- 1Department of Marine Sciences, University of Connecticut, Groton, CT, United States
- 2Naval Research Laboratory, Washington, DC, United States
- 3College of Science and Engineering / TropWATER, James Cook University Nguma-bada Campus, Cairns, QLD, Australia
- 4Civil & Environmental Engineering, University of California Merced, Merced, CA, United States
- 5Protistology and Aquatic Ecology, Ghent University, Ghent, Belgium
- 6Pixalytics Ltd., Plymouth, United Kingdom
- 7Department of Oceanography, University of Hawai’i at Mānoa, Honolulu, HI, United States
Intensifying pressure on global aquatic resources and services due to population growth and climate change is inspiring new surveying technologies to provide science-based information in support of management and policy strategies. One area of rapid development is hyperspectral remote sensing: imaging across the full spectrum of visible and infrared light. Hyperspectral imagery contains more environmentally meaningful information than panchromatic or multispectral imagery and is poised to provide new applications relevant to society, including assessments of aquatic biodiversity, habitats, water quality, and natural and anthropogenic hazards. To aid in these advances, we provide resources relevant to hyperspectral remote sensing in terms of providing the latest reviews, databases, and software available for practitioners in the field. We highlight recent advances in sensor design, modes of deployment, and image analysis techniques that are becoming more widely available to environmental researchers and resource managers alike. Systems recently deployed on space- and airborne platforms are presented, as well as future missions and advances in unoccupied aerial systems (UAS) and autonomous in-water survey methods. These systems will greatly enhance the ability to collect interdisciplinary observations on-demand and in previously inaccessible environments. Looking forward, advances in sensor miniaturization are discussed alongside the incorporation of citizen science, moving toward open and FAIR (findable, accessible, interoperable, and reusable) data. Advances in machine learning and cloud computing allow for exploitation of the full electromagnetic spectrum, and better bridging across the larger scientific community that also includes biogeochemical modelers and climate scientists. These advances will place sophisticated remote sensing capabilities into the hands of individual users and provide on-demand imagery tailored to research and management requirements, as well as provide critical input to marine and climate forecasting systems. The next decade of hyperspectral aquatic remote sensing is on the cusp of revolutionizing the way we assess and monitor aquatic environments and detect changes relevant to global communities.
Introduction
In response to mounting ecological stressors, natural resource managers look to remote sensing as a means of providing timely and spatially coherent environmental information that can inform decision making. However, current products provided by the ocean color community do not satisfy the needs required for management of aquatic ecosystems spanning the open ocean to inland waters. As water surfaces are much darker than terrestrial surfaces, the signal emerging from the water is obscured by the atmosphere, when viewed from high altitude or space, and dedicated sensors with large dynamic ranges and longer integration times are often required to achieve the measurement sensitivity needed (Mouroulis et al., 2008). For many applications, the spatial footprint of space-based ocean color sensors can be too large (typically 0.5–1 km) and the revisit time of land-imaging sensors, which have the capability to resolve coasts and inland waters, is often too infrequent (weekly to monthly). Further, increases in spatial resolution typically go hand-in-hand with decreases in spectral resolution, and the products afforded by limited spectral imagery are high in uncertainty and low in specificity (Dekker and et al., 2018). The tradeoffs between spectral, spatial, and temporal sampling often mean that no single sensor or platform can provide all of the needs for any single user community or application (Muller-Karger et al., 2018). As a result, current capabilities for monitoring water quality and biodiversity across diverse habitats, from lakes and wetlands to coastal pelagic and benthic communities, have only been demonstrated on limited scales and have yet to achieve routine operational use by environmental managers.
