- 1Faculty of Economics, Hunan University of Finance and Economics, Changsha, China
- 2School of Public Administration, Zhongnan University of Economics and Law, Wuhan, China
- 3Centre for Blue Governance, Faculty of Economics and Law, University of Portsmouth, Portsmouth, United Kingdom
Planning for the research and development (R&D) of renewable energy resources (RERs) has not received enough attention. This paper aims to study the planning for the R&D of RERs in order to avoid bottlenecks and ensure sustainable development in developing marine economies. We have established a triple difference model (DDD) model and a wise pig game model between the theoretical government and enterprise. The data on RERs come from the World Bank and International Energy Agency databases. We have three contributions on the basis of distinguishing between mature and immature marine RERs technologies. First, it emphasizes the importance of developing R&D planning for marine RERs immature technology in the future. Second, the DDD model is used to empirically establish whether RERs planning has a significant positive impact on RERs’ output, which explains the importance of existing RERs planning. Third, the wise pig game model is used to analyze the welfare benefits to the government brought by the R&D planning of marine RERs which proves the importance of future RERs R&D planning.
Introduction
As long as the world can develop 0.1% of total marine wave energy, it will be able to achieve 5 times the current world energy demand (Kumar et al., 2015). Marine renewable energy resources (RERs) are the largest undeveloped RERs in the world (Kumar et al., 2015). Most of marine developing countries are facing the problem of insufficient energy, thus they need to plan for the research and development (R&D) of marine RERs. Otherwise, if they encounter major natural disasters and epidemic disasters, such as earthquakes or COVID-19, they may be blocked intentionally or unintentionally at the key technology or sale point by developed countries and encounter a crisis of energy cut-off. Consequently, the theory of RERs becomes an important part of the theory of marine developing economies (Aktas and Kircicek, 2020; Coughlan et al., 2020; Isaksson et al., 2020; Raoux et al., 2020; Taveira-Pinto et al., 2020). Marine RERs include waves, tides, ocean currents, salinity, thermal gradients, marine biomass, offshore wind energy, and offshore solar energy (Taveira-Pinto et al., 2020). Much progress has been made in literature and research toward understanding the relation of RERs with developing marine economies. It has always been known that marine RERs has great potential, but developing countries need to distinguish their theoretical potential from technological potential in order to plan marine energy research and development (Liao and Drakeford, 2019; He and Walheer, 2020). Furthermore, it is necessary to distinguish between mature RERs and immature RERs, as well as technologies required for the development of related supply chains (Gorelick and Walmsley, 2020; Taveira-Pinto et al., 2020). Most previous studies have assumed that apart from wind power, which is a relatively mature technology, the basic theory of renewable energy technology is difficult to develop. Recent research, however, has found that only when marine developing economies plan how to conduct R&D of RERs (Davies et al., 2014; O’Hagan, 2016; Li Y. B. et al., 2020), are they able to reach a breakthrough in basic theory of the RERs and ensure not to be blocked at the key technology or sale. The objectives of this research include: (1) distinguishing between mature and immature technologies for marine RERs; (2) analyzing the impact of triple difference variables on output of RERs and analyze the internal economic mechanism of the rapid development of RERs by DDD model; (3) establishing a smart pig game model to analyze governments’ benefits in the process of the game between government and enterprises based on the R&D planning of RERs. Based on this, it is first necessary to establish what research has been covered in this field.
Experts in the field of marine RERs applied technology prefer to obtain technology application methods from research. In the study of wave and storm surges, a method of generating electricity using artificial lagoons was discovered. In their research of RERs in Lake Ontario and Lake Michigan, Sogut et al. (2018) found that wave power is more than10 KW/m at its peak during the winter and about 1 KW/m during the spring and the summer, while the power generation potential contained in storm surges is very high, such as, the highest is 2.088 × 1011 KW/m (58 GWh). Based on their results, using man-made lagoons to generate synthesize storm surges can contribute to power generation (Sogut et al., 2019). Another useful mechanism for research of RERs is sensor buoy systems which can be used for monitoring marine RERs, such as energy coming from wind, waves, and marine currents (Garcia et al., 2018). Further research has been carried out through the use of a non-hydrostatic hydrodynamic model used to assess suitable areas for the extraction of marine currents’ energy (Quesada et al., 2014). Following this, Deep et al. (2020) used the three-parameter Weibull model to measure the actual wind power of wind turbines, which is an improvement in the quality of wind speed measurement method of wind turbines, where the previous method by Quesada et al. (2014) overestimates wind speed by 25%.
In many cases, basic theory researchers tend to focus their plan on the basic theoretical research of renewable energy. However, in the marine RERs plan, it is necessary to build a variety of energy infrastructure paths that meet legal energy goals, in order to measure the impact of its land use and evaluate its performance relative to electricity demand (Thomas and Racherla, 2020). Furthermore, the scheduling problem of the energy hub system should also be planned (Dolatabadi et al., 2019), which is rationalized by scholars through the use of models such as the optimal expansion planning model for an energy hub with multiple energy systems (Zhang et al., 2015), optimization models for residential energy hubs (Bozchalui et al., 2012), and an uncertain model of optimal energy hub operation (Pazouki et al., 2014). It is also necessary to carefully calculate the costs of technology, equipment, power transmission and maintenance in the process of planning the use of marine RERs (Liu et al., 2015). Furthermore, a virtual power plant, which uses coordinated control technology, smart metering technology and information communication technology, can integrate a large number of distributed energy sources in the smart grid, and can effectively solve the instability problem of wind power and solar power (Han et al., 2019).
This article differs from research in basic technology and applied technology of marine RERs. Our first contribution is to study the marine RERs R&D plan of developing marine countries from the perspective of economics (Matei, 2020). We distinguish between mature technologies and immature technologies of marine RERs, and attempt to provide research in basic technology and applied technology with a marine RERs a global economics perspective of mature and immature technology, and provide a collection of entrepreneurial opportunities’ ideas for the development of the marine RERs industry chain.
Furthermore, extensive work has been done by economists, focusing on the economy policies of RERs. The marine RERs of some developing marine countries are showing a positive development trend (Varlas et al., 2017). The residents of few developing countries are often unwilling to use RERs and prefer to use wood as fuel (Okwanya et al., 2020). Often there is protest against the use of RERs, as the general population of developing countries cannot afford the price of RERs, especially where government policies do not consider the high installation and maintenance. Based on this, the governments of developing marine countries need to formulate policies on RERs investment and promote their use (Wang Q. et al., 2020), especially under the circumstance that the cost of traditional renewable energy such as wind and solar energy continues to fall. Energy system integration is also a difficult problem, which lacks effective policy and legal support in the EU (Cambini et al., 2020), let alone in developing countries. Thus, Odam and de Vries (2020) suggest that governments should carefully implement RERs policies guided by learning curve estimation. The more common RERs policies include low-carbon policies (Wendling et al., 2020), RERs production subsidies (Ravetti et al., 2020), RERs portfolio standards (Wendling et al., 2020), clean energy-related economic policies (Chen and Kim, 2020), and so on. At present, the application of RERs is recognized by the vast majority of residents in most of developing countries, and they have a willingness to consume RERs. With the continuous decline of RERs costs, most residents will soon have access to RERs. In order to quickly promote the application of renewable energy, governments of developing countries should consider the public’s future acceptance or market adaptability (Chen and Kim, 2020) and corporate investment income according to their own economic development level (Bakhtavar et al., 2020; Sukharev, 2020).
However, most of the economics academic research in these policy fields have not studied the R&D planning of RERs in the developing marine economy and have not paid attention to the bottleneck problems that marine RERs may face in the sense that they may easily be cut off from energy exporting countries. Fewer economic studies use a difference in difference in difference (DDD) model to assess the impact of energy planning. Therefore, our second contribution is that we choose the DDD model to study the impact of RERs planning on the related indicator. Economic research has not yet widely studied the marine RERs R&D planning game between government and enterprises from the perspective of game theory. Our third contribution is that we establish a wise pig game model to analyze the benefits obtained by the governments of developing marine countries in the R&D planning of marine RERs.
We get our desired results of the paper. Firstly, governments, enterprises, and researchers should pay attention to the distinction between mature and immature technologies for marine RERs, especially the R&D planning in the field of immature technologies. Secondly, the triple difference variable only has a significant positive impact on output of RERs which shows that RERs planning plays a great role in promoting its development. Thirdly, the growth of per capita GDP, R&D expenditure, R&D personnel and carbon dioxide emissions have a positive effect on the output of RERs. Lastly, under certain probability conditions, the government can maximize the welfare of the society by R&D planning of RERs. The results of the research can provide reference for governments, technical and economic theory researchers, and energy companies in various marine developing economies, stressing the importance of R&D planning of RERs and the need for further R&D planning.
This paper is structured into four sections. The following section provides theories which include RERs distribution theory, mature technology theory, immature technology theory and RERs planning theory. The section thereafter covers the data and DDD model, which shows the importance of RERs planning. Following that, the next section uses a wise pig game model to analyze the government’s planning of RERs in marine developing countries, and the payment or benefits brought by it. The field of RERs R&D planning is also discussed. The last section concludes this work.
Mature and Immature RERs Technology
The mature area of marine RERs includes mainly offshore wind energy (Held et al., 2019; Deep et al., 2020) and offshore solar energy (Hurst, 1990), within which the technologies are well developed and widely implemented. In this section, we distinguish between mature technologies and immature technologies of marine RERs based on high-quality literature, commencing with the distribution of marine RERs in two major developing marine countries, China and India.
