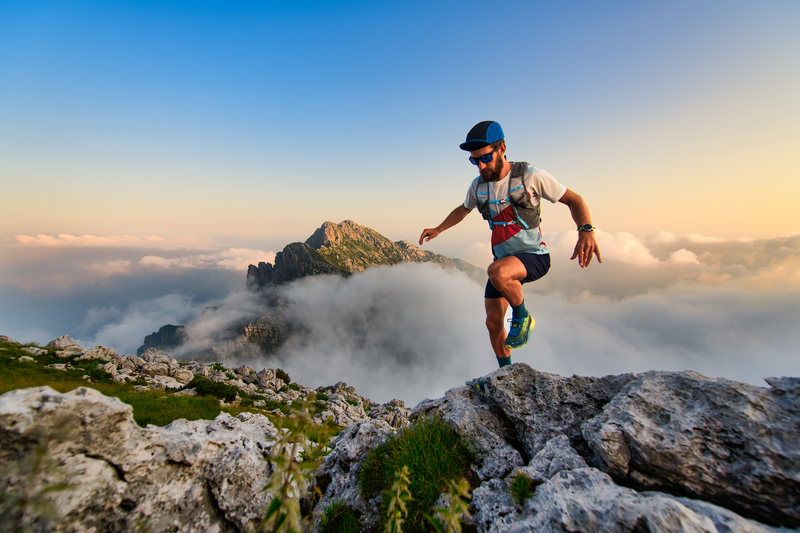
95% of researchers rate our articles as excellent or good
Learn more about the work of our research integrity team to safeguard the quality of each article we publish.
Find out more
ORIGINAL RESEARCH article
Front. Environ. Sci. , 07 July 2021
Sec. Environmental Economics and Management
Volume 9 - 2021 | https://doi.org/10.3389/fenvs.2021.717737
This article is part of the Research Topic Green Finance, Renewable and Non-Renewable Energy, and COVID-19 View all 26 articles
Reducing energy intensity is conducive to the sustainable use of non-renewable fossil energy, and is also one of the main strategies to deal with climate change and environmental degradation. The effect of national macro-level factors on energy intensity has been basically confirmed, but the effect of regional low-carbon policy remains to be investigated. Based on this, our analysis exploits China’s “low-carbon city pilot” policy as a quasi-natural experiment and conducts the difference-in-difference resign. We collect the panel data of 271 cities in China from 2006 to 2016. The empirical results show that: first, the low-carbon city pilot policy can effectively reduce the energy intensity. Second, there exist heterogeneous effects on energy intensity among different cities, and the inhibition effects in eastern cities, high economic development cites, and non-old industrial-base cities are more significant. Third, the policy mainly affects regional energy intensity through technological innovation rather than industrial structure optimization mechanism.
Energy intensity relates to the sustainable utilization of non-renewable resources such as fossil energy. Reducing energy intensity is also one of the main strategies to deal with current problems of climate change and environmental degradation. As the main habitat of human production and life, cities are also the main places of carbon emissions. According to the BP statistical review of world energy in 2020, China has become the largest energy consumer, whose energy consumption accounts for 24.5% of the total global consumption in 2019, but its energy efficiency is still low, though always improving(Liang et al., 2020). Moreover, China’s energy intensity is unbalanced among different regions that the energy intensity in the eastern coastal areas is far lower than that in the central and western regions (Jiang et al., 2017). Although Chinese policy makers have always attached great importance to reducing energy intensity, China’s energy intensity has been increased in the process of rapid urbanization (Yan, 2015).
Most scholars mainly study the determinants of energy intensity from the national macro level, which includes factors affecting energy demand (per capita income, green technology innovation, etc.), energy supply (energy tax, etc.) and the energy price factor. For example, Filipović et al. (2015) and Samargandi (2019) have examined the determinants of energy intensity in EU countries and OPEC countries, respectively, and concluded that energy price, energy tax and per capita GDP can affect energy intensity. Wurlod and Noailly (2018) analyzed the impact of green technology innovation on energy intensity in 17 OECD countries, and concluded that green technology innovation leads to the reduction of energy intensity in most industries. Canh et al. (2020) used the data of 81 countries and examine the heterogeneous impacts of financial development on the energy intensity among countries with different incomes. Bashir et al. (2021) examined the impact of environmental protection tax on energy consumption and energy intensity in OECD countries.
As far as we know, few scholars have studied the determinants of energy intensity from the local micro level, especially from the city level. Yuxiang and Chen (2010) used China’s provincial panel data and concluded that the increase of Chinese government consumption expenditure has significantly improved the energy intensity. In China, the mayor (the chief executive for the city) is the first person responsible for the quality of urban environmental, and also possess the power to formulate environmental policies. Therefore, it is more meaningful to study the impact of policies on energy intensity from the perspective of the cities. Unfortunately, this research is still relatively rare except for the researches of Ma and Yu (2017) and Zhang et al. (2020). Ma and Yu (2017) found that changes in industrial structure (including the increase in the proportion of state-owned enterprises and small-scale enterprises) can reduce urban energy intensity. Zhang et al. (2020a) found that local government environmental regulations can mitigate the financing-energy relationship and reduce energy intensity. Therefore, differ from their researches, we attempt to evaluate the effect of local government policies on energy intensity from the city level.
