- 1State Key Laboratory of Infectious Disease Prevention and Control, National Institute for Communicable Disease Control and Prevention, Chinese Center for Disease Control and Prevention, Beijing, China
- 2Zhejiang Provincial Centers for Disease Control and Prevention, Hangzhou, China
- 3Department of Personnel, Qilu Hospital of Shandong University, Jinan, China
Background: Hemorrhagic fever with renal syndrome (HFRS) is an endemic in China, accounting for 90% of HFRS cases worldwide and growing. Therefore, it is urgent to monitor and predict HFRS cases to make control measures more effective. In this study, we applied generalized additive models (GAMs) in Liaoning Province, an area with many HFRS cases. Our aim was to determine whether GAMs could be used to accurately predict HFRS cases and to explore the association between meteorological factors and the incidence of HFRS.
Methods: HFRS data from Liaoning were collected from January 2005 to May 2019 and used to construct GAMs. Generalized cross-validation (GCV) and adjusted R-square (R2) values were used to evaluate the constructed models. The interclass correlation coefficient (ICC) was used as an index to assess the quality of the proposed models.
Results: HFRS cases of the previous month and meteorological factors with different lag times were used to construct GAMs for three cities in Liaoning. The three models predicted the number of HFRS cases in the following month. The ICCs of the three models were 0.822, 0.832, and 0.831. Temperature and the number of cases in the previous month had a positive association with HFRS.
Conclusion: GAMs applied to HFRS case data are an important tool for HFRS control in China. This study shows that meteorological factors have an effect on the occurrence of HFRS. A mathematical model based on surveillance data could also be used in forecasting. Our study will inform local CDCs and assist them in carrying out more effective measures for HFRS control and prevention through simple modeling and forecasting.
Background
Hemorrhagic fever with renal syndrome (HFRS) is caused by a negative-sense single-stranded RNA virus known as hantavirus (genus Hantavirus, family Bunyavirdae). More than ten thousand cases have been reported annually in China in recent years (Bai and Huang, 2002). Since HFRS was first recognized in northeast China in 1931 (Zhang et al., 2014), this disease had been reported in only two provinces until the 1950s, while the disease has been on the rise since then and has now been reported in 29 of 31 provinces (Chen et al., 1986; Zhang et al., 2004). More than 1.5 million HFRS cases were reported from 1950 to 2007 (He et al., 2018; Zhang Y.Z et al., 2010), and more than 50,000 cases were reported from 2013 to 2018 in mainland China. Several factors are thought to be related to the increasing HFRS cases and expanding endemic trend, including urbanization, human migration, economic development, and the effect of climate change (Zuo et al., 2011). In China, Hantaan virus (HTNV) and Seoul virus (SEOV) are two dominant hantaviruses, which are transmitted by the rodent species Apodemus agrarius and Rattus norvegicus, respectively (Zuo et al., 2014). The epidemic of HTNV infection shows a rapid peak in autumn and winter, while the epidemic caused by SEOV infection always shows a long-lasting peak in spring and summer (Chen et al., 1986). To date, HFRS has also been reported in Europe, the United States, Africa, and the Middle Eastern countries (Klempa, 2009; Knust and Rollin, 2013; Zelená et al., 2013; Witkowski et al., 2014). In China, the majority of HFRS cases occur in Shaanxi, Heilongjiang, Jilin, and Liaoning (Zhang Y.Z et al., 2010). Liaoning is an important HFRS epidemic area in China, with 17,335 reported cases from 2005 to 2017. Because of the transmission by rodents and the seasonal distribution of HFRS epidemics, HFRS was chosen as a means of studying the effect of climate factors on the epidemiology of infectious diseases. The seasonal autoregressive integrated moving average (SARIMA) model and seasonal autoregressive integrated moving average with exogenous variables (SARIMAX) model are the common models used to predict the incidence of HFRS (He et al., 2018). They evolved from the classic prediction model ARIMA, which is constructed using changes, periodic trends, and random disturbances in time series (Liu et al., 2011a). A complex algorithm is applied to reveal the factors driving disease epidemics for the purposes of predicting future outbreaks. The generalized additive model (GAM) is a classical method and was applied in our study to predict the incidence of HFRS with meteorological factors and previous HFRS cases with controlling for nonlinear confounding effects (Dominici et al., 2002).
Furthermore, we chose three areas in Liaoning with high numbers of HFRS cases—Dandong, Huludao, and Shenyang—to examine and compare. Among the three areas, Dandong is a typical HFRS epidemic area, with infections caused mainly by the HTNV viral strain; Huludao is a typical epidemic area for the SEOV strain; and Shenyang, the province’s capital city, is an epidemic area for both viruses. The epidemics of the three cities show different seasonal distributions of cases (Supplementary Figures S1–S3), and there may be different associations between the incidence of HFRS and meteorological factors. Thus, individual forecasts for these cities must be made.
The relationship between climate factors and HFRS has been examined in many previous studies (Fang et al., 2010; Liu et al., 2011b; Xiao et al., 2013a; Li et al., 2013). However, few studies have focused on the relationships between meteorological factors and HFRS incidence in areas with different epidemic seasons. In this study, we constructed three GAMs to analyze the associations between meteorological factors and HFRS and created models to make early predictions.
Methods
Study Sites
Liaoning is situated in northeastern China, located between latitudes 38°43′ and 43°26′ north and longitudes 118°53′ and 125°46′ east. The province has 14 cities, three of which we chose to study, namely, Dandong, Huludao, and Shenyang. (Figure 1).
