- 1State Key Laboratory of Urban and Regional Ecology, Research Center for Eco-Environmental Science, Chinese Academy of Sciences, Beijing, China
- 2International College, University of Chinese Academy of Sciences, Beijing, China
- 3Key Laboratory of Ecosystem Network Observation and Modeling, Institute of Geographic Sciences and Natural Resources Research, Chinese Academy of Sciences, Beijing, China
- 4College of Forestry, Beijing Forestry University, Beijing, China
Widely distributed shrubs in drylands can locally alter soil physicochemical properties, which distinguish soil under plant canopy from soil outside the canopy. In the present study, we used a dominant shrub species Artemisia gmelinii in a semiarid land, SW China, to investigate the consequences of “shrub resource islands” for soil microbial communities and enzymatic activities. Such investigation was made at four sites that differed in rates of rainfall to examine how the consequences were altered by variation in the local climate. The results showed that A. gmelinii enhanced fungal abundance but did not influence bacterial abundance, resulting in higher total microbial abundance and fungal-to-bacterial ratio in under-canopy soil compared to outside-canopy soil. Microbial community composition also differed between the two soils, but this difference only occurred at sites of low rainfall. Redundancy analysis revealed that such composition was attributed to variation in soil water content, bulk density, and total phosphorus as a result of shrub canopy and varying rates of rainfall. Activities of hydrolytic enzymes (β-1,4-glucosidase, β-1,4-N-acetylglucosaminidase, alkaline phosphatase, and leucine aminopeptidase) were higher in under-canopy soil than in outside-canopy soil, among which C-acquisition enzyme, β-1,4-glucosidase, and P-acquisition enzyme, alkaline phosphatase, were also higher in the soil of high rainfall. The overall pattern of enzyme activities did not show differences between under- and outside-canopy soils, but it separated the sites of high rate from that of low rates of rainfall. This pattern was primarily driven by variation in soil physicochemical properties rather than variation in soil microbial community, suggesting that the distribution pattern of enzyme activities may be more sensitive to variation in rainfall than to shrub canopy. In conclusion, our study shows that shrub species A. gmelinii can shift the soil microbial community to be fungal-dominant and increase hydrolytic enzyme activities, and such effect may depend on local climatic variation, for example, rainfall changes in the semiarid land. The findings of this study highlight the important roles of shrub vegetation in soil biological functions and the sensitivity of such roles to climatic variation in semiarid ecosystems.
Introduction
Soil microbial community is a key component of underground biota and recognized vital roles in a wide range of soil processes, such as litter decomposition and nutrient cycling (Aislabie et al., 2013). Different soil microbial groups in the community are responsible for specific functions. For instance, soil fungi mostly participate in the early stage of litter decomposition, while bacteria play the primary roles in later stages (Hu et al., 2017). Thus, structural shifts of a soil microbial community are often accompanied by its functional changes. Soil microbial communities can secrete extracellular enzymes that take part in numerous soil processes (Brzostek et al., 2013). Among these enzymes, hydrolases are involved in C (e.g., β-glucosidases), N (e.g., β-1,4-N-acetylglucosaminidase and leucine aminopeptidase), and P (e.g., alkaline phosphatases) cycling and thus widely investigated in previous studies (Martinez and Tabatabai 1997; Roberts et al., 2007; Zhao et al., 2016; Li et al., 2019; Thapa et al., 2021). The activities of these enzymes can quickly respond to changes in microbial communities and environmental conditions, and are, therefore, considered as sensitive indicators of microbial dynamics and environmental changes (Bowles et al., 2014). Accordingly, environmental variations that affect a microbial community structure or hydrolytic enzyme activities in a soil may substantially alter the consequences of microbial-based soil processes (Ushio et al., 2008; Dick et al., 2015).
Vegetation provides organic inputs and inorganic nutrients in the form of litter and root exudates. The quality and quantity of these plant-derived residues are important foraging substrates for soil microbes and thus critical for the dynamics of microbial communities and enzyme activities (Shahbaz et al., 2017; Zhao et al., 2019). On the other hand, vegetation may compete for available nutrients with soil microbes, also contributing to steering microbial communities and metabolic activities (Deng et al., 2019). Many studies have shown that vegetation can pronouncedly influence organic matter and nutrient content in the soil, driving compositional shift and metabolic changes of soil microbial communities (e.g., Yin et al., 2014; Li et al., 2015; Wang et al., 2020). This is particularly true in arid and semiarid lands where vegetation coverage and organic matter content in the soil are relatively low (Okin et al., 2001).
Precipitation is also documented to strongly influence soil microbial community and enzyme activities in many terrestrial ecosystems (Zhou et al., 2013; Ren et al., 2017; Ma et al., 2020). This is because precipitation can regulate plant productivity and litter inputs, thereby influencing the amount of available substrates for soil microbial communities (Nielsen and Ball 2015; Luo et al., 2017). In addition, since the migratory ability of microorganisms toward the foraging substrates is limited by diminished pore connectivity under dry conditions (Manzoni et al., 2014), precipitation may also alter microbial community and activities by changing water availability in soils (Ren et al., 2017). Moreover, soil fungi possess complex hyphal networks and are usually less sensitive to drought stress than bacteria, and thus, precipitation may determine microbial community and associated metabolic activities by changing their relative abundance (Sun et al., 2020). The abovementioned potential effects of precipitation on soil microbes are thought to be particularly strong in arid and semiarid systems where they can be magnified by the sporadic and scarce rainfall events (Reynolds et al., 2004; Collins et al., 2008; Ouyang and Li 2020).
Recent years witness increasing research interests studying regulatory roles of vegetation or altered precipitation in steering soil microbial community and enzyme activities (Hortal et al., 2015; Xu et al., 2014; Rath et al., 2019; Ramírez et al., 2020). However, the interactive effects of such roles are rarely investigated: it is yet unknown that whether vegetation roles in regulating soil microbial community and metabolic activities vary with climatic changes, for example, varying rates of rainfall. This question is particularly necessary to be answered in arid and semiarid lands given that these drylands often experience severe soil erosion as a result of variable regime of rainfall (Yu et al., 2019), and standing vegetation is recognized as the key to restoring these eroded lands (Gu et al., 2019).
In the present study, we selected a dominant shrub species Artemisia gmelinii in a semiarid land, southwest China, and collected soils under and outside the canopy of this shrub species from four sites that vary in rates of rainfall, to investigate shrub effects on soil microbial community composition and enzyme activities as well as the extent to which such shrub effects may change with the varying rainfall. We hypothesize that 1) A. gmelinii will alter soil physicochemical properties under the canopy, 2) by which microbial community composition and enzymatic activities in under-canopy soil will differ from that in outside-canopy soil, 3) and the effects of shrubs on soil physicochemical and microbial properties will vary with rates of rainfall in sites where soils are from.
Materials and Methods
Study Area
The study region is located at the basin of the upper Minjiang River (30°44′–32°24′ N; 102°41′–103°58′ E), a first-order branch of the Yangtze River. The Minjiang River is 735 km in length and has an altitude range of 3,560 m. This basin is a 337 km2 region located in the northwestern corner of the Sichuan Province, southwest China. The elevation of this basin ranges from 900 to 3,600 m, and the study area is located between 1,700 and 1800 m in elevation and has a mean annual temperature of 11.2°C. Mean annual precipitation is 500 mm and mean annual evaporation is 1,332 mm in this area, representing a typical semiarid climate. Soil is classified as Calcic Cambisols (FAO–UNESCO, 1988) with shallow depths of 10–30 cm. The coarse texture was composed of 28.7% sand, 68.7% silt, and 2.6% clay by volume, and the parent material is phyllite (Xu et al., 2008). Typical vegetation is patchy dwarf shrubs, with very few herbaceous plants under the canopy. No plants were found at the interspace between the canopies.
Experimental Design and Soil Sampling
In early July 2012, we selected four experimental sites, including Lianghekou (LH), Fenghong (FH), Cuoji (CJ), and Moutuo (MT) that differed in mean annual precipitation along the upper Minjiang River. LH and FH are dry sites with a mean annual precipitation of 335.5 mm, while CJ and MT are wet sites in relation to LH and FH where the mean annual precipitation is 560.6 mm. These sites were formerly farmland and have been abandoned since the 1990s due to perturbation of anthropogenic activities, such as improper grazing activities. The occurring plant species in each site were as follows: Artemisia gmelinii, Bauhinia faberi, and Sophora davidii in LH; A. gmelinii and Ajania potaninii in FH; A. gmelinii, Pulicaria chrysantha, and S. davidii in CJ; and A. gmelinii, B. faberi, and Caryopteris tangutica in MT, respectively. Among these shrub species, A. gmelinii was the most abundant and occurred in all four sites and thus selected as the focal species in this study. A. gmelinii is a perennial tussock and widely distributed in central and eastern Asia. It is usually 50–100 cm tall with a dense, umbrella-shaped canopy that often reaches the ground. This species is commonly found in semiarid ecosystems, at abandoned cropland, and the stand age often varies over time after abandonment.
