganic forms of phosphorus, while the other three are organic. A detailed description of the phosphorus cycle can be found in the SWAT theoretical documentation (https://swat.tamu.edu/docs/). The SWAT model is running on ArcGIS used for exchanging data. In the study, the SWAT model divides the study region into 32 sub-basins with 162 hydrological response units (HRUs), and thresholds of soil, land use, and slope are 5, 20, and 20%, respectively.
Daily Bias Correction (DBC)
In this study, the bias correction for GCMs adopts the daily bias correction (DBC) (Chen et al., 2013), which is a hybrid method combining the local intensity scaling method (LOCI) and the daily conversion method (DT). First, the LOCI method is used to correct the precipitation occurrence, assuring that the frequency of precipitation occurrence of corrected data for the reference period is the same as observed data in a month. Then the quantile-based DT method is used to correct the empirical distribution of daily precipitation quantity and daily temperature based on the observed and GCM-simulated during the reference period. Moreover, the relationship acquired applies to the future climate. Finally, all GCMs used in the study are bias-corrected to obtain the suitable future climate data (2041–2080). Before the bias correction, all GCMs with different resolutions (Table 1) are interpolated into corresponding meteorological stations using Inverse Distance Weight (IDW). Especially, the adjusted daily precipitation (P) and temperature (T) for the future period are obtained using Eqs 1, 2, respectively.
where
CA-Markov
CA-Markov of IDRISI integrated the Markov chain and cellular automata (CA), using multi-criteria evaluation to define the transfer rules between land-use types. Markov chain is a stochastic method only related to the state at a previous time that simulates the transition probability between land-use types in two periods, while CA can predict spatial distribution, and the calculation efficiency is very high. The principle of the CA-Markov model is based on the land-use transformation area of the reference period and a previous time and the suitable land-use type of the pixel represented by the suitability atlas to predict the temporal-spatial distribution of land use through neighborhood relationship analysis. The land-use types are redistributed until the land-use area predicted by the CA-Markov is satisfied. The transition probability matrix and the transition area matrix of land use are calculated using Markov Chain Model based on observed land-use maps (LULC 2005 and LULC 2015). Based on the DEM, road, water, and historical land-use (LULC 2015) information of the study area, the suitability atlas of land-use types was obtained by the MCE module in IDRISI. The key assumption of the model is that the annual evolution trend of land use in the future is the same as the historical period, and the land use in 2055 and 2075 must satisfy the requirements of General Planning of Land Use in Nanyang city (2006–2020) in which the key driving factor is the development of local society and economy. Then, the land-use maps in 2055 and 2075 are projected based on the transition probability matrix, transition area matrix, and suitability atlas. Furthermore, the Kappa metrics is used to measure the performance of the model. This method has been applied in previous studies (Wu et al., 2012; Andaryani et al., 2019; Chanapathi and Thatikonda, 2020; Zhou et al., 2020).
The land-use change in 2015 is predicted based on LULC of 1995 and 2005 and three potential road, water, and DEM factors. The simulated land use in 2015 is compared with the observed land-use map to check the prediction accuracy. The Kappa is 0.95, which means the simulation is accurate (Pontius, 2000), so the model can predict future land-use changes in China. The study predicts the future land-use maps in 2055, and 2075, representing the land use of future two periods (2040–2060, 2060–2080), respectively, based on the transition area matrix LULC2005 and LULC 2015. From Table 3, it is suggested that the agricultural areas are reduced, and the urban areas are increased due to urbanization in the future. In addition, the other areas have not changed significantly compared with historical periods. Those results satisfied the constraint of General Planning of Land Use in Nanyang city (2006–2020) except for the forest areas that are less than the area required by the General Planning of Land Use in Nanyang city (2006–2020). But, the forest areas in 2055 and 2075 are increased compared to LULC 2015, which satisfies the regulation trend of the conversion of farmland to forest. Figure 1 gives the spatial distribution of land-use maps for 2015, 2055, and 2075.
Scenarios Setup
Owing to the great uncertainty of future climate change, multi-emission scenarios and multi-climate models are adopted to reduce the uncertainty caused by different models and scenarios (Molina-Navarro et al., 2018; Schürz et al., 2018). Eight GCMs corresponding to the two emission scenarios RCP4.5 (CMCC-CMS, GFDL-ESM2G, IPSL-CM5A-LR, and MPI-ESM-LR) and RCP8.5 (BCC-CSM1-1-m, CanESM2, MIROC-ESM, and MRI-CGCM3) are adopted by using 10 and 90% quantiles of the change of average rainfall and temperature in the future predicted by each GCM relative to the baseline period (1961–2005) (Immerzeel et al., 2013) (Figure 2). The GCMs are corrected by the daily bias correction (DBC) (Chen et al., 2013) based on the historical climate data (1961–2005), including daily temperature (maximum, minimum) and precipitation, before predicting the future climate scenarios (2041–2080). Four twenty periods are selected (1980–1999, 2000–2019, 2041–2060, 2061–2080), representing the historical period, baseline period, and the two future periods, respectively. To preliminarily understand the impact of climate change and land-use change on yearly streamflow, sediment, and TP loads, the inter-combination of climate/land-use in the historical period and the baseline period is used as the input of the SWAT model. Eight GCMs outputs for the future period (2041–2060, 2061–2080) are combined with land use assumed to be the same as the baseline period (LULC 2015) to assess the impact of individual climate change on yearly streamflow, sediment, and TP loads. To analyze the impact of individual land-use change on yearly streamflow, sediment, and TP loads, the climate for the future period is assumed to be the same as the baseline period (2000–2019). To assess the combined impact of climate and land-use change on yearly streamflow, sediment, and TP loads, the projected land use (LULC 2055, LULC 2075) and eight GCMs are used as the input of SWAT. In this study, a total of 46 scenarios are designed (Table 4). The following are detailed scenario settings:
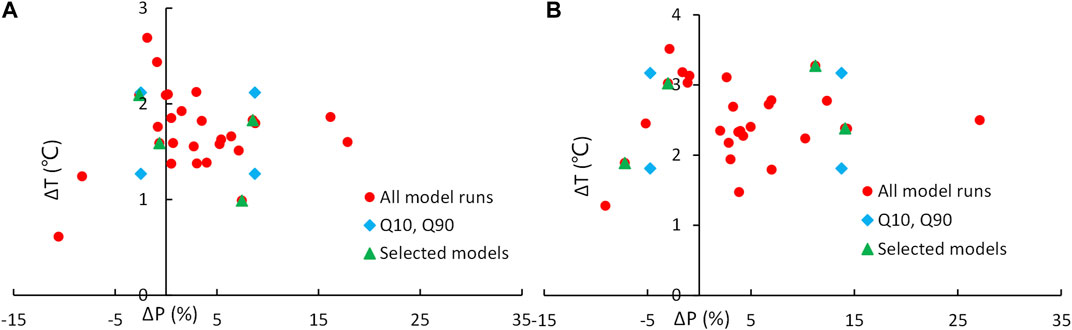
FIGURE 2. Model selection in this study for RCP4.5 and RCP8.5. ΔP, ΔT are the change of average annual precipitation and temperature in 2040–2080 relative to the historical period (1961–2019) (The selected models in RCP4.5: CMCC-CMS, GFDL-ESM2G, IPSL-CM5A-LR, MPI-ESM-LR; and in RCP8.5: CanESM2, BCC-CSM1.1m, MIROC-ESM, MRI-CGCM3).
Historical period: the historical/baseline period land-use (LULC 1995, LULC 2015) and historical/baseline period climate data (1980–1999, 2000–2019) are inter-combined to run the SWAT model (2 LULC×2 climate = 4).
Baseline period: the observed historical climate data (2000–2019) and observed land-use (LULC 2015) are used to run the calibrated SWAT model.
Future period: two future periods (2041–2060 and 2061–2080) are used to analyze the future impact of climate and land-use change.
Individual climate change scenarios: the bias-corrected eight GCMs of two representative concentration pathways (RCP4.5, RCP8.5), the multi-model ensemble means of two representative concentration pathways (RCP4.5, RCP8.5) for two future periods, and land-use for the baseline period (LULC 2015) are used (1 LULC 2015× [8 GCMs + 1 ensemble mean×2 pathways] ×2 = 20).
Individual land-use change scenarios: the projected land-uses (LULC 2055, LULC 2075) with climate for the baseline period are applied (2 LULC×1 climate = 2).
Combined scenarios: the bias-corrected eight GCMs of two representative concentration pathways (RCP4.5, RCP8.5), the multi-model ensemble means under two representative concentration pathways (RCP4.5, RCP8.5) with the projected land-uses (LULC 2055, LULC 2075) are considered ([8 GCMs + 1 ensemble mean×2 pathways] ×2 = 20).