The “low-carbon zone” policy is a major measure for countries or regions to achieve environmental goal and deal with the global warming crisis. Since 1990, many countries have reached consensus on international agreements of low-carbon development. In 2003, the British government issued the energy white paper “our future energy: creating a low carbon economy” (DTI, 2003). The UN started international climate negotiations in 1990 and signed the Kyoto Protocol in February 2005. In 2007, Japan also put forward the concept of low-carbon society, and pointed out that “without a low-carbon society, there would be no low-carbon economy”. Then there are a few scholars who start to quantitatively evaluate the effect of the “low-carbon zone” policy. Difference-in-difference (hereafter called DID) method is a most frequently used method for policy evaluation. For example, Wolff (2014) used the DID method to examine the impact of European low-carbon area policy. Gehrsitz (2017) used the DID method to evaluate the impact of Germany’s low-carbon zone policy on air quality and infant mortality. Their researchers concluded that the low-carbon zone policy has significantly improved local air quality and reduced infant mortality. China also has carried out a kind of low-carbon zone policy called low-carbon city pilot policy. In August 2010 and December 2012, China’s national development and Reform Commission (NDRC) issued two batches of low-carbon city pilot notices, requiring the pilot cities to combine the adjustment of industrial structure with the optimization of energy structure and energy efficiency, reduce carbon intensity and promote green development. This pilot are considered an inevitable choice for China (Yang and Li, 2013), whose actual effect remains to be verified. This constitutes the research goal of our study.
Our study makes several contributions to exist literature. Firstly, we try to evaluate the impact of “low-carbon city pilot” policy on energy intensity. Previous researchers have found that low-carbon zone policy can effectively improve air quality, but its impact on energy intensity is still to be verified. We use China’s low-carbon city pilot policy as a quasi-natural experiment and evaluate its effects on energy intensity. In particular, most of the literature about energy intensity are transnational and focus on developed countries. There are few studies focusing on the regional energy intensity within a country. Therefore, it is innovative to use the samples of Chinese cities and conduct an empirical research on cities’ energy intensity. The results show that China’s low-carbon city pilot policy has reduced energy intensity remarkably. Secondly, we try to study the heterogeneous impacts of “low-carbon city pilot” policy. As an emerging market country, China’s development stage is different from that of developed countries. Low-carbon regional policy may show heterogeneity in China. Especially, China’s regional economic development is unbalanced, the per capita GDP of the eastern developed cities has reached the level of developed countries, while the central and western regions are still at the level of developing countries. Cities in different stages of economic development may exhibit different energy intensity. The empirical results show that: low carbon city pilot policy has a more significant inhibitory effect on the energy intensity of Eastern, high economic development and non-heavy industrial cities. Finally, we try to explore the mechanism of “low-carbon city pilot” policy affecting energy intensity. Relevant empirical studies show that green technology innovation and industrial structure optimization can reduce energy intensity. However, whether low-carbon city policy can affect energy intensity through green technology innovation or industrial structure optimization has not been demonstrated. Therefore, we conduct a mediating effect model to test this mechanism. Our results show that the “low-carbon city pilot” policy can reduce the energy intensity by promoting green technology innovation rather than industrial structure optimization.
The rest of our paper is organized as follows: In Econometrical Tests on the Effect of the “Low-Carbon City Pilot” Policy on Energy Intensity we conduct the econometric tests on the effect of the “low-carbon city pilot” policy on energy intensity, which mainly includes model design, variables and data and empirical analysis. In The Heterogeneous Impact of “Low-Carbon City Pilot” Policy on Energy Intensity is the heterogeneous impact of “low-carbon city pilot” policy on energy intensity. In Robust Tests we test the robustness of the previous research conclusions. In Further Analysis: Impact Mechanism we further explore the impact mechanism of “low-carbon city pilot” policy on energy intensity. And Conclusion concludes the paper.
Different levels of environmental regulation have heterogeneous effects on regional energy intensity (Hou et al., 2018). When the level of environmental regulation is low, enterprises tend to reduce pollutant emissions and increase the “end treatment” investment such as sewage and waste gas treatment equipment (Jorgenson and Wilcoxen, 1990; Nur Utomo et al., 2020). At this time, “compliance cost effect” plays a leading role, leading to a positive correlation between environmental regulation and energy intensity. With the continuous optimization and increasing intensity of environmental regulation, enterprises will be forced to pay attention to “prior prevention”. For example, they will increase the investment in energy conservation and energy efficiency (Porter and Linde, 1995; Hong et al., 2020). At this time, the “innovation compensation effect” plays a leading role, leading to a negative correlation between environmental regulation and energy intensity. Therefore, the relationship between environmental regulation and energy intensity depends on the size of “compliance cost effect” and “innovation compensation effect”.
Some scholars have evaluated the effect of “low-carbon zone” policy. They think that low-carbon technology plays an increasingly significant role in the process of economic development. In practice, developed cities all over the world regard the construction of low-carbon city policy as an important way to improve competitiveness (Hamamoto, 2006). And most of them affirmed its positive role. For example, Cheng et al. (2019) find that “low-carbon city pilot” policy has a positive effect on regional green economic development. According to the notices of China’s “low-carbon city pilot” policy, the core of this policy lies in: faced with a growing energy demand, we need to strive to construct an industrial system and consumption mode characterized by low-carbon emissions while developing economy and improving people’s livelihood. Therefore, we believe that as an environmental regulation measurement, China’s “low-carbon city pilot” policy can force enterprises to save energy and reduce energy intensity, and its “innovation compensation effect” is more prominent than “compliance cost effect”. In short, China’s “low-carbon city pilot” policy is negatively correlated with energy intensity.
In order to explore the development of economy, realize energy conservation and emission reduction, and promote green development, Chinese government has issued a series of policies and requirements to promote energy conservation and emission reduction. Among them, the most important one is the “low-carbon city pilot” policy. In 2010, NDRC started the first batch of low-carbon city pilot policy, and started the second and third batch of low-carbon pilot policy in 2012 and 2017, respectively. In July 2010, NDRC selected eight cities as the first batch of low-carbon pilot cities. In December 2012, NDRC selected 28 cities (districts and counties) as low-carbon pilot cities. In 2017, the number of low-carbon pilot cities reached a climax, and a total of 45 cities (districts and counties) were selected as low-carbon pilot cities. Figure 1 plots a map of the two batch of low-carbon pilot cities in China.