Traditional ocean color remote sensing involves multi-spectral sensors that have a small number of broad discontiguous spectral bands measuring portions of the visible and infrared electromagnetic spectrum. These bands were placed at key wavelength regions mainly designed to detect the concentration of the primary pigment in phytoplankton, chlorophyll a (Gordon and Morel, 1983). Airborne and spaceborne sensor technology has advanced rapidly in the last few decades to include imaging spectrometers with continuous spectral coverage throughout the visible and near-infrared spectrum (VNIR), typically between 400 and 1,000 nm, and sometimes extending to the shortwave infrared regions as well (up to 2,500 nm). To differentiate from multi-spectral sensors with discrete, often broad wavebands, such imaging spectrometers are commonly referred to as “hyperspectral” imaging systems. The terms “hyperspectral imaging” and “imaging spectrometry” are interoperable. No standard definitions have been adopted as to the number of bands and associated bandwidths required for a sensor to be considered “hyperspectral.” Still, generally, hyperspectral sensors for coastal applications have bandwidths less than 15 nm and >20 bands in visible wavelengths. However, sensitivity studies spanning a range of diverse aquatic water bodies recommend hyperspectral sensors bandwidths of 5 nm (Wolanin et al., 2016; Vandermeulen et al., 2017; Dekker et al., 2018). Consequently, the majority of the present and future hyperspectral sensors have at least 60 bands within the VNIR spectral range (Figure 1).
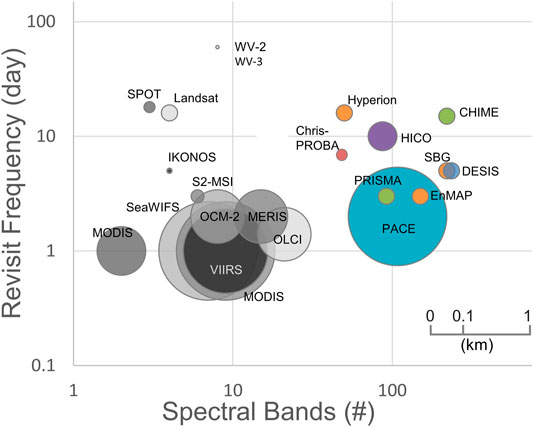
FIGURE 1. Architecture of various international missions showing the tradeoff between number of spectral bands, revisit frequency (X and Y axes, respectively) and the spatial footprint (approximated as the size of circle). Gray represent multi-spectral missions and colors represent the hyperspectral missions. Modified from Hestir et al. (2015a).
The field of aquatic hyperspectral remote sensing is advancing rapidly, and new products are informing water quality monitoring, as well as the biodiversity of organisms swimming and floating in pelagic habitats and dwelling on and near the bottom in benthic habitats (reviewed in Tyler et al., 2016; Giardino et al., 2019; Kutser et al., 2020). The continuous spectrum can always be sub-sampled to yield any multispectral band combination to take advantage of legacy algorithms or to amplify the signal to noise ratio. However, hyperspectral data provides potential new applications that are not limited by gaps in the spectrum based on historic notions of utility in open ocean ecosystems. Indeed, several multispectral ocean color sensors are programmable spectrometers resampled onboard to specific wavebands (e.g., Sentinel-3 OLCI). Utilizing the full spectrum, even simple approaches that characterize the spectral dimensionality and variability can be used to identify and track subtle differences between water masses and over time that could not be tracked by bulk chlorophyll a estimates (Vandermeulen et al., 2020). Full-spectrum estimates of the diffuse attenuation coefficient (Kd) may lead to new insights about aquatic ecosystems and biodiversity processes like spectral niche partitioning and complementarity (Striebel et al., 2009), or behavior and evolutionary traits of aquatic invertebrates and vertebrates (Russell and Dierssen, 2015; Cummings and Endler, 2018). Thus, imaging spectrometry can inspire and support new algorithm concepts that require continuous and dense spectral sampling. The benefits of merging hyperspectral imagery with other types of remote sensing imagery and environmental parameters through modeling (IOCCG, 2020) will also be an avenue for the future to address a wide range in societal problems including the response of coastal ocean ecosystems to population growth and climate change (Muller-Karger et al., 2018).
