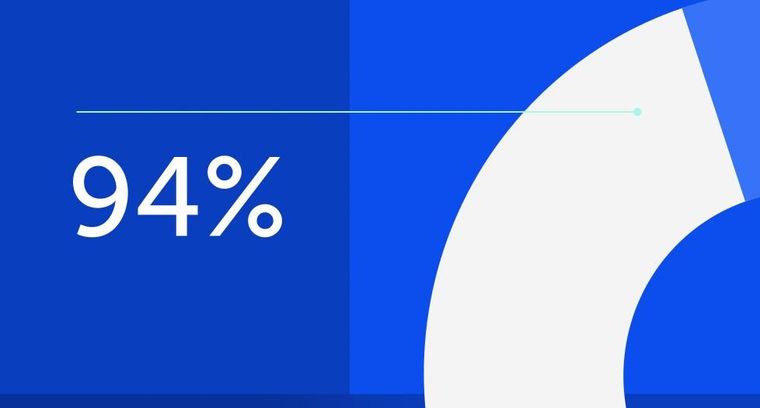
94% of researchers rate our articles as excellent or good
Learn more about the work of our research integrity team to safeguard the quality of each article we publish.
Find out more
ORIGINAL RESEARCH article
Front. Environ. Sci., 22 March 2021
Sec. Atmosphere and Climate
Volume 9 - 2021 | https://doi.org/10.3389/fenvs.2021.654651
This article is part of the Research TopicAtmospheric Aerosols and their Interactions with Clouds, Radiation, and Climate on Regional ScalesView all 7 articles
COVID-19 has a tremendous impact on both human life and the environment due to the unprecedented large-scale shutdown of economic activities at the beginning of 2020. While it was widely expected to see a dramatic reduction in air pollution, reality appears to be much more complex due to the joint influences of emissions and meteorology in dictating air pollution. By analyzing ample meteorological and environmental observational data, this study attempts to evaluate the contribution of an economic lockdown or at a well-below normal level across China to air pollution during the COVID-19 pandemic in the Beijing-Tianjin-Hebei region. Besides the unprecedented emission reductions that helped to improve air quality, multiple other factors came into play, such as high humidity and low wind speed that are favorable for haze formation. After separating long-term trends, seasonal signals, holiday effects, and meteorological contributions concerning climatology, we estimated that the relative contributions of human activities to changes in particulate matter with a diameter of less than 2.5 μm and nitrogen dioxide during the epidemic were −17.13 μg/m3 and −0.03 μg/m3, respectively, with negative quantities denoting reductions to air pollution. Furthermore, comparing the changes in PM2.5 and NO2 concentrations after lockdown revealed that for short-term control measures, meteorological factors mainly affected pollutant particles.
COVID-19 has been the most detrimental public crisis affecting mankind thus far in the twenty-first century. Shortly after its outbreak in Wuhan, China, the Chinese government took the exceptional measure to lock down Wuhan city beginning on January 23, 2020. Meanwhile, most economic activities elsewhere were also paused or at a much lower level. The move greatly reduced emissions of pollutants from vehicles, factory production, and human activities (Wang P. et al., 2020). At the beginning of the lockdown, the number of commercial vehicles and private cars on the road in North China dropped by 77 and 39%, respectively (MEP, 2020). Since then, the epidemic has been greatly suppressed (Wang C. et al., 2020; Chinazzi et al., 2020; Tian et al., 2020), and isolation has proved to be one of the most effective large-scale preventative measures taken over much of the world (Kraemer et al., 2020), triggering a wave of studies on its impact on the environment.
Previous studies have stated that air pollutant concentrations are dictated by both anthropogenic emissions and meteorological factors (Wu et al., 2008; Jhun et al., 2015; Li et al., 2017). Even if emissions were to remain consistent, unfavorable meteorological conditions, such as weak wind and circulation (Yang et al., 2018), low planetary boundary layer (PBL) (Liu et al., 2018; Su et al., 2020b), a stable atmosphere especially temperature inversion (Guo et al., 2020) also lead to the formation of heavy pollution (Mahmud et al., 2012; Ghude et al., 2016). Exceptionally high concentrations of particulate matter with a diameter of less than 2.5 μm (PM2.5) were often observed in winter (Zhang et al., 2018). There have been several studies on the impact of some major social events in China during which strict emission control measures were enforced, such as during the Beijing Summer Olympics, the Asia-Pacific Economic Cooperation, and the China Victory Day Parade (Witte et al., 2009; Wang et al., 2010; Huang et al., 2015). As natural experiments, these studies reinforced the impact of meteorology on air quality (Wang et al., 2009; Zhang et al., 2012; Liang et al., 2017). On the other hand, air pollution rarely happens in regions with little emissions. Therefore, it is thus important to differentiate the impact of meteorology and anthropogenic emission controls on air quality for improving emission-control strategies.
