- 1UCD College of Business, University College Dublin, Albany, Ireland
- 2Business Administration Department, Faculty of Management Sciences, ILMA University, Karach, Pakistan
- 3The Finance and Accounting Department, GEF2A-Lab, Higher Institute of Management of Tunis, University of Tunis, Tunis, Tunisia
- 4Finance Department, College of Business, King Abdulaziz University, Rabigh, Saudi Arabia
- 5Finance, Control and Law, Montpellier Business School, France
- 6Department of Accounting, Analysis and Audit, South Ural State University, Chelyabinsk, Russia Federation
COVID-19 has morphed from a health crisis to an economic crisis that affected the global economy through several channels. This paper aims to study the impact of COVID-19 on the time-frequency connectedness between Green Bonds and other financial assets. Our sample includes the global stock market, bond market, oil, USD index, and two popular hedging alternatives, namely Gold and Bitcoin, from May 2013 to August 2020. First, we apply the methodologies of Diebold and Yilmaz (International Journal of Forecasting, 2012, 28(1), 57–66) and Baruník and Křehlík (Journal of Financial Econometrics, 2018, 16(2), 271–296). Then, we estimate hedge ratios and hedge effectiveness of green bonds for other financial assets. Green bonds are found to have a great weight in the overall network, particularly strongly connected with the USD index and bond index. While the bi-directional relationship with USD persists during COVID, the connectedness with conventional bonds is also strengthened. Notably, we find a weak relationship between Green bonds and Bitcoin, both in the short and long run. As portfolio implications, Gold and USD have the highest hedge ratio, which is confirmed by the hedging effectiveness. In contrast, oil and stocks exhibit the lowest hedging effectiveness. Our findings imply that financial assets might have a heterogeneous relationship with green bonds. Furthermore, despite its infancy, it seems that the role of green bond during a crisis should not be ignored, as it can be a hedger for some assets, while a contagion amplifier during crisis times.
Introduction
COVID-19 has morphed from a health crisis to an economic crisis that affected the global economy through several channels. The unexpected coronavirus that started in Wuhan city in China in late 2019 spread globally in a matter of a few months. The World Health Organization (WHO) classified COVID-19 as a global pandemic on March 11 2020 (World Health Organization, 2020). The affected countries by COVID-19 imposed strict implications and policies to lock down the borders. This lockdown has impacted international trade (imports and exports), resulting in slower economic growth. Moreover, many studies confirmed that the COVID-19 outbreak influenced financial markets’ returns significantly and inversely, which affect the economy negatively (Al-Awadhi et al., 2020; Ashraf, 2020; Mazur et al., 2020). On the other side, Haldar and Sethi. (2020) found an insignificant correlation between COVID-19 and socio-economic factors like GDP per capita and the human development index for the countries: India, United States, Brazil, Argentina, France, Colombia, Russia, Israel, United Kingdom, and Peru. Another case by Dash et al. (2021) on BRICS economies (Brazil, Russia, India, China, and South Africa) shows that economic growth is insignificant with COVID-19. This result encourages the policymakers of BRICS economies to invest more in the health care sector. Several reasons have been highlighted in recent literature that could justify the drop in financial markets’ returns during the period of COVID-19, such as the appearance of uncertainty and fear (Lyócsa and Molnár, 2020), investor sentiment (Narayan et al., 2020), and systematic risks (Zhang et al., 2020), which influenced economies negatively. Moreover, COVID-19 can also increase the risk of volatility of stock markets, which decrease stocks return significantly and negatively (Haldar and Sethi, 2021). Therefore, focusing on the integration between financial markets and national and global economies is important (Nasir and Du, 2018). COVID-19 not only impacted stock markets negatively but affected the oil market significantly. Global oil prices have been experiencing a significant decline since the start of COVID-19 due to many reasons, such as a reduction in oil demand, specifically, the cut in airline sectors and the stoppage of global and national flights. This collapse in demand for transportation fuel (Salisu et al., 2020; Sharif et al., 2020). Another reason that international import (demand) of oil faced a sharp slump resulted in lower oil prices (Dutta et al., 2020). Throughout COVID-19, gold and Bitcoin represent safe-haven (hedgers), which encourages investors to trade more in gold and Bitcoin rather than invest in financial markets (Dutta et al., 2020). Recently, the global population is expanding rapidly and increasing the consumption of energy with more CO2 emissions. This increase in consumption causes a higher threat to the environment (Dogan and Seker, 2016), human health (Anser et al., 2020), and economies (Rasool et al., 2019). Therefore, governments and corporates encourage the use of clean energy. An example to enhance clean energy is to finance and invest in green projects such as trading green bonds, which can effectively mitigate the risks of CO2 emissions. Green bonds are similar to conventional corporate bonds, but green bonds focus only on projects that can be friendly to the environment (Nguyen et al., 2020; Reboredo and Ugolini, 2020; Saeed et al., 2020). Previous studies argue that more green bond trading can support low-carbon projects, which reduces environmental degradation significantly (Monasterolo and Raberto, 2018). This results in having a cleaner environment and lower negative effects of climate change (Gevorkyan et al., 2016; Flaherty et al., 2017; Orlov et al., 2018). Globally, countries have been taking action against climate change, global warming, and mitigating greenhouse emissions, (e.g. CO2) through signing many agreements like Kyoto Protocol (1997) and Paris Agreement (2015). Our study contributes to the United Nations' Sustainable Development Goals particularly, Goal 7: Affordable and Clean Energy, Goal 11: Sustainable Cities and Communities, and Goal 13: Climate Action (United Nations, 2016). According to Goal 7: Affordable and Clean Energy, this study encourages using clean energy and investing in green bonds. Regarding Goal 11: Sustainable Cities and Communities, higher usage of clean energy would help to achieve sustainability effectively, that can enhance economy, society and environment. Focusing on Goal 13: Climate Action, this study followed the call, policies and implication of Kyoto Protocol (1997) and Paris Agreement (2015) through the mitigation of the global pollution (CO2 emissions).
International financial investors would trade using two main strategies through hedging and diversification. The most important markets that drive the global economy are the global stock market, bond market, oil market, USD index, Gold, and Bitcoin, which is the focus of our study. Regarding financial (stocks and bonds) markets, they are playing important roles to finance corporates and governments. This finance significantly supports economic growth, but again, maybe this finance would lead to more pollution as higher finance would increase production. As a result, green finance aims to promote green energy and the environment. According to oil, it is important due to oil is a source of energy to supply production (industries). Moreover, transportations depend highly on oil products such as fuel. However, oil is still a non-renewable source for energy, and international organizations, e.g., United Nations, are encouraging using renewable energy, which threats oil prices, but at least the world would have a cleaner environment. Focusing on USD index, the reason behind choosing USD to be examined is that USD is linked to main global commodities, e.g., oil, gold, and Bitcoin. Concentrating on hedging alternatives such as Gold and Bitcoin over periods of crisis, e.g., global financial crisis, gold found being a safe-haven asset (Baur and McDermott, 2010; Ciner et al., 2013; Reboredo, 2013). A recent study by Das et al., 2020 shows that Bitcoin is not a superior haven asset (hedging) than Gold and USD. Importantly, our study investigates whether gold and Bitcoin are safe-haven assets during Covid- 19 turmoil. To summarize, focusing on the above variables contributes to the field of the study. In our study, we employ a time-frequency analysis to find the connectedness between the study variables. The time-frequency analysis is important for portfolio managers and investors in financial markets. Specifically, this approach is significant for short-term (active investors/day traders) and long-term investors (passive investors). Additionally, disconnection in the short-term means an asset is a safer haven during the crisis, whereas disconnection in the long-term indicates diversification benefit. Investors have different time horizons’ preferences and frequencies, and some investors prefer to invest at short- (hours/days), medium- (weekly/monthly), and long-term (yearly) periods (Nguyen et al., 2020). Moreover, the time-frequency analysis measures spillovers and dependencies effectively and tracks the spillovers at all levels, based on the vector autoregressive model (VAR model) (Diebold and Yilmaz, 2012). The VAR computes the forecast error variance decompositions (FEVD) from a generalized vector autoregression. Moreover, this study also aims to find if the impact of COVID-19 on the connectedness between green bonds and other financial markets using the time-frequency domain with portfolio implications. Based on the discussions above, we can conclude that this study makes several contributions: 1) a limited number of studies in the literature examined the relation between green bonds and financial markets. 2) To our knowledge, there is no study in the literature focused on the effects of COVID-19 impact on the connectedness among green bonds and financial markets. However, the main objective of this study is to analyze the effects of COVID-19 on the connectedness between Green Bonds and some important financial assets (global stock market, bond market, oil, USD index, and two popular hedging alternatives, namely Gold and Bitcoin). The study is structured into four sections. Literature Review presents the literature review. The methodology and data are explained in Methodology and Data. The findings are reported and discussed in Empirical Findings and Conclusion concludes the paper.
