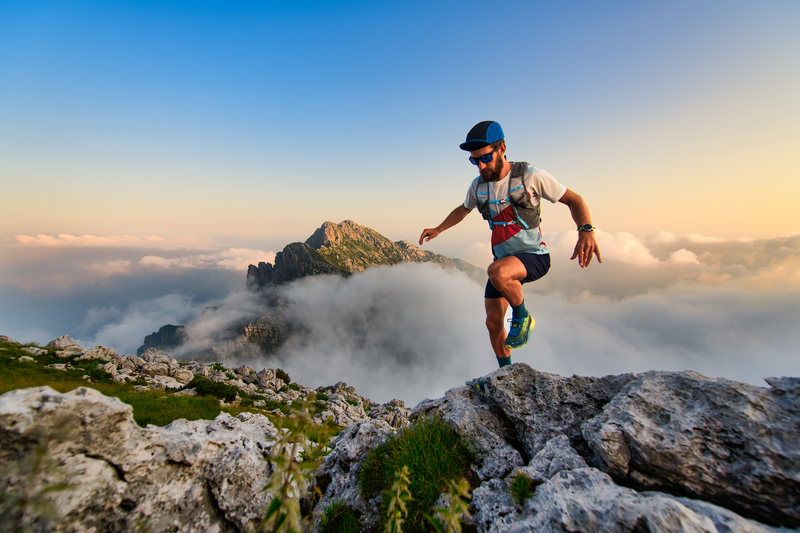
95% of researchers rate our articles as excellent or good
Learn more about the work of our research integrity team to safeguard the quality of each article we publish.
Find out more
ORIGINAL RESEARCH article
Front. Environ. Sci. , 28 May 2021
Sec. Land Use Dynamics
Volume 9 - 2021 | https://doi.org/10.3389/fenvs.2021.657969
This article is part of the Research Topic Nature-Based Solutions for Urban Areas View all 10 articles
Recently, pocket green spaces (PGS), i.e., small green spaces, have attracted growing attention for their various ecological and social services. As a crucial part of urban green spaces in high-density urban areas, PGS facilitates recreation and relaxation for neighborhoods and thus improves the livability of cities at the local scale. However, whether and how the PGS cools the urban heat island effect is still unclear. This research was performed in the highly developed areas of the city of Shanghai during hot summer daytime. We applied a set of cooling effect indicators to estimate the cooling extent, cooling intensity, and cooling efficiency of PGS. We further examined whether and how landscape features within and surrounding the PGS influence its cooling effects. The results showed that 90% of PGS are cooler than their surroundings. Among the landscape features, the land surface temperature of PGS logarithmically decreased with its area, and the maximum local cool island intensity and maximum cooling area logarithmically increased with the area of PGS. The vegetation types and their composition within the PGS also influenced their surface temperature and the cooling effect. The PGS dominated by tree-shrub-grass showed the highest cooling efficiency. The surrounding landscape patterns, especially the patch density and the landscape shape index, influence the cooling effect of PGS at both class and landscape levels. These findings add new knowledge on factors influencing the cooling effect of PGS, and provide the biophysical theoretical basis for developing nature-based cooling strategies for urban landscape designers and planners.
Urbanization is a primary driver of conversion of vegetated areas to impervious surfaces globally (Grimm et al., 2008; Weng, 2012). A primary environmental risk following urbanization is the urban heat island (UHI) effect (Oke, 1982). Urban heat island effect and its associated amplified heatwave could pose extraordinary heat stress on urban organisms (Harlan et al., 2006) and cause considerable heat-related health risks for urban residents, such as increasing heat-related morbidity and mortality (Tan et al., 2010; Gabriel and Endlicher, 2011; Harlan and Ruddell, 2011; Chan et al., 2012; Dong et al., 2020). In addition, UHI can lead to an increase in energy and water consumption (Santamouris, 2014). Therefore, how to mitigate the UHI effect is vital to urban public health and sustainable development.
Several heat mitigation strategies, such as using high albedo material, vegetation or green space, and water bodies, have been proposed to alleviate the UHI effect in different climatic contexts (Akbari et al., 2003; Norton et al., 2015; Zhao and Fong, 2017; Morini et al., 2018). Among these, urban green space has been widely used to mitigate the UHI effect due to its cooling effects via evapotranspiration and shading (Kong et al., 2014b; Cheng et al., 2014; Jaganmohan et al., 2016; Sun and Chen, 2017). Urban green space has been considered as one of the nature-based solutions approaches to help improve climate change adaption and sustainable land management (Ferrari et al., 2019; Haase, 2021). Major findings from previous studies on the effects of urban green space on UHI can be summarized in the following: 1) the vast majority of urban green spaces have cooling effect on their surroundings, mitigating the UHI, but a few green spaces were warmer than their surroundings (Chang et al., 2007; Cao et al., 2010; Cheng et al., 2014); 2) linear relationships existed between land surface temperature and biophysical parameters such as the normalized difference vegetation index (NDVI), vegetation fraction at city scales (Weng et al., 2004; Li et al., 2011); 3) nonlinear relationship existed between the size of urban green spaces and their cooling effect when considering the local cool island intensity at patch and class scales (Cao et al., 2010; Cheng et al., 2014; Yu et al., 2017); 4) the landscape pattern of urban green space influenced urban land surface temperature (Li et al., 2011; Zhou et al., 2011; Kong et al., 2014a; Sun and Chen, 2017; Masoudi and Tan, 2019); 5) the surrounding landscape pattern of urban green space affected its cooling effect (Cao et al., 2010; Cheng et al., 2014).
These studies are helpful for understanding the cooling effects of urban green space and provide insights for urban planning. However, most of the previous investigations were mainly focused on different types of urban green space, including parks (Chang et al., 2007; Lin and Lin, 2010), gardens, and residential green spaces (Wu et al., 2016; Zhen et al., 2019), and forests (Kong et al., 2014a). These studies were generally focused on relatively large green spaces. Less attention has been paid to urban small green spaces (Lin et al., 2017).
Due to space limitations in urban areas, cities once characterized by extensive green spaces are ever shifting to be more compact and dense (Armato, 2017). Often large urban green space is encroached or fragmented. Therefore, small urban green spaces, here named as pocket green spaces (PGS), have become one of the major components of open spaces in high-density urban areas. The PGS plays an important role in providing various ecosystem services, which the large greenspace used to provide (Peschardt et al., 2016; Lin et al., 2017; Wei and Lu, 2018).
Currently, there is no consensus on the definition of PGS. It was generally defined in terms of its size (Peschardt and Stigsdotter, 2013; Lin et al., 2017). According to some studies (Table 1), green space with a size less than 2 ha in urban and suburban areas can be regarded as a PGS (Currie, 2017; Amaya-Espinel et al., 2019). In recent years, increasingly more studies had been dedicated to the diverse ecosystem services that PGS provide, such as serving as wildlife habitat for biodiversity conservation of birds (Ikin et al., 2013; Jasmani et al., 2017; Amaya-Espinel et al., 2019), fostering community interaction and recreation (Gibson and Canfield, 2016), and generating health benefits (Cohen et al., 2014; Peschardt et al., 2016; Lin et al., 2019), as PGS can be effectively deployed in urban areas due to its small size. PGS has started becoming an important component in modern urban planning and design (Nordh and Østby, 2013; Armato, 2017; Danford et al., 2018; Hu, 2018; Labuz, 2019; Balai Kerishnan et al., 2020). However, few studies have paid attention to the cooling effect of urban PGS. A recent research by Lin et al. (2017) in Hong Kong showed that urban pocket parks could help to mitigate the UHI intensity at the microscale in the high-rise, high-density urban environment. More studies on the cooling effects of urban PGS are needed in many more cities to further understand its role in mitigating UHI.
Numerous studies use cooling intensity, i.e., the temperature difference between the green space interior and its nearby surroundings, to evaluate the cooling effect of urban green space (Chang et al., 2007; Cao et al., 2010; Kong et al., 2014b). Studies found that urban green space can cool well beyond its own physical boundaries (Upmanis et al., 1998; Cheng et al., 2014; Lin et al., 2015; Andersson et al., 2020). However, few studies quantified how far the cooling effect of urban green space could reach. Therefore, more indicators of cooling distance or extent are needed. Recently, Cheng et al. (2014) established a multi-indicator evaluation framework to estimate the cooling intensity, distance and area, and efficiency of urban parks, which has been used to comprehensively measure the cooling effect of urban green and blue spaces from different perspectives (Wu et al., 2019; Peng et al., 2021). However, whether such indicators and framework are appropriate for urban PGS remains to be verified. The cooling effects of PGS and the influence of landscape characteristics within and surrounding PGS on their cooling effect are not well understood.
