- 1Leverhulme Centre for Wildfires, Environment and Society, Department of Life Sciences, Imperial College London, London, United Kingdom
- 2Department of Geography and Environmental Science, University of Reading, Reading, United Kingdom
- 3Centre for Environmental Policy, Imperial College London, London, United Kingdom
- 4Department of Geography, King’s College London, London, United Kingdom
- 5Department of Geography, Royal Holloway University of London, London, United Kingdom
- 6Division of Ecosystem-Atmosphere Interactions, KIT/IMK-IFU, Garmisch-Partenkirchen, Germany
- 7Department of Environmental Sciences, Rutgers University, New Brunswick, NJ, United States
- 8Department of Mechanical Engineering, Imperial College London, London, United Kingdom
- 9Department of Geography and Environment, London School of Economics and Political Science, London, United Kingdom
Although it has long been recognised that human activities affect fire regimes, the interactions between humans and fire are complex, imperfectly understood, constantly evolving, and lacking any kind of integrative global framework. Many different approaches are used to study human-fire interactions, but in general they have arisen in different disciplinary contexts to address highly specific questions. Models of human-fire interactions range from conceptual local models to numerical global models. However, given that each type of model is highly selective about which aspects of human-fire interactions to include, the insights gained from these models are often limited and contradictory, which can make them a poor basis for developing fire-related policy and management practices. Here, we first review different approaches to modelling human-fire interactions and then discuss ways in which these different approaches could be synthesised to provide a more holistic approach to understanding human-fire interactions. We argue that the theory underpinning many types of models was developed using only limited amounts of data and that, in an increasingly data-rich world, it is important to re-examine model assumptions in a more systematic way. All of the models are designed to have practical outcomes but are necessarily simplifications of reality and as a result of differences in focus, scale and complexity, frequently yield radically different assessments of what might happen. We argue that it should be possible to combine the strengths and benefits of different types of model through enchaining the different models, for example from global down to local scales or vice versa. There are also opportunities for explicit coupling of different kinds of model, for example including agent-based representation of human actions in a global fire model. Finally, we stress the need for co-production of models to ensure that the resulting products serve the widest possible community.
Introduction
Naturally occurring landscape fires, or wildfires, have been an integral component of the Earth System for 350–400 million years, since the development of vegetation on land (Scott, 2000; Bowman et al., 2009). Humans have used fire for domestic purposes for about one million years (e.g., Goren-Inbar et al., 2004; Karkanas et al., 2007; Berna et al., 2012) and fire has been used as a management tool to facilitate land clearance and pasture improvement at least since the Neolithic (Piperno, 1994; Arroyo-Kalin, 2012). Fire continues to be used today in subsistence activities and for maintaining cultural identity, where traditional fire knowledge governs burning (e.g., Mistry et al., 2005; Eriksen, 2007). It has been estimated that about 280 million hectares of land, mostly in the tropics and subtropics, are used for swidden agriculture (Heinimann et al., 2017) — much of which is facilitated by the use of fire for land clearance.
Fire is the most important cause of natural disturbance (Pausas et al., 2017), influencing vegetation patterns and biogeochemical cycles (Scott, 2000) and promoting biodiversity in fire-prone ecosystems (He et al., 2019). Fires also provide important ecosystem services, including helping to regulate the occurrence of catastrophic fires (Pausas and Keeley, 2019). However, alongside these benefits, there are considerable negative impacts from wildfires on human safety and health (e.g., Johnston et al., 2012; Liu et al., 2015; Yu et al., 2020), economic costs from fire management, disaster relief, property and forestry damage, tourism loss and health costs (Butry et al., 2001), severe impacts on forest recovery (Stevens-Rumann et al., 2018) and especially in ecosystems that are not well adapted to fire (Whitman et al., 2019; Kelly et al., 2020), and significant feedbacks from fires to climate (Liu et al., 2014; Harrison et al., 2018; Walker et al., 2019). Human-induced deforestation fires in tropical fire-resistant biomes also have noticeable and largely negative effects on biodiversity, human health and climate (e.g., Van der Werf et al., 2009; Reddington et al., 2014; Spracklen and Garcia-Carreras, 2015; Crippa et al., 2016; Exbrayat et al., 2017; Ellwanger et al., 2020), although this is most characteristic of the deforestation fires that are used to promote more intensive land-use practices rather than facilitating swidden agriculture (Murdiyarso and Adiningsih, 2007).
The frequency and severity of wildfires are heterogenous over space and time, influenced by interactions between climatic conditions, ignitions and available fuel (Moritz et al., 2005; Krawchuck et al., 2009; Harrison et al., 2010). It is becoming increasing evident that anthropogenic climate warming promotes the conditions for wildfire, through extending the periods of fire weather, which occurs through a combination of high temperatures, low humidity and rainfall, and often high winds (Jolly et al., 2015; Jones et al., 2020). Future climate projections indicate that there will be an increase in the likelihood of fire weather and this has been seen as another motivation for political action to limit climate change to below 2°C (e.g., Burton et al., 2018; Turco et al., 2018). However, despite the increased prevalence of fire weather, satellite datasets show a decrease in burned area in recent years (Van Lierop et al., 2015; Doerr and Santín, 2016; Andela et al., 2017; Lizundia-Loiola et al., 2020a; Lizundia-Loiola et al., 2020b). This trend is not statistically significant at the global scale (Forkel et al., 2019a) but is important in certain regions, most noticeably in sub-Saharan Africa. The causes of this decline remain uncertain: Andela et al. (2017) argued that the decline was a reflection of human-induced land-use changes but more recent analyses suggest that changes in climate and natural vegetation cover also play a role, leading to both increased and decreased fire, and can offset the carbon losses from land-use change at a regional scale (Forkel et al., 2019a). Whatever the cause of the recent decline in burnt area, the implications for environmental policy and fire management are far different from those that would emerge from a consideration of fire weather trends alone.
Predictions of the future trajectory of wildfires are required in order to predict the consequences of these changes for the Earth System (e.g., Kloster et al., 2010; Kloster and Lasslop, 2017), and these models must necessarily include some consideration of the role of anthropogenic fires and the complex role of humans in fire management (Lavorel et al., 2007; Knorr et al., 2016; Rabin et al., 2017). Projections are also required of how changes in wildfires might impact human activities and, in turn, how human activities might be modified in the light of these impacts and how political, economic, social or cultural factors might affect these responses. Models of human-fire relationships can also illuminate our understanding of the potential for human fire practices to have positive ecological outcomes, and of the role that fire plays in sustaining cultures and livelihoods.
Many different types of models have been employed to examine human-fire interactions, ranging from informal or conceptual models to formal or mathematical models (Edmonds, 2018), on scales ranging from local to global. These models have generally been developed and deployed to answer specific questions, and thus are rooted within disciplinary perspectives and understanding. Despite the recognition that integration of these different perspectives and approaches would be beneficial, and promote a better understanding of pyrogeography (Bowman et al., 2009), little progress has been made towards such an integration. This is a serious concern because, as the differences in the trends in fire weather versus burnt area amply illustrate, consideration of limited aspects of the climate-fire-human nexus could lead to radically different approaches to fire management and the development of policies for adaptation/mitigation actions. As in many areas of global change science, policy and management choices will need to reflect trade-offs between costs and benefits and this will require integrating the different perspectives gained by deploying multiple kinds of models.
In this paper, we address the following questions: i) what kinds of models are currently being used to address human-fire interactions? ii) what can we learn from each of the different kinds of models?; and iii) can we reconcile the different modelling perspectives and build more comprehensive fire-system models? To answer these questions, we first describe a number of different types of models that have been used to describe the interactions between humans and fire, encompassing both formal and informal models that operate at different spatial scales and emerge from different disciplinary or social perspectives. We describe what they are designed to do, their key characteristics, the assumptions that underpin them, how the models are currently implemented and their data requirements, and explicitly examine their current uncertainties and simplifications. We then explore commonalities between these models, if and how different approaches can be reconciled or integrated, and if and when it is helpful to do so. Finally, we outline the major challenges and provide a basic roadmap for integrating insights from different types and scales of models. Through doing so, we propose a way forward for improving our understanding of human-fire interactions, providing a more solid foundation for predicting future fire regimes and a more comprehensive basis for building fire management plans and policies.
Approaches to Modelling Fire
Interest in human-fire relationships spans many disciplines, each asking very different types of questions, grounded in different philosophical approaches and drawing on a different knowledge base. The tools used to address these questions, and specifically the informal or formal models developed within different research domains, can therefore be expected to be different from one another. Here, as a basis for exploring commonalities across models, we describe some of the types of models that are currently being used to examine human-fire interactions. These models differ in the spatial and temporal scale at which they operate, and the resolution and complexity that bio-physical processes and humans are represented (Figure 1). We structure this discussion moving from the most people-centric models (place-based models of fire knowledge, agent-based models, economic models), which are differentiated to some extent by the spatial scale at which they are developed and used, to the physics-centric models that incorporate human-fire interactions to some extent (wildfire spread models, global fire models). In the last subsection, we look at how policies are developed and implemented in the context of modelling human-fire interactions.
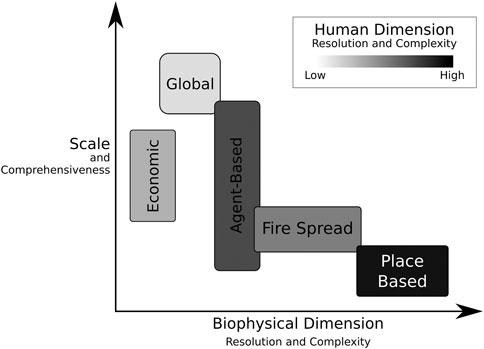
FIGURE 1. Graphical illustration of the relationships between scale (spatial and temporal) and comprehensiveness (number of components in a model), with bio-physical resolution and complexity, and with the resolution and complexity of the representation of humans and human activities, for different fire models.
People-Centric Models
Place-Based Models of Fire Knowledge
Place-based models of fire knowledge represent the relationships between people, fire and the landscape in a local context in verbal, visual, written, or numerical form. Humans may be represented in such models as i) users of fire for specific subsistence activities such as farming or pastoralism, ii) fire managers, controlling fire in the landscape, often within non-governmental or governmental institutions, or iii) holistic fire users, embedded within social-ecological systems in which fire is understood to have agency. Place-based models of fire knowledge can be used to understand historical and current patterns of fire use, the status of fire knowledge, the influence of social, environmental, economic and political factors on fire use, fire cosmologies and fire governance.
The methodologies by which place-based models of fire knowledge are constructed and documented vary in the extent of involvement by local people in the research process. At one end of the spectrum, there are models constructed by researchers without involving local people, where the human influence on fire regimes is inferred from collated datasets. For example, Van Wilgen et al. (2004) combined a 45 years spatial dataset of fire occurrence in the Kruger National Park with climate data and information about management policies in different time periods to model the fire-return time and variability resulting from different management approaches. Models may be constructed by researchers using data about local people through ethnographic observation, interviews, questionnaires or participatory research methodologies. For example, Sorrensen (2004) used data from interviews with swidden farmers in the Brazilian Amazon to develop a model linking the calendar week when farmers chose to burn an area to the length of the preceding fallow period. Where local people are involved, the research process attempts to elicit the mental models that fire users and other stakeholders have of their external reality and their place within it (Jones et al., 2011). Models co-produced by researchers with local people may involve amalgamation of models constructed by individuals or involve a group modelling process.
Modelling processes also vary greatly in the extent to which they are structured around model production and the particular form of model envisaged. To model fire use in Bolivia, for example, Devisscher et al. (2016) followed a structured process of fuzzy cognitive mapping, involving focus group discussions to identify model variables and quantify the strength of relationships between them. Conceptual models may emerge post-hoc, drawing upon data from several sources. Monzón-Alvarado et al. (2014), for example, used insights from research interviews and participatory mapping with swidden farmers in Mexico to develop a qualitative model of the cascading effects of early rains on agricultural burn outcomes across ecological, economic and cultural domains.
There are many participatory modelling techniques that explicitly aim to co-produce a model with local people, many of which produce qualitative models (Voinov et al., 2018). A number of these techniques have been used to develop place-based models of fire knowledge. Seasonal calendars, for example, have been constructed to understand different fire practices in the context of annual ecological and social cycles (e.g., Rodríguez et al., 2011; McKemey et al., 2020). Rich pictures, artistic impressions that can include pictures, text, and symbols representing particular situations or issues from the viewpoint(s) of the person or people who create them (Bell et al., 2016), have been used to develop shared understanding of fire management between Indigenous community members and representatives of governmental and non-governmental organisations (Bilbao et al., 2019, Figure 2). Causal loop diagrams, that depict causal or correlative relationships between different variables in a system (Lane, 2000), have been used to model responses of swidden agriculture to climate and social change in Panama (Li and Ford, 2019) and the role of fire use in land cover change in Indonesia (Medrilzam et al., 2014). Quantitative or semi-quantitative approaches have also been used to co-produce models. In fuzzy cognitive mapping, participants construct a diagram showing the direction and strength of relationships between variables, where the variables are defined by the participants (Özesmi and Özesmi, 2004). This methodology has been used, for example, to model fire use and wildfire risk in Bolivia (Devisscher et al., 2019). Constructing Bayesian belief networks, participants relate elements with discrete possible states to one another in a hierarchy, such that the state of elements higher up the hierarchy probabilistically influences the state of those lower down (Düspohl et al., 2012). This approach has been used to understand adaptive fire management in conservation areas in Australia (Smith et al., 2007). Different methodologies are appropriate in different social contexts. Barber and Jackson (2015), for example, argue that, to Aboriginal Australians, visual and relational modelling approaches make better intuitive sense than quantitative approaches, because these societies have both strong traditions of using artwork to represent relationships between people and the wider cosmological landscape and also keep track of elaborate kinship systems.
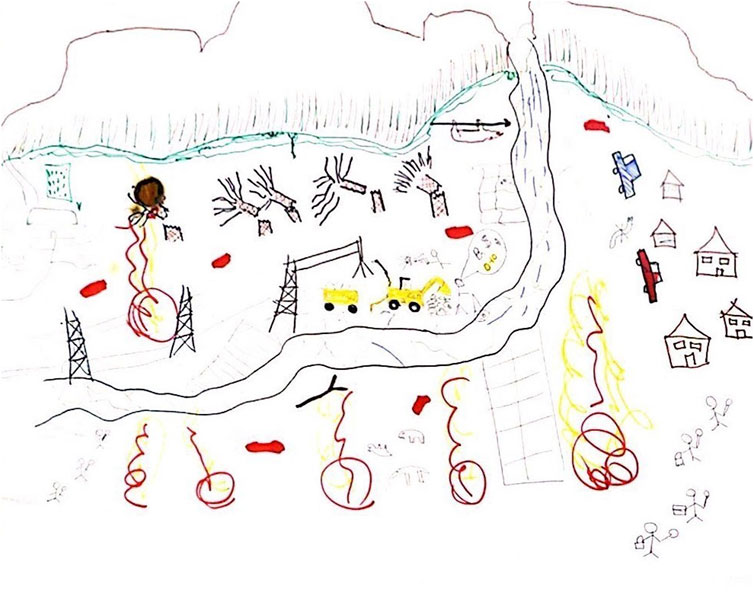
FIGURE 2. Rich picture exploring fire management in the Canaima National Park, Venezuela, made by Indigenous participants of a climate change workshop in 2017 (Source: Jay Mistry).
Some forms of local knowledge, and some socio-ecological entities, relationships and processes, cannot be represented using participatory research frameworks. The biophysical elements of socio-ecological systems are generally more easily represented than socially-constructed elements (Crane, 2010; Jones et al., 2011). Models may also not account for the multiple ways in which people understand causality simultaneously: offered explanations for using fire might be proximate, functional, ontogenetic, evolutionary, or all four simultaneously (see Bliege et al. commentary in Scherjon et al., 2015, p. 315). Participatory modelling processes are affected by the power relations inherent in any local context, and between researchers and local people (Cooke and Kothari, 2001). Trust is important, particularly in cases where fire use has been delegitimised by the state and local fire users may therefore be wary of discussing the issue. The generalisation and simplification involved in model creation may impede consensus when people in a community have different understandings and practices. It is important to understand whether participating individuals have the right to share collective knowledge or speak on behalf of a group (Davis and Wagner, 2003).
Constructing place-based models of fire knowledge can benefit local fire users. While modelling codifies what is already known, it can also be a creative process of knowledge making (Barber and Jackson, 2015). Workshops to elicit understandings of fire and socio-ecological change among the Pemón in Venezuela, for example, exposed conflicting perspectives among elders and young people and allowed communities to develop new shared understandings (Rodríguez et al., 2018). Formalising knowledge and practices in model form can also give local people credibility and visibility. In the case of the Pemón, articulation of local knowledge in this way promoted dialogue with resource managers, shifting official narratives away from fire suppression towards management (Bilbao et al., 2019). However, there is considerable debate about whether rules derived from locally-specific knowledge can be applied in other places or at broader scales. When rules derived from Aboriginal fire knowledge were applied to institutional fire management in Australia, for example, they were criticised as prescriptive and ecologically detrimental (Wohling, 2009). Using place-based models of fire knowledge outside of their context also carries ethical implications because local people may not understand, and may therefore not be able to give informed consent to, the way their knowledge, in model form, is used.
Agent-Based Models
Agent-based models (ABMs) belong to a class of computational models that represent individual, heterogeneous, and often interacting, entities using sets of computational rules or relationships (e.g., Grimm et al., 2005; Bithell et al., 2008). The individual entities or agents in an ABM represent individual humans or groups of humans (i.e., institutions) and their activities in the social and physical worlds (Figure 3). Agents have the ability to make decisions about future actions based on their state, the state of other agents, and/or the state of the simulated environment (O’Sullivan et al., 2012). Decision-making is represented using explicit rules or decision-trees. Actions that result from specific decisions may modify the state of the decision-making agent, other agents, or the environment.
