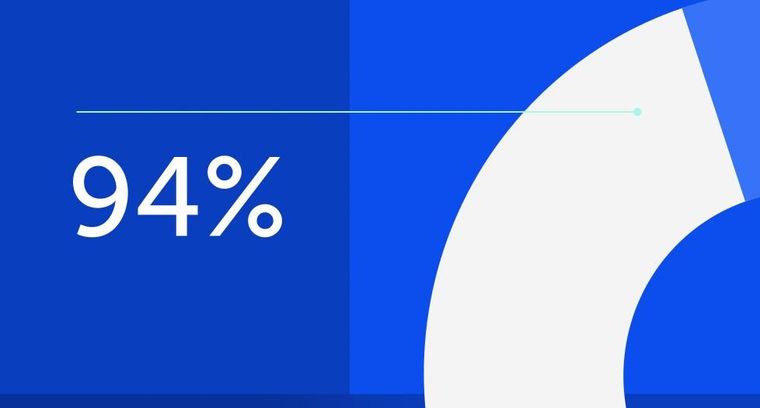
94% of researchers rate our articles as excellent or good
Learn more about the work of our research integrity team to safeguard the quality of each article we publish.
Find out more
ORIGINAL RESEARCH article
Front. Environ. Sci., 04 June 2021
Sec. Environmental Informatics and Remote Sensing
Volume 9 - 2021 | https://doi.org/10.3389/fenvs.2021.667018
This article is part of the Research TopicFood-Energy-Water Systems: Achieving Climate Resilience and Sustainable Development in the 21st CenturyView all 21 articles
Interconnected food, energy, and water (FEW) nexus systems face many challenges to support human well-being (HWB) and maintain resilience, especially in arid and semiarid regions like New Mexico (NM), United States (US). Insufficient FEW resources, unstable economic growth due to fluctuations in prices of crude oil and natural gas, inequitable education and employment, and climate change are some of these challenges. Enhancing the resilience of such coupled socio-environmental systems depends on the efficient use of resources, improved understanding of the interlinkages across FEW system components, and adopting adaptable alternative management strategies. The goal of this study was to develop a framework that can be used to enhance the resilience of these systems. An integrated food, energy, water, well-being, and resilience (FEW-WISE) framework was developed and introduced in this study. This framework consists mainly of five steps to qualitatively and quantitatively assess FEW system relationships, identify important external drivers, integrate FEW systems using system dynamics models, develop FEW and HWB performance indices, and develop a resilience monitoring criterion using a threshold-based approach that integrates these indices. The FEW-WISE framework can be used to evaluate and predict the dynamic behavior of FEW systems in response to environmental and socioeconomic changes using resilience indicators. In conclusion, the derived resilience index can be used to inform the decision-making processes to guide the development of alternative scenario-based management strategies to enhance the resilience of ecological and socioeconomic well-being of vulnerable regions like NM.
The availability and consumption of food, energy, and water (FEW) resources heavily rely on one another, and there are numerous ways in which these three systems overlap and interconnect. For example, food production accounts for ∼30% of global energy consumption and ∼92% of the human water footprint (Finley and Seiber, 2014); 15% of global water withdrawals are used for energy production (IEA, 2012) and 70% for food production (Ritchie, 2017); 3% of global electricity is required for water provision (Liu et al., 2015) and 6% for food production (Ritchie, 2017). However, currently, about 0.8 billion people are hungry (FAO, 2017), 1.4 billion people have no access to energy (Alstone et al., 2015), and 0.7 billion people have no access to water (FAO, 2011). The demand for FEW resources is projected to increase, further adding management, sustainability, and resilience challenges as there is a need to increase food production by ∼70% (FAO, 2014; World Bank, 2016) along with 57% more water (WWAP, 2015) and 40% more energy (OECD, 2012; World Energy Outlook, 2014; Rasul, 2016) in the next 20 years. The combination of factors that include global population growth, which is projected to grow from 7.7 billion people in 2019 to 9.7 billion in 2050 and 10.9 billion in 2100 (UNDES, 2016), increased economic development, and rapidly changing climate amplifies the scarcity of water supply, the decline in food production, and the depletion of fossil fuels that humans heavily rely on for socioeconomic development (Gerland et al., 2014; Karandish and Mousavi, 2016). Providing a framework that allows proper modeling and evaluation of FEW system components is a first step toward improving the ability to better manage and allocate these resources.
These factors which can generally be grouped into two categories—socioeconomic and climate change—act as external drivers of vulnerability of FEW systems to related disturbances and shocks (Wisner et al., 2004). Major challenges to FEW systems are those imposed by climate change–induced extreme events, such as droughts (Mpandeli et al., 2018), increased temperature, and variable precipitation patterns, especially in semiarid regions with low adaptive capacity [e.g., New Mexico (NM) in the Southwest United States (US)] (Niang et al., 2014). Increased temperatures and declining precipitation can increase the demand for water supply—mostly for irrigation to ensure food security (FAO, 2012), lead to environmental degradation, and eventually deteriorate human livelihood, well-being, and economic development (Sun and Yang, 2016). Additionally, significant socioeconomic changes (e.g., food and energy prices) can influence the availability and accessibility of FEW resources, making large portions of the population unable to afford and access these basic needs (Mohtar and Daher, 2010; Ringler et al., 2016). The effects of these external drivers are complex, span the three FEW nexus components, and can drastically affect the sustainability and resilience of FEW resources.
As it is important to provide an improved understanding of the functionality of individual components of FEW systems, it is also critical to have an elaborate characterization of the interconnections and behavior of these systems as a nexus as they evolve and transform these climatic and socioeconomic setbacks (Grafton et al., 2017; Rosa et al., 2017; Scanlon et al., 2017; D’Odorico et al., 2018; Nhamo et al., 2018; Rosa et al., 2018). These interconnections are among the most important ones of nature that are essential for human well-being (IRENA, 2015; EIA, 2018; Nhamo et al., 2018; Ding et al., 2019). A distinct framework is required to investigate FEW system dynamics and assess the resilience of such coupled natural–human systems (Carpenter et al., 2001; Gunderson and Holling, 2001; Holling, 2001; Folke, 2006). The scope of this conceptual analysis was to develop a framework to assess FEW system relationships, identify external drivers, and conduct a threshold-based resilience evaluation.
The resilience challenges related to FEW systems and human well-being are particularly amplified in semiarid regions such as the state of NM in the southwest of the United States. NM, with its unique characteristics, can be considered as an informative case of FEW nexus and human well-being. NM has recently experienced frequent and prolonged drought events (Gonzalez et al., 2018), and it is projected to experience extremely high–water scarcity conditions in the future (WRI, 2015). NM is a major fossil fuel (e.g., crude oil and natural gas) producer, and the energy sector significantly supports its economy. The resilience of NM’s FEW nexus is challenged due to the increased pressure on its water resources, food production (Sawalhah et al., 2019; Zaied et al., 2019; Zaied et al., 2020), economic shocks from energy markets, and fluctuations in ranchers’ and farmers’ income. These NM challenges further highlight the need to appropriately conduct qualitative and quantitative analysis, and integrate and evaluate the dynamic behavior of FEW systems to enhance their resilience.
This study used the “nexus” concept because it has emerged as an effective means to address these challenges by properly describing the complex linkages and inseparable interactions between multiple distinct but interconnected systems (McGrane et al., 2019). The concept has long been used in philosophy, cell biology, and economics. It was introduced to natural resources disciplines in the Food–Energy Nexus Programme (Sachs and Silk, 1990), to provide integrated solutions to food and energy scarcity (McGrane et al., 2019). It was highlighted at the Bonn 2011 Nexus Conference to promote the understanding of FEW resources, provisioning basic needs, and ensuring security (Hoff, 2011). The nexus concept can be defined as an integrated systems approach to qualitatively describe and identify interconnected subsystems and to quantitatively account for their functionality and resource availability, enhance their synergistic use, and minimize trade-offs with the main goal of sustaining the well-being of human societies and resilience of FEW systems. The concept has been explored to promote effective management of FEW resources qualitatively and quantitatively (Hoff, 2011; Fischer et al., 2015; Keairns et al., 2016; Scanlon et al., 2017), for example, to assess the effects of irrigation practices on water resources (de Vito et al., 2017; Cai et al., 2018), assess human livelihood under climate and socioeconomic impacts (Ding et al., 2019), and integrate FEW and human systems to promote environmental security and sustainability (Biggs et al., 2014; Biggs et al., 2015; de Grenade et al., 2016).
Most previous research efforts that focused on qualitative and (to some extent) quantitative assessments of the FEW nexus lacked modeling of its dynamic behavior (Keairns et al., 2016; Albrecht et al., 2018; Givens et al., 2018). A clear quantitative description is required to adequately integrate such coupled natural–human systems, develop effective management decisions (Ramankutty et al., 2018; Rosa et al., 2018; Mohtar and Daher, 2019), and improve resource use efficiency and synergies (Kan et al., 2016; Fang and Chen, 2017). However, most traditional modeling approaches may not be suitable for such complex systems (CE, 2010; Bazilian et al., 2011; ADB, 2013; WB, 2013; NSF, 2014) because their parametrization limits their application to local scales (Miralles-Wilhelm, 2016); hinders their ability to integrate more than two subsystems (Dubreuil et al., 2013); and lack in coupling socioeconomic systems. On the other hand, these limitations provide the opportunity to apply system dynamics (SD) (Forrester, 1994; Sterman, 2000) which allows characterizing the dynamic behavior systems. The SD approach is unique for integrating physical (e.g., FEW) and socioeconomic (e.g., employment) systems (Bagheri and Hjorth, 2007; Winz et al., 2008; Tidwell et al., 2018). An SD model can help visualizing the interrelationships between discrete sectors, the dynamic changes, and the interdependences among FEW systems (Simonovic and Fahmy, 1999). It also allows the use of scenario-based analysis to simulate past, current, and forecast future resource availability and consumption (Fiksel, 2003; Bieber et al., 2018; Laspidou et al., 2020).
It is important to monitor the dynamic behavior of the FEW nexus over time in response to disturbances. Ecological (e.g., FEW) and social (e.g., human society) systems can, mostly, adapt to external stresses over time until a resource availability threshold is surpassed, beyond which these systems can undergo significant shifts—either transform into a new equilibrium state (Angeler and Allen, 2016) or become nonresilient (Angeler and Allen, 2016). The temporal behavior of these systems can be effectively captured using the resilience concept that was introduced in the field of ecology beginning in the 1970s (Holling, 1973). Resilience thinking is a generic approach that has been increasingly adopted to understand socio-ecological systems (Carpenter et al., 2001; Folke et al., 2002; Folke, 2006). Achieving resilience across FEW and human systems signifies the amount of disturbance that these systems can withstand before shifting into a new stable state. It is important to identify the factors that allow maintaining resilience and those that can introduce risks and undesired resource scarcities.
Additionally, performance indicators that couple FEW and human well-being (HWB) systems need to be developed. HWB is a subset of social well-being and economic growth that can be measured using a number of indicators such as employment, education, and income, among others (OECD, 2012). FEW and HWB systems can seamlessly be integrated using their relevant indices—FEW Index and Human Well-being Index (HWBI), respectively. The goal of this analysis was to develop an integrated framework referred to as FEW-WISE including FEW, human Well-beIng, and reSiliEnce. The objectives were to 1) provide an improved characterization of the integrated relationships in FEW systems, 2) provide a quantitative assessment approach for the FEW nexus based on relevant indices and thresholds that can identify its dynamic equilibrium status, and 3) propose a resilience simulation framework to assess the response of FEW resources in the presence of external stresses. These objectives can be achieved using the FEW-WISE framework that consists of five steps (FEW-WISE Framework section below). In this article, the first two steps were described in detail to lay out the baseline information needed to model and conduct indicators-based analysis. The other three steps were individually conceptualized and will be described in separate modeling, indices, and resilience assessments. The SD modeling results, predicted parameters of FEW nexus, and resilience index will be reported separately in a follow-up article that shows the application of the FEW-WISE framework. This framework was developed within the context as part of an INFEWS project using NM as a case study but can be generalized for other vulnerable regions with similar conditions.