The experiment was carried out at the end of July, when A. gmelinii reached their maximum photosynthetic rates, and two consecutive weeks of no rain was available for sampling. Three plots (10 m × 10 m) were randomly set in each site at the south-facing hillside with a slope of 37±2 degree. In each plot, three individuals of A. gmelinii with similar-sized canopy were selected. Five soil cores were randomly sampled in the vicinity of individual A. gmelinii stand beneath the canopy and combined to be an under-canopy soil sample. Similarly, five soil cores were also taken in the interspace between canopies of two individual stands in the same plot and combined to be an outside-canopy soil sample. Each sample was collected into a separate new polyethylene bag. The distance between each shrub individual was minimally 3 m to prevent the potential interactions between neighboring plants. All soil cores were collected from a depth of 0–15 cm using a 2 cm diameter soil corer that was thoroughly cleaned with 70% ethanol between samplings to avoid cross-contamination. Consequently, nine under-canopy and nine outside-canopy soil samples were obtained from each site. Soil samples were stored in iceboxes and immediately transported to the laboratory, where samples were thoroughly mixed, and plant and animal materials were removed before going through a sterilized 2 mm mesh sieve. Each sieved sample was used for three soil analyses: 40 g soil was lyophilized and stored at −80°C for PLFA analyses, 20 g soil was stored at 4°C to determine enzyme activities, and 150 g soil was air-dried to measure other soil physicochemical properties.
Physicochemical Analyses
The soil water content (SWC) was determined at 105°C for 24 h in an oven and soil pH was measured in soil suspension (soil: water = 1:2.5 w/w) using a pH meter (FE20K, Mettler Toledo, Switzerland). Soil organic carbon (SOC) was determined colorimetrically (Sims and Haby 1971) by estimating sulfuric acid (H2SO4) and potassium dichromate (K2Cr2O7) wet digestion under low external heat (100°C) for 90 min. Total nitrogen (TN) was measured using an element analyzer (VarioMax C/N, Elementar, Germany), and total phosphorus (TP) was analyzed using perchloric acid–sulfuric acid combined with molybdenum stibium anticolorimetry (Eisenreich et al., 1975). Available nitrogen (AN) was determined using a microdiffusion method after alkaline hydrolysis (Conway 1978). Available phosphorus (AP) was extracted using 0.5 mol/L NaHCO3 and measured using a molybdenum stibium anticolorimetry method (Watanabe and Olsen 1965).
PLFA Analyses
Microbial abundance was assessed using phospholipid fatty acid (PLFA) analyses. Lipids were extracted and purified from 4 g freeze-dried soil and analyzed using methods described by Bligh and Dyer 1959) and Frostegård et al. (1993). Fatty acid methyl esters were identified using a Sherlock Microbial Identification System (MIDI Inc. Newark, DE, United States) and calculated according to the internal 19:0 standard peak area and a known concentration. The extracted fatty acid methyl esters were quantified as peak areas by an Agilent 6850 N gas chromatograph using a flame ionization detector (GC–FID).
All the detected PLFAs (expressed in nmol g−1) were used to calculate the total PLFAs (total PLFA) and measured as an estimate of the total viable microbial abundance. The abundance of an individual PLFA was expressed as the percentage of total PLFAs in each soil sample. Fatty acids i14:0, 14:0, i15:0, a15:0, 15:0, i16:0, i17:0, 17:0, 15:1ω6c, 16:1ω7c, 17:1ω8c, 18:1ω5c, 16:1 2OH, cy17:0, and cy19:0 were selected to represent bacterial PLFA (Bossio and Scow 1998; Frostegård et al. 1993). Of which, the fatty acids i14:0, i15:0, a15:0, i16:0, i17:0, and a17:0 were used to calculate Gram-positive (GP) bacterial abundance and the fatty acids 15:1ω6c, 16:1ω7c, 17:1ω8c, 18:1ω5c, 16:1 2OH, cy17:0, and cy19:0 were used to calculate Gram-negative (GN) bacterial abundance. Fatty acids 18:2ω6c and 16:1ω5c were used to estimate the abundance of saprophytic fungi (F) and arbuscular mycorrhizal fungal (AMF), respectively. The fungal-to-bacterial ratio (F/B) was calculated using total bacterial and saprophytic fungal abundance (Bardgett et al., 1996).
Enzyme Assays
Soil enzymes β-1,4-glucosidase (BG), β-1,4-N-acetylglucosaminidase (NAG), leucine aminopeptidase (LAP), and alkaline phosphatase (AlkP) were measured for their potential activities in this study given their key roles in soil organic matter decomposition and nutrient cycling (Grandy et al., 2013; Xu et al., 2014). The enzymes were bioassayed using a microplate method adapted from studies by Saiya-Cork et al. (2002) and German et al. (2011). A separate standard curve was included for each soil sample to minimize potential quenching effects. The homogenate was prepared with 1 g fresh soil sample suspended in 125 ml Tris buffer (50 mM) at pH 7.5. Slurries were homogenized and fully stirred. A subset of 200 μl obtained homogenates dispensed in each well of a 96-well assay plate. Subsequently, 50 μl fluorometric substrate solution and 200 μl soil homogenate were added to each sample well. Blank control wells received 250 μl Tris buffer, whereas each homogenate control well received 50 μl Tris buffer plus 200 μl soil homogenate. The substrate control well received 50 μl substrate solution and 200 μl Tris buffer. Eight replicate wells were set for each sample and respective control (blank, homogenate, or substrate control). A reference standard curve and a quench standard curve were accordingly created. The concentration gradient of 0, 1, 2.5, 5, 10, 20, and 30 μM was used for NAG, BG, and AlkP measurements, while a different gradient of 0, 2, 4, 8, 16, 24, 32, and 40 mM was used for LAP. Each concentration level was duplicated.
All the bioassays were incubated at 20°C for 2.5 h followed by the addition of 10 ml 1.0 M NaOH to each well and measured for 60 s in a fluorometer (BioTek Synergy H4, Winooski, VT, United States) at 365 nm excitation and 450 nm emission.
Statistical Analyses
Experimental data on soil physicochemical properties, microbial abundance, and enzyme activities (BG, NAG, LAP, and AlkP) were analyzed using a linear mixed-effect model (LMM) with a maximum-likelihood (ML) iterative algorithm in R version 3.6.1 (R Core Team, 2014). In this model, “soil” following shrub effects (Outside-canopy vs. Under-canopy) and “rainfall” (Low vs. High) of soil origin as well as their interaction were included as fixed factors. The identity of “site” of soil sampled (LH, FH, CJ, and MT) was included as a random factor. To meet the assumptions of normality and homogeneity of data residuals in the models, the data on enzyme activities and soil physicochemical properties were square-root-transformed and data on microbial abundance were logarithm-transformed. Data on ratios including GP/GN, C/N, C/P, and N/P were square-root-transformed. The model was run using lmer function in the “lme4” package (Bates et al., 2015), and the tests of significance were estimated using anova function in the “lmerTest” package (Kuznetsova et al., 2017).
Principal component analyses (PCA) were used to analyze soil microbial community composition and pattern of enzyme activities between the two soils (Outside-canopy vs. Under-canopy) and rainfall levels (Low vs. High). The PCAs were conducted based on a correlation similarity matrix. Grouped PLFA markers and the activity of individual enzyme were subjected to their respective PCA after scaling the unit variance. Redundancy analyses (RDA) were used to reveal the relationships among soil physicochemical properties, microbial community, and pattern of enzyme activities. Prior to the RDA, the Monte Carlo permutation tests (999 permutations) were used to select soil physicochemical variables that were significantly correlated with variations in microbial community and soil physicochemical and microbial variables that were correlated with variations in the pattern of enzyme activities. All multivariate analyses (PCA and RDA) were conducted in the “vegan” package (Oksanen et al., 2015). Pearson correlation analysis was used to examine the relationships between soil physicochemical properties and the abundance of soil microbial groups or enzyme activities.