This study aims to quantitatively evaluate the cooling effect of PGS on urban surface temperature and to identify the key factors that determine the cooling effect. We specifically focus on the following questions: 1) What is the relationship between landscape features of PGS and its land surface temperature? 2) How do the landscape features of PGS influence its cooling effect? and 3) How do the surrounding landscape patterns impact the cooling effect of PGS? The answers to these questions will provide novel insights for urban landscape designing and planning regarding the use of PGS for UHI mitigation.
Shanghai is a megacity in China, located in 30°40′N-31°53′N, 120°52′E-122°12′E. The city faces the East China Sea on the east, bordered by Hangzhou Bay on the south, and Yangtze River on the north, enjoying a superior geographical position with convenient traffic settings on both land and sea. Shanghai covers an area of 6,340.5 km2 (SMBS, 2019). After thirty years of rapid urbanization since 1990, the proportion of the build-up area has reached 46% in 2015 (The Master Plan of Shanghai 2017-2035). Nearly 50% of the permanent resident population of the city live in the central urban area. Shanghai situates in the subtropical monsoon climate zone. Previous studies found a distinct UHI effect in the summer (Tan et al., 2010; Li et al., 2011). More hot days and heatwaves were observed in urban areas than in rural areas, bringing adverse effects on human health (Tan et al., 2010). The frequency of heat wave events in Shanghai is increasing in the 21st century (Chen et al., 2013). Therefore, how to effectively use urban green space as a nature-based solution to mitigate the UHI effect and to ameliorate the urban thermal environment are of great interest to urban residents and urban planners and designers. We focus on an area located within the Outer-Ring Road of Shanghai, encompassing the entire central urban area. Therefore, the study area is suitable to explore the cooling effect of PGS in a high-density metropolitan area.
There are more than ten thousand small green spaces in the urban area of Shanghai. In this study, We define a PGS as an urban public green space whose area is larger than 0.09 ha but less than 3.5 ha, including mini urban parks and street and roadside greenways (excluding the single isolated tree or single row street trees). Peng et al. (2021) demonstrated that the cooling effect of urban parks could overlap each other. Thus, to identify the UHI mitigation effect of individual green spaces, it is necessary to select green spaces, which are not overwhelmed by the other green or blue spaces (Cheng et al., 2014). To do so, the selected green spaces have to meet the following criteria: 1) The area of the green space must be more than 0.09 ha but less than 3.5 ha, which can be identified in the Landsat Thermal image; 2) There should be no larger green spaces within 100 m away from the surrounding green spaces; 3) There should to be no large blue spaces (river, lake, etc.) within 300 m in the vicinity; 4) the total vegetation (trees, shrubs, and grass) coverage within green space should exceed 80%. Consequently, a total number of 41 PGS were identified via visual interpretation from aerial photos in 1-meter resolution, and their boundaries were digitized with a geographic information systems tool (Figure 1). The areas of selected PGS range from 0.17 to 3.5 ha with mean of 0.81 ha.
FIGURE 1. Spatial distribution of sampled PGS in the study area (the high-resolution image was one of the PGS in RGB).
In this study, the landscape features of the PGS mainly refer to its area and the dominant vegetation type within the green space. We identify three vegetation types in PGS, including trees, shrubs, and grass. These three types of vegetation can make up seven vegetation structure combinations, i.e., trees, shrubs, grass, tree-shrub, tree-grass, shrub-grass, and tree-shrub-grass, respectively. We first identified the vegetation types within each PGS via visual interpretation based on high spatial resolution aerial photos, images on Google Earth, and Baidu Panoramic Map (http://quanjing.baidu.com/), then further verified on-screen visual interpretation through field visits. We did not find PGS with a pure shrub type.
The land surface temperature (LST) was retrieved from a cloud-free Landsat 8 thermal image. Although the original spatial resolution of Landsat 8 thermal band is 100 m, it was resampled to 30 m spatial resolution using cubic convolution resampling method at EROS Data Center when downloaded. The image was acquired on August 3, 2015. Firstly, the at-sensor spectral radiance was converted to effective at-sensor brightness temperature using the following equation (Chander et al., 2009):
where TB is the effective at-sensor brightness temperature (oK);
The brightness temperature calculated with Eq. 1 is for a black body. Therefore, it needs to be converted to LST with the correction for spectral emissivity (ε) of a gray body. The emissivity-corrected land surface temperature (Ts) is computed as in the following (Artis and Carnahan, 1982):
where λ is the wavelength of emitted radiance, taking a value of 10.90 μm (the center of the bandwidth for Landsat 8 TIRS 10); and α = hc/b (1.438 × 10–2 mK); h is the Planck’s constant (h = 6.626 × 10–34 Js); b is the Boltzmann constant (b = 1.38 × 10–23 J/K); c is the speed of light (2.998 × 108 m/s); ε is the surface emissivity and was estimated using the equation proposed by Li et al. (2011), ε = 0.02644Fv + 0.96356, where Fv is the vegetation fraction, and estimated from NDVI according to Yu et al. (2014).
The land surface temperature in the study area ranges from 27 to 50°C in the thermal image, as shown in Figure 2. The hotspots with a high land surface temperature higher than 40°C were dispersed and spatially associated with high-density built-up area. In comparison, the relatively cool spots with a temperature lower than 34°C were mainly concentrated in urban green and blue spaces, such as Huangpu River and the green Urban Parks.
Land-cover types were classified using the Landsat 8 OLI image by the following steps. First, water bodies were extracted using a modified normalized difference water index with the threshold value of 0.05 (Wu et al., 2019). Second, the built-up land and green land were classified using maximum likelihood. Finally, three land-cover types, including build-up land, water body, and green land, were mapped with a Kappa coefficient for an overall accuracy of 0.79. They were shown in Figure 2.
The evaluation of the cooling effect of green space was based upon the concept of the local cool island intensity (LCII), which was initially proposed by Chang et al. (2007), defined as the temperature difference between the inner park and its nearby surroundings. We employed the four cooling indicators proposed by Cheng et al. (2014) to measure the cooling effect of PGS in Shanghai, i.e., the maximum local cool island intensity (MLCII), the maximum cooling distance (MCD), the maximum cooling area (MCA), and the maximum cooling efficiency (MCE). To calculate the cooling effect indicators, we create a series of 10 m-width buffer rings up to 500 m starting from the boundary of each PGS, then calculate the mean land surface temperature within each buffer ring and that of the PGS. It is inevitable that some of the buffer rings of the PGS could partially overlapped each other when the distance between the PGS is within 1,000 m. The LST in the overlapping buffer rings may be influenced by both PGS, therefore, must be corrected. The temperature in the overlapping areas will be estimated using the following equation (Wu et al., 2019):
where, Tbi is the mean LST of a given buffer i of the target PGS A. Tij is the LST of a pixel in the buffer i; N is the total number of pixels in the buffer i; fij is the weight that a pixel carries for buffer i. If a pixel is located in a non-overlapping buffer, fij takes a value of 1. If pixel j is in an overlap zone with another PGS B, fij is calculated with the following equation:
where dAij is the distance of pixel j in buffer i to target PGS A, and dBij is the sample pixel to PGS B.
To estimate the cooling effect of the PGS, the four indicators were calculated using the equations developed by Cheng et al. (2014) as follows.
The first indicator is the maximum cooling distance (MCD). It is obvious that the MCD depends on the local cool island intensity (LCII), which is strongly affected by the surrounding landscape setting. LCII is the difference between the mean land surface temperature in each buffer ring and the mean land surface temperature of PGS. Generally, the LCII of a green space decreases with the distance from the boundary of the green space and eventually reaches equilibrium with its surroundings. For each pocket green space, there may exist a relationship between LCII and the cooling distance, and there may be several peaks along the way due to the influence of the surrounding landscape. The distance between the PGS and the first LST peak in its surrounding is defined as the maximum cooling distance (Cheng et al., 2014).
The second indicator is the maximum local cool island intensity (MLCII), which was defined as the difference between the land surface temperature corresponding to the MCD and the mean land surface temperature within the PGS, and was calculated using Eq. 5:
where Ts is the land surface temperature corresponding to where the MCD occurred, Tp is the mean surface temperature of the PGS.
We identify the LST peaks along the buffer zones by fitting a polynomial function of the temperatures within the buffers with the buffer distance for each of the 41 PGS. Among the 41 selected PGS, there are 2 PGS which presented no robust fitting curves and 2 PGS that behave as weak heat islands. Therefore, we rely on the 37 remaining PGS to calculate their maximum cooling distance and the associated cooling area and efficiency. The final polynomial fittings for the 39 PGS (excluding 2 PGS with no fitting curves) were showed in Supplementary Table S1.
The third indicator, the maximum cooling area (MCA), was referred to as the largest area, through which the PGS’s cooling effect would extend (Cheng et al., 2014). To calculate the MCA, we need to set two threshold values. The one is the minimum threshold value of Ta, refers to the average land surface temperature of the PGS. The other is the threshold value of Tmax, refers to the maximum land surface temperature corresponding to the maximum cooling distance. The sum of all pixels within the MCD with their land surface temperature between Ta and Tmax is the MCA. Pixels with temperatures outside this range are not counted toward MCA.