From Figure 1, we can find that the distribution of these two batch of low-carbon pilot cities is relatively scattered from the west to the east, from the north to the south in China. They not only include the developed coastal cities, but also include the under-developed western cities.
In order to solve the endogeneity problem in literature, we use this pilot policy as a “natural experiment” to construct a DID model. This pilot policy is relatively exogenous and not easily affected by other factors. Therefore, the endogenous problems can be largely avoided. Using the DID research design, we compare the change in energy intensity among low-carbon pilot cities with the change among non-low-carbon pilot cities. The specific model is as follows:
In Eq. 1, the subscipt
In addition, we introduce other control variables according to the relevant theories: 1) the economic development. It is generally believed that countries with higher economic development often exhibit lower energy intensity. This is because the industrial competition in developed countries is more intense, and their R&D department is more higher developed, which makes the energy intensity decrease more (Wu, 2012; Filipović et al., 2015; Li and Liao, 2020). Of course, there are some scholars who believe that the relationship between economic development and energy intensity is uncertain. On the one hand, higher income will increase energy demand and then thus energy intensity; On the other hand, different incomes reflects different development stage, and higher income is often accompanied by the improvement of energy efficiency, which will reduce energy intensity (Jimenez and Mercado, 2014).2) Industrial structure. China’s economy has experienced high speed development, followed by the optimization of industrial structure(Li et al., 2020). Luan et al. (2021) found that when the proportion of tertiary industry increase 1%, energy intensity decrease by about 0.03%. Li and Lin (2014) denoted industrial structure by the proportion of secondary industry, and conclude that there is a non-linear (rather than simply promoting or restraining) relationship between industrial structure and energy intensity. 3) Population density. It is generally believed that higher population density will increase the energy demand, and thus the energy intensity (Sadorsky, 2013; Rafiq et al., 2016). 4) Foreign Direct investment (FDI). Scholars believe that the entry of FDI can bring advanced management experience, corporate management system and business philosophy, which will be helpful to the decrease of energy intensity in host countries (Li et al., 2019; Cao et al., 2020; Zhong and Li, 2020). 5) City dummy variable. It is used to eliminate the influence of missing invariable variables such as city characteristic. 6) Time dummy variable. It is used to exclude the impact of macroeconomic events (such as the 2008 financial crisis, etc.).
Referring to the common method of scholars such as Chang (2014), Lin and Wang (2021) and so on, we measure the energy intensity by energy consumption over GRP. And from the perspective of Chinese cities, the data of the energy use over GRP is more displayed by the change rate, so we use the reduction rate of energy consumption over GRP to indicate the energy intensity. In the control variables, the economic development is expressed in terms of per capita GRP. The industrial structure is denoted by the proportion of second and tertiary industries in GRP, the population density is denoted by the number of permanent residents per unit area, and foreign direct investment is denoted by the actually utilized foreign capital which is converted into RMB according to the average exchange rate in the year.
Table 1 shows the variable measurement and data sources. Due to the data availability and comparability, we mainly collected panel data of 271 cities from 2006 to 2016. The comparability refers to that since low-carbon pilot policy has been spread to lots of cities, there would be not enough samples as the control group if the samples in 2017 were included.” But it may be not clearly expounded. So we add in the paper that “Due to the issue of comparability, we mainly want to examine the impact of the first batch (July 2010) and the second batch (November 26, 2012) of “low-carbon city pilot” with a relatively close time, but not the impact of the third batch (2017). There are two specific reasons: first, in 2017, the lack of data in some regions is more serious after 2018. Second, the third batch of “low-carbon city pilot” are carried out on a large scale. After 2017, most cities have carried out “low-carbon city pilot”, so there are not enough reference objects for the comparative analysis of difference-in-difference. The data used in our study are mainly from the statistical yearbook. Specially, the data of energy intensity and economic development are mainly from statistical yearbooks of provinces and cities. The data of industrial structure, population density and actual utilization of foreign capital are from China’s urban statistical yearbook, and the average exchange rate of the year is from China’s statistical yearbook.
Before the empirical analysis, we need to conduct descriptive statistical analysis on all variables, and the results are shown in Table 2.
It can be seen from Table 2 that there are 271 sample enterprises in this paper, and the reduction rate of energy intensity is between −10.34 and 4.13%. The proportion of the secondary industry and the tertiary industry in the GRP changes greatly. The proportion of the secondary industry ranges from 14.95 to 85.64, and the proportion of the tertiary industry ranges from 11.22 to 80.23, which indicates that there are great differences in the industrial structure among different cities in China. The minimum value of per capita GRP is 5.4 and the maximum value is 12.28, which indicates that the per capita income of Chinese cities with better economic development has reached the level of high-income countries or regions, while the per capita income of Chinese cities with poor economic development is still at the level of low-income countries or regions, which may lead to the heterogeneous impact of low-carbon city pilot on the energy intensity of these different regions. In addition, we also find that there are great differences between the actual level of foreign investment and population density in different regions.
Before testing the impact of low-carbon city pilot on energy intensity, we need to examine the degree of correlation between various variables to avoid multicollinearity in parameter estimation. Based on this, this paper calculates the correlation coefficient between various variables as shown in Table 3. Table 3 is the Pearson correlation coefficient matrix, which shows the correlation among the variables in the model. Among them, the energy intensity has a significant negative correlation with the proportion of the secondary industry and the level of economic development. But in the whole there is no high correlation between the variables. Therefore, we think that there is no serious multicollinearity among the variables, so we can directly conduct the ordinary least squares regression.