Results
Soil Physicochemical Properties
Soil organic carbon (SOC) was significantly higher in under-canopy soil of A. gmelinii than in outside-canopy soil and also higher in soil collected from sites of high rainfall than in soil collected from sites of low rainfall. In contrast, bulk density (BD) was lower in soil under the canopy than in outside-canopy soil. Total phosphorus (TP) was overall higher in under-canopy soil, which was site-specific. Other soil physicochemical properties did not differ between outside- and under-canopy soils, regardless of rates of rainfall for these soils (Tables 1, 2).
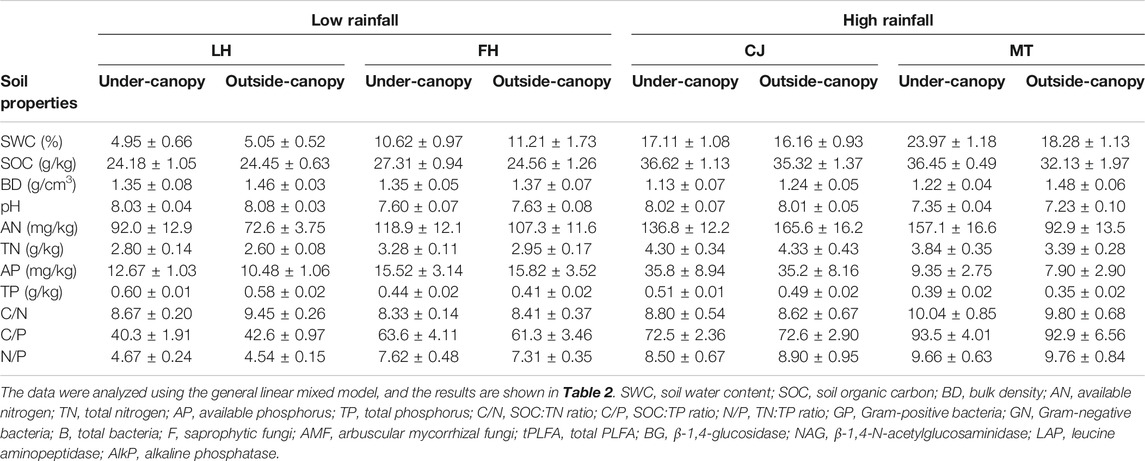
TABLE 1. Mean (±CI) of soil physiochemical properties in under-canopy and outside-canopy soils of shrub species Artemisia gmelinii collected from four sites (LH, FH, CJ, and MT) that varied in rates of rainfall at the basin of the Minjiang River Valley, SW China.
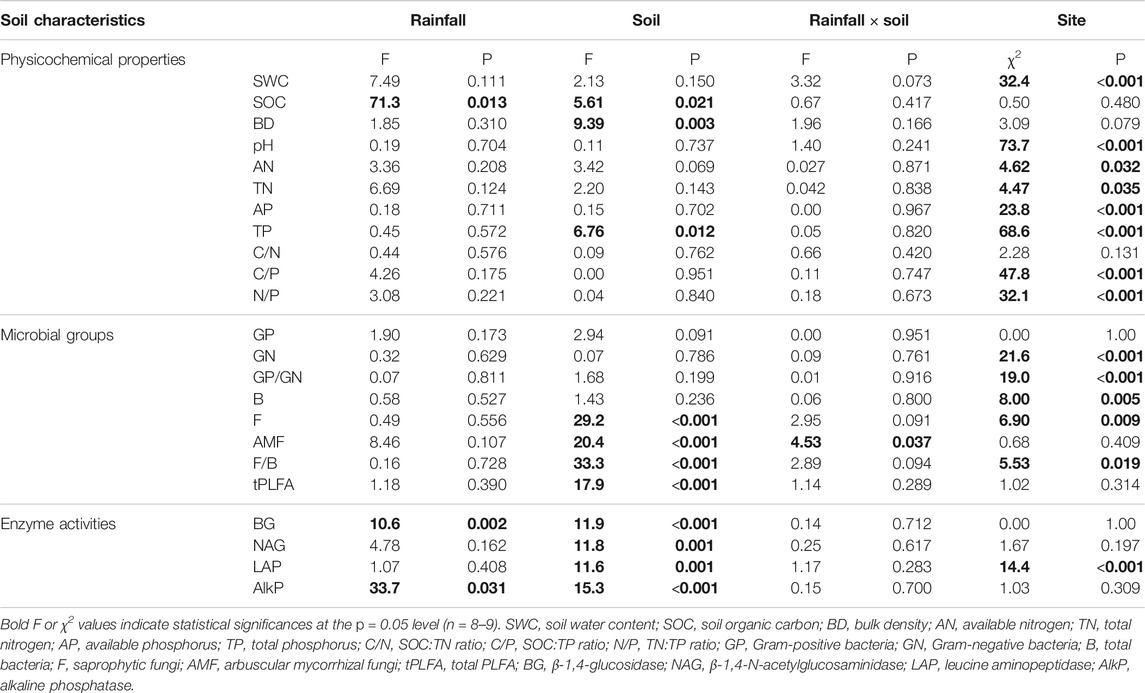
TABLE 2. Results for the general linear mixed model of soil physicochemical properties, microbial functional groups, and enzyme activities in soils from under- or outside-canopy of shrub species Artemisia gmelinii at sites of varying rates of rainfall in the Minjiang River Valley, SW China..
Soil Microbial Abundance and Enzyme Activities
Biomarkers for bacterial abundance (GP, GN, GP/GN, and B) did not differ between outside- and under-canopy soils of A. gmelinii, independent of rates of rainfall (Table 2; Figures 1A–D). Biomarkers for saprotrophic fungal abundance (F), its relative abundance to bacteria (F/B), and biomarker for arbuscular mycorrhizal fungi (AMF) and total soil microbial abundance (tPLFA) were higher in under-canopy soil than in outside-canopy soil (Table 2; Figures 1E–H). The biomarker for arbuscular mycorrhizal fungi (AMF) was also higher in under-canopy soil, but only when the soils were collected from sites of low rainfall (Table 2; Figure 1G).
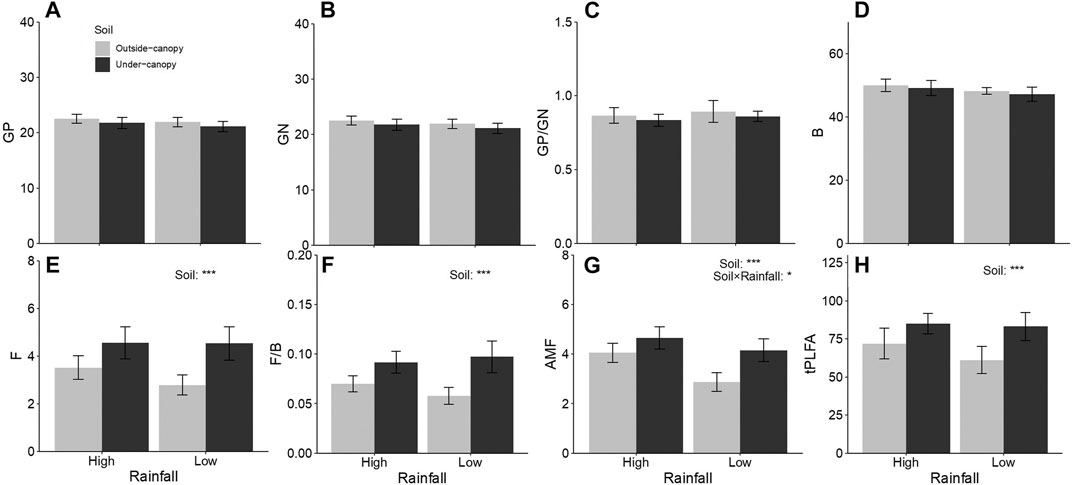
FIGURE 1. Difference (mean ± CI) in abundance of microbial groups indicated by PLFA (phospholipid fatty acids) biomarkers in soil under (Under-canopy, dark gray bars) and outside the canopy (Outside-canopy, light gray bars) of shrub species Artemisia gmelinii from sites of low and high rainfall. Total abundance of soil microbes (tPLFA) was expressed in nanomoles per gram of dry soils, and abundance of each microbial group was expressed as its percentage of the tPLFA. GP, Gram-positive bacteria; GN, Gram-negative bacteria; B, total bacteria; F, saprotrophic fungi; F/B, ratio of saprotrophic fungal to bacterial abundance and AMF, arbuscular mycorrhizal fungi. Statistical results are shown in Table 1.