The fourth indicator is the maximum cooling efficiency (MCE), which was defined as the ratio of maximum cooling area to the size of the PGS.
where MCA is the maximum cooling area, A is the area of the PGS.
Landscape metrics have been widely utilized to characterize landscape patterns (Wu et al., 2002; Li and Wu, 2004; Weber et al., 2014a; Weber et al., 2014b), and landscape composition and configuration are two aspects of landscape patterns that can be linked to PGS to examine their impacts on PGS’ cooling effects. Many landscape pattern metrics have been developed in literature at both the landscape and class levels, and many provide similar information. We carefully selected eight metrics at the landscape level and seven metrics at the class level to characterize the composition and configuration of the areas surrounding the PGS and examine their impacts on PGS’ cooling effects. The eight metrics at the landscape level include patch density (PD), edge density (ED), landscape shape index (LSI), aggregate index (AI), mean Euclidean nearest neighbor distance (ENN_MN), Contagion(CONTAG), Shannon’s diversity index (SHDI), and Shannon’s evenness index (SHEI), and the seven metrics at the class level are Percentage of landscape (PLAND), clumpiness (CLUMPY), patch density (PD), edge density (ED), landscape shape index (LSI), aggregate index (AI), mean Euclidean nearest neighbor distance (ENN_MN). The description and calculation of the landscape metrics were listed in Supplementary Table S1. These metrics are calculated based on the land-cover map using Fragstats version 4.2 (McGarigal et al., 2012).
To quantify the relationships, the logarithmic regression analysis was conducted to relate the area of PGS to its own land surface temperature, the maximum local cool island intensity, and the maximum cooling area as:
where Y is the mean LST, maximum local cool island intensity, or maximum cooling area of PGS, and X represents the area of PGS.
Pearson correlation analysis was used to measure the association between surrounding landscape patterns and the cooling effect of PGS. Pearson correlation analysis method requires the variables to satisfy normal distribution, so the variable that did not follow normal distribution were transformed using logarithmic transformation. These steps were done in SPSS software.
Among the 41 PGS, the lowest mean land surface temperature (LST) of pocket green space (PGS) was 35.2°C with an area of approximately 3.5 ha, while the highest mean land surface temperature of PGS was 39.6°C with the size of 0.6 ha. The average of the mean land surface temperature of all PGS was 37.6°C.
There is a negative relationship between the land surface temperature of PGS and its area. The mean land surface temperature of PGS decreased logarithmically with the increase of PGS area (Figure 3).
It was shown that the mean LST varied with the PGS’s vegetation type (Figure 4). The tree-shrub type PGS had the lowest mean LST, followed by the shrub-grass type. Their mean LSTs were 36.6 and 37.8°C, respectively. The highest mean LST was occurred in the grass-type PGS.
FIGURE 4. The boxplot of mean LST for PGS dominant with different vegetation type (the dashed line is the mean value, the solid line is the median, Tr., Sh. and Gr. represents PGS dominated with vegetation type of Tree, Shrub and Grass, respectively).
We found that the PGS’ maximum local cool island intensity could range from −0.4 to 3.6°C with a mean of 1.2°C. The lower bound is negative because there is three PGS functioning as heat islands in the study area. The maximum cooling distance could reach 213 m with a mean of 132 m. The maximum cooling areas varied from 0.1 to 16.8 ha, averaging 5.7 ha. In contrast, the maximum cooling efficiency varied greatly from less than 0.3 to 24.6 times of the area of the PGS with a mean of 6.8 (Table 2).
TABLE 2. Statistics of the cooling effect indicators of the 41 PGS selected in the central urban area of Shanghai based on remotely sensed data from Landsat 8 collected on August 3, 2015.
There was a significant relationship between the PGS area and its maximum local cool island intensity. The maximum local cool island intensity increased logarithmically with the area (Figure 5A). There were three PGS whose maximum local cool island intensity was less than zero, meaning that they were hotter than the surroundings. There was no significant relationship between the PGS area and its maximum cooling distance (Figure 5B). The maximum cooling distance of the largest PGS was 190.5 m, and the largest maximum cooling distance was 213 m and was observed in the PGS with an area of 1.2 ha. The area of PGS had a significant impact on the maximum cooling area (Figure 5C). The maximum cooling area increased with the PGS’ area, and a positive logarithmic relationship existed between them. No significant statistical relationship existed between the area of PGS and the maximum cooling efficiency (Figure 5D). However, most PGS have cooling efficiencies that are more than five times their own area.
FIGURE 5. Relationship between the area and cooling effect indicators of PGS (the dash line in (A) means that parks above it have cooling effect, the dash line in (B) indicates the mean value of Max. cooling distance).
The dominant vegetation type in the PGS also influences the cooling effect of the PGS (Figure 6). We found that tree-shrub PGS has the highest MLCII with an average value of 2.1°C (SD = 0.8°C), and the grass PGS has the lowest MLCII at 0.8°C (SD = 0.5°C). The ranking of MLCII from high to low for PGS with different vegetation types was tree-shrub, tree, tree-shrub-grass, shrub-grass, tree-grass, and grass type, respectively (Figure 6A). Tree type of PGS had the largest MCD with an average value of 152.8 m (SD = 55.9 m), and grass type had the smallest MCD with an average of 86.7 m (SD = 61.0 m). The order of MCD from large to small for PGS with different vegetation types was tree-shrub, tree, tree-shrub-grass, shrub-grass, tree-grass, and grass (Figure 6B). The tree-shrub-grass PGS had the largest MCA with a mean of 8.2 ha (SD = 5.4 ha), while the grass PGS had the smallest MCA with an average of 1.7 ha (SD = 1.1 ha). MCA for PGS with different vegetation types decreased sequentially in tree, tree-shrub, tree-shrub-grass, tree-grass, shrub-grass, and grass (Figure 6C). For MCE, PGS with tree-shrub-grass and grass have the highest and lowest values at 11.5 (SD = 9.2) and 4.2 (SD = 3.8), respectively. MCE for PGS decreases with tree-shrub-grass, tree, tree-shrub, shrub-grass, tree-grass, and grass type (Figure 6D). Generally, the tree-shrub-grass was the best vegetation composition that had the best cooling effect.
FIGURE 6. The boxplot of cooling effect indicators for PGS with different vegetation types (the dashed line is the mean value, the solid line is the median, Tr., Gr., Tr.Sh., Tr.Gr, Sh.Gr., Tr.Sh.Gr. represent the vegetation type of tree, grass, tree-shrub, tree-grass, shrub-grass, tree-shrub-grass, respectively).
The cooling effect of PGS were significantly affected by the surrounding landscape pattern at the landscape level (Table 3). Significant negative correlations were found between four cooling effect indicators of maximum local cool island intensity (MLCII), maximum cooling distance (MCD), maximum cooling area (MCA), maximum cooling efficiency (MCE), and patch density (PD) of the surrounding landscape. These relationships imply that the increasing landscape fragmentation of the PGS surroundings would enhance the LST, weakening the cooling effect of PGS. There were significant positive correlations between landscape shape index (LSI), edge density (ED), and the MCE, indicating that the more shape irregularity and complexity of surrounding landscape, the better the cooling efficiency of PGS. Mean Euclidean nearest neighbor distance (ENN_MN) of the surrounding landscape had significant positive influences on the MLCII, the MCD, and the MCA. Thus, the farther away of the patches in the same land-cover type at the landscape level, the better the cooling effect of PGS is.
TABLE 3. Pearson correlation coefficients between cooling effect indicators of PGS and landscape metrics at landscape scale.
The cooling effect of PGS was also affected by the landscape patterns of surrounding land-cover types at the class level (Table 4). Among the class level pattern metrics, patch density of all classes shows consistent negative effects on all PGS cooling indicators, although not all are statistically significant. There were statistically significant negative correlations between the maximum cooling distance (MCD), the maximum cooling area (MCA), and patch density (PD) of green and built-up land types, respectively. The PD of green and built-up land significantly negatively influenced the maximum local cool island intensity (MCLII) and maximum cooling efficiency (MCE). The landscape shape index (LSI) of green space was positively correlated to the four cooling effect indicators. The LSI of built-up land also positively relate to the MCD and MCA. The mean Euclidean nearest neighbor distance (ENN_MN) of green land had impacts on the MCLII, and the same trend for the ENN_MN of built-up land to MCD. The aggregate index (AI) and the clumpiness (CLUMPY) of the water body significantly positively affected the MCD. In addition, the CLUMPY of built-up land also positively impacted the MCD.
TABLE 4. Pearson correlation coefficients between cooling effect indicators of PGS and landscape metrics at class scale.