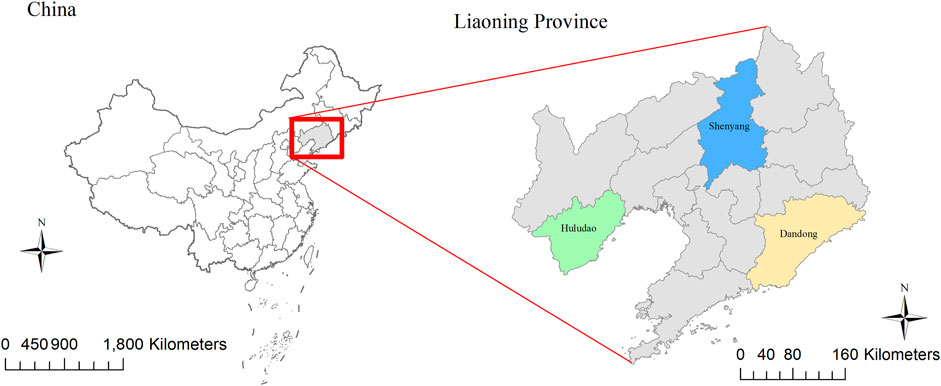
FIGURE 1. Geographical distribution of study areas in China. The map was created by ArcGIS 10.3 (Environmental Systems Research Institute; Redlands, CA, USA). The base map was acquired from data center for geographic sciences and natural sources research, CAS (http://www.resdc.cn/).
Dandong is located in southeast Liaoning adjacent to the Yellow Sea. It had a population of 2.34 million in 2014 and an area of 15,222 km2. The city has an annual mean rainfall of 800–1,200 mm and an annual mean temperature of 8–10°C. Huludao is a coastal city located southwest of Liaoning, with a population of 2.78 million in 2017. The annual mean temperature is 8–9°C, and the annual mean rainfall is approximately 500–610 mm. Shenyang, the capital city of Liaoning, is the only megacity in northern China, with a total area of 12,948 km2 and a population of 8.11 million in 2018. The city has an annual rainfall of 600–800 mm, and a mean temperature range of 6–9°C.
Data Collection
Meteorological data were collected from the China Meteorological Data Sharing Service System (http://data.cma.cn), including monthly means for temperature, minimum temperature, maximum temperature, relative humidity, precipitation, and sunshine hours. HFRS is a class B notifiable infectious disease in China (Zou et al., 2016) and was diagnosed according to the criteria issued by the Ministry of Health in China. Local healthcare staff must report new HFRS cases to the Center for Disease Control and Prevention (CDC) daily through the Nationwide Notifiable Infectious Diseases Reporting Information System (NIDRIS) within 24 h after diagnosis. HFRS case-level records from January 2005 to May 2019 for our study were obtained from the NIDRIS.
Data Analysis
First, a descriptive analysis was used to define the overall epidemiological characteristics of HFRS cases reported in the three cities during the study period (Figure 2). Second, a Pearson correlation analysis including lags of up to 6 months was performed to select the lag time. In addition, an autocorrelation analysis was used to explore whether the monthly HFRS cases were correlated with the HFRS cases in previous months.
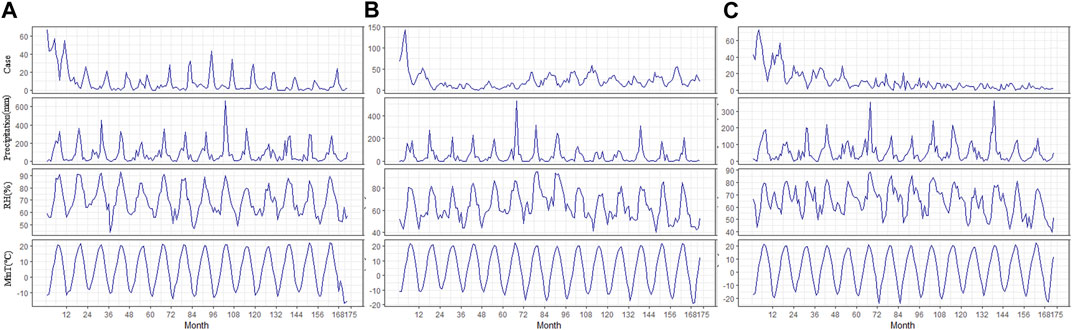
FIGURE 2. Time series of HFRS cases and meteorological factors in the study areas from 2005-2019. [(A): Dandong; (B): Huludao; (C): Shenyang].
Third, a GAM was used to quantify nonlinear associations between meteorological factors and HFRS, as the effect of climate factors on the epidemic of HFRS is considered commonly lagged with certain months, including the time for the development of rodent populations and the incubation period of sensitive populations (Jiang et al., 2017; Li et al., 2019). The number of monthly HFRS cases in the whole population is generally assumed to be small (Li et al., 2011; Sun et al., 2018; Li et al., 2019).
The GAM was formulated as follows:
where
In the process of model selection, we first performed a correlation analysis of the climate variables at certain lag times of 1–6 months (Zhang W.Y et al., 2010; Supplementary Table S1). Second, all significant variables with specific delays were included in the model. After permutation and combination, many models were obtained. Among these models, better-fitting models had a higher adjusted R2 and deviance explained value but a lower GCV score (Wood, 2006).
Data in this study were split by using the first 12 years (from January 2005 to December 2016) for model fitting and the last time period of less than 3 years (from January 2017 to May 2019) to test the constructed model and make a prediction (Tian et al., 2017; Xu et al., 2017). The interclass correlation coefficient (ICC) was applied to measure the consistency between the predicted and actual values (Dayama and Kameshwaran, 2013; Sun et al., 2018).
The package “mgcv” (Wood, 2004) in R Project 3.4.4 (R Development Core Team, 2012) was used to construct the GAM. Geographic maps for the study sites were generated using ArcGIS 10.3 software (ESRI, Redlands, CA, United States).