One of the aftermaths of the lockdown was that air pollution levels in many areas greatly reduced (Bao and Zhang, 2020; Liu et al., 2020; Sharma et al., 2020; Shehzad et al., 2020). Data obtained by satellites reveal that nitrogen dioxide (NO2) levels in eastern China dropped by 71.9% during the epidemic, compared with the same period in 2019 (Le et al., 2020). The TROPOspheric Monitoring Instrument revealed a 40% reduction (Bauwens et al., 2020). Through the analysis of real-time monitoring data, similar reductions in multiple pollutants have also been reported (Bao and Zhang, 2020). Despite the drastic reduction in precursor gases, a severe smog episode erupted after the lockdown (Sun et al., 2020). This was attributed to the formation of secondary pollution (Huang et al., 2020; Le et al., 2020), while others believed that it was caused by regional transport (Chang et al., 2020) and a low PBL height (Su et al., 2020b).
Given the complex meteorological changes witnessed during this period, the aforementioned processes may all have played some roles, but their relative contributions are unclear. As a method to eliminate meteorological effects, some previous studies have compared the same period with that of climatology (Bauwens et al., 2020; Shi and Brasseur, 2020). Su et al. (2020b) revealed that compared with PM2.5 concentrations during other similar shallow PBL periods in climatology, the average PM2.5 concentration during the lockdown period was the lowest and pointed out the impact of aerosol-PBL interactions on pollution. Sun et al. (2020) further found that the reduction in primary aerosols was higher than that of secondary aerosols at the same relative humidity (RH) level during the Lunar New Year (LNY). However, in these studies, the threshold of meteorological conditions was determined subjectively, and meteorological factors were analyzed individually. Considering these limitations, some studies have applied models to simulate pollution changes caused by lockdown and meteorology. Wang P. et al. (2020) used a model to show that in Beijing, unfavorable weather conditions caused a 40% increase in PM2.5. However, the model assumed constant emissions, incurring some uncertainties. In general, meteorological variables (such as RH, temperature, and wind speed) that affect pollution are usually interrelated, and to a certain extent, are controlled by synoptic circulations (Zhang et al., 2012). Therefore, the classification of circulation patterns can be adopted as a downscaling tool to evaluate the effects of lockdown control measures under different meteorological conditions (Demuzere et al., 2009; Liao et al., 2017).
The research aims to isolate the effect of lockdown control strategies in the short term rather than interannual trends, seasonality, LNY, and meteorology on changes in pollutant concentration. More specifically, its objective is to find the characteristics of pollutants under different meteorological conditions and the variation in pollutants under similar meteorological conditions. This study identifies the role of meteorology in the formulation of emission-control strategies in the future. In Data and Methods Section, various datasets and the methods used in this study are briefly introduced. Results Section shows the air quality and meteorological factors in different synoptic circulation patterns, trying to single out the role of meteorology. Conclusion Section summarizes the findings.
The study used real-time and continuous measurement of the daily average PM2.5 and NO2 concentrations in the Beijing-Tianjin-Hebei (BTH) region from January 2015 to December 2020. Supplementary Figure S1 provides the digital-elevation-model-derived topography of the study area and locations of the monitoring stations. The China National Environmental Monitoring Center provided the pollutant concentration data measured at the surface (Wang et al., 2014; Wang et al., 2017). Cross-validation with data collected by United States embassies demonstrated their reliability (Hu et al., 2015; Liang et al., 2016). In this study, the stations with valid data of duration shorter than 90% of the total duration have been eliminated. Taking into account the availability of pollutant observational data, the effective data period is 2015–2020. The National Climate Data Center provided daily meteorological observations, including RH and maximum wind direction and wind speed at 10 m. Other parameters from the European Center for Medium-Range Weather Forecasts reanalysis considered in this study include sea-level pressure (SLP) and PBL height (PBLH). Horizontal winds in the reanalysis dataset are daily averages. PBLH and SLP were both measured at 1,400 h, Beijing time (BJT = UTC +8 h) because the strong turbulent mixing, which occurs at noontime, best represents daily atmospheric mixing patterns (Su et al., 2020a; Shi and Brasseur, 2020).