NM encompasses a large geographic area with diverse interior–continental ecosystems, including mountain ranges, forests, grasslands, and deserts (NOAA, 2017). NM population has shown a sustained growth during 2001–2005 after a leveled growth around the 2000 Census (BBER, 2008). However, NM has experienced a slow growth in population during 2010–2019 when most of the counties saw a decrease in population (NMEDD, 2010); migration and economic trends provide real historical impetus toward population dynamics (USBC, 2019). NM has a considerable reserve of fossil fuel, mineral, and renewable energy resources. Crude oil and natural gas place the state among the top 10 energy producing states in the United States (EIA, 2019; EIA, 2020). NM’s abundant land makes it the fifth largest state in the United States with 314,850 m2 of land of which 175,230 m2 are classified as farms and ranches with 88% of this area identified as rangeland (Figure 1) (Goodwin and McDermott, 2017).
FIGURE 1. Study area showing county-wise distribution of food–energy–water systems and climate over New Mexico that includes livestock production (NASS, 2015), rangeland cover (USFS, 2019), total crops sold (NASS, 2015), total water withdrawal (Molly et al., 2015), Oil & Gas production in Oil Conservation Division (OCD) Districts, and 30-year (1981–2010) mean annual temperature and rainfall (PRISM, 2010).
NM has an arid to semiarid climate and is ranked as the fifth driest state in the United States. NM average annual precipitation is ∼380 mm (1895–2019) and ranges from less than 254 mm over much of the southern region to more than 508 mm at higher elevations (NOAA-NCEI, 2020). NM temperatures vary widely with a monthly average temperature in the northern mountainous regions ranging from 6.7°C in January to 15.6°C in July, while in the lower elevations in the south, the range is from 4.4°C in January to 26.7°C in July. The state has a limited and variable water supply due to its normally dry conditions and frequent droughts that add challenges to explore its full potential in food and energy resources, thus affecting the sustainability of these systems and New Mexicans’ livelihood and well-being.
The major industries in NM include agriculture and energy. Within the agricultural sector, NM is known for its considerable production of livestock (e.g., beef), diary (e.g., milk), and crop (pecan and hay) commodities. The livestock industry is an integral economic component for NM that adds ∼ $2.5 billion to its gross state product (GSP) and employs over 32,000 workers as of 2012 (Diemer et al., 2012). Most irrigated lands are located along the Rio Grande River corridor running from north to south through the middle of the state. The energy sector provides significant revenue to the state, which then supports New Mexicans in different ways (e.g., schools, hospitals, and state government and other services) (EMNRD, 2015). NM has plentiful energy resources (fossil fuels and renewables) that make it the eighth largest energy producer in the United States with more than 6 and 4% of the United States total proved crude oil and natural gas reserves in 2018, respectively (EIA, 2018). This wealth of energy resources also creates economic development opportunities, from attracting manufacturing to additional opportunities for energy exports. Both food and energy production are water-intensive activities.
The FEW-WISE framework follows five steps: 1) qualitatively identify the relationships between FEW nexus components and quantitatively assess resource exchanges, 2) identify the drivers of the systems, 3) integrate FEW systems using SD models to evaluate their response to the identified drivers, 4) develop FEW and HWB performance indices, and 5) develop a resilience monitoring criterion using a threshold-based approach that integrates these indices (Figure 2). The qualitative and quantitative assessments of NM’s FEW nexus along with the external drivers can be used as inputs to simulation and prediction of FEW systems’ temporal response to identified changes and can be used to develop the corresponding FEW and HWB resilience indices at the county and state levels. These five steps are discussed and explained in more detail in NM FEW Nexus Description to Resilience section below.
FIGURE 2. Integrated FEW-WISE framework that couples food–energy–water systems and drought impact, socioeconomic, and human well-being components.
To operationalize the analysis of NM FEW systems, the interactions between FEW subsystems under external drivers were first qualitatively characterized, as depicted in Figure 3. Such depiction was needed to promote cross-sector collaboration, develop coherent decisions, and enhance HWB and resilience (Keskinen et al., 2016). This qualitative description of NM FEW systems was based on resource use, for example, food commodities produced using NM land, water, and energy. Much of the food commodities produced in NM are exported, and thus, additional supplies are imported to meet local demands.
FIGURE 3. New Mexico food–energy–water (FEW) nexus describing the relationships and interconnections of its components under climate and socioeconomic drivers.
In NM, energy is used in harvesting, production, processing, and transportation of agricultural (e.g., crop and livestock) commodities. Likewise, agricultural by-products (e.g., biofuels and biopower) can be used, to a limited extent, to generate energy. The important variables that link these two sectors were identified based on a few factors including the total amount and economic value of production as well as the amount of resources used or needed from the other sector to produce these variables.
NM food production systems include crops and livestock commodities. Major crops include field crops (e.g., hay, winter wheat, sorghum, corn, cotton, peanuts, and dry beans), vegetables, and nuts (e.g., chili, onions, and pecans). In 2017, the total crop production in equivalent metric tons was about 4.012 million (NASS, 2018). Corn (grain and silage) accounted for about 53%, followed by hay (all types) and onions about 28 and 6% of the total production, respectively. While pecans accounted for only 1% of the total crop production by weight, its economic value outpaced that of corn and hay (Figure 4). NM was among the largest producers of pecans (a high value cash crop) as the state ranked second in 2017, with a 28% increase from 2016. In 2018, NM surpassed Georgia in pecan production for the first time and ranked as the top producer nationally. Most of the pecan acreage is in Doña Ana county, followed by Eddy and Chavez counties (NMDA, 2018).
NM livestock production systems consist of beef cattle, dairy, sheep, and lambs. Except for dairy, most of NM livestock production depends on pasture and rangelands. Based on January 2018 inventory, the total number of all cattle and calves was ∼1.51 million and that of milk cows was ∼483 thousand. The economic value of dairy production (mainly milk) was more than that of all meat animals as they accounted for 41.4 and 31.5% of the total cash receipts, respectively, of all agriculture commodities (NASS, 2018). Nationally, in 2012, NM was ranked ninth for the value of milk sold and the number of milk cows. Most of this production occurs in Chavez, Curry, and Roosevelt counties.
In terms of energy production, NM has a diverse energy portfolio, and it is considered a major producer of crude oil and natural gas as it was ranked third and eighth in the United States in 2020, respectively. NM natural gas and crude oil account for close to one-tenth and over 3% of the United States total production, respectively. Major crude oil and natural gas reserves are in the Permian Basin in the southeast and in the San Juan Basin in the northwest (see oil and gas production districts map in Figure 1). The Permian Basin has supplied more than 5.7 billion m3 of crude oil and about 3.75 trillion m3 of natural gas as of January 2020. As of 2018, the U.S. Energy Information Administration (EIA) estimated the remaining proven reserves in the Permian Basin exceed 11 billion barrels of crude oil and 1.38 trillion m3of natural gas, making it one of the largest hydrocarbon-producing basins in the United States and globally (EIA, 2019). In 2010, EIA ranked the combined San Juan Basin Gas Area in Colorado and NM as the second largest natural gas field in the United States in terms of proven reserves, with a production of 54 billion m3 in 2019. Other sources of energy in NM include coal and renewables (e.g., geothermal, hydroelectric, biomass, wind, and solar) but have minimal contribution to the state’s total energy production. NM is the seventh largest net supplier of energy in the United States, with a total annual production of 2,820 trillion Btu in 2017 (mostly crude oil and natural gas).
The total demand for energy by the agriculture sector was 1,700 trillion Btu in 2009 (all sources of energy), which decreased to 1,500 trillion Btu in 2012, and has been increasing since 2014 reaching 1,714 trillion Btu accounting for about 1.74% of the total U.S. primary (e.g., coal, natural gas, and oil) energy consumption (Hitaj and Suttles, 2016). The secondary form of energy (e.g., electricity) is also used at all the stages of food production, including pumping water for irrigation (7.5% US cropland and pastureland were irrigated using electricity in 2007), powering tractors for tillage and harvesting; transporting and distributing food products, and for heating and cooling in livestock activities (23.7% in the year 2002) (Miranowski, 2005; Nord et al., 2005).
Fossil fuels are used throughout the feed chain of the livestock production systems (production, transportation, storage, processing), farm operations (machinery, equipment, climate control), and products chain (transportation, processing, storage) (Sainz, 2003). (Patrick, 1977) estimated the energy demand for dairy, range beef, and feedlots beef productions as 57.56 million Btu, 57.17 million Btu, and 39.30 million Btu per animal unit, respectively. NM dairy production systems consistently showed the highest energy demand. NASS (2018) reported 465,000 beef cattle head and national average beef cow weight as 608 kg for the year 2017 that is equivalent to 6,28,215 animal unit (AU). Energy requirement of beef cattle in the year 2017 is approximately 60,652 billion Btu.
Energy requirements of alfalfa, corn, wheat, and sorghum were estimated using energy requirements reported by Patrick (1977) using NM crop yields of the year 2018. The energy requirements of crop (production) for alfalfa (4,230 kg), corn grain (4,712 kg), sorghum (957 kg), and wheat (405 kg) are expected to be around 15.8, 27.4, 8.0, and 4.2 million Btu, respectively. Overall, NM energy consumption varies widely by sectors defined by the EIA (Figure 5). NM agricultural industries spent nearly $90 million for petroleum fuel and oils and another $49.6 million for electricity in 2016 to power farm equipment, manufacture fertilizer, and transport products (CEA, 2016). The transportation sector is the largest energy consumer in NM, followed closely by the industrial sector, where more energy is consumed per capita than in three-fourths of the United States (EIA, 2017) (Figure 5).
Generally, energy production relies on water availability, and the supply and distribution of water require energy (King et al., 2008; Sanders and Webber, 2012). In NM, extraction of energy resources including crude oil and natural gas involves the use of hydraulic fracturing technology—a process of high-pressure injection of a fluid mixture that consists of water, sand, and chemicals into bedrock formation for increased production. Also, power generation is a major source of water consumption (e.g., mainly electricity) for cooling (specifically coal and natural gas). There are no nuclear power generation plants in NM. Minimal amounts of water are used in other mining activities. On the other hand, energy is needed for pumping, conveyance of water for agriculture (from surface and/or groundwater resources), purification, treatment, and distribution of freshwater and wastewater, and other domestic uses at the household level (e.g., water heating and laundry).
To account for how much water is used for energy production, it is important to evaluate the exchange of quantities between these two systems. NM water supply depends on surface and groundwater resources relatively equally to meet its demands. During a typical year, almost half of NM water comes from groundwater aquifers (Longworth et al., 2019)—about 2.1 billion m3 per year, pumped from the five major freshwater aquifers underlying NM. More groundwater pumping may occur during drought years to make up for the deficit in surface water supply. Groundwater withdrawal is more energy-intensive than that of surface water. NM uses about 12.87 billion m3 per day (Maupin et al., 2010) as mining and irrigation account for 1 and 76%, respectively, of total groundwater use (Longworth et al., 2013) (Figure 6). In NM, about 1.65 billion m3 of water were applied to irrigate 2.73 billion m2 in 2018 (US DOE, 2014).
The growing practice of multistage hydraulic fracturing has increased the amount of water used per well in recent years, which, in NM, can range from 1.9 to 30 million liters per well. While this amount of water used for energy extraction (crude oil and natural gas) represents a small fraction of the total water use, it can trigger local stress on freshwater supply in high-production areas. Withdrawal in all water use categories combined was about 3.84 billion m3, of which surface water and groundwater accounted for 52.34 and 47.66%, respectively. In 2015, water use under the mining category accounted for 52.17 million m3 (51.26 million m3 in 2010) (EIA, 2017). Power generation accounted for 71.96 million m3 in 2010 compared to 72.06 AF (1.62%) of total water use in 2015. Compared to 2015, power generation in 2010 used 81.3 and 18.7% surface and groundwater, respectively, out of the 71.96 million m3. Mining activities use mostly groundwater (∼97% in 2015), while power generation uses mostly surface water (∼79% in 2015) (Longworth et al., 2013). However, in the United States, irrigation accounted for 42% of freshwater withdrawal in 2015 as compared to 84% in NM. Therefore, the data about the nature and volume of the aquifers are important to manage NM water resources, economics, and environment.