The PGS indeed could be cool islands and lower the urban land surface temperature. First, our results showed that the vast majority of the PGS (37 out of 41 PGS, or 90%) were cooler than their surroundings, and only two PGS with an area less than 1 ha (accounting for 4.8%) were hotter than their surroundings. This finding is similar to previous studies on urban parks, particularly in the summer daytime. For example, a study conducted in Taipei, China, reported that most of 61 city parks were cooler than their surroundings, but nearly one-fifth of the parks were warmer than their surroundings due to more than 50% paved coverage and low tree- and shrub-cover (Chang et al., 2007). A study in Nagoya, Japan, showed that parks’ cool island intensity varies with seasons, and most of the 92 sampled urban parks were cooler than their urban surroundings in spring, summer, and autumn. However, there nearly 21 parks in spring, 26 parks in summer, and 32 parks in autumn appeared hotter than their surroundings because the higher buildings’ shadow and higher vegetation coverage resulted in lower LST of the surroundings (Cao et al., 2010). While in Shanghai, two of the 39 urban parks were warmer than their surroundings because of the disturbance from the nearby river and higher vegetation coverage (Cheng et al., 2014). After careful examination of the two hot spot PGS that we encountered in this study, we found that they were located near residential areas with higher green space coverage, which functions as a cool island, overwhelming the PGS’ cooling effects. Therefore, The PGS were warmer than their surroundings.
Second, the LST of urban green space is affected by the landscape components or composition, such as the vegetation types and their coverage, and landscape configuration, such as area and shape etc. (Chang et al., 2007; Kong et al., 2014a). Our study confirmed these findings. The mean land surface temperature of PGS decreased logarithmically with the area of PGS (Figure 3). This finding is very similar to the findings in urban parks in Nagoya, Japan (Cao et al., 2010) and Shanghai, China (Cheng et al., 2014), in which the park area could explain nearly 60 and 37% of the land surface temperature variations within PGS, respectively. However, our results showed that the PGS’ area only explained 31% of surface temperature variation, suggesting that the area of PGS cannot satisfactorily explain its LST, indicating more factors contribute to the LST of PGS due to its small size. Our findings also showed that vegetation components of PGS could influence the land surface temperature within the PGS (Figure 4). The tree-shrub dominated PGS have the lowest surface temperature, followed by the PGS with tree-grass, tree-shrub-grass, and tree-grass types with increasing temperature. PGS with the single vegetation type exhibit relatively higher land surface temperature, suggesting that a composite vegetation within a PGS will be more effective in lowering its LST than the ones with single vegetation type. Therefore, a composite vegetation composition for PGS should be preferred by urban green landscape design when considering its cooling effect.
Urban green space can mitigate urban thermal environment during the hot summer days (Maimaitiyiming et al., 2014; Weber et al., 2014c; Zhou et al., 2017; Xiao et al., 2018; Cârlan et al., 2020), however, most of the previous studies utilized single indicator, i.e., local cool intensity, to measure the cooling effect of green spaces (Chang et al., 2007; Cao et al., 2010; Kong et al., 2014b). Our study utilized four cooling effect indicators proposed by Cheng et al. (2014) to quantitatively measure the cooling effect of PGS. We found that PGS has considerable cooling effects at the local scale. The average maximum local cool island intensity of all the 41 PGS examined in this study reached 1.2°C, which was equivalent to that in urban parks in Nagoya, Japan (Cao et al., 2010), but less than that (1.8°C) observed in urban green spaces in Fuzhou, China (Yu et al., 2017), that (2.2°C) of urban green spaces in Bengaluru, India (Shah et al., 2021), and that (3.0°C) of large urban parks in Shanghai (Cheng et al., 2014). The maximum cooling distance could reach 213 m with an average of 132 m in our study (Table 2). The previous studies reported that the average cooling distance could be 276.7 m for urban parks in Shanghai (Cheng et al., 2014), 104 m for urban green spaces in Fuzhou (Yu et al., 2017), 347 m for urban green spaces in Bengaluru, India (Shah et al., 2021). The maximum cooling distance was estimated at 240 m in Addis Ababa, Ethiopia (Feyisa et al., 2014). The average maximum cooling area of PGS was 5.7 ha which was much greater than the area of PGS themselves, but this was less than that of 46.5 ha observed in urban parks in Shanghai (Cheng et al., 2014). While the average cooling efficiency of PGS was 6.8, which is much larger than those of 1.36 and 2.54 observed in urban parks in Shenzhen (Peng et al., 2021) and Shanghai (Cheng et al., 2014), respectively. It is important to note the much larger cooling efficiency of PGS compared to urban green space in general because the PGS can be easily imbedded in the high-density urban environment, enhancing its cooling efficiency. Overall, The PGS can significantly and effectively lower the LST of their surroundings, ameliorating urban thermal environment.
Previous studies demonstrated that land cover components drove the variations of land surface temperature (Weng, 2009; Buyantuyev et al., 2010). In addition, the composition/configuration of the urban landscape could greatly affect land surface temperature (Zhou et al., 2011). Zhu et al. (2002) reported that the green space with shrub-grass or tree-grass composition had the best cooling effect in Harbin, China. The tree-covered urban green space had a stronger cooling effect than the grass-covered in both the Temperate Monsoon Climate and Mediterranean Climate (Yu et al., 2018b). Our result showed that the cooling effect indicators were affected by the landscape features of the PGS itself. For example, the maximum local cool island intensity and maximum cooling area were logarithmically increased with the area of PGS. The cooling effect was also influenced by vegetation types and their composition in the PGS. For instance, the largest maximum cool island intensity occurred in the PGS dominated with the tree-shrub type, and the PGS dominated with tree type had the longest maximum cooling distance, and the tree-shrub-grass possessed the largest cooling area and cooling efficiency.
The tree-dominated vegetation composition exhibited better cooling effect may be due to 1) tree-dominated vegetation composition have a larger and thicker canopy, therefore, can provide more shading with higher evapotranspiration than those of the other vegetation types; 2) the taller tree-dominated vegetation composition can effectively reduce wind speed and generate more eddies within the boundary layer to facilitate heat dispersion, generating stronger cooling effect (Erell et al., 2011); and 3) multiple vegetation types enhance the surface roughness, providing greater cooling effect (Zhao et al., 2014).
The spatial pattern of the landscape can significantly affect the land surface temperature (Li et al., 2011; Zhou et al., 2011). Most previous studies revealed the relationship between the cooling effect of green space and the landscape pattern itself (Zhou et al., 2011; Kong et al., 2014a; Zhou et al., 2017). However, since the urban green space has been greatly promoted for mitigation of the local urban heat island, it is important to understand the impacts of the landscape composition and configuration in their surrounding landscape on the cooling effects. Recent studies have demonstrated that the landscape pattern around the green space significantly affects the cooling effect (Oliveira et al., 2011; Cheng et al., 2014). Our results also supported these findings for PGS at both landscape and class levels.
We found that the surrounding landscape pattern could significantly influence the cooling effect of green space. At the landscape level, the patch density and mean Euclidean nearest neighbor distance had significant impacts on the cooling effect of PGS. Our results showed that increased shape complexity indicated by landscape shape index and the isolation distance between patches measured by the mean Euclidean nearest neighbor distance within the nearby surroundings would increase the cooling effect of PGS. In contrast, the cooling effect would decrease with the increasing fragmentation of the nearby surroundings indicated by patch density. At the class level, the cooling effect of PGS was also significantly affected by patch density and landscape shape index of the surrounding landscape. The increase of the complexity of the shape of surrounding green land would enhance the cooling effect of PGS. The results were consistent with the previous study. As the landscape shape of natural or semi-natural green spaces, such as water bodies and green spaces, became more complex, it enhances the cooling effect of urban parks (Cheng et al., 2014).
The landscape pattern of the surroundings of PGS influenced the cooling effect of PGS may be due to the following mechanisms. First, our study using the local cool island intensity concept to quantify the local cooling effect, which greatly depends on the LST difference between the surroundings and PGS, while the surrounding landscape pattern facilitates or impedes heat exchange, influencing the LST itself. Second, The previous studies demonstrated that the ecological context of the surroundings, especially the climatic conditions (Wang et al., 2020), the shading, and transpiration (Jiao et al., 2017) had significant effects on urban trees’ cooling efficiency. Third, the landscape structure, such as edge complexity and the wider street canyon, facilitates or impedes the energy exchanges between PGS and their surroundings, therefore, influencing the cooling effect of PGS (Yan et al., 2019). Further studies are needed to better quantify the impact of the surrounding landscape pattern on the PGS cooling efficiency.