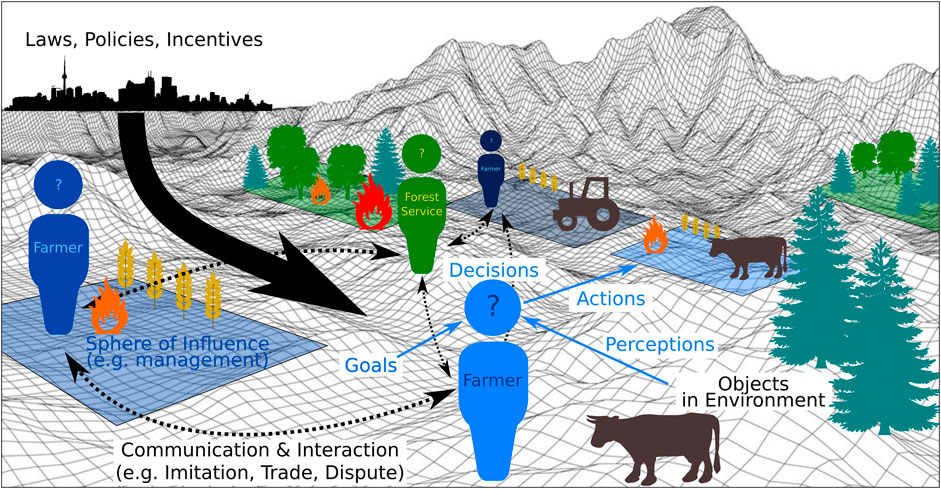
FIGURE 3. Elements of a possible agent-based model at the landscape-scale. Agents make decisions about future actions based on their state (including their goals and available resources), their interactions with other agents, constraints or incentives due to laws, policies and markets. and/or their perceived state of the simulated environment. As in the real world, agents often have a limited sphere of influence over the environment (e.g., which they own and/or manage).
ABMs provide considerable flexibility in the representation of decision making. For example, they can represent decision-making i) with multiple alternative rules or strategies; ii) that are imperfectly rational, for example because of incomplete availability of information; iii) that pursue non-economically advantageous goals, for example because of cultural norms; or iv) are adaptive through time, simulating learning processes. Furthermore, different decision-making rules can be used for different members of a heterogeneous agent population. This flexibility has promoted the use of ABMs in studies of socio-ecological systems, in which interactions between agents and individual and environmental heterogeneity are understood to be essential features (Levin et al., 2013). For example, ABMs have been used widely to examine land use and cover (e.g., Parker et al., 2003; Groeneveld et al., 2017), with specific consideration of land management systems including agriculture, (e.g., Huber et al., 2018), rangelands (Walker and Janssen, 2002) and forests (e.g., Rammer and Seidl, 2015). Most of these studies have been conducted at the human landscape-scale (i.e., 100–10,000 km2), and combine qualitative and quantitative data to structure and parameterise the models. Brändle et al. (2015), for example, examined processes of land abandonment and re-forestation in the Visp area of the Central Valais of Switzerland, developing an agent decision-making typology via a farm household survey in conjunction with the national agricultural census.
In the context of fire, an ABM offers the potential to represent the decision-making in anthropogenic fire use and management as a process explicitly. However, so far, they have only been deployed to investigate the management and use of fire in land systems at a landscape-scale in the global north (e.g., Spain, United States; Wainwright and Millington, 2010; Hulse et al., 2016; Spies et al., 2017). Although these studies demonstrate the utility of ABMs for examining landscape-scale processes and locally-relevant policies and management strategies, the transferability of fire ABMs to other locations or their application beyond the landscape scale has yet to be demonstrated. Agent-based approaches to represent human behaviour have been used, however, to examine land-systems at a continental scale through defining Agent Functional Types (AFTs) (Brown et al., 2019). AFTs build on theoretically-informed typologies to create generalisations about human–environment interactions through two essential human characteristics: roles (e.g., forester, farmer) and behaviours (e.g., imitation, conservatism). They therefore allow representation not only of direct human impacts on the environment, but also behaviourally-mediated responses people might make to consequent environmental change.
The advantages that ABMs provide for representing heterogeneous individual agents and their interactions have high data costs for both model parameterisation and evaluation. Data concerning the beliefs, values and/or objectives that shape human decision-making, for example, are needed to construct ABMs (Robinson et al., 2007; Smajgl et al., 2011) but are difficult to collect even at local scales. Most land-system ABMs have been placed-based and landscape-scale because the empirical data required to construct and use these models are difficult to obtain at broader scales (Verburg et al., 2019). Furthermore, the inherent complexity of dynamics resulting from multiple interacting, heterogeneous individuals can make identifying the causes of emergent patterns and outcomes challenging. It can be difficult to establish which features are common to the system of study and which are contingent on particular boundary conditions or structures in a case study (Millington et al., 2012; Manson et al., 2020). In the face of limited data for parameterisation and the high degrees of freedom in model structure, there may be considerable subjectivity involved in ABM development (Lee et al., 2015) and robust empirical verification or validation of a model is challenging (Schulze et al., 2017). As with all models, the quality of representation is dependent on the quality of data and theory available; in the case of ABMs for agency of individual humans these can be both lacking and contested.
The adoption of more general assumptions, such as the use of AFTs, may facilitate the application of ABMs to understand human-fire interactions at a global scale. However, this will require a substantial improvement in the availability of data on human activities in relation to fire. Information about fire use in the context of agriculture, hunting and pastoralism is widespread in the literature, but fragmented across numerous academic disciplines including anthropology, sociology, development studies, ecology, and agronomy (Coughlan and Petty, 2012). Synthesising such data into a global dataset to provide the empirical basis for improved modelling of anthropogenic fire is an important research priority. However, such synthesis could be used with tools such as cluster analysis to define AFT roles (Malek et al., 2019); this would be equivalent to gathering primary data e.g., through social surveys for using ABMs at finer spatial scales (Smajgl et al., 2011). Determining the global spatial distribution and variation of AFTs defined through a series of small-scale case studies represents a further challenge, but could be solved through comparison with secondary data sets and previous attempts to map land use and land use intensity at the global scale (e.g., Haberl et al., 2007; Malek and Verburg, 2020).
Representation of the policy development process (see Fire and Policy) is a major challenge in computational modelling of socio-ecological systems (Brown et al., 2019). Whilst agent-based modelling of policy outcomes and their (un-)intended consequences is comparatively widespread, few models include an explicit representation of the policymaking process itself (Castro et al., 2020). Policy is generally represented, at the regional to global scale, as a weighting towards a given outcome or ecosystem service provision within land user calculations (e.g., Holzhauer et al., 2018). One important shortcoming of this approach for wildfire models is the inability to account for abrupt policy changes in response to catastrophic fire regime shocks. Even when representing policy simply through input parameters, combining consideration of local, national, and global policy influences with land user preferences in a model could lead to highly complex emergent phenomena, with consequences for model interpretation and utility (Caillault et al., 2013).
Economic Models of Wildfire
Economic analysis examines the human drivers of wildfire occurrence, its effects on economic activity, and quantifies wildfire costs and benefits in terms of changes in human welfare (Figure 4). Economic models of wildfire are largely empirical and aim to establish relationships between variables describing human behaviour and wildfire, estimate marginal effects, or monetise impacts. Modelling can be at the micro-level of individual agents including persons and firms, or at the aggregate macro-level of jurisdictions, states or countries.
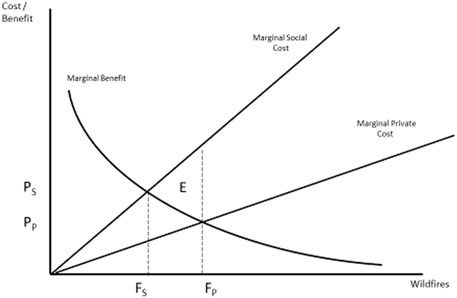
FIGURE 4. External costs of wildfires. Note: The horizontal axis measures wildfire incidents or area burned. The vertical axis measures costs and benefits in £. The marginal benefit line shows the additional benefit from one extra wildfire. Marginal Social and Marginal Private cost lines show the additional private and social costs from an extra wildfire. FS and FP represent the social and private optimal wildfire occurrence. Area E shows the external costs from oversupplying wildfires.
Wildfire can be the intended outcome of the behaviour of economic agents, where its timing and frequency is determined to optimise an objective function (Varma, 2003; Yoder, 2004; Prestemon and Butry, 2005; Purnomo et al., 2017). Economic models can be used to assess the efficiency of wildfire use, the extent to which its occurrence aligns with its socially desirable level. Many wildfire costs are not borne by the agents enjoying the benefits, and compensation mechanisms are not in place. These external costs are ignored by fire users, leading to overutilization of wildfire. At the same time, failure to acknowledge external benefits can lead to oversupply of suppression and under-provision of wildfire. Wildfires can occur as a result of seemingly unrelated economic activity and distorted incentives (Warziniack et al., 2019; Champ et al., 2020; Kountouris, 2020). Economic models characterise the wildfire-human interaction, highlight the presence of external costs and study agents’ incentives, to assist in the development of effective wildfire policy.
Econometric models of wildfire combine variables on natural and human systems. Models may use time series data, samples of a population at a given time (cross-sections) or repeated sampling of the population through time (panel) data. Wildfire occurrence and burned area information typically come from earth observation or international, national and local incident databases. There are multiple sources of economic variables. Macroeconomic models to assess the relationship between wildfire and the aggregate economy usually employ data on national or subnational income, employment, land use, or other economy-wide metrics (Wibbenmeyer et al., 2019; Boustan et al., 2020; Liao and Kousky, 2020). Microeconometric models examining the relationship between individual agent behaviour and wildfire use consumer, household, firm or farm level survey, census and administrative data, and contrast the behaviour of units that do, or do not experience wildfire and its consequences.
Models assessing either wildfire impacts on economic outcomes or economic impacts on wildfires typically control for factors affecting both human behaviour and wildfire. Unobserved confounders simultaneously determining wildfire and economic outcomes introduce biases in the estimation of causal relationships. Researchers utilize variation in space and time for estimation (Jayachandran, 2009; Moeltner et al., 2013). Biases due to individual (agent or jurisdiction) specific, time-invariant unobserved characteristics can be addressed through differencing or fixed effects estimators, which utilize the time dimension to remove both observed and unobserved constant-over-time characteristics, and employ within variation to estimate coefficients of interest (Michetti and Pinar, 2019; Bayham and Yoder, 2020).
Endogeneity concerns remain from individual-specific time-varying unobserved variables, while panel data are not always available. Assessing the effect of wildfire smoke on health and behaviour, for example, is challenging as agents can self-select their degree of exposure. To address this type of problem, researchers leverage plausibly exogenous variation in agents’ exposure (Angrist et al., 1996). Zivin et al. (2019), for example, used variation in wind direction to compare examination performance in schools located upwind and downwind of a wildfire.
Estimates of the economic value of wildfire impacts can be used for developing wildfire management policy, and for comparing different mitigation and adaptation interventions in a cost-benefit analysis framework. A good or service is considered to have economic value to the extent it contributes to human welfare. Economic valuation techniques are used to estimate the change in human welfare resulting from experiencing wildfire or its aftermath, and translate this into monetary units. Valuation assumes substitutability: consumers are willing to sacrifice income to avoid the negative consequences of wildfire, or are willing to accept compensation for wildfire damages. The economic value of some impacts can be inferred directly using market prices and estimations of the cost of replacing lost infrastructure and production (Butry et al., 2001; Richardson et al., 2012; Stephenson et al., 2013). It is harder to capture the total economic value of wildfire, however, either because there are no markets and prices for some ecosystem goods and services, or because observed prices are inaccurate signals of the true marginal social costs and benefits (Freeman et al., 2014). Ignoring the value of non-marketed goods and services, and pricing distortions, reduces the estimated cost of wildfire leading to its overuse.
The non-marketed impacts of wildfire can be monetised using revealed and stated preference valuation methods (Freeman et al., 2014). Revealed preference methods use information from transactions in related markets to infer the value of an ecosystem good or service. The travel cost method is used to monetise the loss in recreational value from wildfire by modelling demand for recreation activities as a function of burned areas (Nobel et al., 2020) or wildfire risk (Hesseln et al., 2003). Hedonic pricing approaches typically use data from property market transactions to infer the influence of wildfire risk (Donovan et al., 2007; McCoy and Walsh, 2018) or the proximity to burned area (Stetler et al., 2010) on property prices. Stated preference valuation asks consumers to participate in hypothetical markets and declare their preference for a non-marketed good or service. In contingent valuation studies consumers state whether they are willing to pay (accept) some price to avoid (as compensation for incurring) the effects or risk of wildfire (Loomis et al., 2005; Loomis et al., 2009; Molina et al., 2019). In choice experiments, consumers make a series of choices among hypothetical good profiles comprising a series of attributes at different levels. When one of the attributes is monetary, the willingness to pay for each characteristic can be estimated (Remoundou et al., 2012; Campbell and Anderson, 2019; Mueller et al., 2019; Alló and Loureiro, 2020). Both stated preference approaches directly model consumers' utility to explain stated choices. Whereas revealed preference methods only capture the effect of wildfire on use values (recreation, amenity etc), stated preference approaches also capture non-use values (existence, bequest etc.). However, values estimated from stated preference studies could over- or underestimate the true costs and benefits of wildfire since they are hypothetical by design and, although careful design of the hypothetical market may go some way to reducing the biases (Carson and Groves, 2007; Vossler et al., 2012), the validity and usefulness of stated preference value estimates for policy making is highly debated (Diamond and Hausman, 1994; Hanemann, 1994; Carson, 2012; Hausman, 2012).
Physics-Centric Models
Wildfire Spread Models
Spread models predict the position and intensity of the wildfire as a fuction of time and explain how it will evolves over a given landscape. They are widely used as tools for wildfire preparedness (e.g., for prescribed burn planning, fuel management, evaluating threats to values-at-risk, ecological applications, and training tools) and for operational firefighting (supporting incident management). They have also been used for post-wildfire investigations into suppression effectiveness and forensic support (Pearce, 2009). Additionally, the outputs of wildfire spread models are also being integrated with fire evacuation models such as EXODUS (Veeraswamy et al., 2018) and open source platforms (Ronchi et al., 2019). However, in contrast to the other types of models described here, wildfire spread models do not seek to address the two-way interactions between humans and fire explicitly.
It was understood more than half a century ago that the principle of energy conservation could provide the basis for simulating the rate at which a fire front spreads across a landscape (Rothermel, 1972; Weber, 1991). In fire spread models, the spread of fire from one grid cell to the next may be based on the physical conservation of energy principle. Physics-centric fire models include models of fuel-flame-plume interactions, such as Wildland Fire Dynamics Simulator (WFDS) (Mell et al., 2009). These models include physical processes such as fluid dynamics, combustion, heat transfer, pyrolysis, microphysics and turbulence, which are generally resolved at a high spatial resolution (cm-scale). Other physics-centric models are concerned with plume-atmosphere interactions, which usually involve coupling relatively simple fire models within a high resolution mesoscale atmospheric model such as the Weather Research and Forecasting model (WRF-FIRE) (Mandel et al., 2011).
Physics-centric models are computationally demanding and are not able to simulate fire spread in real-time (Figure 5). Semi-empirical fire spread models were developed in response to operational needs to simulate the spread of fire across landscapes in real-time, or ideally faster than real-time. Such fire spread or fire growth models use spatial data on fuel characteristics (e.g., vegetation type, loading, moisture content), topography (elevation, slope, aspect), and weather (temperature, relative humidity, wind speed, wind direction). Model outputs (e.g., fire perimeter, fuel consumption) are determined by empirical fire behaviour sub-models, termed fuel models – a suite of empirically-derived fuel-specific equations that describe the relationship between the fuel, topography and weather inputs, and the fire rate-of-spread and/or intensity. The spatially-explicit simulation of a fire spread across a landscape uses Huygens’ physical principle of elliptical wave propagation (Richards, 1990; Finney, 1998). Examples of semi-empirical fire spread models include the Fire Area Simulator (FARSITE: Finney 1998) and Prometheus (Tymstra et al., 2010). FARSITE was developed by the U.S. Forest Service (USFS) as a National System for predicting wildland fire behaviour and spread in areas of the United States and is widely used by federal/state land management agencies as an operational tool for planning land management fires, responding to escaped fires, and responding to wildfire incidents. FARSITE’s empirical fuel models rely upon the surface fire predictions of Rothermel (1972), whose equations were derived from a series of small-scale laboratory burns based on homogeneous dead fuel beds. In contrast, the Prometheus model uses empirical fuel models representing 16 fuel types typical of Canadian ecosystems based on measurements of landscape-scale fires (Tymstra et al., 2010). Prometheus is mainly used to provide a decision-support tool to aid fire managers planning prescribed fires, and in responding to escaped fires which necessitate the need to fight fire on the landscape (Suffling et al., 2008), but has also been used to examine landscape fire risk (Parisien et al., 2005; Stralberg et al., 2018). Other examples of semi-empirical fire spread models include Phoenix (Tolhurst et al., 2008) and SPARK (Miller et al., 2015) developed for Australian bushfires (Neale and May, 2018).