The motivation for this review emerged from a recent workshop focused on the advances and challenges of hyperspectral remote sensing technology applied to coastal aquatic environments. The workshop, conducted at the University of Hawai'i at Mānoa on 15 and 16 May 2018, was convened by the Alliance for Coastal Technologies (ACT) and sponsored by the National Oceanic and Atmospheric Administration (NOAA)/U.S. Integrated Observing System (US.IOOS) (workshop report available at http://www.act-us.info/workshops.php). The overarching goal of this article is to present advances and resources relevant to hyperspectral remote sensing in aquatic ecosystems for those newly diving into the field, as well as for those already deeply submerged within remote sensing and aquatic optics. With the advent of new sensor capabilities across all platforms from orbiting in space, to flying autonomously over reefs (Joyce et al., 2019), to cruising along the dark, cold ocean floor (Johnsen et al., 2013), hyperspectral remote sensing is rapidly growing its user base. Many resources are already available for algorithm developers, and users of the data depending on the specific user need. Here, we have compiled lists of reviews, software, and databases relevant to developing and implementing aquatic hyperspectral remote sensing approaches. Finally, we discuss how remote sensing of coastal aquatic systems might advance in the coming decade.
A Blast From the Past: A Brief History of Hyperspectral Imaging
Many excellent reviews have been written in the last five years alone about the history and requirements for aquatic remote sensing that relate to hyperspectral imaging spectroscopy (Table 1). Some reviews focus on specific habitats from open ocean to coastal and inland waters; some review techniques relevant for data collection or modeling; and, others focus on applications including water quality assessment, phytoplankton composition, bathymetry and benthic cover in optically shallow waters. Here, we build on those studies to provide a summary of advances made specifically in imaging spectroscopy for aquatic applications. This overview is meant to show the breadth of the field and theoretical studies from the vast oligotrophic ocean to coral reefs to lakes and rivers.
The origins of the discipline are challenging to pinpoint, as it arose from a merging of technology and research coming from limnology and oceanography, as well as building from advances made in terrestrial, atmospheric, and cryospheric remote sensing. Wernand (2011) provides a comprehensive overview of the scientists, their hypothesis and experiments in the historical development of hydrologic optics since the seventeenth century that culminated in the theoretical foundations provided by Raman (Raman, 1922) and Shoulejkin (Shoulejkin, 1923). Field experiments were also conducted at that time, such as those done by the limnologist Edison Pettit who conducted spectroscopic investigations into the volume scattering and absorption processes underlying the color of Crater Lake, OR, USA (Pettit, 1936). Modern optical oceanography is considered to have originated around the 1950s, with the pioneering research by the Danish oceanographer Nils Jerlov who established a continuum of optical water types from the clear pelagic ocean to the turbid coast (Jerlov, 1951, 1963). However, as noted below, aquatic remote sensing really began in earnest in the late 1960s and 1970s with theoretical and experimental research spanning inland, coastal and open ocean environments (reviewed in Acker, 2015; Kutser et al., 2020).
In the late 1960s, Clarke et al. (1970) detail an airborne spectroradiometer flown in North Atlantic waters that demonstrated how increasing amounts of chlorophyll were found to be associated with a relative decrease in the blue portion of the spectra and an increase in the green in backscattered light (Clarke et al., 1970). Concomitantly in 1970, international collaboration on the development of spectrometers culminated in a sea trial as part of Scientific Committee on Oceanographic Research (SCOR) Working Group 15 that included optical measurements in combination with phytoplankton species composition and sizes, primary productivity, chlorophyll and nutrients with researchers from Australia, Denmark, France, Japan, Norway, Russia, Scotland, and the United States (Tyler, 1970). Collectively, such international partnerships provided proof-of-concept evidence for satellite-based ocean color remote sensing. This underpinning work led to the first ocean color mission, the Coastal Zone Color Scanner (CZCS), and continues today with the International Ocean Color Coordinating Group (IOCCG).