Synergistic scenarios: the combined effect is more significant than the linear superposition of the single climate effect and the single land-use effect, and the additional part is the synergistic effect between climate and land-use change as a result of the interaction between climate and land-use (Dosdogru et al., 2020). Understanding the synergistic impact (combined impact minus sum of individual impacts) of climate and land-use change on streamflow, sediment, and TP loads can help to realize the interaction of climate and land-use and take adequate measures for resources management. The following is the impact of synergistic between climate and land-use on parameter Y:
Results and Discussions
Calibration and Validation
The SWAT model is calibrated and validated in SWAT-Calibration Uncertainty Program (SWAT-CUP), where the Sequential Uncertainty Fitting (SUFI-2) equipped with great performance is selected. The selected calibration period and validation period for streamflow, sediment, and TP loads must include typical peak years and normal years, respectively. Therefore, the average monthly data from the only XinDianPu station is used for calibration and validation, where period 2018–2019 for streamflow, sediment, and TP loads is used to validate, period 2010–2017 for streamflow, 2013–2017 for sediment, and 2012–2017 for TP loads is used to calibrate. The Nash-Sutcliffe Efficiency (NSE) and coefficient of determination (R2) are used as the performance metrics for streamflow, sediment, and TP loads. Owing to the sequential influence relationship between variables, the model is first calibrated for streamflow, second calibrated for sediment, and finally calibrated for TP loads. Figure 3 shows that the performance of the model for streamflow, sediment, and TP loads is satisfied, where the NSE > 0.5 and the R2 > 0.6 (Moriasi et al., 2007; Anand et al., 2018). The result shows that the SWAT model for the Baihe River watershed can be used to simulate and predict the future.
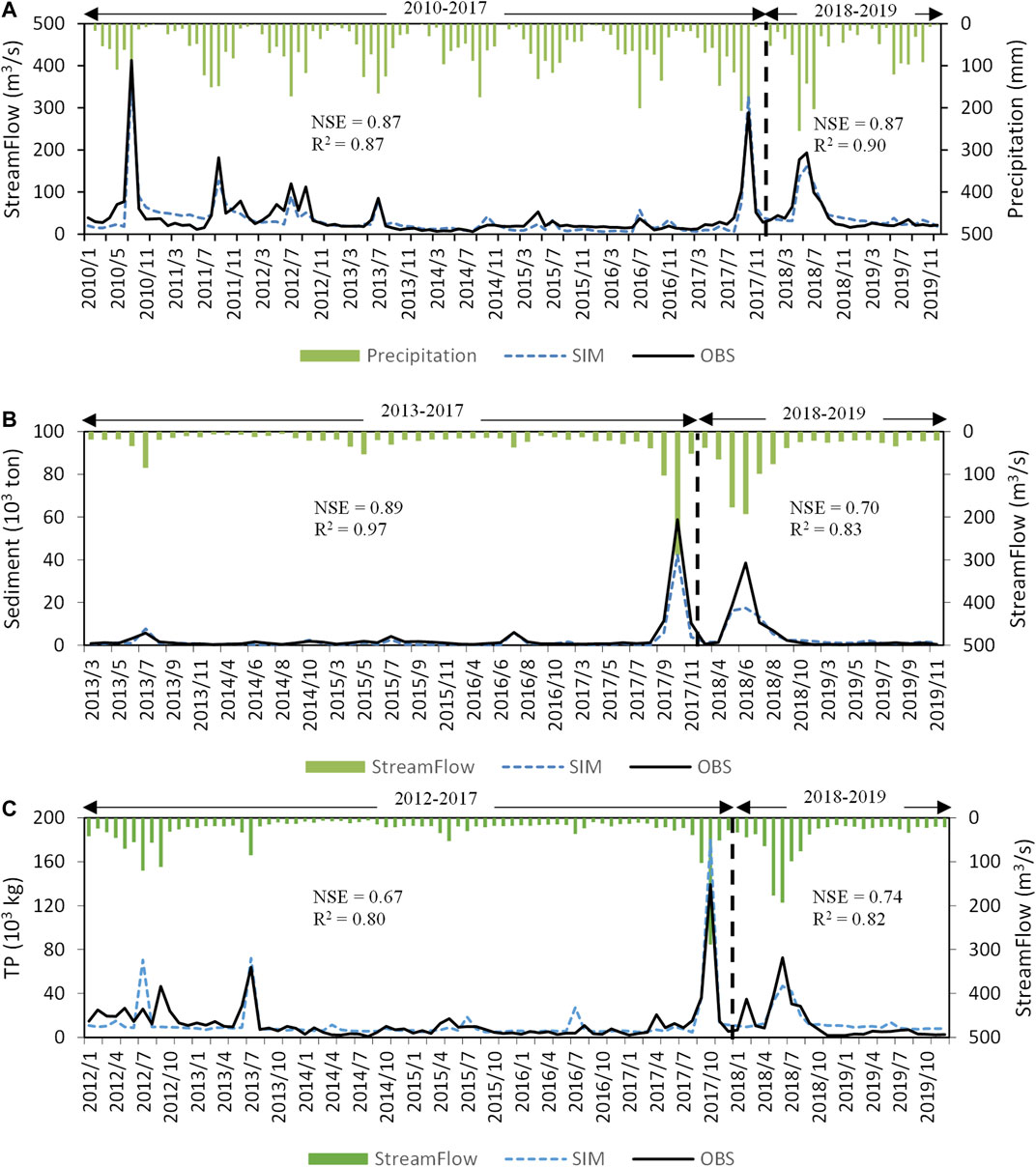
FIGURE 3. Comparison of the simulated and observed streamflow, sediment, and TP loads at the Baihe river basin (Dotted line segment calibration and validation period).
The Impact of Past Land-Use and Climate Change on Streamflow, Sediment, and TP Loads
To study the impact of individual land-use change in the past on streamflow, sediment, and TP loads, the land-use scenarios for S1 and S2 (Table 4) are simulated. The land-use change has a negative effect on average annual streamflow, sediment, and TP loads, whereas the result is more negligible on streamflow (−4%) and TP loads (−2%) compared to sediment (−6%) (Table 5). The study in the Valapattanam River Basin in India also indicated that land-use changes have a more predominant impact on sediment than runoff (Kreiling et al., 2020). The minor reduction in mean streamflow is due to the fewer urban areas in 1995 and 2005 compared to the historical period (Table 3). Urbanization is the primary factor increasing the streamflow due to increasing impervious areas (Aghsaei et al., 2020; Makhtoumi et al., 2020). Furthermore, some studies have reported similar conclusions (Nie et al., 2011; Yan et al., 2013). The decrease of sediment is attributed to the reduction of streamflow and increase in grassland (pasture). In the current study, the streamflow is the main driving factor of the sediment transport which shows significant correlation (R2 = 0.711, p < 0.01) (Wagena and Easton, 2018; Aghsaei et al., 2020), and vegetation interception will also reduce soil erosion (Wang et al., 2020). Forests contribute to the maintenance of soil and water; instead, it is found that the sediment output is still high when the forest area is an increase in the historical period, which may attribute to the higher slope of the forest land (Haregeweyn et al., 2017; Ouyang et al., 2018). Both streamflow and sediment decide the routing of TP loads (Tang et al., 2018), so TP loads are showing the same decreasing trend and ignoring slight increase of agricultural areas (1.5%) that received much fertilizer, the main source of TP loads (Liu et al., 2013; Teshager et al., 2016). In terms of the inter-annual variation of streamflow, sediment, and TP loads, land-use change can increase the variability of streamflow (0.57), sediment (0.95), and TP loading (1.19) under the S2 scenario (Table 5). Moreover, the inter-annual variation of TP loads is the greatest, followed by sediment and the streamflow is the least.
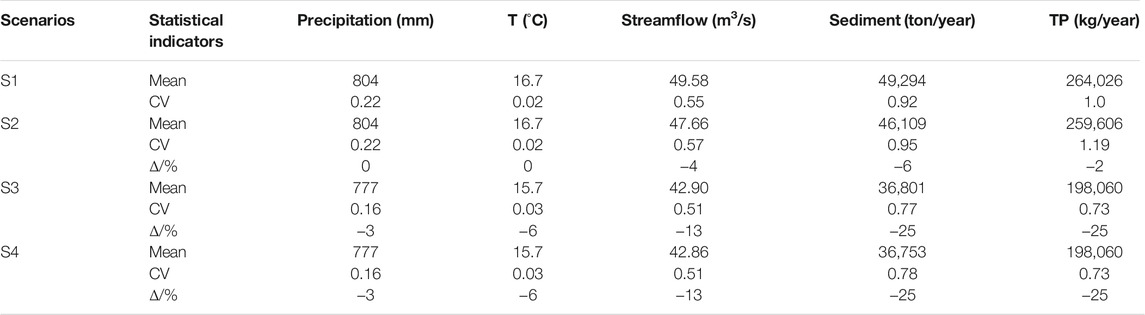
TABLE 5. The impact of climate change/land-use change on multi-annual average and Coefficient of Variation (CV) of precipitation, temperature, streamflow, sediment, and TP loads compared to the baseline period (2000–2019).