Literature Review
There are limited studies in the literature review that tested the correlation between green bonds and other financial markets (Hammoudeh et al., 2020; Nguyen et al., 2020; Reboredo and Ugolini, 2020). Hammoudeh et al. (2020) examined the link between green bonds, financial assets, and the environment. The financial assets are United States conventional bonds and WilderHill clean energy (equity) index, while CO2 emissions represent the environment from July 30 2014 to February 10 2020. Statistically, the data analyzed in this study through the novel time-varying Granger causality test. The findings show a significant causality running from the United States 10 years Treasury bond index to green bonds from the end of 2016 to February 10 2020. Also, CO2 emission allowances price affected green bonds significantly (time-varying causality) from July 30 2014 to the end of 2015. Moreover, the time-varying causality showed a low effect from the clean energy index to green bonds in 2019. Reboredo and Ugolini. (2020) analyzed the price connectedness between the green bond and financial markets from October 2014 to December 2018. Identifying the direct and indirect financial shocks was through the model of structural vector autoregressive (VAR). This study’s empirical results suggest that the green bond market is associated with currency and fixed-income markets. On the other side, the green bond market has a weak link with energy, stock, and high-yield corporate bond markets. The VAR method has been employed in the literature review to examine the effects of credit risk on several international financial institutions (Yang and Zhou, 2013) and analyze volatility spillovers on some global financial markets (Yang and Zhou, 2017). Focusing on the correlation between environment and green bonds, Zerbib. (2019) estimates the differentiation of yield amongst green bond conventional bonds over July 2013 to December 2016. The yield of green bonds was found to be lower than the conventional bond. The results also indicate that investors’ preferences considering pro-environment are low, which means that the investors prefer profits rather than supporting green bond markets (cleaner environment). Another study by Glomsrød and Wei. (2018) shows the influence of green bonds in mitigating global non-renewable energy consumption, (e.g. coal), which strongly recommended reducing CO2 emissions. Tang and Zhang. (2020) approve that when green bond issuance appears (announces), the stock market indices respond positively; more liquidity and trust can be shown on the financial markets after the issuance of green bonds. Flammer (2018) also points out that green bonds’ issuance has influenced the firm’s financial performance and environment that issued green bonds. Broadstock and Cheng. (2019) argue a significant link between green and black bond markets. Moreover, the financial market's volatility, economic policy uncertainty, daily economic activity, and oil prices significantly affected green bonds. Some studies confirmed a significant link between oil prices and green energy stocks (Sadorsky, 2012; Wen et al., 2014), but other studies argued an insignificant correlation between oil and renewable energy corporate prices, (e.g. Reboredo et al., 2017). However, Reboredo and Ugolini (2020) identify the network connectedness of green bonds and asset classes in Europe and the United States. This study concluded that green, treasury and corporate bonds are strongly connected in the short- and long-run. In contrast, a weak connectedness approved between green bonds and high yield corporate bond, stock, and energy assets. Recently, Le et al. (2021) test the connectedness between green bonds, fintech, and cryptocurrencies utilizing daily basis data from November 2018 to June 2020. This study is very important as it covers the period of COVID-19. This study’s findings showcase a strong link between technology assets and common stock. Additionally, Bitcoin, MSCIW, MSCI US, and KFTX caused strong volatility shocks, whereas the USD index, gold, oil, and green bonds were found to be good hedgers. To summarize, we highlight the past literature on connectedness, comovement and spillovers between green bonds and other financial markets in Table 1.
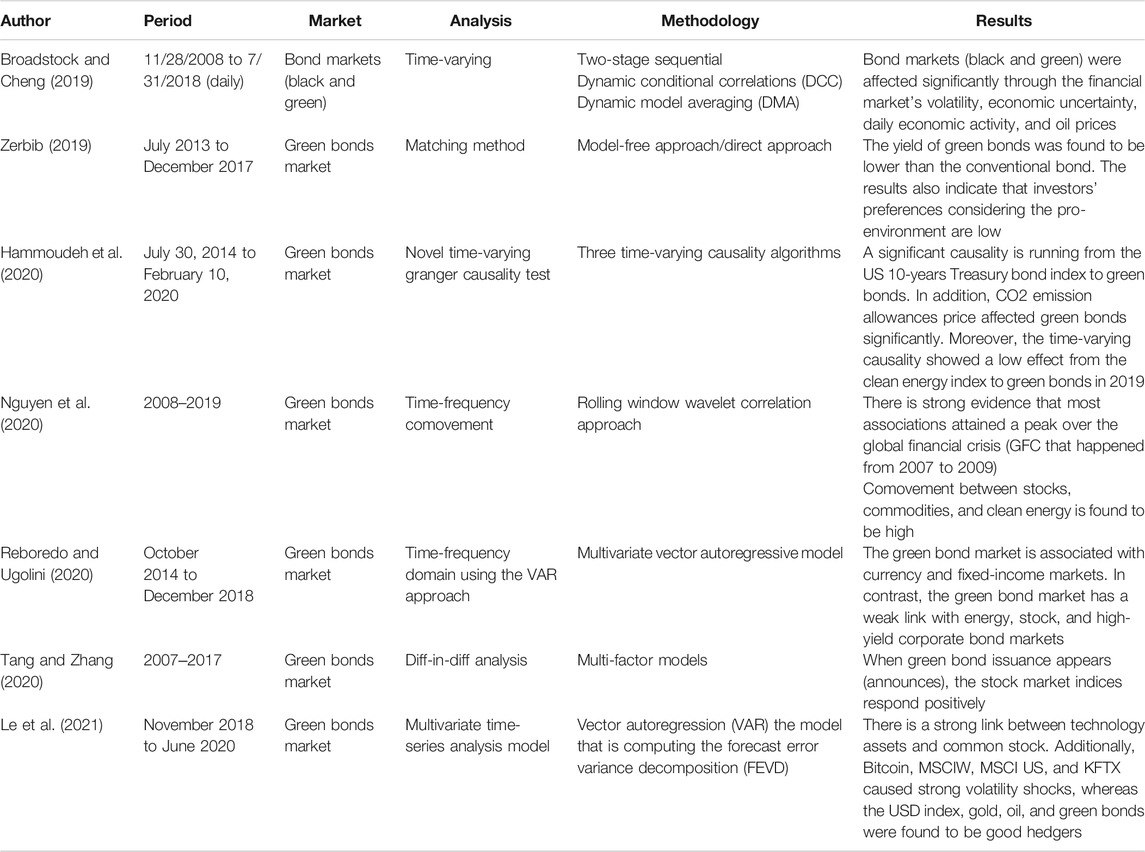
TABLE 1. Summary of studies in the literature review on connectedness between green bonds and other financial markets.
Methodology and Data
This paper aims to examine the impact of COVID on the connectedness of green bonds with other financial assets within a time-frequency framework. This methodology describes how connectedness evolves in both time and across different investment horizons, namely the short and long run. In line with literature that studies the impact of crises, we first estimate connectedness on a full sample that provides a full picture of the connectedness over the entire period of the sample. Then, we estimate connectedness on sub-samples, pre-COVID and post-COVID. The pre-COVID starts from the start of our sample (May 2013) to December 2019. The post-COVID starts at January 2020 to the end of the sample, as the pandemic is ongoing.
We present first the time-frequency methodologies, and then we explain the hedge ratio and hedging effectiveness.
GFEVD and Connectedness in the Time-Domain
In order to estimate the transmission of return between green bonds and other assets, we first apply the methodology of Diebold and Yilmaz. (2012) to describe how this transmission evolves in time. The spillover measure is based on the forecast error variance decomposition (FEVD) matrix centered on the generalized vector-autoregressive (VAR) model. The idea of this decomposition is that each forecast error variance of a particular asset process is constituted by its own contribution and a contribution from another asset process with which it correlates. An advantageous feature of the method is that it is invariant to the ordering of the variables. Consider an n-variate covariance stationary VAR(p) model,
where
where
We find the generalized approach of Koop et al. (1996) and Pesaran and Shin. (1998) a better approach for considering orthogonality, given that the Cholesky factor relies on the ordering of the variables.