Furthermore, we make parameter estimation on the impact of low-carbon city pilot on energy intensity, and the results of parameter estimation are shown in Table 4. It can be seen from Table 4 that the “low-carbon city pilot” policy can reduce the energy intensity. Specifically, in column 1–3 the coefficients of the interaction items are from −0.4177 to −0.4659, and pass the 5% significance level test. It is shown that the pilot policy has inhibitory effect on energy consumption per unit of GRP, which confirms the innovation compensation effect of the pilot policy. In addition, in column 2–3 the coefficients of the regional economic development (lnpgrp) are significantly negative, indicating that with the regional economic development, energy efficiency will be improved and the energy intensity reduced. The coefficients of the variables such as the proportion of the secondary industry and the proportion of the tertiary industry are both significantly in column 2–3. According to the theory of industrial economics, the increase of the proportion of the secondary and tertiary industries reflects the general law of industrial structure evolution, which will bring about the improvement of energy efficiency. This is consistent with our empirical conclusion. The coefficient of FDI are significantly negative, indicating that the higher the foreign investment, the energy intensity is lower. This is due to that the entry of foreign direct investment can bring advanced management experience, enterprise management system and business philosophy, which will improve the energy efficiency in China, and then reduce the energy intensity. The coefficient of the population density fails to pass the statistical test. It may be due to that after controlling variables such as the economic development, the population density will not increase the energy demand and improve the energy intensity.
There are differences in energy intensity among cities with different geographic locations, economic development, and characters such as whether old industrial bases are or not. So we further distinguish cities according to geographic locations, regional economic development, and whether they are old industrial bases or not, and analyze the heterogeneous effects of “low-carbon city pilot” policy on energy intensity.
Since the reform and opening up, economic gaps between the eastern regions, the central regions and the western regions in China have always existed and never narrowed. The pace of development in the eastern region continues to accelerate, while the development of central and western regions are restricted by related factors such as backward infrastructure, imperfect market economy system, and backward education development, thus the economic development gap between the East and the west is widening. Generally speaking, the level of China’s economic and technological development gradually decreases from east to west, while the abundance of natural resources increases from east to west. Objectively, there are three major economic regions such as the east, middle and west. Therefore, we refer to the research of (Elliott et al., 2013; Zhang et al., 2020) and divide China’s cities into eastern, central and western regions according to their geographic location. Among them, the eastern region mainly includes Beijing, Tianjin, Jiangsu, Zhejiang, Fujian, Liaoning, Shandong, Guangdong, Hainan, and Hebei. The central region includes Shanxi, Inner Mongolia, Jilin, Hubei, Hunan, Anhui, Heilongjiang, Jilin, and Jiangxi. The western region includes Sichuan, Guizhou, Yunnan, Tibet, Shaanxi, Gansu, Qinghai, Ningxia, Xinjiang, and Guangxi. Then we conduct a regression analysis on sub-samples. The results are shown in Table 5.
TABLE 5. The heterogeneous impacts of the pilot policy on the energy intensity in different geographical locations.
According to Table 5, the “low-carbon city pilot” policy have a heterogeneous impact on the energy intensity of different regions, and its impact on the energy intensity of the eastern region is more significant. Specifically, the coefficients of the interaction terms in column 1 are negative and pass the 1% significance test, while the corresponding coefficients in column 2 and 3 are not significant, indicating that after controlling other regional factors, the “low-carbon city pilot” policy can significantly reduce the energy intensity in eastern regions, but has no significant impact on the energy intensity in central and western regions. This may be due to the fact that China’s eastern regions have better technical foundation and more talents which are the basis for technology innovation. Specifically, China’s eastern regions have superior geographical conditions, agricultural intensification, industrialization and commercialization relative to the central and western regions (Zheng et al., 2020), so they have obvious advantages in attracting talent and foreign investment and technological innovation. Therefore, the low-carbon city pilot policy is easier to promote the eastern regions to increase investment in energy conservation and play an innovative compensation effect to reduce energy intensity.
Furthermore, we divide the samples into regions with high economic development and regions with medium and low economic development level according to the median of the per capita GRP of the region, and conduct sub-sample regression analysis. At the same time, considering the correspondence to different geographical locations in China, we also use the tertiles of per capita GRP and divide the samples into regions with high economic development, medium economic development, and low economic development. Then we conduct a sub-sample regression analysis. The results are shown in Table 6.
TABLE 6. The heterogonous impact of “low-carbon city pilot” policy on the energy intensity in regions with different economic development.
The analysis in Table 6 shows that, the “low-carbon city pilot” policy has heterogeneous impacts on the energy intensity of the regions with different economic development, and the impacts of the regions with high economic development and medium economic development are significantly negative, while those of the regions with low economic development are not significant. Specially, from Table 6, it is shown that the coefficients of the interaction terms in column 1, 3, and 4 are all negative, and pass the significance test of 5, 10 and 1%, respectively, while those in column 2 and 5 are insignificant. This further confirms previous conclusions that with fiercer industrial competition and higher professional R&D departments, enterprises in economically developed regions are able to respond quickly once policies introduced, which in turn bring down energy intensity even more. Admittedly, this will make the problem worse. Because in fact, compared with energy intensity in the regions with low economic development, those in middle and high economic development regions are generally lower. And the effect of the “low-carbon city pilot” policy is more significant in middle and high economic development regions. The result will worsen with larger differences of energy intensity between the medium-high economic development regions and the low economic development regions.