To represent the variation in water use for energy production, water intensity of the power generation variable can be developed and used to account for the combined water use in mining and power generation. For the United States, water intensity of the total power generation was about 57.2 L per kilowatt-hour in 2014 (49.2 L per kilowatt-hour in 2017). Water intensity referred to here is the average amount of water withdrawn per unit of total net electricity generated. The total energy used for irrigation and sprinkler operation accounted for 1,160 GWh or approximately 3% of NM’s total energy use in 2011. This estimate was based on acres of land using groundwater and sprinklers. In 2010, the total water used for energy production accounted for about 3% of NM’s overall withdrawal from surface and groundwater sources (Tatro, 2018). The amount of energy needed for public supply was about 26 MWh of electric power for groundwater pumping from an average depth of 150 m.
NM’s water, which supports its crop and livestock production and food processing, is limited due to variable precipitation and recurring drought conditions that consequently create production uncertainties, inhibit farmer and rancher livelihood, and can result in more fallow land or land transitions out of agriculture. For the last half century, water use by agriculture was roughly evenly split between groundwater and surface water. However, in 2013, out of 1.62 billion m3 irrigation water, 0.99 billion m3 ground water from wells, 0.18 billion m3 on-farm surface water, and 0.45 billion m3 off-farm water from other sources were used (USDA, 2013).
In addition to the water for food needs for growing crops, water is also essential for livestock production—beef cattle and dairy are an important source of food in NM. Globally, irrigated crops and raising animals consume ∼70% of the total freshwater. Livestock production needs water for animal watering, growing feeds, and on-farm needs such as cleaning, sanitation, cooling, and waste disposal systems; however, direct water consumption for drinking accounts only for 0.5% of the total livestock water footprint (Sawalhah et al., 2021). Most water requirement in livestock production is to grow feeds (95% of the total water footprint). In NM, 28,203 L of green (precipitation) and blue (surface and ground) water are required to produce one kilogram of beef, where blue water accounted for only 18% of the total water footprint (Sawalhah et al., 2021). In 2015, livestock production accounted for 1.1% from NM total freshwater withdrawals compared to 82.5% withdrawals by irrigation (USGS, 2015). The highest annual blue water footprint in NM’s livestock production is associated with dairy cows. Marston et al. (2018) reported that dairy cows, beef cattle, sheep, goats, and hogs consumed around 23, 597; 20, 610; 247; 78; and 7 thousand m3 per year, respectively. Additionally, more water is being used to produce beef than anything else humans eat, requiring 15, 415 liters of water (primarily to grow feed for animals).
Crops also affect natural flow regimes, yet these effects are not well-understood. Return flow from irrigation systems (nonconsumptive portion of water withdrawals) affects flow balance and water use accounting at a basin scale (Cai et al., 2003; Ochoa et al., 2020). Determining return flow, especially the utilizable return flow volume, is important for not only understanding water balance in streams and aquifers but also determining water availability for the development of more reasonable (and sustainable) water rights at the river basin scale (Grafton et al., 2012). For instance, the conversion of water-intensive crops to low-water crops (e.g., sorghum) has been identified as one of the effective methods to ease pressure on an increasingly limited water supply. Water managers face a continual challenge to meet the needs of multiple users.
FEW nexus components act as endogenous factors influencing one another (Chang et al., 2016). External (exogenous) drivers can simultaneously affect their behavior; thus, they need to be considered to address resilience challenges in terms of opportunities and trade-offs. External drivers can include climate variables and related extreme events—that is, temperature, precipitation, and drought—and socioeconomic stresses such as economic growth, population growth, poverty, political stability (Hameed et al., 2019). They can directly be linked in the FEW-WISE framework through multiple interactions and feedbacks across spatial and temporal scales (Chenoweth et al., 2011; King and Jaafar, 2015; Scanlon et al., 2017), thus creating risks and management challenges.
NM annual precipitation showed a slight decreasing trend, while an increasing trend in mean annual temperature was observed for the period between 1895 and 2019 (Figure 7A). This combination can have a profound negative impact on mountain snowpack that feeds water supply reservoirs by reducing water flow to the river basins and thus affecting water availability during the growing season. Even if snowpack accumulation was not to decrease, the projected higher temperatures will lead to an earlier initiation and end of snowmelt, potentially necessitating changes in water management.
FIGURE 7. (A) Annual mean precipitation and temperature of the United States and New Mexico from 1895 to 2019 (Source: www.ncdc.noaa.gov/temp-and-precip/) and (B) Elephant Butte Reservoir storage levels (m3) in New Mexico from 1915 to 2020 (Source: USBR, 2021).
Multiyear periods of high and low precipitation have resulted in very large swings in reservoir water supplies for agriculture. For example, the water levels in the Elephant Butte Reservoir were high from the 1920s to the 1940s before dropping to low levels during the mega drought of the 1950s until the 1980s. High levels remained throughout the 1980s and 1990s until falling again in the first part of the 21st century to about 10–15% of its capacity (Figure 7B).
NM’s precipitation and temperature trends (Figure 7A) demonstrate that the deficits between potential evapotranspiration from freshwater bodies or vegetated surfaces and precipitation are increasing. This increased deficit can reduce streamflow and reservoir storage (Figure 7B) and increase drought severity (Figure 8). With persisting drought conditions, farmers are increasingly dependent on pumping groundwater, rather than surface water sources (e.g., reservoirs and streams) to irrigate their crops and make up for the deficit that results in high production costs. Subsequently, the variable water supply threat has led farmers to plant more drought-resistant crops like beans, or to abandon their fields altogether. In NM, the most extensively pursued in terms of agriculture land area used is livestock grazing. Due to increased variability in precipitation, the amount of water is not sufficient for the optimum growth of forage on rangeland. Thus, this limited and variable rangeland productivity has resulted in reduced feed for livestock, and some ranchers adopt risk-averse strategies of selling their cattle to reduce the cost of buying additional feed supplements (e.g., hay) (Holechek et al., 2020; Gedefaw et al., 2021). At the same time, ranchers significantly reduce cattle herd sizes, allowing grasslands to recover from drought conditions (Uyttebrouck, 2013).
The southwestern United States is particularly vulnerable to drought, and even a small decrease in water availability in this already arid region can stress natural systems and further threaten water supplies. Drought poses a persistent risk to NM, added to its normally arid to semiarid climatic conditions. Drought events have broken historical records in recent years (NOAA, 2017). The extended record indicates that droughts were frequent in NM and more severe in recent years (Figure 8) (NOAA, 2017; Johnson et al., 2020), and this trend is projected to continue (Schwalm et al., 2012).
Drought can directly and indirectly disrupt the state’s most vulnerable economic activities including farming, ranching, and other sectors linked to agriculture. Recent extreme droughts have negatively impacted NM ecosystems such as the Chihuahuan Desert, causing grassland degradation and resulting in reduced grazing capacity for livestock (Gedefaw et al., 2021). Indirect impacts include, for example, reduced employment opportunities and increased agricultural input purchases. Drought also has indirect economic impacts on other sectors including public water supply, industry (e.g., mining, crude oil, and natural gas production), tourism and recreation, and tribal economies. Recurrence of a multiyear severe drought like that of the 1950s would have greater impacts on the food and water resources and the economy of the state than in the 1950s because of warmer temperatures, population growth, and increased demand for water since the 1950s. Nevertheless, climate change will affect NM food systems and could undermine food security by reducing farmlands by 20–25% and irrigation water by more than 90% (USEPA, 1998; Voiland, 2013).
NM experiences lack of funding for short- and long-term water planning, which can increase water sustainability risks compounded by the general lack of awareness of predicted future declines in water availability (Gonzalez et al., 2018). NM is not alone as it is facing the same challenges experienced in the Western United States with increasing demand, reduced supply, and an inadequate legal and regulatory framework that was set up over 115 years ago when the conditions were different. There is a concern that the lack of funding and appropriate efforts will result in prolonged periods of extremely low water flow as warmer temperatures increase water losses due to increased evaporation. With increased reliance on groundwater, deeper groundwater wells would be needed to accommodate declining groundwater table, posing additional accessibility challenges and risks as groundwater often contains higher levels of salinity and other minerals that can reduce crop production and increase soil contamination (Frisvold et al., 2013).
NM FEW nexus functionality has direct and indirect impacts on the state’s revenue and expenses, employment, and gross development product (GDP). Specifically, NM energy and food sectors have wide-ranging benefits to the state economy and New Mexican’s livelihood and well-being. Together, agriculture and food processing support almost 50,000 jobs and contribute $10.6 billion to NM gross state product (GSP) in 2012 (Diemer et al., 2012). The agriculture and food processing industries directly created 32,578 jobs and 18,308 jobs in related support activities for a total of 50,886 jobs statewide (Diemer et al., 2012). These socioeconomic indicators have varied over the years based on several factors that include price fluctuations (crude oil and natural gas) and natural hazards such as drought. For example, the economic well-being of farmers and ranchers, which can be evaluated using net gains and losses in cash income, decreased by 36% between 2007 and 2012. Also, from 2002 to 2012, the number of farms in the United States with net losses increased by 0.2%, while that for NM increased by about 81.4% (New Mexico First and New Mexico State University, 2016).
The total value of the NM agriculture sector in 2018 was ∼$3.17 billion, while that of livestock production was $2.18 billion (NASS, 2018). Beef cattle and dairy are the most important agricultural activities in NM, contributing ∼42 and 56%, respectively, in cash receipts (NASS, 2016). Major portions of NM agricultural and processed food products (∼97%) and cattle (∼99%) are processed out of the state (NMDA, 2010). To meet NM consumers domestic demands, locally produced agricultural products of $13 million were sold directly to consumers in 2010. The prices of these food commodities coincided with higher energy consumption required for their production and processing.
Crop production requires significant direct (fuel and electricity) and indirect (fertilizers and pesticides) energy inputs that are mostly based on crude oil and natural gas. Price fluctuations of these energy sources directly impact agricultural production. Energy prices during 2011–2014 were 20 times higher than those of the 1970s and can be largely attributed to a combination of increasing global demand and increasing market fluctuations (Figure 9A). On the other hand, a huge drop in crude oil prices from June 2014 to March 2016 negatively impacted NM’s economy through declines in revenue that ultimately affected services provided to New Mexicans and resulted in a state fiscal crisis (Figure 9B).
FIGURE 9. (A) Prices of crude oil and natural gas from 1967 to 2019 (Source: U.S. EIA, 2020) and (B) Unemployment rate from 1976 to 2020 in New Mexico (source: U.S. BLS, 2021).
Regarding the energy sector, price fluctuations have a direct impact on the state’s economic growth and stability. During 2012–2014, there was considerable economic growth that was attributed to increased crude oil and natural gas revenues primarily because of increased prices and production (EMNRD, 2015). Depending on the demand and prices, NM generally receives over $2 billion annually in direct revenue from crude oil and natural gas industry through severance taxes, property taxes, and royalty and rental income. Additional indirect income comes from sales and income taxes on crude oil and natural gas drilling and services, which can generate ∼$300 million. In NM, the revenue generated by the crude oil and natural gas industry can directly and indirectly impact New Mexicans in multiple ways as it contributes about 35% of funding toward the state’s public education, health, and other services.
Since the economic downturn of 2007, NM GDP has increased by 20% until 2017. The GDP in 2017 of $94.2 billion increased by 1% from that of 2016 (Moskowitz, 2017; BEA, 2018) and by 3.7% in the year 2019 from that of 2018. On average, the total impact of agricultural production is about 7.4% of NM GDP and that of food processing is about 5.7%. These two broad industries accounted for $10.6 billion (around 12.3%) toward NM GDP by state of $86.5 billion in the year 2012. The agriculture sector provided 41,961 jobs in NM in 2012 (Diemer et al., 2012). Figure 10 shows the contribution of different industries to NM GDP 2019.
In 2017, the largest contributor (∼7% of $11.3 billion) to real GDP growth was natural gas and crude oil production (BEA, 2018). Mining, quarrying, and crude oil and natural gas extraction were the state’s largest growth sectors, contributing about 2.2% to NM GDP and 1.7% to GDP change from 2018 to 2019, while this only contributed about 0.33% to the GDP of the entire United States. One-third of the annual revenues and contributions from crude oil and natural gas industry are used to provide services in NM. Energy-related high-paying jobs are estimated as 6.4% of employment in the state (EMNRD, 2019). Accounting for the impacts of FEW nexus on New Mexicans’ well-being needs to consider the abovementioned factors to develop resilient social life before, during, and after climate extreme events and socioeconomic stresses.