All the investigated enzymes (BG, NAG, LAP, and AlkP) showed higher activities in soil under canopy of A. gmelinii than in outside-canopy soil (Table 2; Figures 2A–D). Among them, BG and AlkP activities were also higher in soil of sites from high rainfall than in soil from low rainfall (Table 2; Figures 2A,D).
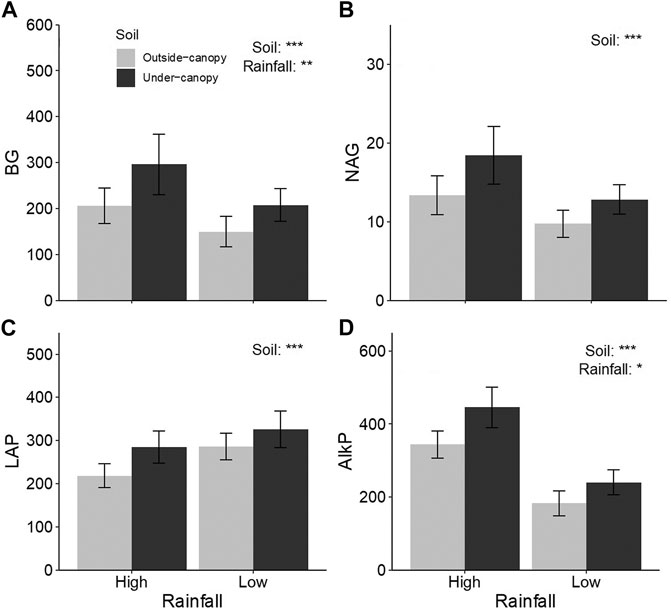
FIGURE 2. Difference (mean ± CI) in potential activities of extracellular enzymes (A) β-1,4-glucosidase (BG), (B) β-1,4-N-acetylglucosaminidase (NAG), (C) leucine aminopeptidase (LAP), and (D) alkaline phosphatase (AlkP) in soil under (Under-canopy, dark gray bars) and outside the canopy (Outside-canopy, light gray bars) of shrub species Artemisia gmelinii from sites of low and high rainfall. Statistical results are shown in Table 1.
Soil Microbial Community and Its Relation to Soil Physicochemical Properties
PCA results showed that the first three axes accounted for 39.5% (PC1), 26.6% (PC2), and 22.3% (PC3) of the total variation of microbial community, respectively (Figure 3A). Data on microbial communities were separated between outside- and under-canopy soils of shrub A. gmelinii, but only when the soils were collected from sites of low rainfall (Figures 3A,B). Results of redundancy analyses (RDA) showed that the first and second canonical axes accounted for 17.4 and 6.6% of the total variance in data on soil microbial community, respectively (Figure 3B), in which only RD1 significantly explained the data variance. Along RD1, biomarkers for GN, B, and AMF were positively correlated with SWC, and biomarker for saprophytic fungi (F and F/B) positively correlated with TP. The biomarkers for the majority of microbial groups, including GN, B, F, AMF, and tPLFA, were negatively correlated with BD (Table 3).
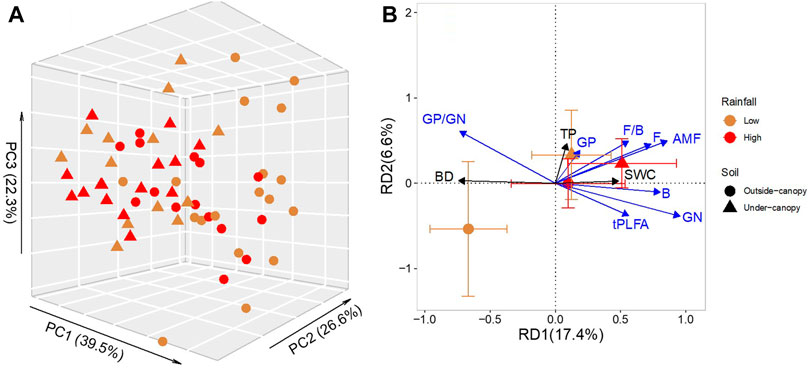
FIGURE 3. (A) 3-dimensional scatterplots for the principal component analysis (PCA) of microbial community composition and (B) biplot for the redundancy analysis (RDA) of relationships between the microbial community and physicochemical properties in soil under (Under-canopy, filled triangle) and outside the canopy (Outside-canopy, filled circle) of shrub species Artemisia gmelinii from sites of low (brown) and high rainfall (red). Percentage of data variation explained by each principal component is shown in its respective parentheses.
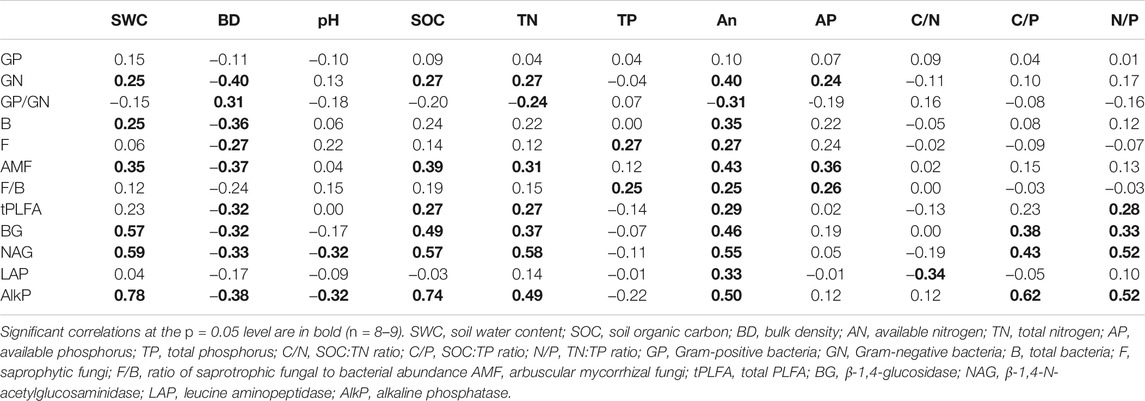
TABLE 3. Pearson correlation coefficient matrix between enzyme activities, microbial functional groups, and soil physicochemical properties in soils from under- or outside-canopy of shrub species Artemisia gmelinii at sites of varying rates of rainfall in the Minjiang River Valley, SW China.
Pattern of Enzyme Activities and Its Relation to Soil Physicochemical and Microbial Properties
The first two axes of PCAs accounted for 64.5 and 22.4% of the total variation of data on enzyme activities, respectively (Figure 4A). There was no clear separation of data on enzyme activities between outside- and under-canopy soils of A. gmelinii, but a significant separation was observed between soils from high and low rainfall. RDA results showed that the first two axes accounted for 49.0 and 12.7% of the total variation in the data, respectively, and both axes significantly explained the variation (Figure 4B). Along the axis RD1, activities of BG, NAG, and AlkP were positively correlated with SWC, SOC, C/P, and the soil N content (TN and AN) and negatively with soil BD. The activities of NAG and AlkP were also negatively correlated with soil pH (Table 3). Along the axis RD2, LAP activity was positively correlated with AN but negatively with C/N (Table 3; Figure 4B). Soil bacterial abundance slightly contributed to the overall pattern of enzyme activities, but it did not significantly correlate with the activities of any individual enzymes (Supplementary Table S1; Figure 4B).
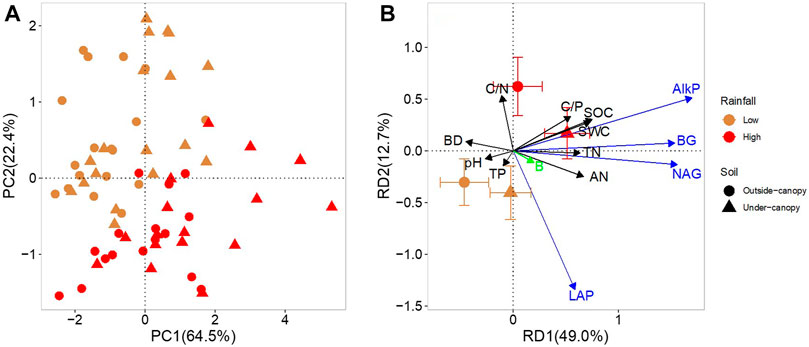
FIGURE 4. (A) Biplot for distribution pattern of enzyme activities and (B) the relationships between the pattern of enzyme activities and the physicochemical or microbial properties in soil under (Under-canopy, filled triangle) and outside the canopy (Outside-canopy, filled circle) of shrub species Artemisia gmelinii from sites of low (brown) and high rainfall (red). Shown are soil physicochemical or microbial properties that significantly explained data variation of enzyme activities. Black and green arrows represent soil physiochemical and microbial properties, respectively, and the blue arrows represent enzymatic parameters. Percentages of total variation of data explained by the first two axes are given in parentheses. BG, β-1,4-glucosidase (BG); NAG, β-1,4-N-acetylglucosaminidase; NAP, leucine aminopeptidase; and AlkP, alkaline phosphatase.