In addition to these oceanic studies, many other parallel lines of research were occurring in the 1970s in the broader field of experimental and theoretical aquatic optics. Investigations were beginning in shallow water optics with the mapping of the Great Barrier Reef using Landsat data (Smith et al., 1975) and the seminal work of Polcyn et al. (1970) and Lyzenga (1978), which provided methods to retrieve bathymetry and benthic composition of sand, mud and vegetation from passive remote sensing imagery (Polcyn et al., 1970; Lyzenga, 1978). Some of the first laboratory reflectance measurements of phytoplankton cultures were summarized in a 1977 report entitled “Assessment of Aquatic Environments by Remote Sensing” demonstrating that blue-green algae, green algae and diatoms could be differentiated from “laboratory reflectance fingerprints” (Adams et al., 1977). Multi-spectral and thermal airborne remote sensing of algal blooms was initiated in the Great Lakes (Strong, 1974). Goldman et al. (1974) used color and multispectral aerial photography to delineate sediment plumes into Lake Tahoe, California and correlate them with suspended sediment, inorganic carbon, light penetration and primary productivity (Goldman et al., 1974). Concurrently, radiative transfer simulations studies helped to provide a qualitative and quantitative interpretation of the water leaving signal (Gordon et al., 1975; Gordon and McCluney, 1975). Further theoretical and mathematical developments were established when Preisendorfer culminated his research on radiative transfer theory in 1976 with a six-volume treatize entitled Hydrologic Optics (Preisendorfer, 1976). Preisendorfer’s method for radiative transfer solution is implemented in the Hydrolight software, that became the community standard for the simulation of scalar aquatic spectral reflectance (Mobley, 1994). This decade of independent and parallel research provided the foundations for many of the techniques and applications for the broader field of aquatic hyperspectral remote sensing today.
Over the past three decades, the emergence of imaging spectroscopy with the ability to produce a spectrally resolved image of a scene has advanced rapidly in sensor technology, calibration, deployment modes, and applications as briefly outlined below. With the limited availability of satellite data, the period between the early 1980s and 1999 ushered in the age of operational aircraft-based hyperspectral from AVIRIS to CASI (reviewed in Giardino et al., 2019). Other relevant airborne hyperspectral sensors were the Operational Modular Imaging Spectrometer (OMIS) and the Pushbroom Hyperspectral Imaging (PHI) developed in China (Tong et al., 2001). These airborne imagers were designed primarily for terrestrial applications but were nonetheless successfully used to identify brighter aquatic targets such as identifying sediment plumes (e.g. Moore et al. (1999) that became the basis for the MERIS bright pixel atmospheric correction) and bathymetry and benthic composition in shallow water. Collins and Pattiaratchi (1984) used the Daedalus Airborne Thematic Mapper, which was operated alongside CASI by the Natural Environment Research Council Airborne Research Facility for its thermal capabilities, noting that both relative and absolute increases in suspended sediment produced a shift toward longer (red) wavelengths.
However, these initial aquatic applications had limited success due to the airborne sensors having a relatively low signal-to-noise ratio (SNR) and a limited dynamic range (reviewed in Kutser 2020). The operators needed to set the appropriate integration time for the low aquatic signal and develop robust procedures for instrument calibration. Therefore, data from these sensors were often aggregated spectrally and spatially to obtain higher signal or smoothed considerably in post-processing to reduce spectral noise (Carder et al., 1993) and provided only multispectral data over aquatic targets (Mumby et al., 1997; Sathyendranath et al., 1997). As a result, little was gained from the hyperspectral signal.
The 2000s were a time for targeting new sensors and programs designed to facilitate aquatic applications. Even though the potential for imaging spectrometry was recognized early on (Dekker and Donze, 1994), inland and coastal waters provide critical challenges to spectrometer system design including reflectance of targets varying from <1% for dark water to over 90% for bright sand. The Ocean Portable Hyperspectral Imager for Low-Light Spectroscopy (PHILLS) was a pushbroom-scanning instrument designed specifically for aquatic applications (Davis et al., 2002). Early deployments of Ocean PHILLS include those as part of the Coastal Benthic Optical Properties (CoBOP) program at Lee Stocking Island, Bahamas (May/June 1999 and May 2000), the Hyperspectral Coupled Ocean Dynamics Experiments (HyCODE) program on the West Florida Shelf (2000 and 2001) and at the LEO-15 site in New Jersey (July 2000 and July 2001). The field and airborne efforts from those projects provided some of the foundational hyperspectral algorithms for evaluating optically important constituents, estimating vertical structure in the near-surface ocean, developing benthic cover and bathymetry algorithms, and refining treatment of optical properties in coupled ocean-atmosphere models (Davis, 2001; Mazel, 2002; Chang et al., 2004; Wang, 2004).