The SD approach is defined as “the study of the information–feedback characteristic of industrial activity to show how organization structure, amplification, and time delays interact to influence the success of the enterprises” (Forrester, 1958; Forrester, 1961). It has been used since the 1950s in diverse applications including economics, sociology, ecology, and engineering. It is based on the notion of system thinking (Forrester, 1961; Sterman, 2000) and allows to comprehensively review the structure and dynamics of complex systems (Tenza et al., 2017). It emerged as an innovative approach to facilitate holistic analysis of coupled human–environmental systems such as FEW systems (Kotir et al., 2016; Turner et al., 2016; Tenza et al., 2017; Xu and Szmerekovsky, 2017).
SD has the ability to integrate disparate systems such as FEW and HWB (Green et al., 2011; Qin et al., 2011; Shannak et al., 2018; Sušnik et al., 2018). It was therefore proposed to develop the FEW-WISE framework to identify the interactions between FEW’s drivers at multiple spatial and temporal scales (Dietz et al., 2003). Specifically, development of SD models uses causal loop diagrams (CLD) to qualitatively represent systems’ interactions. CLD can visually represent the links between the system variables using arrows and describe their effects. For example, a positive relationship indicates that an increase in a variable brings about an increase in the other (i.e., both variables change in the same direction). In contrast, a negative relationship indicates that an increase in a variable brings about a decrease in the other (i.e., the variables change in opposite directions). Another feature of SD is its ability to model feedbacks and delays in systems’ response over time.
A generalized CLD for NM FEW nexus (Figure 11) highlights multiple loops that describe how food production and prices; energy production and prices; and state revenue and severance tax incentives interact. Qualitative CLD can be translated to quantitative estimation of FEW nexus parameters. An SD simulation and prediction can offer a “virtual world” to analyze the influence of interconnected variables on FEW systems’ behavior through scenario-based sensitivity analyses (Richardson, 1995; Ford, 1999). This SD approach will be further used to develop livestock, forage, dairy, energy, and water interconnected relationships for NM.
FIGURE 11. Casual loop diagram for NM’s FEW systems. The arrows describe cause-and-effect behavior between variables. For example, an arrow from A to B indicates that A causes B. Pair-wise variable polarities represented as positive (+) (i.e., an increase or decrease in one factor causes an increase or decrease in the other factor) or (-), which is the opposite of a positive influence (i.e., an increase or decrease in one factor causes a decrease or increase in the other).
As a part of FEW-WISE framework, the qualitative description of FEW systems’ component linkages (NM FEW Nexus Description and NM FEW Nexus Drivers section) will inform the flows and connections of stocks and drivers in the SD model. The time series output of SD modeling will further be utilized to derive indices to monitor the FEW nexus, assess its adaptive capacity and resilience, assess potential resource management scenarios, and guide the decision-making process to develop effective policies to enhance resilience. The indices considered in this analysis represent and integrate two elements: FEW resource use (and availability) and HWB. The availability and use of FEW resources will be quantified based on the qualitative description of the interactions among different FEW system components (Food and Energy to Water and Food sections). Enhancing HWB can be achieved mostly through economic development but at the cost of degrading FEW resources (Foreman et al., 2003; Overpeck et al., 2005; Carpenter et al., 2006)—a process that acts as a barrier to resilience. A limited number of indices in nexus research have recently emerged (Willis et al., 2016) such as the Notre Dame Global Adaptation Index (Notre Dame Global Adaptation Index (ND-GAIN, 2015), the Environmental Performance Index (Wendling et al., 2020), the Human Insecurity Index (Werthes et al., 2011), and the Pardee RAND FEW Security Index (Willis et al., 2016).
However, these indices focus on resource vulnerability, use, or accessibility but lacked in integrating HWB. For instance, the FEW Security Index is based on the principle that sufficient, widely accessible FEW systems are required for human development based on the fact that high security in one or two of these components cannot completely compensate for low security in others. This research focuses on developing an adapted index that couples FEW and HWB systems based on the principle that measuring only the aggregated amount of available resources to support human development is an insufficient measure of security and that the distribution of FEW resources is also an important consideration. The linkage between resources and human development can directly be determined by whether an adequate amount and good quality of resources are provided to meet the needs of growing population.
The concept that will be followed in developing these indices is based on the availability, sustainability, and resiliency of FEW resource use to account for short- and long-term changes and shocks. To calculate these indices, it is important to describe the quality and quantity of FEW resources in terms of use, production, and availability through indicators. The qualitative description of the interlinkages (NM FEW Nexus Description section) can be used to guide the selection of the most important indicators to represent the FEW systems under investigation, expressing the linkages between variables using a process-based approach as exemplified in a CLD (Figure 11). This study, for example, considered the amount of food commodities produced (regardless of their respective nutritive values and ability to meet the demand) and required, in one way or the other, a form of resources (water and energy). For each subsystem, various indicators will be chosen to provide information about the behavior of the integrated system for which high-quality historical data are needed at the NM county and state levels (Table 1). These indicators of FEW index will be context-dependent as they are developed considering the qualitative and quantitative description of FEW system components of NM. However, the calculation and derivation of this index are more generic that can be applied and used in other regions and other contexts like food security.
The FEW indices can be calculated as follows:
where
The above three indices will be combined using the weighted average to derive a single indicator explaining the status of FEW systems as follows:
where i is the number of FEW indicators. The selection of an appropriate time scale based on the indicators is required to develop a consistent time series of FEW indices. The availability of such time series is key in monitoring and evaluating the behavior FEW nexus in response to the abovementioned stresses. In other words, these indices can be compared with drought indices and energy prices.
Humans benefit from their interaction with the Earth’s natural resources base as it provides environmental, economic, and social capital to enhance their well-being. HWB is a broad concept that cannot be observed directly from surveys or measured independent of various social factors. It could possibly have multiple domains, and social scientists have developed broad categories to draw general distinctions between them. Within each domain, there is a set of subcategories (or indicators) that identify specific components of HWB. There is no general agreement on a set of domains and indicators to describe HWB. In the literature, seven domains have been identified to be broad enough to encompass most research frameworks such as (Hagerty et al., 2001) relationships with family and friends, emotional well-being, material well-being, health, work and productivity, feeling part of one’s community, and personal safety (Cummins et al., 1994; Cummins, 1996). The list of potential indicators is even longer, and no comprehensive list exists (e.g., education, employment, energy, population, and leisure activities.) (Boelhouwer and Stoop, 1999; Diener et al., 1999; Marks et al., 2006; Costanza et al., 2007). These domains and indicators are used to understand and categorize the concepts, status, and trends of HWB based on a set of indices. One such index is the Human Wellbeing Index (HWBI) of the U.S. Environmental Protection Agency that characterizes HWB at multiple scales (e.g., national, regional, or local) (Summers et al., 2017). The HWBI can use a substantial group of indicators to evaluate the influence of social, economic, and environmental domains in an integrated fashion based on well-being, applicable to communities at multiple scales. In this study, a subset of social and economic domains defined by Summers et al. (2017) will be modified to estimate the HWB index for NM (i.e.,
Using the following equation, three indicators associated with the economic domain of HWB will be combined to calculate
where
and
where i is the number of HWB indicators. The indicators (e.g., adults working long hours spending less leisure time, degradation of natural FEW systems, and educated population associated with specific age-groups) associated with the social domain of the HWB system will be defined objectively to determine the time series HWB index. A qualitative nonmeasurable criterion or scores from low to high will be given due to their related effectiveness to the quality of life. The selected economic and social domains of HWB are reflective of NM economy and social conditions due to contributions of energy (e.g., crude oil and natural gas) and food (e.g., livestock) industries toward revenues, degradation of FEW, or natural resources (Gedefaw et al., 2020) that contributes indirectly to the well-being of the human society by promoting and attracting tourism-based economic and social welfare.
Both FEW and HWB indices are generic, and the weights are dimensionless, varying from 0 – 1, but they need to be evaluated regionally and contextually. The units of different indicators of the two indices (i.e.,
NM FEW nexus is strained by some of the most challenging risks toward achieving resilience. To address these risks, resource managers heavily rely on these socioecological systems’ capacities to absorb disturbances while maintaining essential functions (Holling, 1973; Folke et al., 2002). But when a critical threshold is surpassed, a system under stress can undergo catastrophic changes and reorganize into a different state (Angeler and Allen, 2016). An improved understanding of the boundaries of a system resilience (i.e., thresholds) that separate one state from another alternative potentially undesirable state can help in developing adequate management practices.
The concept of resilience was introduced more than 40 years ago in ecological sciences and was defined as the amount of disturbance that a system can withstand before shifting into an alternative stable state (Holling, 1973). The concept has been used in human development (Brown and Westaway, 2011) and socioecological systems (Folke, 2006; Curtin and Parker, 2014; Desjardins et al., 2015) among others. It emphasizes that socioecological systems need to be managed and governed for flexibility and emergence, rather than for maintaining stability (Peterson et al., 2003; Carpenter et al., 2015). Hence, resilience is concerned with navigating complexity, uncertainty, and changes across scales (Berkes et al., 2003; Cash et al., 2006; Cumming et al., 2013) on a human-dominated planet (Lubchenco, 1998; Steffen et al., 2007) by combining the concepts of adaptability and transformation. Adaptation is a process of deliberate change in anticipation of or in reaction to external stimuli and stress (Nelson et al., 2007). Transformability is shifting development into new pathways and even creating novel ones.
Given this concept, the FEW-WISE framework adopted the ecological resilience concept (Holling and Meffe, 1996; Quinlan et al., 2016), which refers to the magnitude of disturbance (i.e., distance to critical transition) that a system can absorb before shifting to an alternate system state (Holling and Meffe, 1996). It assumes that a system has multiple alternate equilibria and the capacity to maintain its essential structure and functions through reorganization—a key property of complex adaptive systems. Disturbances slow down system’s processes through abrupt, gradual turns, or bifurcate toward an alternate state. These turning points are referred to as tipping points as the system passes critical thresholds (Dakos et al., 2015). A system becomes unstable once reaching such thresholds but afterward should attain a new stable, adaptive, and transformed state (Kuehn, 2011). A dynamic behavior of a system consisting of two different states, A and B, before and after a disturbance, respectively, is depicted in Figure 12. The recovery rate (resilience) of a system is high when the distance to a critical transition (h) is higher and low when the distance to critical threshold (ds) is shorter. An FEW nexus can reorganize (Ullah et al., 2015) and attain multiple resilience states, while undergoing abrupt shifts (Scheffer and Carpenter, 2003; Scheffer et al., 2009) through human interventions that can introduce different adaptive measures (Folke et al., 2004; Biggs et al., 2012; Schoon and Cox, 2012). The concept described in Figure 12 can be used to assess NM FEW systems’ resilience by quantitatively characterizing and predicting their response to the current and future climatic and socioeconomic changes.
FIGURE 12. (A) Stages in the performance of FEWS toward resilience in response to disturbances with (A) tipping points t1 and t2 and critical thresholds f1 and f2 which are overcome by changing the state of a system in response to drivers, and (B) and (C) show the systems with low and high resilience and recovery rate, respectively.
Resilience as a system property should not be reduced to a simple index, but different types of indicators need to be combined to capture facets of resilience. A Resilience Index (RI) needs to reflect the complex adaptive behavior of socioecological systems—combining biophysical (e.g., FEW) feedbacks and trade-offs with socioeconomic (HWB) drivers and outcomes (Givens et al., 2018).
SD can be the most appropriate approach to model resilience with its structured framework that allows simulation of simulate complex feedbacks of key resource variables (Luna-Reyes and Andersen, 2003; Tenza et al., 2017), can integrate numerous interactions into a set of nonlinear expressions similar to those governing coupled FEW–HWB systems, and most importantly, focuses on the evolution of a process of interest, rather than achieving a specific equilibrium or optimal solution. Particularly, what is more pertaining to resilience is that SD models are essentially systems of equations with no “closed-form” solution, where comparison of the evolution of different scenarios is the primary mode of analysis.