Results
Descriptive Analysis
Dandong, Huludao, and Shenyang reported 1,503; 3,832; and 1,876 HFRS cases, respectively, with averages of 8.69, 22.15, and 10.84 new HFRS cases every month during the study period (Table 1). We also observed that the incidence of HFRS is seasonal and that the epidemic months are different in these three cities. Dandong had an autumn peak of HFRS in 2005–2018, and HFRS cases spiked in October to December (Supplementary Figure S1), accounting for 51.63% (776/1,503) of the total number of cases; November was the month with the highest incidence of HFRS. The number of HFRS cases in Huludao peaked in spring in 2005–2018, with cases concentrated in February to May (Supplementary Figure S2), accounting for 50.10% (1,918/3,832) of the city cases. Shenyang had two HFRS epidemic peaks: a spring peak and an autumn–winter peak (Supplementary Figure S3) in February–May and October–December, respectively. The two peaks accounted for 71.70% of the total reported cases in the whole year (1,345/1,876). The temporal variation trend of HFRS cases and meteorological factors in the study areas are shown in Figure 2.
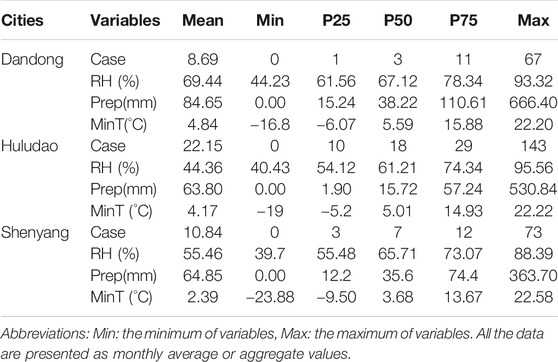
TABLE 1. Monthly meteorological variables and HFRS occurrence in the three cities from 2005 to 2019.
Correlation Analysis
In the three study areas, RH, prep, and MinT were significantly correlated with monthly HFRS cases and had different lag times (Supplementary Table S1). In addition, for the three study areas, the p value of the Ljung-Box Q statistic of HFRS incidence for each lagged month was <0.05, and the values of the autocorrelation coefficient and partial autocorrelation coefficient during the first lagged month were greater than those of other lagged months, which indicated that the HFRS cases had a strong autocorrelation with the first one-month lag (Supplementary Table S2).
Model Construction and Evaluation
For Dandong, the monthly mean minimum temperature at a lag of 3 months, monthly cumulative precipitation at a lag of 2 months, and monthly relative humidity at a lag of 4 months were included in the final best-fitting model of HFRS, with a GCV score of 2.33, adjusted R2 of 0.863, explained value of deviance of 87.3%, and ICC of 0.822 (Figure 3B). The model suggests that the HFRS cases in the previous month, Prep and MinT, are positively correlated with HFRS cases, while RH has a negative relationship with the occurrence of HFRS cases (Figure 3A). Residuals under different lags of autocorrelation and partial autocorrelation were not significant (Supplementary Figure S4).
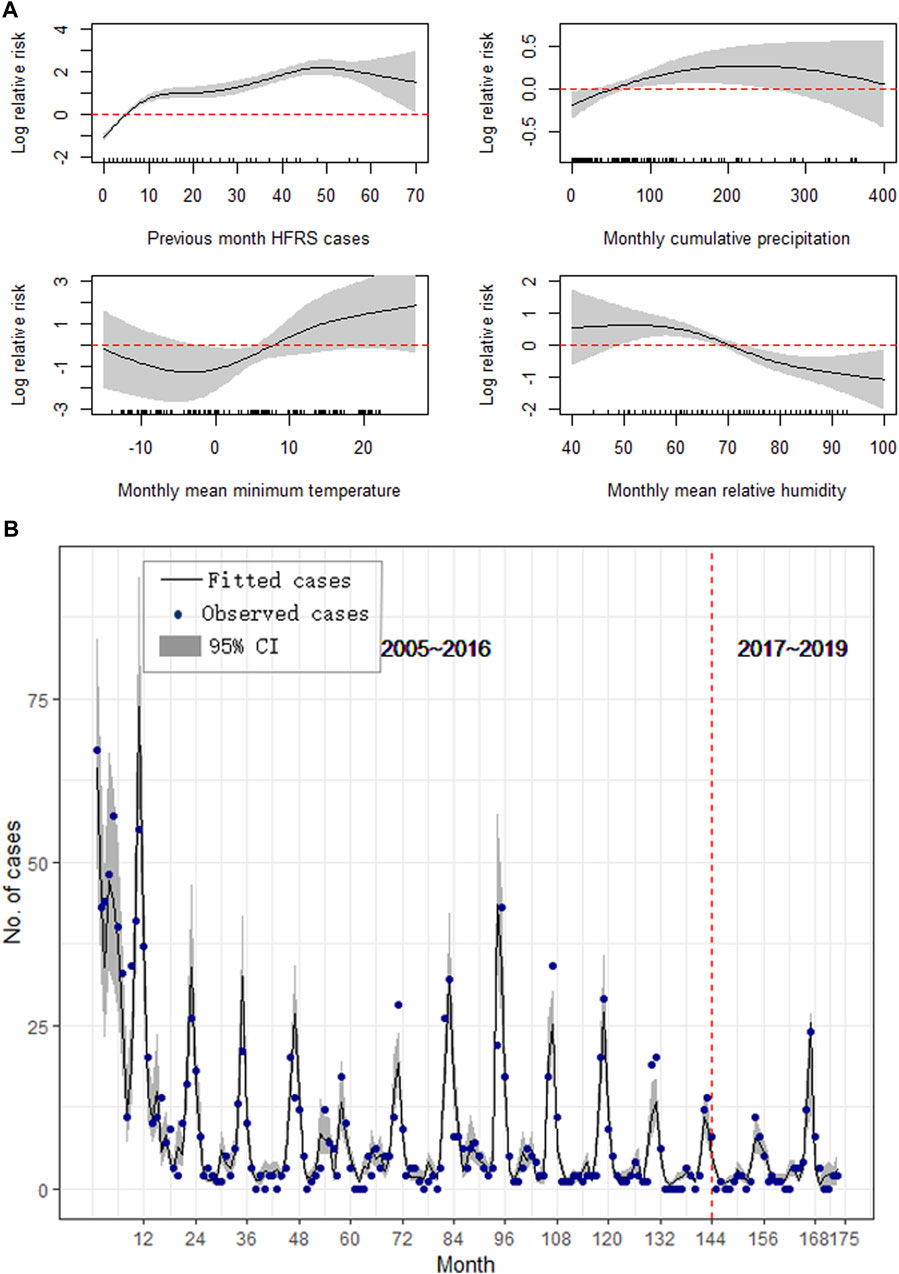
FIGURE 3. Results of the GAM for Dandong. (A) The effect of meteorological on the epidemics of HFRS, (B) Forecast and comparison of the fitted values.