More technological advances were also brought about by the National Aeronautics and Space Administration (NASA) Jet Propulsion Laboratory (JPL) Portable Hyperspectral Imaging SpectroMeter (PRISM) developed in 2008 designed to handle the dynamic range of aquatic surfaces, with an improved spectral resolution, polarization sensitivity, response uniformity, and minimal spectrometer distortions (Mouroulis et al., 2008). First flight results over calibration sites in Elkhorn Slough, California, demonstrated good agreement between in situ and remotely sensed data (Heupel et al., 2013; Mouroulis et al., 2014) and the sensor has found modern use in applications from differentiating floating vegetation, water contaminants, chlorophyll fluorescence, and coral reef assessments (Dierssen et al., 2015; Fichot et al., 2016; Garcia et al., 2018; Erickson et al., 2019). Similar high-quality imagery is now available in sensors like Airborne Prism Experiment (APEX) and from commercial vendors (reviewed in Giardino et al., 2019).
Moving from suborbital to orbital platforms, the first decade of the 21st Century also involved the launch of several hyperspectral imagers with applications to aquatic ecosystems including: Hyperion launched aboard the EO-1 spacecraft in 2000 (Dekker et al., 2001), the Compact High Resolution Imaging Spectrometer (CHRIS) launched on PROBA-1 in 2001 (Cutter et al., 2004), the Scanning Imaging Absorption Spectrometer for Atmospheric Chartography (SCIAMACHY) launched on ENVISAT in 2002 (Bracher et al., 2009) and the Hyperspectral Imager for the Coastal Ocean (HICO) installed on the International Space Station in 2009 (Ryan et al., 2014). Except for SCIAMCHY, these sensors had smaller footprints optimized for coastal applications (20–90 m) and were targeted mappers acquiring data over particular areas based on data acquisition requests with limited collections per day (Figure 1). Similar to the early airborne instruments, the data exhibited low signals over many aquatic targets and were often binned spatially and spectrally for aquatic applications (Hestir et al., 2015). SCIAMACHY provided global mapping capabilities at much finer spectral resolution (<0.5 nm bands), but at much larger spatial resolutions of 30 km pixels. Such applications demonstrated that hyperspectral signals could move beyond typical remote sensing methods and be used to assess bathymetry, benthic cover, and phytoplankton composition. These applications are summarized in many different reviews of hyperspectral and aquatic remote sensing provided in Table 1.
Launching the Future of Hyperspectral Imaging
Here we discuss both the orbital and suborbital systems proposed for hyperspectral imaging of aquatic ecosystems including advances in sensor miniaturization and autonomous sampling platforms.