Climate change has a more significant impact on annual streamflow and sediment, which results in the decreasing of streamflow (13%), sediment (625%), and TP loading (25%) in S3 compared to the baseline period. The decreasing rainfall source and the rising temperature amplified evaporation for S3 may explain the decrease in streamflow. Notably, in streamflow and sediment decreasing, TP loads also present the same trend. Conversely, because of the significant positive correlation (R2 = 0.71, p < 0.01) between streamflow and sediment, the sediment has a similar trend with streamflow under S3. On the other hand, the mean annual sediment decreasing by 25% for S3, which is also due to the change in maximum rainfall intensity (1980–1999: 113 mm/d) compared to a baseline period (119 mm/d), altered the soil and stream erosion (Molina-Navarro et al., 2018; Wagena and Easton, 2018). There exists a significant correlation between sediment and rainfall intensification (R2 = 0.519, p < 0.01). Furthermore, the similar magnitude of streamflow, sediment, and TP loads between the combined scenario and the climate change scenario can be found due to the minor variation tendency of land-use (Table 3). In addition, the inter-annual variation of flow, sediment, and TP loads is changed under climate change. In general, climate change has more effect on annual streamflow, sediment, and TP loads and can decrease the inter-annual variation of streamflow, sediment, and TP loads.
Individual or Combined Impact of Land-Use/Climate Change on Streamflow in the Future
Individual Impact of Land-Use Change
S5 and S6 are used to predict the change of annual streamflow under individual land-use change scenarios and compare it with the baseline period (S1). Future land-use change has no effect on multi-annual average streamflow for LULC2055 and has little effect (1%) for LULC 2075 (Figures 4, 5). The coefficient of variation (CV) (0.759, 0.756, and 0.759) in S5, S6, and baseline period are similar, which means the intra-annual variation of streamflow is the same with the baseline period under land-use change (Figure 6). In addition, the CV of annual streamflow for S5 (0.551) and S6 (0.543) is slightly smaller than the baseline period (0.552), which means the inter-annual variation of streamflow is reduced slightly under future land-use change (Figure 7). These phenomena indicate that the future land-use change has minimal influence on the annual average streamflow (Tang et al., 2011; Guse et al., 2015), intra-annual variation, and inter-annual variation of streamflow. In addition, the intra-annual variation of streamflow is always higher than inter-annual variation with less coefficient of variation.
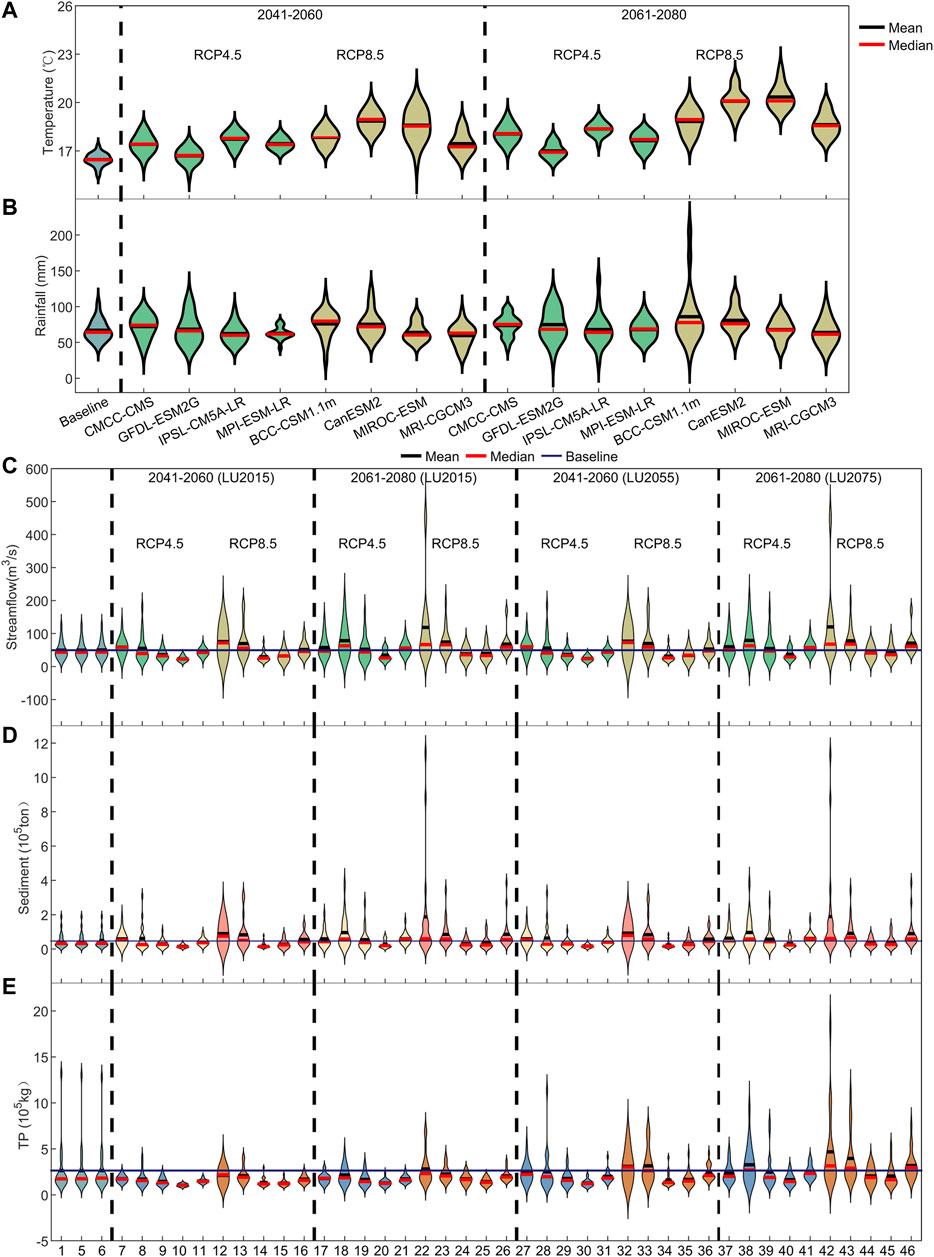
FIGURE 4. The individual and combined impacts of climate change and land use on the multi-annual average temperature, precipitation, streamflow, sediment, and TP under various scenarios (X-axis represents the scenario number corresponding to Table 4).
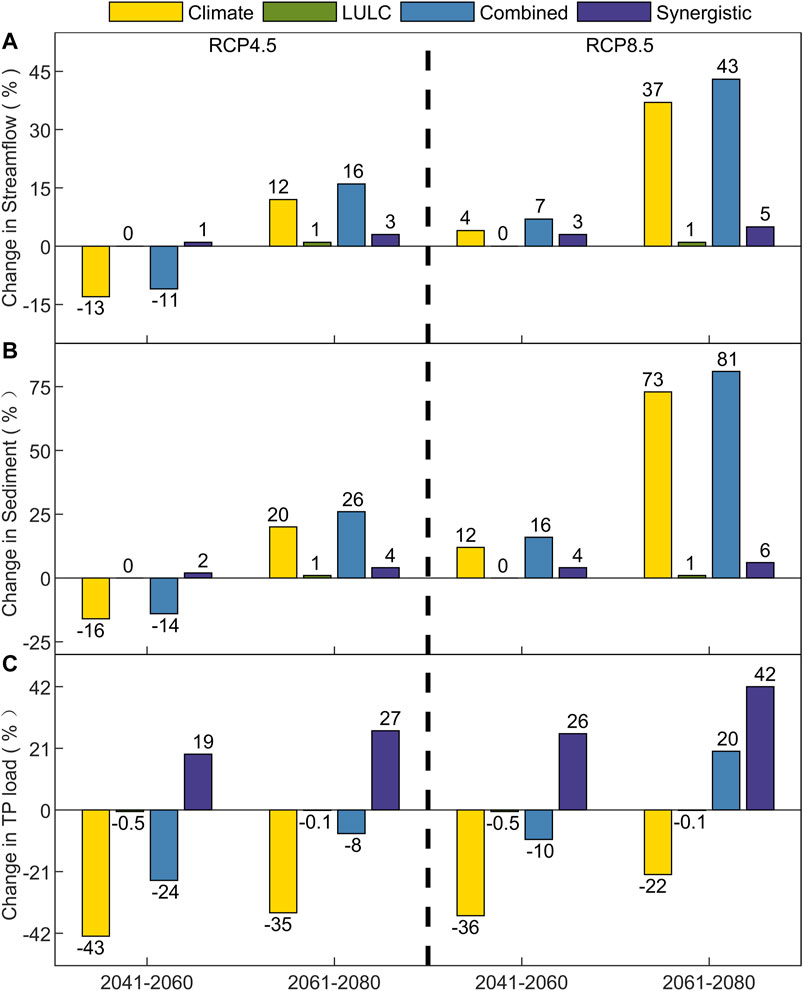
FIGURE 5. The individual and combined impacts of climate change and land use on the Coefficient of Variation of monthly temperature, precipitation, streamflow, sediment, and TP under various scenarios (X-axis represents the scenario number corresponding to Table 4).