Discussion
Soil Physicochemical Properties due to Shrub Canopy and Varying Rainfall
Plants provide litter inputs or exudates to soil, often resulting in enhancement of soil organic matter and nutrient availability under the canopy (Yadav et al., 2008; Vallejo et al., 2012; Bright et al., 2017). In agreement with this hypothesis, shrub species Artemisia gmelinii indeed induced a higher SOC under the canopy in our study. TP was also higher in under-canopy soil, which is likely due to shrub roots that either penetrate into deep soils to lift phosphorus up to the soil surface or explore phosphorus stored in soil aggregation that eventually returns to soils as litter inputs (Rutigliano et al., 2004). Shrubs also improved soil physical quality as indicated by a lower BD, which might result from the accumulation of organic matter under the canopy (Chaudhari et al., 2013; Vannoppen et al., 2017) as increases in the organic carbon content usually enhance soil macroaggregate content and macroporosity, leading to a decrease in bulk density (Dunkerley and Brown 1995). It is noteworthy that higher SOC was also seen in the soil of high rainfall, and this is in consistent with the general pattern that chemical and biochemical processes in relation to soil carbon gain and loss are sensitive to change in precipitation (Li et al., 2021).
Soil Microbial Community and Its Relationship with Soil Properties
Shrubs can provide residues to soil in the form of root debris and rhizodeposited organic matters and improve soil physics via root expansion (Udawatta et al., 2009). Such shrub-induced increase in soil resource quality or quantity and favorable environment can selectively promote the growth of specific microbial groups under the canopy. In the current study, the abundance of saprophytic fungi (F) was enhanced, but that of soil bacteria was not influenced by A. gmelinii, resulting in a higher F/B ratio and total microbial abundance (tPLFA) in under-canopy soil than in outside-canopy soil. The variation of these microbial properties should result from the larger SOC in under-canopy soil since soil fungi and bacteria often differ in response to changing environmental conditions and fungi tended to utilize litter-derived C more than bacteria as food sources (Preusser et al., 2019). This is particularly true for saprophytic fungi that dominate in the decomposition of plant litter and soil organic matter (Zhou et al., 2017) and recalcitrant C fraction in SOC when compared to bacteria (Kramer and Gleixner 2008). The increase in total microbial abundance and relative abundance of fungi to bacteria in under-canopy soil indicates improved substrate quantity and system sustainability due to shrub canopy (de Vries et al., 2006). Interestingly, AMF abundance in soil was also enhanced by shrubs in our study, which, however, only occurred in sites of low rainfall. This result suggests that shrubs may promote the abundance AMF mainly by removing the constraints on AMF growth as a result of low rainfall, for example, SOC in this study.
Many soil properties are shown to influence microbial communities, such as organic matter content, nutrient availability, soil pH, or soil structure (DeForest et al., 2012; Prayogo et al., 2014). In the present study, the structure of microbial community was significantly driven by soil properties including SWC, TP, and BD. Further analyses suggested that the TP content was related to the abundance of saprotrophic fungi (F and F/B), which is in line with early studies reporting that saprotrophic fungi can break inorganic soil phosphates and release P from insoluble forms to soil for plant use (Ceci et al., 2018). SWC was positively correlated with the abundance of soil bacteria (B and GN), which is in agreement with other results that bacteria are more vulnerable and sensitive to drought than fungi as the latter can take advantage of their extended hyphae to explore water from water-filled micropores (Yuste et al., 2011). Compared to GN, it seemed GP was much less sensitive to soil moisture and thereby not related to SWC. This contradiction may be due to their stronger cell wall and more advanced osmoregulatory strategy than GN that confers GP higher tolerance to drought stress (Schimel et al., 2007). We were surprised to observe the positive correlation between AMF and SWC as AMF are typically drought-tolerant and not sensitive to change in SWC (Evans and Wallenstein 2012). Obviously, this relationship may be regulated by the shrubs that can provide more fresh carbon inputs in the form of residues in moist condition, which favors more abundant AMF in soil (Drenovsky et al., 2004). Soil BD is often used as an indicator of soil quality and has an influence on the growth of most soil microbes; therefore, it is certainly related to the abundance of the majority of soil microbial groups as many other studies showed (e.g., Rincon-Florez et al., 2016; Chen et al., 2020).
Shrub Effects on Enzyme Activities under Varying Rainfall
In our study, shrub species A. gmelinii enhanced activities of all measured soil hydrolytic enzymes under the canopy. This effect was consistent with the larger amount of SOC in under-canopy soil that provides more substrate for microbial use to synthesize enzymes (Yin et al., 2014; Kotroczó et al., 2014). The lower BD in under-canopy soil may also contribute to the higher enzyme activities by enhancing the diffusion of those substrates to enzymes in the soil (Mayor et al., 2016). In addition, BG and AlkP also showed higher activities in the soil of high rainfall, indicating a favorable soil environment for microbial metabolism following high rainfall (Xiao et al., 2018). Indeed, the larger amount of SOC under high rainfall can certainly induce higher BG activities to decompose cellulose and other carbohydrate polymers and provide sugars for microbial use (Ren et al., 2017). The rock-derived nutrient P can be more easily lost due to leaching or occlusion than atmospherically derived element N in weathered soils following rainfall events (Sinsabaugh et al., 2008), which may explain the higher activities of P-acquisition AlkP and the irresponsive activities of N-acquisition enzymes.
The pattern of enzyme activities can reflect microbial allocation to resource acquisition, indicating the metabolic and stoichiometric requirements of microbial communities in a soil (Mori 2020). In our study, the pattern of enzyme activities was not influenced by shrub canopy, suggesting that shrubs may provide organic substrates for the acquisition of targeting elements by all enzymes. On the other hand, separate clusters of data on enzyme activities in soils of high and low rainfall suggest higher sensitivity of enzyme activities to change in rainfall than in shrub canopy at semiarid systems. The redundancy analysis showed that this pattern was driven by the majority of soil physicochemical properties, including soil physical (SWC and BD), chemical traits (pH), and nutritional traits (SOC, TN, AN, C/N, TP, and C/P), which indicates a strong effect on enzyme activities (Acosta-Martinez et al., 2007; Shen et al., 2019). It is surprising to notice that the majority of these soil properties were mostly related to activities of BG, NAG, and AlkP, but only C/N related to LAP activities. This is possibly due to the difference of these enzymes in their sensitivity to environmental changes, in particular the difference of targeting substrates between LAP and NAG that are both N-acquisition enzymes (Zhao et al., 2016). In contrast to these soil physicochemical properties that have a profound contribution to the pattern of enzyme activities, microbial properties tended to play a relatively minor role (Ushio et al., 2010). These results showed that soil enzyme activities corresponded with abiotic factors more than with soil microbial community composition in our study (Kivlin and Treseder 2014; Yao et al., 2019), which suggests decoupling of potential enzyme activities from microbial biomass in soils under dry conditions (Geisseler et al., 2011).
Conclusion
The dominant shrub species A. gmelinii in semiarid lands, SW China, enhanced soil microbial abundance and drove soil microbial community toward fungal-dominant, by promoting organic carbon inputs and improving soil physicochemical properties under the canopy. Soil enzyme activities were also enhanced by the shrubs. The effects on both soil microbial community and enzyme activities due to shrubs varied with rates of rainfall, suggesting the dependence of vegetation on the local environment or climate in regulating soil biological activities. Our study suggests the necessity to take local environmental variation into account when conserving shrub vegetation to restore soil biological functions in semiarid lands.
Data Availability Statement
The raw data supporting the conclusion of this article will be made available by the authors, without undue reservation.
Author Contributions
LQ conceived the idea and wrote the manuscript, BW and XZ performed the study, and MW analyzed the data and wrote the manuscript.
Funding
This study was funded by the National Key Research and Development Program of China (no. 2017YFC0504802) and the National Natural Science Foundation of China (no. 31170581).
Conflict of Interest
The authors declare that the research was conducted in the absence of any commercial or financial relationships that could be construed as a potential conflict of interest.