A critical aspect of resilience is to monitor the evolution of processes and feedback loops. The analysis of CLD can provide insights into the root causes of a system behavior; help in identifying changes in variables, processes, and drivers; and indicate threshold of a state variable that is prone to be overpassed. For example, the occurrence of trade-offs, synergies, and cross-sector dependencies in a system can indicate new technology (oil and gas hydraulic fracturing), policy, or environmental changes that transform it into a new state, demonstrating the adaptive management of FEW resources. Therefore, an RI needs to couple multiple indices (e.g.,
The FEW-WISE framework (Figure 2) involves resilience assessment based on an RI (Eq. 9) that integrates and evaluates coupled FEW-HWB systems. The proposed RI
where the weights
Based on
The goal of this analysis was to develop the FEW-WISE framework for an improved assessment of FEW systems resilience in response to environmental and socioeconomic shocks. The state of New Mexico was used as a case study to develop this framework in terms of its unique characteristics and challenges in terms of climate change impacts that affect its FEW resources and New Mexicans’ well-being. To achieve this goal, FEW-WISE followed five steps: 1) qualitatively identify interconnections between the FEW system components and quantitatively assess resource exchanges, 2) identify important systems drivers, 3) integrate FEW systems using system dynamics (SD) models to evaluate their response to the identified drivers, 4) develop FEW-HWB performance indices, and 5) develop a resilience monitoring criterion using a threshold-based approach that integrates these indices. The first two steps have been described in detail here. The other three steps were individually conceptualized and will be carried out and presented in separate modeling, indices, and resilience assessments. With the application of the framework, the expected results will include time series of predicted values of FEW components, FEW-HWB, and resilience indices ranging from 0 to 1.
The SD modeling is a key feature of the framework as it simulates the nexus as a holistic multi-sectoral system, providing insights into the vulnerability of resources to stresses, demonstrating how the nexus will respond to changes and transition to absorb the effects from these stresses. The SD modeling along with the resilience indices potentially provides the needed linkages to the decision-making processes. It should be noted that the HWB and FEW indices can be developed based on a wide range of variables or indicators. However, data availability challenges exist, especially those related to the links to food resources in water and energy systems, and water used for crop production, and human well-being. While the use of variables with most available data is important, some variables may not be the best ones to represent the systems or regions under consideration.
In conclusion, the FEW-WISE framework with its indices can be used to develop alternative scenario-based management strategies for FEW resources to enhance resilience and sustainability of ecologically and socioeconomically vulnerable regions. As NM has FEW systems that are sensitive to drought and fluctuations in energy prices, this framework would allow the state to effectively manage its resources. The framework is currently proposed at county and state scales over a semiarid region and context; however, its methodology can effectively be adapted, redefined, and transferred to operate over different regions and contexts in other parts of the world as needed.
The original contributions presented in the study are included in the article/Supplementary Material, further inquiries can be directed to the corresponding author.
KY and HG conceived the idea for this study. The FEW nexus components and drivers were described by KY and HG. The indices for human well-being and resilience were developed by HG. MS, LJ, and AZ, and MG provided datasets related to FEW components. Tables and figures were generated by KY along with the first draft of the writing. The final version of the manuscript was edited by all the authors. The final edits were compiled by KY who converted the manuscript to the final format for this journal.
This research was funded with a grant from the National Science Foundation awards # 1739835 and # IIA-1301346” to HG and collaborators, and New Mexico State University. The funders had no role in the design of the study; in the collection, analyses, or interpretation of data; in the writing of the manuscript, or in the decision to publish the results.
Any opinions, findings, and conclusions or recommendations expressed in this material are those of the author(s) and do not necessarily reflect the views of the National Science Foundation.
The authors declare that the research was conducted in the absence of any commercial or financial relationships that could be construed as a potential conflict of interest.
The authors would like to thank the entire INFEWS team.
ADB (2013). Thinking about Water Differently: Managing the Water, Energy and Food Nexus. Asian Development Bank. Publication Stock No. RPT125184, Mandaluyong City, Philippines.
Albrecht, T. R., Crootof, A., and Scott, C. A. (2018). The Water-Energy-Food Nexus: A Systematic Review of Methods for Nexus Assessment. Environ. Res. Lett. 13. doi:10.1088/1748-9326/aaa9c6
Alstone, P., Gershenson, D., and Kammen, D. M. (2015). Decentralized Energy Systems for Clean Electricity Access. Nat. Clim. Chang. 5, 305–314. doi:10.1038/nclimate2512
Angeler, D. G., and Allen, C. R. (2016). Quantifying Resilience. J. Appl. Ecol. 53, 617–624. doi:10.1111/1365-2664.12649
Bagheri, A., and Hjorth, P. (2007). A Framework for Process Indicators to Monitor for Sustainable Development: Practice to an Urban Water System. Environ. Dev. Sustain. 9, 143–161. doi:10.1007/s10668-005-9009-0
Bazilian, M., Rogner, H., Howells, M., Hermann, S., Arent, D., Gielen, D., et al. (2011). Considering the Energy, Water and Food Nexus: Towards an Integrated Modelling Approach. Energy Policy 39, 7896–7906. doi:10.1016/j.enpol.2011.09.039
BBER (2008). A Report on Historical and Future Population Dynamics in New Mexico Water Planning Regions. Available at:http://www.ose.state.nm.us/Pub/ISCreports/BBER-WPR-Estimates-Projections-Aug2008.pdf.
BEA (2018). Gross Domestic Product. Available at:http://www.statssa.gov.za/publications/P0441/P04414thQuarter2017.pdf.
Berkes, F., Colding, J., and Folke, C. (2003). Introduction. Navig. Soc. Syst., 1–30. doi:10.1017/cbo9780511541957.003
Bieber, N., Ker, J. H., Wang, X., Triantafyllidis, C., van Dam, K. H., Koppelaar, R. H. E. M., et al. (2018). Sustainable Planning of the Energy-Water-Food Nexus Using Decision Making Tools. Energy Policy 113, 584–607. doi:10.1016/j.enpol.2017.11.037
Biggs, E., Boruff, B., and Bruce, E. (2014). Environmental Livelihood Security in Southeast Asia and Oceania: a Water-Energy-Food-Livelihoods Nexus Approach for Spatially Assessing Change. Available at: https://hdl.handle.net/10568/71199doi:10.5337/2014.231
Biggs, E. M., Bruce, E., Boruff, B., Duncan, J. M. A., Horsley, J., Pauli, N., et al. (2015). Sustainable Development and the Water-Energy-Food Nexus: A Perspective on Livelihoods. Environ. Sci. Pol. 54, 389–397. doi:10.1016/j.envsci.2015.08.002
Biggs, R., Blenckner, T., Folke, C., Gordon, L., Norström, A., Nyström, M., et al. (2012). “Regime Shifts,” in Encyclopedia of Theoretical Ecology. Editors L. Hastings, and A. Gross (Ewing, NJ, USA): University of California Press).
Boelhouwer, J., and Stoop, I. (1999). Measuring Well-Being in the Netherlands: The SCP index from 1974 to 1997. Soc. Indic. Res. 48, 51–75. doi:10.1023/A:1006931028334
Brown, K., and Westaway, E. (2011). Agency, Capacity, and Resilience to Environmental Change: Lessons from Human Development, Well-Being, and Disasters. Annu. Rev. Environ. Resour. 36, 321–342. doi:10.1146/annurev-environ-052610-092905
Cai, X., Rosegrant, M. W., and Ringler, C. (2003). Physical and Economic Efficiency of Water Use in the River basin: Implications for Efficient Water Management. Water Resour. Res. 39. doi:10.1029/2001WR000748
Cai, X., Wallington, K., Shafiee-Jood, M., and Marston, L. (2018). Understanding and Managing the Food-Energy-Water Nexus – Opportunities for Water Resources Research. Adv. Water Resour. 111, 259–273. doi:10.1016/j.advwatres.2017.11.014
Carpenter, S. R., Brock, W. A., Folke, C., Van Nes, E. H., Scheffer, M., and Polasky, S. (2015). Allowing Variance May Enlarge the Safe Operating Space for Exploited Ecosystems. Proc. Natl. Acad. Sci. U. S. A. 112, 14384–14389. doi:10.1073/pnas.1511804112
Carpenter, S. R., DeFries, R., Dietz, T., Mooney, H. A., Polasky, S., Reid, W. V., et al. (2006). Millennium Ecosystem Assessment: Research Needs. Sci. (80- 314, 257–258. doi:10.1126/science.1131946
Carpenter, S., Walker, B., Anderies, J. M., and Abel, N. (2001). From Metaphor to Measurement: Resilience of what to what? Ecosystems 4, 765–781. doi:10.1007/s10021-001-0045-9
Cash, D. W., Adger, W. N., Berkes, F., Garden, P., Lebel, L., Olsson, P., et al. (2006). Scale and Cross-Scale Dynamics: Governance and Information in a Multilevel World. Ecol. Soc. 11. doi:10.5751/es-01759-110208
CE (2010). A Scoping Study on the Macroeconomic View of Sustainability—Final Report for the European Commission. (Cambridge: DG Environment).
CEA (2016). Energy for New Mexico. Available at: www.conwww.consumerenergyalliance.orgsumerenergyalliance.org
Chang, Y., Li, G., Yao, Y., Zhang, L., and Yu, C. (2016). Quantifying the Water-Energy-Food Nexus: Current Status and Trends. Energies 9, 1–17. doi:10.3390/en9020065
Chenoweth, J., Hadjinicolaou, P., Bruggeman, A., Lelieveld, J., Levin, Z., Lange, M. A., et al. (2011). Impact of Climate Change on the Water Resources of the Eastern Mediterranean and Middle East Region: Modeled 21st century Changes and Implications. Water Resour. Res. 47. doi:10.1029/2010WR010269
Costanza, R., Fisher, B., Ali, S., Beer, C., Bond, L., Boumans, R., et al. (2007). Quality of Life: An Approach Integrating Opportunities, Human Needs, and Subjective Well-Being. Ecol. Econ. 61, 267–276. doi:10.1016/j.ecolecon.2006.02.023
Cumming, G. S., Olsson, P., Chapin, F. S., and Holling, C. S. (2013). Resilience, Experimentation, and Scale Mismatches in Social-Ecological Landscapes. Landsc. Ecol. 28, 1139–1150. doi:10.1007/s10980-012-9725-4
Cummins, R. A. (1996). The Domains of Life Satisfaction: An Attempt to Order Chaos. Soc. Indic. Res. 38, 303–328. doi:10.1007/BF00292050
Cummins, R. A., Mccabe, M. P., Romeo, Y., and Gullone, E. (1994). Validity Studies the Comprehensive Quality of Life Scale (Comqol): Instrument Development and Psychometric Evaluation on College Staff and Students. Educ. Psychol. Meas. 54, 372–382. doi:10.1177/0013164494054002011
Curtin, C. G., and Parker, J. P. (2014). Foundations of Resilience Thinking. Conserv. Biol. 28, 912–923. doi:10.1111/cobi.12321
Dakos, V., Carpenter, S. R., van Nes, E. H., and Scheffer, M. (2015). Resilience Indicators: Prospects and Limitations for Early Warnings of Regime Shifts. Philos. Trans. R. Soc. B Biol. Sci. 370, 1–10. doi:10.1098/rstb.2013.0263
de Grenade, R., House-Peters, L., Scott, C. A., Thapa, B., Mills-Novoa, M., Gerlak, A., et al. (2016). The Nexus: Reconsidering Environmental Security and Adaptive Capacity. Curr. Opin. Environ. Sustain. 21, 15–21. doi:10.1016/j.cosust.2016.10.009
de Vito, R., Portoghese, I., Pagano, A., Fratino, U., and Vurro, M. (2017). An index-based Approach for the Sustainability Assessment of Irrigation Practice Based on the Water-Energy-Food Nexus Framework. Adv. Water Resour. 110, 423–436. doi:10.1016/j.advwatres.2017.10.027
Desjardins, E., Barker, G., Lindo, Z., Dieleman, C., and Dussault, A. (2015). Promoting Resilience. The Quarterly Review of Biology, (Chicago, Illinois: The University of Chicago Press). doi:10.1080/14635240.2017.1279764
Diemer, J., Crawford, T., and Patrick, M. (2012). Agriculture’s Contribution to New Mexico’s Economy, Circular. 675, 1–8.