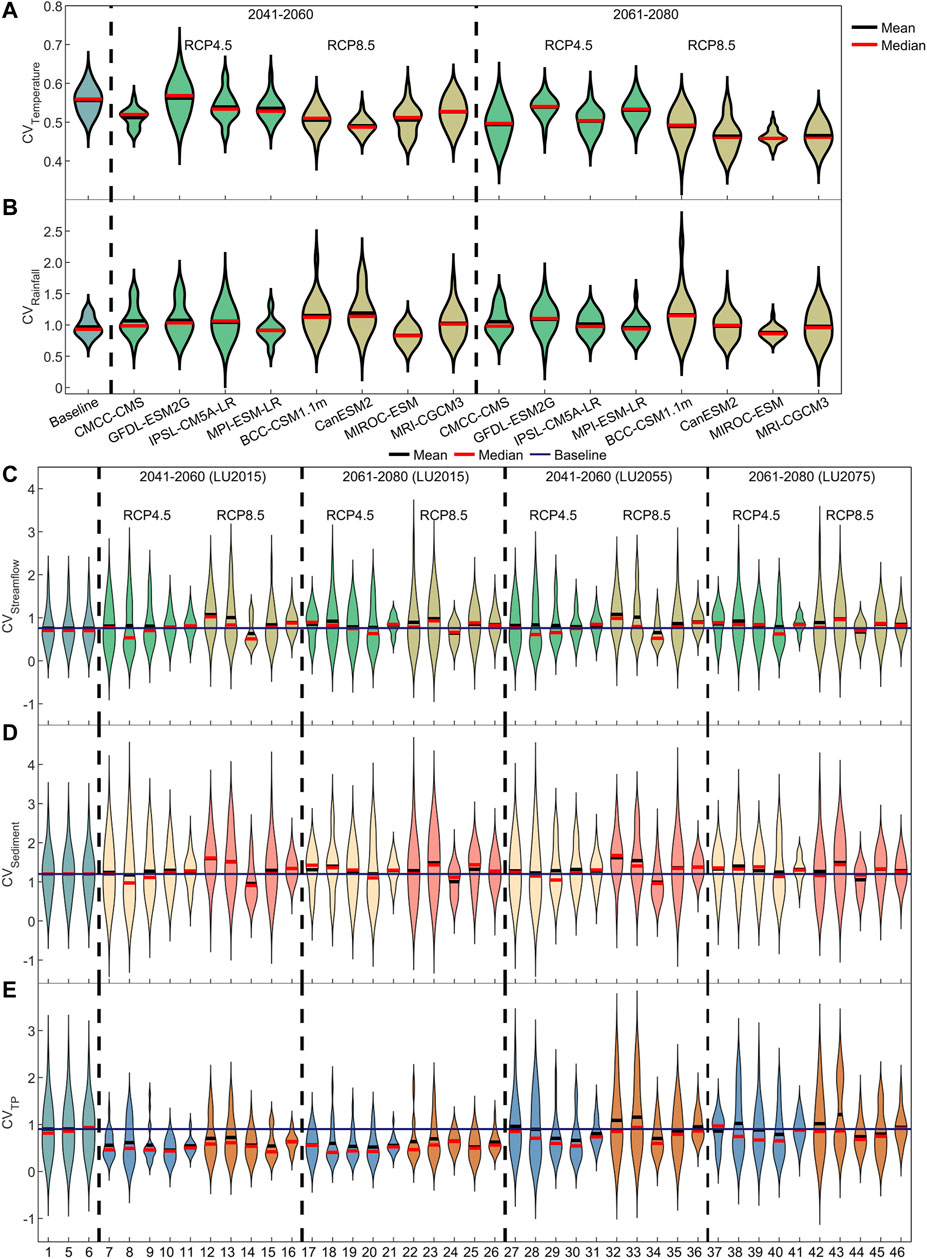
FIGURE 6. The individual, combined, and synergetic impact of land-use change and climate change on the percentage change of multi-model ensemble average annual streamflow, sediment, and TP loads compared to the baseline period (2000–2019).
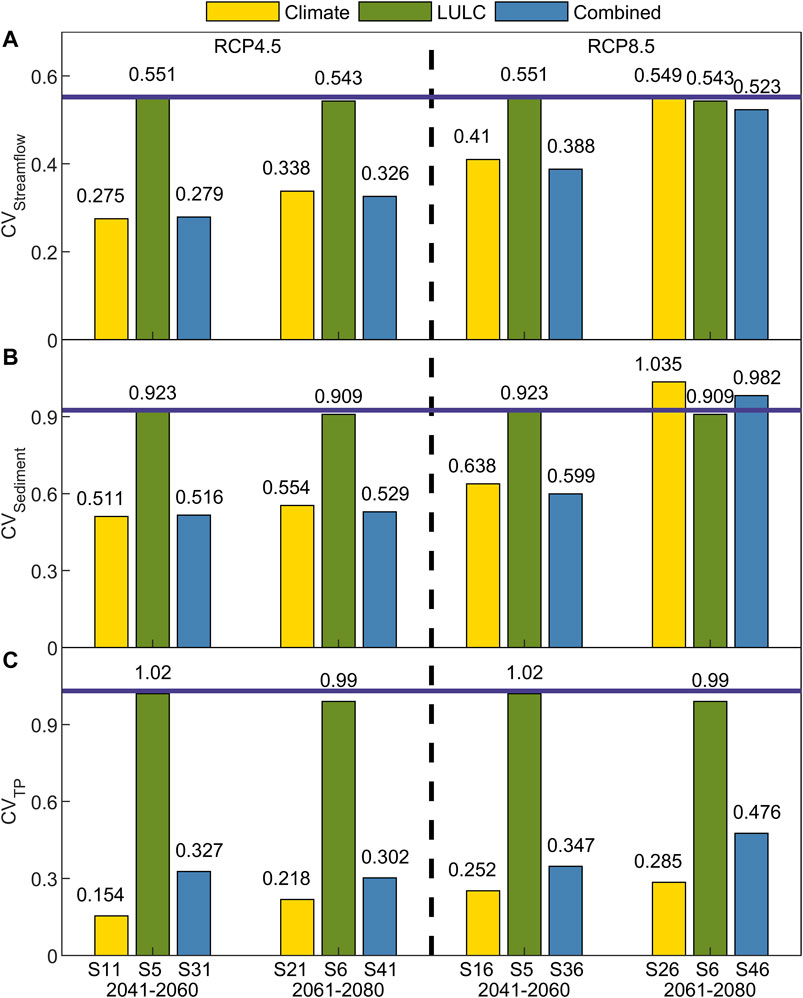
FIGURE 7. The Coefficient of Variation of annual streamflow, sediment, and TP loads under various scenarios (Thick solid line represents the coefficient of variation in baseline period).
Individual Impact of Climate Change
The historical land-use (LU 2015) is used to estimate and compare the impact of individual climate change on streamflow under various climate scenarios S7-S26. The baseline period (S1) annual average streamflow is approximately 49.58 m3/s. However, the impact of each only climate change scenario in RCP4.5 (RCP8.5) on streamflow is very different and variable with a range of −53 – −19% (−40 – −52%) and −31% – −58% (−22 – −139%) change compared to the baseline period during 2041–2060 and 2061–2080, respectively. Nevertheless, the multi-model ensemble annual average streamflow is expected to increase under both RCP4.5 and RCP8.5 compared to S1. And annual streamflow is always greater in RCP8.5 (51.68 m3/s, 67.85 m3/s) than in RCP4.5 (43.3 m3/s, 55.72 m3/s) in both 2041–2060 and 2061–2080, which is attributed to the greater rainfall in RCP8.5 (Figure 4).
As shown in Figure 4, the inter-annual distribution of annual streamflow of each GCM is significantly increased with time under RCP4.5 and RCP8.5, especially for BCC-CSM1-1-m, and high variation is projected among different GCMs. However, the variation is expected to be more stable under individual climate change (CV: 0.275–0.549) when compared to baseline (CV: 0.552); the CV of annual streamflow for the ensemble mean further confirmed the conclusion (Figure 7). The intra-annual variation is various among different GCMs (2041–2060 RCP4.5: 0.683–0.815, 2041–2060 RCP8.5: 0.633–1.073, 2061–2080 RCP4.5: 0.736–0.919, 2061–2080 RCP8.5: 0.643–0.977) (Figure 6) and are more significant than baseline period which may attribute to the greater rainfall variation (Figure 6) (Ye et al., 2020).
Combined Impact of Land-Use and Climate Change
Based on S27–S46, streamflow changes in the next two periods under the combined influence of climate and land-use are predicted. The multi-annual average streamflow varies in the range of −51 – −20% (−37 – −55%) and −26%–60% (−14 – −57%) under the combined impact of RCP4.5 (RCP8.5) and land-use in the corresponding period during 2041–2060 and 2061–2080, respectively. For multi-model ensemble mean, the multi-annual average flow is expected to increase in 2061–2080 (RCP4.5:16%, RCP8.5:43%) and is uncertain in 2041–2060 (RCP4.5: −11%, RCP8.5: 7%) when compared to baseline. In addition, the multi-annual average streamflow is larger than individual climate change scenarios for each corresponding GCM. Obviously, the decreasing amplitude of multi-annual average of multi-model ensemble average streamflow is narrowed, while the increasing amplitude is amplified in each combined scenario, especially for RCP8.5 compared to individual climate change.