Publisher’s Note
All claims expressed in this article are solely those of the authors and do not necessarily represent those of their affiliated organizations, or those of the publisher, the editors, and the reviewers. Any product that may be evaluated in this article, or claim that may be made by its manufacturer, is not guaranteed or endorsed by the publisher.
Acknowledgments
We thank the staff in Maoxian experimental station of Chinese Academy of Sciences for technical assistance in the field survey and two reviewer for comments on previous versions of the manuscript.
Supplementary Material
The Supplementary Material for this article can be found online at: https://www.frontiersin.org/articles/10.3389/fenvs.2021.725960/full#supplementary-material
References
Acosta-Martínez, V., Cruz, L., Sotomayor-Ramírez, D., and Pérez-Alegría, L. (2007). Enzyme Activities as Affected by Soil Properties and Land Use in a Tropical Watershed. Appl. Soil Ecol. 35, 35–45. doi:10.1016/j.apsoil.2006.05.012
Aislabie, J., Deslippe, J. R., and Dymond, J. R. (2013). “Soil Microbes and Their Contribution to Soil Services,” in Ecosystem Services in New Zealand: Conditions and Trends. Editor J. Dymond (Lincoln: Manaaki Whenua Press), 143–161.
Bardgett, R. D., Hobbs, P. J., and Frostegård, S. (1996). Changes in Soil Fungal:bacterial Biomass Ratios Following Reductions in the Intensity of Management of an upland Grassland. Biol. Fert Soils 22, 261–264. doi:10.1007/bf00382522
Bates, D., Mӓchler, M., Bolker, B., and Walker, S. (2015). Fitting Linear Mixed-Effects Models Using Lme4. J. Stat. Softw. 67, 1–48. doi:10.18637/jss.v067.i01
Bossio, D. A., and Scow, K. M. (1998). Impacts of Carbon and Flooding on Soil Microbial Communities: Phospholipid Fatty Acid Profiles and Substrate Utilization Patterns. Microb. Ecol. 35, 265–278. doi:10.1007/s002489900082
Bowles, T. M., Acosta-Martínez, V., Calderón, F., and Jackson, L. E. (2014). Soil Enzyme Activities, Microbial Communities, and Carbon and Nitrogen Availability in Organic Agroecosystems across an Intensively-Managed Agricultural Landscape. Soil Biol. Biochem. 68, 252–262. doi:10.1016/j.soilbio.2013.10.004
Bright, M. B. H., Diedhiou, I., Bayala, R., Assigbetse, K., Chapuis-Lardy, L., Ndour, Y., et al. (2017). Long-term Piliostigma Reticulatum Intercropping in the Sahel: Crop Productivity, Carbon Sequestration, Nutrient Cycling, and Soil Quality. Agric. Ecosyst. Environ. 242, 9–22. doi:10.1016/j.agee.2017.03.007
Brzostek, E. R., Greco, A., Drake, J. E., and Finzi, A. C. (2013). Root Carbon Inputs to the Rhizosphere Stimulate Extracellular Enzyme Activity and Increase Nitrogen Availability in Temperate forest Soils. Biogeochemistry 115, 65–76. doi:10.1007/s10533-012-9818-9
Ceci, A., Pinzari, F., Russo, F., Maggi, O., and Persiani, A. M. (2018). Saprotrophic Soil Fungi to Improve Phosphorus Solubilisation and Release: In Vitro Abilities of Several Species. Ambio 47, 30–40. doi:10.1007/s13280-017-0972-0
Chaudhari, P. R., Ahire, D. V., Ahire, V. D., Chkravarty, M., and Maity, S. (2013). Soil Bulk Density as Related to Soil Texture, Organic Matter Content and Available Total Nutrients of Coimbatore Soil. Int. J. Sci. Res. Publ. 3, 2250–3153.
Chen, L.-F., He, Z.-B., Zhao, W.-Z., Liu, J.-L., Zhou, H., Li, J., et al. (2020). Soil Structure and Nutrient Supply Drive Changes in Soil Microbial Communities during Conversion of virgin Desert Soil to Irrigated Cropland. Eur. J. Soil Sci. 71, 768–781. doi:10.1111/ejss.12901
Collins, S. L., Sinsabaugh, R. L., Crenshaw, C., Green, L., Porras-Alfaro, A., Stursova, M., et al. (2008). Pulse Dynamics and Microbial Processes in Aridland Ecosystems. J. Ecol. 96, 413–420. doi:10.1111/j.1365-2745.2008.01362.x
Conway, B. E. (1978). The Evaluation and Use of Properties of Individual Ions in Slution. J. Solution Chem. 7, 721–770. doi:10.1007/bf00643580
de Vries, F. T., Hoffland, E., van Eekeren, N., Brussaard, L., and Bloem, J. (2006). Fungal/bacterial Ratios in Grasslands with Contrasting Nitrogen Management. Soil Biol. Biochem. 38, 2092–2103. doi:10.1016/j.soilbio.2006.01.008
DeForest, J. L., Smemo, K. A., Burke, D. J., Elliott, H. L., and Becker, J. C. (2012). Soil Microbial Responses to Elevated Phosphorus and pH in Acidic Temperate Deciduous Forests. Biogeochemistry 109, 189–202. doi:10.1007/s10533-011-9619-6
Deng, L., Peng, C., Huang, C., Wang, K., Liu, Q., Liu, Y., et al. (2019). Drivers of Soil Microbial Metabolic Limitation Changes along a Vegetation Restoration Gradient on the Loess Plateau, China. Geoderma 353, 188–200. doi:10.1016/j.geoderma.2019.06.037
Dick, R. P., Breakwell, D. P., and Turco, R. F. (2015). Soil Enzyme Activities and Biodiversity Measurements as Integrative Microbiological Indicators. Methods Assessing Soil Qual., 247–271. doi:10.2136/sssaspecpub49.c15
Drenovsky, R. E., Vo, D., Graham, K. J., and Scow, K. M. (2004). Soil Water Content and Organic Carbon Availability Are Major Determinants of Soil Microbial Community Composition. Microb. Ecol. 48, 424–430. doi:10.1007/s00248-003-1063-2
Eisenreich, S. J., Bannerman, R. T., and Armstrong, D. E. (1975). A Simplified Phosphorus Analysis Technique. Environ. Lett. 9, 43–53. doi:10.1080/00139307509437455
Evans, S. E., and Wallenstein, M. D. (2012). Soil Microbial Community Response to Drying and Rewetting Stress: Does Historical Precipitation Regime Matter?. Biogeochemistry 109, 101–116. doi:10.1007/s10533-011-9638-3
Frostegård, Å., Bååth, E., and Tunlio, A. (1993). Shifts in the Structure of Soil Microbial Communities in Limed Forests as Revealed by Phospholipid Fatty Acid Analysis. Soil Biol. Biochem. 25, 723–730. doi:10.1016/0038-0717(93)90113-p
Geisseler, D., Horwath, W. R., and Scow, K. M. (2011). Soil Moisture and Plant Residue Addition Interact in Their Effect on Extracellular Enzyme Activity. Pedobiologia 54, 71–78. doi:10.1016/j.pedobi.2010.10.001
German, D. P., Weintraub, M. N., Grandy, A. S., Lauber, C. L., Rinkes, Z. L., and Allison, S. D. (2011). Optimization of Hydrolytic and Oxidative Enzyme Methods for Ecosystem Studies. Soil Biol. Biochem. 43, 1387–1397. doi:10.1016/j.soilbio.2011.03.017
Grandy, A. S., Salam, D. S., Wickings, K., McDaniel, M. D., Culman, S. W., and Snapp, S. S. (2013). Soil Respiration and Litter Decomposition Responses to Nitrogen Fertilization Rate in No-Till Corn Systems. Agric. Ecosyst. Environ. 179, 35–40. doi:10.1016/j.agee.2013.04.020
Gu, C., Mu, X., Gao, P., Zhao, G., Sun, W., Tatarko, J., et al. (2019). Influence of Vegetation Restoration on Soil Physical Properties in the Loess Plateau, China. J. Soils Sediments 19, 716–728. doi:10.1007/s11368-018-2083-3
Hortal, S., Bastida, F., Moreno, J. L., Armas, C., García, C., and Pugnaire, F. I. (2015). Benefactor and Allelopathic Shrub Species Have Different Effects on the Soil Microbial Community along an Environmental Severity Gradient. Soil Biol. Biochem. 88, 48–57. doi:10.1016/j.soilbio.2015.05.009
Hu, Z., Xu, C., McDowell, N. G., Johnson, D. J., Wang, M., Luo, Y., et al. (2017). Linking Microbial Community Composition to C Loss Rates during wood Decomposition. Soil Biol. Biochem. 104, 108–116.