Finally, we also analyze the heterogeneous impacts of the policy between the samples according to whether they are old industrial-base areas. Specifically, according to the National Development and Reform Commission’s “National Old Industrial Base Adjustment and Transformation Plan (2013-2022)” issued in 2013, we identify 120 old industrial base cities or provincial capital cities. Among them, some of the old industrial-bases are important national energy bases, and usually undertake major technical equipment or the supply of products related to the national economy and people’s livelihood. On the whole, the old industrial-bases have the characteristics of high energy consumption and high pollution, and often exhibit higher energy intensity. Therefore, our concern is whether the low-carbon city pilot policy can restrain the energy intensity of the old industrial bases and achieve high-quality development of the old industrial-bases. In order to solve this problem, we divide the sample into old industrial-base areas and non-old industrial-base areas, and perform sub-sample regression analysis. The results are shown in Table 7.
TABLE 7. The heterogeneous impacts of “low-carbon city pilot” policy on energy intensity: old industrial-bases and non-old industrial-bases.
The analysis in Table 7 shows that the “low-carbon city pilot” policy has heterogeneous impacts between old industrial-base area and the non-old industrial-base area, and its impact on the non-old industrial-base is more significant. Specifically, the coefficient of the interaction term in column 1 is insignificant, while the coefficient of the interaction term in column 2 is −0.522 and pass the 5% significance level test. It shows that the “low-carbon city pilot” policy has a significant inhibitory effect on the energy intensity of non-old industrial-bases, but has no significant effect on non-old industrial-bases areas. The reason lies in that their different concerns about environment quality. Specially, most of the non-old industrial bases are cities with a relatively developed economy and a higher degree of marketization, and they have more demands for environmental quality, thus more enthusiastic about participating in energy conservation and emission reduction (Li et al., 2019), so with the “low-carbon city pilot” policy introduced, the public in these areas consciously strengthen the supervision of corporate behavior, prompting corporations to improve energy efficiency and reduce energy intensity.
The premise of the difference-in-difference method is that the experimental group and the control group are comparable, that is, if there is no low-carbon city pilot policy, the energy intensity of the treat group and the control group will not change significantly over time and meet the parallel trend assumption. In order to verify this premise, we use the method of (Chen et al., 2018; Hong et al., 2020), use 2009 as the reference year, and add variables before and after the implementation of the policy into the model. The interaction term of the variable is used for event analysis, and the specific model is shown in Eq. 2. The coefficients of each interaction term are shown in Figure 2.
It can be seen from Figure 2 Results of parallel trend test that before the implementation of the low-carbon city policy, there was no significant difference between the control group and the treatment group, but after the implementation of the policy, the energy consumption per GRP of the pilot cities in 2011 and 2013 dropped significantly. Specifically, before the policy implementation, the coefficients of the interaction term are not significant, indicating that the difference in energy intensity between pilot cities and non-pilot cities is not significant before the policy is implemented, that is, the parallel trend assumption is satisfied. It is suitable to use the difference-in-difference method. After the implementation of the policy, the coefficient of the interaction term in 2011 and 2013 significantly dropped to a negative value, and passed the significance test of 10 and 5%, respectively, indicating that with the implementation of the “low-carbon city pilot” policy, the energy intensity was significantly reduced. In other words, the “low-carbon city pilot” policy have a certain inhibitory effect on energy intensity.
In order to further rule out the differences in energy intensity between pilot cities and non-pilot cities caused by other multiple compound factors, we also conduct a placebo test by constructing a false treatment group and a control group. From the overall sample of 271 cities, 61 cities were randomly selected as the treatment group, and other cities were used as the control group. Through random sampling, a group of false pilot cities and non-pilot cities are constructed. The dummy variable of the pilot city is assigned a value of 1 while the dummy variable of the false non-pilot city is assigned a value of 0, and then make a regression analysis with other control variables added. In this way, random sampling is repeated 500 times. The coefficients of the interaction term are used, and we plot the kernel density distribution diagram as shown by Figure 3.
It can be seen from Figure 3 that the estimation result of the benchmark model has passed the placebo test, indicating that the impact of the “low-carbon city pilot” policy on energy intensity has little correlation with other unknown factors, that is, the estimation result of the benchmark model is robust. Specifically, Figure 3 shows that the estimated coefficients obtained by 500 random processing are distributed near zero, indicating that the unobserved regional characteristics will not affect the estimated results.
Considering that our research period selected are 4 years before and after the implementation of the policy, is somewhat arbitrary. Therefore, in order to enhance the credibility of the conclusions, we further relax this assumption and set the research period as 3 years before and after the policy implementation and 2 years before and after the implementation of the policy for empirical analysis. The results are shown in Table 8.
Table 8 shows that when we change the previous research period 2006–2016 to two other period 2007–2016, and 2006–2015, which covers the period 3 years before and after the policy implementation, and the period 2 years before and after the policy implementation, the research conclusions have not substantially changed. This further confirmed the robustness of previous research conclusions. Specifically, the coefficients of the interaction terms in column 1 and column 2 are −0.6615, and −0.8295, respectively, and both pass the 1% significance test. It shows that after changing the research period, the low-carbon city pilot policy still have a significant inhibitory effect on energy intensity.
The theoretical analysis part of the previous article pointed out that “low-carbon city pilot” policy may contribute to energy conservation and emission reduction by encouraging enterprises to strengthen R&D and innovation, and may also reduce energy intensity by promoting the upgrading of industrial structure. To this end, we further explore the impact mechanism of “low-carbon city pilot” policy based on two perspectives of technological innovation and industrial structure upgrading.