The contribution of variable j to the H-step-ahead generalized variance of forecast error of variable i is denoted as
where the
The pairwise directional transmission,
The total directional transmission from others to i is defined by the ratio of the off-diagonal sum of rows to the sum of all the elements as:
Moreover, the total directional transmission to others from j is given by the ratio of the off-diagonal sums of columns to the sum of all the elements as:
Finally, the total connectedness is defined by the ratio of the sum of the from-others (to-others) elements of the variance decomposition matrix to the sum of all its elements:
GFEVD in the Frequency Domain
To explain the frequency connectedness’ dynamics over both frequencies short and long terms, we employ the spectrum description of variance decomposition. These decompositions are based on frequency responses to shocks instead of impulse responses to shocks. Consequently, the present theory's building block calls the frequency response feature,
Knowing the dynamics of frequency depends on the main power spectrum of quantity
Following Barunik and Krehlik. (2018), we explain the measurement of connectedness indicators at varying frequencies. Therefore, spectral quantities are estimated by the regular Fourier transform. Cross-spectral density of the interval
for
and
At a given frequency band, the decomposition of the impulse response function is calculated as
where,
Hedge Ratio and Hedge Effectiveness
The hedging ability of green bond (GB) for other financial assets (A) permits to investigate the amount of reduced risk when including GB along with another financial asset (A) among the six used in this study. The hedging ability is thus examined, in this study, through hedge effectiveness measures. Particularly, consider
where
Referring to Baillie and Myers. (1991), we define the optimal coefficient by minimizing the conditional variance of the hedged portfolio as:
The hedge ratio is estimated using the extracted covariance and conditional volatility series estimated from an asymmetric generalized DCC-GARCH model, as suggested by Kroner and Sultan (1993). The expression of hedging a long position in a financial asset A by a short position in the green bond index is as follows:
where
Data and Preliminary Analysis
We use daily spot prices indexes of a diversified Green bond index (GB) with six financial asset classes. We use the S&P green bond index (SPGRBND), United States dollar index (USDXY), MSCI world index (MSWRLD), Bond index (BOND) along with three popular hedging alternatives (Gold, “GOLD”; WTI crude oil, “OIL” and Bitcoin, “BTC”).
Several green bond indices are available, (e.g. the Barclays MSCI Green Bond Index, the S and P Dow Jones Green Bond Index, and the Bank of America Merrill Lynch Green Bond Index), but there is a high correlation among them (Reboredo and Ugolini, 2020; Nguyen et al., 2020; Saeed et al., 2020). In this paper, we use the S and P Dow Jones Green Bond Index. It is a market value-weighted index that comprises bonds issued in any country and any currency, provided they are labeled green by the Climate Bonds Initiative. The index is calculated in USD, and weights are updated every month. It includes treasury, government-related, corporate, and securitized issues (Reboredo, 2018). The MSCI world index is included as a measure of international financial performance and West Texas Intermediate oil and gold as “international flight to safety assets” (Akyildirim et al., 2020, Naeem et al., 2020a,b; Shahzad et al., 2019, Shahzad et al., 2020). Regarding Bitcoin, since the boom of cryptocurrencies in 2013, the market attractiveness for this new digital asset has increased. Several recent empirical studies have documented that it is negatively correlated to some major financial assets and would provide valuable diversification benefits (Naeem et al., 2020a,b, and Shahzad et al., 2019, Shahzad et al., 2020).
All data are extracted from Datastream except for Bitcoin from coinmarketcap.com. Log transformation returns are used for empirical analyses. All indices are in USD. In our study, we use daily data from May 2013 to August 2020, yielding 1908 observations. Table 2 reports summary statistics. All return series are leptokurtic and have negative skewness value. Jarque-Bera test statistic points to the non-normality of the return distribution. All return series are stationary, as confirmed by the Augmented Dickey-Fuller (ADF) test statistic. Unsurprisingly, Bitcoin has the highest average return and, at the same time, the highest variability. In contrast, the Green bond index reveals to be the most stable asset as it has the lowest standard deviation, which increases its potential as a diversifier.
We report the unconditional correlation in Figure 1. As our main aim in this paper is to study the impact of COVID on the connectedness of Green Bond with other major financial assets, we thus report our results first for the whole sample than for the sub-samples: pre- and post-COVID. December 31, 2019, was the first time China reported to the WHO that there were multiple respiratory infection cases. Therefore, we consider the data sample from January 1, 2020 to August 30, 2020 as the post -COVID-19 period, in line with many other studies (Zaremba et al., 2020; Bouri et al., 2021; Corbet et al., 2020; Han and Li, 2020).
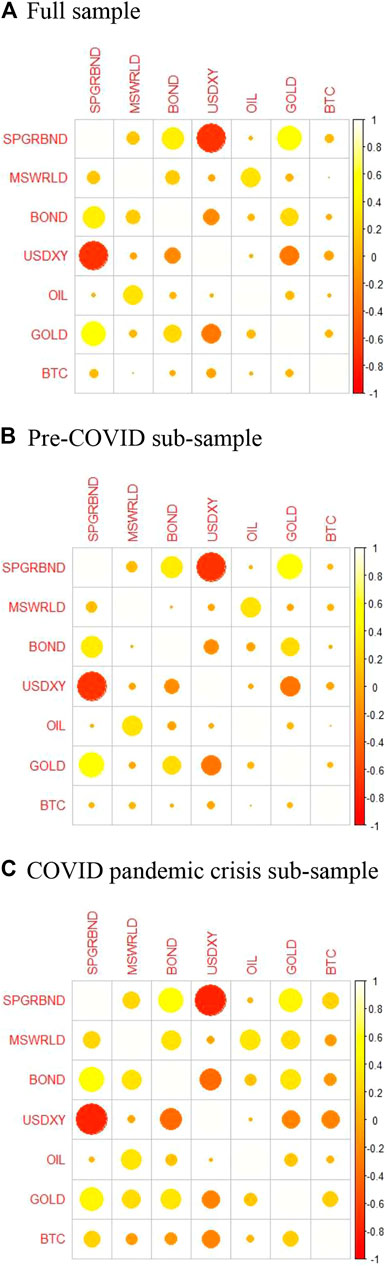
FIGURE 1. Correlation heatmaps. (A) Full sample. (B) Pre-COVID sub-sample. (C) COVID pandemic crisis sub-sample
A strong negative unconditional correlation is found between GB and the United States dollar index (USD), suggesting a substantial potential risk diversification benefit of GB in a portfolio with USD. This negative correlation is also maintained during the COVID times, suggesting that the Green Bond index is a safe-haven for the United States dollar during the crisis period. Nevertheless, we find that the Green bond index is weakly and negatively correlated to the MSCI index. Hence, the green bond index might be used as a diversifier in a stock portfolio.
Comparing the pre- and post-COVID time periods, a sheltered strong negative correlation is found between GB and USD, whereas a peculiar correlation with oil. However, a noticeable increase in correlation is found between GB and BTC, also a subdued increase with the MSCI index. As to gold, the correlation is even decreased during the pandemic time. We argue that the increase in correlation for some assets seems obvious as an expected response to market conditions. As to hedging alternatives (WTI, gold, and BTC), the Green Bond index's correlation pattern is not the same for all of them.
After having a preliminary idea of the impact of COVID-19 on the unconditional correlation between Green bonds and other financial assets, we analyze dynamic connectedness within a time-frequency framework and its portfolio implications.
Empirical Findings
Total Return Connectedness and COVID-19
First, we analyze the dynamic total connectedness between all the variables, based on the Diebold and Yilmaz (2012) [DY] framework. We utilize the rolling window approach with a window size of 262 days and a forecast horizon of H = 100 days, and the lag length of order one, as determined by the Schwarz Information Criterion (BIC).