In the study area (30°N-50°N, 107°E-127°E), a self-organizing map (SOM) cluster analysis (Liang et al., 2016) was used to analyze large-scale abnormal atmospheric circulations in the BTH region. Compared with the subjective circulation-classification method, SOM can help study the continuity and evolution of daily weather events in two-dimensional space through unsupervized learning algorithms (Hewitson and Crane, 1996; Hewitson and Crane, 2002; Horton et al., 2015). It is also an effective method to directly link-local pollution parameters with atmospheric circulation (Liu et al., 2006; Sheridan and Lee, 2011; Bei et al., 2016). A typical SOM network structure consists of an input layer and a competing layer (output layer), as shown in Figure 1. The variable
FIGURE 1. The schematic plot of a self-organizing map (SOM) cluster analysis model with its input and output layers. The input layer is the normalized sea level pressure. Through the unsupervized learning algorithm, each neuron in the output layer is matched with the input sample. The finally winning neuron is the classification of sea level pressure, and the nodes in the output layer represent the types of circulation.
For the analysis of the local source and regional transportation of pollutants in the BTH region during the lockdown period, the HYSPLIT model uses NCEP/GDAS reanalysis data with a spatial resolution of 1° × 1° to simulate a 24-h backward trajectory. During the lockdown periods, the backward trajectories of PM2.5 were calculated at the height of 10 m, beginning at 00:00, 06:00, 12:00, 18:00 BJT every day. The potential source contribution function (PSCF) and concentration weighted trajectory (CWT) based on the HYSPLIT model are used to analyze the local sources of pollutants and the relative magnitudes of the contributions from different regions, respectively. As a conditional probability function, PSCF gives the probability of wind from each wind direction associated with the specific pollutants (here defined as PM2.5
Following the positive response to COVID-19, air quality has greatly ameliorated over China. Satellites have monitored a significant reduction in anthropogenic emissions over the BTH region (Le et al., 2020). Is this change solely related to the economic slowdown? Here, we mainly focus on the BTH region, which experiences high aerosol loading. Figure 2 shows the fractional differences in PM2.5 concentration, i.e., differences between mean values in 2020 and mean values from the same periods in the climatology for the years 2015–2019, from 1 to January 20, 2020 (before the COVID-19 lockdown), 21 January to February 9, 2020 (the start of the COVID-19 lockdown), and 10 to February 29, 2020 (during the COVID-19 lockdown). Perplexedly, PM2.5 concentrations did not decline as expected at the beginning of the lockdown but increased by 23.38% compared with the same period in the climatology (Figure 2B).
FIGURE 2. The anomaly of PM2.5 in 2020 relative to the same period in 2015–2019 for 1–20 January (A), 21 January to 9 February (B), and 10–29 February (C).
Fractional Changes in near-surface wind speed and RH are shown in Figure 3 based on observations. At the beginning of the lockdown, high RH and relatively low wind speeds occurred simultaneously, conducive to the retention of pollution. We averaged the WS and RH anomalies at the observation site from January to February 2020. During the first 20 days of the city lockdown, the average RH was 62.6%, an increase of 33% from the same period in climatology. The frequency of RH levels higher than ∼60% was significantly higher (Figure 3D), which promoted the multiphase reaction of aerosol formation and growth (Huang et al., 2020; Le et al., 2020). In a humid environment, high RH can promote the conversion of gaseous pollutants into particulate pollutants through an aqueous reaction, thereby increasing PM pollution (Wang et al., 2016). In the BTH region, the average wind speed dropped by 28.9%. We also checked the pressure field anomalies (Supplementary Figure S2). At the beginning of the lockdown, BTH was located between a low-pressure system and a high-pressure system. The warm-air advection brought by the southwest wind heated the upper atmosphere, stabilizing the atmosphere (Su et al., 2020b). Over the next 20 days, the increase in wind speed led to the removal of pollutants from the region.
FIGURE 3. Fractional changes in RH (A–C) and in wind speed (E–G) wind speed. (A, E) before the lockdown; (B, F) at the beginning of the lockdown; (C, G) during the lockdown. Panels (D) and (H) show the probability density functions (PDFs) of RH and wind speed, respectively. Blue and red shaded areas represent the beginning of the COVID-19 lockdown and the climatology, respectively.