Nature-based solutions, such as natural systems agriculture, ecosystem-based approach, green infrastructures, and their combinations, are innovative approaches to ameliorate adverse environmental changes and hazards caused by human activities in the long term (Eggermont et al., 2015). Currently, urban green space is widely employed as a vital natural element in sustainable urban development, as it could effectively improve urban local climate and outdoor thermal comfort (Erell, 2017; Andersson et al., 2019; Pauleit et al., 2019). Therefore, how to utilize the PGS to mitigate the urban thermal environment, especially through optimizing its vegetation composition in high-density urban areas, is very important.
This study identified some strong relationships between the landscape patterns in the PGS surrounding areas and its cooling effect. It provides novel insights relevant to urban planning and landscape design. Firstly, the cooling effect of PGS was particularly significantly influenced by its size, which explained 30 and 26% variations in the maximum local cool island intensity and maximum cooling area, respectively. Increasing the size of the PGS would enhance its cooling effect. Secondly, the logarithmic relationship between the maximum local cooling island intensity and the size of PGS means that there is a certain threshold for the area of the PGS, exceeding which the cooling efficiency would no longer increase much (Cheng et al., 2014; Yu et al., 2018a; Wu et al., 2019). Such a threshold value should be highly valuable in design PGS in urban landscape design for the economical use of green space. Due to space limitations in the high-density urban area, the construction of large urban green spaces is unrealistic (Rotem-Mindali et al., 2015). Thirdly, our result showed that tree-dominated composite vegetation compositions, such as tree-shrub-grass, tree-shrub, and tree-grass types, had a better cooling effect than non-tree vegetation and thus should be preferred for PGS (Zhang et al., 2017) to maximize its cooling effect. Lastly, the cooling effect of the PGS was also affected by the surrounding landscape patterns. Thus, synergizing the effects of the green space and the surrounding landscape could optimize the mitigation effect of PGS on urban surface heat islands.
From the application perspective, our study used land surface temperature derived from remotely sensed data of Landsat 8, which are now widely available free charge, and our threshold concept and research approach could serve as a “template” to be adopted for similar studies in large cities around the world. In addition, the results could be valuable information for urban planning and management. In accordance with the New Leipzig Charter as most current urban policy document of the European Union, well-designed, managed, and connected urban green and blue areas are a requirement for healthy living environments, which help adapt to climate change, enable climate-neutral, resilient, and environmentally sound urban development, and improve air quality. The high pressure on land-use intensification in large cities around the world increases the importance of the PGS for their complex ecosystem services, such as UHI mitigation and urban resilience enhancement.
There were several limitations in our study that should require attention in the future. First, our study used the land surface temperature derived from Landsat 8 thermal band instead of the traditional air temperature. Therefore, we could not capture and evaluate the cooling effect of PGS at night. Although there are several satellites, such as MODIS, which provide nighttime land surface temperature products, they cannot clearly identify the thermal performance of our PGS due to their coarse spatial resolutions.
Second, the original spatial resolution of the remote sensing thermal image from Landsat 8 is 100 m and is relatively coarse for exploring the cooling effect of PGS. Although the thermal image was resampled to 30 m, it still has the following limitations: one is that the PGS with areas less than 0.09 ha cannot be well delineated, and the other is that the PGS with areas less than 0.09 ha, even larger than 0.09 ha, may manifest as a mixed pixel on the thermal image, therefore, influencing the accuracy of their LST estimation. A series of buffer widths were used to study the cooling effect of urban green space, ranging from 20 m for urban parks (Cheng et al., 2014), to 30 m for urban green spaces (Yu et al., 2017), and to 50 m for urban wetlands (Sun et al., 2012). In this study, we delineated 10 m width buffer rings around the PGS due to the small size of PGS and our intention to capture detailed landscape patterns in the surroundings of PGS. The small buffer width may result in land surface temperature homogeneity for some pixels across neighboring buffer rings, but it is able to capture the horizontal variations of land surface temperature within a buffer ring.
Third, there are some residential areas surrounding some of the selected PGS, within which vegetation cover can be high, lowering the surface temperature of the surroundings. But the residential areas are not designated as green space. Therefore, some of the green space within the residential areas will inevitably interfere with the cooling effect of the PGS, leading to overestimate the cooling effect of PGS. But it is difficult to separate the interference of the residential green space from the cooling effects of PGS. In recent years, with the advent of Unmanned Aerial Vehicle (UAV) remote sensing, the UAV-borne very-high spatial resolution, hyperspectral and thermal images (Zarco-Tejada et al., 2012; Feng and Li, 2019; Schiefer et al., 2020), such as those used for grapevine (Maimaitiyiming et al., 2020), can be employed to characterize the detailed, diurnal real-time canopy and thermal information of PGS in the future.
This paper investigated the cooling effect of PGS and its influencing factors. We found that the vast majority of the PGS had a cooling effect on their surroundings. The area and vegetation types of the PGS could influence its cooling effects, which were quantified by the maximum local cool island intensity, maximum cooling distance, maximum cooling area, and maximum cooling efficiency. The land surface temperature of PGS logarithmically decreased with its area and was also affected by the composition of vegetation types. The lowest land surface temperature occurred in PGS with the tree-shrub composition.
The maximum local cool island intensity could reach 3.6°C with an average of 1.2°C, and the maximum cooling area could be as large as 5.7 ha on average, both of which increased logarithmically with the area of PGS. The maximum cooling distance reached 132 m on average, while the maximum cooling efficiency was 6.8 times the size of PGS. The cooling effect was also affected by the vegetation type within the PGS. Patch density in the surroundings of PGS negatively correlated with cooling effect indicators. Landscape shape index and mean Euclidean nearest neighbor distance positively correlated with the cooling effect, which demonstrated that fragmentation, connection, and complexity of the surrounding landscape also played vital roles in affecting the cooling effect at class and landscape levels, respectively. Our findings provide novel and practical insights for urban landscape optimization in urban planning and design in the early stage of park and neighborhood development.
The raw data supporting the conclusions of this article will be made available by the corresponding author upon reasonable request.
JL and CWU conceived and designed the research, CWU and CWA performed the research, CWU wrote the manuscript, JL, CS, DH, JB, and MF reviewed and edited the manuscript.
This research was partly supported by the Natural Science Foundation of China (Grant No. 31870453 to JL, Grant No. 32001162 to CWU, and Grant No. 31971485 to CWA). Dagmar benefited from the GreenCityLabHue Project (FKZ 01LE 1910A) and the CLEARING HOUSE (Collaborative Learning in Research, Information-sharing and Governance on How Urban forest-based solutions support Sino-European urban futures) Horizon 2020 project (No. 821242). Dagmar further contributed to this paper as part of the EU Horizon 2020 project CONNECTING Nature-COproductioN with NaturE for City Transitioning, Innovation and Governance (Project Number: 730222).
The authors declare that the research was conducted in the absence of any commercial or financial relationships that could be construed as a potential conflict of interest.
The Supplementary Material for this article can be found online at: https://www.frontiersin.org/articles/10.3389/fenvs.2021.657969/full#supplementary-material
Akbari, H., Levinson, R., and Berdahl, P. (2003). A Review of Methods for the Manufacture of Residential Roofing Materials. Berkeley, CA: Lawrence Berkeley National Laboratory.