For Huludao, the best-fitting GAM contained variables including the one-month lag of cases, monthly cumulative precipitation at a lag of 4 months, monthly mean minimum temperature at a lag of 3 months, and monthly mean relative humidity at a lag of 2 months, with a GCV value of 2.81, an adjusted R2 of 0.878, an explained value of deviance of 85.8%, and an ICC of 0.832 (Figure 4B). The number of HFRS cases was positively associated with the previous-month HFRS cases, monthly cumulative precipitation, monthly mean minimum temperature, and monthly mean relative humidity (Figure 4A). No significant autocorrelation or partial autocorrelation was found between residuals at different lags (Supplementary Figure S5).
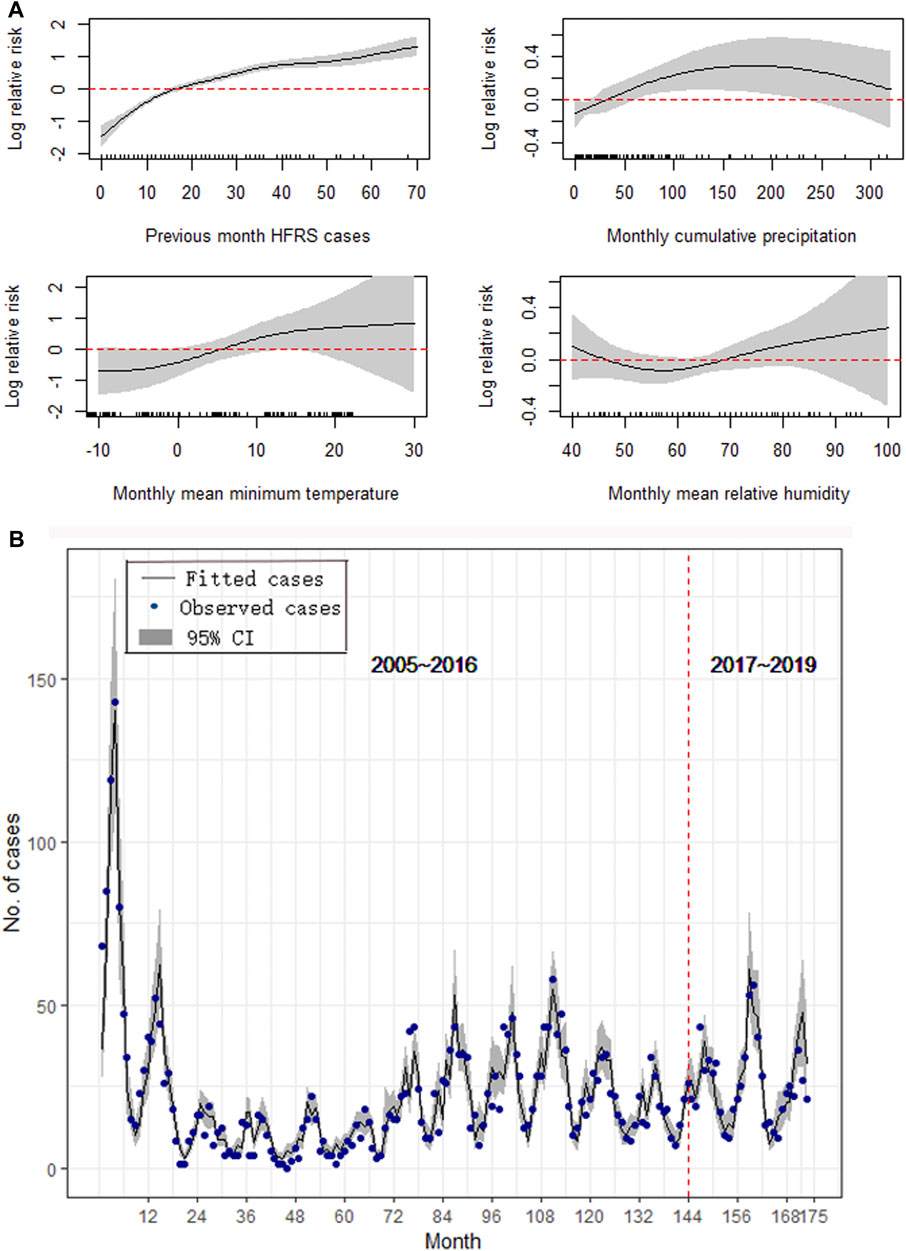
FIGURE 4. Results of the GAM for Huludao. (A) The effect of meteorological on the epidemics of HFRS. (B) Forecast and comparison of the fitted values.