Diener, E., Suh, E. M., Lucas, R. E., and Smith, H. L. (1999). Subjective Well-Being: Three Decades of Progress. Psychol. Bull. 125, 276–302. doi:10.1037/0033-2909.125.2.276
Dietz, T., Ostrom, E., and Stern, P. C. (2003). The Struggle to Govern the Commons. Science 302(5652), 1907–1912. doi:10.1126/science.1091015
Ding, K. J., Gunda, T., and Hornberger, G. M. (2019). Prominent Influence of Socioeconomic and Governance Factors on the Food-Energy-Water Nexus in Sub-saharan Africa. Earth’s Futur 7, 1071–1087. doi:10.1029/2019EF001184
D’Odorico, P., Davis, K. F., Rosa, L., Carr, J. A., Chiarelli, D., Dell’Angelo, J., et al. (2018). The Global Food-Energy-Water Nexus. Rev. Geophys. 56, 456–531. doi:10.1029/2017RG000591
Dubreuil, A., Assoumou, E., Bouckaert, S., Selosse, S., and Maı¨zi, N. (2013). Water Modeling in an Energy Optimization Framework – the Water-Scarce Middle East Context. Appl. Energ. 101, 268–279. doi:10.1016/j.apenergy.2012.06.032
EIA (2017). AEO 2017 with Projections to 2050Annu. Energy Outlook 2017, 1–127. Available at:www.eia.gov/aeo.
EIA (2018). Annual Energy Outlook 2018 with Projections to 2050. 11, 581–588. Available at:www.eia.gov/aeo.
EIA (2019). Annual Energy Outlook 2019 with Projections to 2050. Annu. Energy Outlook 2019 with Proj, 44. (Washington D.C: EIA), 1–64.
EIA (2020). Annual Energy Outlook 2020. 1–81. Available at: www.eia.gov/aeo.
EMNRD (2015). Seizing Our Energy Potential : Creating a More Diverse Economy in New Mexico. (State of New Mexico: New Mexico Energy Policy and Implementation Plan).
Fang, D., and Chen, B. (2017). Linkage Analysis for the Water–Energy Nexus of City. Appl. Energ. 189, 770–779. doi:10.1016/j.apenergy.2016.04.020
FAO (2012). Irrigation Water Requirement and Water Withdrawal by Country. (Rome: Food Agric. Organ. United Nations), 264. Available at:http://www.fao.org/nr/water/aquastat/water_use_agr/IrrigationWaterUse.pdf%0Ahttp://www.fao.org/nr/water/aquastat/water_use_agr/index.stm.
FAO (2017). The State of Food Security and Nutrition in the World. Building resilience for peace and food security. (Rome: Food and Agriculture Organization of the United States). doi:10.1080/15226514.2012.751351
FAO (2011). The State of the World’s Land and Water Resources for Food and Agriculture (SOLAW) - Managing Systems at Risk. Available at:http://www.fao.org/3/i1688e/i1688e03.pdf.
FAO (2014). The Water-Energy-Food Nexus: A New Approach in Support of Food Security and Sustainable Agriculture (Rome: Food Agric. Organ. United Nations)Available at: http://www.fao.org/3/a-bl496e.pdf.
Fiksel, J. (2003). Designing Resilient, Sustainable Systems. Environ. Sci. Technol. 37, 5330–5339. doi:10.1021/es0344819
Finley, J. W., and Seiber, J. N. (2014). The Nexus of Food, Energy, and Water. J. Agric. Food Chem. 62, 6255–6262. doi:10.1021/jf501496r
Fischer, J., Gardner, T. A., Bennett, E. M., Balvanera, P., Biggs, R., Carpenter, S., et al. (2015). Advancing Sustainability through Mainstreaming a Social-Ecological Systems Perspective. Curr. Opin. Environ. Sustain. 14, 144–149. doi:10.1016/j.cosust.2015.06.002
Folke, C., Carpenter, S., Elmqvist, T., Gunderson, L., Holling, C. S., and Walker, B. (2002). Resilience and Sustainable Development: Building Adaptive Capacity in a World of Transformations. Ambio 31, 437–440. doi:10.1579/0044-7447-31.5.437
Folke, C., Carpenter, S., Walker, B., Scheffer, M., Elmqvist, T., Gunderson, L., et al. (2004). Regime Shifts, Resilience, and Biodiversity in Ecosystem Management. Annu. Rev. Ecol. Evol. Syst. 35, 557–581. doi:10.1146/annurev.ecolsys.35.021103.105711
Folke, C. (2006). Resilience: The Emergence of a Perspective for Social-Ecological Systems Analyses. Glob. Environ. Chang. 16, 253–267. doi:10.1016/j.gloenvcha.2006.04.002
Ford, D. N. (1999). A Behavioral Approach to Feedback Loop Dominance Analysis, Syst. Dyn. Rev., 15. (Hoboken, New Jersey: John Wiley – Sons, Ltd.), 3–36. doi:10.1002/(SICI)1099-1727
Foreman, D., Daly, K., Noss, R., Clark, M., Menke, K., Parsons, D. R., et al. (2003). New Mexico Highlands Wild lands Network Vision Connecting the Sky Islands to the Southern Rockies. (Vermont, US: New Mexico Wilderness Alliance, Wildlands Project).
Forrester, J. W. (1958). Industrial Dynamics: A Major Breakthrough for Decision Makers. Harv. Bus. Rev. 26, 37–66.
Frisvold, G. B., Jackson, L. E., Pritchett, J. G., and Ritten, J. P. (2013). “Agriculture and Ranching,” in Assessment of Climate Change in the Southwest United States: A Report Prepared for the National Climate Assessment. Editors A. Garfin, A. Jardine, R. Merideth, and M. Black (Washington, DC:Island Press), 218–239.
Gedefaw, M. G., Geli, H. M. E., and Abera, T. A. (2021). Assessment of Rangeland Degradation in New Mexico Using Time Series Segmentation and Residual Trend Analysis. Remote Sens. 13(9), 1618. doi:10.3390/rs13091618
Gedefaw, M. G., Geli, H. M. E., Yadav, K., Zaied, A. J., Finegold, Y., and Boykin, K. G. (2020). A Cloud-Based Evaluation of the National Land Cover Database to Support New Mexico’s Food-Energy-Water Systems. Remote Sens 12. doi:10.3390/rs12111830
Gerland, P., Raftery, A. E., Ševčíková, H., Li, N., Gu, D., Spoorenberg, T., et al. (2014). World Population Stabilization Unlikely This century. Science, 346, 234–237. doi:10.1126/science.1257469
Givens, J. E., Padowski, J., Guzman, C. D., Malek, K., Witinok-Huber, R., Cosens, B., et al. (2018). Incorporating Social System Dynamics in the Columbia River Basin: Food-Energy-Water Resilience and Sustainability Modeling in the Yakima River Basin. Front. Environ. Sci. 6, 1–19. doi:10.3389/fenvs.2018.00104
Gonzalez, P., Garfin, G. M., Breshears, D. D., Brooks, K. M., Brown, H. E., Elias, E. H., et al. (2018). “Southwest,” in Impacts, Risks, and Adaptation in the United States: Fourth National Climate Assessment. Editors B. C. S. Reidmiller, D. R. C. W. Avery, D. R. Easterling, K. E. Kunkel, K. L. M. Lewiset al. (Washington, DC, USA: U.S. Global Change Research Program), 1101–1184. doi:10.7930/NCA4.2018.CH25
Grafton, R. Q., Libecap, G. D., Edwards, E. C., O’Brien, R. J., Bob), , and Landry, C. (2012). Comparative Assessment of Water Markets: Insights from the Murray–Darling Basin of Australia and the Western USA. Water Policy 14, 175–193. doi:10.2166/wp.2011.016
Grafton, R. Q., Williams, J., and Jiang, Q. (2017). Possible pathways and tensions in the food and water nexus. Earth’s Future 5, 449–462. doi:10.1002/2016EF000506
Green, T. R., Taniguchi, M., Kooi, H., Gurdak, J. J., Allen, D. M., Hiscock, K. M., et al. (2011). Beneath the Surface of Global Change: Impacts of Climate Change on Groundwater. J. Hydrol. 405, 532–560. doi:10.1016/j.jhydrol.2011.05.002
Gunderson, L. H., and Holling, C. S. (2001). “Panarchy: Understanding Transformations in Human and Natural Systems,” In Ecological Economics (Washington, DC, sland Press), 450. doi:10.1016/j.ecolecon.2004.01.010
Hagerty, M. R., Cummins, R., Ferriss, A. L., Land, K., Michalos, A. C., Peterson, M., et al. (2001). Quality of Life Indexes for National Policy: Review and Agenda for Research. Bull. Méthodologie Sociol. 71, 58–78. doi:10.1177/075910630107100104
Hameed, M., Moradkhani, H., Ahmadalipour, A., Moftakhari, H., Abbaszadeh, P., and Alipour, A. (2019). A Review of the 21st century Challenges in the Food-Energy-Water Security in the Middle East, Water (Switzerland), 11. doi:10.3390/w11040682
Heckbert, S., Costanza, R., Parrott, L., and Director, I. (2014). Achieving Sustainable Societies: Lessons from Modelling the Ancient Maya. Solutions 5(5), 55–64.
Hitaj, C., and Suttles, S. (2016). Trends in US Agriculture’s Consumption and Production of Energy: Renewable Power, Shale Energy and Cellulosic Biomass (Washington, D.C.: Economic Research Service, USDA).
Hoff, H. (2011). “Understanding the Nexus,” in Background Paper for the Bonn2011 Conference: The Water, Energy and Food Security Nexus, 16–18 November 2011. Stockholm: Stockholm Environment Institute.
Holechek, J. L., Geli, H. M. E., Cibils, A. F., and Sawalhah, M. N. (2020). Climate Change, Rangelands, and Sustainability of Ranching in the Western United States. Sustain 12. doi:10.3390/su12124942
Holling, C. S., and Meffe, G. K. (1996). Command and Control and the Pathology of Natural Resource Management. Conserv. Biol.J. Soc. Conserv. Biol. 10, 328–337. doi:10.1046/j.1523-1739.1996.10020328.x
Holling, C. S. (2001). Understanding the Complexity of Economic, Ecological, and Social Systems. Ecosystems 4, 390–405. doi:10.1007/s10021-001-0101-5
IEA (2012). CO2 Emissions from Fuel Combustion 2012 (Paris: OECD Publishing). doi:10.1787/co2_fuel-2012-en
IRENA (2015). Renewable Energy in the Water, Energy and Food Nexus. Int. Renew. Energ. Agency. 1–125.