Amaya-Espinel, J. D., Hostetler, M., Henríquez, C., and Bonacic, C. (2019). The Influence of Building Density on Neotropical Bird Communities Found in Small Urban parks. Landscape Urban Plann. 190, 103578. doi:10.1016/j.landurbplan.2019.05.009
Andersson, E., Haase, D., Scheuer, S., and Wellmann, T. (2020). Neighbourhood Character Affects the Spatial Extent and Magnitude of the Functional Footprint of Urban green Infrastructure. Landscape Ecol. 35 (7), 1605–1618. doi:10.1007/s10980-020-01039-z
Andersson, E., Langemeyer, J., Borgström, S., McPhearson, T., Haase, D., Kronenberg, J., et al. (2019). Enabling Green and Blue Infrastructure to Improve Contributions to Human Well-Being and Equity in Urban Systems. BioScience 69 (7), 566–574. doi:10.1093/biosci/biz058
Armato, F. (2017). Pocket Park: Product Urban Design. Des. J. 20 (Suppl. 1), S1869–S1878. doi:10.1080/14606925.2017.1352705
Artis, D. A., and Carnahan, W. H. (1982). Survey of Emissivity Variability in Thermography of Urban Areas. Remote Sensing Environ. 12 (4), 313–329. doi:10.1016/0034-4257(82)90043-8
Balai Kerishnan, P., Maruthaveeran, S., and Maulan, S. (2020). Investigating the Usability Pattern and Constraints of Pocket parks in Kuala Lumpur, Malaysia. Urban For. Urban Green. 50, 126647. doi:10.1016/j.ufug.2020.126647
Borland, J. (2019). Small parks, Big Designs: Reconstructed Tokyo's New green Spaces, 1923-1931. Urban Hist. 47 (1), 106–125. doi:10.1017/S0963926819000567
Buyantuyev, A., Wu, J., and Gries, C. (2010). Multiscale Analysis of the Urbanization Pattern of the Phoenix Metropolitan Landscape of USA: Time, Space and Thematic Resolution. Landscape Urban Plann. 94 (3-4), 206–217. doi:10.1016/j.landurbplan.2009.10.005
Cao, X., Onishi, A., Chen, J., and Imura, H. (2010). Quantifying the Cool Island Intensity of Urban parks Using ASTER and IKONOS Data. Landscape Urban Plann. 96 (4), 224–231. doi:10.1016/j.landurbplan.2010.03.008
Cârlan, I., Haase, D., Große-Stoltenberg, A., and Sandric, I. (2020). Mapping Heat and Traffic Stress of Urban Park Vegetation Based on Satellite Imagery - A Comparison of Bucharest, Romania and Leipzig, Germany. Urban Ecosyst. 23 (2), 363–377. doi:10.1007/s11252-019-00916-z
Chan, E. Y. Y., Goggins, W. B., Kim, J. J., and Griffiths, S. M. (2012). A Study of Intracity Variation of Temperature-Related Mortality and Socioeconomic Status Among the Chinese Population in Hong Kong. J. Epidemiol. Community Health 66 (4), 322–327. doi:10.1136/jech.2008.085167
Chander, G., Markham, B. L., and Helder, D. L. (2009). Summary of Current Radiometric Calibration Coefficients for Landsat MSS, TM, ETM+, and EO-1 ALI Sensors. Remote Sensing Environ. 113 (5), 893–903. doi:10.1016/j.rse.2009.01.007
Chang, C.-R., Li, M.-H., and Chang, S.-D. (2007). A Preliminary Study on the Local Cool-Island Intensity of Taipei City parks. Landscape Urban Plann. 80 (4), 386–395. doi:10.1016/j.landurbplan.2006.09.005
Chen, M., Geng, F., Ma, L., Zhou, W., Shi, H., and Ma, J. (2013). Analysis on the Heat Wave Events in Shanghai in Recent 138 Years. Plateau Meteorology 32 (2), 2597–2607. doi:10.7522/j.issn.1000-0534.2012.00058
Cheng, X., Wei, B., Chen, G., Li, J., and Song, C. (2014). Influence of Park Size and its Surrounding Urban Landscape Patterns on the Park Cooling Effect. J. Urban Plann. Develop. 141 (3), A4014002. doi:10.1061/(ASCE)UP.1943-5444.0000256
Cohen, D. A., Marsh, T., Williamson, S., Han, B., Derose, K. P., Golinelli, D., et al. (2014). The Potential for Pocket Parks to Increase Physical Activity. Am. J. Health Promot. 28 (3_Suppl. l), S19–S26. doi:10.4278/ajhp.130430-QUAN-213
Currie, M. A. (2017). A Design Framework for Small parks in Ultra-urban, Metropolitan, Suburban and Small Town Settings. J. Urban Des. 22 (1), 76–95. doi:10.1080/13574809.2016.1234334
Danford, R. S., Strohbach, M. W., Warren, P. S., and Ryan, R. L. (2018). Active Greening or Rewilding the City: How Does the Intention behind Small Pockets of Urban green Affect Use? Urban For. Urban Green. 29, 377–383. doi:10.1016/j.ufug.2017.11.014
Dong, J., Peng, J., He, X., Corcoran, J., Qiu, S., and Wang, X. (2020). Heatwave-induced Human Health Risk Assessment in Megacities Based on Heat Stress-Social Vulnerability-Human Exposure Framework. Landscape Urban Plann. 203, 103907. doi:10.1016/j.landurbplan.2020.103907
Eggermont, H., Balian, E., Azevedo, J. M. N., Beumer, V., Brodin, T., Claudet, J., et al. (2015). Nature-based Solutions: New Influence for Environmental Management and Research in Europe. GAIA - Ecol. Perspect. Sci. Soc. 24 (4), 243–248. doi:10.14512/gaia.24.4.9
Erell, E., Pearlmutter, D., and Williamson, T. (2011). Urban Microclimate: Designing the Spaces between Buildings. London: Routledge.
Erell, E. (2017). “Urban Greening and Microclimate Modification,” in Greening Cities. Editors P. Y. Tan, and C. Y. Jim (Singapore: Springer), 73–93. doi:10.1007/978-981-10-4113-6_4
Feng, X., and Li, P. (2019). A Tree Species Mapping Method from UAV Images over Urban Area Using Similarity in Tree-Crown Object Histograms. Remote Sensing 11 (17), 1982. doi:10.3390/rs11171982
Ferrari, B., Quatrini, V., Barbati, A., Corona, P., Masini, E., and Russo, D. (2019). Conservation and Enhancement of the green Infrastructure as a Nature-Based Solution for Rome's Sustainable Development. Urban Ecosyst. 22 (5), 865–878. doi:10.1007/s11252-019-00868-4
Feyisa, G. L., Dons, K., and Meilby, H. (2014). Efficiency of parks in Mitigating Urban Heat Island Effect: An Example from Addis Ababa. Landscape Urban Plann. 123, 87–95. doi:10.1016/j.landurbplan.2013.12.008
Gabriel, K. M. A., and Endlicher, W. R. (2011). Urban and Rural Mortality Rates during Heat Waves in Berlin and Brandenburg, Germany. Environ. Pollut. 159 (8), 2044–2050. doi:10.1016/j.envpol.2011.01.016
Gibson, H., and Canfield, J. (2016). Pocket parks as Community Building Blocks: A Focus on Stapleton, CO. Community Develop. 47 (5), 732–745. doi:10.1080/15575330.2016.1220965
Grimm, N. B., Faeth, S. H., Golubiewski, N. E., Redman, C. L., Wu, J., Bai, X., et al. (2008). Global Change and the Ecology of Cities. Science 319 (5864), 756–760. doi:10.1126/science.1150195
Haase, D. (2021). “Integrating Ecosystem Services, Green Infrastructure and Nature-Based Solutions-New Perspectives in Sustainable Urban Land Management,” in Sustainable Land Management in a European Context: A Co-design Approach. Editors T. Weith, T. Barkmann, N. Gaasch, S. Rogga, C. Strauß, and J. Zscheischler (Cham: Springer International Publishing), 305–318. doi:10.1007/978-3-030-50841-8_16
Harlan, S. L., Brazel, A. J., Prashad, L., Stefanov, W. L., and Larsen, L. (2006). Neighborhood Microclimates and Vulnerability to Heat Stress. Soc. Sci. Med. 63 (11), 2847–2863. doi:10.1016/j.socscimed.2006.07.030
Harlan, S. L., and Ruddell, D. M. (2011). Climate Change and Health in Cities: Impacts of Heat and Air Pollution and Potential Co-benefits from Mitigation and Adaptation. Curr. Opin. Environ. Sustainability 3 (3), 126–134. doi:10.1016/j.cosust.2011.01.001
Hu, Y. (2018). “A Novel Planning of Vest-Pocket Park in Historic Urban Area in Metropolis: A Case Study of Beijing,” in Green Intelligent Transportation Systems. Editors W. Wang, K. Bengler, and X. Jiang (Singapore: Springer Singapore), 1035–1053.