The Figure 2 starts at a moderate level of 28% in 2013–2014, then a slight increase with weak peaks during 2016 and 2017. Nguyen et al. (2020) argued that the progressing massive development of green bonds, from 2013 onwards, is a plausible factor that drives the connectedness of green bonds with other markets. However, some downturns are also shown during 2018, but the deeper decrease in connectedness level (about 22%) occurred during late 2019. Noticeably, since the start of 2020, we see a sharp increase, reaching the highest level in the period of nearly (45%). Undoubtedly, this increase in connectedness is an expected response to COVID19. This alteration in connectedness level is in line with the contagion theory literature, which stipulates a peak in correlation among different assets as a response to extreme economic shocks (Benhmad, 2013; Narayan et al., 2014). More recent COVID empirical studies confirm that statement (Bouri et al., 2021; Adekoya and Olyide, 2020). It emerged primarily as a health crisis; COVID-19 has rapidly turned into an unprecedented economic crisis because of the higher uncertainty and ambiguity surrounding this crisis (Baker et al., 2020; OECD, 2020). Consequently, fears ‘investors led them to rebalance their portfolios among different financial assets for diversification and hedging against expected losses, causing hence the increased connectedness. More notably, this value is higher than 27.2% reported by Bouri et al. (2021) for a combination of the conventional bond index, USD index, gold, MSCI World, and crude oil during COVID times. We suggest that the inclusion of green bonds in the combination has made the difference implying thus the influential weight of green bonds in the overall financial system. As a new financial asset that is environment friendly, we argue that investors may perceive green bonds as a safer asset and hence shift their investments from traditional assets toward this market through the main currency, which is the US dollar. Following the peak during the onset of the pandemic, we also see a rapid decrease. Following that peak, the connectedness magnitude remains at a high level of around 35%, though, which is expected as uncertainty is continuing.
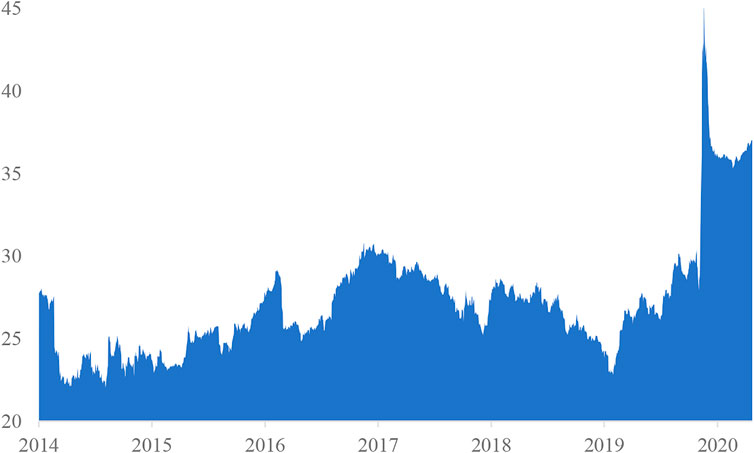
FIGURE 2. Rolling window using Diebold and Yilmaz. (2012).Note: Window length of 260 days
Next, we decompose the total return connectedness into short- (5 days) and long-term (6–262 days), applying the time-frequency method of Baruník and Křehlík. (2018). We plot the result in Figure 3. The red-shaded area indicates total connectedness at the higher frequency band, which corresponds to movements up to five trading days (one week). On the other hand, the green-shaded area reflects total connectedness at the lower frequency band, which refers to movements from 6 to 262 days. Throughout the sample period, the red-shaded areas outweigh the green-shaded areas, which implies that total connectedness among green bonds and other financial assets looks to be more driven by short-term shocks. We suggest that such a finding might reflect a speculative behavior of active traders who are more guided by short-term benefits and are shifting across financial assets, increasing their connectedness. In contrast, passive traders are more concerned with long-term investments and thus are focused on a specific asset. Our finding corroborates with Nguyen et al. (2020) on connectedness between green bonds, stocks, and commodities.
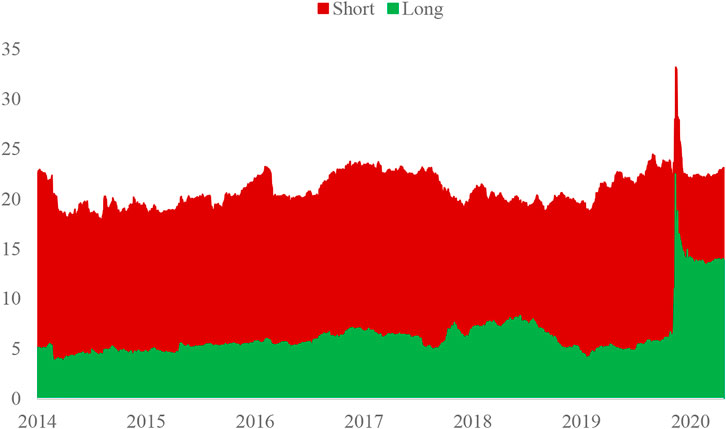
FIGURE 3. Rolling window using Barunik and Krehlik (2018).Note: Window length 260 days
Network Connectedness and COVID-19
While the dynamic approach can be informative and provide an overall picture of the network variables over time, it conceals some interesting information regarding the pairwise connectedness between the study variables. Results over the full sample for both DY and BK are plotted in Figures 4, 5, respectively.
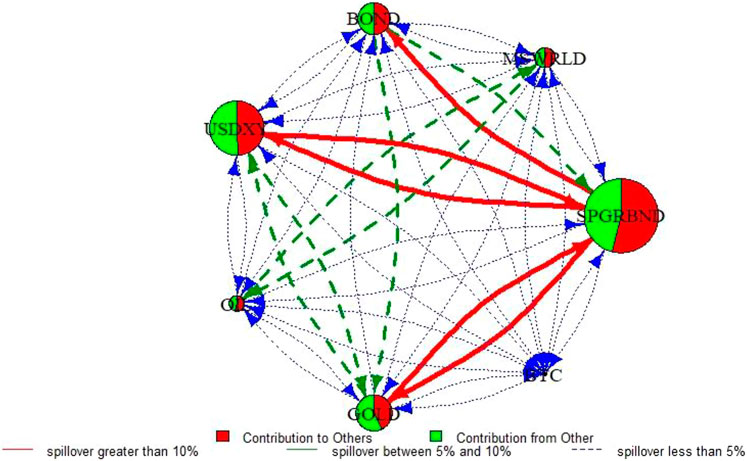
FIGURE 4. Network diagram of connectedness table using Diebold and Yilmaz (2012). Lags = 1 based on SIC. Forecast horizon of 100 days. Note: This network graph illustrates the degree of total connectedness in a system that consists of the S and P Green Bonds, MSCI World index, Bonds, United States Dollar index, Oil, Gold, and Bitcoin returns over the full sample period. Total connectedness is measured using the Diebold-Yilmaz framework. The size of the node shows the magnitude of the contribution of each variable to system connectedness, while the color indicates the origin of connectedness. In particular, the red color implies contribution from the variable under consideration to the other variables of the system, and the green color means contribution from the other variables to the variable under analysis. The color and shape of the arrows refer to the strength of connectedness. The red color and full line arrows represent strong spillovers while green and blue color arrows show medium and weak liquidity spillovers.
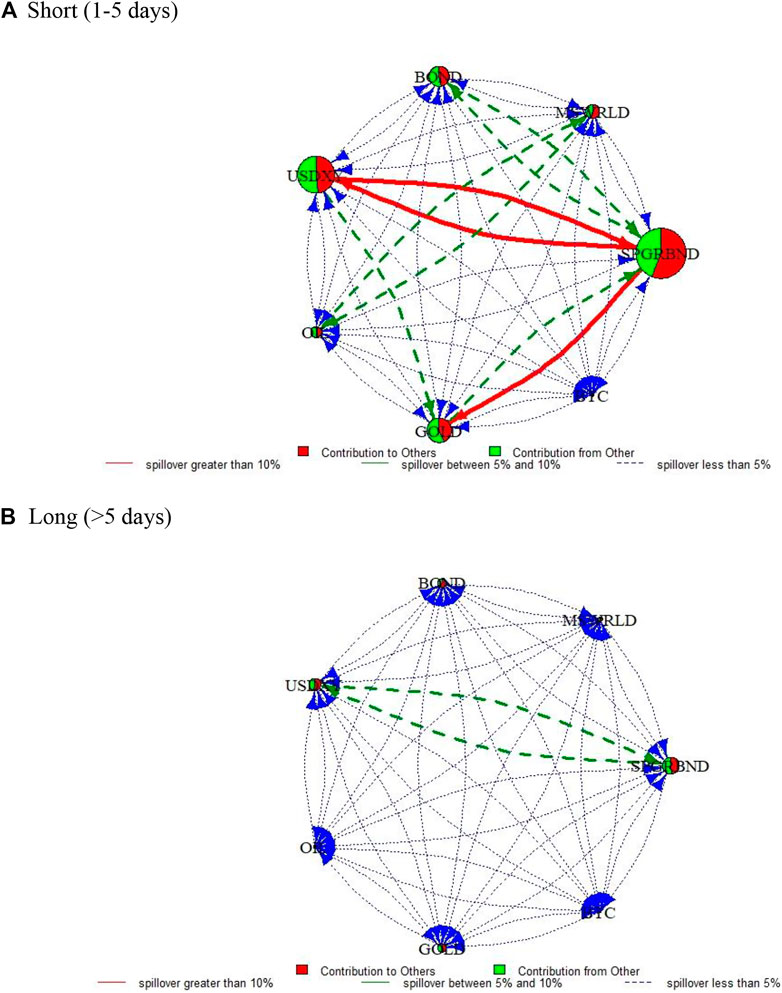
FIGURE 5. Network diagram of frequency-domain connectedness table using Barunik and Krehlik (2018). (A) Short (1-5 days). (B) Long (>5 days). Lags = 1 based on SIC. Forecast horizon of 100 days. Note: These network graphs illustrate the degree of total connectedness in a system that consists of the S and P Green Bonds, MSCI World index, Bonds, United States Dollar index, Oil, Gold, and Bitcoin returns over the full sample period for a) short-run and b) long-run connectedness. Total connectedness is measured using the Barunik-Krehlik framework. The size of the node shows the magnitude of the contribution of each variable to system connectedness, while the color indicates the origin of connectedness. In particular, the red color implies contribution from the variable under consideration to the other variables of the system, and the green color means contribution from the other variables to the variable under analysis. The color and shape of the arrows refer to the strength of connectedness. The red color and full line arrows represent strong spillovers while green and blue color arrows show medium and weak liquidity spillovers.