Kivlin, S. N., and Treseder, K. K. (2014). Soil Extracellular Enzyme Activities Correspond with Abiotic Factors More Than Fungal Community Composition. Biogeochemistry 117, 23–37. doi:10.1016/j.soilbio.2016.10.017
Kotroczó, Z., Veres, Z., Fekete, I., Krakomperger, Z., Tóth, J. A., Lajtha, K., et al. (2014). Soil Enzyme Activity in Response to Long-Term Organic Matter Manipulation. Soil Biol. Biochem. 70, 237–243. doi:10.1016/j.soilbio.2013.12.028
Kramer, C., and Gleixner, G. (2008). Soil Organic Matter in Soil Depth Profiles: Distinct Carbon Preferences of Microbial Groups during Carbon Transformation. Soil Biol. Biochem. 40, 425–433. doi:10.1016/j.soilbio.2007.09.016
Kuznetsova, A., Brockhoff, P. B., and Christensen, R. H. B. (2017). LmerTest Package: Tests in Linear Mixed Effects Models. J. Stat. Softw. 82, 1–26. doi:10.18637/jss.v082.i13
Li, J., Zhou, X., Yan, J., Li, H., and He, J. (2015). Effects of Regenerating Vegetation on Soil Enzyme Activity and Microbial Structure in Reclaimed Soils on a Surface Coal Mine Site. Appl. Soil Ecol. 87, 56–62. doi:10.1016/j.apsoil.2014.11.010
Li, Y., Li, Z., Cui, S., Liang, G., and Zhang, Q. (2021). Microbial-derived Carbon Components Are Critical for Enhancing Soil Organic Carbon in No-Tillage Croplands: A Global Perspective. Soil Tillage Res. 205, 104758. doi:10.1016/j.still.2020.104758
Li, Y., Nie, C., Liu, Y., Du, W., and He, P. (2019). Soil Microbial Community Composition Closely Associates with Specific Enzyme Activities and Soil Carbon Chemistry in a Long-Term Nitrogen Fertilized Grassland. Sci. Total Environ. 654, 264–274. doi:10.1016/j.scitotenv.2018.11.031
Luo, S., Wang, S., Tian, L., Li, S., Li, X., Shen, Y., et al. (2017). Long-term Biochar Application Influences Soil Microbial Community and its Potential Roles in Semiarid farmland. Appl. Soil Ecol. 117-118, 10–15. doi:10.1016/j.apsoil.2017.04.024
Ma, W., Li, J., Gao, Y., Xing, F., Sun, S., Zhang, T., et al. (2020). Responses of Soil Extracellular Enzyme Activities and Microbial Community Properties to Interaction between Nitrogen Addition and Increased Precipitation in a Semi-arid Grassland Ecosystem. Sci. Total Environ. 703, 134691. doi:10.1016/j.scitotenv.2019.134691
Manzoni, S., Schaeffer, S. M., Katul, G., Porporato, A., and Schimel, J. P. (2014). A Theoretical Analysis of Microbial Eco-Physiological and Diffusion Limitations to Carbon Cycling in Drying Soils. Soil Biol. Biochem. 73, 69–83. doi:10.1016/j.soilbio.2014.02.008
Martinez, C. E., and Tabatabai, M. A. (1997). Decomposition of Biotechnology By-Products in Soils. J. Environ. Qual. 26, 625–632. doi:10.2134/jeq1997.00472425002600030006x
Mayor, Á. G., Goirán, S. B., Vallejo, V. R., and Bautista, S. (2016). Variation in Soil Enzyme Activity as a Function of Vegetation Amount, Type, and Spatial Structure in Fire-Prone Mediterranean Shrublands. Sci. Total Environ. 573, 1209–1216. doi:10.1016/j.scitotenv.2016.03.139
Mori, T. (2020). Does Ecoenzymatic Stoichiometry Really Determine Microbial Nutrient Limitations?. Soil Biol. Biochem. 146, 107816. doi:10.1016/j.soilbio.2020.107816
Nielsen, U. N., and Ball, B. A. (2015). Impacts of Altered Precipitation Regimes on Soil Communities and Biogeochemistry in Arid and Semi-arid Ecosystems. Glob. Change Biol. 21, 1407–1421. doi:10.1111/gcb.12789
Okin, G. S., Roberts, D. A., Murray, B., and Okin, W. J. (2001). Practical Limits on Hyperspectral Vegetation Discrimination in Arid and Semiarid Environments. Remote Sensing Environ. 77, 212–225. doi:10.1016/s0034-4257(01)00207-3
Oksanen, J., Blanchet, F. G., Kindt, R., Legendre, P., Minchin, P. R., O’Hara, R. B., et al. (2015). The Vegan Package. Community ecology package. R package version 2.5-7 Available at: https://github.com/vegandevs/vegan.
Ouyang, Y., and Li, X. (2020). Effect of Repeated Drying-Rewetting Cycles on Soil Extracellular Enzyme Activities and Microbial Community Composition in Arid and Semi-arid Ecosystems. Eur. J. Soil Biol. 98, 103187. doi:10.1016/j.ejsobi.2020.103187
Prayogo, C., Jones, J. E., Baeyens, J., and Bending, G. D. (2014). Impact of Biochar on Mineralisation of C and N from Soil and Willow Litter and its Relationship with Microbial Community Biomass and Structure. Biol. Fertil. Soils 50, 695–702. doi:10.1007/s00374-013-0884-5
Preusser, S., Poll, C., Marhan, S., Angst, G., Mueller, C. W., Bachmann, J., et al. (2019). Fungi and Bacteria Respond Differently to Changing Environmental Conditions within a Soil Profile. Soil Biol. Biochem. 137, 107543. doi:10.1016/j.soilbio.2019.107543
R Core Development Team (2014). R: A Language and Environment for Statistical Computing. Vienna, Austria: R Found. Stat. Comput. Available at: http//www.R-project.org/.
Ramírez, P. B., Fuentes-Alburquenque, S., Díez, B., Vargas, I., and Bonilla, C. A. (2020). Soil Microbial Community Responses to Labile Organic Carbon Fractions in Relation to Soil Type and Land Use along a Climate Gradient. Soil Biol. Biochem. 141, 107692. doi:10.1016/j.soilbio.2019.107692
Rath, K. M., Murphy, D. N., and Rousk, J. (2019). The Microbial Community Size, Structure, and Process Rates along Natural Gradients of Soil Salinity. Soil Biol. Biochem. 138, 107607. doi:10.1016/j.soilbio.2019.107607
Ren, C., Zhao, F., Shi, Z., Chen, J., Han, X., Yang, G., et al. (2017). Differential Responses of Soil Microbial Biomass and Carbon-Degrading Enzyme Activities to Altered Precipitation. Soil Biol. Biochem. 115, 1–10. doi:10.1016/j.soilbio.2017.08.002
Reynolds, J. F., Kemp, P. R., Ogle, K., and Fernández, R. J. (2004). Modifying the 'pulse-reserve' Paradigm for Deserts of North America: Precipitation Pulses, Soil Water, and Plant Responses. Oecologia 141, 194–210. doi:10.1007/s00442-004-1524-4
Rincon-Florez, V. A., Ng, C., Dang, Y. P., Schenk, P. M., and Carvalhais, L. C. (2016). Short-term Impact of an Occasional Tillage on Microbial Communities in a Vertosol after 43 Years of No-Tillage or Conventional Tillage. Eur. J. Soil Biol. 74, 32–38. doi:10.1016/j.ejsobi.2016.03.001
Rutigliano, F. A., D'Ascoli, R., and Virzo De Santo, A. (2004). Soil Microbial Metabolism and Nutrient Status in a Mediterranean Area as Affected by Plant Cover. Soil Biol. Biochem. 36, 1719–1729. doi:10.1016/j.soilbio.2004.04.029
Saiya-Cork, K. R., Sinsabaugh, R. L., and Zak, D. R. (2002). The Effects of Long Term Nitrogen Deposition on Extracellular Enzyme Activity in an Acer Saccharum forest Soil. Soil Biol. Biochem. 34, 1309–1315. doi:10.1016/s0038-0717(02)00074-3
Schimel, J., Balser, T. C., and Wallenstein, M. (2007). Microbial Stress-Response Physiology and its Implications for Ecosystem Function. Ecology 88, 1386–1394. doi:10.1890/06-0219
Shahbaz, M., Kuzyakov, Y., Sanaullah, M., Heitkamp, F., Zelenev, V., Kumar, A., et al. (2017). Microbial Decomposition of Soil Organic Matter Is Mediated by Quality and Quantity of Crop Residues: Mechanisms and Thresholds. Biol. Fertil. Soils 53, 287–301. doi:10.1007/s00374-016-1174-9