Ikin, K., Beaty, R. M., Lindenmayer, D. B., Knight, E., Fischer, J., and Manning, A. D. (2013). Pocket parks in a Compact City: How Do Birds Respond to Increasing Residential Density?. Landscape Ecol. 28 (1), 45–56. doi:10.1007/s10980-012-9811-7
Jaganmohan, M., Knapp, S., Buchmann, C. M., and Schwarz, N. (2016). The Bigger, the Better? the Influence of Urban green Space Design on Cooling Effects for Residential Areas. J. Environ. Qual. 45 (1), 134–145. doi:10.2134/jeq2015.01.0062
Jasmani, Z., Ravn, H. P., and van den Bosch, C. C. K. (2017). The Influence of Small Urban parks Characteristics on Bird Diversity: A Case Study of Petaling Jaya, Malaysia. Urban Ecosyst. 20 (1), 227–243. doi:10.1007/s11252-016-0584-7
Jiao, M., Zhou, W., Zheng, Z., Wang, J., and Qian, Y. (2017). Patch Size of Trees Affects its Cooling Effectiveness: A Perspective from Shading and Transpiration Processes. Agric. For. Meteorology 247, 293–299. doi:10.1016/j.agrformet.2017.08.013
Kong, F., Yin, H., James, P., Hutyra, L. R., and He, H. S. (2014a). Effects of Spatial Pattern of Greenspace on Urban Cooling in a Large Metropolitan Area of Eastern China. Landscape Urban Plann. 128, 35–47. doi:10.1016/j.landurbplan.2014.04.018
Kong, F., Yin, H., Wang, C., Cavan, G., and James, P. (2014b). A Satellite Image-Based Analysis of Factors Contributing to the green-space Cool Island Intensity on a City Scale. Urban For. Urban Green. 13 (4), 846–853. doi:10.1016/j.ufug.2014.09.009
Labuz, R. (2019). Pocket Park - A New Type of Green Public Space in Kraków (Poland). IOP Conf. Ser. Mater. Sci. Eng. 471, 112018. doi:10.1088/1757-899x/471/11/112018
Lau, S. S., Lin, P., and Qin, H. (2012). A Preliminary Study on Environmental Performances of Pocket parks in High-Rise and High-Density Urban Context in Hong Kong. Int. J. Low-carbon Tech. 7 (3), 215–225. doi:10.1093/ijlct/cts033
Li, H., and Wu, J. (2004). Use and Misuse of Landscape Indices. Landscape Ecol. 19 (4), 389–399. doi:10.1023/B:LAND.0000030441.15628.d6
Li, J., Song, C., Cao, L., Zhu, F., Meng, X., and Wu, J. (2011). Impacts of Landscape Structure on Surface Urban Heat Islands: A Case Study of Shanghai, China. Remote Sensing Environ. 115 (12), 3249–3263. doi:10.1016/j.rse.2011.07.008
Lin, B.-S., and Lin, Y.-J. (2010). Cooling Effect of Shade Trees with Different Characteristics in a Subtropical Urban Park. HortScience: a Publ. Am. Soc. Hortic. Sci. 45 (1), 83–86. doi:10.1590/S0102-0536201000010002410.21273/hortsci.45.1.83
Lin, P., Lau, S. S. Y., Qin, H., and Gou, Z. (2017). Effects of Urban Planning Indicators on Urban Heat Island: a Case Study of Pocket parks in High-Rise High-Density Environment. Landscape Urban Plann. 168, 48–60. doi:10.1016/j.landurbplan.2017.09.024
Lin, W., Chen, Q., Jiang, M., Zhang, X., Liu, Z., Tao, J., et al. (2019). The Effect of green Space Behaviour and Per Capita Area in Small Urban green Spaces on Psychophysiological Responses. Landscape Urban Plann. 192, 103637. doi:10.1016/j.landurbplan.2019.103637
Lin, W., Yu, T., Chang, X., Wu, W., and Zhang, Y. (2015). Calculating Cooling Extents of green parks Using Remote Sensing: Method and Test. Landscape Urban Plann. 134, 66–75. doi:10.1016/j.landurbplan.2014.10.012
Maimaitiyiming, M., Ghulam, A., Tiyip, T., Pla, F., Latorre-Carmona, P., Halik, Ü., et al. (2014). Effects of green Space Spatial Pattern on Land Surface Temperature: Implications for Sustainable Urban Planning and Climate Change Adaptation. ISPRS J. Photogrammetry Remote Sensing 89, 59–66. doi:10.1016/j.isprsjprs.2013.12.010
Maimaitiyiming, M., Sagan, V., Sidike, P., Maimaitijiang, M., Miller, A. J., and Kwasniewski, M. (2020). Leveraging Very-High Spatial Resolution Hyperspectral and Thermal UAV Imageries for Characterizing Diurnal Indicators of Grapevine Physiology. Remote Sensing 12 (19), 3216. doi:10.3390/rs12193216
Masoudi, M., and Tan, P. Y. (2019). Multi-year Comparison of the Effects of Spatial Pattern of Urban green Spaces on Urban Land Surface Temperature. Landscape Urban Plann. 184, 44–58. doi:10.1016/j.landurbplan.2018.10.023
McGarigal, K., Cushman, S. A., and Ene, E. (2012). FRAGSTATS V4: Spatial Pattern Analysis Program for Categorical and Continuous Maps. Amherst: University of Massachusetts.
Morini, E., Castellani, B., De Ciantis, S., Anderini, E., and Rossi, F. (2018). Planning for Cooler Urban Canyons: Comparative Analysis of the Influence of Façades Reflective Properties on Urban canyon thermal Behavior. Solar Energy 162, 14–27. doi:10.1016/j.solener.2017.12.064
Motazedian, A., Coutts, A. M., and Tapper, N. J. (2020). The Microclimatic Interaction of a Small Urban Park in central Melbourne with its Surrounding Urban Environment during Heat Events. Urban For. Urban Green. 52, 126688. doi:10.1016/j.ufug.2020.126688
Nordh, H., and Østby, K. (2013). Pocket parks for People - A Study of Park Design and Use. Urban For. Urban Green. 12 (1), 12–17. doi:10.1016/j.ufug.2012.11.003
Norton, B. A., Coutts, A. M., Livesley, S. J., Harris, R. J., Hunter, A. M., and Williams, N. S. G. (2015). Planning for Cooler Cities: A Framework to Prioritise green Infrastructure to Mitigate High Temperatures in Urban Landscapes. Landscape Urban Plann. 134, 127–138. doi:10.1016/j.landurbplan.2014.10.018
Oke, T. (1982). The Energetic Basic of the Urban Heat Island. Q. J. R. Meteorol. Soc. 108 (455), 1–24. doi:10.1002/qj.4971084550210.1256/smsqj.45501
Oliveira, S., Andrade, H., and Vaz, T. (2011). The Cooling Effect of green Spaces as a Contribution to the Mitigation of Urban Heat: A Case Study in Lisbon. Building Environ. 46 (11), 2186–2194. doi:10.1016/j.buildenv.2011.04.034
Park, J., Kim, J.-H., Lee, D. K., Park, C. Y., and Jeong, S. G. (2017). The Influence of Small green Space Type and Structure at the Street Level on Urban Heat Island Mitigation. Urban For. Urban Green. 21, 203–212. doi:10.1016/j.ufug.2016.12.005
Pauleit, S., Andersson, E., Anton, B., Buijs, A., Haase, D., Hansen, R., et al. (2019). Urban green Infrastructure - Connecting People and Nature for Sustainable Cities. Urban For. Urban Green. 40, 1–3. doi:10.1016/j.ufug.2019.04.007
Peng, J., Dan, Y., Qiao, R., Liu, Y., Dong, J., and Wu, J. (2021). How to Quantify the Cooling Effect of Urban parks? Linking Maximum and Accumulation Perspectives. Remote Sensing Environ. 252, 112135. doi:10.1016/j.rse.2020.112135
Peschardt, K. K., Schipperijn, J., and Stigsdotter, U. K. (2012). Use of Small Public Urban Green Spaces (SPUGS). Urban For. Urban Green. 11 (3), 235–244. doi:10.1016/j.ufug.2012.04.002
Peschardt, K. K., and Stigsdotter, U. K. (2013). Associations between Park Characteristics and Perceived Restorativeness of Small Public Urban green Spaces. Landscape Urban Plann. 112, 26–39. doi:10.1016/j.landurbplan.2012.12.013
Peschardt, K. K., Stigsdotter, U. K., and Schipperrijn, J. (2016). Identifying Features of Pocket Parks that May Be Related to Health Promoting Use. Landscape Res. 41 (1), 79–94. doi:10.1080/01426397.2014.894006
Rotem-Mindali, O., Michael, Y., Helman, D., and Lensky, I. M. (2015). The Role of Local Land-Use on the Urban Heat Island Effect of Tel Aviv as Assessed from Satellite Remote Sensing. Appl. Geogr. 56, 145–153. doi:10.1016/j.apgeog.2014.11.023
Santamouris, M. (2014). On the Energy Impact of Urban Heat Island and Global Warming on Buildings. Energy and Buildings 82, 100–113. doi:10.1016/j.enbuild.2014.07.022
Schiefer, F., Kattenborn, T., Frick, A., Frey, J., Schall, P., Koch, B., et al. (2020). Mapping forest Tree Species in High Resolution UAV-Based RGB-Imagery by Means of Convolutional Neural Networks. ISPRS J. Photogrammetry Remote Sensing 170, 205–215. doi:10.1016/j.isprsjprs.2020.10.015
Shah, A., Garg, A., and Mishra, V. (2021). Quantifying the Local Cooling Effects of Urban green Spaces: Evidence from Bengaluru, India. Landscape Urban Plann. 209, 104043. doi:10.1016/j.landurbplan.2021.104043
Sun, R., Chen, A., Chen, L., and Lü, Y. (2012). Cooling Effects of Wetlands in an Urban Region: The Case of Beijing. Ecol. Indicators 20 (0), 57–64. doi:10.1016/j.ecolind.2012.02.006
Sun, R., and Chen, L. (2017). Effects of green Space Dynamics on Urban Heat Islands: Mitigation and Diversification. Ecosystem Serv. 23, 38–46. doi:10.1016/j.ecoser.2016.11.011
Tan, J., Zheng, Y., Tang, X., Guo, C., Li, L., Song, G., et al. (2010). The Urban Heat Island and its Impact on Heat Waves and Human Health in Shanghai. Int. J. Biometeorol. 54 (1), 75–84. doi:10.1007/s00484-009-0256-x
Upmanis, H., Eliasson, I., and Lindqvist, S. (1998). The Influence of green Areas on Nocturnal Temperatures in a High Latitude City (Göteborg, Sweden). Int. J. Climatol. 18 (6), 681–700. doi:10.1002/(sici)1097-0088(199805)18:6<681::aid-joc289>3.0.co;2-l
Wang, J., Zhou, W., Jiao, M., Zheng, Z., Ren, T., and Zhang, Q. (2020). Significant Effects of Ecological Context on Urban Trees' Cooling Efficiency. ISPRS J. Photogrammetry Remote Sensing 159, 78–89. doi:10.1016/j.isprsjprs.2019.11.001
Weber, N., Haase, D., and Franck, U. (2014a). Assessing Modelled Outdoor Traffic-Induced Noise and Air Pollution Around Urban Structures Using the Concept of Landscape Metrics. Landscape Urban Plann. 125, 105–116. doi:10.1016/j.landurbplan.2014.02.018
Weber, N., Haase, D., and Franck, U. (2014b). Traffic-induced Noise Levels in Residential Urban Structures Using Landscape Metrics as Indicators. Ecol. Indicators 45, 611–621. doi:10.1016/j.ecolind.2014.05.004
Weber, N., Haase, D., and Franck, U. (2014c). Zooming into Temperature Conditions in the City of Leipzig: How Do Urban Built and green Structures Influence Earth Surface Temperatures in the City?. Sci. Total Environ. 496, 289–298. doi:10.1016/j.scitotenv.2014.06.144
Wei, J., and Lu, S. (2018). “Research on Landscape Space Perception of Pocket Park,” in 2018 International Conference on Engineering Simulation and Intelligent Control (ESAIC), Changsha, China, August 10–11, 2018 (IEEE), 290–294.