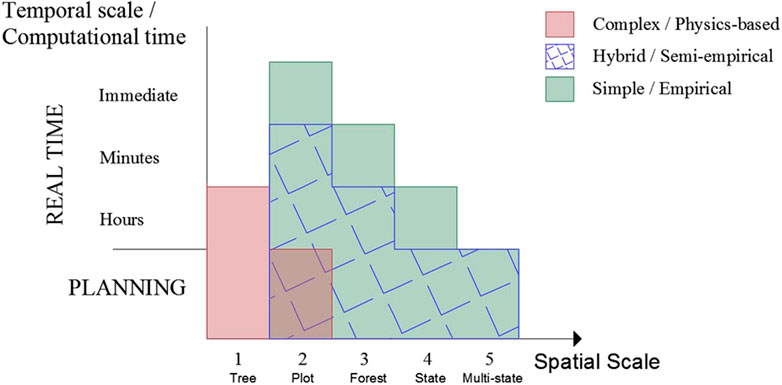
FIGURE 5. Typical time and space scales for different types of wildfire spread models Figure by Ronchi et al. (2019), CC BY.
As well as providing information on spatially-explicit fire perimeters, most of the semi-empirical models will also provide information on fireline intensity (kW m−1), flame length (m), rate of spread (m min−1), heat release density (kJ m−2), reaction intensity (kW m−2), along with information about such behaviours as crown fire activity (e.g., FARSITE). Depending on their use, fire spread models may include a number of additional physical or parameterised sub-models. Some examples include the parameterisation of embers-driven spotting behaviour (Finney, 1998) and emissions modules that may be used for subsequent modelling of smoke plume dispersion (Volkova et al., 2018).
Global Fire Models
Global fire models are mathematical representations of processes that determine the occurrence and extent of fire, including ignition, spread, fuel combustion, vegetation mortality and natural suppression (Hantson et al., 2016). Global fire models are designed to interface with dynamic global vegetation models (DGVMs) to explicitly model the impact of fire on ecosystems and large-scale vegetation distribution. However, since DGVMs are increasingly included in the land-surface component of climate or earth system models, fire-enabled global vegetation models are also used to make predictions of how changes in fire regime impact pyrogenic emissions, biogeochemical cycles and ultimately climate.
The vegetation model in which the fire model is embedded provides information about the vegetation, generally in terms of the proportions of different plant functional types present, and this in turn determines the amount of both live and dead fuel loads. Climate affects both vegetation growth and the probability of fire. Temperature and precipitation determine what plant types can grow and their productivity, for example, and hence determine fuel availability; they also determine the rate at which fuel dries out and therefore whether it is susceptible to burn. Wind speed, fuel continuity and the atmospheric vapour pressure deficit are important factors determining the rate at which a fire spreads and hence how large an area is burnt.
The most fundamental assumption underpinning the representation of fire in global vegetation-fire models is that processes can be represented mathematically and are universal in space and time. Spatial or temporal patterns in the expression of these processes should arise explicitly from the patterns in the controls on these processes. Thus, for example, a lightning strike will trigger an ignition if it produces a long continuing current sufficient to reach a given temperature threshold. Spatial differences in the number and energy of cloud-to-ground lightning strikes then determine how many lightning ignitions will take place in a given region. Similarly, an ignition will cause a fire only if the fuel bed is sufficiently dry; spatial differences in the occurrence of fire after ignition are then determined by climate factors that affect fuel dryness in a given location.
The implementation of universal processes modulated by spatial or temporal differences in their controls is not always straightforward. In practice, many global vegetation-fire models use simplifications (parameterisations) of complex processes or represent these processes through empirical relationships. For example, fuel drying rates are determined not only by the atmospheric vapour pressure deficit and wind speed but also by the size and arrangement of the fuel (fuel packing). The influence of fuel packing on drying is represented through empirical relationships that relate packing to the size of the fuel, which in turn is related to the rate of drying and fire spread. Simplifications may also be introduced because of inadequacies in the available input data. For example, until recently the only global lightning data set available provided information about the total number of lightning strikes (Cecil et al., 2014), requiring assumptions about the partitioning between cloud-to-cloud and cloud-to-ground strikes and about the energetic efficiency of cloud-to-ground strikes (Latham and Schlieter, 1989). In the absence of other information, it is usually assumed that these relationships are constant in space and time (see e.g., Thonicke et al., 2010).
Further simplifications are introduced (see Hantson et al., 2016; Rabin et al., 2017) because global vegetation-fire models are designed to operate at a coarse resolution (0.5° × 0.5° or coarser). This has three consequences. Firstly, the input data are specified at this resolution which means the model is run using average conditions. For example, the influence of wind speed on fire spread does not take account of variable wind gustiness or of pyrogenically induced winds. Secondly, models do not predict the precise location of a fire, but rather the proportion of a grid cell that is affected. Finally, there are no interactions between grid cells: fires do not spread from grid cell to grid cell.
Although most global fire-vegetation models include some consideration of the role of humans in fire regimes (Rabin et al., 2017), this is the area which is treated most simplistically in the current generation of models. Humans are considered as a source of ignitions in many models (Figure 6). The number of ignitions is generally specified as a function of population density, increasing up to a threshold value where there are no additional ignitions with increasing population. Both the strength of the relationship between population density and number of ignitions and the threshold value are empirically tuned and vary between models. Some models employ different relationships between population density and number of ignitions depending on human economic systems (e.g., LPJ-LMfire: Pfeiffer et al., 2013), although again the values are empirically tuned. Humans may also be considered as a source of landscape modification (Figure 6), both promoting (e.g., agricultural fires, deforestation fires) and suppressing (e.g., through landscape fragmentation) fire. However, the failure to identify a universal process susceptible to mathematical formulation and modification through changes in easily obtained inputs means that these treatments are not fully prognostic. For example, deforestation fires are currently simulated using observed patterns of deforestation as an input. This allows the ecological, biogeochemical and climatic consequences of recent deforestation to be quantified, but the model has no predictive power because it does not incorporate process understanding about the causes (and therefore the possible future occurrence) of deforestation. Similarly, although most models exclude fire in cropland areas and thus account for the contribution of agricultural expansion to landscape fragmentation, models which include fire as an agricultural management tool are not fully prognostic, relying on data derived from remote sensing for burned cropland fraction (Rabin et al., 2018) or for empirical (non-process-based) parameterization of crop fires (Li et al., 2013).
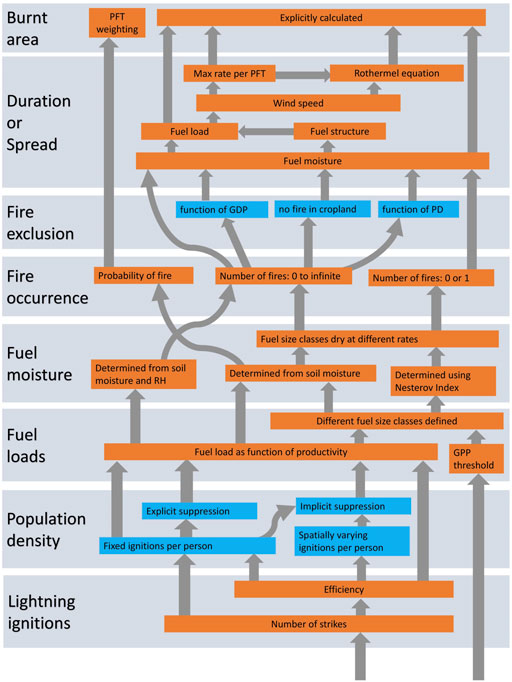
FIGURE 6. Simplified representation of the structure of a global fire model. The orange boxes represent natural processes and the blue boxes human activities that impact fire starts, spread or duration, exclusion and/or suppression. The grey arrows show pathways taken by individual global fire models where arrows to a specific box show that this process is included explicitly. GPP, gross primary production; RH, relative humidity; PD, population density; GDP, gross domestic product: PFT, plant functional type. The Nesterov Index is one example of a fire danger index where ignition probability is calculated as a function of climate. The Rothermel equation is a quasi-empirical expression for the rate of fire spread based on the conservation of energy.
Global vegetation-fire models are useful tools for investigating the impact of changes in climate on fire regimes and feedbacks to climate. In a world increasingly affected by changes in land use and land management, it is imperative to incorporate more realistic treatments of human-fire interactions (Andela et al., 2017; Forkel et al., 2019a; Teckentrup et al., 2019; Hantson et al., 2020). Improved understanding of the processes involved, identification of which drivers could be specified from global data sources, and the creation of appropriate driving data sets are key to implementing human-fire interactions in global models in a realistic way.
Fire and Policy
Policies are a set of ideas or guidelines for the actions undertaken by many types of organisation including governments, non-governmental organisations (NGOs) and businesses. Fire policies may draw on the information provided by the various fire models described in the preceding sections and – through their impact on human behaviour and vegetation – change the input data for these models. Thus, when thinking about modelling human-fire interactions, it is helpful to consider how policies are developed and implemented (Figure 7).
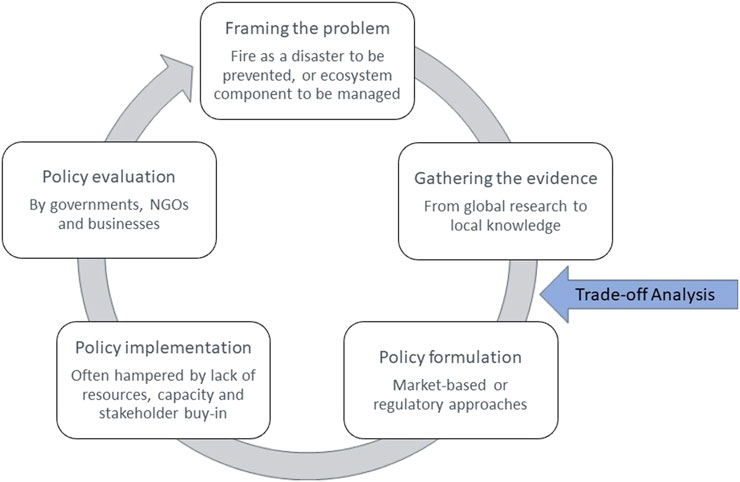
FIGURE 7. Simplified policy cycle: Far from being a technocratic exercise, politics is embedded in the cycle, from the framing of the problem to determining which evidence to use, weighing up trade-offs between different policy options, ensuring resources and buy-in for policy implementation and undertaking and reacting to evaluation.
Policies are usually developed in response to a problem. However, the problem of fire can be framed very differently: for example, ecologists may focus on maintaining biodiversity by managing the size and timing of fires in line with the needs of an ecosystem or species of interest, whereas town councils will focus on preventing damage to people and infrastructure. In response to the 2015 Paris Climate Agreement, managing land use to reduce carbon emissions or increase sequestration has become an increasingly powerful driver for many fire-related policies (Eloy et al., 2019). However, land use management decisions necessarily involve trade-offs between the aspirations of different groups of people (Mace et al., 2018). Thus although policies to reduce emissions from deforestation and forest degradation and enhance carbon stocks (REDD+) are promoted as win-win solutions, their implementation could lead to significant carbon-biodiversity trade-offs in fire-prone old-growth grassland ecosystems and reverse progress made in decentralising forest management to local communities (Phelps et al., 2010; Phelps et al., 2012).
Policy formulation is a messy and unpredictable process (Cairney, 2015) that involves weighing up and negotiating trade-offs, that are essentially about power, relationships, responsibility and accountability (Nunan et al., 2018). In many cases, policy is determined more by politics than evidence. In Australia, for example, the compulsory purchase of land by government recommended by the Victoria Bushfire Royal Commission on the catastrophic fires of 2009 (Teague et al., 2010) was not adopted because of its political unpopularity (Bowman et al., 2011). Similarly, an investigation into a fire in which five fire-fighters died in Spain terminated discussion of the limitations of fire control (González-Hidalgo et al., 2014). The adoption of evidence-based policy may also be limited because the underpinning bodies of evidence, whether local or global, are rarely neutral. Fire experiments, which informed both colonial and post-colonial fire policy in West Africa, were designed to test the belief that the savanna was the product of generations of anthropogenic burning (Laris and Wardell, 2006), a view that has been widely contested both by social scientists (Amanor, 2002; Leach and Scoones, 2015) and by ecologists (West et al., 2000; Bond and Zaloumis, 2016). Knowledge-production is fundamentally political in nature, as is evident in the privileging of remote sensing and quantitative analyses over traditional ecological knowledge in supporting fire suppression policies (Sletto, 2008; Leach and Scoones, 2015).
Fire-related policies broadly fall into two categories, state-enforced regulations and market-based mechanisms, though the boundaries between them are increasingly blurred (Sikor et al., 2008; Lambin et al., 2014). Regulatory policies include land use zoning associated with rules about whether and when fires can be set. Market-based mechanisms, such as commodity roundtables (e.g., the Roundtable on Sustainable Palm Oil) may be implemented by the private sector but enforced by NGOs. Payments for Environmental Services schemes, like REDD+, may involve both governments and NGOs in implementation and enforcement (Lambin et al., 2014). The different approaches can co-exist. Carmenta et al. (2017), for example, report that severe peat fires in Sumatra led to the development of a variety of fire management interventions across scales, sectors and stakeholders, ranging from new regulations to technical innovations, developments in fire monitoring and the provision of incentives to communities for fire-free practices.
Once policies are formulated, there is an assumption that they will be implemented (Figure 7). In reality, there is often a large policy-implementation gap. This may arise because responsible authorities lack the capacity or resources to enforce new rules. It can also be the result of poor policy development processes which have not effectively addressed the trade-offs experienced by some stakeholders. The fire exclusion policy adopted in Bale National Park in Ethiopia, for example, forced local communities to stop small-patch burning practices and resort to illlicit fires, often set late in the dry season when ignition is more likely, to maintain the grazing landscape, with the inadvertent result that the size of fires in the park has increased (Johansson et al., 2019). In contrast, wide acceptance allows Indigenous Tagbanua farmers in the Philippines to practise traditional fire-based swidden farming despite the practice being criminalised for decades by both state and non-state actors (Dressler et al., 2020). Political interests may also undermine policy intentions. In Tanzania, for example, community forest and fire management initiatives must defend collectively owned lands from the hunting fires set by more powerful and politically well-connected stakeholders who hold hunting concessions (Khatun et al., 2017). Understanding the diverse motivations for fire use is essential if interventions are to succeed (Carmenta et al., 2017). In the case of Sumatran peat fires, for example, no single stakeholder group is primarily responsible for fire-setting and there are many nuanced motivations for setting fires (Carmenta et al., 2017). Co-production of fire policies through the involvement of local stakeholders (Laris and Wardell, 2006; Monzón-Alvarado et al., 2014; Humphrey et al., 2020) and the recognition of traditional knowledge in the environmental policy making process (Rodríguez et al., 2018; Bilbao et al., 2019; Devisscher et al., 2019; Mistry et al., 2019) may be important ways to narrow the policy-implementation gap. When policies are evaluated (and possibly adapted) there is a need to disaggregate across societal groups, with particular attention paid to the voices of often marginalised stakeholders, such as the poorest, Indigenous peoples and women (Schreckenberg et al., 2018).
Issues and Commonalities
The models discussed here originate from diverse disciplines, were developed for different purposes and address different questions, include different types of processes, and operate on different time and space scales, resolution and complexity (Figure 1). Nevertheless, they share some common features.
Each type of model has a theoretical basis for the representation of the relationship between humans, the biological, physical, and in some cases, spiritual, attributes of the environment, and fire. The underlying theory may be incomplete, the theoretical basis of some individual models developed within each class of models may even be wrong, but there is an assumption that the models should embody causal relationships. Data analysis or machine-learning approaches have been used to derive empirical relationships or parameterisations for economic models (Papakosta et al., 2017; Storm et al., 2020) and global fire models (Forkel et al., 2017; Stralberg et al., 2018; Forkel et al., 2019b), and may even be useful for the development of typologies (e.g., Delgado et al., 2018), but these are not unsupervised analyses and the types of data are selected based on the underlying theory involved.
Each type of model is a simplification of the complexities of the real world. Even place-based models of fire knowledge represent a partial view, because participants are selected or self-select, because people understand and relate to fire in multiple ways which may not always be elicited in the process of model construction, or because some social constructs are difficult to represent. Practical considerations also lead to simplification, whether this is the limited computer power available for global-scale fire modelling which precludes ultra-high resolution in order to simulate individual fires (see e.g., Toivanen et al., 2019) or the limited data availability that necessitates substituting travel costs to monetise loss of recreational value in economic modelling (e.g., Hesseln et al., 2003; Nobel et al., 2020). Simplification may be driven by considerations of the relative importance of specific processes at a given time or space scale, informed by theory, or by lack of appropriate data. Lack of quantitative data on the timing and extent of human burning and fire suppression in agricultural areas, for example, underpins the widespread use of population density as a surrogate measure. However, all of the types of model require extensive data inputs, and in many cases data limitations are the strongest constraint on model development.
The models differ in potential for co-production by scientists and stakeholders that use or manage fire or are directly impacted by wildfires. In some cases, stakeholder knowledge is incorporated by scientists into a pre-determined model structure. This is the case, for example where stated preference approaches are used in economic modelling (e.g., Loomis et al., 2005), or interview and social survey data feeds into an agent-based model (e.g., Spies et al., 2017). Stakeholders may also participate more directly, potentially playing a part in defining the structure of the model. Place-based models of fire knowledge have been most amenable to such participatory modelling approaches (e.g., Bilbao et al., 2019). Generally, co-production is more likely and feasible where models operate at smaller spatial scales, and can incorporate information from individuals or communities, and where power differentials between stakeholders are less extreme.