For Shenyang, the monthly cumulative precipitation at a lag of 2 months, monthly mean minimum temperature at a lag of 2 months, and monthly mean relative humidity at a lag of 1 month were significantly associated with HFRS and used to construct the best-fitting model, with a GCV value of 2.81, an adjusted R2 of 0.88, an explained deviation value of 85.5%, and an ICC of 0.83 (Figure 5B). Positive associations of HFRS cases were found with the previous-month HFRS cases, monthly mean minimum temperature, monthly mean relative humidity, and monthly cumulative precipitation below 200 mm. However, the HFRS cases were negatively correlated with monthly cumulative precipitation higher than 200 mm (Figure 5A). There was no significant autocorrelation or partial autocorrelation between residuals for the final model of Shenyang under different lag months (Supplementary Figure S6).
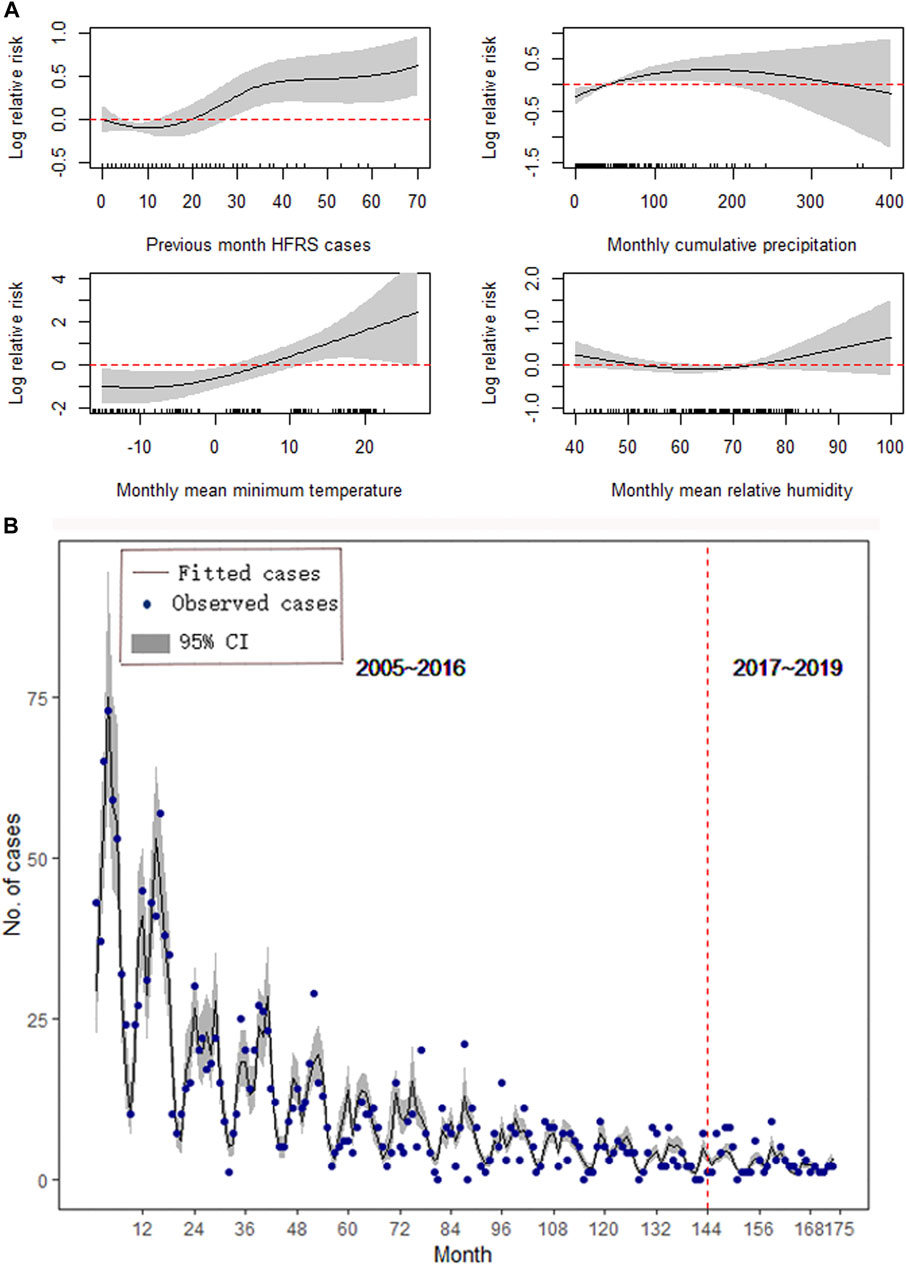
FIGURE 5. Results of the GAM for Shenyang. (A) The effect of meteorological conditions on the epidemics of HFRS. (B) Forecast and comparison of the fitted values.
Evaluation of Forecast Models
We validated our models by comparing the predicted results with the surveillance HFRS data from January 2017 to May 2019, and the results showed good performances in all three study areas. Figures 3B, 4B, 5B also show that the majority of the predicted data agreed closely with the observed data; however, the results for Huludao City presented better ICCs (Table 2).
Discussion
Time-series analysis is widely used in the field of epidemiology to explore the relationships between climate variables and vector-borne viral infectious diseases, and HFRS is among the most sensitive of all diseases to climate change (Liu et al., 2011b; Xiao et al., 2013b). Compared with SARIMA and SARIMAX, the GAM is a flexible statistical method that uses smoothing functions on the predictor variable and whichever link function may be appropriate (Dominici et al., 2002). The results of our study indicated that the GAM method was efficient in constructing models for forecasting HFRS occurrence and exploring the effects of weather variables on HFRS.