RERs Distribution
According to data from the 2014 to 2017 China Ocean Statistical Yearbook of total marine renewable energy power generation (billion kilowatt hours) of 48 coastal countries, we are able to establish RERs distribution in developing marine economies as shown Figure 1. Herein, showing the world’s total RERs power generation data from 2010 to 2014. From the data of Figure 1, it is apparent that China has the largest renewable energy generation capacity in the world. The power generation from marine renewable energy in other developing countries is only about 0.003--5% of that of China in 2014. Such countries being Mexico, Argentina, Chile, Cuba, Ecuador, Haiti, Honduras, Jamaica, Nicaragua, Panama, Paraguay, Peru, Albania, Belgium, Saudi Arabia, Mauritius, Morocco, Nigeria, Reunion, South Africa, Tunisia, Myanmar, Cambodia, North Korea, Laos, Malaysia, Thailand, and Vietnam. While the renewable energy generation capacity of China was 23%1 of total generation capacity of China in 2014. The renewable energy generation capacity of several developed countries such as the Unite States, Brazil, Canada, India, Russia, German, Japan, Italy and France, are, respectively, 43, 33, 31, 15, 13, 13, 11, 9, and 7% of that of China in 2014. These data show that no matter whether it is a developing or developed country, their total amount of RERs is still relatively low. The maps of Figure 1 can more intuitively show the gap in the total RERs power generation of countries from 2010 to 2014. Similarly, the data shows that total renewable electricity consumption (billion kilowatt hours) of 48 countries are equal to total RERs power generation (billion kilowatt per hour) of those countries from 2010 to 2012. These data show that marine renewable energy generation has been completely consumed and there is no surplus. Therefore, it is feasible to increase the generation of ocean renewable energy in market demand.
Next, we use the data of two major maritime developing countries, China and India, to illustrate the RERs situation. Figure 2 was developed on the basis of the data from the 2018 China Ocean Statistical Yearbook, which shows the hydroelectric power generation of China’s coastal provinces from 2013 to 2017. Hydropower has the highest utilization rate of marine RERs in China. Between 2013 and 2017, the province with the largest hydropower generation in China’s coastal provinces is Guangxi, the second Fujian, the third Guangdong and the fourth Zhejiang from 2013 to 2017. It is notable, in the 2018 China Energy Statistical Yearbook, only hydro power and wind power data for 2017 are available. Hydro power made up 35837.39 × 104 tce (ton coal equivalent) and wind power 8885.95 × 104 tce in 2017. The official statistical data for China’s biochemical energy, congen biomass power, waste to energy etc. is unavailable, which shows that this part of China’s renewable energy has not produced scale. This indicates that China’s renewable energy has room for planning in terms of regions and types.
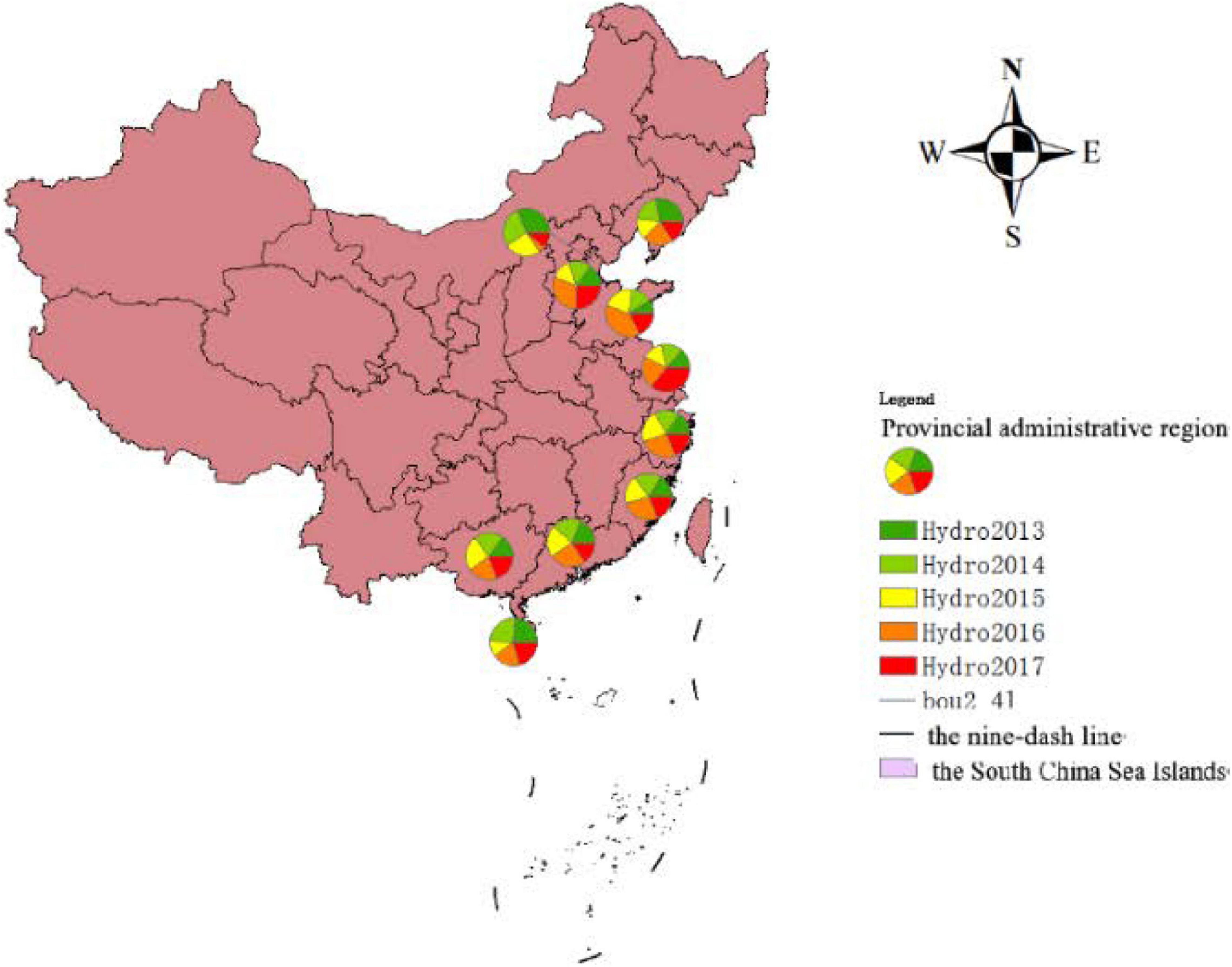
Figure 2. Hydroelectric power generation of China’s coastal provinces from 2013 to 2017. The dark green is the 2013 data, the light green is the 2014 data, the yellow is the 2015 data, the orange is the 2016 data, and the red is the 2017 data.
The capacity of grid interactive renewable power (in megawatts) in India is shown in Figure 3. According to the 2018 India Statistical Yearbook, in 2017, the biggest small hydropower generating state of India was Karnataka, the second biggest Himachal Pradesh, and the third biggest Maharashtra. For the case of wind power in 2017, the largest source was in the Tamil Nadu state, the second Gujarat, the third Maharashtra, and the fourth Rajasthan. The amount of biochemical energy is very small in India. The largest biochemical energy producing state for 2017 was Maharashtra, the second Uttar Pradesh, and the third Tamil Nadu for congen biomass power. It is distributed in a wide area. The amount of Waste to Energy is less than the amount of bioenergy, which is compared with the total amount of renewable energy, it is not worth mentioning. The top states in terms of solar energy in 2017 were Andhra Pradesh, Rajasthan, Tamil Nadu, Telangana, Gujarat, and Karnataka. The top states in terms of total energy were Tamil Nadu, Karnataka, Gujarat, Rajasthan, Andhra Pradesh, Uttar Pradesh, and Telangana in 2017. The total RERs power in 2017 was 45,924 megawatts, while total installed generating capacity of electricity was about 350,367 megawatts. Therefore, the ratio of renewable energy power generation to total power generation was about 16% in 2017. India’s data of RERs show that India have developed various RERs, but each type of RERs is not used on a large scale. Moreover, India’s total RERs accounted for only 15.22% of China’s total RERs in 2014.
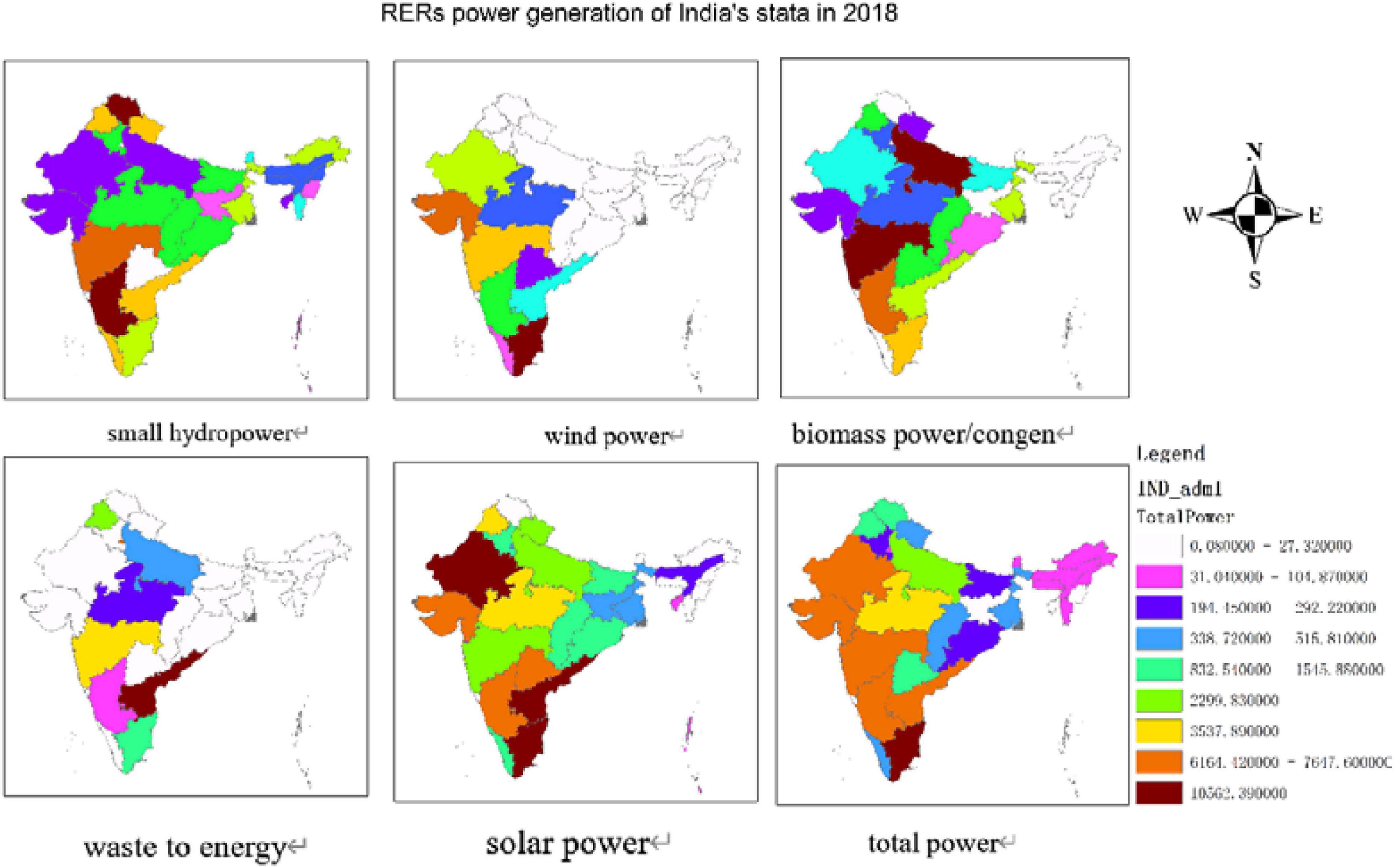
Figure 3. The generation (megawatts) of small hydropower, wind power, biomass power/congen, waste to energy, solar power, and total power in India’s states in 2018.