Johnson, L. E., Geli, H. M. E., Hayes, M. J., and Smith, K. H. (2020). Building an Improved Drought Climatology Using Updated Drought Tools: A New Mexico Food-Energy-Water (FEW) Systems Focus. Front. Clim. 2. doi:10.3389/fclim.2020.576653
Kan, G., Zhang, M., Liang, K., Wang, H., Jiang, Y., Li, J., et al. (2016). Improving Water Quantity Simulation & Forecasting to Solve the Energy-Water-Food Nexus Issue by Using Heterogeneous Computing Accelerated Global Optimization Method. Appl. Energ. 210, 420–433. doi:10.1016/j.apenergy.2016.08.017
Karandish, F., and Mousavi, S. (2016). Climate Change Uncertainty and Risk Assessment in Iran during Twenty-First century: Evapotranspiration and green Water Deficit Analysis. Theor. Appl. Climatol. 131, 777–791. doi:10.1007/s00704-016-2008-2
Keairns, D. L., Darton, R. C., and Irabien, A. (2016). The Energy-Water-Food Nexus. Annu. Rev. Chem. Biomol. Eng. 7, 239–262. doi:10.1146/annurev-chembioeng-080615-033539
Keskinen, M., Guillaume, J. H. A., Kattelus, M., Porkka, M., Räsänen, T. A., and Varis, O. (2016). The Water-Energy-Food Nexus and the Transboundary Context: Insights from Large Asian Rivers. Water (Switzerland) 8. doi:10.3390/w8050193
King, C., and Jaafar, H. (2015). Rapid Assessment of the Water–Energy–Food–Climate Nexus in Six Selected Basins of North Africa and West Asia Undergoing Transitions and Scarcity Threats. Int. J. Water Resour. Dev. 31, 343–359. doi:10.1080/07900627.2015.1026436
King, C. W., Holman, A. S., and Webber, M. E. (2008). Thirst for Energy. Nat. Geosci. 1, 283–286. doi:10.1038/ngeo195
Kotir, J. H., Smith, C., Brown, G., Marshall, N., and Johnstone, R. (2016). A System Dynamics Simulation Model for Sustainable Water Resources Management and Agricultural Development in the Volta River Basin, Ghana. Sci. Total Environ. 573, 444–457. doi:10.1016/j.scitotenv.2016.08.081
Kuehn, C. (2011). A Mathematical Framework for Critical Transitions: Bifurcations, Fastslow Systems and Stochastic Dynamics. Phys. D Nonlinear Phenom. 240, 1020–1035. doi:10.1016/j.physd.2011.02.012
Laspidou, C. S., Mellios, N. K., Spyropoulou, A. E., Kofinas, D. T., and Papadopoulou, M. P. (2020). Systems Thinking on the Resource Nexus: Modeling and Visualisation Tools to Identify Critical Interlinkages for Resilient and Sustainable Societies and Institutions. Sci. Total Environ. 717. doi:10.1016/j.scitotenv.2020.137264
Liu, J., Mooney, H., Hull, V., Davis, S. J., Gaskell, J., Hertel, T., et al. (2015). Systems Integration for Global Sustainability. Science 80, 347. doi:10.1126/science.1258832
Longworth, J. W., Valdez, J. M., Magnuson, M. L., and Richard, K. (2013). New Mexico Water Use by Categories 2010. New Mexico State Engineer Office, Technical Report 54, October 2013., 144.
Lubchenco, J. (1998). Entering the century of the Environment: A New Social Contract for Science. Science (80-. ) 279, 491–497. doi:10.1126/science.279.5350.491
Luna-Reyes, L. F., and Andersen, D. L. (2003). Collecting and Analyzing Qualitative Data for System Dynamics: Methods and Models. Syst. Dyn. Rev. 19, 271–296. doi:10.1002/sdr.280
Marks, N., Abdallah, S., Simms, A., and Thompson, S. (2006). THE (Un)HAPPY PLANET INDEX: An index of Human Well-Being and Environmental Impact. Agenda. New Economics Foundation.
Marston, L., Ao, Y., Konar, M., Mekonnen, M. M., and Hoekstra, A. Y. (2018). High-Resolution Water Footprints of Production of the United States. Water Resour. Res. 54, 2288–2316. doi:10.1002/2017WR021923
Maupin, M. A., Kenny, J. F., Hutson, S. S., Lovelace, J. K., Barber, N. L., and Linsey, K. S. (2010). Estimated Use of Water in the United States in 2010 U.S. Geological Survey Circular 1405, 56. Available at:http://pubs.usgs.gov/circ/1405/.
McGrane, S. J., Acuto, M., Artioli, F., Chen, P. Y., Comber, R., Cottee, J., et al. (2019). Scaling the Nexus: Towards Integrated Frameworks for Analysing Water, Energy and Food. Geogr. J. 185, 419–431. doi:10.1111/geoj.12256
Miralles-Wilhelm, F. (2016). Development and Application of Integrative Modeling Tools in Support of Food-Energy-Water Nexus Planning—A Research Agenda. J. Environ. Stud. Sci. 6, 3–10. doi:10.1007/s13412-016-0361-1
Miranowski, J. A. (2005). Chapter 3 Energy Consumption in US Agriculture. In Agriculture as a Producer and Consumer of Energy (Cambridge, MA: CABI Publishing) 68–111.
Mohtar, R. H., and Daher, B. (2019). Lessons Learned: Creating an Interdisciplinary Team and Using a Nexus Approach to Address a Resource Hotspot. Sci. Total Environ. 650, 105–110. doi:10.1016/j.scitotenv.2018.08.406
Mohtar, R. H., and Daher, B. (2010). Water, Energy, and Food: The Ultimate Nexus. Encycl. Agric. Food Biol. Eng., 2nd edition, 1–5. doi:10.1081/e-eafe2-120048376
Molly, M. L., Valdez, J. M., Lawler, C. R., Nelson, R., and Petronis, L. (2015). New Mexico State Engineer Office, Technical Report 55 Available at: https://www.ose.state.nm.us/WUC/wuc_waterUseData.php.
Moskowitz, R. (2017). Gross Domestic Product of New Mexico (Mexico: Labor Market Review. U.S. Bureau of Economic Analysis).
Mpandeli, S., Naidoo, D., Mabhaudhi, T., Nhemachena, C., Nhamo, L., Liphadzi, S., et al. (2018). Climate Change Adaptation through the Water-Energy-Food Nexus in Southern Africa. Int. J. Environ. Res. Public Health 15, 1–19. doi:10.3390/ijerph15102306
NASS (2015). New Mexico Agricultural Statisitics - New Mexico Annual Bulletin2015. Available at: https://www.nass.usda.gov/Statistics_by_State/New_Mexico/Publications/Annual_Statistical_Bulletin/2015/2015_NM_Ag_Statistics.pdf.(Accessed February 13, 2020).
NASS (2016). New Mexico Agricultural Statistics, New Mexico Annual Bulletin. Available at: https://www.nass.usda.gov/Statistics_by_State/New_Mexico/Publications/Annual_Statistical_Bulletin/2016/2016_NM_Ag_Statistics.pdf(Accessed February 13, 2020).
NASS (2018). New Mexico Agricultural Statistics. New Mexico Annual Bulletin, 2018, USDAM National Agricultural Statistics Service. Available at: https://www.nass.usda.gov/Statistics_by_State/New_Mexico/Publications/Annual_Statistical_Bulletin/2018/2018-NM-Ag-Statistics.pdf. (Accessed on February 13, 2020).
Gonzalez, P., Garfin, G. M., Breshears, D. D., Brooks, K. M., Brown, H. E., Elias, E. H., et al. (2018). “Southwest,” in Impacts, Risks, and Adaptation in the United States: Fourth National Climate Assessment, Volume II. Editors B. C. S. Reidmiller, D. R. C. W. Avery, D. R. Easterling, K. E. Kunkel, K. L. M. Lewiset al. (Washington, DC, USA: U.S. Global Change Research Program), 1101–1184. doi:10.7930/NCA4.2018.CH25
ND-Gain, (2015). ND GAIN. Available at: https://gain.nd.edu/our-work/country-index/.
Nelson, D. R., Adger, W. N., and Brown, K. (2007). Adaptation to Environmental Change: Contributions of a Resilience Framework. Annu. Rev. Environ. Resour. 32, 395–419. doi:10.1146/annurev.energy.32.051807.090348
New Mexico First and New Mexico State University, (2016). Resilience in New Mexico Agriculture: Opportunities, Challenges and Realities for New Mexico ’ S Farming and Ranching Future (Las Cruces and Albuquerque, NM: Thornburg Foundation and New Mexico Department of Agriculture) Available at: https://nmfirst.org/initiatives/agricultural-resilience-in-new-mexico/.
Nhamo, L., Ndlela, B., Nhemachena, C., Mabhaudhi, T., Mpandeli, S., and Matchaya, G. (2018). The Water-Energy-Food Nexus: Climate Risks and Opportunities in Southern Africa. Water (Switzerland) 10, 1–18. doi:10.3390/w10050567
Niang, I., Ruppel, O. C., Abdrabo, M. A., Ama, E., Christopher, L., Padgham, J., et al. (2014). “Chapter 22 Africa,” in Climate Change 2014: Impacts, Adaptation, and Vulnerability. Part B: Regional Aspects. Contribution of Working Group II to the Fifth Assessment Report of the Intergovernmental Panel on Climate Change,” in Contribution Of Working Group II to the Fifth Assessment Report Of the Intergovernmental Panel On Climate Change. Editors V. R. Barros, C. B. Field, D. J. Dokken, M. D. Mastrandrea, K. J. Mach, T. E. Biliret al. (Cambridge, United Kingdom and New York, NY, USA: Cambridge University Press), 1199–1265.
NMDA (2018). New Mexico Pecan County Estimates. USDA, National Agricultural Statistics Service. Available at: https://www.nass.usda.gov/Statistics_by_State/New_Mexico/Publications/Special_Interest_Reports/2018-Pecan-CE.pdf.
NMEDD (2010). Census Data, New Mexico Population. Available at: https://gonm.biz/site-selection/census-data.
NOAA (2017). National Centers for Environmental Information, State of the Climate: National Climate Report for Annual 2017, Published online January 2018, retrieved on May 18, 2020 from. https://www.ncdc.noaa.gov/sotc/national/201713.
NOAA-NCEI, (2020). Climate of New Mexico. Available at: https://wrcc.dri.edu/Climate/narrative_nm.php.
Nord, M., Andrews, M., and Carlson, S. (2005). Household Food Security in the United States, (2004). USDA ERS Economic Research Report No. 11. Available at SSRN: https://ssrn.com/abstract=878333 or http://dx.doi.org/10.2139/ssrn.878333
NSF (2014). Food, Energy and Water: Transformative Research Opportunities in the Mathematical and Physical Sciences. Mathematical and Physical Sciences Advisory Committee, National Science Foundation.
Ochoa, C., Guldan, S., and Fernald, A. (2020). Surface Water and Groundwater Interactions in Acequia Systems of Northern New Mexico. Acequias of the Southwestern United States: Elements of Resilience in a Coupled Natural and Human System, Acequia Hydrology and Society (New Mexico: Ecohydrology Lab), 33–40.
OECD (2012). OECD Environmental Outlook to 2050 : The Consequences of Inaction. OECD Publishing, Paris SE - 349 Pages : Color Illustrations, Color Maps ; 27 Cm. doi:10.1787/9789264122246-en
Overpeck, J., Garfin, G., Jardine, A., Busch, D. E., Cayan, D., Dettinger, M., et al. (2005). Ecosystems and Human Well-Being: A Framework for Assessment. Washington: Island Press. doi:10.5822/978-1-61091-484-0_1
Patrick, N. A. (1977). Energy Use Patterns for Agricultural Productionin New Mexico. AGRIS, Food and Agriculture Organization of the United Nations. Available at: https://agris.fao.org/agris-search/search.do?recordID=US19780294771.
Peterson, G. D., Cumming, G. S., and Carpenter, S. R. (2003). Scenario Planning: A Tool for Conservation in an Uncertain World. Conserv. Biol. 17, 358–366. doi:10.1046/j.1523-1739.2003.01491.x
PRISM (2010). 30-Year Normal Temperature and Precipitation. Available at:https://prism.oregonstate.edu/normals/.
Qin, H.-P., Su, Q., and Khu, S.-T. (2011). An Integrated Model for Water Management in a Rapidly Urbanizing Catchment. Environ. Model. Softw. 26, 1502–1514. doi:10.1016/j.envsoft.2011.07.003
Quinlan, A. E., Berbés-Blázquez, M., Haider, L. J., and Peterson, G. D. (2016). Measuring and Assessing Resilience: Broadening Understanding through Multiple Disciplinary Perspectives. J. Appl. Ecol. 53, 677–687. doi:10.1111/1365-2664.12550
Ramankutty, N., Mehrabi, Z., Waha, K., Jarvis, L., Kremen, C., Herrero, M., et al. (2018). Trends in Global Agricultural Land Use: Implications for Environmental Health and Food Security. Annu. Rev. Plant Biol. 69, 789–815. doi:10.1146/annurev-arplant-042817-040256
Rasul, G. (2016). Managing the Food, Water, and Energy Nexus for Achieving the Sustainable Development Goals in South Asia. Environ. Dev. 18, 14–25. doi:10.1016/j.envdev.2015.12.001
Richardson, G. P. (1995). Loop Polarity, Loop Dominance, and the Concept of Dominant Polarity (1984). Syst. Dyn. Rev. 11, 67–88. doi:10.1002/sdr.4260110106
Ringler, C., Willenbockel, D., Perez, N., Rosegrant, M., Zhu, T., and Matthews, N. (2016). Global Linkages Among Energy, Food and Water: an Economic Assessment. J. Environ. Stud. Sci. 6, 161–171. doi:10.1007/s13412-016-0386-5
Ritchie, H. (2017). Water Use and Stress Our World Data. (England, Wales.: Global Change Data Lab). Available at: https://ourworldindata.org/water-use-stress.