In combined scenarios, the inter-annual variation of the annual streamflow of ensemble mean tends to be more stable with a smaller coefficient of variation (Figure 7) than the baseline period and individual climate change scenarios in the corresponding period except for 2041–2060 RCP4.5. However, in terms of intra-annual variation, there is much difference between different GCMs (2041–2060 RCP4.5: 0.676–0.833, 2041–2060 RCP8.5: 0.653–1.078, 2061–2080 RCP4.5: 0.731–0.924, 2061–2080 RCP8.5: 0.669–0.972) (Figure 6) which are all higher than the baseline period. In general, the results show that climate change may increase intra-annual variation and decrease inter-annual variation in the future.
Individual or Combined Impact of Land-Use/Climate Change on Sediment Load in the Future
Individual Impact of Land-Use Change
As shown in Figures 4, 5, the individual land-use change has a negligible impact on annual sediment changes of approximately 0 and 1% for S5 and S6 (Figure 5) compared to the baseline period (49,294 ton), the same as streamflow. The coefficient of variation (CV) of monthly sediment (1.200 and 1.199) in 2000–2019 for LULC2055 and LULC2075 is similar to the baseline period (1.200), which meant the intra-annual variation of sediment is the same as the baseline under land-use change (Figure 6). In addition, the inter-annual variation of annual sediment on both LULC 2055 (0.923) and LULC 2075 (0.909) is relatively stable than baseline (0.925) (Figure 7). These phenomena indicate that the future land-use change has negligible influence on annual sediment load and intra-annual variation of sediment load and is expected to slightly diminish the inter-annual variation of annual sediment. From the CV of sediment, it has concluded that the inter-annual variation of sediment is more stable than the intra-annual variation under both baseline and land-use change scenarios.
Individual Impact of Climate Change
The simulated multi-model ensemble annual sediment for S11 and S16 decreases by 16% and increases by 12% compared to the baseline period with annual average sediment of approximately 49294 kg, and for S21 and S26, increases by 20 and 73%, respectively. There is a big divergence about average annual sediment among different GCMs with a range of −68–−24% (−58–−81%) and 42–94% (−37–−281%) under 2041–2060 RCP4.5 (RCP8.5) and 2061–2080 RCP4.5 (RCP8.5) respectively, especially for BCC-CSM1.1m when compared with the baseline period. The results show that the sediment load is almost greater in RCP8.5 than RCP4.5 for both periods and is expected to increase for multi-model ensemble average annual sediment, which is similar to the streamflow with a smaller variation range.
The CV of monthly sediment loads for different GCMs is different. The CV of multi-model ensemble average monthly sediment load decreases with time period under both RCP4.5 and RCP8.5, which is also greater (smaller) than baseline and land-use change scenarios for S11 and S16 (S21 and S26) (Figure 6). The CV of annual sediment load under multi-model ensemble mean of individual climate change scenarios for both RCP4.5 and RCP8.5 is less than the baseline (0.925) and land-use scenarios (S5: 0.923, S6: 0.909) except the 2061–2080 RCP8.5 (1.035), which also increases with the time period (Figure 7). The CV results for monthly sediment loads and annual sediment loads show that climate change is expected to increase intra-annual variation and diminish inter-annual variation of sediment loads at greater probability.
Combined Impact of Land-Use and Climate Change
There is a high variation in annual sediment load among eight GCMs for each of the combined scenarios of land use and climate change (S27–S46). The predicted future change in the multi-annual average sediment load ranges from −66% to −28% (−53 – −87%) and −36% – −96% (−28 – −283%) under the combined impact of RCP4.5 (RCP8.5) and the corresponding land use (LULC 2055, LULC 2075) during 2041–2060 and 2061–2080, respectively (Figure 4). For multi-model ensemble average sediment load, the sediment load is expected to increase in 2061–2080 (RCP4.5: 26%, RCP8.5: 81%) and is uncertain in 2041–2060 (RCP4.5: −14%, RCP8.5: 16%). The decreasing amplitude of multi-annual average of multi-model ensemble average sediment load is narrowed, while the increasing amplitude is amplified in each combined climate scenario, especially for RCP8.5 compared with individual climate change (Figure 5). The combined impact of climate change and land-use change is expected to increase the annual average sediment load at greater probability in the future.
Under the combined effect of land use and climate change, the CV of monthly sediment load for each scenario is various, greater than baseline for CMCC-CMS, BCC-CSM1-1m, and CanESM2, and almost the same with the individual climate change scenarios. For the multi-model ensemble average, the CV of monthly sediment loads is less than the baseline and land-use scenarios (Figure 6). Moreover, the CV of multi-model ensemble average annual sediment load under a combined scenario is more minor than individual climate change scenarios except for 2041–2060 LULC 2055, and less than individual land-use scenarios and baseline for both time periods except for 2061–2080 LULC 2075. Those results show that the combined effect of climate change and land-use change is expected to increase the intra-annual variation and reduce the inter-annual variation of annual sediment load in the future. In addition, the inter-annual variation of sediment is more petite than intra-annual variation.
Individual or Combined Impact of Land-Use/Climate Change on TP Loads in the Future
Individual Impact of Land-Use Change
The predicted changes in average annual TP loads under future individual land-use change scenarios (S5, S6) are negligible compared to the baseline period (264026 kg), changing by approximately −0.5 and 0.1% for S5 and S6 (Figure 5). In addition, the distribution of annual TP loads for both S5 and S6 is similar to the baseline period, which means the land-use change has a negligible effect on annual TP loads (Figure 4). The coefficients of variation (0.905 and 0.918) of monthly TP loads for S5 and S6 are similar and a little greater than the baseline period (0.903), which means the intra-annual variation of TP loads is relatively low under land-use change. And the inter-annual variation of annual TP loads on both S5 (1.020) and S6 (0.990) is relatively stable than baseline (1.031) (Figure 5). These phenomena indicate that the future land-use change has negligible influence on annual TP loads and is expected to slightly increase the intra-annual variation of TP loads and to slightly minimize the inter-annual variation of annual TP loads. The inter-annual variation of TP loads is also more stable than the intra-annual variation under baseline and all change scenarios.
Individual Impact of Climate Change
The simulated multi-model ensemble annual TP loads for S11 and S16 decrease by 43 and 36% compared to the baseline period, and S21 and S26 decrease by 35 and 22%, respectively. There exists high uncertainty in multi-annual average annual TP loads among GCMs, with the changes ranging from −16% to −15% (−18 – −69%) and −51 – −19% (−46 – −6%) under the 2041–2060 RCP4.5 (RCP8.5) and 2061–2080 RCP4.5 (RCP8.5) respectively compared with the baseline period. The results show that TP loads in RCP8.5 tend to be more significant than RCP4.5 for both periods and are reduced with the time period for the multi-model ensemble average annual TP loads.
As shown in Figure 6, the CV of monthly TP loads for different GCM is various, which is decreased with time period under the ensemble mean of RCP4.5 and RCP8.5 at the same level and all smaller than baseline. Furthermore, the CV of monthly TP loads for each GCM has uncertainty but is all less than the baseline (longer whisky) (Figure 6). The CV of annual TP loads under multi-model ensemble average of individual climate change scenarios for RCP4.5 and RCP8.5 is less than the baseline and increases with time (Figure 7). CV results for monthly TP loads and annual TP loads show that climate change is expected to diminish the intra-annual and inter-annual variation of TP loads with greater probability in the future.
Combined Impact of Land-Use and Climate Change
The annual TP loads vary among 8 GCMs for each of the combined scenarios of land-use and climate change (S27–S46). The predicted future change in multi-annual average TP loads ranges from 26 to −0% (−32 – −33%) and from −52% to −5% (−39 – −19%) under the combined impact of RCP4.5 (RCP8.5) and corresponding land use during 2041–2060 and 2061–2080, respectively (Figure 4). The multi-annual average TP loads for multi-model ensemble average TP loads are expected to decrease in 2041–2060 (RCP4.5: −24%, RCP8.5: −10%) and exist uncertain in 2061–2080 (RCP4.5: −8%, RCP8.5: 20%). The decreasing amplitude of multi-annual average of multi-model ensemble average TP loads is narrowed. In contrast, the increasing amplitude is amplified in each combined climate scenario, especially for RCP8.5 compared to individual climate change. The combined impact of climate change and land-use change is expected to decrease the annual average TP loads and increase the instability of annual TP loads in the future.
Under the combined impact of land-use and climate change, the distribution of CV of monthly TP loads is highly uneven for all GCMs compared to corresponding individual scenarios, and is more uneven than baseline period for BCC-CSM1.1m and CanESM2. However, for a multi-model ensemble, the CV of monthly TP loads are less than the baseline and greater than individual climate change scenarios for both time periods. As shown in Figure 6, the CV of annual TP loads under the combined scenarios is greater than individual climate scenarios and less than the baseline. These results show that the combined effect of climate change and land-use change is expected to further amplify the intra-annual variation, the uncertainty of TP loads for each GCM, and the inter-annual variation of annual TP loads in the future compared to individual climate change. These results can also indicate that climate change has a significant impact on the variation of TP loads.