The above analysis of Figures 1–3 show that RERs are an important part of the national plan of developing coastal countries, but great potential for marine RERs still exists. Because big energy producing and consuming countries such as China, the chairman’s important speech at the Climate Ambition Summit in December 2020 specifically emphasized increasing the total installed capacity of wind and solar power generation, and did not mention the plan for marine RERs. Small energy countries and energy weak countries are more worried about energy shortages than developed countries. Many small developing marine economies rely on imports for energy. When they encounter earthquakes, natural disasters or major epidemics such as COVID-19, they may be cut off from the energy giants. If the small marine developing economies plan the R&D of RERs, they can alleviate the problem of energy shortages in the long term.
Hypothesis 1: In developing marine countries, there is more room to plan for marine renewable energy in both the types and geographical areas, which include waves, tides, ocean currents, salinity, thermal gradients, marine biomass, offshore wind energy and offshore solar energy.
Mature Technology
Offshore Wind Energy
Wind energy technology is the most mature technology and the fastest growing of all RERs in the world (Deep et al., 2020) but does have life cycles. The turning point of wind energy growth was in 2011, where wind energy in the world reached saturation and the distribution of the wind energy network was not planned in practice (Zhang and Guan, 2019). This lead to fierce competition in the wind power product market. Its mature technology includes nacelle (Madvar et al., 2019), the analysis of gust characteristics (Fan et al., 2020), the novel hybrid wind-solar-compressed air energy storage system which can solve the intermittent problems of wind and solar energy (Ji et al., 2017), the technology development of scavenging wind energy by using the electromagnetic effect (Chen et al., 2018), and the manufacturing of various parts of wind power generators, to name a few. The top three countries in the world for offshore wind power installations are Britain, Germany and China. The Chinese government will no longer subsidize the installation of wind turbines from 2021.
Tidal Energy
There are three evolving forms of tidal power plants. Single-storage one-way power stations, that generate power when the tide rises or falls; single-storage two-way power station, capable of generating power during both rising and falling tides; double-storage two-way power stations, which through the establishment of upper and lower reservoirs can generate electricity 24 h a day. However, none of these reservoirs can generate electricity at low tide.
Offshore Solar Energy
The technology of solar power generation is notably mature, and the photovoltaic industry in China is already saturated. Solar power generation directly converts light energy into electric energy, or converts thermal energy into electric energy. Solar cells are the intermediary for this conversion of electrical energy. However, the application of offshore solar power is still not extensive. It is necessary to create new materials to convert ocean solar energy into electricity, so that ocean renewable solar energy can be widely used.
Hypothesis 2: Mature technology provides a technological platform for the R&D planning of marine renewable energy resources in developing marine countries.
Immature Technology
Variability, uncertainty, low power density, harsh environmental conditions and distribution are always RERs’s challenges, which coupled with regulatory barriers, environmental barriers and technology costs barriers (Osorio et al., 2020), therefore, the widespread use of offshore RESs is greatly restricted.
Offshore Wind Energy
Due to the non-linear and random characteristics of wind energy, wind energy prediction is still a challenging task (Zendehboudi et al., 2018; Yang et al., 2019). Wind energy conversion is also a complex technology that has not been fully controlled. Near-inertial wind energy has always been overestimated, and it is necessary to use surface floating objects for accurate estimation (Liu F. et al., 2019). Determining how to accurately estimate ocean wind speed by the cyclone celestial navigation system requires the development of professional knowledge. Since it is difficult to forecast wind energy, only a breakthrough in the forecasting method can solve the problem. The prediction method that scientists prefer is that of artificial neural networks, but there is still a certain technical gap between this method and accurate prediction of wind energy (Marugan et al., 2018). Offshore wind power construction, maintenance, equipment life, impact on navigation safety and fishery, fatigue load of wind turbine blades, resistance to strong earthquakes, and resistance to strong typhoons are all issues that need to be resolved. The development of offshore energy is limited by the geotechnical structure, as it is difficult to establish a complete geological and geotechnical classification map suitable for all offshore RESs, because the map will vary greatly depending on the geotechnical structure type of RERs (Coughlan et al., 2020; Taveira-Pinto et al., 2020).
Tidal Energy
In order to utilize tidal energy, it is necessary to evaluate it. Determining how the high-resolution sounding method, tidal components, and high-order harmonic components affect the quantification of tidal flow energy is a research trend (Mejia-Olivares et al., 2020). The grid-connected tidal stream turbine (TST) flexible control method for grid failure is under study (Toumi et al., 2020). In Maine, United States, there is the world’s first tidal power generating unit without a dam, but it has not yet contributed electricity to users. Establishing how to break through the limitations of tidal energy site selection, how to promote tidal power stations without dams and reduce the loss of tidal energy generation are difficult problems that need to be overcome.
Hydrogen Energy
At present, the four issues that need to be researched and developed for water-to-light complementary power generation include the ratio of water-to-light capacity, the issue of absorption and access to the system, the issue of coordination with conventional power sources, and the issue of impact on grid operation (China Energy News, 2020).
Offshore Solar Energy and Thermal Gradients
The R&D of new materials to reduce the cost of solar energy, to alternate use of ocean thermal energy and offshore solar energy, to reduce the intermittent problem of combining wave energy and solar energy (Oliveira-Pinto et al., 2020), which requires scientists to spend more time on research (Straatman and Van Sark, 2008).
Marine Biomass
Marine bioenergy is the energy contained in marine algae. For example, macroalgae are chopped up and decomposed and fermented by bacteria to produce methane and hydrogen, which can be used as fuel to replace petrochemical energy. It is necessary to establish how to widely grow some invertebrate organisms in the ocean as raw materials for the production of biogas and biological fertilizers. Few scholars have conducted in-depth studies relating to this (Hackl et al., 2018).
Ocean Currents
Ocean current or ocean current velocity detection is on-site measurement by a staff member in a boat. It is important to note that it is inconvenient to adjust the position of the detector during the on-site measurement process, and new methods of the detection need to be created (Zhao et al., 2020). The generation mechanism, instability, variability, life cycle, destructive force, turbulence cascades, internal wave interaction and the choice of the best location of ocean currents are all areas where there are more questions than answers (McWilliams, 2016; Barnier et al., 2020). How to make ocean currents impact on the genetic structure of biota and provide solutions for marine biological biofouling is also a problem worth exploring (White et al., 2010).
Marine Biofouling
The marine pollution of offshore renewable energy equipment includes biofouling and non-biofouling. Biofouling mainly includes acorn barnacles, mussels, calcareous tuberculosis, bryozoans and kelp. Non-biofouling factors include physical and chemical characteristics of seawater such as temperature, PH, dissolved oxygen and organic content, hydrodynamic conditions such as current speed, wave exposure, distance from shore and depth to water, and underlying characteristics such as material composition, color, roughness, immersion time, and exercise time (Vinagre et al., 2020). Non-biofouling and biological pollution are intertwined, affect each other and aggravate each other. How to reduce biological and the factors which aggravate marine biological pollution, and how to accurately draw a map of biological pollution are problems that need to be solved.
Salinity and Thermal Gradients
The difference in ocean salinity can be used to generate electricity, but the technology for measuring salinity at high latitudes is not yet mature at the time of writing (Supply et al., 2020). The impact of the salinity difference between the two bodies of water on kinetic energy and how to use it for power generation requires further research (Lee et al., 2016). Few scientists have studied the use of ocean thermal gradients to generate electricity.
Energy Storage Systems
The energy storage system is the most effective solution to the instability of marine renewable energy and can minimize power fluctuations in the hybrid power system. However, this effective energy storage system has not been used in practice (Aktas and Kircicek, 2020). The improvement of the energy storage system can increase the utilization rate of RERs (De Quevedo et al., 2019). Battery energy storage systems is a suitable technology to eliminate the uncertainty and instability of renewable energy (Hemmati, 2018). If marine developing countries are to establish a new paradigm that meets RERs power system, microgrid integration, synchrophasor-driven automation technology, flexibility and safety requirements, and robustness and reliability methods for generation and dispatch, etc., it will be also large-scale technology challenge (Aminifar et al., 2019; Failler et al., 2019).
Monitoring Marine RERs
In the monitoring of marine RERs, technical experts need to develop multi-sensor floating system energy parameters for monitoring the marine environment, and establish a dedicated floating sensor device that can easily sample wind, wave and ocean current energy (Garcia et al., 2018). In addition, they need to study how to use sound emission to monitor the health of marine renewable energy equipment (Walsh et al., 2017).
The Impact of RERs on the Marine Environment
There are many areas that have not been studied in depth on the impact of renewable energy on the marine environment, such as the influence of tidal underwater kites on the depth of fish distribution, the impact of offshore wind power plants on the living environment of marine birds and fish, and the influence of ocean salinity on the distribution pattern of anaerobic bacteria (Li Z. et al., 2020). Additionally, there has been a lack of research into the effect of thermal gradients on marine bacteria (Sollich et al., 2020) and the effect of natural thermal gradients on protein synthesis in marine organisms (Rastrick and Whiteley, 2020). How triple salinity shapes the water masses of the basin-scale oceans and affects the climate is also a problem that needs long-term tracking (Hu et al., 2020).