Each type of model is oriented towards and designed to lead to practical outcomes. Place-based models of fire knowledge, for example, can be used to engineer dialogues between different groups of stakeholders or help promote cultural identity. ABMs can be used to develop locally relevant policies for landscape management, while economic models can be used to ensure that the hidden costs and benefits of wildfire are factored into the development of effective fire management policies. Global fire models provide a way to predict changes in fire regimes and fire impacts in response to future climate and land-use scenarios. However, different types of models may yield different recommendations for fire policy and management because of their very different foci and scales. The need to develop more holistic fire-related policies and practices provides a good motivation for combining the strengths and benefits of different types of models.
Some differences between the models may be more apparent than real. There is an apparent tension for example between the assumption made by global fire models that processes are universal and the place-centred focus of place-based models of fire knowledge. This translates into the perception that policies and practices based on local knowledge cannot be usefully applied elsewhere. However, global fire models also assume that universal processes such as ignition, fire spread and extinction are governed by factors that vary spatially, such as climate or vegetation properties or land use, thus giving rise to different fire regimes (Hantson et al., 2016). The use of agent typologies (e.g., Lauk and Erb, 2016) makes a similar assumption - that there are universal activities or types of behaviour, although the mix of different agent types may change through time or across space as do climate and vegetation properties.
Moving Forward: Key Challenges and a Roadmap for Model Use and Integration
The major challenge for developing better models of human-fire interaction is lack of data that can be used to develop heuristic, globally-applicable schemes in a modelling framework. Collecting field data at a local level is time-consuming. The synthesis of data from multiple local studies into a global data set could provide one route to obtaining sufficient data to parameterise models, whether these are ABMs, economic models or fire-enabled vegetation models, but the quality of such a synthesis depends to some extent on whether the same information has been collected and whether the same data-collection methods have been applied (Costafreda-Aumedes et al., 2017). Many place-based models of fire knowledge are not framed in the quantitative way required for use by global fire models, for example. There is also an issue about representativeness. In the physical sciences, there are standard methods that are used to judge what is the minimum data set required to provide global metrics (see e.g., Mann et al., 2008). Such an assessment is likely to be more difficult given the diversity of human socio-economic and cultural systems and the heterogeneity of their influences on fire. Furthermore, research on human use and management of fire is fragmented across many disciplines and heterogenous in the methods used and data produced. However, this situation is not uncommon in studies of socio-ecological systems, and meta-study methods to synthesise diverse case-studies have been developed (Magliocca et al., 2015; van Vliet et al., 2016). Large scale syntheses using such methods are ongoing (e.g., Perkins et al., 2021; Smith and Mistry, 2021), with the aim of improving systematic understanding of human use and management of fire, which in turn will be useful in developing improved human-fire models.
The scaling-up of models that function at a local scale to the global scale is also a major challenge. Models of fire spread, for example, explicitly deal with the influence of topography, whereas global fire-enabled vegetation models ignore topographic influences. To some extent, this is because of the computational cost that working at sufficiently high resolution would entail but it also reflects decisions about the importance of specific factors at different scales, and whether these processes can be represented probabilistically rather than deterministically.
To some extent, scaling up can be seen as a process of simplification. For example, scaling ABMs to the global scale could involve defining a limited number of Agent Functional Types (AFTs) based on theoretical typologies derived for example from local fire studies. This would be parallel to the use of Plant Functional Types (PFTs) in most of the current generation of dynamic global vegetation models (and indeed in most land-surface models). This parallel is informative about the potential traps involved in such simplification. The original idea behind the use of PFTs to represent plant functional diversity involved classifying plants in terms of adaptations to climate (Prentice et al., 1992; Harrison et al., 2010), drawing inspiration from the seminal work of Raunkiær (1909). PFTs defined in this way do not necessarily represent plant functional diversity with respect to other traits, for example they do not distinguish between fire-adapted, fire-tolerant and fire-intolerant species (Pausas et al., 2004; Brando et al., 2012; Clarke et al., 2013). Furthermore, recent empirical (Diaz et al., 2015) and theoretical (Wang et al., 2017; Smith and Keenan, 2020; Wang et al., 2021) developments suggest that there are alternative ways to treat plant diversity, including simulating the adaptive traits directly (e.g., Scheiter et al., 2013; Fyllas et al., 2014; Berzaghi et al., 2020) or as emergent properties of the system (Wang et al., 2017; Franklin et al., 2020; Harrison et al., 2021). Thus, in the creation of simplified representations of human-fire interactions, it will be important to consider both the purpose of the model which will use these relationships and to test the theoretical basis for such representations rigorously. Furthermore, in so far as the development of typologies of fire users assumes there are universal rules governing how specific fire-user types behave across time and space, or that certain fire practices are associated with certain environmental conditions, there is a danger of adopting outdated concepts including environmental determinism or societal evolution through linear stages of economic development, with corresponding land management practices, from primitive to modern (Coughlan and Petty, 2013; Coughlan, 2015).
Despite these challenges, there are ways to move forward. Climate projections are made using scenarios of changes in anthropogenic emissions and land use developed using alternative assumptions about human activities in the future, for example a continued dependence on fossil fuels or the widespread adoption of deliberate strategies to reduce emissions (O'Neill et al., 2017; Riahi et al., 2017). Global fire-enabled vegetation models can use these climate projections to examine the consequences of climate changes for future fire regimes, but they could also use the underlying scenarios about human activities to modulate the treatment of human ignitions and/or suppression.
Notwithstanding the highly political nature of much policy-formulation, the potential for different types of models to lead to radically different outcomes for policy and management means that some form of model integration is vital. The primary step is the development of ways to use outputs from one model to inform other models. For example, global vegetation-fire models could be used to make projections of the probability of climate-induced changes in fire regimes. These projections could be used in the context of economic models to determine the costs and benefits of fire management or fire reduction at a regional scale. Agent-based models could then be used to establish whether specific mitigation actions are likely to be taken up in these regions. The predicted changes in human behaviour could then be used to construct new scenarios for incorporation into global vegetation-fire models simulations. The enchaining of models in this way is already integral to impact modelling, for example in the framework of the ISIMIP project (Rosenzweig et al., 2017). The challenge is either to develop consistent terminology and standardised protocols that allow outputs from one model to feed into another model or to provide appropriate tools that translate these outputs into appropriate formats.
Another step in using multiple kinds of models to address human-fire interactions could focus on the implicit coupling of global and regional models. Global climate simulations, for example, are used to provide the boundary conditions for regional climate models which because of their higher resolution can be used to project the influence of local features, such as large lakes, on regional climate (e.g., Diallo et al., 2018). Higher-resolution regional models can also reduce the need for parameterisation of individual processes (Giorgi, 2019), but since they operate on a limited spatial domain they can do so without excessive computational costs. Similar approaches could be adopted with fire modelling, either to link similar types of models across scales (e.g., fire spread models and global vegetation-fire models) or to derive probabilistic representations of human-fire interactions derived from local studies into global-scale models. Improved coupling between regional and global scales would facilitate addressing fire policy questions since wildfires are not typically confined to a single jurisdiction. Fire management has a global dimension, as illustrated by climate change mitigation concerns and transboundary pollution associated with forest fires in the tropics (Khatun et al., 2017). Scale is a challenge for natural resource governance, since ecological and social/administrative processes rarely occur on the same spatial or temporal scale (Nunan et al., 2018) and politics and power often determines the scale at which decisions are made (Zulu, 2009). Effective multi-level governance requires both vertical coordination between actors at different levels and horizontal cooperation, e.g. between different sectors (Nunan et al., 2018).
It is difficult to envisage creating a single model that addresses all the different questions and scales currently addressed by different types of human-fire models. Nevertheless, there are obvious avenues for integration. The use of local conceptual or placed-based models of fire knoweldge and of global fire-use typologies to develop agent classifications for use in global ABMs seems a fruitful avenue to explore. ABM can be readily combined with economic models (e.g., Bert et al., 2015), and building economic constraints into land- and fire-use decision-making would be useful. There is also an important gap in linking place-based models of fire knowledge with ABM. Given that landscape-scale ABMs have already drawn on interviews, surveys and workshops with fire managers in developed-world contexts (e.g., Millington et al., 2008; Spies et al., 2017), there is no reason why models could not be derived from data generated in similar ways with indigenous fire practitioners. Pursuing such model integration to understand human-fire interactions in developing world landscapes will be important for improving fire management and ensuring sustainability under changing socio-economic and climate conditions.
There is potential for global ABM predictions to be incorporated in fire-enabled global vegetation models: given that some global models already allow relationships between population density and number of ignitions to vary depending on changes in human economic systems through time (e.g., Pfeiffer et al., 2013), this could be extended to include other human-fire interactions, such as suppression. Including humans as agents within a fire-enabled global vegetation model would also lay the groundwork for incorporating feedbacks between changing fire regimes and changing human activities (Figure 8). For example, under a loose model coupling, the ABM would provide static inputs to the fire-enabled global vegetation model, for example by replacing anthropogenic ignitions from population density with an ABM output. Under a tighter-coupling (Figure 8), the ABM would be run alongside the fire-enabled global vegetation model, allowing cross-system feedbacks to be captured though at the expense of significant additional model complexity. In a loose coupling, the ABM’s ecological inputs such as land cover and NPP would come from secondary data, whilst under a tighter model coupling, these could come from the dynamic outputs of the fire-enabled global vegetation model (Antle et al., 2001). Designing rigorous methods of evaluating these coupled human-fire models, as is already done for fire-enabled vegetation models (Hantson et al., 2020), will require careful consideration.
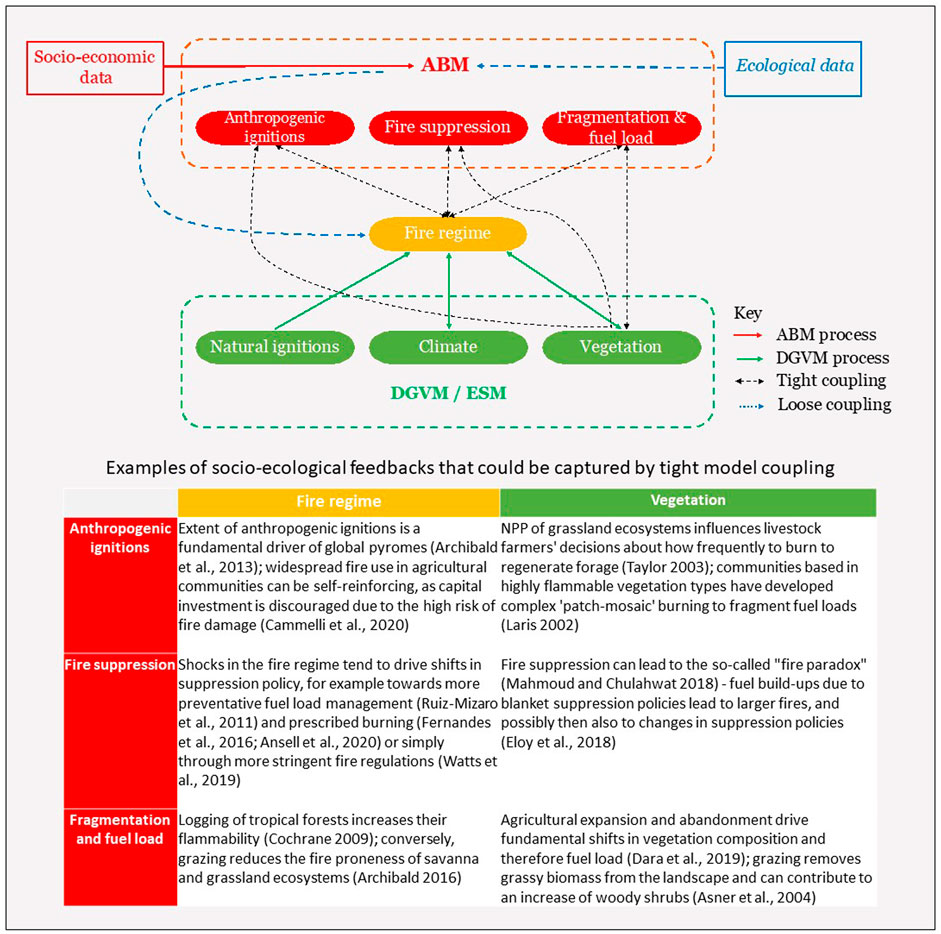
FIGURE 8. Options for integrating an agent-based model of anthropogenic fire impacts into a fire-enabled global vegetation model or Earth System Model (ESM). The table shows examples of socio-ecological feedbacks that could be captured by tight model coupling.
The enchaining of models is not unidirectional. While it may be useful to think how insights gained at local scales or models that consider a single component of the human-fire system can contribute to improving global fire models, there could also be a useful flow of information from global models to other kinds of fire modelling. When modelling closely coupled human-natural systems, it is common to explore the uncertainty associated with policy decisions, socioeconomic trends, and technological development by exploring discrete scenarios for the future. These scenarios, or storylines, can be used to set up simulations from one kind of model that can then be fed into other kinds of models, in order to evaluate various aspects of the changing Earth system. The Inter-Sectoral Impacts Model Intercomparison Project (ISIMIP), for example, uses scenario-based trajectories from climate, economic, and land-use models as inputs to models that simulate the impacts of climate change on sectors including agriculture, human health, fisheries, and many others (Frieler et al., 2017). The outputs from global fire models could be used in a similar way. For example, global fire models driven by different scenarios of climate change could provide information about potential changes in fire frequency, burned area and fire intensity. These outputs could then be used in economic modelling, as the environmental constraints for ABM modelling at a regional or local scale, or as what-if scenarios for local fire modelling. This can be illustrated by considering simulations of the response of burned area to changes in climate and land use under the SSP2-RCP4.5 scenario made with the CESM2-WACCM coupled climate model (Danabasoglu et al., 2020). By the middle of the 21st century (2040–2049), the model predicts a decrease in burnt area in western Africa although there is a substantial increase in burnt area in a limited area of north-central Africa (Figure 9A). In contrast, the model predicts a generalised increase in burnt area along the southern margin of Amazonia (Figure 9B). The projections of decreased burnt area in western Africa suggest that rigorous fire suppression policies may not be required as part of conservation measures to protect forested areas. On the other hand, the projected increase in burnt area in South America would have major economic and policy implications. These kind of scenario maps could also form the basis for exploring how local populations could adapt their current use of fire.
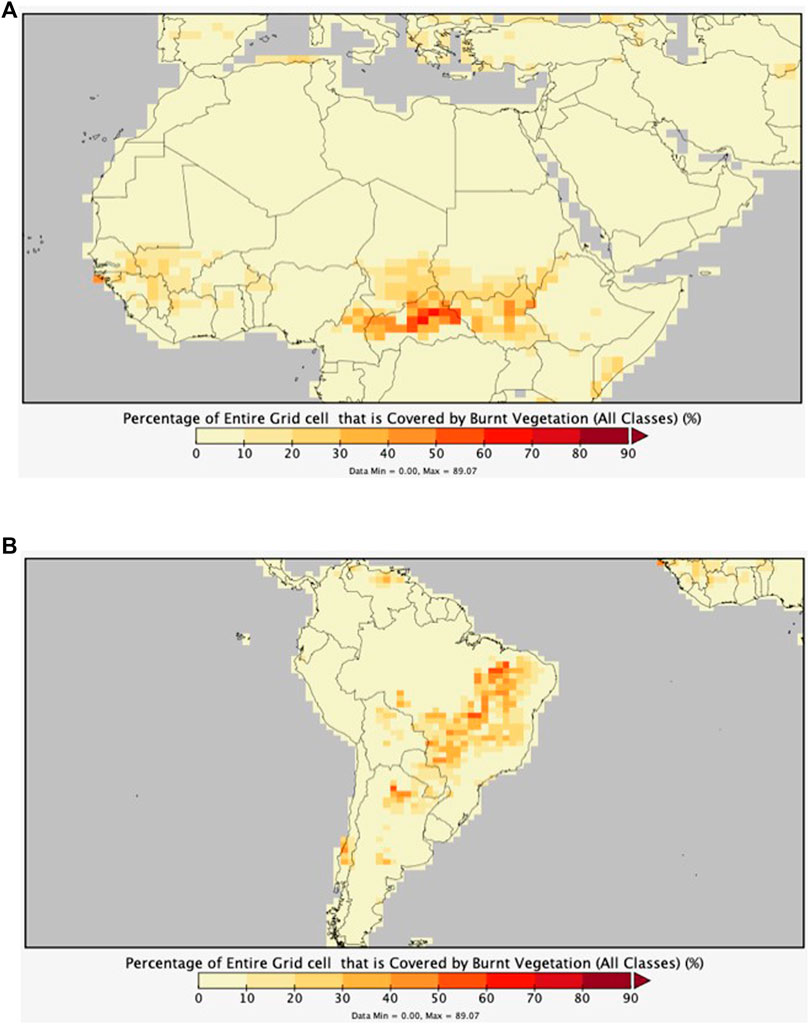
FIGURE 9. Simulated burned area by the middle of the 21st century (2040–2049) in response to the SSP2-RCP4.5 scenario made with the CESM2-WACCM coupled climate model, (A) for northern Africa, and (B) for South America.