Satellite Systems
Building on these past successes, we now find ourselves on the verge of significant global advances in hyperspectral remote sensing with the recent and pending launch of an array of hyperspectral satellites poised to sense the Earth from different platforms and orbits (Figure 1). Most recently, several targeted mappers have been launched, of which we highlight a few missions with publicly available imagery such as Deutsches Zentrum fur Luft–und Raumfahrt German Aerospace Center (DLR) Earth Sensing Imaging Spectrometer (DESIS) (Müller et al., 2016) installed on the International Space Station and the launch of China’s Advanced Hyperspectral Imager (AHSI) onboard the GaoFeng-5 satelite (Liu et al., 2019) in 2018, followed by the launch of the PRecursore IperSpettrale della Missione Applicativa (PRISMA) sensor by the Italian Space Agency in 2019 (Giardino et al., 2020) and HyperScout instruments launched on nanosatellites (Esposito and Zuccaro Marchi (2019). On the future horizon are low-Earth orbiting global mapping hyperspectral missions including NASA’s Plankton Erosol Cloud and ocean Ecosystem (PACE) and Surface Biology and Geology (SBG) missions, and the European Space Agency’s Copernicus Hyperspectral Imaging Mission for the Environment (CHIME), as well as geostationary missions like NASA’s Geosynchronous Littoral Imaging and Monitoring Radiometer (GLIMR). Recognizing that no single mission can satisfy all applications, concepts have started to formulate among space agencies, researchers, resource managers, and policy experts for combining multiple instruments into a virtual constellation that meets many observation needs, as well as combining hyperspectral imagery with other sources of remote, in situ, and modeled data to better assess ecosystem health and biodiversity (Duffy et al., 2013; Greb et al., 2018; Muller-Karger et al., 2018).
Suborbital Systems
It is exciting that sensor design has also moved toward smaller, solid state systems requiring less power for deployment on small satellites (Doubleday et al., 2015; Bender et al., 2018), aircraft, autonomous unoccupied aerial systems (UAS) (Wu et al., 2019), or small watercraft dedicated to specific problems (Klemas, 2015; Ackleson et al., 2017). This represents an emerging dimension to how modern remote sensing data are acquired. Traditional sources of coverage by orbiting sensors are inherently limited by cloud cover and orbital dynamics, and engineering trades made in sensor design between spatial, spectral and radiometric resolution. Portable sensors flown on aircraft or drones provide a critical sampling niche distinct from satellite-borne sensors that are particularly well suited for coastal and inland water applications Such sensors can sample at fine spatial scales, can operate under clouds and with nearly unlimited repeat coverage, and are effective platforms for high resolution active sensors (e.g., lidar). Aircraft overflights are also subject to limitations of transporting gear, aircraft and personnel to the study area, as well as being subject to local weather conditions and flight restrictions. Autonomous systems are more portable and completely controlled by the individual research team, permitting imaging schedule and geometry, e.g., relative to the Sun location, to be adjusted in real time.
Unoccupied Aerial Systems
Perhaps some of the greatest future advances will come with the rapid advances in UAS technology. The term UAS is used to include both the unoccupied aerial vehicle (UAV) or drone, as well as the ground-based control system. UAS for civilian applications started in the early 1980s using remote controlled aircraft equipped with aerial mapping cameras (Wester-Ebbinghaus, 1980). Since that time, both sensor and drone technology have advanced significantly (Colomina and Molina, 2014; Aasen et al., 2018) with decreasing expense and mission planning, and navigation software requiring less experience from the remote operator. Users can readily create custom UAS systems or purchase complete systems, including sensors, aircraft, and mission planning and data analysis software, tailored to applications. A recent review of UAS remote sensing systems in environmental biology found that publication rates from studies using this technology increased 10 fold between 2000 and 2018 with most of the increase occurring since 2011 (Nowak et al., 2018).
Compact hyperspectral imaging sensors appropriate for small UAV operations are a fairly recent development, starting in the 2010 time frame based on published reports. Advances in gimbaling systems have allowed for better image quality to minimize artifacts from pitch and roll of the drone and vibrations (Wu et al., 2019). UAV flight lines and scanning geometries can also be oriented to optimize retrievals (e.g., avoid Sun glint) and their range can be greatly expanded by launching from ships. Because of cloud cover and orbital constraints, the temporal resolution from satellites is often not sufficient to track episodic events like harmful algal blooms (HABs) compared to a drone that can be deployed in a rapid response during an outbreak. Imagery from UAS can provide details at the centimeter scale and temporal frequencies at the hourly scale with relatively low costs. Moreover, the technology can be closely synchronized with water sampling in order to characterize phytoplankton types and quantify the concentrations of cells and toxins (Wu et al., 2019).