Hypothesis 3: Immature technologies provide technical and environmental challenges for the R&D planning of marine renewable energy resources in developing marine countries.
RERs Planning
The purpose of RERs planning is to reduce dependence on fossil energy consumption and reduce the pressure on the environment caused by carbon dioxide emissions (Su et al., 2020). Most countries in Africa have set targets to support RERs, among which the most successful country in attracting wind energy investment is Kenya (Kazimierczuk, 2019). The implementation of the European Union’s renewable energy policy is very effective, because the European Union’s has been committed to energy planning, and governments of various countries have also provided appropriate policy support for RERs. Contrastingly, in some countries, such as Finland, RERs producers had little say in the formulation of energy policies (Ratinen, 2019). Therefore, if the developing marine economy countries want to develop renewable energy, they must provide certain support and planning for RERs (Gnatowska and Moryn-Kucharczyk, 2019), and further involve companies that produce RERs in the planning process.
Planning Basis
Wind resources in coastal areas are relatively rich, which can provide a theoretical basis for the planning of regions and seasons of wind power (Liu Y. Z. et al., 2019). The wind energy planning framework includes long-term wind speed sampling, wind speed prediction reliability, energy commercialization, wind farm profitability (Bernardes et al., 2018), electric vehicle charging networks (Mehrjerdi and Hemmati, 2020), etc. Technology, environmental protection and energy prices are the most influential driving factors for the development of RERs (Chen et al., 2020). The improvement of the efficiency of renewable energy planning is also an issue that must be considered in the planning process.
Microgrid Planning
It is necessary for the government to properly plan the construction of microgrids in areas suitable for the development of RERs, because the flexible areas of microgrids can effectively improve the renewable energy utilization rate of microgrids, improve the reliability of microgrid operations, and reduce the load of large-scale grids (Su et al., 2020). Governments can integrate renewable energy into the microgrid energy supply system of the community to reduce carbon emissions (Bakhtavar et al., 2020), and create a multi-energy microgrid with optimal performance (Yang et al., 2020). In the planning of the microgrid balanced energy network, technical experts need to consider the characteristics of renewable energy and multi-energy loads, time series, auto-correlation and cross-correlation (Lei et al., 2020).
Excess Energy Planning
In the case of excess wind and solar energy, it is important to understand how to use them to produce hydrogen and promote sustainable energy development (Nadaleti et al., 2020). The energy storage system and the transmission network are combined to avoid the imbalance in the performance of RERs power generation, and the excess RERs can be transmitted to the power market for digestion (Zhang et al., 2020). But the uncertainties in the supply and demand of renewable energy resources pose intractable problems for planners.
The planning of RER requires the participation of the government, and the market for RERs requires appropriate laws, regulations and government documents. For the planning, design, operation and control of RERs systems, its technical optimization has become very important (Li et al., 2018; Aminifar et al., 2019). Scientists need to turn the problem list of RERs bottleneck technologies into a problem list of scientific research (Maciel et al., 2018), plan various RERs standards (Maciel et al., 2018), combine RERs to overcome their instability and intermittent characteristics (Zhang et al., 2019), plan the optimal configuration of multi-energy systems, and consider demand response when planning RERs (Asensio et al., 2018).
Hypothesis 4: Different countries have certain plans for renewable energy resources, but there is no plan for the research and development of renewable energy resources. After a period of economic development in the previous plans, it is necessary to increase plans for new renewable energy technologies and remove some plans for mature and backward technologies.
Methdology
Samples and Data
For this research, 232 developing countries and developed countries were used as samples, in which there are 204 developing countries, 62 developing marine countries and 28 developed countries. The time span of the panel data is from 1990 to 2016. RERs output and consumption data come from the Sustainable Energy database of the World Bank. CO2 emissions data comes from the International Energy Agency (IEA). Various GDP and R&D data come from the Sustainable Development Goals of the World Bank. Countries with coastlines belong to marine areas, and countries without coastlines belong to non-marine areas. Every country with a coastline is verified by Google Maps. The choice of planning time comes from Wikipedia2. We use “country name + renewable energy + Wikipedia” or “country name + energy + Wikipedia” as search keywords on Google. For countries with renewable energy plans, the planning time is shown on Wikipedia. In some countries, there are several planning time nodes, then we just select the first planned time node as the planned time. For countries that do not have a renewable energy plan, we regard the establishment of their first renewable energy power plant as the planned time. Their first renewable energy power plant may be wind power, hydropower, etc., or any other form of RERs power plant. Data on CO2 emissions comes from International Energy Agency (IEA).
DDD Model
The triple difference model is also called the difference in difference in differences (DDD) model. There are two reasons for the establishment of the DDD model. First, the sample does not meet the common time trend. Energy planning policies can be seen as a prerequisite for randomized trials, thus building a model is needed to evaluate the impact of renewable energy planning policies on renewable energy output. The difference in differences (DID) model and is usually used for the evaluation of policy shocks, but the prerequisite is that the sample must meet a common time trend. We had tried to use DID model but the samples do not conform to common trends in RERs planning because some countries plan earlier while others plan later, and the speed of RERs technology progress is also different. The DID model furthermore failed to pass the placebo test, which determines that the best alternative model to the DID model for policy impact assessment is the DDD model. Second, before using the triple difference model, it is necessary to check whether the time trend is significant. If the significance is not 0, the triple difference model can be used. From the regression analysis results in Table 1, it can be seen that the time trend variable is significantly not zero, which is a reason for utilizing the DDD model.
Based on the DDD method of Fu et al. (2015) and Kim et al. (2015), we build the DDD model in (1) to analyze the impact of planning for R&D on the output and consumption of RERs. There are two treatment groups and one control group in the DDD model.
Considering that the implementation of RERs planning will be affected by GDP, CO2 emissions, research and development expenditure (RDEX) and researchers in R&D (RDRE), these variables need to be entered into the DDD model in order to avoid the endogenous problem. Thus, Eq. (1) changes into Eq. (2).
Variable
The explained variable is OUTPRERs. O’Hagan (2016), Maciel et al. (2018), and Salvador et al. (2019) establish that the final result of R&D planning is the output of RERs. Thus, we take OUTPRERs as the explained variable.
The dummy variables are DevelopingMari, RERsPlan, and DevelopedMari. Because we want to study the R&D planning of developing marine RERs, we regard developing marine countries as the first treatment group. Secondly, marine RERs planning in developed countries has an impact on developing countries, because developed countries will extend the idea of developing marine RERs planning to developing countries, and developing countries will also refer to relevant plans of developed countries. Thus, the developing countries are regarded as the second treatment group. The other countries are the control group. Therefore, we have three dummy variables in Eqs. (3–5), which also act as the explanatory variables. Further, the DDD model derived several new explanatory variables, that is, DevelopingMari × RERsPlan, DevelopingMari × DevelopedMari, RERsPlan × DevelopingMari, and DevelopingMari × RERsPlan × DevelopedMari.
Covariate GDP, PerGDP, and CO2E
There is a long positive correlation or the causal relationship between renewable energy and GDP, GDP per capita (PerGDP) and CO2 emission (CO2E) (Apergis and Payne, 2014; Ohler and Fetters, 2014), thus GDP, PerGDP, and CO2E will have an impact on marine renewable energy and should be included as control variables in the DDD model.
Covariate RDEX and RDRE
R&D spending per GDP is an indicator of renewable energy R&D (Ragwitz and Miola, 2004), which can drive the output of RERs (Adedoyin et al., 2020). By their own strength and expansion of social networks, researchers can promote the output of renewable energy (Kumar et al., 2013). Thus, we take R&D expenses (RDEX) and R&D personnel (RDRE) as control variables in the DDD model. The variables of the DDD models and the descriptive statistics are shown in Table 1.
Result of DDD
We regress both model (1) and model (2) by two methods, Diff and OLS. The regression results are shown in Table 2.
For the regression results of the DDD model, the effectiveness of the policy can only be judged by the significance of the triple difference crossover variable. If the triple difference crossover variable is significant, the policy is effective. The OLS method and Diff command are used to estimate the model (1), and the triple difference variables are not significant, which indicates that the endogenous problem causes the model (1) to not obtain significant results. For the model (2) with covariate added, the regression results of both methods show that triple difference variables DevelopingMari × RERsPlan × DevelopedMari have a significantly positive impact on OUTPRERs at the significance level of 1%. The economic significance of this result is that the RERs plan of developing marine countries to increase the amount and the share of RERs power generation from 1990 to 2016. In other words, the RERs planning policies of developing marine countries have a positive impact on the output of RERs. The rapid development of RERs has its inherent economic mechanism. At the same time, new problems will arise in the development process.
First, Government Economic Policy
The government of each developing marine country has different motivations to promote the use of RERs by economic policy. Their goal may be to discover new economic growth points, or to reduce CO2 emissions in order to meet climate agendas and foster a better environment for the population. It may be that traditional energy deficiency or the awareness raised by environmental science and advocacy, that governments pay attention to aiming for low-carbon environments (Gallagher, 2013). Under the advocacy of the government RERs policy, the installation cost of RERs generator sets is declining, and the operating costs are falling (Nazir et al., 2019), alongside the increasing saturation of solar and wind energy. Under these circumstances, the government has less and less support for RERs (Schaffer and Bernauer, 2014). For example, the Chinese government have canceled financial subsidies for the installation of wind turbines in 2021. This requires the governments of developing marine countries to make breakthroughs for RERs planning, and the opportunity for this breakthrough planning lies in the R&D plans for marine RERs.
Second, the Growth of GDP per Capita
According to the results of the OLS regression in Table 2, per capita GDP has a significant positive impact on RERs output, that is, for every one percentage point increase in per capita GDP, the output of RERs will increase by 0.126 percentage points, which is consistent with the conclusion of Simionescu et al. (2019). However, because Simionescu et al. (2019) uses EU data, the 0.126 percentage point in this article is higher than the 0.009 percentage point of their research, which is 0.117 percentage points.