Above all, the process of coupling different types of model will be a learning exercise, in part because it challenges narrow discipline-bound assumptions and in part because it provides the opportunity to assess the strengths and weaknesses of different approaches to understanding human-fire interactions (Antle et al., 2001; Voinov and Shugart, 2013). Coupling different types of models should facilitate detailed representation of individual elements of human-environment systems and also exploration of the links and feedbacks between those elements. However, there are technical, conceptual and semantic challenges in model coupling (Janssen et al., 2011), and these challenges are linked to important issues of model uncertainty and assessment (Millington et al., 2017). Semantic integration of models requires ensuring language, understanding, and perspectives on the entities and processes being modelled are shared between modellers from different disciplinary backgrounds. Conceptual integration requires ensuring alignment and consistency of concepts and units as information is passed between models, likely requiring conversion of units but also potentially concepts. Assessing the types and magnitudes of uncertainty introduced through model coupling will be an ongoing issue, although tools are available to assist in its management (e.g., Bastin et al., 2013). Coupling models of human activity with biophysical models to improve our understanding of fire regimes can benefit from the previous experience to couple models rooted in different scientific disciplines (e.g., Janssen et al., 2011; Voinov and Shugart, 2013; Calvin and Bond-Lamberty, 2018). Ultimately, model coupling should provide a stronger foundation for making predictions about future fire regimes and how these are influenced and will influence human actions. It will also provide a basis for the co-production of fire-related policies that take account of the aspirations of all sectors of society, thus promoting environmental justice.
Author Contributions
SH, AF, JayM, and JamM contributed to the conception and design of this study. SH and AF wrote the first draft of Introduction; JayM and CS drafted Place-Based Models of Fire Knowledge; JamM and OP drafted Agent-Based Models; YK drafted Economic Models of Wildfire; GR and TS drafted Wildfire Spread Models, SH and SR drafted Global Fire Models; KS and KY drafted Fire and Policy; SH drafted Issues and Commonalities and Moving Forward: Key Challenges and a Roadmap for Model Use and Integration. JamM and AF produced Figure 1; JamM and OP produced Figure 8; and SH and SR produced Figure 9. AF coordinated and edited the manuscript, and all authors contributed to the final version.
Funding
This research was funded by the Leverhulme Centre for Wildfires, Environment and Society through the Leverhulme Trust, grant number RC-2018-023. Additionally, the time of TS was funded by NERC grant number NE/T003553/1.
Conflict of Interest
The authors declare that the research was conducted in the absence of any commercial or financial relationships that could be construed as a potential conflict of interest.
Publisher’s Note
All claims expressed in this article are solely those of the authors and do not necessarily represent those of their affiliated organizations, or those of the publisher, the editors and the reviewers. Any product that may be evaluated in this article, or claim that may be made by its manufacturer, is not guaranteed or endorsed by the publisher.
Acknowledgments
We would like to thank our many colleagues from the Leverhulme Centre for Wildfires, Environment and Society and from the FURNACES project for fruitful discussions around this theme, and most especially Apostolos Voulgarakis and Matt Kasoar for discussions about the incorporation of ABMs in a climate modelling framework.
References
Alló, M., and Loureiro, M. L. (2020). Assessing Preferences for Wildfire Prevention Policies in Spain. For. Pol. Econ. 115, 102145. doi:10.1016/j.forpol.2020.102145
Amanor, K. S. (2002). Bushfire Management, Culture and Ecological Modernisation in Ghana. IDS Bull. – Dev. Stud. 33 (1), 65–74. doi:10.1111/j.1759-5436.2002.tb00008.x
Andela, N., Morton, D. C., Giglio, L., Chen, Y., van der Werf, G. R., Kasibhatla, P. S., et al. (2017). A Human-Driven Decline in Global Burned Area. Science 356, 1356–1362. doi:10.1126/science.aal4108
Angrist, J. D., Imbens, G. W., and Rubin, D. B. (1996). Identification of Causal Effects Using Instrumental Variables. J. Am. Stat. Assoc. 91, 444–455. doi:10.1080/01621459.1996.10476902
Ansell, J., Evans, J., Rangers, A., Rangers, A. S., Rangers, D., Rangers, J., et al. (2019). Contemporary Aboriginal savanna Burning Projects in Arnhem Land: a Regional Description and Analysis of the Fire Management Aspirations of Traditional Owners. Jounral Int. Assoc. Wildland Fire 29 (5), 371–385. doi:10.1071/WF18152
Antle, J. M., Capalbo, S. M., Elliott, E. T., Hunt, H. W., Mooney, S., and Paustian, K. H. (2001). Research Needs for Understanding and Predicting the Behavior of Managed Ecosystems: Lessons from the Study of Agroecosystems. Ecosystems 4, 723–735. doi:10.1007/s10021-001-0041-0
Archibald, S. (2016). Managing the Human Component of Fire Regimes: Lessons from Africa. Philos. T. R. Soc. B. 371, 20150346. doi:10.1098/rstb.2015.0346
Archibald, S., Lehmann, C. E. R., Gómez-Dans, J. L., and Bradstock, R. A. (2013). Defining Pyromes and Global Syndromes of Fire Regimes. Proc. Natl. Acad. Sci. 110 (16), 6442–6447. doi:10.1073/pnas.1211466110
Arroyo-Kalin, M. (2012). Slash-burn-and-churn: Landscape History and Crop Cultivation in Pre-columbian Amazonia. Quat. Int. 249 (6), 4–18. doi:10.1016/j.quaint.2011.08.004
Asner, G. P., Elmore, A. J., Olander, L. P., Martin, R. E., and Harris, A. T. (2004). Grazing Systems, Ecosystem Responses, and Global Change. Annu. Rev. Environ. Resour. 29, 261–299. doi:10.1146/annurev.energy.29.062403.102142
Barber, M., and Jackson, S. (2015). 'Knowledge Making': Issues in Modelling Local and Indigenous Ecological Knowledge. Hum. Ecol. 43 (1), 119–130. doi:10.1007/s10745-015-9726-4
Bastin, L., Cornford, D., Jones, R., Heuvelink, G. B. M., Pebesma, E., Stasch, C., et al. (2013). Managing Uncertainty in Integrated Environmental Modelling: The UncertWeb Framework. Environ. Model. Softw. 39, 116–134. doi:10.1016/j.envsoft.2012.02.008
Bayham, J., and Yoder, J. K. (2020). Resource Allocation under Fire. Land Econ. 96, 92–110. doi:10.3368/le.96.1.92
Bell, S., Berg, T., and Morse, S. (2016). Rich Pictures: Encouraging Resilient Communities. London: Routledge.
Berna, F., Goldberg, P., Horwitz, L. K., Brink, J., Holt, S., Bamford, M., et al. (2012). Microstratigraphic Evidence of In Situ Fire in the Acheulean Strata of Wonderwerk Cave, Northern Cape Province, South Africa. Proc. Natl. Acad. Sci. 109 (20), E1215–E1220. doi:10.1073/pnas.1117620109
Bert, F., North, M., Rovere, S., Tatara, E., Macal, C., and Podestá, G. (2015). Simulating Agricultural Land Rental Markets by Combining Agent-Based Models with Traditional Economics Concepts: The Case of the Argentine Pampas. Environ. Model. Softw. 71, 97–110. doi:10.1016/j.envsoft.2015.05.005
Berzaghi, F., Wright, I. J., Kramer, K., Oddou-Muratorio, S., Bohn, F. J., Reyer, C. P. O., et al. (2020). Towards a New Generation of Trait-Flexible Vegetation Models. Trends Ecol. Evol. 35, 191–205. doi:10.1016/j.tree.2019.11.006
Bilbao, B., Mistry, J., Millán, A., and Berardi, A. (2019). Sharing Multiple Perspectives on Burning: towards a Participatory and Intercultural Fire Management Policy in Venezuela, Brazil, and Guyana. Fire 2 (3), 39. doi:10.3390/fire2030039
Bithell, M., Brasington, J., and Richards, K. (2008). Discrete-element, Individual-Based and Agent-Based Models: Tools for Interdisciplinary Enquiry in Geography? Geoforum 39 (2), 625–642. doi:10.1016/j.geoforum.2006.10.014
Bond, W., and Zaloumis, N. P. (2016). The Deforestation story: Testing for Anthropogenic Origins of Africa's Flammable Grassy Biomes. Phil. Trans. R. Soc. B. 371, 20150170. doi:10.1098/rstb.2015.0170
Boustan, L. P., Kahn, M. E., Rhode, P. W., and Yanguas, M. L. (2020). The Effect of Natural Disasters on Economic Activity in US Counties: A century of Data. J. Urban Econ. 118, 103257. doi:10.1016/j.jue.2020.103257
Bowman, D. M. J. S., Balch, J., Artaxo, P., Bond, W. J., Cochrane, M. A., D’Antonio, C. M., et al. (2011). The Human Dimension of Fire Regimes on Earth. J. Biogeogr. 38 (12), 2223–2236. doi:10.1111/j.1365-2699.2011.02595.x
Bowman, D. M. J. S., Balch, J. K., Artaxo, P., Bond, W. J., Carlson, J. M., Cochrane, M. A., et al. (2009). Fire in the Earth System. Science 324, 481–484. doi:10.1126/science.1163886
Brändle, J., Langendijk, G., Peter, S., Brunner, S., and Huber, R. (2015). Sensitivity Analysis of a Land-Use Change Model with and without Agents to Assess Land Abandonment and Long-Term Re-forestation in a Swiss Mountain Region. Land 4 (2), 475–512. doi:10.3390/land4020475
Brando, P. M., Nepstad, D. C., Balch, J. K., Bolker, B., Christman, M. C., Coe, M., et al. (2012). Fire-induced Tree Mortality in a Neotropical forest: the Roles of Bark Traits, Tree Size, wood Density and Fire Behavior. Glob. Change Biol. 18, 630–641. doi:10.1111/j.1365-2486.2011.02533.x
Brown, C., Seo, B., and Rounsevell, M. (2019). Societal Breakdown as an Emergent Property of Large-Scale Behavioural Models of Land Use Change. Earth Syst. Dynam. 10, 809–845. doi:10.5194/esd-10-809-2019
Burton, C., Betts, R. A., Jones, C. D., and Williams, K. (2018). Will Fire Danger Be Reduced by Using Solar Radiation Management to Limit Global Warming to 1.5 °C Compared to 2.0 °C? Geophys. Res. Lett. 45, 3644–3652. doi:10.1002/2018GL077848
Butry, D. T., Mercer, D. E., Prestemon, J. P., Pye, J. M., and Holmes, T. P. (2001). What Is the price of Catastrophic Wildfire? J. For. 99, 9–17. doi:10.1093/jof/99.11.9
Caillault, S., Mialhe, F., Vannier, C., Delmotte, S., Kêdowidé, C., Amblard, F., et al. (2013). Influence of Incentive Networks on Landscape Changes: A Simple Agent-Based Simulation Approach. Environ. Model. Softw. 45, 64–73. doi:10.1016/j.envsoft.2012.11.003
Cairney, P. (2015). How Can Policy Theory Have an Impact on Policymaking? the Role of Theory-Led Academic-Practitioner Discussions. Teach. Public Adm. 33 (1), 22–39. doi:10.1177/0144739414532284
Calvin, K., and Bond-Lamberty, B. (2018). Integrated Human-Earth System Modeling—State of the Science and Future Direction. Environ. Res. Lett. 13, 063006. doi:10.1088/1748-9326/aac642
Cammelli, F., Garrett, R. D., Barlow, J., and Parry, L. (2020). Fire Risk Perpetuates Poverty and Fire Use Among Amazonian Smallholders. Glob. Environ. Change 63, 102096. doi:10.1016/j.gloenvcha.2020.102096
Campbell, R. M., and Anderson, N. M. (2019). Comprehensive Comparative Economic Evaluation of Woody Biomass Energy from Silvicultural Fuel Treatments. J. Environ. Manage. 250, 109422. doi:10.1016/j.jenvman.2019.109422
Carmenta, R., Zabala, A., Daeli, W., and Phelps, J. (2017). Perceptions across Scales of Governance and the Indonesian Peatland Fires. Glob. Environ. Change 46, 50–59. doi:10.1016/j.gloenvcha.2017.08.001
Carson, R. T. (2012). Contingent Valuation: A Practical Alternative when Prices Aren't Available. J. Econ. Perspect. 26, 27–42. doi:10.1257/jep.26.4.27
Carson, R. T., and Groves, T. (2007). Incentive and Informational Properties of Preference Questions. Environ. Resource Econ. 37, 181–210. doi:10.1007/s10640-007-9124-5
Castro, J., Drews, S., Exadaktylos, F., Foramitti, J., Klein, F., Konc, T., et al. (2020). A Review of Agent-Based Modeling of Climate-Energy Policy. Wires Clim. Change 11 (4), e647. doi:10.1002/wcc.647
Cecil, D. J., Buechler, D. E., and Blakeslee, R. J. (2014). Gridded Lightning Climatology from TRMM-LIS and OTD: Dataset Description. Atmos. Res. 135-136, 404–414. doi:10.1016/j.atmosres.2012.06.028
Champ, P. A., Meldrum, J. R., Brenkert-Smith, H., Warziniack, T. W., Barth, C. M., Falk, L. C., et al. (2020). Do actions Speak Louder Than Words? Comparing the Effect of Risk Aversion on Objective and Self-Reported Mitigation Measures. J. Econ. Behav. Organ. 169, 301–313. doi:10.1016/j.jebo.2019.11.019
Clarke, P. J., Lawes, M. J., Midgley, J. J., Lamont, B. B., Ojeda, F., Burrows, G. E., et al. (2013). Resprouting as a Key Functional Trait: How Buds, protection and Resources Drive Persistence after Fire. New Phytol. 197, 19–35. doi:10.1111/nph.12001
Cochrane, M. (2009). “Fire Land Use, Land Cover Dynamics, and Climate Change in the Brazillian Amazon,” in Tropical Fire Ecology: Climate Change, Land Use, and Ecosystem Dynamics. Editor M. Cochrane (Chichester: Praxis Publishing Ltd.), 389–426.
Cooke, B., and Kothari, U. (2001). Participation: The New Tyranny? London and New York: Zed Books Ltd.
Costafreda-Aumedes, S., Comas, C., and Vega-Garcia, C. (2017). Human-caused Fire Occurrence Modelling in Perspective: a Review. Int. J. Wildland Fire 26, 983–998. doi:10.1071/WF17026
Coughlan, M. R., and Petty, A. M. (2013). Fire as a Dimension of Historical Ecology: a Response to Bowmanet al.(2011). J. Biogeogr. 40 (5), 1010–1012. doi:10.1111/j.1365-2699.2012.02767.x
Coughlan, M. R., and Petty, A. M. (2012). Linking Humans and Fire: a Proposal for a Transdisciplinary Fire Ecology. Int. J. Wildland Fire 21 (5), 477–487. doi:10.1071/WF11048
Coughlan, M. R. (2015). Traditional Fire-Use, Landscape Transition, and the Legacies of Social Theory Past. Ambio 44 (8), 705–717. doi:10.1007/s13280-015-0643-y
Crane, T. A. (2010). Of Models and Meanings: Cultural Resilience in Social–Ecological Systems. Ecol. Soc. 15 (4), 19. doi:10.5751/es-03683-150419
Crippa, P., Castruccio, S., Archer-Nicholls, S., Lebron, G. B., Kuwata, M., Thota, A., et al. (2016). Population Exposure to Hazardous Air Quality Due to the 2015 Fires in Equatorial Asia. Sci. Rep. 6, 37074. doi:10.1038/srep37074
Danabasoglu, G., Lamarque, J.-F., Bacmeister, J., Bailey, D. A., DuVivier, A. K., Edwards, J., et al. (2020). The Community Earth System Model Version 2 (CESM2). J. Adv. Model. Earth Sy. 12, e2019MS001916. doi:10.1029/2019MS001916
Dara, A., Baumann, M., Hölzel, N., Hostert, P., Kamp, J., Müller, D., et al. (2019). Post-soviet Land-Use Change Affected Fire Regimes on the Eurasian Steppes. Ecosystems 23, 943–956. doi:10.1007/s10021-019-00447-w
Davis, A., and Wagner, J. R. (2003). Who Knows? on the Importance of Identifying “Experts” when Researching Local Ecological Knowledge. Hum. Ecol. 31 (3), 463–489. doi:10.1023/a:1025075923297
Delgado, R., Gonzalez, J.-L., Sotoca, A., and Tibau, X.-A. (2018). “Archetypes of Wildfire Arsonists: An Approach by Using Bayesian Networks,” in Forest Fire. Editor J. Szmyt (London: IntechOpen). doi:10.5772/intechopen.72615
Devisscher, T., Boyd, E., and Malhi, Y. (2016). Anticipating Future Risk in Social-Ecological Systems Using Fuzzy Cognitive Mapping: the Case of Wildfire in the Chiquitania, Bolivia. Ecol. Soc. 21 (4), 18. doi:10.5751/ES-08599-210418
Devisscher, T., Malhi, Y., and Boyd, E. (2019). Deliberation for Wildfire Risk Management: Addressing Conflicting Views in the Chiquitania, Bolivia. Geogr. J. 185 (1), 38–54. doi:10.1111/geoj.12261
Diallo, I., Giorgi, F., and Stordal, F. (2018). Influence of Lake Malawi on Regional Climate from a Double-Nested Regional Climate Model experiment. Clim. Dyn. 50, 3397–3411. doi:10.1007/s00382-017-3811-x
Diamond, P. A., and Hausman, J. A. (1994). Contingent Valuation: Is Some Number Better Than No Number? J. Econ. Perspect. 8 (4), 45–64. doi:10.1257/jep.8.4.45
Díaz, S., Kattge, J., Cornelissen, J. H. C., Wright, I. J., Lavorel, S., Dray, S., et al. (2015). The Global Spectrum of Plant Form and Function. Nature 529, 167–171. doi:10.1038/nature16489
Doerr, S. H., and Santín, C. (2016). Global Trends in Wildfire and its Impacts: Perceptions versus Realities in a Changing World. Phil. Trans. R. Soc. B. 371, 20150345. doi:10.1098/rstb.2015.0345
Donovan, G. H., Champ, P. A., and Butry, D. T. (2007). Wildfire Risk and Housing Prices: A Case Study from Colorado Springs. Land Econ. 83, 217–233. doi:10.3368/le.83.2.217
Dressler, W. H., Smith, W., Kull, C. A., Carmenta, R., and Pulhin, J. M. (2020). Recalibrating Burdens of Blame: Anti-swidden Politics and green Governance in the Philippine Uplands. Geoforum 124, 348. doi:10.1016/j.geoforum.2020.01.024
Düspohl, M., Frank, S., and Döll, P. (2012). A Review of Bayesian Networks as a Participatory Modeling Approach in Support of Sustainable Environmental Management. J. Sust. Dev. 5, 1–18. doi:10.5539/jsd.v5n12p1:
Edmonds, B. (2018). The "formalist Fallacy". Review of Artificial Societies and Social Simulation. 11th June 2018 Available at: https://rofasss.org/2018/07/20/be/. (Accessed December 16, 2020).