In order to identify the existence of low-carbon city pilot impact mechanism, we use the mediating effect model to test (Hayes, 2009), the model is set as follows:
In Eqs. 3, 4,
It can be seen from Table 9 that low-carbon city pilot policies can reduce energy intensity by promoting regional green technology innovation and technological innovation, which supports the Porter Hypothesis. That is to say, as a favorable environmental regulation, the low-carbon city pilot policy could generate “innovation compensation”, eventually leading to the decrease of energy intensity. Specifically, the coefficients of the interaction terms in column 1 and column 3 are both positive, and have passed the 1 and 5% significance tests, respectively, indicating that the low-carbon city pilot policies can significantly promote regional green technological innovation and technological innovation; The coefficients of the interaction terms in column 2 and column 4 are significantly negative, while the coefficients in front of green technological innovation and technological innovation are negative, but they fail the significance test. Therefore, the Sobel test is required, and the test results are significant, indicating that the mediation effect exists.
“Low-carbon city pilot” policy may affect energy intensity by forming “environmental barriers” to industrial structure optimization. Environmental regulations require recourses re-allocation in the process of economic development, set up environmental barriers for industrial access, further eliminate backward industries with high energy consumption and high pollution, promote the development of low energy consumption and low pollution industries, and accelerate the “energy saving” upgrade of the economic structure. The entropy index is often used to measure income inequality between regions. We refer to the research of (Zhou et al., 2013; Cheng et al., 2018), and use the entropy index to measure the rationality of the industrial structure. Specifically, the construction of the index is as follows:
In Eq. 5,
It can be seen from Table 10 that “low-carbon city pilot” policies cannot reduce energy intensity through the influencing mechanism of the industrial structure. Specifically, the coefficient of the interaction term in column 1 is negative and has passed the 1% significance test, indicating that the “low-carbon city pilot” policy can significantly reduce the deviation of the industrial structure, that is, it can promote the upgrading of the industrial structure in each city. Neither the coefficient of the interaction term or the industry deviation variable in column 2 are significant. Furthermore, we performed the Sobel test, and the test result is not significant, indicating that the mediating effect does not exist.
Energy intensity is related to the sustainable use of fossil energy, and reducing energy intensity is one of the main strategies to deal with practical problems such as climate change and environmental degradation. The role of national macro-level factors on energy intensity has been basically confirmed, but the role of low-carbon policies at the regional level remains to be investigated. Based on the panel data of 271 cities in China from 2006 to 2016, this paper uses the low-carbon city pilot as a quasi-natural experiment to study the impact of regional-level low-carbon policies on energy intensity, and the following main conclusions are obtained:
First, low-carbon city pilot policies can effectively reduce regional energy intensity. As far as we know, pervious studies have shown that low-carbon city policies can effectively reduce carbon emissions and improve air quality, but whether it can reduce energy intensity remains to be verified. This paper empirically provide evidence for the positive effects of “low-carbon city pilot” policy on energy conservation.
Second, the effects of the “low-carbon city pilot” policy on energy intensity are heterogeneous. This heterogeneity is mainly reflected in different geographical locations, different economic development levels and whether it is a heavy industrial base. Compared with other regions, the low-carbon city pilot policies have a more significant inhibitory effect on the energy intensity of the eastern region, high economic development, and non-heavy industrial regions. This is mainly because, on the one hand, compared with the central and western regions and economically underdeveloped areas, these regions have superior geographical conditions and are easy to attract inflows of people and property. Therefore, low-carbon city pilot policies can more easily promote energy conservation and enhancement in the eastern region. Investment in energy efficiency and other aspects will play an innovative compensation effect to reduce energy intensity. On the other hand, the competition among industries in these areas is more intense, the R&D departments are more professional, and the public’s environmental appeals are stronger, which enables related industries to respond quickly after the policy is introduced, which in turn reduces energy intensity.
Finally, “low-carbon city pilot” policy mainly affect regional energy intensity through technological innovation, rather than the mechanism of industrial structure optimization. Through mechanism analysis, we further demonstrated that “low-carbon city pilot” policy will mainly bring innovation compensation effects, promote technological innovation and green technology innovation, and reduce energy intensity. In addition, “low-carbon city pilot” policy have also brought about some problems. For example, “low-carbon city pilot” policy will further increase the energy intensity difference between medium and high economic development areas and low economic development areas.
Based on the research conclusions, we propose the following policy recommendations: First, the government can further promote “low-carbon city pilot” policy nationwide by refining pilot experience, and effectively supervise pilot cities and induce their technological innovation, so as to achieve the goal of energy saving, emission reduction and green development. China’s low-carbon city policy is introduced by government departments, and maybe other countries can also introduce this kind of environmental regulation policies to reduce the energy intensity of cities. Second, comparing the results of different types of regions, it can be seen that government should implement differentiated regulations according to local economic conditions, especially for the energy intensity of the central and western regions, low economic development regions, and heavy industrial base regions. Third, the government can formulate policies that encourage technological innovation and green technological innovation, improve the technological innovation, and thus enhance the inhibitory effect of “low-carbon city pilot” policy on energy intensity.
We have evaluated the impacts of “low-carbon city pilot” policy on energy intensity, but due to the availability of data, this article still has some limitations. The optimization and upgrading of the industrial structure is not limited to the evolution of the first, second and third industries, but includes the internal adjustments of various sub-industries. Therefore, the mechanism of industrial structure optimization still needs further research. Moreover, the low-carbon city pilot policy itself is still in the process of gradual advancement, and more in-depth research on this policy can be carried out in future.
The original contributions presented in the study are included in the article/Supplementary Material, further inquiries can be directed to the corresponding author.
Conceptualization, methodology, and writing—original draft preparation: MH and SC; Data curation: MH and KZ.
This research was funded by the Project of Hunan Social Science Achievement Evaluation Committee in China (XSP19YBC271) and National Social Science Fund (No. 20BTJ022).
The authors declare that the research was conducted in the absence of any commercial or financial relationships that could be construed as a potential conflict of interest.