Third, Carbon Dioxide (CO2) Emissions
CO2 emissions do not affect a single country, but affect the global environment and climate, thus reducing CO2 emissions requires the joint efforts of all countries in the world (Mendonca et al., 2020). Generally speaking, the more CO2 emissions there are, the output of RERs will increase rapidly (Mendonca et al., 2020). From Table 2, we establish that the relationship between RERs output and CO2 emissions is positive, which is consistent with the conclusion of Ikram et al. (2020). For every unit increase in carbon dioxide emissions, the output of renewable energy resources will increase by 83.724 units. In Table 2, GDP does not have a significant impact on the output of RERs. The real GDP growth will increase the output of RERs through the indirect way of increasing carbon emissions (Dogan, 2017).
Fourth, the Support of RERS R&D
The development of basic technology of RERs is a long, uncertain and extensive process (Jacobsson and Johnson, 2000). Therefore, technological innovation of RERs in developing marine countries is mainly imitative innovation or direct introduction of RERs technologies from developed countries. China’s RERS R&D expenditures are mainly government expenditures which bring about few breakthroughs in the basic technology of RERs, and private companies have no incentive to conduct RERS R&D. R&D personnel are mainly in universities and scientific research institutions, who promote the application technology of RERS (Huang et al., 2012). The empirical results of OLS confirmed this mechanism. The results in Table 2 show that R&D expenditure has no significant positive impact on the output of RERs. R&D personnel have a significant positive impact on RERs output. For every percentage point increase in R&D personnel, the output of renewable energy can increase by 2.449 percentage points. If there are R&D funds and R&D personnel who specialize in marine RERS research, the R&D quality of marine RERs can be rapidly improved, and R&D bottlenecks can be quickly broken through. That is, R&D expenditure which brings knowledge accumulation and knowledge spillover, all affect the RERs innovation in every developing marine country, but knowledge spillovers will reduce domestic RERs innovation and increase domestic knowledge reserves of RERs(Miremadi et al., 2019).
Following this, the use of a placebo test is needed to test the robustness of the model. We randomly selected 115 countries as developing marine economies and 116 countries as developed marine economies as the treatment group. The results of the placebo test are shown in Table 2. We judge whether the DDD model can pass the placebo test by the significance of the triple difference crossover variable. If the triple difference crossover variable is not significant, the DDD model passes the placebo test. The triple difference crossover variable DevelopingMari × RERsPlan × DevelopedMari is not significant at 1, 5, and 10 in Table 2, so the DDD model in Table 2 can pass the placebo test.
Discussion
Smart Pig Game Model
Based on hypotheses 1–4, the government’s R&D plan for RERs research and development is essentially a smart pig game between the government and enterprises. Table 3 is the result of the game between the government and enterprises in renewable energy planning. The government acts as a “big pig” and the firms act as “piggy.” In the smart pig game, the government has two strategies, that is, planning and not planning. The probability of planning is ν, and the probability of not planning is 1 − ν. In this case, the government’s planning refers to the R&D planning of key technologies, basic technologies and immature technology for RERs. The firm has two strategies too, and they can choose to carry out R&D or not. The probability of R&D is μ, and the probability of not undertaking R&D is 1 − μ. The meaning of variables and strategies is shown in Table 4.
If the government conducts R&D plans for key technologies, basic technologies and immature technology for the production of RERs, the government will designate some universities, research institutions or firms to conduct R&D on these technologies for RERs through a project tender. R&D costs are afforded by the government, not by enterprises. The government plans for R&D of RERs (instead of enterprises), because the R&D plan of RERs can bring about a green environment, eliminate haze, return fresh air to people’s lives, meet goals for low-carbon green growth, and save petrochemical energy consumption (Hong et al., 2009). Furthermore, R&D can dominate the consumption of RERs, whose effect on consumption is higher than the impact of policy and energy intensity (Wang R. et al., 2020). Additionally, the impact of R&D on RERs consumption is affected by the level of GDP, and the impact will be greater at a high level of GDP per capita (Kocsis and Kiss, 2014). The government may also subsidize firms that research and develop RERs, which can promote the development of RERs (Wu et al., 2020). However, if they are not used for R&D of basic RERs technologies, government subsidies will have a certain crowding-out effect on the R&D of RERs (Yu et al., 2016).
From Table 3, we establish that if the government chooses planning, its expected benefits are as shown in Eq. (6).
If the government chooses not to plan, its expected benefits are as in Eq. (7).
From (6) and (7), we get (8).
From (8), we develop (9).
If we find the first derivative of (9) and assume that the first derivative is 0, we develop (10–13).
From (15), we develop (14).
From (16) and (14), we obtain (15).
From (13–15), we develop (16).
From (20), we suppose η = 1. According (17), (14), and (15),we establish (17).
If (14–17) are established at the same time, △Egov can reach a maximum, and the government chooses the planning of R&D.
Planned Area
From the share, output and consumption of RERs electricity, we can see where planning should take place to avoid bottlenecks in marine developing countries. According to the data of RERs in 203 countries around the world in 2014, sourced from the World Bank, the RERs electricity share of total electricity output can be established (Figure 4). In the World Bank database, some countries have no statistics. We treat missing data as 0. Countries where renewable energy power generation accounts for more than 90% of the national total power generation include Albania, Bhutan, Central African Republic, Congo Dem. Rep., Ethiopia, Iceland, Laos, Lesotho, Namibia, Nepal, Paraguay, and Tajikistan. However, RERs electricity share of total electricity output in countries of the world in 2014 is less than 20% in more than 90 countries around the world, and China’s share is around 22%.
Countries with Access to electricity (% of total population) below 50% include Angola, Benin, Burkina Faso, Burundi, Central African Republic, Chad, Congo, Eritrea, Ethiopia, French Guiana, Gambia The, Guadeloupe, Guinea, Guinea-Bissau, Haiti, Kenya, South Korea (Peoples Republic of), Lesotho, Liberia, Madagascar, Malawi, Martinique, Mauritania, Mozambique, Namibia, Niger, Papua New Guinea, Reunion, Rwanda, Sierra Leone, Solomon Islands, Somalia, Sudan, Tanzania, Togo, Uganda, Vanuatu, Western Sahara, Zambia, and Zimbabwe.
Countries where urban electricity supply accounts for less than 60% of the total population are mainly Angola, Benin, Burkina Faso, Burundi, Central African Republic, Chad, Congo, French Guiana, Guadeloupe, Guinea-Bissau, South Korea (Peoples Republic of), Liberia, Malawi, Martinique, Mozambique, Reunion, Sierra Leone, Somalia, Tanzania,, Uganda, Western, and Sahara.
Countries where the percentage of rural population with electricity is below 50% are mainly Angola, Benin, Bermuda, Botswana, Burkina Faso, Burundi, Cambodia, Cameroon, Central African Republic, Chad, Congo, Djibouti, Eritrea, Ethiopia, French Guiana, Gabon, Gambia, The Guadeloupe, Guinea, Guinea-Bissau, Haiti, Kenya, South Korea (Peoples Republic of), Lesotho, Liberia, Madagascar, Malawi, Martinique, Mauritania, Monaco, Mongolia, Mozambique, Myanmar(Burma), Namibia, Niger, Nigeria, Papua New Guinea, Reunion, Rwanda, Senegal, Sierra Leone, Singapore, Solomon Islands, Somalia, Sudan, Tanzania, Togo, Turks and Caicos Islands, Uganda, Vanuatu, Western Sahara, Zambia, and Zimbabwe, as shown in Figure 5.
According to the conversion where 1TJ(terajoule) = 0.2778 GWh (gigawatt hours), we changed the number of units consumed, as stated by the World Bank in 2014, and compared the output and consumption of renewable energy with a pie chart, as shown in Figure 6. From this, we can intuitively see that the consumption of renewable energy in most countries is higher than the output.
The above data analysis shows that there is huge room for the development of RERs in all countries in the world. The utilization rate of marine RERs is lower, so all marine developing countries have room for the planning of R&D of marine RERs. The potential for the development of marine RERs is huge, because energy giants, like China, have been advocating residents to save electricity during the peak period of power consumption in winter, indicating that electricity supply is far from meeting demand. If there is an abundance of marine RERs power supply, the cost of electricity for enterprises can be reduced, and there would be no need for residents to save electricity. The government would then not have to worry about the exhaustion of fossil energy, and fossil energy conservation and CO2 emission reduction targets can be achieved.
In short, there are its inherent economic mechanism for the increase in marine RERs output. Marine RERs planning and R&D are direct mechanisms that have a positive impact on RERs output. An indirect mechanism for increased output of marine RERs is as follows. Increasing GDP has led to an increase in CO2 emissions, and an increase in per capita GDP has necessitated an environment of higher quality. This urges governments to implement the CO2 emission reduction systems. The key to this implementation is to increase the output of RERs by planning marine RERs R&D.
However, in the process of marine RERs development, some problems will arise. For example, there are not enough and professional RERs R&D expenditures and R&D personnel. Neither the government nor enterprises pay attention to marine RERs R&D, particularly developing marine countries’ governments lack marine RERs R&D planning. The existence of these problems will hinder the increase in marine RERs output.
Conclusion
This study focused on four theoretical hypotheses. Firstly, there is more room to plan R&D of RERs in developing marine countries. Secondly, mature technology provides a technological platform for planning the R&D of RERs. Thirdly, immature technologies provide technical and environmental challenges for planning of R&D of RERs. Fourthly, the previous plan of RERs needs to be continuously updated to increase the content of the marine R&D plan of RERs.
From this, four conclusions were established by using a DDD model and wise pig game model. First, the existing RERs planning of marine developing countries has a positive impact on the output of RERs, which shows that marine developing countries are correct in planning for RERs. Second, CO2 emission, GDP per capita and R&D personnel also have a positive impact on the output of marine RERs, which are variables that affect the output of RERs in addition to RERs planning policies. Third, the difference between the expected return of the government planning for R&D of RERs and the expected return of the government not planning for R&D of RERs is maximized, when the government has a probability of 2/3 for planning the R&D of RERs and the probability of a successful planning is 1/2, while the company conducts R&D of RERs with the probability of 1 − (GDP+2CR&D–gl)/(SC-Cswl) and the probability of R&D successful is any value. The game payment that the government can obtain is the sum of GDP, social welfare (such as from having a green environment) and the cost of social welfare losses (such as the cost of haze, air pollution, the consumption of petrochemical energy, etc.), minus the cost paid for R&D of RERs by the government, which is GDP+SC-CR&D–g1+Cswl. That is, if the government successfully plans for the R&D of RERs, and at the same time the enterprise joins the government’s planned R&D of RERs’ immature technology, the government can obtain the maximum benefits of the R&D plan of RERs’ immature technology. Fourthly, all marine developing countries or their alliances need to plan for R&D of marine RERs. RERs’ R&D planning needs to be carried out on the theoretical basis of this article, and RERs need to seriously consider R&D plans in immature technical fields.