Ellwanger, J. H., Kulmann-Leal, B., Kaminski, V. L., Valverde-Villegas, J. M., Veiga, A. B. G. D., Spilki, F. R., et al. (2020). Beyond Diversity Loss and Climate Change: Impacts of Amazon Deforestation on Infectious Diseases and Public Health. Acad. Bras Cienc 92 (1), e20191375. doi:10.1590/0001-3765202020191375
Eloy, L., A. Bilbao, B., Mistry, J., and Schmidt, I. B. (2018). From Fire Suppression to Fire Management: Advances and Resistances to Changes in Fire Policy in the Savannas of Brazil and Venezuela. Geogr. J. 185 (1), 10–22. doi:10.1111/geoj.12245
Eloy, L., Hecht, S., Steward, A., and Mistry, J. (2019). Firing up: Policy, Politics and Polemics under New and Old Burning Regimes. Geogr. J. 185 (1), 2–9. doi:10.1111/geoj.12293
Eriksen, C. (2007). Why Do They Burn the ?bush?? Fire, Rural Livelihoods, and Conservation in Zambia. Geographical J. 173 (3), 242–256. doi:10.1111/j.1475-4959.2007.00239.x
Exbrayat, J. F., Smallman, T. L., Bloom, A. A., Hutley, L. B., and Williams, M. (2017). Inverse Determination of the Influence of Fire on Vegetation Carbon Turnover in the Pantropics. Glob. Biogeochem. Cy. 32, 1776–1789. doi:10.1029/2018GB005925
Fernandes, P., Rossa, C., Madrigal, J., and Rigolot, E. (2016). “Updated State-Of-The-Art on the Uses of Prescribed Burning,”. Technical Report. Vila Real: UTAD doi:10.13140/RG.2.2.31837.82400
Finney, M. A. (1998). “FARSITE: Fire Area Simulator-Model Development and Evaluation,”. Res. Pap. RMRS-RP-4, Revised 2004 (Ogden, UT: U.S. Department of Agriculture, Forest Service, Rocky Mountain Research Station). doi:10.2737/RMRS-RP-4
Forkel, M., Andela, N., Harrison, S. P., Lasslop, G., van Marle, M., Chuvieco, E., et al. (2019b). Emergent Relationships with Respect to Burned Area in Global Satellite Observations and Fire-Enabled Vegetation Models. Biogeosciences 16, 57–76. doi:10.5194/bg-16-57-2019
Forkel, M., Dorigo, W., Lasslop, G., Chuvieco, E., Hantson, S., Heil, A., et al. (2019a). Recent Global and Regional Trends in Burned Area and Their Causes. Environ. Res. Comm. 1, 051005. doi:10.1088/2515-7620/ab25d2
Forkel, M., Dorigo, W., Lasslop, G., Teubner, I., Chuvieco, E., and Thonicke, K. (2017). A Data-Driven Approach to Identify Controls on Global Fire Activity from Satellite and Climate Observations (SOFIA V1). Geosci. Model. Dev. 10 (12), 4443–4476. doi:10.5194/gmd-10-4443-2017
Franklin, O., Harrison, S. P., Dewar, R., Farrior, C. E., Brännström, Å., Dieckmann, U., et al. (2020). Organizing Principles for Vegetation Dynamics. Nat. Plants 6, 444–453. doi:10.1038/s41477-020-0655-x
Freeman, M., Herriges, J. A., and Kling, C. L. (2014). The Measurement of Environmental and Resource Values: Theory and Methods. 3rd ed. Oxford: Routledge.
Frieler, K., Lange, S., Piontek, F., Reyer, C. P. O., Schewe, J., Warszawski, L., et al. (2017). Assessing the Impacts of 1.5 °C Global Warming - Simulation Protocol of the Inter-sectoral Impact Model Intercomparison Project (ISIMIP2b). Geosci. Model. Dev. 10, 4321–4345. doi:10.5194/gmd-10-4321-2017
Fyllas, N. M., Gloor, E., Mercado, L. M., Sitch, S., Quesada, C. A., Domingues, T. F., et al. (2014). Analysing Amazonian forest Productivity Using a New Individual and Trait-Based Model (TFS v.1). Geosci. Model. Dev. 7, 1251–1269. doi:10.5194/gmd-7-1251-2014
Giorgi, F. (2019). Thirty Years of Regional Climate Modeling: Where Are We and where Are We Going Next? J. Geophys. Res.-Atmos 124, 5696–5723. doi:10.1029/2018JD030094
González-Hidalgo, M., Otero, I., and Kallis, G. (2014). Seeing beyond the smoke: the political ecology of fire in Horta de Sant Joan (Catalonia). Environ. Plan. A. 46 (5), 1014–1031. doi:10.1068/a45600
Goren-Inbar, N., Alperson, N., Kislev, M. E., Simchoni, O., Melamed, Y., Ben-Nun, A., et al. (2004). Evidence of Hominin Control of Fire at Gesher Benot Ya`aqov, Israel. Science 304 (5671), 725–727. doi:10.1126/science.1095443
Grimm, V., Revilla, E., Berger, U., Jeltsch, F., Mooij, W. M., Railsback, S. F., et al. (2005). Pattern-oriented Modeling of Agent-Based Complex Systems: Lessons from Ecology. Science 310 (5750), 987–991. doi:10.1126/science.1116681
Groeneveld, J., Müller, B., Buchmann, C. M., Dressler, G., Guo, C., Hase, N., et al. (2017). Theoretical Foundations of Human Decision-Making in Agent-Based Land Use Models - A Review. Environ. Model. Softw. 87, 39–48. doi:10.1016/j.envsoft.2016.10.008
Haberl, H., Erb, K. H., Krausmann, F., Gaube, V., Bondeau, A., Plutzar, C., et al. (2007). Quantifying and Mapping the Human Appropriation of Net Primary Production in Earth's Terrestrial Ecosystems. Proc. Natl. Acad. Sci. 104 (31), 12942–12947. doi:10.1073/pnas.0704243104
Hanemann, W. M. (1994). Valuing the Environment through Contingent Valuation. J. Econ. Perspect. 8, 19–43. doi:10.1257/jep.8.4.19
Hantson, S., Arneth, A., Harrison, S. P., Kelley, D. I., Prentice, I. C., Rabin, S. S., et al. (2016). The Status and challenge of Global Fire Modelling. Biogeosciences 13, 3359–3375. doi:10.5194/bg-13-3359-2016
Hantson, S., Kelley, D. I., Arneth, A., Harrison, S. P., Archibald, S., Bachelet, D., et al. (2020). Quantitative Assessment of Fire and Vegetation Properties in Simulations with Fire-Enabled Vegetation Models from the Fire Model Intercomparison Project. Geosci. Model. Dev. 13, 3299–3318. doi:10.5194/gmd-13-3299-2020
Harrison, S. P., Bartlein, P. J., Brovkin, V., Houweling, S., Kloster, S., and Prentice, I. C. (2018). The Biomass Burning Contribution to Climate-Carbon-Cycle feedbackBiomass Burning Contribution to Global Climate-Carbon Cycle Feedback. Earth Syst. Dynam. 9, 663–677. doi:10.5194/esd-9-663-2018
Harrison, S. P., Cramer, W., Franklin, O., Prentice, I. C., Wang, H., Brännström, Å., et al. (2021). Eco‐evolutionary Optimality as a Means to Improve Vegetation and Land‐surface Models. New Phytol. 231 (6), 2125–2141. doi:10.1111/nph.17558
Harrison, S. P., Prentice, I. C., Barboni, D., Kohfeld, K. E., Ni, J., and Sutra, J.-P. (2010). Ecophysiological and Bioclimatic Foundations for a Global Plant Functional Classification. J. Veg. Sci. 21, 300–317. doi:10.1111/j.1654-1103.2009.01144.x
Hausman, J. (2012). Contingent Valuation: From Dubious to Hopeless. J. Econ. Perspect. 26, 43–56. doi:10.1257/jep.26.4.43
He, T., Lamont, B. B., and Pausas, J. G. (2019). Fire as a Key Driver of Earth's Biodiversity. Biol. Rev. 94, 1983–2010. doi:10.1111/brv.12544
Heinimann, A., Mertz, O., Frolking, S., Egelund Christensen, A., Hurni, K., Sedano, F., et al. (2017). A Global View of Shifting Cultivation: Recent, Current, and Future Extent. PLoS ONE 12, e0184479. doi:10.1371/journal.pone.0184479
Hesseln, H., Loomis, J. B., González-Cabán, A., and Alexander, S. (2003). Wildfire Effects on Hiking and Biking Demand in New Mexico: a Travel Cost Study. J. Environ. Manage. 69, 359–368. doi:10.1016/j.jenvman.2003.09.012
Holzhauer, S., Brown, C., and Rounsevell, M. (2018). Modelling Dynamic Effects of Multi-Scale Institutions on Land Use Change. Reg. Environ. Change 19, 733–746. doi:10.1007/s10113-018-1424-5
Huber, R., Bakker, M., Balmann, A., Berger, T., Bithell, M., Brown, C., et al. (2018). Representation of Decision-Making in European Agricultural Agent-Based Models. Agric. Syst. 167, 143–160. doi:10.1016/j.agsy.2018.09.007
Hulse, D., Branscomb, A., Enright, C., Johnson, B., Evers, C., Bolte, J., et al. (2016). Anticipating surprise: Using Agent-Based Alternative Futures Simulation Modeling to Identify and Map Surprising Fires in the Willamette Valley, Oregon USA. Landscape Urban Plann. 156, 26–43. doi:10.1016/j.landurbplan.2016.05.012
Humphrey, G. J., Gillson, L., and Ziervogel, G. (2020). How Changing Fire Management Policies Affect Fire Seasonality and Livelihoods. Ambio 50, 1–17. doi:10.1007/s13280-020-01351-7
Janssen, S., Athanasiadis, I. N., Bezlepkina, I., Knapen, R., Li, H., Domínguez, I. P., et al. (2011). Linking Models for Assessing Agricultural Land Use Change. Comput. Elect. Agric. 76 (2), 148–160. doi:10.1016/j.compag.2010.10.011
Jayachandran, S. (2009). Air Quality and Early-Life Mortality: Evidence from Indonesia's Wildfires. J. Hum. Resour. 44, 916–954. doi:10.3368/jhr.44.4.91610.1353/jhr.2009.0001
Johansson, M., Senay, S., Creathorn, E., Kassa, H., and Hylander, K. (2019). Change in Heathland Fire Sizes inside vs. Outside the Bale Mountains National Park, Ethiopia, over 50 Years of Fire-Exclusion Policy: Lessons for REDD+. Ecol. Soc. 24 (4), 26. doi:10.5751/ES-11260-240426
Johnston, F. H., Henderson, S. B., Chen, Y., Randerson, J. T., Marlier,Defries, M. R. S., DeFries, R. S., et al. (2012). Estimated Global Mortality Attributable to Smoke from Landscape Fires. Environ. Health Perspect. 120 (5), 695–701. doi:10.1289/ehp.1104422
Jolly, W. M., Cochrane, M. A., Freeborn, P. H., Holden, Z. A., Brown, T. J., Williamson, G. J., et al. (2015). Climate-induced Variations in Global Wildfire Danger from 1979 to 2013fire Danger from 1979 to 2013. Nat. Commun. 6, 7537. doi:10.1038/ncomms8537
Jones, M. W., Smith, A., Betts, R., Canadell, J. G., Prentice, I. C., and Le Quéré, C. (2020). Climate Change Increases the Risk of Wildfire. Sci. Brief. Available at: www.sciencebrief.org/briefs/wildfires. (Accessed December 14, 2020).
Jones, N. A., Ross, H., Lynam, T., Perez, P., and Leitch, A. (2011). Mental Models: an Interdisciplinary Synthesis of Theory and Methods. Ecol. Soc. 16 (1), 46. doi:10.5751/es-03802-160146
Karkanas, P., Shahackgross, R., Ayalon, A., Barmatthews, M., Barkai, R., Frumkin, A., et al. (2007). Evidence for Habitual Use of Fire at the End of the Lower Paleolithic: Site-Formation Processes at Qesem Cave, Israel. J. Hum. Evol. 53, 197–212. doi:10.1016/j.jhevol.2007.04.002
Kelly, L. T., Giljohann, K. M., Duane, A., Aquilué, N., Archibald, S., Batllori, E., et al. (2020). Fire and Biodiversity in the Anthropocene. Science 370, eabb0355. doi:10.1126/science.abb0355
Khatun, K., Corbera, E., and Ball, S. (2017). Fire Is REDD+: Offsetting Carbon through Early Burning Activities in South-Eastern Tanzania. Oryx 51 (1), 43–52. doi:10.1017/S0030605316000090
Kloster, S., and Lasslop, G. (2017). Historical and Future Fire Occurrence (1850 to 2100) Simulated in CMIP5 Earth System Models. Glob. Planet. Change 150, 58–69. doi:10.1016/j.gloplacha.2016.12.017
Kloster, S., Mahowald, N. M., Randerson, J. T., Thornton, P. E., Hoffman, F. M., Levis, S., et al. (2010). Fire Dynamics during the 20th century Simulated by the Community Land Model. Biogeosciences 7 (6), 1877–1902. doi:10.5194/bg-7-1877-2010
Knorr, W., Arneth, A., and Jiang, L. (2016). Demographic Controls of Future Global Fire Risk. Nat. Clim Change 6, 781–785. doi:10.1038/NCLIMATE2999
Kountouris, Y. (2020). Human Activity, Daylight Saving Time and Wildfire Occurrence. Sci. Total Environ. 727, 138044. doi:10.1016/j.scitotenv.2020.138044
Krawchuk, M. A., Moritz, M. A., Parisien, M. A., Van Dorn, J., and Hayhoe, K. (2009). Global Pyrogeography: the Current and Future Distribution of Wildfire. PLOS ONE 4 (4), e5102. doi:10.1371/journal.pone.0005102
Lambin, E. F., Meyfroidt, P., Rueda, X., Blackman, A., Börner, J., Cerutti, P. O., et al. (2014). Effectiveness and Synergies of Policy Instruments for Land Use Governance in Tropical Regions. Glob. Environ. Change 28, 129–140. doi:10.1016/j.gloenvcha.2014.06.007
Lane, D. C. (2000). Diagramming Conventions in System Dynamics. J. Oper. Res. Soc. 51 (2), 241–245. doi:10.1057/palgrave.jors.2600864
Laris, P. (2002). Burning the Seasonal Mosaic: Preventative Burning Strategies in the Wooded Savanna of Southern Mali. Hum. Ecol. 30, 155–186. doi:10.1023/a:1015685529180
Laris, P., and Wardell, D. A. (2006). Good, Bad or 'necessary Evil'? Reinterpreting the Colonial Burning Experiments in the savanna Landscapes of West Africa. Geographical J. 172 (4), 271–290. doi:10.1111/j.1475-4959.2006.00215.x
Latham, D. J., and Schlieter, J. A. (1989). “Ignition Probabilities of Wildland Fuels Based on Simulated Lightning Discharges,”. Research Paper INT-411 (Ogden, UT: Intermountain Research Station).
Lauk, C., and Erb, K. H. (2016). “A Burning Issue: Anthropogenic Vegetation Fires,” in Social Ecology. Human-Environment Interactions. Editors H. Haberl, M. Fischer-Kowalski, F. Krausmann, and V. Winiwarter (Springer, Cham), Vol. 5. doi:10.1007/978-3-319-33326-7_15
Lavorel, S., Flannigan, M. D., Lambin, E. F., and Scholes, M. C. (2007). Vulnerability of Land Systems to Fire: Interactions Among Humans, Climate, the Atmosphere, and Ecosystems. Mitig. Adapt. Strat. Glob. Change 12, 33–53. doi:10.1007/s11027-006-9046-5
Leach, M., and Scoones, I. (2015). “Political Ecologies of Carbon in Africa,” in Carbon Conflicts and Forest Landscapes in Africa. Editors M. Leach, and I. Scoones (Abingdon, UK: Routledge), 1–42.