The node's size shows the magnitude of the contribution of each variable to system connectedness, while the color indicates the origin of connectedness. In particular, the red color implies contribution from the variable under consideration to the other variables of the system, and the green color indicates the contribution from the other variables to the variable under analysis. The color and shape of the arrows refer to the strength of connectedness. The red color and full line arrows represent strong spillovers while green and blue color arrows show medium and weak spillovers. In terms of size, Figure 4 shows that the Green bond and United States dollar have the strongest contribution to the return connectedness network, followed by the bond market and gold, whereas oil and global stock market are the smallest contributors. We find that the green bond index is strongly connected with the USD and bond during the full sample, ket, which is in line with Reboredo and Ugolini (2020). However, the GB is found weakly linked to oil, in contrast to Reboredo and Ugolini (2020) and Bouri et al. (2021). Furthermore, we find a weak relationship between GB and MSCI, suggesting that green bonds can serve as a diversifier in a stock portfolio, corroborating Han and Li (2020).
As to net spillovers, only GB and Gold appear as net transmitters of return spillover while the US dollar, bond market, stock market, and oil have a balanced contribution to the return network as their contributing spillover to the network is equal to their received spillover. GB is the leading net transmitter of return spillover. GB transmits strong return spillover to the United States dollar, bond market, and gold but weak spillover to others. While it receives strong spillover from US dollar and Gold, but moderate spillover from Bond and weak spillovers from the rest of assets. Hence, GB seems to interact mainly with the US dollar, bond market, and less degree gold. The strong connectedness between GB and bond index stocks looks intuitive since the former constitutes a part of the overall fixed income market. Hence, we expect such a relationship. As to the USD, the GB index includes many green bonds issued by governments from different countries and expressed in USD. Hence the GB index is significantly influenced by movements in treasury and currency markets (Reboredo and Ugolini, 2020). Meanwhile, our findings are different from Huynh et al. (2020), who find that green bonds and Bitcoin are shock senders to oil, gold, and the MSCI world equity index. US dollar has a strong spillover to Green bond and backward during a moderate spillover with gold in both directions.
Figure 5 plots the decomposition of the network connectedness into the short and long term. In the short run, we see a similar pattern. GB is the leading net transmitter of return spillover while its return spillover to the bond market is moderate, and its contribution to gold is as well decreased comparing to the static network. In the long run, overall, we find a very weak connectedness among the network that seems mainly driven by a moderate connectedness between GB and USD.
Since our aim in this paper is to examine the impact of COVID-19 on the network connectedness of green bonds with other financial assets. After having described, above, the network connectedness for the full sample, we now continue our analysis focusing on two sub-samples, pre-COVID and post-COVID. Results are plotted in Figures 6, 7. As shown in Figures 6A, in the pre-COVID, GB is the leading contributor in the network connectedness, followed by the USD index, then with lesser weight Gold and Bond index. Stock market and oil contribute weakly to the network connectedness while merely any contribution from BTC. Particularly, Figures 6B shows that COVID appears to have a noticeable impact on the whole connectedness network through several features. First, while the green bond index remains the most contributor, all the other six assets increased their contribution, except for oil, which maintains a small contribution. This is consistent with the global economic feature of COVID-19 that affects all the markets. The contribution of GB to the rest of the markets has declined in favor of a strong return spillover received from the bond market and stock market. We suggest that investors search for alternative financial instruments to hedge against COVID risk, other than conventional ones provided by the bond and stock market. US dollar showed increased connectedness and received a strong return spillover from bond and stock markets. Meanwhile, an increased connectedness is also found among the other individual assets, in magnitude between bond and stock market or bitcoin and gold. Overall, it seems that the impact of COVID significantly increased the connectedness between green bonds and other financial markets.
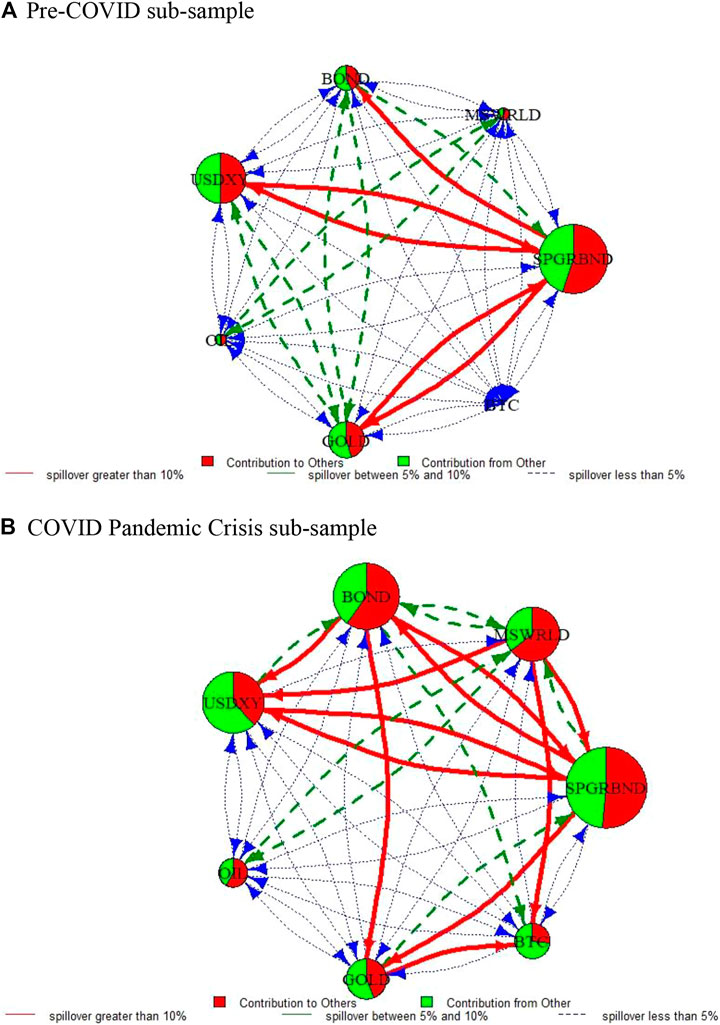
FIGURE 6. Network diagram of connectedness table using Diebold and Yilmaz (2012). (A) Pre-COVID sub-sample. (B) COVID Pan9demic Crisis sub-sample. Note: Refer to note with Figure 2.