The lag effect between climate and HFRS was also proved in some other studies (Kallio et al., 2006; Bai et al., 2015; Jiang et al., 2017), and models including temperature, rainfall, humidity in the previous several months, and the number of cases in the previous month seemed more reliable and practical in predicting HFRS in endemic areas than other previously reported models (Li et al., 2012; Li et al., 2016). Some previous studies reported that the occurrence of HFRS was associated with meteorological factors. Our results for the three cities consistently revealed that there is an obvious inverted-U shape between the monthly cumulative rainfall and the incidence of HFRS. When the monthly total rainfall is less than 200 mm, the effect of HFRS increases with increasing rainfall. When the monthly rainfall exceeds 200 mm, the effect decreases with increasing rainfall. The results of this study are partially consistent with those of other studies. The studies of Yan et al. and Bi et al. revealed that wetlands with moist soil are an ideal habitat for rodents and present an increased risk for disease transmission (Bi et al., 2005; Yan et al., 2007). Adequate rainfall is essential to the reproduction of rodents (Bi et al., 1998). This means that moderate monthly precipitation is good for rodent growth, while monthly rainfall that exceeds 200 mm may destroy the microenvironment of rodents, including their nests, and thus reduce the chance that they will survive to infect humans. Liu et al. found that precipitation ranging between 10 and 120 mm is the most favorable condition for the occurrence of HFRS (Liu et al., 2013).
The relationship between temperature and HFRS is S-shaped. At the three study sites, with increasing temperature, the effect of temperature on HFRS increases with no maximum effect. Temperature influences rodent pregnancy rate, litter size, birth rate, and survival rate and promotes the activity of both rodents and humans (Zhang et al., 1993). Jiang et al. found that the pregnancy rate of rodents was 25% at 10–25°C and 90.63% at 15–20°C (Jiang et al., 2017). However, our study showed no maximum effect of temperature on the incidence of HFRS at our study sites.
The relationship between relative humidity and the incidence of HFRS seemed inconsistent among the three cities. There was a negative relationship between relative humidity and the incidence of HFRS in Dandong but a positive one in Huludao and Shenyang. This may partly be because, compared with Dandong, the other two cities have similar lower monthly mean relative humidity values, which creates a special environment for rodents and the occurrence of HFRS. However, the mechanism needs further study.
We validated our models by comparing the predictive results with the surveillance HFRS data from January 2017 to May 2019. The ICC, which ranges from 0 to 1, was used to verify the consistency between the predicted and actual data. The closer the ICC value is to 1, the smaller the difference between the predicted value and the actual value, and the better the model’s predictive effect (Shrout and Fleiss, 1979; Koo and Li, 2016). In this study, the ICC values for the three cities were larger than 0.8, and the results showed good performance for all three models.
There are several limitations in our study that should also be acknowledged. First, this study followed an ecological design. We only added meteorological variables and lacked data on factors such as rat density and local economic factors; thus, important unknown and unmeasured factors may have existed. In addition, tourism and transportation may also have effects on the transmission of HFRS, especially in these tourist cities; however, due to data availability, they were not included in this study.
Conclusion
In summary, our study indicated that the seasonal dynamics of HFRS are correlated with meteorological factors; when the monthly rainfall is 200 mm, the effect on HFRS incidence is the largest, and temperature has a significant positive correlation with the incidence rate of HFRS. By including meteorological variables in the model and adjusting their lag time, a well-fitted prediction model was established. The prediction results of our study will be helpful in developing an early warning system, and this study on the relationships between meteorological factors and HFRS is helpful for disease prevention and control staff seeking to better understand the relationship between these factors and to develop corresponding measures in advance under specific meteorological conditions.
Data Availability Statement
The original contributions presented in the study are included in the article/Supplementary Material; further inquiries can be directed to the corresponding author's.
Author Contributions
WS: data curation, conception, software, and writing - original draft. ZL: conception, methodology, and software. WL: data curation and software. QL: supervision. LL: data collection, visualization, and writing - reviewing and editing.
Funding
The study was supported by the Special Foundation of Basic Science and Technology Resources Survey of the Ministry of Science and Technology, China (grant No. 2017FY101202).
Conflict of Interest
The authors declare that the research was conducted in the absence of any commercial or financial relationships that could be construed as a potential conflict of interest.
Acknowledgments
We thank the staff members from the various CDCs and the National Meteorological Information Center of China for their valuable assistance in collecting the data that we used.
Supplementary Material
The Supplementary Material for this article can be found online at: https://www.frontiersin.org/articles/10.3389/fenvs.2021.707960/full#supplementary-material
References
Bai, X., and Huang, C. (2002). Study Farther on Hemorrhagic Fever with Renal Syndrome. Chin. J. Infect. Dis. 20, 197–198.
Bai, Y., Xu, Z., Lu, B., Sun, Q., Tang, W., Liu, X., et al. (2015). Effects of Climate and Rodent Factors on Hemorrhagic Fever with Renal Syndrome in Chongqing, China, 1997-2008. PLoS One 10 (7), e0133218. doi:10.1371/journal.pone.0133218
Bi, P., Parton, K. A., and Tong, S. (2005). El Nino-Southern Oscillation and Vector-Borne Diseases in Anhui, China. Vector-Borne Zoonotic Dis. 5 (2), 95–100. doi:10.1089/vbz.2005.5.95
Bi, P., Wu, X., Zhang, F., Parton, K. A., and Tong, S. (1998). Seasonal Rainfall Variability, the Incidence of Hemorrhagic Fever with Renal Syndrome, and Prediction of the Disease in Low-Lying Areas of China. Am. J. Epidemiol. 148 (3), 276–281. doi:10.1093/oxfordjournals.aje.a009636
Chen, H.-X., Qiu, F.-X., Dong, B.-J., Ji, S.-Z., Li, Y.-T., Wang, Y., et al. (1986). Epidemiological Studies on Hemorrhagic Fever with Renal Syndrome in China. J. Infect. Dis. 154 (3), 394–398. doi:10.1093/infdis/154.3.394
Dayama, P., and Kameshwaran, S. (2013). Predicting the Dengue Incidence in Singapore Using Univariate Time Series Models. AMIA Ann. Symp. Proc. 8.