Considering that long-distance transportation is an important factor altering pollutant concentration, the distribution and contribution of potential pollution sources in Beijing were calculated using PSCF and CWT models. The potential sources of pollutants are shown in Figure 4A. Generally, when the winds come from southwest, southeast, and south, the possibility of increasing PM2.5 concentrations is highest. The WCWT plots in Figure 4B highlight key pollution source areas affecting PM2.5 concentrations in Beijing. The WCWT
In recent years, China has committed to reducing air pollution (Jin et al., 2016; Wei et al., 2019). From 2013 to 2018, the annual trend in PM2.5 in the BTH region reached −6.23 μg/m3 (Wei et al., 2020; Wei et al., 2021) and the lockdown happened to be the LNY holiday period exactly. Therefore, to gain the effect of meteorology on air pollution during COVID-19, the 2015–2019 pollutant concentration time series were successively extracted as interannual trends, seasonality, and LNY, same as Silver et al. (2020). Figure 5 shows the climatological trend, climatological seasonal cycle, and climatological LNY effect of PM2.5 in the BTH region. Although not shown, the same trend analyses were done for NO2 in the same way. PM2.5 and NO2 are reduced by 7.3 and 2.04 μg/m3 per year, respectively. The daily average of climatology is used to extract seasonal signals. The pollutant concentration during the Lunar New Year has been deleted, and interpolated data are used, avoiding double-counting the LNY effect. LNY is defined as two weeks before and three weeks after the Lunar New Year. In the two weeks before LNY, PM2.5 usually declines over BTH regions due to muted economic and human activity (clearer plot in Figure 5C). On the first day of LNY and the day after the Lantern Festival, the PM2.5 concentration increased by 79.89 and 44.68 μg/m3, respectively, which may be caused by fireworks.
FIGURE 5. Time series of (A) inter-annual trends, (B) seasonal signals and (C) LNY for PM2.5 (µg/m3). A 30-days smoothing window is applied to (A) and (B), and a 7-days smoothing window is applied to (C). The graph in (C) is the PM2.5 concentration two weeks before and three weeks after the Lunar New Year.
Residuals of interannual trends, seasonality, and LNY continue to be used to estimate the impact of meteorology on pollutants. Here, SOMs were used to identify 12 major circulation patterns in the climatological SLP over the BTH region during the same period of the lockdown (January and February of 2015–2019). After testing different map sizes, 4 × 3 nodes were selected in this study, best reflecting the impact of circulation patterns on air quality and providing an appropriate reasonable compromise between complex circulation patterns and air pollution. Figure 6 shows the 12 circulation types identified in this study. There are three circulation types associated with higher levels of pollution in the BTH region (Supplementary Figure S3): 1) Type 7, denoted as T7 (Figure 6G), when a weak pressure field was present, and 2) T8 and T12 (Figures 6H,L), before the passage of a cold front. T7, with its relatively weak pressure gradient, resulted in the lowest wind speed and the most stable noontime PBLH among the 12 circulation types (Supplementary Figure S4). Under these weather conditions, weak vertical mixing forms a strong barrier to inhibit aerosols in the PBL. By contrast, T6 had high wind speeds and a higher noontime PBLH. According to Zhang et al. (2009), for the T6 circulation type, i.e., a high-pressure system, vertical mixing, and horizontal transportation are rapid, diluting aerosols in both vertical and horizontal directions, resulting in clean air. Based on the observations, Supplementary Figure S5 shows the wind rose diagrams of the twelve circulation types for climatological samples. T11 and T12 bring in moist air via the easterly wind, facilitating pollution. The t-test revealed that air pollution parameters among the different circulation types generally had statistical differences (Supplementary Figure S6) at the 0.05 level, suggesting that this circulation classification is effective. Then the classification is applied to 2020.
FIGURE 6. Mean patterns of normalized SLP and frequencies of occurrence (numbers above each panel) of 12 circulation types (A–L) based on ERA5 reanalysis data for the climatology. All samples are normalized before being input into the input layer.
It is assumed that for any given predetermined circulation type
FIGURE 7. Changes in PM2.5(A) and NO2(B) concentrations driven by the lockdown. The red (blue) area shows the decreasing (increasing) concentrations. For the lockdown period, Met is defined as the residuals from the corresponding
Since the outbreak of the epidemic, almost all non-essential human activities in China have been suspended. However, serious pollution incidents still happened in North China during this period, instigating extensive investigations. To further understand the role of anthropogenic emissions and meteorology in regulating air pollution during this period, we quantified the contribution of control strategies in reducing pollutants by comparing pollutant concentrations under similar synoptic circulation conditions.
The abnormal PM2.5 in the BTH region was 31.1 and −0.03 μg/m3 at the beginning of and during the lockdown. At the beginning of the lockdown, the formation of secondary aerosols was caused by heterogeneous chemistry promoted by the increase in humidity, which eventually exacerbated PM2.5 (Le et al., 2020). During the next period, favorable weather conditions enabled control strategies to improve PM2.5 pollutions. Concerning NO2, lockdown drives a continuous reduction. From −1.33 μg/m3 at the beginning of the lockdown to −17.13 μg/m3 during the lockdown period, indicating that the lockdown did drive negative anomaly.