Shen, F., Wu, J., Fan, H., Liu, W., Guo, X., Duan, H., et al. (2019). Soil N/P and C/P Ratio Regulate the Responses of Soil Microbial Community Composition and Enzyme Activities in a Long-Term Nitrogen Loaded Chinese Fir forest. Plant Soil 436, 91–107. doi:10.1007/s11104-018-03912-y
Sims, J. R., and Haby, V. A. (1971). Simplified Colorimetric Determination of Soil Organic Matter. Soil Sci. 112, 137–141. doi:10.1097/00010694-197108000-00007
Sinsabaugh, R. L., Lauber, C. L., Weintraub, M. N., Ahmed, B., Allison, S. D., Crenshaw, C., et al. (2008). Stoichiometry of Soil Enzyme Activity at Global Scale. Ecol. Lett. 11, 1252–1264. doi:10.1111/j.1461-0248.2008.01245.x
Thapa, V. R., Ghimire, R., Acosta-Martínez, V., Marsalis, M. A., and Schipanski, M. E. (2021). Cover Crop Biomass and Species Composition Affect Soil Microbial Community Structure and Enzyme Activities in Semiarid Cropping Systems. Appl. Soil Ecol. 157, 103735. doi:10.1016/j.apsoil.2020.103735
Udawatta, R. P., Kremer, R. J., Garrett, H. E., and Anderson, S. H. (2009). Soil Enzyme Activities and Physical Properties in a Watershed Managed under Agroforestry and Row-Crop Systems. Agric. Ecosyst. Environ. 131, 98–104. doi:10.1016/j.agee.2008.06.001
Ushio, M., Kitayama, K., and Balser, T. C. (2010). Tree Species Effects on Soil Enzyme Activities through Effects on Soil Physicochemical and Microbial Properties in a Tropical Montane forest on Mt. Kinabalu, Borneo. Pedobiologia 53, 227–233. doi:10.1016/j.pedobi.2009.12.003
Ushio, M., Wagai, R., Balser, T. C., and Kitayama, K. (2008). Variations in the Soil Microbial Community Composition of a Tropical Montane forest Ecosystem: Does Tree Species Matter?. Soil Biol. Biochem. 40, 2699–2702. doi:10.1016/j.soilbio.2008.06.023
Vallejo, V. E., Arbeli, Z., Terán, W., Lorenz, N., Dick, R. P., and Roldan, F. (2012). Effect of Land Management and Prosopis Juliflora (Sw.) DC Trees on Soil Microbial Community and Enzymatic Activities in Intensive Silvopastoral Systems of Colombia. Agric. Ecosyst. Environ. 150, 139–148. doi:10.1016/j.agee.2012.01.022
Vannoppen, W., De Baets, S., Keeble, J., Dong, Y., and Poesen, J. (2017). How Do Root and Soil Characteristics Affect the Erosion-Reducing Potential of Plant Species?. Ecol. Eng. 109, 186–195. doi:10.1016/j.ecoleng.2017.08.001
Wang, L., Pang, X., Li, N., Qi, K., Huang, J., and Yin, C. (2020). Effects of Vegetation Type, fine and Coarse Roots on Soil Microbial Communities and Enzyme Activities in Eastern Tibetan Plateau. Catena 194, 104694. doi:10.1016/j.catena.2020.104694
Watanabe, F. S., and Olsen, S. R. (1965). Test of an Ascorbic Acid Method for Determining Phosphorus in Water and NaHCO3 Extracts from Soil. Soil Sci. Soc. America J. 29, 677–678. doi:10.2136/sssaj1965.03615995002900060025x
Xiao, W., Chen, X., Jing, X., and Zhu, B. (2018). A Meta-Analysis of Soil Extracellular Enzyme Activities in Response to Global Change. Soil Biol. Biochem. 123, 21–32. doi:10.1016/j.soilbio.2018.05.001
Xu, X., Ma, K., Fu, B., Song, C., and Liu, W. (2008). Relationships Between Vegetation and Soil and Topography in a Dry Warm River Valley, SW China. Catena. 75, 138–145. doi:10.1016/j.catena.2008.04.016
Xu, Z., Yu, G., Zhang, X., Ge, J., He, N., Wang, Q., et al. (2014). The Variations in Soil Microbial Communities, Enzyme Activities and Their Relationships with Soil Organic Matter Decomposition along the Northern Slope of Changbai Mountain. Appl. Soil Ecol. 86, 19–29.
Yadav, R. S., Yadav, B. L., and Chhipa, B. R. (2008). Litter Dynamics and Soil Properties under Different Tree Species in a Semi-arid Region of Rajasthan, India. Agroforest Syst. 73, 1–12. doi:10.1007/s10457-008-9106-9
Yao, Y., Shao, M., Fu, X., Wang, X., and Wei, X. (2019). Effects of Shrubs on Soil Nutrients and Enzymatic Activities over a 0-100 Cm Soil Profile in the Desert-Loess Transition Zone. Catena 174, 362–370. doi:10.1016/j.catena.2018.11.031
Yin, R., Deng, H., Wang, H.-l., and Zhang, B. (2014). Vegetation Type Affects Soil Enzyme Activities and Microbial Functional Diversity Following Re-vegetation of a Severely Eroded Red Soil in Sub-tropical China. Catena 115, 96–103. doi:10.1016/j.catena.2013.11.015
Yu, Y., Wei, W., Chen, L., Feng, T., and Daryanto, S. (2019). Quantifying the Effects of Precipitation, Vegetation, and Land Preparation Techniques on Runoff and Soil Erosion in a Loess Watershed of China. Sci. Total Environ. 652, 755–764. doi:10.1016/j.scitotenv.2018.10.255
Yuste, J. C., Peñuelas, J., Estiarte, M., Garcia-Mas, J., Mattana, S., Ogaya, R., et al. (2011). Drought-resistant Fungi Control Soil Organic Matter Decomposition and its Response to Temperature. Glob. Chang. Biol. 17, 1475–1486. doi:10.1111/j.1365-2486.2010.02300.x
Zhao, C., Long, J., Liao, H., Zheng, C., Li, J., Liu, L., et al. (2019). Dynamics of Soil Microbial Communities Following Vegetation Succession in a Karst Mountain Ecosystem, Southwest China. Sci. Rep. 9. doi:10.1038/s41598-018-36886-z
Zhao, C., Miao, Y., Yu, C., Zhu, L., Wang, F., Jiang, L., et al. (2016). Soil Microbial Community Composition and Respiration along an Experimental Precipitation Gradient in a Semiarid Steppe. Sci. Rep. 6. doi:10.1038/srep24317
Zhou, X., Chen, C., Wang, Y., Xu, Z., Han, H., Li, L., et al. (2013). Warming and Increased Precipitation Have Differential Effects on Soil Extracellular Enzyme Activities in a Temperate Grassland. Sci. Total Environ. 444, 552–558. doi:10.1016/j.scitotenv.2012.12.023
Keywords: semiarid, shrub canopy, Artemisia gmelinii, soil microbial communities, enzyme activities
Citation: Qu L, Wang B, Zhang X and Wang M (2021) Responses of Soil Microbial Community and Enzyme Activities to Shrub Species Artemisia gmelinii in Relation to Varying Rainfall in a Semiarid Land, SW China. Front. Environ. Sci. 9:725960. doi: 10.3389/fenvs.2021.725960
Received: 16 June 2021; Accepted: 28 July 2021;
Published: 15 September 2021.
Edited by:
Rosa Francaviglia, Council for Agricultural and Economics Research (CREA), ItalyReviewed by:
Shikha Singh, Oregon State University, United StatesLois S. Taylor, The University of Tennessee, Knoxville, United States
Copyright © 2021 Qu, Wang, Zhang and Wang. This is an open-access article distributed under the terms of the Creative Commons Attribution License (CC BY). The use, distribution or reproduction in other forums is permitted, provided the original author(s) and the copyright owner(s) are credited and that the original publication in this journal is cited, in accordance with accepted academic practice. No use, distribution or reproduction is permitted which does not comply with these terms.
*Correspondence: Laiye Qu, bHlxdUByY2Vlcy5hYy5jbg==; Minggang Wang, bWluZ2dhbmd3YW5nQGJqZnUuZWR1LmNu