Much of the excellent work undertaken in the early 2000s with autonomous field spectrometers can now be expanded using spectrometers from UAS. Where early work focused on spectral characteristics of marine and aquatic features at very high temporal scales at a single point scale from moorings (Dickey and Chang, 2002) it is possible to now include similar spectrometers on a UAS payload, rapidly capturing thousands of point-based spectroscopic measurements traversing large areas at very low cost from small off the shelf platforms (Cornet and Joyce, in review). For more advanced solutions, imaging technology over broader scales allows researchers to explore spatial and temporal dynamics in even greater detail. UAS have become a popular tool for monitoring the emergence and extent of intense phytoplankton blooms, particularly those that are considered HABs (Becker et al., 2019; Wu et al., 2019). For example, drone imagery, coupled with satellite technology, was used to effectively detect, forecast, and manage the green tides in South Yellow Sea, China (Xu et al., 2017). Researchers also used a drone to track and quantify an intense phytoplankton bloom in Weitou Bay in the western Taiwan Strait (Shang et al., 2017). Similarly, several studies have used RGB imagery from drones to map intertidal coral reefs (Murfitt et al., 2017; Muslim et al., 2019). Application of hyperspectral UAS for aquatic environments, including water quality (Zeng et al., 2017), HABs (Becker et al., 2019), shallow water benthic mapping (Parsons et al., 2018), and marine fauna surveys (Colefax et al., 2018).
Deriving quantitative estimates of reflectance can be particularly challenging from UAVs, particularly under glint or variable cloud cover when the downwelling irradiance at the sea surface can be quite different from that measured at the drone. Shang et al. (2017) provide an innovative method to calibrate the signal and derive hyperspectral water-leaving reflectance from drones. Joyce et al. (2019) provide data collection workflows for planning UAS campaigns to ensure that necessary pre-planning and safety steps are considered, as well as requirements including licensing, data processing and logistical considerations. As noted further in Section 8, we are on the cusp of achieving significant advances in hyperspectral remote sensing across the aquatic landscape or “aquascape”.
Autonomous Floating and Underwater Sampling
The availability of high quality in situ observations suitable for remote sensing algorithm development and product validation is key to any quantitative remote sensing activity. Unfortunately, coastal and inland aquatic systems are notoriously difficult to sample, especially in coordination with airborne or spaceborne remote sensing. Water constituent concentration and bottom depth vary across a wide range in temporal and spatial scale. Correlation scales of water and benthic properties are typically <10 m and often less than 1 m and traditional ship-based operations are limited and often prohibited by shallow bathymetry. Thus, there are significant challenges resulting from inadequate in situ knowledge that currently limits progress in any application of remote sensing to coastal and inland aquatic problems, regardless of sensor configuration. To address these issues, researchers have begun exploring autonomous methods of collecting in situ observations. Autonomous platforms, primarily underwater versions, have been in development for several decades and have focused on bathymetry, chlorophyll, and water physical properties associated with optically deep water (Moline et al., 2005; Ryan et al., 2010; Johnsen et al., 2013). More recently, attention has turned to instrumented surface systems designed to support interdisciplinary applications of hyperspectral remote sensing operations in shallow coastal environments (Ackleson et al., 2017).
Looking Under the Hood
Hyperspectral imagery is generated in a “Datacube” with spatial dimensions in the X and Y axis and wavelength as the Z axis. With >100 bands, hyperspectral images are notoriously large files that can be challenging to download and process with some of the traditional software tools employed in multi-spectral remote sensing. For example, 12 flight lines from the PRISM airborne sensor covering 100 km2 of Elkhorn Slough, California and two long flight lines out 40 km into Monterey Bay, California provided over 400 GB of raw imagery (Heupel et al., 2013). Similarly, 16 flightlines covering 250 km2 of a wetland near Sacramento, California collected by a UAS-mounted Headwall Nano-Hyperspec pushbroom sensor provided roughly 40 GB of raw imagery (Bolch et al., 2020). An entire image cannot be fully loaded into a typical computer memory and the image must be treated as data tiles, either spectrally or spatially, such that only a subset of bands or pixels are loaded into memory at one time. For rendering, an RGB representation of the image is often shown where a single red, green and blue wavelength is selected to mimic the human eye as a pseudo-true color. For image processing, spatial subsets are processed sequentially with some overlap typically required for certain functions. Software has been developed and optimized for processing such large imagery and automatically conducting data tiling spatially or spectrally as needed for the user (Table 2).