Synergetic Impact
As shown in Figure 5, the individual land-use change has negligible impact on multi-model ensemble average annual streamflow, sediment, and TP loads ranging from 0% to 1%, 0% to −1%, and −0.5% to −0.1%, respectively. The result is different from previous studies (Abdulkareem et al., 2018) that revealed the pollutant loads were expected to increase with urban areas. This difference can be because of the study area being dominated by agricultural areas with the direct pollution source from fertilizer (Niraula et al., 2013) and urbanization will reduce the major pollution source from fertilizer (Liu et al., 2013). The individual climate change is likely to amplify the annual streamflow and sediment and reduce the annual TP loads (Kalcic et al., 2019), owing to increasing plant phosphorus uptake spurred by higher CO2 (Culbertson et al., 2016). The combined impact of climate and land-use change on the average annual streamflow, sediment, and TP loads are all greater than individual climate change scenarios. Furthermore, the streamflow, sediment, and TP loads can be further amplified under the land-use change in combined scenarios compared to individual climate change to some extent. In addition, the combined effect is larger than the linear superposition of the individual climate effect and the individual land use effect, and the additional part is the synergistic effect between climate and land-use change (Dosdogru et al., 2020). The existence of the synergistic effect means an interaction between climate and land use (Castillo et al., 2014). For example, the land-use change can cause an increase (1%) in annual streamflow, and climate change is expected to increase approximately 12%. When combined simultaneously, the final increase in annual streamflow is approximately 16% higher than the sum of individual effects (Figure 5). In addition, different variables suffer from synergistic effects, among which TP loads have the largest synergistic effect, followed by sediment, and streamflow has the least. The synergistic effect between climate and land use also increases with the time period, and the synergistic effect of RCP8.5 on annual streamflow, sediment, and TP loads is greater than RCP4.5. Therefore, even if the individual land-use change makes a little effect on streamflow, sediment, and TP loads, it is significantly necessary to simultaneously consider both climate and land-use change due to the synergistic impact of climate and land-use change on streamflow, sediment, and TP loads in the future climate change studies (Wagner et al., 2019). The analysis of synergistic impact resulting from climate and land-use change can contribute to understanding impact of both climate and land-use change and help managers to take effective countermeasures to protect the local water environment and resources.
Conclusion
This study explores and predicts the individual and combined impact of land-use change and climate change on streamflow, sediment, and TP loads in the future by systematically aggregating two representative emission pathways (RCP4.5 and RCP8.5), eight GCMs, three 20-years temporal blocks (baseline, near, and far future), three land-use maps (LULC 2015, LULC 2055, and LULC 2075). Moreover, this study compares the individual impact of past land-use map (LULC 1995) and climate (1980–1999) on streamflow, sediment, and TP loads at the 20-years temporal block with the baseline period. The following conclusions can be drawn from the results of this study:
1) Generally, urbanization (10.01 and 10.7%) and loss of agricultural land (−9.52% and −10.21%) are projected in the future (2055 and 2075). The annual average temperature is projected to rise for the eight GCMs in the next two periods, and the temperature of RCP4.5 is lower than RCP8.5, with the values of 1.1°C and 2.0°C, respectively. In addition, the inter-annual variation of temperature is also projected to increase in the future (with a greater variation coefficient) except the MPI-ESM-LR in 2041–2060 and IPSL-CM5A-LR in 2061–2080 compared to baseline. However, the intra-annual variation of temperature is projected to decrease in the future (with a smaller monthly temperature variation coefficient) except for GFDL-ESM2G in 2041–2060. A higher uncertainty exists in the change of mean annual rainfall in the future and is projected to increase over time. The inter-/intra-annual variation of rainfall will increase at a higher probability in the future, which is greater than temperature changes.
2) Based on the SWAT simulation, the individual land-use change has a negligible impact on multi-model ensemble average annual streamflow, sediment, and TP loads ranging from 0% to −1%, 0% to −1%, and −0.5% to −0.1%, respectively. The individual climate change is likely to amplify the annual streamflow and sediment and to reduce the annual TP loads. The combined impact of climate and land-use change on the average annual streamflow, sediment, and TP loads is more significant than that of individual climate change scenarios. Furthermore, the magnitude of variation for TP loads is greater than streamflow, the same as Shrestha et al. (2018). The land-use change also has a negligible effect on the inter-annual variation and intra-annual variation of streamflow and sediment, but the intra-annual variation of TP loads is predicted to grow up. Instead, individual climate change is expected to reduce the inter-/intra-annual variation of TP loads. And the intra-annual variation of streamflow and sediment is magnified as opposed to the inter-annual variation. The change direction of inter-/intra-annual variation of streamflow, sediment, and TP loads under individual climate change is the same as that under the combined scenario. In addition, the average and inter-annual variation of annual streamflow, sediment, and TP loads in RCP8.5 are higher than in RCP4.5.
3) There are remarkable synergistic effects in streamflow, sediment, and TP load when combining the land-use change and climate change scenarios, which means the interplay of climate and land use will exacerbate the individual effect. And TP loads suffer the largest synergistic effect, followed by sediment, and streamflow suffers the least. The synergistic effect between climate and land use also increases with the time period, and the synergistic effect of RCP8.5 on annual streamflow, sediment, and TP loads is greater than RCP4.5. Therefore, even if the individual land-use change has a minor effect on streamflow, sediment, and TP loads, it is significantly necessary to simultaneously consider both climate change and land-use change in the future due to the non-negligible synergistic effect resulting from the combined scenarios.
This is rare research that simultaneously studies the individual/combined/synergistic effects of land-use change and climate change on streamflow, sediment, and TP loads. Based on the results of this study, the conclusion that it is essential to integrate land-use changes and climate change to predict and assess non-point source pollution and streamflow changes in the future environment is drawn. Thus, this study will help to understand the interaction of climate and land-use change and take effective climate change mitigation policy and land-use management policy to mitigate the non-point source pollution. Furthermore, this study does not consider dynamic changes in land use during the simulation period to obtain a more realistic development of land use, and we will investigate it further in the next step.
Data Availability Statement
The original contributions presented in the study are included in the article/Supplementary Material, further inquiries can be directed to the corresponding authors.
Author Contributions
KX and HC carried out the study design. KX download the CMIP5 data. YL and ZS provided the hydrology and TP data. KX carried out the calculation. HC was responsible for supervision, project administration and funding acquisition. KX and HC checked the data processing progress. HC and JK reviewed and edited the manuscript after KX wrote the original draft. All authors contributed to the article and approved the final manuscript.
Funding
This study was supported by National Key Research and Development Program (2017YFA0603702). This is also a collaborative research achievement of the Smart Water Institute (SWI) and Seoul Institute of Technology (SIT), supported by the Seoul Institute of Technology (2021-AB-008), Seoul, South Korea.
Conflict of Interest
The authors declare that the research was conducted in the absence of any commercial or financial relationships that could be construed as a potential conflict of interest.
Publisher’s Note
All claims expressed in this article are solely those of the authors and do not necessarily represent those of their affiliated organizations, or those of the publisher, the editors and the reviewers. Any product that may be evaluated in this article, or claim that may be made by its manufacturer, is not guaranteed or endorsed by the publisher.
References
Abdulkareem, J. H., Sulaiman, W. N. A., Pradhan, B., and Jamil, N. R. (2018). Long-Term Hydrologic Impact Assessment of Non-Point Source Pollution Measured through Land Use/Land Cover (LULC) Changes in a Tropical Complex Catchment. Earth Syst. Environ. 2 (1), 67–84. doi:10.1007/s41748-018-0042-1
Aghsaei, H., Mobarghaee Dinan, N., Moridi, A., Asadolahi, Z., Delavar, M., Fohrer, N., et al. (2020). Effects of Dynamic Land Use/land Cover Change on Water Resources and Sediment Yield in the Anzali Wetland Catchment, Gilan, Iran. Sci. Total Environ. 712, 136449. doi:10.1016/j.scitotenv.2019.136449
Anand, J., Gosain, A. K., and Khosa, R. (2018). Prediction of Land Use Changes Based on Land Change Modeler and Attribution of Changes in the Water Balance of Ganga basin to Land Use Change Using the SWAT Model. Sci. Total Environ. 644, 503–519. doi:10.1016/j.scitotenv.2018.07.017
Andaryani, S., Trolle, D., Nikjoo, M. R., Moghadam, M. H. R., and Mokhtari, D. (2019). Forecasting Near-Future Impacts of Land Use and Climate Change on the Zilbier River Hydrological Regime, Northwestern Iran. Environ. Earth Sci. 78 (6), 188. doi:10.1007/s12665-019-8193-4
Arnold, J. G., Srinivasan, R., Muttiah, R. S., and Williams, J. R. (1998). Large Area Hydrologic Modeling and Assessment Part I: Model Development 1. J. Am. Water Resour. Assoc. 34 (1), 73–89. doi:10.1111/j.1752-1688.1998.tb05961.x
Berka, C., Schreier, H., and Hall, K. (2001). Linking Water Quality with Agricultural Intensification in a Rural Watershed. Water Air Soil Pollut. 127 (1), 389–401. doi:10.1023/A:1005233005364
CARD: SWAT literature database for peer-reviewed journal articles (2021). Center for Agricultural and Rural Development, Iowa State University. Ames, Iowa. Available at: https://www.card.iastate.edu/swat_articles/(Accessed may, 2021).