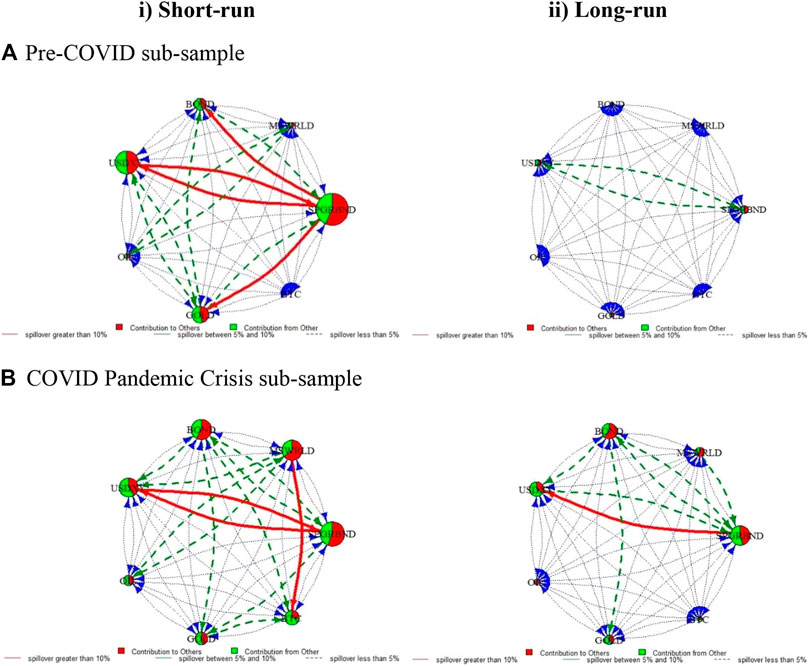
FIGURE 7. Network diagram of frequency-domain connectedness table using Barunik and Krehlik (2018). i) Short-run ii) Long-run. (A) Pre-COVID sub-sample. (B) COVID Pandemic Crisis sub-sample. Note: Refer to note with Figure 3.
The network connectedness during Pre-COVID (Figure 7A) looks similar to that observed in the full sample (Figure 5). However, some interesting findings need to be mentioned during the COVID sample (Figure 7B). While during pre-COVID, GB and US dollar were the most contributors to the network connectedness, after COVID, all the other assets increase their contribution. Accordingly, we find a significant connectedness among all the assets that seems to persist over the short-run. Particularly, during COVID, GB holds a strong bi-directional connectedness with the United States dollar and a moderate connectedness with the bond market in the short run. Likewise, it is shown a moderate return spillover from GB to gold. It is worth noting that the impact of COVID on the spillovers between GB and other assets reveals persists in the long run, although the magnitude is weakened about USD and gold. However, for the rest of the assets, COVID impact looks rather a short term.
Consequently, GB is found to play a significant role in the return network connectedness, during crisis time, both in the short and long run. However, during the normal time, the impact of GB on the network connectedness looks rather short-run. Particularly, during COVID time, GB maintains a strong bi-directional connectedness with the United States dollar and a moderate spillover with the bond market. Hence, investors should take into consideration this finding when diversifying their portfolios during a crisis.
Portfolio Implications
Table 3 reports the results of the hedge ratios (HR) and hedge effectiveness (HE). The negative mean value of HR indicates that investors should take the same position for both assets in the same portfolio (either short or long), while the positive mean value indicates that inverse positions are needed to hedge against the risk of each asset. It is worth noting that we find negative mean values of HR only for the pair GB and USD. Particularly, a long position of USD 1000 is hedged by taking a long position of US$953 in GB. For all the other assets, we find positive mean values of the HR. For instance, the positive mean value between gold and GB indicates that a short position on GB of US$1588 is required to hedge a long position of US$1000 in gold. Comparing the hedging ability of Green bond toward United States dollar and Gold, our results indicate that an investor who holds 000$ cash needs to buy 953 United States dollars of Green bond to hedge his portfolio risk. However, an investor who holds the same amount of $000 in gold should sell $1588 in Green bonds.
Likewise, positive HR for each of BTC, Bond, oil, and MSCI world implies that a short position in Gb is required to minimize the risk of a long position in each of these assets. As to HR values, gold has the highest ratio that exceeds 1.5, followed by the US dollar near the unit. For all the rest, HR values do not exceed 0.5. The HE values confirm the HR implications. The highest HE score for USD shows the highest effectiveness of this currency. Gold also has a high HE value. However, oil and stocks exhibit the lowest hedging effectiveness. Such results confirm that financial assets might be heterogeneous about Green bond. Hence, investors and portfolio managers need to carefully select which asset to include as hedger in a portfolio, including Green bond.
Conclusion
This paper aims to study the impact of COVID-19 on the dynamic connectedness of Green Bonds with various substantial financial assets, namely the Bond market, global stock market, USD index, and three hedging alternatives, oil, gold, and Bitcoin. We use a time-frequency framework, applying first the DY approach and then BK approaches. Our empirical analyses were based on a full sample, then on two sub-samples: pre and post- COVID.
We find a high total connectedness among the different assets. As net spillovers, Green bond and USD dollar are the leading contributors in the network connectedness. Moreover, the time-frequency analysis unveils that return connectedness is more pronounced in the short-run than the long term. This suggests that the connectedness between the Green bond and other assets seems to be more driven by the speculative behavior of active investors.
Furthermore, our time-varying analysis reveals an increasing trend of return connectedness over time. Based on the network connectedness, although all our sample assets are in United States dollars, we find that only green bonds and United States dollar are the most contributors to the return network. We then argue that the overall increasing trend might reflect the increased role of the green bonds in the whole system. One plausible explanation that we suggest is that increased connectedness reflects a progressive demand for this new asset by investors. Interested in good portfolio diversification potential, investors progressively accept green bonds as a good alternative to conventional bonds. In doing so, the United States dollar is excessively traded across investors, flying from conventional bonds to green bonds, United States dollar, which are both in the United States dollar.
Mainly interested in the impact of COVID on network connectedness, our analyses show a significant impact on the total network connectedness through a noticeable peak following the onset of the pandemic. The magnitude of connectedness has then reached an unprecedented level of 45%. The magnitude has slightly decreased afterward, but the level remains high, reflecting a common response among the assets to the continuing uncertainty induced by the global pandemic. Green bonds reveal to play a significant role in the network connectedness both during pre-COVID and post-COVID. Noticeably, during pre-COVID, green bond, United States dollar, and bond markets were the most connected network contributors. However, during the post-COVID sub-sample, all the other assets increased their contribution to the network connectedness, except for oil, which maintains a small contribution. Overall, it seems that COVID affects not only the return connectedness of green bonds but also the return connectedness of other major financial assets. Particularly, the contribution of GB to the network connectedness has declined in favor of a strong return spillover received from the bond and stock markets. We suggest that investors search for alternative financial instruments to hedge against COVID risk, other than conventional ones provided by the bond and stock market. United States dollar showed increased connectedness. Meanwhile, an increased connectedness is also found among the other individual assets, in magnitude between bond and stock market or Bitcoin and gold.
The impact of COVID on the spillovers between GB and other assets reveals persistence in the long run, although the magnitude is weakened for USD and gold. However, the impact of COVID seems more pronounced in the short term. Consequently, GB is found to play a significant role in the return network connectedness, during crisis time, both in the short and long run. However, during the normal time, the impact of GB on the network connectedness looks rather short-run. Particularly, during COVID time, GB maintains a strong bi-directional connectedness with the US dollar and a moderate spillover with the bond index. Hence, investors should take into consideration this finding when diversifying their portfolios during crisis periods.
Last, we also examined the portfolio implications of return connectedness results. We computed the hedge ratios and hedge effectiveness. We find that the hedging results confirm the green bonds as the best hedger for the United States dollar.
Our findings have some practical implications for environment-friendly investors. They may benefit from adding strongly connected assets in their portfolio and GB, such as the United States dollar, when the market is bullish. When the market is bearish, investors may diversify their portfolios by including the least connected assets such as oil, gold, and Bitcoin.
Within the increasing flow of empirical studies interested in Green bond and the impact of COVID, we claim that our study completes those focusing on the connectedness of Green bond with other financial assets and examining their hedging ability (Reboredo, 2018; Reboredo and Ugolini, 2020, Jin et al., 2020 and Bouri et al., 2021). Also, we add to Goodell (2020), who call to examine the impact of COVID on the connectedness of different financial assets. For future research, investigating which specific macroeconomic variables drive the return connectedness of green bonds with these selected assets constitutes a relevant research idea, particularly within the context of the COVID pandemic. We also suggest extending our work to volatility connectedness and alternative methods such as quantile connectedness.
Data Availability Statement
The data analyzed in this study is subject to the following licenses/restrictions: All the data, except Bitcoin, was gathered using the subscription to Thompson Reuters Datastream. Requests to access these datasets should be directed to MN, m.ab.naeem@gmail.com.
Author Contributions
All authors listed have made a substantial, direct, and intellectual contribution to the work and approved it for publication.
Funding
MN gratefully acknowledges the support of Science Foundation Ireland under grant number 16/SPP/3347.
Conflict of Interest
The authors declare that the research was conducted in the absence of any commercial or financial relationships that could be construed as a potential conflict of interest.