Moreover, results reveal that in the short term, at the beginning and during the lockdown, changes in gaseous pollutants had the same sign, and changes in particulate pollution had opposite signs. The difference confirms that lockdown helps improve air quality, especially gaseous pollutants. Our work emphasizes the importance of meteorological conditions because even if NOx-enhanced ozone concentrations during the epidemic were consistent with those at the beginning of the epidemic, PM2.5 concentrations did not increase due to favorable meteorological conditions. The role of the synergy between meteorological conditions and heterogeneous chemistry in particulate pollution formation is currently uncertain. This requires further study.
The COVID-19 lockdown in China provided a natural experiment to study the impact of emissions control on air quality. The reported studies mainly focus on individual meteorological factors, but it is still not enough to understand the synergistic effects of various meteorological factors in reducing pollution. Even if satellite observations, such as those from the European Space Agency and NASA, have provided evidence of unprecedented reductions in NO2 concentrations during the epidemic (Bauwens et al., 2020), in the long term, pollutant concentrations are still subject to long-term emission measures, seasonal changes, LNY and meteorological effects. This study emphasizes that under adverse meteorological conditions, the role of short-term emission control strategies is limited in improving particulate pollution. More research is still needed to explore the impact of meteorology and emissions on PM composition.
Publicly available datasets were analyzed in this study. This data can be found here: http://data.cma.cn/.
Conceptualization, ZL, TX; methodology, ZL, TX; software, TX; validation, ZL; formal analysis, ZL, TX; investigation, ZL, TX; resources, TX; data curation, TX; writing—original draft preparation, ZL, TX; writing-review and editing, ZL, JW; visualization, TX; supervision, ZL; project administration, ZL; funding acquisition, ZL. All authors have read and agreed to the published version of the manuscript.
This research was funded by the National Natural Science Foundation (42030606) and National Key Project (2017YFC1501702).
The authors declare that the research was conducted in the absence of any commercial or financial relationships that could be construed as a potential conflict of interest.
The Supplementary Material for this article can be found online at: https://www.frontiersin.org/articles/10.3389/fenvs.2021.654651/full#supplementary-material.
Bao, R., and Zhang, A. (2020). Does lockdown reduce air pollution? Evidence from 44 cities in northern China. Sci. Total Environ. 731, 139052. doi:10.1016/j.scitotenv.2020.139052
Bauwens, M., Compernolle, S., Stavrakou, T., Muller, J. F., van Gent, J., Eskes, H., et al. (2020). Impact of coronavirus outbreak on NO2Pollution assessed using TROPOMI and OMI observations. Geophys. Res. Lett. 47. doi:10.1029/2020gl087978
Bei, N., Li, G., Huang, R.-J., Cao, J., Meng, N., Feng, T., et al. (2016). Typical synoptic situations and their impacts on the wintertime air pollution in the Guanzhong basin, China. Atmos. Chem. Phys. 16, 7373–7387. doi:10.5194/acp-16-7373-2016
Chang, Y., Huang, R.-J., Ge, X., Huang, X., Hu, J., Duan, Y., et al. (2020). Puzzling haze events in China during the coronavirus (COVID-19) shutdown. Geophys. Res. Lett. 47, e2020GL088533. doi:10.1029/2020gl088533
Chinazzi, M., Davis, J. T., Ajelli, M., Gioannini, C., Litvinova, M., Merler, S., et al. (2020). The effect of travel restrictions on the spread of the 2019 novel coronavirus (COVID-19) outbreak. Science 368, 395–400. doi:10.1126/science.aba9757
Demuzere, M., Trigo, R. M., Vila-Guerau de Arellano, J., and van Lipzig, N. P. M. (2009). The impact of weather and atmospheric circulation on O3 and PM10 levels at a rural mid-latitude site. Atmos. Chem. Phys. 9, 2695–2714. doi:10.5194/acp-9-2695-2009
Ghude, S. D., Chate, D. M., Jena, C., Beig, G., Kumar, R., Barth, M. C., et al. (2016). Premature mortality in India due to PM2.5 and ozone exposure. Geophys. Res. Lett. 43, 4650–4658. doi:10.1002/2016gl068949