Lee, J. S., Filatova, T., Ligmann-Zielinska, A., Hassani-Mahmooei, B., Stonedahl, F., Lorscheid, I., et al. (2015). The Complexities of Agent-Based Modeling Output Analysis. Jasss- J. Artif. Soc. S 18 (4), 1–4. doi:10.18564/jasss.2897
Levin, S., Xepapadeas, T., Crépin, A.-S., Norberg, J., De Zeeuw, A., Folke, C., et al. (2013). Social-ecological Systems as Complex Adaptive Systems: Modeling and Policy Implications. Envir. Dev. Econ. 18 (2), 111–132. doi:10.1017/S1355770X12000460
Li, A., and Ford, J. (2019). Understanding Socio-Ecological Vulnerability to Climatic Change through a Trajectories of Change Approach: a Case Study from an Indigenous Community in Panama. Weather Clim. Soc. 11 (3), 577–593. doi:10.1175/WCAS-D-18-0093.1
Li, F., Levis, S., and Ward, D. S. (2013). Quantifying the Role of Fire in the Earth System - Part 1: Improved Global Fire Modeling in the Community Earth System Model (CESM1). Biogeosciences 10, 2293–2314. doi:10.5194/bg-10-2293-2013
Liao, Y., and Kousky, C. (2020). The Fiscal Impacts of Wildfires on California Municipalities. Rochester: SSRN. doi:10.2139/ssrn.3612311
Liu, J. C., Pereira, G., Uhl, S. A., Bravo, M. A., and Bell, M. L. (2015). A Systematic Review of the Physical Health Impacts from Non-occupational Exposure to Wildfire Smoke. Environ. Res. 136, 120–132. doi:10.1016/j.envres.2014.10.015
Liu, Y., Goodrick, S., and Heilman, W. (2014). Wildland Fire Emissions, Carbon, and Climate: Wildfire-Climate Interactions. For. Ecol. Manag. 317 (1), 80–96. doi:10.1016/j.foreco.2013.02.020
Lizundia-Loiola, J., Otón, G., Ramo, R., and Chuvieco, E. (2020a). A Spatio-Temporal Active-Fire Clustering Approach for Global Burned Area Mapping at 250 m from MODIS Data. Remote Sensing Environ. 236, 111493. doi:10.1016/j.rse.2019.111493
Lizundia-Loiola, J., Pettinari, M. L., and Chuvieco, E. (2020b). Temporal Anomalies in Burned Area Trends: Satellite Estimations of the Amazonian 2019 Fire Crisis. Remote Sensing 12, 151. doi:10.3390/rs12010151
Loomis, J. B., Le, H. T., and Gonzales-Caban, A. (2005). Testing Transferability of Willingness to Pay for forest Fire Prevention Among Three States of California, Florida and Montana. Jfe 11, 125–140. doi:10.1016/j.jfe.2005.07.003
Loomis, J. B., Le Trong Hung, L. T., and González-Cabán, A. (2009). Willingness to Pay Function for Two Fuel Treatments to Reduce Wildfire Acreage Burned: A Scope Test and Comparison of White and Hispanic Households. For. Pol. Econ. 11, 155–160. doi:10.1016/j.forpol.2008.10.006
Mace, G., Schreckenberg, K., and Poudyal, M. (2018). “Ecosystem Services for Human Wellbeing: Trade-Offs and Governance,” in Ecosystem Services and Poverty Alleviation: Trade-Offs and Governance. Editors K. Schreckenberg, G. Mace, and M. Poudyal (Abingdon: Routledge).
Magliocca, N. R., van Vliet, J., Brown, C., Evans, T. P., Houet, T., Messerli, P., et al. (2015). From Meta-Studies to Modeling: Using Synthesis Knowledge to Build Broadly Applicable Process-Based Land Change Models. Environ. Model. Softw. 72, 10–20. doi:10.1016/j.envsoft.2015.06.009
Mahmoud, H., and Chulahwat, A. (2018). Unraveling the Complexity of Wildland Urban Interface Fires. Sci. Rep. 8, 9315. doi:10.1038/s41598-018-27215-5
Malek, Ž., Douw, B., Van Vliet, J., Van Der Zanden, E. H., and Verburg, P. H. (2019). Local Land-Use Decision-Making in a Global Context. Environ. Res. Lett. 14, 083006. doi:10.1088/1748-9326/ab309e
Malek, Ž., and Verburg, P. H. (2020). Mapping Global Patterns of Land Use Decision-Making. Glob. Environ. Change 65, 102170. doi:10.1016/j.gloenvcha.2020.102170
Mandel, J., Beezley, J. D., and Kochanski, A. K. (2011). Coupled Atmosphere-Wildland Fire Modeling with WRF 3.3 and SFIRE 2011. Geosci. Model. Dev. 4, 591–610. doi:10.5194/gmd-4-591-2011
Mann, M. E., Zhang, Z., Hughes, M. K., Bradley, R. S., Miller, S. K., Rutherford, S., et al. (2008). Proxy-based Reconstructions of Hemispheric and Global Surface Temperature Variations over the Past Two Millennia. Proc. Natl. Acad. Sci. 105 (36), 13252–13257. doi:10.1073/pnas.0805721105
Manson, S., An, L., Clarke, K. C., Heppenstall, A., Koch, J., Krzyzanowski, B., et al. (2020). Methodological Issues of Spatial Agent-Based Models. Jasss- J. Artif. Soc. S. 23 (1), 3. doi:10.18564/jasss.4174
McCoy, S. J., and Walsh, R. P. (2018). Wildfire Risk, Salience & Housing Demand. J. Environ. Econ. Manag. 91, 203–228. doi:10.1016/j.jeem.2018.07.005
McKemey, M., Ens, E., Rangers, Y. M., Costello, O., and Reid, N. (2020). Indigenous Knowledge and Seasonal Calendar Inform Adaptive savanna Burning in Northern Australia. Sustainability 12, 995. doi:10.3390/su12030995
Medrilzam, M., Dargusch, P., Herbohn, J., and Smith, C. (2014). The Socio-Ecological Drivers of forest Degradation in Part of the Tropical Peatlands of Central Kalimantan, Indonesia. Forestry 87 (2), 335–345. doi:10.1093/forestry/cpt033
Mell, W., Maranghides, A., McDermott, R., and Manzello, S. L. (2009). Numerical Simulation and Experiments of Burning douglas Fir Trees. Combustion and Flame 156, 2023–2041. doi:10.1016/j.combustflame.2009.06.015
Michetti, M., and Pinar, M. (2019). Forest Fires across Italian Regions and Implications for Climate Change: A Panel Data Analysis. Environ. Resource Econ. 72, 207–246. doi:10.1007/s10640-018-0279-z
Miller, C., Hilton, J., Sullivan, A., and Prakash, M. (2015). “SPARK–A Bushfire Spread Prediction Tool,” in Environmental Software Systems. Infrastructures, Services and Applications, ISESS 2015, IFIP Advances in Information and Communication Technology. Editors R. Denzer, R. M. Argent, G. Schimak, and J. Hřebíček (Springer, Cham), 448. doi:10.1007/978-3-319-15994-2_26
Millington, J. D. A., O’Sullivan, D., and Perry, G. L. W. (2012). Model Histories: Narrative Explanation in Generative Simulation Modelling. Geoforum 43 (6), 1025–1034. doi:10.1016/j.geoforum.2012.06.017
Millington, J. D. A., Romero-Calcerrada, R., Wainwright, J., and Perry, G. L. W. (2008). An Agent-Based Model of Mediterranean Agricultural Land-Use/cover Change for Examining Wildfire Risk. Jasss-j. Artif. Soc. S. 11 (4), 4.
Millington, J., Xiong, H., Peterson, S., and Woods, J. (2017). Integrating Modelling Approaches for Understanding Telecoupling: Global Food Trade and Local Land Use. Land 6 (3), 56. doi:10.3390/land6030056
Mistry, J., Berardi, A., Andrade, V., Krahô, T., Krahô, P., and Leonardos, O. (2005). Indigenous Fire Management in the Cerrado of Brazil: The Case of the Krahô of Tocantíns. Hum. Ecol. 33 (3), 365–386. doi:10.1007/s10745-005-4143-8
Mistry, J., Schmidt, I. B., Eloy, L., and Bilbao, B. (2019). New Perspectives in Fire Management in South American Savannas: The Importance of Intercultural Governance. Ambio 48 (2), 172–179. doi:10.1007/s13280-018-1054-7
Moeltner, K., Kim, M.-K., Zhu, E., and Yang, W. (2013). Wildfire Smoke and Health Impacts: A Closer Look at Fire Attributes and Their Marginal Effects. J. Environ. Econ. Manag. 66, 476–496. doi:10.1016/j.jeem.2013.09.004
Molina, J. R., Zamora, R., and Rodríguez y Silva, F. (2019). Rodríguez Y Silva, FThe Role of Flagship Species in the Economic Valuation of Wildfire Impacts: An Application to Two Mediterranean Protected Areas. Sci. Total Environ. 675, 520–530. doi:10.1016/j.scitotenv.2019.04.242
Monzón-Alvarado, C., Waylen, P., and Keys, E. (2014). Fire Management and Climate Variability: Challenges in Designing Environmental Regulations. Land Use Policy 39, 12–21. doi:10.1016/j.landusepol.2014.03.003
Moritz, M. A., Morais, M. E., Summerell, L. A., Carlson, J. M., and Doyle, J. (2005). Wildfires, Complexity, and Highly Optimized Tolerance. Proc. Natl. Acad. Sci. 102 (50), 17912–17917. doi:10.1073/pnas.0508985102
Mueller, J. M., Soder, A. B., and Springer, A. E. (2019). Valuing Attributes of forest Restoration in a Semi-arid Watershed. Landscape Urban Plann. 184, 78–87. doi:10.1016/j.landurbplan.2018.12.012
Murdiyarso, D., and Adiningsih, E. S. (2007). Climate Anomalies, Indonesian Vegetation Fires and Terrestrial Carbon Emissions. Mitig. Adapt. Strat. Gl. 12, 101–112. doi:10.1007/s11027-006-9047-4
Neale, T., and May, D. (2018). Bushfire Simulators and Analysis in Australia: Insights into an Emerging Sociotechnical Practice. Environ. Hazards 17, 200–218. doi:10.1080/17477891.2017.1410462
Nobel, A., Lizin, S., Witters, N., Rineau, F., and Malina, R. (2020). The Impact of Wildfires on the Recreational Value of Heathland: A Discrete Factor Approach with Adjustment for On-Site Sampling. J. Environ. Econ. Manag. 101, 102317. doi:10.1016/j.jeem.2020.102317
Nunan, F., Menton, M., McDermott, C., and Schreckenberg, K. (2018). ““Governing for Ecosystem Health and Human Wellbeing”,” in Ecosystem Services and Poverty Alleviation: Trade-Offs and Governance. Editors K. Schreckenberg, G. Mace, and M. Poudyal (Abingdon: Routledge).
O’Neill, B. C., Kriegler, E., Ebi, K. L., Kemp-Benedict, E., Riahi, K., Rothman, D. S., et al. (2017). The Roads Ahead: Narratives for Shared Socioeconomic Pathways Describing World Futures in the 21st century,. Glob. Environ. Change 42, 169–180. doi:10.1016/j.gloenvcha.2015.01.004
O’Sullivan, D., Millington, J., Perry, G., and Wainwright, J. (2012). “Agent-based Models–Because They’re worth it?” in Agent-Based Models of Geographical Systems. Editors A. Heppenstall, A. Crooks, L.M. See, and M. Batty (Dordrecht: Springer).:
Özesmi, U., and Özesmi, S. L. (2004). Ecological Models Based on People’s Knowledge: a Multi-step Fuzzy Cognitive Mapping Approach. Ecol. Model. 176 (1-2), 43–64. doi:10.1016/j.ecolmodel.2003.10.027
Papakosta, P., Xanthopoulos, G., and Straub, D. (2017). Probabilistic Prediction of Wildfire Economic Losses to Housing in Cyprus Using Bayesian Network Analysis. Int. J. Wildland Fire 26 (1), 10. doi:10.1071/WF15113
Parisien, M.-A., Kafka, V., Hirsch, K., Todd, J., Lavoie, S., and Maczek, P. (2005). “Mapping Wildfire Susceptibility with the BURN-P3 Simulation Model,”. Information Report NOR-X-405 (Natural Resources Canada. Edmonton, Alberta: Northern Forestry Centre).
Parker, D. C., Manson, S. M., Janssen, M. A., Hoffmann, M. J., and Deadman, P. (2003). Multi-agent Systems for the Simulation of Land-Use and Land-Cover Change: a Review. Ann. Assoc. Am. Geogr. 93 (2), 314–337. doi:10.1111/1467-8306.9302004
Pausas, J. G., Bradstock, R. A., Keith, D. A., and Keeley, J. E. (2004). Plant Functional Traits in Relation to Fire in Crown-Fire Ecosystems. Ecology 85, 1085–1100. doi:10.1890/02-4094
Pausas, J. G., Keeley, J. E., and Schwilk, D. W. (2017). Flammability as an Ecological and Evolutionary Driver. J. Ecol. 105, 289–297. doi:10.1111/1365-2745.12691
Pausas, J. G., and Keeley, J. E. (2019). Wildfires as an Ecosystem Service. Front. Ecol. Environ. 17 (5), 289–295. doi:10.1002/fee.2044
Pearce, H. (2009). “Review of Fire Growth Simulation Models for Application in New Zealand Client,”. Report, 16246 (Christchurch, NZ: Scion Fire Research Group).
Perkins, O., Smith, C., and Millington, J. D. A. (2021). Human-Fire Interactions: A Global Database. Seattle, USA: American Association of Geographers Annual Meeting (AAG). doi:10.5281/zenodo.4661182
Pfeiffer, M., Spessa, A., and Kaplan, J. O. (2013). A Model for Global Biomass Burning in Preindustrial Time: LPJ-LMfire (v1.0). Geosci. Model. Dev. 6, 643–685. doi:10.5194/gmd-6-643-2013
Phelps, J., Friess, D. A., and Webb, E. L. (2012). Win-win REDD+ Approaches Belie Carbon-Biodiversity Trade-Offs. Biol. Conservation 154, 53–60. doi:10.1016/j.biocon.2011.12.031
Phelps, J., Webb, E. L., and Agrawal, A. (2010). Does REDD+ Threaten to Recentralize forest Governance? Science 328, 312–313. doi:10.1126/science.1187774
Piperno, D. R. (1994). Phytolith and Charcoal Evidence for Prehistoric Slash-And-Burn Agriculture in the Darien Rain forest of Panama. The Holocene 4 (3), 321–325. doi:10.1177/095968369400400312
Prentice, I. C., Cramer, W., Harrison, S. P., Leemans, R., Monserud, R. A., and Solomon, A. M. (1992). Special Paper: A Global Biome Model Based on Plant Physiology and Dominance, Soil Properties and Climate. J. Biogeogr. 19, 117–134. doi:10.2307/2845499
Prestemon, J. P., and Butry, D. T. (2005). Time to Burn: Modeling Wildland Arson as an Autoregressive Crime Function. Am. J. Agric. Econ. 87, 756–770. doi:10.1111/j.1467-8276.2005.00760.x
Purnomo, H., Shantiko, B., Sitorus, S., Gunawan, H., Achdiawan, R., Kartodihardjo, H., et al. (2017). Fire Economy and Actor Network of forest and Land Fires in Indonesia. For. Pol. Econ. 78, 21–31. doi:10.1016/j.forpol.2017.01.001
Rabin, S. S., Melton, J. R., Lasslop, G., Bachelet, D., Forrest, M., Hantson, S., et al. (2017). The Fire Modeling Intercomparison Project (FireMIP), Phase 1: Experimental and Analytical Protocols with Detailed Model Descriptions. Geosci. Model. Dev. 10, 1175–1197. doi:10.5194/gmd-10-1175-2017
Rabin, S. S., Ward, D. S., Malyshev, S. L., Magi, B. I., Shevliakova, E., and Pacala, S. W. (2018). A Fire Model with Distinct Crop, Pasture, and Non-agricultural Burning: Use of New Data and a Model-Fitting Algorithm for FINAL.1. Geosci. Model. Dev. 11, 815–842. doi:10.5194/gmd-11-815-2018
Rammer, W., and Seidl, R. (2015). Coupling Human and Natural Systems: Simulating Adaptive Management Agents in Dynamically Changing forest Landscapes. Glob. Environ. Change 35, 475–485. doi:10.1016/j.gloenvcha.2015.10.003
Raunkiær, C. (1909). Livsformen Hos Planter paa Ny Jord. Kongelige Danske Videnskabernes Selskabs Skrifter - Naturvidenskabelig og Mathematisk Afdeling 7, 1–70.
Reddington, C. L., Yoshioka, M., Balasubramanian, R., Ridley, D., Toh, Y. Y., Arnold, S. R., et al. (2014). Contribution of Vegetation and Peat Fires to Particulate Air Pollution in Southeast Asia. Environ. Res. Lett. 9, 094006. doi:10.1088/1748-9326/9/9/094006
Remoundou, K., Kountouris, Y., and Koundouri, P. (2012). Is the Value of an Environmental Public Good Sensitive to the Providing Institution? Resource Energ. Econ. 34, 381–395. doi:10.1016/j.reseneeco.2012.03.002
Riahi, K., van Vuuren, D. P., Kriegler, E., Edmonds, J., O’Neill, B. C., Fujimori, S., et al. (2017). The Shared Socioeconomic Pathways and Their Energy, Land Use, and Greenhouse Gas Emissions Implications: An Overview. Glob. Environ. Change 42, 153–168. doi:10.1016/j.gloenvcha.2016.05.009
Richards, G. D. (1990). An Elliptical Growth Model of forest Fire Fronts and its Numerical Solution. Int. J. Numer. Meth. Engng. 30, 1163–1179. doi:10.1002/nme.1620300606
Richardson, L. A., Champ, P. A., and Loomis, J. B. (2012). The Hidden Cost of Wildfires: Economic Valuation of Health Effects of Wildfire Smoke Exposure in Southern California. Jfe 18, 14–35. doi:10.1016/j.jfe.2011.05.002
Robinson, D. T., Brown, D. G., Parker, D. C., Schreinemachers, P., Janssen, M. A., Huigen, M., et al. (2007). Comparison of Empirical Methods for Building Agent-Based Models in Land Use Science. J. Land Use Sci. 2 (1), 31–55. doi:10.1080/17474230701201349
Rodríguez, I., Albert, P., La Rose, C., and Sharpe, C. J. (2011). “A Study of the Use of Fire by Amerindian Communities in South Rupununi, Guyana, with Recommendations for Sustainable Land Management,” in Study Prepared for the South Central and South Rupununi District Toshaos Councils (UK: South Central Peoples Development Association (SCPDA), Forest Peoples Programme).