Weng, Q., Lu, D., and Schubring, J. (2004). Estimation of Land Surface Temperature-Vegetation Abundance Relationship for Urban Heat Island Studies. Remote Sensing Environ. 89 (4), 467–483. doi:10.1016/j.rse.2003.11.005
Weng, Q. (2012). Remote Sensing of Impervious Surfaces in the Urban Areas: Requirements, Methods, and Trends. Remote Sensing Environ. 117, 34–49. doi:10.1016/j.rse.2011.02.030
Weng, Q. (2009). Thermal Infrared Remote Sensing for Urban Climate and Environmental Studies: Methods, Applications, and Trends. ISPRS J. Photogrammetry Remote Sensing 64 (4), 335–344. doi:10.1016/j.isprsjprs.2009.03.007
Wu, C., Li, J., Wang, C., Song, C., Chen, Y., Finka, M., et al. (2019). Understanding the Relationship between Urban Blue Infrastructure and Land Surface Temperature. Sci. Total Environ. 694, 133742. doi:10.1016/j.scitotenv.2019.133742
Wu, J., Shen, W., Sun, W., and Tueller, P. T. (2002). Empirical Patterns of the Effects of Changing Scale on Landscape Metrics. Landscape Ecol. 17 (8), 761–782. doi:10.1023/A:1022995922992
Wu, Z., Kong, F., Wang, Y., Sun, R., and Chen, L. (2016). The Impact of Greenspace on Thermal Comfort in a Residential Quarter of Beijing, China. Int. J. Environ. Res. Public Health 13 (12), 1217. doi:10.3390/ijerph13121217
Xiao, X. D., Dong, L., Yan, H., Yang, N., and Xiong, Y. (2018). The Influence of the Spatial Characteristics of Urban green Space on the Urban Heat Island Effect in Suzhou Industrial Park. Sustain. Cities Soc. 40, 428–439. doi:10.1016/j.scs.2018.04.002
Yan, J., Zhou, W., and Jenerette, G. D. (2019). Testing an Energy Exchange and Microclimate Cooling Hypothesis for the Effect of Vegetation Configuration on Urban Heat. Agric. For. Meteorology 279, 107666. doi:10.1016/j.agrformet.2019.107666
Yu, X., Guo, X., and Wu, Z. (2014). Land Surface Temperature Retrieval from Landsat 8 TIRS-Comparison between Radiative Transfer Equation-Based Method, Split Window Algorithm and Single Channel Method. Remote Sensing 6 (10), 9829–9852. doi:10.3390/rs6109829
Yu, Z., Guo, X., Jørgensen, G., and Vejre, H. (2017). How Can Urban green Spaces Be Planned for Climate Adaptation in Subtropical Cities?. Ecol. Indicators 82, 152–162. doi:10.1016/j.ecolind.2017.07.002
Yu, Z., Guo, X., Zeng, Y., Koga, M., and Vejre, H. (2018a). Variations in Land Surface Temperature and Cooling Efficiency of green Space in Rapid Urbanization: The Case of Fuzhou City, China. Urban For. Urban Green. 29, 113–121. doi:10.1016/j.ufug.2017.11.008
Yu, Z., Xu, S., Zhang, Y., Jørgensen, G., and Vejre, H. (2018b). Strong Contributions of Local Background Climate to the Cooling Effect of Urban green Vegetation. Sci. Rep. 8 (1), 6798. doi:10.1038/s41598-018-25296-w
Zarco-Tejada, P. J., González-Dugo, V., and Berni, J. A. J. (2012). Fluorescence, Temperature and Narrow-Band Indices Acquired from a UAV Platform for Water Stress Detection Using a Micro-hyperspectral Imager and a thermal Camera. Remote Sensing Environ. 117, 322–337. doi:10.1016/j.rse.2011.10.007
Zhang, Y., Murray, A. T., and Turner, B. L. (2017). Optimizing green Space Locations to Reduce Daytime and Nighttime Urban Heat Island Effects in Phoenix, Arizona. Landscape Urban Plann. 165, 162–171. doi:10.1016/j.landurbplan.2017.04.009
Zhao, L., Lee, X., Smith, R. B., and Oleson, K. (2014). Strong Contributions of Local Background Climate to Urban Heat Islands. Nature 511 (7508), 216–219. doi:10.1038/nature13462
Zhao, T. F., and Fong, K. F. (2017). Characterization of Different Heat Mitigation Strategies in Landscape to Fight against Heat Island and Improve thermal comfort in Hot-Humid Climate (Part I): Measurement and Modelling. Sustain. cities Soc. 32, 523–531. doi:10.1016/j.scs.2017.05.006
Zhen, M., Hong, F., and Zhou, D. (2019). The Relationship between Spatial Arrangement and Environmental Temperature of Residential Areas in Xi'an. Indoor Built Environ. 28 (9), 1288–1300. doi:10.1177/1420326x19860872
Zhou, W., Huang, G., and Cadenasso, M. L. (2011). Does Spatial Configuration Matter? Understanding the Effects of Land Cover Pattern on Land Surface Temperature in Urban Landscapes. Landscape Urban Plann. 102 (1), 54–63. doi:10.1016/j.landurbplan.2011.03.009
Zhou, W., Wang, J., and Cadenasso, M. L. (2017). Effects of the Spatial Configuration of Trees on Urban Heat Mitigation: A Comparative Study. Remote Sensing Environ. 195, 1–12. doi:10.1016/j.rse.2017.03.043
Keywords: pocket green space, land surface temperature, cooling effect, landscape pattern, landscape features
Citation: Wu C, Li J, Wang C, Song C, Haase D, Breuste J and Finka M (2021) Estimating the Cooling Effect of Pocket Green Space in High Density Urban Areas in Shanghai, China. Front. Environ. Sci. 9:657969. doi: 10.3389/fenvs.2021.657969
Received: 24 January 2021; Accepted: 13 May 2021;
Published: 28 May 2021.
Edited by:
Ioan Cristian Ioja, University of Bucharest, RomaniaReviewed by:
Weiqi Zhou, Research Center for Eco-Environmental Sciences (CAS), ChinaCopyright © 2021 Wu, Li, Wang, Song, Haase, Breuste and Finka. This is an open-access article distributed under the terms of the Creative Commons Attribution License (CC BY). The use, distribution or reproduction in other forums is permitted, provided the original author(s) and the copyright owner(s) are credited and that the original publication in this journal is cited, in accordance with accepted academic practice. No use, distribution or reproduction is permitted which does not comply with these terms.
*Correspondence: Junxiang Li, anVueGlhbmdsaUBzanR1LmVkdS5jbg==
Disclaimer: All claims expressed in this article are solely those of the authors and do not necessarily represent those of their affiliated organizations, or those of the publisher, the editors and the reviewers. Any product that may be evaluated in this article or claim that may be made by its manufacturer is not guaranteed or endorsed by the publisher.
Research integrity at Frontiers
Learn more about the work of our research integrity team to safeguard the quality of each article we publish.