Guo, J., Chen, X., Su, T., Liu, L., Zheng, Y., Chen, D., et al. (2020). The climatology of lower tropospheric temperature inversions in China from radiosonde measurements: roles of black carbon, local meteorology, and large-scale subsidence. J. Clim. 33, 9327–9350. doi:10.1175/jcli-d-19-0278.1
Hewitson, B., and Crane, R. (1996). Climate downscaling: techniques and application. Clim. Res. 7, 85–95. doi:10.3354/cr007085
Hewitson, B., and Crane, R. (2002). Self-organizing maps: applications to synoptic climatology. Clim. Res. 22, 13–26. doi:10.3354/cr022013
Horton, D. E., Johnson, N. C., Singh, D., Swain, D. L., Rajaratnam, B., and Diffenbaugh, N. S. (2015). Contribution of changes in atmospheric circulation patterns to extreme temperature trends. Nature 522, 465–469. doi:10.1038/nature14550
Hu, J., Ying, Q., Wang, Y., and Zhang, H. (2015). Characterizing multi-pollutant air pollution in China: comparison of three air quality indices. Environ. Int. 84, 17–25. doi:10.1016/j.envint.2015.06.014
Huang, K., Zhang, X., and Lin, Y. (2015). The “APEC Blue” phenomenon: regional emission control effects observed from space. Atmos. Res. 164, 65–75. doi:10.1016/j.atmosres.2015.04.018
Huang, X., Ding, A., Gao, J., Zheng, B., Zhou, D., Qi, X., et al. (2020). Enhanced secondary pollution offset reduction of primary emissions during COVID-19 lockdown in China. Natl. Sci. Rev. 8 (2), nwaa137. doi:10.1093/nsr/nwaa137
Jhun, I., Coull, B. A., Schwartz, J., Hubbell, B., and Koutrakis, P. (2015). The impact of weather changes on air quality and health in the United States in 1994-2012. Environ. Res. Lett. 10, 084009. doi:10.1088/1748-9326/10/8/084009
Jin, Y., Andersson, H., and Zhang, S. (2016). Air pollution control policies in China: a retrospective and prospects. Int. J. Environ. Res. Public Health 13, 1219. doi:10.3390/ijerph13121219
Kraemer, M. U. G., Yang, C. H., Gutierrez, B., Wu, C. H., Klein, B., Pigott, D. M., et al. (2020). The effect of human mobility and control measures on the COVID-19 epidemic in China. Science 368, 493–497. doi:10.1126/science.abb4218
Le, T., Wang, Y., Liu, L., Yang, J., Yung, Y. L., Li, G., et al. (2020). Unexpected air pollution with marked emission reductions during the COVID-19 outbreak in China. Science 369, 702–706. doi:10.1126/science.abb7431
Li, Z., Guo, J., Ding, A., Liao, H., Liu, J., Sun, Y., et al. (2017). Aerosol and boundary-layer interactions and impact on air quality. Natl. Sci. Rev. 4, 810–833. doi:10.1093/nsr/nwx117
Liang, P., Zhu, T., Fang, Y., Li, Y., Han, Y., Wu, Y., et al. (2017). The role of meteorological conditions and pollution control strategies in reducing air pollution in Beijing during APEC 2014 and Victory Parade 2015. Atmos. Chem. Phys. 17, 13921–13940. doi:10.5194/acp-17-13921-2017
Liang, X., Li, S., Zhang, S., Huang, H., and Chen, S. X. (2016). PM2.5 data reliability, consistency, and air quality assessment in five Chinese cities. J. Geophys. Res. Atmos. 121, 10220–10236. doi:10.1002/2016jd024877
Liao, Z., Gao, M., Sun, J., and Fan, S. (2017). The impact of synoptic circulation on air quality and pollution-related human health in the Yangtze River Delta region. Sci. Total Environ. 607–608, 838–846. doi:10.1016/j.scitotenv.2017.07.031
Liu, F., Page, A., Strode, S. A., Yoshida, Y., Choi, S., Zheng, B., et al. (2020). Abrupt decline in tropospheric nitrogen dioxide over China after the outbreak of COVID-19. Sci. Adv. 6, eabc2992. doi:10.1126/sciadv.abc2992
Liu, L., Guo, J., Miao, Y., Liu, L., Li, J., Chen, D., et al. (2018). Elucidating the relationship between aerosol concentration and summertime boundary layer structure in central China. Environ. Pollut. 241, 646–653. doi:10.1016/j.envpol.2018.06.008
Liu, Y. G., Weisberg, R. H., and Mooers, C. N. K. (2006). Performance evaluation of the self-organizing map for feature extraction. J. Geophys. Res. Oceans 111. doi:10.1029/2005jc003117
Mahmud, A., Hixson, M., and Kleeman, M. J. (2012). Quantifying population exposure to airborne particulate matter during extreme events in California due to climate change. Atmos. Chem. Phys. 12, 7453–7463. doi:10.5194/acp-12-7453-2012
MEP (2020). Five experts focused on the causes of air pollution in the Beijing-Tianjin-Hebei region and surrounding areas during the control of Covid-19. Available at: http://www.mee.gov.cn (In Chinese).