Our most important contribution is to clarify the direct and indirect economic mechanisms for the increase in marine renewable energy output, and to emphasize the importance of marine RERs R&D planning in developing marine countries for which little work has been carried out by academia thus far. Furthermore, this work points out the main technical fields where RERs R&D planning can be carried out. Ultimately, it provides a basis on which further research and investigation can be pursued.
Data Availability Statement
The original contributions presented in the study are included in the article/supplementary material, further inquiries can be directed to the corresponding author/s.
Author Contributions
XO contributed to the idea of the manuscript, gathered and analyzed the data, designed the models, and drafted the article. PF and PY discussed the model and the results. AM revised the manuscript. All authors contributed to the article and approved the submitted version.
Funding
This research was funded by the key scientific research project of the Education Department of the Hunan Province (19A07) and the National Social Science Fund project (12CJY020).
Conflict of Interest
The authors declare that the research was conducted in the absence of any commercial or financial relationships that could be construed as a potential conflict of interest.
Footnotes
- ^ According to 2016 China Statistical Yearbook, total RERs power generation of China is 5638.18 billion kilowatt per hour in 2014. 1284.326 divided 5638.18 equal about 23%.
- ^ Part of the data on the Wikipedia website come from the public, which will engender doubt about the scientific nature of the data in this article. However, in this case it is important to note the Wikipedia also has data from companies and governments which have been specifically used by this article, such as the establishment time of renewable energy companies and the government’s release of renewable energy plans. These data are accurate.
References
Adedoyin, F. F., Bekun, F. V., and Alola, A. A. (2020). Growth impact of transition from non-renewable to renewable energy in the EU: the role of research and development expenditure. Renewable Energy 159, 1139–1145. doi: 10.1016/j.renene.2020.06.015
Aktas, A., and Kircicek, Y. (2020). A novel optimal energy management strategy for offshore wind/marine current/battery/ultracapacitor hybrid renewable energy system. Energy 199:117425. doi: 10.1016/j.energy.2020.117425
Aminifar, F., Arroyo, J. M., Dey, S. S., Khodayar, M., Phan, D. T., and Sun, A. (2019). Optimization techniques in renewable energy system planning, design, operation, and control. IEEE Trans. Sustain. Energy 10, 330–332. doi: 10.1109/tste.2018.2882979
Apergis, N., and Payne, J. E. (2014). The causal dynamics between renewable energy, real GDP, emissions and oil prices: evidence from OECD countries. Appl. Econ. 46, 4519–4525. doi: 10.1080/00036846.2014.964834
Asensio, M., de Quevedo, P. M., Munoz-Delgado, G., and Contreras, J. (2018). Joint Distribution network and renewable energy expansion planning considering demand response and energy storage-part II: numerical results. IEEE Trans. Smart Grid 9, 667–675. doi: 10.1109/tsg.2016.2560341
Bakhtavar, E., Prabatha, T., Karunathilake, H., Sadiq, R., and Hewage, K. (2020). Assessment of renewable energy-based strategies for net-zero energy communities: a planning model using multi-objective goal programming. J. Clean. Prod. 272:122886. doi: 10.1016/j.jclepro.2020.122886
Barnier, B., Domina, A., Gulev, S., Molines, J. M., Maitre, T., and Penduff, T. (2020). Modelling the impact of flow-driven turbine power plants on great wind-driven ocean currents and the assessment of their energy potential. Nat. Energy 5, 240–249. doi: 10.1038/s41560-020-0580-2
Bernardes, F. G., Vieira, D. A. G., Palade, V., and Saldanha, R. R. (2018). Winds of change: how up-to-date forecasting methods could help change brazilian wind energy policy and save billions of US$. Energies 11, 1–23.
Bozchalui, M. C., Ahsan Hashmi, S., Hassen, H., Canizares, C. A., and Bhattacharya, K. (2012). Optimal operation of residential energy hubs in smart grids. IEEE Trans. Smart Grid 3, 1755–1766. doi: 10.1109/tsg.2012.2212032
Cambini, C., Congiu, R., Jamasb, T., Llorca, M., and Soroush, G. (2020). Energy systems integration: implications for public policy. Energy Policy 143:111609. doi: 10.1016/j.enpol.2020.111609
Chen, B., Yang, Y., and Wang, Z. L. (2018). Scavenging wind energy by triboelectric nanogenerators. Adv. Energy Mater. 8:1702649. doi: 10.1002/aenm.201702649
Chen, K. H., Ren, Z. P., Mu, S. J., Sun, T. Q., and Mu, R. P. (2020). Integrating the Delphi survey into scenario planning for China’s renewable energy development strategy towards 2030. Technol. Forecast. Soc. Change 158:120157. doi: 10.1016/j.techfore.2020.120157
Chen, W. M., and Kim, H. (2020). Energy, economic, and social impacts of a clean energy economic policy: fuel cells deployment in Delaware. Energy Policy 144:111617. doi: 10.1016/j.enpol.2020.111617
China Energy News (2020). The Scientific Promotion of Hydro-Light Complementary Power Generation Will Help Promote the Green and Low-Carbon Transformation Of Energy. China Energy News 15th November 2020.
Coughlan, M., Long, M. K., and Doherty, P. (2020). Geological and geotechnical constraints in the Irish Sea for offshore renewable energy. J. Maps 16, 420–431. doi: 10.1080/17445647.2020.1758811
Davies, I. M., Watret, R., and Gubbins, M. (2014). Spatial planning for sustainable marine renewable energy developments in Scotland. Ocean Coast. Manag. 99, 72–81. doi: 10.1016/j.ocecoaman.2014.05.013
De Quevedo, P. M., Munoz-Delgado, G., and Contreras, J. (2019). Impact of electric vehicles on the expansion planning of distribution systems considering renewable energy, storage, and charging stations. IEEE Trans. Smart Grid 10, 794–804. doi: 10.1109/tsg.2017.2752303
Deep, S., Sarkar, A., Ghawat, M., and Rajak, M. K. (2020). Estimation of the wind energy potential for coastal locations in India using the Weibull model. Renewable Energy 161, 319–339. doi: 10.1016/j.renene.2020.07.054
Dogan, E. (2017). CO2 emissions, real GDP, renewable energy and tourism: evidence from panel of the most-visited countries. Statistika Stat. Econ. J. 97, 63–76.
Dolatabadi, A., Jadidbonab, M., and Mohammadi-ivatloo, B. (2019). Short-Term scheduling strategy for wind-based energy hub: a hybrid stochastic/IGDT approach. IEEE Trans. Sustain. Energy 10, 438–448. doi: 10.1109/tste.2017.2788086
Failler, P., Montocchio, C., de Battisti, A. B., Binet, T., Maréchal, J. P., and Thirot, M. (2019). Sustainable financing of marine protected areas: the case of the Martinique regional marine reserve of “Le Prêcheur”. Green Finance 1, 110–129. doi: 10.3934/gf.2019.2.110
Fan, L., Zheng, C. W., Zhou, H. J., Tang, H. C., Zhang, G., Gao, C. Z., et al. (2020). Gust characteristic analysis of wind energy resource in the Western pacific ocean. J. Coast. Res. 99, 404–410. doi: 10.2112/si99-055.1
Fu, M. W., Ye, J. Y., Meng, M. X., and Lei, Z. (2015). The impact of localization rate protection on independent innovation: evidence from China’s wind power manufacturing industry. Econ. Res. 2, 118–131.
Garcia, E., Quiles, E., Correcher, A., and Morant, F. (2018). Sensor buoy system for monitoring renewable marine energy resources. Sensors 18:945. doi: 10.3390/s18040945
Gnatowska, R., and Moryn-Kucharczyk, E. (2019). Current status of wind energy policy in Poland. Renewable Energy 135, 232–237. doi: 10.1016/j.renene.2018.12.015
Gorelick, J., and Walmsley, N. (2020). The greening of municipal infrastructure investments: technical assistance, instruments, and city champions. Green Finance 2, 114–134. doi: 10.3934/gf.2020007
Hackl, R., Hansson, J., Noren, F., Stenberg, O., and Olshammar, M. (2018). Cultivating Ciona intestinalis to counteract marine eutrophication: environmental assessment of a marine biomass based bioenergy and biofertilizer production system. Renewable Energy 124, 103–113. doi: 10.1016/j.renene.2017.07.053
Han, N. H., Wang, X. Y., Chen, S. S., Cheng, L., Liu, H. T., Liu, Z., et al. (2019). “Optimal configuration of energy storage systems in virtual power plants including large-scale distributed wind power,” in Proceedings of the 5th International Conference on Energy Materials and Environment Engineering (ICEMEE), (Bristol: IOP). doi: 10.1016/j.est.2018.07.010
He, M., and Walheer, B. (2020). Technology intensity and ownership in the Chinese manufacturing industry: a labor productivity decomposition approach. Natl. Accounting Rev. 2, 110–137. doi: 10.3934/nar.2020007
Held, A., Ragwitz, M., Del Rio, P., Resch, G., Klessmann, C., Hassel, A., et al. (2019). Do almost mature renewable energy technologies still need dedicated support towards 2030? Econ. Energy Environ. Policy 8, 101–118.
Hemmati, R. (2018). Optimal design and operation of energy storage systems and generators in the network installed with wind turbines considering practical characteristics of storage units as design variable. J. Clean. Prod. 185, 680–693. doi: 10.1016/j.jclepro.2018.03.062
Hong, J. S., Ryu, Y. S., and Kil, B. J. (2009). “Evaluation of new and renewable energy technology R&D investment strategy in Korea,” in Proceedings of PICMET 09 - Technology Management in the Age of Fundamental Change, (Piscataway, NJ: IEEE).