Rosa, L., Davis, K. F., Rulli, M. C., and D’Odorico, P. (2017). Environmental Consequences of Oil Production from Oil Sands. Earth’s Futur. 5, 158–170. doi:10.1002/2016EF000484
Rosa, L., Rulli, M. C., Davis, K. F., and D’Odorico, P. (2018). The Water-Energy Nexus of Hydraulic Fracturing: A Global Hydrologic Analysis for Shale Oil and Gas Extraction. Earth’s Futur 6, 745–756. doi:10.1002/2018EF000809
Sachs, I., and Silk, D. (1990). Food and Energy: Strategies for Sustainable Development. (Hong Kong: United Nations University Press). doi:10.1016/0306-9192(93)90073-K
Sainz, R. D. (2003). Framework for Calculating Fossil Fuel Use in Livestock Systems. Developed under the auspices of the Livestock, Environment and Development Initiative, Food and Agriculture Organization of the United Nations. Available at: http://www.fao.org/wairdocs/lead/x6100e/x6100e00.htm.
Sanders, K. T., and Webber, M. E. (2012). Evaluating the Energy Consumed for Water Use in the United States. Environ. Res. Lett. 7. doi:10.1088/1748-9326/7/3/034034
Sawalhah, M. N., Geli, H. M. E., Holechek, J. L., Cibils, A. F., Spiegal, S., and Gifford, C. (2021). Water Footprint of Rangeland Beef Production in the Southwestern United States. Submitted in Agricultural Water Management
Sawalhah, M. N., Holechek, J. L., Cibils, A. F., Geli, H. M. E., and Zaied, A. (2019). Rangeland Livestock Production in Relation to Climate and Vegetation Trends in New Mexico. Rangel. Ecol. Manag. 72, 832–845. doi:10.1016/j.rama.2019.03.001
Scanlon, B. R., Ruddell, B. L., Reed, P. M., Hook, R. I., Zheng, C., Tidwell, V. C., et al. (2017). The Food-Energy-Water Nexus: Transforming Science for Society. Water Resour. Res. 53, 3550–3556. doi:10.1002/2017WR020889
Scheffer, M., Bascompte, J., Brock, W. A., Brovkin, V., Carpenter, S. R., Dakos, V., et al. (2009). Early-warning Signals for Critical Transitions. Nature 461, 53–59. doi:10.1038/nature08227
Scheffer, M., and Carpenter, S. R. (2003). Catastrophic Regime Shifts in Ecosystems: Linking Theory to Observation. Trends Ecol. Evol. 18, 648–656. doi:10.1016/j.tree.2003.09.002
Schoon, M. L., and Cox, M. E. (2012). Understanding Disturbances and Responses in Social-Ecological Systems. Soc. Nat. Resour. 25, 141–155. doi:10.1080/08941920.2010.549933
Schwalm, C. R., Williams, C. A., Schaefer, K., Baldocchi, D., Black, T. A., Goldstein, A. H., et al. (2012). Reduction in Carbon Uptake during Turn of the century Drought in Western North America. Nat. Geosci. 5, 551–556. doi:10.1038/ngeo1529
Shannak, S., Mabrey, D., and Vittorio, M. (2018). Moving from Theory to Practice in the Water–Energy–Food Nexus: An Evaluation of Existing Models and Frameworks. Water-Energy Nexus 1, 17–25. doi:10.1016/j.wen.2018.04.001
Simonovic, S. P., and Fahmy, H. (1999). A New Modeling Approach for Water Resources Policy Analysis. Water Resour. Res. 35, 295–304. doi:10.1029/1998WR900023
Steffen, W., Crutzen, P. J., and McNeill, J. R. (2007). The Anthropocene: Are Humans Now Overwhelming the Great Forces of Nature, AMBIO A. J. Hum. Environ., 36 (8), 614–621. doi:10.1579/0044-7447(2007)36[614
Sterman, J. D. (2000). in Business Dynamics : Systems Thinking and Modeling for Complex WorldScott Isenberg Jeffrey J. Shelsfud (New York, US: McGraw-Hill Education). doi:10.1108/13673270210417646
Summers, J. K., Smith, L. M., Harwell, L. C., and Buck, K. D. (2017). “The Development of a Human Well-Being Index for the United States,” in Quality of Life and Quality of Working Life, Editors L. M. Smith (Rijeka: Ana Alice Vilas Boas, IntechOpen, London, UK), Ch. 6. doi:10.5772/intechopen.68596
Sun, J., and Yang, K. (2016). The Wicked Problem of Climate Change: A New Approach Based on Social Mess and Fragmentation. Sustain 8. doi:10.3390/su8121312
Sušnik, J., Chew, C., Domingo, X., Mereu, S., Trabucco, A., Evans, B., et al. (2018). Multi-stakeholder Development of a Serious Game to Explore the Water-Energy-Food-Land-Climate Nexus: The SIM4NEXUS Approach. Water (Switzerland) 10. doi:10.3390/w10020139
Tatro, M. (2018). New Mexico Energy Roadmap. New Mexico Energy Minerals and Natural Resources Department, Energy Conservation and Management Division, Santa Fe, NM.
Tenza, A., Pérez, I., Martínez-Fernández, J., and Giménez, A. (2017). Understanding the Decline and Resilience Loss of a Long-Lived Socialecological System: Insights from System Dynamics. Ecol. Soc. 22. doi:10.5751/ES-09176-220215
Tidwell, V. C., Moreland, B. D., Shaneyfelt, C. R., and Kobos, P. (2018). Mapping Water Availability, Cost and Projected Consumptive Use in the Eastern United States with Comparisons to the West. Environ. Res. Lett. 13. doi:10.1088/1748-9326/aa9907
Turner, B. L., Menendez, H. M., Gates, R., Tedeschi, L. O., and Atzori, A. S. (2016). System Dynamics Modeling for Agricultural and Natural Resource Management Issues: Review of Some Past Cases and Forecasting Future Roles. Resources 5. doi:10.3390/resources5040040
Ullah, I. I. T., Kuijt, I., and Freeman, J. (2015). Toward a Theory of Punctuated Subsistence Change. Proc. Natl. Acad. Sci. U. S. A. 112, 9579–9584. doi:10.1073/pnas.1503628112
UNDES (2016). United Nations Department of Economic and Social Affairs World Population Prospects; United Nations.
US, DOE (2014). The Water-Energy Nexus: Challenges and Opportunities. Water-Energy Tech Team, U.S. Department of Energy, United States. Available at: https://www.energy.gov/articles/water-energy-nexus-challenges-and-opportunities.
USBC (2019). Population Estimates Program. United States Census Bureau. Available at: https://www.census.gov/data/developers/data-sets/popest-popproj.html.
U.S. BLS (2021). Unemployment Rate in New Mexico [NMUR], Retrieved from FRED, Federal Reserve Bank of St. Louis. Available at: https://fred.stlouisfed.org/series/NMUR. (Accessed February 9, 2021).
USBR (2021). Elephant Butte Reservoir. Available at:https://www.usbr.gov/uc/albuq/water/SanJuanChama/Reservoirs/ebutte_indx.html.
USDA (2013). Farm and Ranch Irrigation Survey. Census Agric. 3, 216 Available at:www.agcensus.usda.gov/Publications/2012/Online_Resources/Ag_Census_Web_Maps/Overview/.
USEPA (1998). Climate Change and New Mexico. United States Environmental Protection Agency, Office of Policy (2111), EPA 236-F-98-007p.
USFS (2019). Extent of U.S. Rangelands. U.S. Forest ServiceAvailable at: https://data.fs.usda.gov/geodata/rastergateway/rangelands/index.php.
Uyttebrouck, O. (2013). Desperate times for N.M. ranchers. Albuquerque Times. May 26. Available at: www.abqjournal.com/203544/news/desperate-times-for-n-m-ranchers.html.
Voiland, A. (2013). Drought Dries Elephant Butte Reservoir. Available at: http://earthobservatory.nasa.gov/IOTD/view.php?id=81714.
WB (2013). Thirsty Energy: Securing Energy in a Water-Constrained World. Available at: www.worldbank.org/en/topic/sustainabledevelopment/brief/water-energy-nexus.
Wendling, Z. A., Emerson, J. W., de Sherbin, A., and Esty, D. C. (2020). Environmental Performance Index. (New Heaven, CT: Yale Center for Environmental Law & Policy). doi:10.4324/9781315226675-5
Werthes, S., Heaven, C., and Vollnhals, S. (2011). Assessing Human Insecurity Worldwide-The Way to A Human. (In)Security Index. Institute for Development and Peace, University of Duisburg-Essen, Essen, Germany (INEF-Report 102/2011).
Willis, H. H., Groves, D. G., Ringel, J. S., Mao, Z., Efron, S., and Abbott, M. (2016). Developing the Pardee RAND Food-Energy-Water Security Index, 60 (Santa Monica, California: RAND Corporation).
Winz, I., Brierley, G., and Trowsdale, S. (2008). The Use of System Dynamics Simulation in Water Resources Management. Water Resour. Manag. 23, 1301–1323. doi:10.1007/s11269-008-9328-7
Wisner, B., Blaikie, P., Blaikie, P. M., Cannon, T., and Davis, I. (2004). At Risk: Natural Hazards, People’s Vulnerability and Disasters. (London: Routledge) 496.
World Bank (2016). Global Economic Monitor (GEM) Commodities, Market Outlook (Washington D.C. World Bank).
World Energy Outlook (2014). World Energy Outlook 2014. Available at:http://www.oecd-ilibrary.org/energy/world-energy-outlook-2014_weo-2014-en.
WRI (2015). Aqueduct Projected Water Stress Country Rankings. Technical Note. Washington, D.C.: World Resources Institute.
WWAP (United Nations World Water Assessment Programme) (2015). The United Nations World Water Development Report, Water for a Sustainable World. Paris, UNESCO.
Xu, Y., and Szmerekovsky, J. (2017). System Dynamic Modeling of Energy Savings in the US Food Industry. J. Clean. Prod. 165, 13–26. doi:10.1016/j.jclepro.2017.07.093
Zaied, A. J., Geli, H. M. E., Holechek, J. L., Cibils, A. F., Sawalhah, M. N., and Gard, C. C. (2019). An Evaluation of Historical Trends in New Mexico Beef Cattle Production in Relation to Climate and Energy. Sustain 11. doi:10.3390/su11236840
Keywords: drought, socioeconomics, FEW nexus performance indicators, resilience index, resilience threshold, system dynamics modeling
Citation: Yadav K, Geli HME, Cibils AF, Hayes M, Fernald A, Peach J, Sawalhah MN, Tidwell VC, Johnson LE, Zaied AJ and Gedefaw MG (2021) An Integrated Food, Energy, and Water Nexus, Human Well-Being, and Resilience (FEW-WISE) Framework: New Mexico. Front. Environ. Sci. 9:667018. doi: 10.3389/fenvs.2021.667018
Received: 11 February 2021; Accepted: 03 May 2021;
Published: 04 June 2021.
Edited by:
Rabi Mohtar, American University of Beirut, LebanonReviewed by:
Bassel Daher, Texas A&M Energy Institute, United StatesCopyright © 2021 Yadav, Geli, Cibils, Hayes, Fernald, Peach, Sawalhah, Tidwell, Johnson, Zaied and Gedefaw. This is an open-access article distributed under the terms of the Creative Commons Attribution License (CC BY). The use, distribution or reproduction in other forums is permitted, provided the original author(s) and the copyright owner(s) are credited and that the original publication in this journal is cited, in accordance with accepted academic practice. No use, distribution or reproduction is permitted which does not comply with these terms.
*Correspondence: Hatim M. E. Geli, aGdlbGlAbm1zdS5lZHU=
†These authors share first authorship
Disclaimer: All claims expressed in this article are solely those of the authors and do not necessarily represent those of their affiliated organizations, or those of the publisher, the editors and the reviewers. Any product that may be evaluated in this article or claim that may be made by its manufacturer is not guaranteed or endorsed by the publisher.
Research integrity at Frontiers
Learn more about the work of our research integrity team to safeguard the quality of each article we publish.