The Supplementary Material for this article can be found online at: https://www.frontiersin.org/articles/10.3389/fenvs.2021.717737/full#supplementary-material
Bashir, M. F., Ma, B., Shahbaz, M., Shahzad, U., and Vo, X. V. (2021). Unveiling the Heterogeneous Impacts of Environmental Taxes on Energy Consumption and Energy Intensity: Empirical Evidence from OECD Countries. Energy 226, 120366. doi:10.1016/j.energy.2021.120366
Canh, N. P., Thanh, S. D., and Nasir, M. A. (2020). Nexus between Financial Development & Energy Intensity: Two Sides of a coin?. J. Environ. Manage. 270, 110902. doi:10.1016/j.jenvman.2020.110902
Cao, W., Chen, S., and Huang, Z. (2020). Does Foreign Direct Investment Impact Energy Intensity? Evidence from Developing Countries. Math. Probl. Eng. 2020, 1–11. doi:10.1155/2020/5695684
Chang, M.-C. (2014). Energy Intensity, Target Level of Energy Intensity, and Room for Improvement in Energy Intensity: An Application to the Study of Regions in the EU. Energy Policy 67, 648–655. doi:10.1016/j.enpol.2013.11.051
Chen, Y.-C., Hung, M., and Wang, Y. (2018). The Effect of Mandatory CSR Disclosure on Firm Profitability and Social Externalities: Evidence from China. J. Account. Econ. 65 (1), 169–190. doi:10.1016/j.jacceco.2017.11.009
Cheng, J., Yi, J., Dai, S., and Xiong, Y. (2019). Can Low-Carbon City Construction Facilitate green Growth? Evidence from China's Pilot Low-Carbon City Initiative. J. Clean. Prod. 231, 1158–1170. doi:10.1016/j.jclepro.2019.05.327
Cheng, Z., Li, L., and Liu, J. (2018). Industrial Structure, Technical Progress and Carbon Intensity in China's Provinces. Renew. Sust. Energ. Rev. 81, 2935–2946. doi:10.1016/j.rser.2017.06.103
Elliott, R. J. R., Sun, P., and Chen, S. (2013). Energy Intensity and Foreign Direct Investment: A Chinese City-Level Study. Energ. Econ. 40, 484–494. doi:10.1016/j.eneco.2013.08.004
Filipović, S., Verbič, M., and Radovanović, M. (2015). Determinants of Energy Intensity in the European Union: A Panel Data Analysis. Energy 92, 547–555. doi:10.1016/j.energy.2015.07.011
Gehrsitz, M. (2017). The Effect of Low Emission Zones on Air Pollution and Infant Health. J. Environ. Econ. Manag. 83, 121–144. doi:10.1016/j.jeem.2017.02.003
Hamamoto, M. (2006). Environmental Regulation and the Productivity of Japanese Manufacturing Industries. Resource Energ. Econ. 28 (4), 299–312. doi:10.1016/j.reseneeco.2005.11.001
Hayes, A. F. (2009). Beyond Baron and Kenny: Statistical Mediation Analysis in the New Millennium. Commun. Monogr. 76 (4), 408–420. doi:10.1080/03637750903310360
Hong, M., Drakeford, B., Drakeford, B., and Zhang, K. (2020). The Impact of Mandatory CSR Disclosure on green Innovation: Evidence from China. Green. Finance 2 (3), 302–322. doi:10.3934/gf.2020017
Hong, M., Li, Z., and Drakeford, B. (2021). Do the Green Credit Guidelines Affect Corporate Green Technology Innovation? Empirical Research from China. Ijerph 18 (4), 1682. doi:10.3390/ijerph18041682
Hou, J., Teo, T. S. H., Zhou, F., Lim, M. K., and Chen, H. (2018). Does Industrial green Transformation Successfully Facilitate a Decrease in Carbon Intensity in China? an Environmental Regulation Perspective. J. Clean. Prod. 184, 1060–1071. doi:10.1016/j.jclepro.2018.02.311
Jiang, X., Duan, Y., and Green, C. (2017). Regional Disparity in Energy Intensity of China and the Role of Industrial and export Structure. Resour. Conservation Recycling 120, 209–218. doi:10.1016/j.resconrec.2016.12.013
Jimenez, R., and Mercado, J. (2014). Energy Intensity: A Decomposition and Counterfactual Exercise for Latin American Countries. Energ. Econ. 42, 161–171. doi:10.1016/j.eneco.2013.12.015
Jorgenson, D. W., and Wilcoxen, P. J. (1990). Environmental Regulation and U.S. Economic Growth. RAND J. Econ. 21 (2), 314. doi:10.2307/2555426
Li, K., and Lin, B. (2014). The Nonlinear Impacts of Industrial Structure on China's Energy Intensity. Energy 69, 258–265. doi:10.1016/j.energy.2014.02.106
Li, T., Li, X., and Li, X. (2020). Does Structural Deceleration Happen in China? Evidence from the Effect of Industrial Structure on Economic Growth Quality. Natl. Account. Rev. 2 (2), 155–173. doi:10.3934/nar.2020009
Li, T., and Liao, G. (2020). The Heterogeneous Impact of Financial Development on Green Total Factor Productivity. Front. Energ. Res. 8. doi:10.3389/fenrg.2020.00029
Li, W., Zhao, T., Wang, Y., Zheng, X., and Yang, J. (2019). How Does Foreign Direct Investment Influence Energy Intensity Convergence in China? Evidence from Prefecture-Level Data. J. Clean. Prod. 219, 57–65. doi:10.1016/j.jclepro.2019.02.025
Liang, H. J., Liu, J. S., Wang, R., Song, Y. Q., and Zhou, Y. Y. (2020). Research on China's Regional Energy Efficiency Evaluation and Influencing Factors Based on the DEA-Tobit Model. Pol. J. Environ. Stud. 29 (5), 3691–3701. doi:10.15244/pjoes/115976