Hu, S. J., Sprintall, J., Guan, C., Hu, D. X., Wang, F., Lu, X., et al. (2020). Observed triple mode of salinity variability in the thermocline of tropical Pacific ocean. J. Geophys. Res. Oceans 125:e2020JC016210.
Huang, C., Su, J., Zhao, X. Y., Sui, J. G., Ru, P., Zhang, H. W., et al. (2012). Government funded renewable energy innovation in China. Energy Policy 51, 121–127. doi: 10.1016/j.enpol.2011.08.069
Hurst, C. (1990). Establishing new markets for mature energy equipment in developing-countries -experience with windmills, hydropowered mills and solar water heaters. World Dev. 18, 605–6159. doi: 10.1016/0305-750x(90)90075-9
Ikram, M., Zhang, Q. Y., Sroufe, R., and Shah, S. Z. A. (2020). Towards a sustainable environment: the nexus between ISO 14001, renewable energy consumption, access to electricity, agriculture and CO2 emissions in SAARC countries. Sustain. Prod. Consump. 22, 218–230. doi: 10.1016/j.spc.2020.03.011
Isaksson, N., Masden, E. A., Williamson, B. J., Costagliola-Ray, M. M., Slingsby, J., Houghton, J. D. R., et al. (2020). Assessing the effects of tidal stream marine renewable energy on seabirds: a conceptual framework. Mar. Pollut. Bull. 157:111314. doi: 10.1016/j.marpolbul.2020.111314
Jacobsson, S., and Johnson, A. (2000). The diffusion of renewable energy technology: an analytical framework and key issues for research. Energy Policy 28, 625–640. doi: 10.1016/s0301-4215(00)00041-0
Ji, W., Zhou, Y., Sun, Y., Zhang, W., An, B. L., and Wang, J. J. (2017). Thermodynamic analysis of a novel hybrid wind-solar-compressed air energy storage system. Energy Convers. Manag. 142, 176–187. doi: 10.1016/j.enconman.2017.02.053
Kazimierczuk, A. H. (2019). Wind energy in Kenya: a status and policy framework review. Renewable Sustain. Energy Rev. 107, 434–445. doi: 10.1016/j.rser.2018.12.061
Kim, S. J., Wang, R. J. H., and Malthouse, E. C. (2015). The effects of adopting and using a brands mobile application on customers subsequent purchase Behavior. J. Interactive Mark. 31, 28–41. doi: 10.1016/j.intmar.2015.05.004
Kocsis, I., and Kiss, J. T. (2014). Renewable energy consumption, R&D and GDP in European Union countries. Environ. Eng. Manag. J. 13, 2825–2830.
Kumar, V., Sagar, R., and Narula, S. A. (2013). “Researcher-guide networking: a case of renewable energy research,” in Proceedings of the 2013 IEEE/ACM International Conference on Advances in Social Networks Analysis and Mining (ASONAM), (New York, NY: ACM).
Kumar, V., Shrivastava, R. L., and Untawale, S. P. (2015). Solar energy: review of potential green & clean energy for coastal and offshore applications. Int. Conf. Water Resour. Coastal Ocean Eng. 4, 473–480. doi: 10.1016/j.aqpro.2015.02.062
Lee, S. K., Chong, H. L. H., Dayou, J., and Saleh, E. (2016). “Experimental assessment of ocean salinity and temperature energy conversion (OSTEC) full-scale prototype,” in Proceedings of the 16th Techno-Ocean Conference (Techno-Ocean), (Piscataway, NJ: IEEE), 16–21.
Lei, Y., Wang, D., Jia, H. J., Chen, J. C., Li, J. R., Song, Y., et al. (2020). Multi-objective stochastic expansion planning based on multi-dimensional correlation scenario generation method for regional integrated energy system integrated renewable energy. Appl. Energy 276:115395. doi: 10.1016/j.apenergy.2020.115395
Li, Y. B., Hong, Y. G., Wu, J. P., Wang, Y., and Ye, F. (2020). Spatial variability pattern of the anaerobic ammonia-oxidizing bacterial community across a salinity gradient from river to ocean. Ecotoxicology doi: 10.1007/s10646-020-02282-5 Online ahead of print.
Li, Z., Liao, G., and Albitar, K. (2020). Does corporate environmental responsibility engagement affect firm value? the mediating role of corporate innovation. Bus. Strategy Environ. 29, 1045–1055. doi: 10.1002/bse.2416
Li, Z., Liao, G., Wang, Z., and Huang, Z. (2018). Green loan and subsidy for promoting clean production innovation. J. Clean. Prod. 187, 421–431. doi: 10.1016/j.jclepro.2018.03.066
Liao, G., and Drakeford, B. (2019). An analysis of financial support, technological progress and energy efficiency: evidence from China. Green Finance 1, 174–187. doi: 10.3934/gf.2019.2.174
Liu, F., Sun, F. B., Liu, W. W., Wang, T. T., Wang, H., Wang, X. M., et al. (2019). On wind speed pattern and energy potential in China. Appl. Energy 236, 867–876. doi: 10.1016/j.apenergy.2018.12.056
Liu, Y. X., Luo, G. L., and Zhuang, Y. (2015). The status and forecast of China’s exploitation of renewable marine energy resources. J. Coastal Res. 73, 193–196. doi: 10.2112/si73-034.1
Liu, Y. Z., Jing, Z., and Wu, L. X. (2019). Wind power on oceanic near-inertial oscillations in the global ocean estimated from surface drifters. Geophys. Res. Lett. 46, 2647–2653. doi: 10.1029/2018gl081712
Maciel, P. N., Alcocer, J. C. A., Pinto, O. R. D., and Dolibaina, L. I. D. (2018). Sustainable energy public policies planning: encouraging the production and use of renewable energies. Recista eletronica em gestao educacao e tecnologia Ambiental 22, 01–15.
Madvar, M. D., Ahmadi, F., Shirmohammadi, R., and Aslani, A. (2019). Forecasting of wind energy technology domains based on the technology life cycle approach. Energy Rep. 5, 1236–1248. doi: 10.1016/j.egyr.2019.08.069
Marugan, A. P., Marquez, F. P. G., Perez, J. M. P., and Ruiz-Hernandez, D. (2018). A survey of artificial neural network in wind energy systems. Appl. Energy 228, 1822–1836. doi: 10.1016/j.apenergy.2018.07.084
Matei, I. (2020). Is financial development good for economic growth? Empirical insights from emerging European countries. Quantitative Finance Economics 4, 653–678. doi: 10.3934/qfe.2020030
McWilliams, J. C. (2016). Submesoscale currents in the ocean. Proc. R. Soc. A Math. Phys. Eng. Sci. 472:20160117. doi: 10.1098/rspa.2016.0117
Mehrjerdi, H., and Hemmati, R. (2020). Stochastic model for electric vehicle charging station integrated with wind energy. Sustain. Energy Technol. Assess. 37:100577. doi: 10.1016/j.seta.2019.100577
Mejia-Olivares, C. J., Haigh, I. D., Lewis, M. J., and Neill, S. P. (2020). Sensitivity assessment of bathymetry and choice of tidal constituents on tidal-stream energy resource characterisation in the Gulf of California, Mexico. Appl. Ocean Res. 102:102281. doi: 10.1016/j.apor.2020.102281
Mendonca, A. K. D., Barni, G. D. C., Moro, M. F., Bornia, A. C., Kupek, E., and Fernandes, L. (2020). Hierarchical modeling of the 50 largest economies to verify the impact of GDP, population and renewable energy generation in CO2 emissions. Sustain. Prod. Consump. 22, 58–67. doi: 10.1016/j.spc.2020.02.001
Miremadi, I., Saboohi, Y., and Arasti, M. (2019). The influence of public R&D and knowledge spillovers on the development of renewable energy sources: the case of the Nordic countries. Techol. Forecast. Soc. Change 146, 450–463.
Nadaleti, W. C., dos Santos, G. B., and Lourenco, V. A. (2020). Integration of renewable energies using the surplus capacity of wind farms to generate H-2 and electricity in Brazil and in the Rio Grande do Sul state: energy planning and avoided emissions within a circular economy. Int. J. Hydrogen Energy 46, 24190–24202. doi: 10.1016/j.ijhydene.2020.06.226
Nazir, M. S., Mahdi, A. J., Bilal, M., Sohail, H. M., Ali, N., and Iqbal, H. M. N. (2019). Environmental impact and pollution-related challenges of renewable wind energy paradigm - a review. Sci. Total Environ. 683, 436–444. doi: 10.1016/j.scitotenv.2019.05.274
Odam, N., and de Vries, F. P. (2020). Innovation modelling and multi-factor learning in wind energy technology. Energy Economics 8:104594. doi: 10.1016/j.eneco.2019.104594
O’Hagan, A. M. (2016). Maritime spatial planning and marine renewable energy. Plan. Lanning Theory Prac. 17, 148–151.