Castillo, C. R., Güneralp, İ., and Güneralp, B. (2014). Influence of Changes in Developed Land and Precipitation on Hydrology of a Coastal Texas Watershed. Appl. Geogr. 47, 154–167. doi:10.1016/j.apgeog.2013.12.009
Chanapathi, T., and Thatikonda, S. (2020). Investigating the Impact of Climate and Land-Use Land Cover Changes on Hydrological Predictions over the Krishna River basin under Present and Future Scenarios. Sci. Total Environ. 721, 137736. doi:10.1016/j.scitotenv.2020.137736
Chen, J., Brissette, F. P., Chaumont, D., and Braun, M. (2013). Performance and Uncertainty Evaluation of Empirical Downscaling Methods in Quantifying the Climate Change Impacts on Hydrology over Two North American River Basins. J. Hydrol. 479, 200–214. doi:10.1016/j.jhydrol.2012.11.062
Chen, Y., Ale, S., Rajan, N., and Srinivasan, R. (2017). Modeling the Effects of Land Use Change from Cotton (Gossypium Hirsutum L.) to Perennial Bioenergy Grasses on Watershed Hydrology and Water Quality under Changing Climate. Agric. Water Manage. 192, 198–208. doi:10.1016/j.agwat.2017.07.011
Culbertson, A. M., Martin, J. F., Aloysius, N., and Ludsin, S. A. (2016). Anticipated Impacts of Climate Change on 21st Century Maumee River Discharge and Nutrient Loads. J. Great Lakes Res. 42 (6), 1332–1342. doi:10.1016/j.jglr.2016.08.008
Dosdogru, F., Kalin, L., Wang, R., and Yen, H. (2020). Potential Impacts of Land Use/cover and Climate Changes on Ecologically Relevant Flows. J. Hydrol. 584, 124654. doi:10.1016/j.jhydrol.2020.124654
El-Khoury, A., Seidou, O., Lapen, D. R., Que, Z., Mohammadian, M., Sunohara, M., et al. (2015). Combined Impacts of Future Climate and Land Use Changes on Discharge, Nitrogen and Phosphorus Loads for a Canadian River basin. J. Environ. Manage. 151, 76–86. doi:10.1016/j.jenvman.2014.12.012
Ervinia, A., Huang, J., Huang, Y., and Lin, J. (2019). Coupled Effects of Climate Variability and Land Use Pattern on Surface Water Quality: An Elasticity Perspective and Watershed Health Indicators. Sci. Total Environ. 693, 133592. doi:10.1016/j.scitotenv.2019.133592
Ervinia, A., Huang, J., and Zhang, Z. (2020). Nitrogen Sources, Processes, and Associated Impacts of Climate and Land-Use Changes in a Coastal China Watershed: Insights from the INCA-N Model. Mar. Pollut. Bull. 159, 111502. doi:10.1016/j.marpolbul.2020.111502
Gao, X., Ouyang, W., Hao, Z., Shi, Y., Wei, P., and Hao, F. (2017). Farmland-Atmosphere Feedbacks Amplify Decreases in Diffuse Nitrogen Pollution in a Freeze-Thaw Agricultural Area under Climate Warming Conditions. Sci. Total Environ. 579, 484–494. doi:10.1016/j.scitotenv.2016.11.070
Guse, B., Pfannerstill, M., and Fohrer, N. (2015). Dynamic Modelling of Land Use Change Impacts on Nitrate Loads in Rivers. Environ. Process. 2 (4), 575–592. doi:10.1007/s40710-015-0099-x
Haregeweyn, N., Tsunekawa, A., Poesen, J., Tsubo, M., Meshesha, D. T., Fenta, A. A., et al. (2017). Comprehensive Assessment of Soil Erosion Risk for Better Land Use Planning in River Basins: Case Study of the Upper Blue Nile River. Sci. Total Environ. 574, 95–108. doi:10.1016/j.scitotenv.2016.09.019
Hartmann, J., Moosdorf, N., Lauerwald, R., Hinderer, M., and West, A. J. (2014). Global Chemical Weathering and Associated P-Release - the Role of Lithology, Temperature and Soil Properties. Chem. Geology. 363, 145–163. doi:10.1016/j.chemgeo.2013.10.025
Immerzeel, W. W., Pellicciotti, F., and Bierkens, M. F. P. (2013). Rising River Flows Throughout the Twenty-First Century in Two Himalayan Glacierized Watersheds. Nat. Geosci 6 (9), 742–745. doi:10.1038/ngeo1896
Kalcic, M. M., Muenich, R. L., Basile, S., Steiner, A. L., Kirchhoff, C., and Scavia, D. (2019). Climate Change and Nutrient Loading in the Western Lake Erie Basin: Warming Can Counteract a Wetter Future. Environ. Sci. Technol. 53 (13), 7543–7550. doi:10.1021/acs.est.9b01274
Khoi, D. N., and Suetsugi, T. (2014). Impact of Climate and Land-Use Changes on Hydrological Processes and Sediment Yield-A Case Study of the Be River Catchment, Vietnam. Hydrological Sci. J. 59 (5), 1095–1108. doi:10.1080/02626667.2013.819433
Kim, J., Choi, J., Choi, C., and Park, S. (2013). Impacts of Changes in Climate and Land Use/Land Cover under IPCC RCP Scenarios on Streamflow in the Hoeya River Basin, Korea. Sci. Total Environ. 452-453, 181–195. doi:10.1016/j.scitotenv.2013.02.005
Kreiling, R. M., Thoms, M. C., Bartsch, L. A., Larson, J. H., and Christensen, V. G. (2020). Land Use Effects on Sediment Nutrient Processes in a Heavily Modified Watershed Using Structural Equation Models. Water Resour. Res. 56 (7), e2019WR026655. doi:10.1029/2019WR026655
Li, S., Zhang, L., Du, Y., Zhuang, Y., and Yan, C. (2020). Anthropogenic Impacts on Streamflow-Compensated Climate Change Effect in the Hanjiang River Basin, China. J. Hydrol. Eng. 25 (1), 04019058. doi:10.1061/(ASCE)HE.1943-5584.0001876
Li, Z., Zhang, R., Liu, C., Zhang, R., Chen, F., and Liu, Y. (2020b). Phosphorus Spatial Distribution and Pollution Risk Assessment in Agricultural Soil Around the Danjiangkou Reservoir, China. Sci. Total Environ. 699, 134417. doi:10.1016/j.scitotenv.2019.134417
Liang, K., Jiang, Y., Qi, J., Fuller, K., Nyiraneza, J., and Meng, F.-R. (2020). Characterizing the Impacts of Land Use on Nitrate Load and Water Yield in an Agricultural Watershed in Atlantic Canada. Sci. Total Environ. 729, 138793. doi:10.1016/j.scitotenv.2020.138793
Lintern, A., Webb, J. A., Ryu, D., Liu, S., Waters, D., Leahy, P., et al. (2018). What Are the Key Catchment Characteristics Affecting Spatial Differences in Riverine Water Quality? Water Resour. Res. 54 (10), 7252–7272. doi:10.1029/2017WR022172
Liu, R., Zhang, P., Wang, X., Chen, Y., and Shen, Z. (2013). Assessment of Effects of Best Management Practices on Agricultural Non-point Source Pollution in Xiangxi River Watershed. Agric. Water Manage. 117, 9–18. doi:10.1016/j.agwat.2012.10.018
Luo, L., Zhou, Q., He, H. S., Duan, L., Zhang, G., and Xie, H. (2020). Relative Importance of Land Use and Climate Change on Hydrology in Agricultural Watershed of Southern China. Sustainability 12 (16), 6423. doi:10.3390/su12166423
Makhtoumi, Y., Li, S., Ibeanusi, V., and Chen, G. (2020). Evaluating Water Balance Variables under Land Use and Climate Projections in the Upper Choctawhatchee River Watershed, in Southeast US. Water 12 (8), 2205. doi:10.3390/w12082205
McDonough, L. K., Santos, I. R., Andersen, M. S., O’Carroll, D. M., Rutlidge, H., Meredith, K., et al. (2020). Changes in Global Groundwater Organic Carbon Driven by Climate Change and Urbanization. Nat. Commun. 11 (1), 1279. doi:10.1038/s41467-020-14946-1
Molina-Navarro, E., Andersen, H. E., Nielsen, A., Thodsen, H., and Trolle, D. (2018). Quantifying the Combined Effects of Land Use and Climate Changes on Stream Flow and Nutrient Loads: A Modelling Approach in the Odense Fjord Catchment (Denmark). Sci. Total Environ. 621, 253–264. doi:10.1016/j.scitotenv.2017.11.251
Moriasi, D. N., Arnold, J. G., Van Liew, M., Bingner, R. L., Harmel, R. D., and Veith, T. L. (2007). Model Evaluation Guidelines for Systematic Quantification of Accuracy in Watershed Simulations. Trans. Asabe 50 (3), 885–900. doi:10.13031/2013.23153
Mukundan, R., Hoang, L., Gelda, R. K., Yeo, M.-H., and Owens, E. M. (2020). Climate Change Impact on Nutrient Loading in a Water Supply Watershed. J. Hydrol. 586, 124868. doi:10.1016/j.jhydrol.2020.124868
Ndulue, E., and Mbajiorgu, C. (2018). Modeling Climate and Landuse Change Impacts on Streamflow and Sediment Yield of an Agricultural Watershed Using SWAT. Agric. Eng. Int. : CIGR e-journal 20, 15–25. https://www.researchgate.net/publication/330635281.