Rodríguez, I., Sletto, B., Bilbao, B., Sánchez-Rose, I., and Leal, A. (2018). Speaking of Fire: Reflexive Governance in Landscapes of Social Change and Shifting Local Identities. J. Environ. Pol. Plann. 20 (6), 689–703. doi:10.1080/1523908X.2013.766579
Ronchi, E., Gwynne, S. M. V., Rein, G., Intini, P., and Wadhwani, R. (2019). An Open Multi-Physics Framework for Modelling Wildland-Urban Interface Fire Evacuations. Saf. Sci. 118, 868–880. doi:10.1016/j.ssci.2019.06.009
Rosenzweig, C., Arnell, N. W., Ebi, K. L., Lotze-Campen, H., Raes, F., Rapley, C., et al. (2017). Assessing Inter-sectoral Climate Change Risks: the Role of ISIMIP. Environ. Res. Lett. 12, 010301. doi:10.1088/1748-9326/12/1/010301
Rothermel, R. C. (1972). “A Mathematical Model for Predicting Fire Spread in Wildland Fuels,”. Res. Pap. INT-115 (Ogden, UT: U.S. Department of Agriculture, Intermountain Forest and Range Experiment Station).
Ruiz-Mirazo, J., Robles, A., and Gonzalez-Rebollar, J-L. (2011). Two-year Evaluation of Fuelbreaks Grazed by Livestock in the Wildfire Prevention Program in Andalusia (Spain). Agr. Ecosyst. Environ. 141 (1-2), 13–22. doi:10.1016/j.agee.2011.02.002
Scheiter, S., Langan, L., and Higgins, S. I. (2013). Next‐generation Dynamic Global Vegetation Models: Learning from Community Ecology. New Phytol. 198 (3), 957–969. doi:10.1111/nph.12210
Scherjon, F., Bakels, C., MacDonald, K., and Roebroeks, W. (2015). Burning the Land: an Ethnographic Study of Off-Site Fire Use by Current and Historically Documented Foragers and Implications for the Interpretation of Past Fire Practices in the Landscape. Curr. Anthropol. 56 (3), 314–315. doi:10.1086/681561
Schreckenberg, K., Mace, G., and Poudyal, M. (2018). Ecosystem Services and Poverty Alleviation: Trade-Offs and Governance. Abingdon: Routledge.
Schulze, J., Müller, B., Groeneveld, J., and Grimm, V. (2017). Agent-based Modelling of Social-Ecological Systems: Achievements, Challenges, and a Way Forward. Jass- J. Artif. Soc. S. 20 (2), 8. doi:10.18564/jasss.3423
Scott, A. C. (2000). The Pre-quaternary History of Fire. Palaeogeogr. Palaeoclimatol. Palaeoecol. 164, 281–329. doi:10.1016/S0031-0182(00)00192-9
Sikor, T., Barlösius, E., and Scheumann, W. (2008). “Introduction: Public-Private Relations and Key Policy Issues in Natural Resource Governance,” in Public and Private in Natural Resource Governance: A False Dichotomy? Editor T. Sikor (London: Earthscan), 1–18.
Sletto, B. (2008). The Knowledge that Counts: Institutional Identities, Policy Science, and the Conflict over Fire Management in the Gran Sabana, Venezuela. World Dev. 36 (10), 1938–1955. doi:10.1016/j.worlddev.2008.02.008
Smajgl, A., Brown, D. G., Valbuena, D., and Huigen, M. G. A. (2011). Empirical Characterisation of Agent Behaviours in Socio-Ecological Systems. Environ. Model. Softw. 26 (7), 837–844. doi:10.1016/j.envsoft.2011.02.011
Smith, C., Felderhof, L., and Bosch, O. J. H. (2007). Adaptive Management: Making it Happen through Participatory Systems Analysis. Syst. Res. Behav. Sci. 24 (6), 567–587. doi:10.1002/sres.835
Smith, C., and Mistry, J. (2021). “Collating Case Study Data on Human Fire Use at the Global Scale,” in 16th International Wildland Fire Safety Summit & 6th Human Dimensions of Wildland Fire Virtual Conference, May 24-27, 2021.
Smith, N. G., and Keenan, T. F. (2020). Mechanisms Underlying Leaf Photosynthetic Acclimation to Warming and Elevated CO 2 as Inferred from Least‐cost Optimality Theory. Glob. Change Biol. 26, 5202–5216. doi:10.1111/gcb.15212
Sorrensen, C. (2004). Contributions of Fire Use Study to Land Use/cover Change Frameworks: Understanding Landscape Change in Agricultural Frontiers. Hum. Ecol. 32 (4), 395–420. doi:10.1023/b:huec.0000043513.47895.a8
Spies, T. A., White, E., Ager, A., Kline, J. D., Bolte, J. P., Platt, E. K., et al. (2017). Using an Agent-Based Model to Examine forest Management Outcomes in a Fire-Prone Landscape in Oregon, USA. Ecol. Soc. 22 (1), 25. doi:10.5751/ES-08841-220125
Spracklen, D. V., and Garcia-Carreras, L. (2015). The Impact of Amazonian Deforestation on Amazon basin Rainfall. Geophy. Res. Lett. 42 (2), 9546–9552. doi:10.1002/2015GL066063
Stephenson, C., Handmer, J., and Betts, R. (2013). Estimating the Economic, Social and Environmental Impacts of Wildfires in Australia. Environ. Hazards 12, 93–111. doi:10.1080/17477891.2012.703490
Stetler, K. M., Venn, T. J., and Calkin, D. E. (2010). The Effects of Wildfire and Environmental Amenities on Property Values in Northwest Montana, USA. Ecol. Econ. 69, 2233–2243. doi:10.1016/j.ecolecon.2010.06.009
Stevens-Rumann, C. S., Kemp, K. B., Higuera, P. E., Harvey, B. J., Rother, M. T., Donato, D. C., et al. (2018). Evidence for Declining forest Resilience to Wildfires under Climate Change. Ecol. Lett. 21, 243–252. doi:10.1111/ele.12889
Storm, H., Baylis, K., and Heckelei, T. (2020). Machine Learning in Agricultural and Applied Economics. Eur. Rev. Agric. Econ. 47 (3), 849–892. doi:10.1093/erae/jbz033
Stralberg, D., Wang, X., Parisien, M.-A., Robinne, F.-N., ólymos, S. P., Mahon, C. L., et al. (2018). Wildfire-mediated Vegetation Change in Boreal Forests of Alberta, Canada. Ecosphere 9, e02156. doi:10.1002/ecs2.2156
Suffling, R., Grant, A., and Feick, R. (2008). Modeling Prescribed burns to Serve as Regional Firebreaks to Allow Wildfire Activity in Protected Areas. For. Ecol. Manag. 256, 1815–1824. doi:10.1016/j.foreco.2008.06.043
Taylor, C. A. (2003). Rangeland Monitoring and Fire: Wildfires and Prescribed Burning, Nutrient Cycling, and Plant Succession. Arid Land Res. Manag. 17 (4), 429–438. doi:10.1080/713936109
Teague, B., Mcleod, R., and Pascoe, S. (2010). The 2009 Victoria Bushfires Royal Commission Final Report. Melbourne: Government Printer for the State of Victoria.
Teckentrup, L., Harrison, S. P., Hantson, S., Heil, A., Melton, J. R., Forrest, M., et al. (2019). Sensitivity of Simulated Historical Burned Area to Environmental and Anthropogenic Controls: A Comparison of Seven Fire Models. BioGeosciences Discuss., 1–39. doi:10.5194/bg-2019-42
Thonicke, K., Spessa, A., Prentice, I. C., Harrison, S. P., Dong, L., and Carmona-Moreno, C. (2010). The Influence of Vegetation, Fire Spread and Fire Behaviour on Biomass Burning and Trace Gas Emissions: Results from a Process-Based Model. Biogeosciences 7, 1991–2011. doi:10.5194/bg-7-1991-2010
Toivanen, J., Engel, C. B., Reeder, M. J., Lane, T. P., Davies, L., Webster, S., et al. (2019). Coupled Atmosphere-Fire Simulations of the Black Saturday Kilmore East Wildfires with the Unified Model. J. Adv. Model. Earth. Sy. 11, 210–230. doi:10.1029/2017MS001245
Tolhurst, K., Shields, B., and Chong, D. (2008). Phoenix: Development and Application of a Bushfire Risk Management Tool. Aust. J. Emerg. Manag. 23, 47.
Turco, M., Rosa-Cánovas, J. J., Bedia, J., Jerez, S., Montávez, J. P., Llasat, M. C., et al. (2018). Exacerbated Fires in Mediterranean Europe Due to Anthropogenic Warming Projected with Non-stationary Climate-Fire Models. Nat. Commun. 9, 3821. doi:10.1038/s41467-018-06358-z
Tymstra, C., Bryce, R., Wotton, B., Taylor, S., and Armitage, O. (2010). “Development and Structure of Prometheus: the Canadian Wildland Fire Growth Simulation Model. Information,”. Report NOR-X-417 (Natural Resources Canada. Edmonton, Alberta: Northern Forestry Centre).
Van der Werf, G. R., Morton, D. C., DeFries, R. S., Olivier, J. G. J., Kasibhatla, P. S., Jackson, R. B., et al. (2009). CO2 Emissions from forest Loss. Nat. Geosci. 2, 737–738. doi:10.1038/ngeo671
Van Lierop, P., Lindquist, E., Sathyapala, S., and Franceschini, G. (2015). Global forest Area Disturbance from Fire, Insect Pests, Diseases and Severe Weather Events. For. Ecol. Manag. 352, 78–88. doi:10.1016/j.foreco.2015.06.010
Van Vliet, J., Magliocca, N. R., Büchner, B., Cook, E., Rey Benayas, J. M., Erle, E. C., et al. (2016). Meta-studies in Land Use Science: Current Coverage and Prospects. Ambio 45 (1), 15–28. doi:10.1007/s13280-015-0699-8
Van Wilgen, B. W., Biggs, H. C., Ntsala, D., Funda, X. N., and Funda, X. N. (2004). Response of savanna Fire Regimes to Changing Fire-Management Policies in a Large African national park. Conserv. Biol. 18 (6), 1533–1540. doi:10.1111/j.1523-1739.2004.00362.x
Varma, A. (2003). The Economics of Slash and Burn: a Case Study of the 1997-1998 Indonesian forest Fires. Ecol. Econ. 46, 159–171. doi:10.1016/S0921-8009(03)00139-3
Veeraswamy, A., Galea, E. R., Filippidis, L., Lawrence, P. J., Haasanen, S., Gazzard, R. J., et al. (2018). The Simulation of Urban-Scale Evacuation Scenarios with Application to the Swinley forest Fire. Saf. Sci. 102, 178–193. doi:10.1016/j.ssci.2017.07.015
Verburg, P. H., Alexander, P., Evans, T., Magliocca, N. R., Malek, Z., Rounsevell, M. D., et al. (2019). Beyond Land Cover Change: towards a New Generation of Land Use Models. Curr. Opin. Environ. Sustainability 38, 77–85. doi:10.1016/j.cosust.2019.05.002
Voinov, A., Jenni, K., Gray, S., Kolagani, N., Glynn, P. D., Bommel, P., et al. (2018). Tools and Methods in Participatory Modeling: Selecting the Right Tool for the Job. Environ. Model. Softw. 109, 232–255. doi:10.1016/j.envsoft.2018.08.028
Voinov, A., and Shugart, H. H. (2013). 'Integronsters', Integral and Integrated Modeling. Environ. Model. Softw. 39, 149–158. doi:10.1016/j.envsoft.2012.05.014
Volkova, L., Meyer, C. P., Haverd, V., and Weston, C. J. (2018). A Data - Model Fusion Methodology for Mapping Bushfire Fuels for Smoke Emissions Forecasting in Forested Landscapes of South-Eastern Australia. J. Environ. Manage. 222, 21–29. doi:10.1016/j.jenvman.2018.05.060
Vossler, C. A., Doyon, M., and Rondeau, D. (2012). Truth in Consequentiality: Theory and Field Evidence on Discrete Choice Experiments. Am. Econ. J. Microeconomics 4, 145–171. doi:10.1257/mic.4.4.145
Wainwright, J., and Millington, J. D. A. (2010). Mind, the gap in Landscape-Evolution Modelling. Earth Surf. Proc. Land. 35 (7), 842–855. doi:10.1002/esp.2008
Walker, B. H., and Janssen, M. A. (2002). Rangelands, Pastoralists and Governments: Interlinked Systems of People and Nature. Phil. Trans. R. Soc. Lond. B. 357 (1421), 719–725. doi:10.1002/esp.200810.1098/rstb.2001.0984
Walker, X. J., Baltzer, J. L., Cumming, S. G., Day, N. J., Ebert, C., Goetz, S., et al. (2019). Increasing Wildfires Threaten Historic Carbon Sink of Boreal forest Soils. Nature 572, 520–523. doi:10.1038/s41586-019-1474-y
Wang, H., Prentice, I. C., Keenan, T. F., Davis, T. W., Wright, I. J., Cornwell, W. K., et al. (2017). Towards a Universal Model for Carbon Dioxide Uptake by Plants. Nat. Plants 3, 734–741. doi:10.1038/s41477-017-0006-8
Wang, H., Prentice, I. C., Wright, I. J., Qiao, S., Xu, X., Kikuzawa, K., et al. (2021). Leaf Economics Explained by Optimality Principles. New York: bioRxiv. doi:10.1101/2021.02.07.430028
Warziniack, T., Champ, P., Meldrum, J., Brenkert-Smith, H., Barth, C. M., and Falk, L. C. (2019). Responding to Risky Neighbors: Testing for Spatial Spillover Effects for Defensible Space in a Fire-Prone WUI Community. Environ. Resource Econ. 73, 1023–1047. doi:10.1007/s10640-018-0286-0
Watts, J. D., Tacconi, L., Hapsari, N., Irawan, S., Sloan, S., and Widiastomo, T. (2019). Incentivizing Compliance: Evaluating the Effectiveness of Targeted Village Incentives for Reducing Burning in Indonesia. For. Pol. Econ. 108, 101956. doi:10.1016/j.forpol.2019.101956
Weber, R. (1991). Toward a Comprehensive Wildlife Spread Model. Int. J. Wildland Fire 1, 245. doi:10.1071/WF9910245
West, A. G., Bond, W. J., and Midgley, J. J. (2000). Soil Carbon Isotopes Reveal Ancient Grassland under forest in Hluhluwe, Kwa-Zulu Natal. S. Afr. J. Sci. 96, 252–254.
Whitman, E., Parisien, M.-A., Thompson, D. K., and Flannigan, M. D. (2019). Short-interval Wildfire and Drought Overwhelm Boreal forest Resilience. Sci. Rep. 9, 18796. doi:10.1038/s41598-019-55036-7
Wibbenmeyer, M., Anderson, S. E., and Plantinga, A. J. (2019). Salience and the Government Provision of Public Goods. Econ. Inq. 57, 1547–1567. doi:10.1111/ecin.12781
Wohling, M. (2009). The Problem of Scale in Indigenous Knowledge: a Perspective from Northern Australia. Ecol. Soc. 14, 1.
Yoder, J. (2004). Playing with Fire: Endogenous Risk in Resource Management. Am. J. Agric. Econ. 86, 933–948. doi:10.1111/j.0002-9092.2004.00644.x
Yu, P., Xu, R., Abramson, M. J., Li, S., and Guo, Y. (2020). Bushfires in Australia: a Serious Health Emergency under Climate Change. Lancet Planet. Health 4, e7–e8. doi:10.1016/s2542-5196(19)30267-0
Zivin, J. S. G., Liu, T., Song, Y., Tang, Q., and Zhang, P. (2019). The Unintended Impacts of Agricultural Fires: Human Capital in China. Cambridge, MA: National Bureau of Economic Research. doi:10.3386/w26205
Keywords: pyrogeography, climate and fire projections, fire management, fire policies, human-fire models, agent-based models, fire-enabled global vegetation model, firespread models
Citation: Ford AES, Harrison SP, Kountouris Y, Millington JDA, Mistry J, Perkins O, Rabin SS, Rein G, Schreckenberg K, Smith C, Smith TEL and Yadav K (2021) Modelling Human-Fire Interactions: Combining Alternative Perspectives and Approaches. Front. Environ. Sci. 9:649835. doi: 10.3389/fenvs.2021.649835
Received: 05 January 2021; Accepted: 07 September 2021;
Published: 28 September 2021.
Edited by:
Hayley Jane Fowler, Newcastle University, United KingdomReviewed by:
Anders Bryn, University of Oslo, NorwayChris Guiterman, University of Arizona, United States
Copyright © 2021 Ford, Harrison, Kountouris, Millington, Mistry, Perkins, Rabin, Rein, Schreckenberg, Smith, Smith and Yadav. This is an open-access article distributed under the terms of the Creative Commons Attribution License (CC BY). The use, distribution or reproduction in other forums is permitted, provided the original author(s) and the copyright owner(s) are credited and that the original publication in this journal is cited, in accordance with accepted academic practice. No use, distribution or reproduction is permitted which does not comply with these terms.
*Correspondence: Adriana E. S. Ford, a.ford@imperial.ac.uk
†These authors share first authorship