Ohler, A., and Fetters, I. (2014). The causal relationship between renewable electricity generation and GDP growth: a study of energy sources. Energy Econ. 43, 125–139. doi: 10.1016/j.eneco.2014.02.009
Okwanya, I., Alhassan, A., Migap, J. P., and Sunday, S. A. (2020). Evaluating renewable energy choices among rural communities in Nigeria. an insight for energy policy. Int. J. Energy Sector Manag. 9, 157–172. doi: 10.1108/ijesm-12-2019-0001
Oliveira-Pinto, S., Rosa-Santos, P., and Taveira-Pinto, F. (2020). Assessment of the potential of combining wave and solar energy resources to power supply worldwide offshore oil and gas platforms. Energy Convers. Manag. 223:113299. doi: 10.1016/j.enconman.2020.113299
Osorio, J. D., Panwar, M., Rivera-Alvarez, A., Chryssostomidis, C., Hovsapian, R., Mohanpurkar, M., et al. (2020). Enabling thermal efficiency improvement and waste heat recovery using liquid air harnessed from offshore renewable energy sources. Appl. Energry 275:115351. doi: 10.1016/j.apenergy.2020.115351
Pazouki, S., Haghifam, M. R., and Moser, A. (2014). Uncertainty modeling in optimal operation of energy hub in presence of wind, storage and demand response. Int. J. Electrical Power Energy Syst. 61, 335–345. doi: 10.1016/j.ijepes.2014.03.038
Quesada, M. C. C., Lafuente, J. G., Garrido, J. C. S., Sammartino, S., and Delgado, J. (2014). Energy of marine currents in the Strait of Gibraltar and a renewable energy resource its potential as a renewable energy resource. Renewable Sustain. Energy Rev. 34, 98–109. doi: 10.1016/j.rser.2014.02.038
Ragwitz, M., and Miola, A. (2004). Evidence from RD&D spending for renewable energy sources in the EU. Renewable Energy 30, 1635–1647. doi: 10.1016/j.renene.2004.12.001
Raoux, A., Pezy, J. P., Ernande, B., Niquil, N., Dauvin, J. C., and Grangere, K. (2020). Isotopic analyses, a good tool to validate models in the context of Marine Renewable energy development and cumulative impacts. Estuar. Coastal Shelf Sci. 273:106690. doi: 10.1016/j.ecss.2020.106690
Rastrick, S. P. S., and Whiteley, N. M. (2020). Influence of natural thermal gradients on whole animal rates of protein synthesis in marine gammarid amphipods. PLoS One 8:e60050. doi: 10.1371/journal.pone.0060050
Ratinen, M. (2019). Social embeddedness of policy actors. The failure of consumer-owned wind energy in Finland. Energy Policy 128, 735–743. doi: 10.1016/j.enpol.2019.01.004
Ravetti, C., Theoduloz, T., and Valacchi, G. (2020). Buy coal or kick-start green innovation? energy policies in an open economy. Environ. Resour. Econ. 77, 95–126. doi: 10.1007/s10640-020-00455-8
Salvador, S., Gimeno, L., and Larruga, F. J. S. (2019). The influence of maritime spatial planning on the development of marine renewable energies in Portugal and Spain: legal challenges and opportunities. Energy Policy 128, 316–328. doi: 10.1016/j.enpol.2018.12.066
Schaffer, L. M., and Bernauer, T. (2014). Explaining government choices for promoting renewable energy. Energy Policy 68, 15–27. doi: 10.1016/j.enpol.2013.12.064
Simionescu, M., Bilan, Y., Krajnakova, E., Streimikiene, D., and Gedek, S. (2019). Renewable energy in the electricity sector and GDP per Capita in the European Union. Energies 12:2520. doi: 10.3390/en12132520
Sogut, D. V., Farhadzadeh, A., and Jensen, R. E. (2018). Characterizing the great lakes marine renewable energy resources: lake michigan surge and wave characteristics. Energy 150, 781–796. doi: 10.1016/j.energy.2018.03.031
Sogut, D. V., Jensen, R. E., and Farhadzadeh, A. (2019). Characterizing lake ontario marine renewable energy resources. Mar. Technol. Soc. J. 53, 21–27. doi: 10.4031/mtsj.53.2.3
Sollich, M., Yoshinaga, M. Y., Hausler, S., Price, R. E., Hinrichs, K. U., and Buhring, S. I. (2020). Heat stress dictates microbial lipid composition along a thermal gradient in marine sediments. Front. Microbiol. 8:1550.
Straatman, P. J. T., and Van Sark, W. G. (2008). A new hybrid ocean thermal energy conversion - offshore solar pond (OTEC-OSP) design: a cost optimization approach. Solar Energy 82, 520–527. doi: 10.1016/j.solener.2007.12.002
Su, D., Li, K. C., and Shi, N. (2020). Research on renewable energy planning considering the flexible region of the microgrid. Appl. Sci. Basel 10:7544. doi: 10.3390/app10217544
Sukharev, O. S. (2020). Structural analysis of income and risk dynamics in models of economic growth. Quantitative Finance and Economics 4, 1–18.
Supply, A., Boutin, J., Vergely, J. L., Kolodziejczyk, N., Reverdin, G., Reul, N., et al. (2020). Insights into SMOS sea surface salinity new retrievals in the Arctic Ocean. Remote Sens. Environ. 249:112027. doi: 10.1016/j.rse.2020.112027
Taveira-Pinto, F., Rosa-Santos, P., and Fazeres-Ferradosa, T. (2020). Marine renewable energy. Renewable Energy 150, 1160–1164. doi: 10.1016/j.renene.2019.10.014
Thomas, A., and Racherla, P. (2020). Constructing statutory energy goal compliant wind and solar PV infrastructure pathways. Renewable Energy 161, 1–19. doi: 10.1016/j.renene.2020.06.141
Toumi, S., Elbouchikhi, E., Benbouzid, M., and Amirat, Y. (2020). Grid fault-resilient control of a PMSG-based tidal stream turbine. J. Electrical Syst. 16, 429–447.
Varlas, G., Christakos, K., Cheliotis, I., Papadopoulos, A., and Steeneveld, G. J. (2017). Spatiotemporal variability of marine renewable energy resources in Norway. Energy Procedia 125, 180–189. doi: 10.1016/j.egypro.2017.08.171
Vinagre, P. A., Simas, T., Cruz, E., Pinori, E., and Svenson, J. (2020). Marine biofouling: a european database for the marine renewable energy sector. J. Mar. Sci. Eng. 8:495. doi: 10.3390/jmse8070495
Walsh, J., Bashir, I., Garrett, J. K., Thies, P. R., Blondel, P., and Johanning, L. (2017). Monitoring the condition of marine renewable energy devices through underwater acoustic emissions: case study of a wave energy converter in Falmouth Bay, UK. Renewable Energy 102, 205–213. doi: 10.1016/j.renene.2016.10.049
Wang, Q., Li, S. Y., and Pisarenko, Z. (2020). Heterogeneous effects of energy efficiency, oil price, environmental pressure, R&D investment, and policy on renewable energy - evidence from the G20 countries. Energy 209:118322. doi: 10.1016/j.energy.2020.118322
Wang, R., Hsu, S. C., Zheng, S. N., Chen, J. H., and Li, X. R. I. (2020). Renewable energy microgrids: economic evaluation and decision making for government policies to contribute to affordable and clean energy. Appl. Energy 274:115287. doi: 10.1016/j.apenergy.2020.115287
Wendling, Z. A., Warren, D. C., Rubin, B. M., Carley, S., and Richards, K. R. (2020). A scalable energy-economy model for state-level policy analysis applied to a demand-side management program. Econ. Dev. Q. 34, 372–386. doi: 10.1177/0891242420937792
White, C., Selkoe, K. A., Watson, J., Siegel, D. A., Zacherl, D. C., and Toonen, R. J. (2010). Ocean currents help explain population genetic structure. Proc. R. Soc. B Biol. Sci. 277, 1685–1694. doi: 10.1098/rspb.2009.2214
Wu, T., Yang, S. W., and Tan, J. J. (2020). Impacts of government R&D subsidies on venture capital and renewable energy investment - an empirical study in China. Resour. Policy 68:101715.
Yang, D. F., Jiang, C., Cai, G. W., Yang, D. Y., and Liu, X. J. (2020). Interval method based optimal planning of multi-energy microgrid with uncertain renewable generation and demand. Appl. Energy 277:115491. doi: 10.1016/j.apenergy.2020.115491
Yang, W. D., Wang, J. Z., Lu, H. Y., Niu, T., and Du, P. (2019). Hybrid wind energy forecasting and analysis system based on divide and conquer scheme: a case study in China. J. Clean. Prod. 222, 942–959. doi: 10.1016/j.jclepro.2019.03.036
Yu, F. F., Guo, Y., Le-Nguyen, K., Barnes, S. J., and Zhang, W. T. (2016). The impact of government subsidies and enterprises’ R&D investment: a panel data study from renewable energy in China. Energy Policy 89, 106–113.
Zendehboudi, A., Baseer, M. A., and Saidur, R. (2018). Application of support vector machine models for forecasting solar and wind energy resources: a review. J. Clean. Prod. 199, 272–285. doi: 10.1016/j.jclepro.2018.07.164
Zhang, C. M., Cheng, H. Z., Liu, L., Zhang, H., Zhang, X. H., and Li, G. (2020). Coordination planning of wind farm, energy storage and transmission network with high-penetration renewable energy. Int. J. Electrical Power Energy Syst. 120:105944. doi: 10.1016/j.ijepes.2020.105944
Zhang, J. J., and Guan, J. C. (2019). The impact of competition strength and density on performance: the technological competition networks in the wind energy industry. Ind. Mark. Manag. 82, 213–225. doi: 10.1016/j.indmarman.2019.01.011
Zhang, W. P., Maleki, A., Rosen, M. A., and Liu, J. Q. (2019). Sizing a stand-alone solar-wind-hydrogen energy system using weather forecasting and a hybrid search optimization algorithm. Energy Convers. Manag. 180, 609–621. doi: 10.1016/j.enconman.2018.08.102
Zhang, X. P., Shahidehpour, M., Alabdulwahab, A., and Abusorrah, A. (2015). Optimal expansion planning of energy hub with multiple energy infrastructures. IEEE Trans. Smart Grid 6, 2302–2311. doi: 10.1109/tsg.2015.2390640
Keywords: marine renewable energy resources, avoiding bottlenecks, developing marine economies, planning R&D, difference in difference in difference
Citation: Ou X, Ye P, Failler P and March A (2021) Planning the R&D of Marine Renewable Energy Resources: Avoiding Bottlenecks and Ensuring Sustainable Development in Developing Marine Economies. Front. Environ. Sci. 9:654566. doi: 10.3389/fenvs.2021.654566
Received: 16 January 2021; Accepted: 02 March 2021;
Published: 25 March 2021.
Edited by:
Qiang Ji, Chinese Academy of Sciences, ChinaReviewed by:
Bin Mo, Guangzhou University, ChinaAbbi Kedir, The University of Sheffield, United Kingdom
Copyright © 2021 Ou, Ye, Failler and March. This is an open-access article distributed under the terms of the Creative Commons Attribution License (CC BY). The use, distribution or reproduction in other forums is permitted, provided the original author(s) and the copyright owner(s) are credited and that the original publication in this journal is cited, in accordance with accepted academic practice. No use, distribution or reproduction is permitted which does not comply with these terms.
*Correspondence: Xueyin Ou, 1277279006@qq.com