Dominici, F., Mcdermott, A., Zeger, S. L., and Samet, J. M. (2002). On the Use of Generalized Additive Models in Time-Series Studies of Air Pollution and Health. Am. J. Epidemiol. 156 (3), 193–203. doi:10.1093/aje/kwf062
Fang, L.-Q., Wang, X.-J., Liang, S., Li, Y.-L., Song, S.-X., Zhang, W.-Y., et al. (2010). Spatiotemporal Trends and Climatic Factors of Hemorrhagic Fever with Renal Syndrome Epidemic in Shandong Province, China. Plos Negl. Trop. Dis. 4 (8), e789. doi:10.1371/journal.pntd.0000789
He, J., He, J., Han, Z., Teng, Y., Zhang, W., and Yin, W. (2018). Environmental Determinants of Hemorrhagic Fever with Renal Syndrome in High-Risk Counties in China: A Time Series Analysis (2002–2012). Am. J. Trop. Med. Hyg. 99 (5), 1262–1268. doi:10.4269/ajtmh.18-0544
Jiang, F. C., Wang, L., Wang, S., Zhu, L., Dong, L. Y., Zhang, Z. T., et al. (2017). Meteorological Factors Affect the Epidemiology of Hemorrhagic Fever with Renal Syndrome via Altering the Breeding and Hantavirus-Carrying States of Rodents and Mites: a 9 years’ Longitudinal Study. Emerg. Microbes Infect. 6 (11), e104. doi:10.1038/emi.2017.92
Kallio, E. R., Klingström, J., Gustafsson, E., Manni, T., Vaheri, A., Henttonen, H., et al. (2006). Prolonged Survival of Puumala Hantavirus outside the Host: Evidence for Indirect Transmission via the Environment. J. Gen. Virol. 87 (8), 2127–2134. doi:10.1099/vir.0.81643-0
Klempa, B. (2009). Hantaviruses and Climate Change. Clin. Microbiol. Infect. 15 (6), 518–523. doi:10.1111/j.1469-0691.2009.02848.x
Knust, B., and Rollin, P. E. (2013). Twenty-year Summary of Surveillance for Human Hantavirus Infections, United States. Emerg. Infect. Dis. 19 (12), 1934–1937. doi:10.3201/eid1912.131217
Koo, T. K., and Li, M. Y. (2016). A Guideline of Selecting and Reporting Intraclass Correlation Coefficients for Reliability Research. J. Chiropractic Med. 15 (2), 155–163. doi:10.1016/j.jcm.2016.02.012
Li, C.-P., Huang, L.-Y., Cui, Z., Li, S.-L., Magalhaes, R. J. S., Zhang, W.-Y., et al. (2013). Association between Hemorrhagic Fever with Renal Syndrome Epidemic and Climate Factors in Heilongjiang Province, China. Am. J. Trop. Med. Hyg. 89 (5), 1006–1012. doi:10.4269/ajtmh.12-0473
Li, G., Zhou, M., Cai, Y., Zhang, Y., and Pan, X. (2011). Does Temperature Enhance Acute Mortality Effects of Ambient Particle Pollution in Tianjin City, China. Sci. Total Environ. 409 (10), 1811–1817. doi:10.1016/j.scitotenv.2011.02.005
Li, Q., Xu, Y.-G., Qi, S.-X., Zhang, Y.-B., Liu, Y.-Y., Han, Z.-Y., et al. (2012). Application of an Autoregressive Integrated Moving Average Model for Predicting the Incidence of Hemorrhagic Fever with Renal Syndrome. Am. J. Trop. Med. Hyg. 87 (2), 364–370. doi:10.4269/ajtmh.2012.11-0472
Li, R., Xu, L., Bjørnstad, O. N., Liu, K., Song, T., Chen, A., et al. (2019). Climate-driven Variation in Mosquito Density Predicts the Spatiotemporal Dynamics of Dengue. Proc. Natl. Acad. Sci. USA 116 (9), 3624–3629. doi:10.1073/pnas.1806094116
Li, S., Cao, W., Ren, H., Lu, L., Zhuang, D., and Liu, Q. (2016). Time Series Analysis of Hemorrhagic Fever with Renal Syndrome: A Case Study in Jiaonan County, China. PLoS One 11 (10), e0163771. doi:10.1371/journal.pone.0163771
Liu, J., Xue, F. Z., Wang, J. Z., and Liu, Q. Y. (2013). Association of Haemorrhagic Fever with Renal Syndrome and Weather Factors in Junan County, China: a Case-Crossover Study. Epidemiol. Infect. 141 (4), 697–705. doi:10.1017/s0950268812001434
Liu, Q., Liu, X., Jiang, B., and Yang, W. (2011). Forecasting Incidence of Hemorrhagic Fever with Renal Syndrome in China Using ARIMA Model. BMC Infect. Dis. 11 (1), 1–7. doi:10.1186/1471-2334-11-218
Liu, X., Jiang, B., Gu, W., and Liu, Q. (2011). Temporal Trend and Climate Factors of Hemorrhagic Fever with Renal Syndrome Epidemic in Shenyang City, China. BMC Infect. Dis. 11 (1), 331. doi:10.1186/1471-2334-11-331
Shrout, P. E., and Fleiss, J. L. (1979). Intraclass Correlations: Uses in Assessing Rater Reliability. Psychol. Bull. 86 (2), 420–428. doi:10.1037/0033-2909.86.2.420
Wood, S. N. (2006). Generalized Additive Models, An Introduction with R[M] England: Chapman & Hall/CRC.