Nie, W., Yuan, Y., Kepner, W., Nash, M. S., Jackson, M., and Erickson, C. (2011). Assessing Impacts of Landuse and Landcover Changes on Hydrology for the Upper San Pedro Watershed. J. Hydrol. 407 (1), 105–114. doi:10.1016/j.jhydrol.2011.07.012
Niraula, R., Kalin, L., Srivastava, P., and Anderson, C. J. (2013). Identifying Critical Source Areas of Nonpoint Source Pollution with SWAT and GWLF. Ecol. Model. 268, 123–133. doi:10.1016/j.ecolmodel.2013.08.007
Ouyang, W., Wu, Y., Hao, Z., Zhang, Q., Bu, Q., and Gao, X. (2018). Combined Impacts of Land Use and Soil Property Changes on Soil Erosion in a Mollisol Area under Long-Term Agricultural Development. Sci. Total Environ. 613-614, 798–809. doi:10.1016/j.scitotenv.2017.09.173
Peters, M. K., Hemp, A., Appelhans, T., Becker, J. N., Behler, C., Classen, A., et al. (2019). Climate-Land-Use Interactions Shape Tropical Mountain Biodiversity and Ecosystem Functions. Nature 568 (7750), 88–92. doi:10.1038/s41586-019-1048-z
Pontius, R. (2000). Quantification Error Versus Location Error in Comparison of Categorical Maps. Photogrammetric Eng. Remote Sensing 66, 1011–1016. https://www.researchgate.net/publication/330635281.
Ridwansyah, I., Yulianti, M., Apip, , Onodera, S.i., Shimizu, Y., Wibowo, H., et al. (2020). The Impact of Land Use and Climate Change on Surface Runoff and Groundwater in Cimanuk Watershed, Indonesia. Limnology 21 (3), 487–498. doi:10.1007/s10201-020-00629-9
Schürz, C., Hollosi, B., Matulla, C., Pressl, A., Ertl, T., Schulz, K., et al. (2019). A Comprehensive Sensitivity and Uncertainty Analysis for Discharge and Nitrate-Nitrogen Loads Involving Multiple Discrete Model Inputs under Future Changing Conditions. Hydrol. Earth Syst. Sci. Discuss. 23 (3), 1211–1244. doi:10.5194/hess-23-1211-2019
Shrestha, S., Bhatta, B., Shrestha, M., and Shrestha, P. K. (2018). Integrated Assessment of the Climate and Landuse Change Impact on Hydrology and Water Quality in the Songkhram River Basin, Thailand. Sci. Total Environ. 643, 1610–1622. doi:10.1016/j.scitotenv.2018.06.306
Tang, L., Yang, D., Hu, H., and Gao, B. (2011). Detecting the Effect of Land-Use Change on Streamflow, Sediment and Nutrient Losses by Distributed Hydrological Simulation. J. Hydrol. 409 (1), 172–182. doi:10.1016/j.jhydrol.2011.08.015
Tang, X., Wu, M., and Li, R. (2018). Distribution, Sedimentation, and Bioavailability of Particulate Phosphorus in the Mainstream of the Three Gorges Reservoir. Water Res. 140, 44–55. doi:10.1016/j.watres.2018.04.024
Teshager, A. D., Gassman, P. W., Schoof, J. T., and Secchi, S. (2016). Assessment of Impacts of Agricultural and Climate Change Scenarios on Watershed Water Quantity and Quality, and Crop Production. Hydrol. Earth Syst. Sci. 20 (8), 3325–3342. doi:10.5194/hess-20-3325-2016
Trang, N. T. T., Shrestha, S., Shrestha, M., Datta, A., and Kawasaki, A. (2017). Evaluating the Impacts of Climate and Land-Use Change on the Hydrology and Nutrient Yield in a Transboundary River basin: A Case Study in the 3S River Basin (Sekong, Sesan, and Srepok). Sci. Total Environ. 576, 586–598. doi:10.1016/j.scitotenv.2016.10.138
Tu, J. (2009). Combined Impact of Climate and Land Use Changes on Streamflow and Water Quality in Eastern Massachusetts, USA. J. Hydrol. 379 (3), 268–283. doi:10.1016/j.jhydrol.2009.10.009
Wagena, M. B., and Easton, Z. M. (2018). Agricultural Conservation Practices Can Help Mitigate the Impact of Climate Change. Sci. Total Environ. 635, 132–143. doi:10.1016/j.scitotenv.2018.04.110
Wagner, P. D., Bhallamudi, S. M., Narasimhan, B., Kantakumar, L. N., Sudheer, K. P., Kumar, S., et al. (2016). Dynamic Integration of Land Use Changes in a Hydrologic Assessment of a Rapidly Developing Indian Catchment. Sci. Total Environ. 539, 153–164. doi:10.1016/j.scitotenv.2015.08.148
Wagner, P. D., Bhallamudi, S. M., Narasimhan, B., Kumar, S., Fohrer, N., and Fiener, P. (2019). Comparing the Effects of Dynamic versus Static Representations of Land Use Change in Hydrologic Impact Assessments. Environ. Model. Softw. 122, 103987. doi:10.1016/j.envsoft.2017.06.023
Wang, S., Zhang, Z., McVicar, T. R., Guo, J., Tang, Y., and Yao, A. (2013). Isolating the Impacts of Climate Change and Land Use Change on Decadal Streamflow Variation: Assessing Three Complementary Approaches. J. Hydrol. 507, 63–74. doi:10.1016/j.jhydrol.2013.10.018
Wang, W., Chen, L., and Shen, Z. (2020). Dynamic export Coefficient Model for Evaluating the Effects of Environmental Changes on Non-point Source Pollution. Sci. Total Environ. 747, 141164. doi:10.1016/j.scitotenv.2020.141164
Wu, L., Long, T.-y., Liu, X., and Guo, J.-s. (2012). Impacts of Climate and Land-Use Changes on the Migration of Non-point Source Nitrogen and Phosphorus during Rainfall-Runoff in the Jialing River Watershed, China. J. Hydrol. 475, 26–41. doi:10.1016/j.jhydrol.2012.08.022
Yan, B., Fang, N. F., Zhang, P. C., and Shi, Z. H. (2013). Impacts of Land Use Change on Watershed Streamflow and Sediment Yield: An Assessment Using Hydrologic Modelling and Partial Least Squares Regression. J. Hydrol. 484, 26–37. doi:10.1016/j.jhydrol.2013.01.008
Ye, S., Sivapalan, M., and Ran, Q. (2020). Synergistic Impacts of Rainfall Variability and Land Use Heterogeneity on Nitrate Retention in River Networks: Exacerbation or Compensation? Water Resour. Res. 56 (8), e2018WR024226. doi:10.1029/2018WR024226
Zhou, L., Dang, X., Sun, Q., and Wang, S. (2020). Multi-Scenario Simulation of Urban Land Change in Shanghai by Random forest and CA-Markov Model. Sustain. Cities Soc. 55, 102045. doi:10.1016/j.scs.2020.102045
Zhuang, M., Zhang, J., Kong, Z., Fleming, R. M., Zhang, C., and Zhang, Z. (2020). Potential Environmental Benefits of Substituting Nitrogen and Phosphorus Fertilizer with Usable Crop Straw in China during 2000-2017. J. Clean. Prod. 267, 122125. doi:10.1016/j.jclepro.2020.122125
Keywords: climate change, land-use change, streamflow, sediment, total phosphorus loads, SWAT
Citation: Xie K, Chen H, Qiu Y, Kim J-S, Yoon S-K, Lin Y, Liu B, Wang J, Chen J and Zhang S (2021) Exploring and Predicting the Individual, Combined, and Synergistic Impact of Land-Use Change and Climate Change on Streamflow, Sediment, and Total Phosphorus Loads. Front. Environ. Sci. 9:726793. doi: 10.3389/fenvs.2021.726793
Received: 19 June 2021; Accepted: 06 September 2021;
Published: 06 October 2021.
Edited by:
Ioan Cristian Ioja, University of Bucharest, RomaniaReviewed by:
Ketema Tilahun Zeleke, Charles Sturt University, AustraliaSorin Cheval, Henri Coanda Air Force Academy, Romania
Copyright © 2021 Xie, Chen, Qiu, Kim, Yoon, Lin, Liu, Wang, Chen and Zhang. This is an open-access article distributed under the terms of the Creative Commons Attribution License (CC BY). The use, distribution or reproduction in other forums is permitted, provided the original author(s) and the copyright owner(s) are credited and that the original publication in this journal is cited, in accordance with accepted academic practice. No use, distribution or reproduction is permitted which does not comply with these terms.
*Correspondence: Hua Chen, Y2h1YUB3aHUuZWR1LmNu; Yunfeng Qiu, eWZxaXVAd2h1LmVkdS5jbg==