References
Adekoya, O. B., and Oliyide, J. A. (2020). How COVID-19 Drives Connectedness Among Commodity and Financial Markets: Evidence from TVP-VAR and Causality-In-Quantiles Techniques. Resour. Pol. 70, 101898. doi:10.1016/j.resourpol.2020.101898
Akyildirim, E., Corbet, S., Efthymiou, M., Guiomard, C., O'Connell, J. F., and Sensoy, A. (2020). The Financial Market Effects of International Aviation Disasters. Int. Rev. Financial Anal. 69, 101468. doi:10.1016/j.irfa.2020.101468
Al-Awadhi, A. M., Alsaifi, K., Al-Awadhi, A., and Alhammadi, S. (2020). Death and Contagious Infectious Diseases: Impact of the COVID-19 Virus on Stock Market Returns. J. Behav. Exp. Finance 27, 100326. doi:10.1016/j.jbef.2020.100326
Anser, M. K., Hanif, I., Vo, X. V., and Alharthi, M. (2020). The Long-Run and Short-Run Influence of Environmental Pollution, Energy Consumption, and Economic Activities on Health Quality in Emerging Countries. Environ. Sci. Pollut. Res. 27, 32518–32532. doi:10.1007/s11356-020-09348-1
Ashraf, B. N. (2020). Economic Impact of Government Interventions during the COVID-19 Pandemic: International Evidence from Financial Markets. J. Behav. Exp. Finance 27, 100371. doi:10.1016/j.jbef.2020.100371
Baillie, R. T., and Myers, R. J. (1991). Bivariate GARCH Estimation of the Optimal Commodity Futures Hedge. J. Appl. Econ. 6 (2), 109–124. doi:10.1002/jae.3950060202
Baker, S. R., Bloom, N., Davis, S. J., and Terry, S. J. (2020). Covid-induced Economic Uncertainty (No. w26983). Cambridge, MA: National Bureau of Economic Research. doi:10.3386/w26983
Baruník, J., and Křehlík, T. (2018). Measuring the Frequency Dynamics of Financial Connectedness and Systemic Risk*. J. Financial Econom. 16 (2), 271–296. doi:10.1093/jjfinec/nby001
Basher, S. A., and Sadorsky, P. (2016). Hedging Emerging Market Stock Prices with Oil, Gold, VIX, and Bonds: A Comparison between DCC, ADCC and GO-GARCH. Energ. Econ. 54, 235–247. doi:10.1016/j.eneco.2015.11.022
Baur, D. G., and McDermott, T. K. (2010). Is Gold a Safe haven? International Evidence. J. Banking Finance 34 (8), 1886–1898. doi:10.1016/j.jbankfin.2009.12.008
Benhmad, F. (2013). Bull or bear Markets: A Wavelet Dynamic Correlation Perspective. Econ. Model. 32, 576–591. doi:10.1016/j.econmod.2013.02.031
Bouri, E., Cepni, O., Gabauer, D., and Gupta, R. (2021). Return Connectedness Across Asset Classes Around the COVID-19 Outbreak. Int. Rev. Financ. Anal. 73, 101646. doi:10.1016/j.irfa.2020.101646
Broadstock, D. C., and Cheng, L. T. W. (2019). Time-varying Relation between Black and green Bond price Benchmarks: Macroeconomic Determinants for the First Decade. Finance Res. Lett. 29, 17–22. doi:10.1016/j.frl.2019.02.006
Ciner, C., Gurdgiev, C., and Lucey, B. M. (2013). Hedges and Safe Havens: An Examination of Stocks, Bonds, Gold, Oil and Exchange Rates. Int. Rev. Financial Anal. 29, 202–211. doi:10.1016/j.irfa.2012.12.001
Corbet, S., Larkin, C., and Lucey, B. (2020). The Contagion Effects of the Covid-19 Pandemic: Evidence from Gold and Cryptocurrencies. Finance Res. Lett. 35, 101554. doi:10.1016/j.frl.2020.101554
Das, D., Le Roux, C. L., Jana, R. K., and Dutta, A. (2020). Does Bitcoin Hedge Crude Oil Implied Volatility and Structural Shocks? A Comparison with Gold, Commodity and the US Dollar. Finance Res. Lett. 36 (2019), 101335. doi:10.1016/j.frl.2019.101335
Dash, D. P., Sethi, N., and Dash, A. K. (2021). Infectious Disease, Human Capital, and the BRICS Economy in the Time of COVID-19. MethodsX 8, 101202. doi:10.1016/j.mex.2020.101202
Diebold, F. X., and Yilmaz, K. (2012). Better to Give Than to Receive: Predictive Directional Measurement of Volatility Spillovers. Int. J. Forecast. 28 (1), 57–66. doi:10.1016/j.ijforecast.2011.02.006
Dogan, E., and Seker, F. (2016). Determinants of CO2 Emissions in the European Union: The Role of Renewable and Non-renewable Energy. Renew. Energ. 94 (2016), 429–439. doi:10.1016/j.renene.2016.03.078
Dutta, A., Das, D., Jana, R. K., and Vo, X. V. (2020). COVID-19 and Oil Market Crash: Revisiting the Safe haven Property of Gold and Bitcoin. Renew. Energ. 69 (April), 101816. doi:10.1016/j.renene.2016.03.078
Flaherty, M., Gevorkyan, A., Radpour, S., and Semmler, W. (2017). Financing Climate Policies through Climate Bonds - A Three Stage Model and Empirics. Res. Int. Business Finance 42, 468–479. doi:10.1016/j.ribaf.2016.06.001
Gevorkyan, A., Flaherty, M., Heine, D., Mazzucato, M., and Semmler, W. (2016). Financing Climate Policies through Carbon Taxation and Climate Bonds--Theory and Empirics. New York, NY: New School of Economics.
Goodell, J. W. (2020). COVID-19 and Finance: Agendas for Future Research. Finance Res. Lett. 35, 101512. doi:10.1016/j.frl.2020.101512
Glomsrød, S., and Wei, T. (2018). Business as Unusual: The Implications of Fossil Divestment and green Bonds for Financial Flows, Economic Growth and Energy Market. Energ. Sust. Dev. 44 (2017), 1–10. doi:10.1016/j.esd.2018.02.005
Haldar, A., and Sethi, N. (2020). The Effect of Country-Level Factors and Government Intervention on the Incidence of COVID-19. Asian Econ. Lett. 1 (2), 17804. doi:10.46557/001c.17804
Haldar, A., and Sethi, N. (2021). The News Effect of COVID-19 on Global Financial Market Volatility. Bemp 24, 33–58. doi:10.21098/bemp.v24i0.1464
Hammoudeh, S., Ajmi, A. N., and Mokni, K. (2020). Relationship between green Bonds and Financial and Environmental Variables: A Novel Time-Varying Causality. Energ. Econ. 92, 104941. doi:10.1016/j.eneco.2020.104941
Han, Y., and Li, P. (2020). Do Green Bonds Add Value in Multi-Asset Portfolios: Evidence from China. Available at SSRN: https://ssrn.com/abstract=3639753 (Accessed July 1, 2020).