Lin, B., and Wang, M. (2021). What Drives Energy Intensity Fall in China? Evidence from a Meta-Frontier Approach. Appl. Energ. 281, 116034. doi:10.1016/j.apenergy.2020.116034
Luan, B., Zou, H., Chen, S., and Huang, J. (2021). The Effect of Industrial Structure Adjustment on China's Energy Intensity: Evidence from Linear and Nonlinear Analysis. Energy 218, 119517. doi:10.1016/j.energy.2020.119517
Ma, B., and Yu, Y. (2017). Industrial Structure, Energy-Saving Regulations and Energy Intensity: Evidence from Chinese Cities. J. Clean. Prod. 141, 1539–1547. doi:10.1016/j.jclepro.2016.09.221
Nur Utomo, M., Rahayu, S., Rahayu, S., Kaujan, K., and Agus Irwandi, S. (2020). Environmental Performance, Environmental Disclosure, and Firm Value: Empirical Study of Non-financial Companies at Indonesia Stock Exchange. Green. Finance 2 (1), 100–113. doi:10.3934/gf.2020006
Porter, M. E., and Linde, C. v. d. (1995). Toward a New Conception of the Environment-Competitiveness Relationship. J. Econ. Perspect. 9 (4), 97–118. doi:10.2307/213839210.1257/jep.9.4.97
Rafiq, S., Salim, R., and Nielsen, I. (2016). Urbanization, Openness, Emissions, and Energy Intensity: A Study of Increasingly Urbanized Emerging Economies. Energ. Econ. 56, 20–28. doi:10.1016/j.eneco.2016.02.007
Sadorsky, P. (2013). Do urbanization and Industrialization Affect Energy Intensity in Developing Countries?. Energ. Econ. 37, 52–59. doi:10.1016/j.eneco.2013.01.009
Samargandi, N. (2019). Energy Intensity and its Determinants in OPEC Countries. Energy 186, 115803. doi:10.1016/j.energy.2019.07.133
Wolff, H. (2014). Keep Your Clunker in the Suburb: Low‐Emission Zones and Adoption of Green Vehicles. Econ. J. 124 (578), F481–F512. doi:10.1111/ecoj.12091
Wu, Y. (2012). Energy Intensity and its Determinants in China's Regional Economies. Energy Policy 41, 703–711. doi:10.1016/j.enpol.2011.11.034
Wurlod, J.-D., and Noailly, J. (2018). The Impact of green Innovation on Energy Intensity: An Empirical Analysis for 14 Industrial Sectors in OECD Countries. Energ. Econ. 71, 47–61. doi:10.1016/j.eneco.2017.12.012
Yan, H. (2015). Provincial Energy Intensity in China: The Role of Urbanization. Energy Policy 86, 635–650. doi:10.1016/j.enpol.2015.08.010
Yang, L., and Li, Y. (2013). Low-carbon City in China. Sust. Cities Soc. 9, 62–66. doi:10.1016/j.scs.2013.03.001
Yuxiang, K., and Chen, Z. (2010). Government Expenditure and Energy Intensity in China. Energy Policy 38 (2), 691–694. doi:10.1016/j.enpol.2009.10.031
Zhang, D., Li, J., and Ji, Q. (2020a). Does Better Access to Credit Help Reduce Energy Intensity in China? Evidence from Manufacturing Firms. Energy Policy 145, 111710. doi:10.1016/j.enpol.2020.111710
Zhang, H., Fan, L. W., and Zhou, P. (2020b). Handling Heterogeneity in Frontier Modeling of City-Level Energy Efficiency: The Case of China. Appl. Energ. 279, 115846. doi:10.1016/j.apenergy.2020.115846
Zheng, Y., Chen, S., Chen, S., and Wang, N. (2020). Does Financial Agglomeration Enhance Regional green Economy Development? Evidence from China. Green. Finance 2 (2), 173–196. doi:10.3934/gf.2020010
Zhong, J., and Li, T. (2020). Impact of Financial Development and its Spatial Spillover Effect on Green Total Factor Productivity: Evidence from 30 Provinces in China. Math. Probl. Eng. 2020, 1–11. doi:10.1155/2020/5741387
Keywords: the low-carbon city pilot policy, energy intensity, cities, China, difference-in-difference
Citation: Hong M, Chen S and Zhang K (2021) Impact of the “Low-Carbon City Pilot” Policy on Energy Intensity Based on the Empirical Evidence of Chinese Cities. Front. Environ. Sci. 9:717737. doi: 10.3389/fenvs.2021.717737
Received: 31 May 2021; Accepted: 25 June 2021;
Published: 07 July 2021.
Edited by:
Qiang Ji, Institutes of Science and Development (CAS), ChinaReviewed by:
Yan Zeng, Sun Yat-Sen University, ChinaCopyright © 2021 Hong, Chen and Zhang. This is an open-access article distributed under the terms of the Creative Commons Attribution License (CC BY). The use, distribution or reproduction in other forums is permitted, provided the original author(s) and the copyright owner(s) are credited and that the original publication in this journal is cited, in accordance with accepted academic practice. No use, distribution or reproduction is permitted which does not comply with these terms.
*Correspondence: Shuanglian Chen, Y2hlbnNsQGd6aHUuZWR1LmNu
Disclaimer: All claims expressed in this article are solely those of the authors and do not necessarily represent those of their affiliated organizations, or those of the publisher, the editors and the reviewers. Any product that may be evaluated in this article or claim that may be made by its manufacturer is not guaranteed or endorsed by the publisher.
Research integrity at Frontiers
Learn more about the work of our research integrity team to safeguard the quality of each article we publish.