Polissar, A. V., Hopke, P. K., and Harris, J. M. (2001). Source regions for atmospheric aerosol measured at Barrow, Alaska. Environ. Sci. Technol. 35, 4214–4226. doi:10.1021/es0107529
Sharma, S., Zhang, M., Anshika, , Gao, J., Zhang, H., and Kota, S. H. (2020). Effect of restricted emissions during COVID-19 on air quality in India. Sci. Total Environ. 728, 138878. doi:10.1016/j.scitotenv.2020.138878
Shehzad, K., Sarfraz, M., and Shah, S. G. M. (2020). The impact of COVID-19 as a necessary evil on air pollution in India during the lockdown. Environ. Pollut. 266, 115080. doi:10.1016/j.envpol.2020.115080
Sheridan, S. C., and Lee, C. C. (2011). The self-organizing map in synoptic climatological research. Prog. Phys. Geogr. Earth Environ. 35, 109–119. doi:10.1177/0309133310397582
Shi, X., and Brasseur, G. P. (2020). The response in air quality to the reduction of Chinese economic activities during the COVID-19 outbreak. Geophys. Res. Lett. 47. doi:10.1029/2020gl088070
Silver, B., He, X., Arnold, S. R., and Spracklen, D. V. (2020). The impact of COVID-19 control measures on air quality in China. Environ. Res. Lett. 15, 084021. doi:10.1088/1748-9326/aba3a2
Su, T., Li, Z., Li, C., Li, J., Han, W., Shen, C., et al. (2020a). The significant impact of aerosol vertical structure on lower atmosphere stability and its critical role in aerosol-planetary boundary layer (PBL) interactions. Atmos. Chem. Phys. 20, 3713–3724. doi:10.5194/acp-20-3713-2020
Su, T., Li, Z., Zheng, Y., Luan, Q., and Guo, J. (2020b). Abnormally shallow boundary layer associated with severe air pollution during the COVID-19 lockdown in China. Geophys. Res. Lett. 47, e2020GL090041. doi:10.1029/2020gl090041
Sun, Y., Lei, L., Zhou, W., Chen, C., He, Y., Sun, J., et al. (2020). A chemical cocktail during the COVID-19 outbreak in Beijing, China: insights from six-year aerosol particle composition measurements during the Chinese New Year holiday. Sci. Total Environ. 742, 140739. doi:10.1016/j.scitotenv.2020.140739
Tian, H., Liu, Y., Li, Y., Wu, C. H., Chen, B., Kraemer, M. U. G., et al. (2020). An investigation of transmission control measures during the first 50 days of the COVID-19 epidemic in China. Science 368, 638–642. doi:10.1126/science.abb6105
Wang, C., Horby, P. W., Hayden, F. G., and Gao, G. F. (2020). A novel coronavirus outbreak of global health concern. Lancet 395, 496. doi:10.1016/s0140-6736(20)30185-9
Wang, G., Zhang, R., Gomez, M. E., Yang, L., Levy Zamora, M., Hu, M., et al. (2016). Persistent sulfate formation from London Fog to Chinese haze. Proc. Natl. Acad. Sci. United States 113, 13630–13635. doi:10.1073/pnas.1616540113
Wang, J., Zhao, B., Wang, S., Yang, F., Xing, J., Morawska, L., et al. (2017). Particulate matter pollution over China and the effects of control policies. Sci. Total Environ. 584-585, 426–447. doi:10.1016/j.scitotenv.2017.01.027
Wang, M., Zhu, T., Zheng, J., Zhang, R. Y., Zhang, S. Q., Xie, X. X., et al. (2009). Use of a mobile laboratory to evaluate changes in on-road air pollutants during the Beijing 2008 Summer Olympics. Atmos. Chem. Phys. 9, 8247–8263. doi:10.5194/acp-9-8247-2009
Wang, P., Chen, K., Zhu, S., Wang, P., and Zhang, H. (2020). Severe air pollution events not avoided by reduced anthropogenic activities during COVID-19 outbreak. Resour.ces Conserv. Recycl. 158. doi:10.1016/j.resconrec.2020.104814
Wang, S., Zhao, M., Xing, J., Wu, Y., Zhou, Y., Lei, Y., et al. (2010). Quantifying the air pollutants emission reduction during the 2008 olympic games in beijing. Environ. Sci. Technol. 44, 2490–2496. doi:10.1021/es9028167
Wang, Y., Ying, Q., Hu, J., and Zhang, H. (2014). Spatial and temporal variations of six criteria air pollutants in 31 provincial capital cities in China during 2013-2014. Environ. Int. 73, 413–422. doi:10.1016/j.envint.2014.08.016