Huynh, T. L. D., Hille, E., and Nasir, M. A. (2020). Diversification in the Age of the 4th Industrial Revolution: The Role of Artificial Intelligence, Green Bonds and Cryptocurrencies. Technol. Forecast. Soc. Change 159, 120188. doi:10.1016/j.techfore.2020.120188
Jin, J., Han, L., Wu, L., and Zeng, H. (2020). The Hedging Effect of Green Bonds on Carbon Market. Int. Rev. Financ. Anal. 71, 101509. doi:10.1016/j.irfa.2020.101509
Koop, G., Pesaran, M. H., and Potter, S. M. (1996). Impulse Response Analysis in Nonlinear Multivariate Models. J. Econom. 74 (1), 119–147. doi:10.1016/0304-4076(95)01753-4
Kroner, K. F., and Sultan, J. (1993). Time-varying Distributions and Dynamic Hedging with Foreign Currency Futures. J. Financial Quantitative Anal. 28, 535–551. doi:10.2307/2331164
Le, T.-L., Abakah, E. J. A., and Tiwari, A. K. (2021). Time and Frequency Domain Connectedness and Spill-Over Among Fintech, green Bonds and Cryptocurrencies in the Age of the Fourth Industrial Revolution. Technol. Forecast. Soc. Change 162 (July 2020), 120382. doi:10.1016/j.techfore.2020.120382
Lyócsa, Š., and Molnár, P. (2020). Stock Market Oscillations during the corona Crash: The Role of Fear and Uncertainty. Finance Res. Lett. 36 (June), 101707. doi:10.1016/j.frl.2020.101707
Mazur, M., Dang, M., and Vega, M. (2020). COVID-19 and the March 2020 Stock Market Crash. Evidence from S&P1500. Financ. Res. Lett. 38, 101690. doi:10.1016/j.frl.2020.101690
Monasterolo, I., and Raberto, M. (2018). The EIRIN Flow-Of-Funds Behavioural Model of Green Fiscal Policies and Green Sovereign Bonds. Ecol. Econ. 144 (May 2016), 228–243. doi:10.1016/j.ecolecon.2017.07.029
Naeem, M. A., Hasan, M., Arif, M., and Shahzad, S. J. H. (2020a). Can Bitcoin Glitter More Than Gold for Investment Styles? SAGE Open 10 (2), 2158244020926508. doi:10.1177/2158244020926508
Naeem, M. A., Farid, S., Balli, F., and Hussain Shahzad, S. J. (2021). Hedging the Downside Risk of Commodities through Cryptocurrencies. Appl. Econ. Lett. 28 (2), 153–160. doi:10.1080/13504851.2020.1739609
Narayan, S., Sriananthakumar, S., and Islam, S. Z. (2014). Stock Market Integration of Emerging Asian Economies: Patterns and Causes. Econ. Model. 39, 19–31. doi:10.1016/j.econmod.2014.02.012
Narayan, P. K., Phan, D. H. B., and Liu, G. (2020). COVID-19 Lockdowns, Stimulus Packages, Travel Bans, and Stock Returns. Financ. Res. Lett. 38, 101732. doi:10.1016/j.frl.2020.101732
Nasir, M. A., and Du, M. (2018). Integration of Financial Markets in Post Global Financial Crises and Implications for British Financial Sector: Analysis Based on A Panel VAR Model. J. Quant. Econ. 16 (2), 363–388. doi:10.1007/s40953-017-0087-2
Nguyen, T. T. H., Naeem, M. A., Balli, F., Balli, H. O., and Vo, X. V. (2020). Time-frequency Comovement Among green Bonds, Stocks, Commodities, Clean Energy, and Conventional Bonds. Finance Res. Lett. 40, 101739. doi:10.1016/j.frl.2020.101739
OECD (2020). Coronavirus: The World Economy at Risk. Paris, France: OECD Interim Economic Assessment.
Orlov, S., Rovenskaya, E., Puaschunder, J. M., and Semmler, W. (2018). Green Bonds, Transition to a Low-Carbon Economy, and Intergenerational Fairness: Evidence from an Extended DICE Model. SSRN J., 1–30. doi:10.2139/ssrn.3086483
Pesaran, H. H., and Shin, Y. (1998). Generalized Impulse Response Analysis in Linear Multivariate Models. Econ. Lett. 58 (1), 17–29. doi:10.1016/s0165-1765(97)00214-0
Rasool, Y., Zaidi, S. A. H., and Zafar, M. W. (2019). Determinants of Carbon Emissions in Pakistan's Transport Sector. Environ. Sci. Pollut. Res. 26 (22), 22907–22921. doi:10.1007/s11356-019-05504-4
Reboredo, J. C. (2018). Green Bond and Financial Markets: Co-Movement, Diversification and Price Spillover Effects. Energy Econ. 74, 38–50. doi:10.1016/j.eneco.2018.05.030
Reboredo, J. C., and Ugolini, A. (2020). Price Connectedness between green Bond and Financial Markets. Econ. Model. 88 (May 2019), 25–38. doi:10.1016/j.econmod.2019.09.004
Reboredo, J. C., Quintela, M., and Otero, L. A. (2017). Do investors Pay a Premium for Going green? Evidence from Alternative Energy Mutual Funds. Renew. Sust. Energ. Rev. 73 (November 2016), 512–520. doi:10.1016/j.rser.2017.01.158
Reboredo, J. C. (2013). Is Gold a Safe haven or a Hedge for the US Dollar? Implications for Risk Management. J. Banking Finance 37 (8), 2665–2676. doi:10.1016/j.jbankfin.2013.03.020
Sadorsky, P. (2012). Correlations and Volatility Spillovers between Oil Prices and the Stock Prices of Clean Energy and Technology Companies. Energ. Econ. 34 (1), 248–255. doi:10.1016/j.eneco.2011.03.006
Saeed, T., Bouri, E., and Tran, D. K. (2020). Hedging Strategies of green Assets against Dirty Energy Assets. Energies 13 (12), 3141. doi:10.3390/en13123141
Salisu, A. A., Ebuh, G. U., and Usman, N. (2020). Revisiting Oil-Stock Nexus During COVID-19 Pandemic: Some Preliminary Results. Int. Rev. Econ. Finance 69, 280–294. doi:10.1016/j.iref.2020.06.023
Shahzad, S. J. H., Bouri, E., Roubaud, D., Kristoufek, L., and Lucey, B. (2019). Is Bitcoin a Better Safe-haven Investment Than Gold and Commodities?. Int. Rev. Financial Anal. 63, 322–330. doi:10.1016/j.irfa.2019.01.002
Shahzad, S. J. H., Bouri, E., Roubaud, D., and Kristoufek, L. (2020). Safe haven, Hedge and Diversification for G7 Stock Markets: Gold versus Bitcoin. Econ. Model. 87, 212–224. doi:10.1016/j.econmod.2019.07.023
Sharif, A., Aloui, C., and Yarovaya, L. (2020). COVID-19 Pandemic, Oil Prices, Stock Market, Geopolitical Risk and Policy Uncertainty Nexus in the US Economy: Fresh Evidence from the Wavelet-Based Approach. Int. Rev. Financial Anal. 70 (May), 101496. doi:10.1016/j.irfa.2020.101496
Tang, D. Y., and Zhang, Y. (2020). Do shareholders Benefit from green Bonds?. J. Corporate Finance 61 (December 2018), 101427. doi:10.1016/j.jcorpfin.2018.12.001
World Health Organization (2020). Coronavirus disease 2019 (COVID-19). Situation Report, 82. Geneva, Switzerland: World Health Organization.
Wen, X., Guo, Y., Wei, Y., and Huang, D. (2014). How Do the Stock Prices of New Energy and Fossil Fuel Companies Correlate? Evidence from China. Energ. Econ. 41, 63–75. doi:10.1016/j.eneco.2013.10.018
Yang, J., and Zhou, Y. (2013). Credit Risk Spillovers Among Financial Institutions Around the Global Credit Crisis: Firm-Level Evidence. Manag. Sci. 59, 2343–2359. doi:10.1287/mnsc.2013.1706
Yang, Z., and Zhou, Y. (2017). Quantitative Easing and Volatility Spillovers across Countries and Asset Classes. Manag. Sci. 63, 333–354. doi:10.1287/mnsc.2015.2305
Zaremba, A., Kizys, R., Aharon, D. Y., and Demir, E. (2020). Infected Markets: Novel Coronavirus, Government Interventions, and Stock Return Volatility Around the Globe. Finance Res. Lett. 35, 101597. doi:10.1016/j.frl.2020.101597
Zerbib, O. D. (2019). The Effect of Pro-environmental Preferences on Bond Prices: Evidence from green Bonds. J. Banking Finance 98, 39–60. doi:10.1016/j.jbankfin.2018.10.012
Keywords: COVID19, green bonds, network connectedness, time and frequency domain, hedging effectiveness
Citation: Naeem MA, Mbarki I, Alharthi M, Omri A and Shahzad SJH (2021) Did COVID-19 Impact the Connectedness Between Green Bonds and Other Financial Markets? Evidence From Time-Frequency Domain With Portfolio Implications. Front. Environ. Sci. 9:657533. doi: 10.3389/fenvs.2021.657533
Received: 23 January 2021; Accepted: 11 May 2021;
Published: 28 May 2021.
Edited by:
Alex Oriel Godoy, Universidad del Desarrollo, ChileReviewed by:
Luis Edwin Gonzales-Carrasco, Pontificia Universidad Católica de Chile, ChileNarayan Sethi, National Institute of Technology Rourkela, India
Copyright © 2021 Naeem, Mbarki, Alharthi, Omri and Shahzad. This is an open-access article distributed under the terms of the Creative Commons Attribution License (CC BY). The use, distribution or reproduction in other forums is permitted, provided the original author(s) and the copyright owner(s) are credited and that the original publication in this journal is cited, in accordance with accepted academic practice. No use, distribution or reproduction is permitted which does not comply with these terms.
*Correspondence: Muhammad Abubakr Naeem, m.ab.naeem@gmail.com