Sun, J.-M., Lu, L., Liu, K.-K., Yang, J., Wu, H.-X., and Liu, Q.-Y. (2018). Forecast of Severe Fever with Thrombocytopenia Syndrome Incidence with Meteorological Factors. Sci. Total Environ. 626, 1188–1192. doi:10.1016/j.scitotenv.2018.01.196
Tian, H., Yu, P., Cazelles, B., Xu, L., Tan, H., Yang, J., et al. (2017). Interannual Cycles of Hantaan Virus Outbreaks at the Human-Animal Interface in Central China Are Controlled by Temperature and Rainfall. Proc. Natl. Acad. Sci. USA 114 (30), 8041–8046. doi:10.1073/pnas.1701777114
Witkowski, P. T., Klempa, B., Ithete, N. L., Auste, B., Mfune, J. K. E., Hoveka, J., et al. (2014). Hantaviruses in Africa. Virus. Res. 187, 34–42. doi:10.1016/j.virusres.2013.12.039
Wood, S. N. (2004). Stable and Efficient Multiple Smoothing Parameter Estimation for Generalized Additive Models. J. Am. Stat. Assoc. 99, 673–686.
Xiao, H., Tian, H. Y., Cazelles, B., Li, X. J., and Tong, S. L. (2013). Atmospheric Moisture Variability and Transmission of Hemorrhagic Fever with Renal Syndrome in Changsha City, Mainland China, 1991–2010. Plos Neglect Trop. Dis. 7 (6), e2260. doi:10.1371/journal.pntd.0002260
Xiao, H., Gao, L. D., Li, X. J., Lin, X. L., Dai, X. Y., Zhu, P. J., et al. (2013). Environmental Variability and the Transmission of Haemorrhagic Fever with Renal Syndrome in Changsha, People's Republic of China. Epidemiol. Infect. 141 (9), 1867–1875. doi:10.1017/s0950268812002555
Xu, L., Stige, L. C., Chan, K.-S., Zhou, J., Yang, J., Sang, S., et al. (2017). Climate Variation Drives Dengue Dynamics. Proc. Natl. Acad. Sci. USA 114 (1), 113–118. doi:10.1073/pnas.1618558114
Yan, L., Fang, L.-Q., Huang, H.-G., Zhang, L.-Q., Feng, D., Zhao, W.-J., et al. (2007). Landscape Elements and Hantaan Virus-Related Hemorrhagic Fever with Renal Syndrome, People's Republic of China. Emerg. Infect. Dis. 13 (9), 1301–1306. doi:10.3201/eid1309.061481
Zelená, H., Mrázek, J., and Kuhn, T. (2013). Tula Hantavirus Infection in Immunocompromised Host, Czech Republic. Emerg. Infect. Dis. 19 (11), 1873–1875. doi:10.3201/eid1911.130421
Zhang, W.-Y., Guo, W.-D., Fang, L.-Q., Li, C.-P., Bi, P., Glass, G. E., et al. (2010). Climate Variability and Hemorrhagic Fever with Renal Syndrome Transmission in Northeastern China. Environ. Health Perspect. 118 (7), 915–920. doi:10.1289/ehp.0901504
Zhang, W.-Y., Wang, L.-Y., Liu, Y.-X., Yin, W.-W., Hu, W.-B., Magalhaes, R. J. S., et al. (2014). Spatiotemporal Transmission Dynamics of Hemorrhagic Fever with Renal Syndrome in China, 2005-2012. Plos Negl. Trop. Dis. 8 (11), e3344. doi:10.1371/journal.pntd.0003344
Zhang, X. L., Zhang, Z., Xu, S. G., and Chen, Y. H. (1993). Occurrence and Control of Apodemus agrarius. China Plant Prot. Guide J. 3, 26–27.
Zhang, Y.Z., Yang, Z., Zhen, F. F., and Plyusnin, A. (2010). Hantavirus Infections in Humans and Animals, China. Emerg. Infect. Dis. 16 (8), 1195–1203. doi:10.3201/eid1608.090470
Zhang, Y. Z., Xiao, D. L., Wang, Y., Wang, H. X., Sun, L., Tao, X. X., et al. (2004). [The Epidemic Characteristics and Preventive Measures of Hemorrhagic Fever with Syndromes in China]. Zhonghua Liu Xing Bing Xue Za Zhi 25 (6), 466–469. doi:10.1016/j.csr.2003.12.006
Zou, L.-X., Chen, M.-J., and Sun, L. (2016). Haemorrhagic Fever with Renal Syndrome: Literature Review and Distribution Analysis in China. Int. J. Infect. Dis. 43, 95–100. doi:10.1016/j.ijid.2016.01.003
Zuo, S.-Q., Fang, L.-Q., Zhan, L., Zhang, P.-H., Jiang, J.-F., Wang, L.-P., et al. (2011). Geo-spatial Hotspots of Hemorrhagic Fever with Renal Syndrome and Genetic Characterization of Seoul Variants in Beijing, China. Plos Negl. Trop. Dis. 5 (1), e945. doi:10.1371/journal.pntd.0000945
Keywords: haemorrhagic fever with renal syndrome, Liaoning Province, meteorological factors, Prediction, lagged effect
Citation: Sun W, Liu Z, Liu Q, Li W and Lu L (2021) Forecast of Hemorrhagic Fever With Renal Syndrome and Meteorological Factors of Three Cities in Liaoning Province, China, 2005–2019. Front. Environ. Sci. 9:707960. doi: 10.3389/fenvs.2021.707960
Received: 11 May 2021; Accepted: 17 June 2021;
Published: 09 July 2021.
Edited by:
Sen Li, Huazhong University of Science and Technology, ChinaReviewed by:
Junyu HE, Zhejiang University, ChinaGuanshi Zhang, Huazhong University of Science and Technology, China
Copyright © 2021 Sun, Liu, Liu, Li and Lu. This is an open-access article distributed under the terms of the Creative Commons Attribution License (CC BY). The use, distribution or reproduction in other forums is permitted, provided the original author(s) and the copyright owner(s) are credited and that the original publication in this journal is cited, in accordance with accepted academic practice. No use, distribution or reproduction is permitted which does not comply with these terms.
*Correspondence: Liang Lu, bHVsaWFuZ0BpY2RjLmNu
†These authors have contributed equally to this work