Wei, J., Li, Z., Cribb, M., Huang, W., Xue, W., Sun, L., et al. (2020). Improved 1 km resolution PM2.5 estimates across China using enhanced space-time extremely randomized trees. Atmos. Chem. Phys. 20, 3273–3289. doi:10.5194/acp-20-3273-2020
Wei, J., Li, Z., Guo, J., Sun, L., Huang, W., Xue, W., et al. (2019). Satellite-derived 1-km-Resolution PM1 concentrations from 2014 to 2018 across China. Environ. Sci. Technol. 53, 13265–13274. doi:10.1021/acs.est.9b03258
Wei, J., Li, Z., Lyapustin, A., Sun, L., Peng, Y., Xue, W., et al. (2021). Reconstructing 1-km-resolution high-quality PM2.5 data records from 2000 to 2018 in China: spatiotemporal variations and policy implications. Remote Sensing Environ. 252, 112136. doi:10.1016/j.rse.2020.112136
Witte, J. C., Schoeberl, M. R., Douglass, A. R., Gleason, J. F., Krotkov, N. A., Gille, J. C., et al. (2009). Satellite observations of changes in air quality during the 2008 Beijing Olympics and Paralympics. Geophys. Res. Lett. 36. doi:10.1029/2009gl039236
Wu, S., Mickley, L. J., Jacob, D. J., Rind, D., and Streets, D. G. (2008). Effects of 2000––2050 changes in climate and emissions on global tropospheric ozone and the policy-relevant background surface ozone in the United States. J. Geophys. Res. Atmos. 113. doi:10.1029/2007jd009639
Yang, Y., Zheng, X., Gao, Z., Wang, H., Wang, T., Li, Y., et al. (2018). Long-term trends of persistent synoptic circulation events in planetary boundary layer and their relationships with haze pollution in winter half year over eastern China. J. Geophys. Research-Atmospheres 123, 10991–11007. doi:10.1029/2018jd028982
Zhang, J. P., Zhu, T., Zhang, Q. H., Li, C. C., Shu, H. L., Ying, Y., et al. (2012). The impact of circulation patterns on regional transport pathways and air quality over Beijing and its surroundings. Atmos. Chem. Phys. 12, 5031–5053. doi:10.5194/acp-12-5031-2012
Zhang, Q., Ma, Q., Zhao, B., Liu, X., Wang, Y., Jia, B., et al. (2018). Winter haze over North China plain from 2009 to 2016: influence of emission and meteorology. Environ. Pollut. 242, 1308–1318. doi:10.1016/j.envpol.2018.08.019
Keywords: COVID-19, meteorology, synoptic circulation, air quality, lockdown
Citation: Xian T, Li Z and Wei J (2021) Changes in Air Pollution Following the COVID-19 Epidemic in Northern China: The Role of Meteorology. Front. Environ. Sci. 9:654651. doi: 10.3389/fenvs.2021.654651
Received: 17 January 2021; Accepted: 17 February 2021;
Published: 22 March 2021.
Edited by:
Yu Gu, University of California, Los Angeles, United StatesReviewed by:
Yuan Wang, California Institute of Technology, United StatesCopyright © 2021 Xian, Li and Wei. This is an open-access article distributed under the terms of the Creative Commons Attribution License (CC BY). The use, distribution or reproduction in other forums is permitted, provided the original author(s) and the copyright owner(s) are credited and that the original publication in this journal is cited, in accordance with accepted academic practice. No use, distribution or reproduction is permitted which does not comply with these terms.
*Correspondence: Zhanqing Li, emxpQGF0bW9zLnVtZC5lZHU=
Disclaimer: All claims expressed in this article are solely those of the authors and do not necessarily represent those of their affiliated organizations, or those of the publisher, the editors and the reviewers. Any product that may be evaluated in this article or claim that may be made by its manufacturer is not guaranteed or endorsed by the publisher.
Research integrity at Frontiers
Learn more about the work of our research integrity team to safeguard the quality of each article we publish.