Computational power, data storage and I/O bottlenecks have been frequent limitations of using hyperspectral data given the large volume of data. Hence, many of the early studies reduce the dimensionality of hyperspectral imagery to a few multi-spectral bands for simple estimates of chlorophyll a and suspended particulate matter. As high-performance computing and easy-to-implement parallel processing workflows have proliferated, increasing data volumes with increasing hyperspectral datasets and the associated challenges remain lock-step with computing advances. Data reduction is also common because of the limited amount of hyperspectral datasets coupled with biogeochemical information for algorithm development and validation (Dierssen et al., 2020). In addition, many approaches are not repeatable because the imagery has not been adequately corrected for atmospheric absorption and scattering and sea surface reflections. In the following sections, we discuss some of the approaches that have been used for atmospheric correction and processing hyperspectral imagery.
Finding the Ocean Needle in the Atmospheric Haystack
Water bodies are typically much darker than the land or atmosphere above and pulling out the signal that represents the color of the water is extremely challenging. Except in highly turbid waters, the water signal is only a few percent in the pool of photons that reaches a spaceborne sensor. The term “Atmospheric correction” refers to the techniques used to adjust at-sensor radiance on airborne and spaceborne platforms for atmospheric effects, though typically includes compensation for solar irradiance, water surface effects and directional dependency of the water leaving signal (Mobley et al., 2016; Frouin et al., 2019). Particular challenges for atmospheric correction include absorbing aerosols, clouds, adjacency effects and identifying when there is interference from bottom reflection (Brando et al., 2009). Atmospheric correction routines consider the absorption properties of atmospheric gases, as well as light scattered within the atmosphere and reflected from the water surface and/or whitecaps. Such approaches can be extra challenging for hyperspectral imagery that includes spectral regions where atmospheric gases absorb and aquatic ecosystems with high particle loads that scatter light in the near infrared parts of the spectrum. Hyperspectral imagery from the ultraviolet to near infrared also holds the promise of improving upon atmospheric correction, particularly for estimating absorbing aerosols (Frouin et al., 2019).
Because contributions of diffuse and direct sunlight are variable and sea surfaces are not flat, removal of reflected light off the sea surface is challenging for both field and remotely sensed measurements. Ideally, missions tend to minimize Sun glint by optimizing viewing geometry and mission scheduling, which may involve designing spaceborne sensors with pre-defined oblique viewing angles and orbital dynamics that result in mid-morning or mid-afternoon overpasses (Hovis et al., 1980). For aircraft operations, flight planning may include avoidance of observations made close to local noon and flight paths that are oriented into or out of the solar direction to avoid cross-track illumination. There is also a need to account for glitter that exists as whole pixels (Lavender and Nagur, 2002). Regardless of sensor design, Sun glint, adjacency effects, and whitecaps can limit the utility of data even with the most advanced correction routines.
Some of the more common approaches applied to multi-spectral and hyperspectral imagery over aquatic water bodies are provided as a resource (Table 3), in addition to a review of hyperspectral atmospheric correction approaches (Gao et al., 2009). However, we also note that historically the atmosphere has been treated as a prior step to ocean techniques, but advanced methods for coupled ocean-atmosphere retrievals are an area of growth in the future (Stamnes, 2003; Jamet et al., 2005; Kuchinke et al., 2009; Steinmetz et al., 2011; Stamnes et al., 2018). Hyperspectral atmospheric correction routines include those that use simulated water leaving reflectance shapes and retrieve spectra based on some type of a priori spectral library (Steinmetz et al., 2011; Thompson et al., 2019). Others attempt to independently retrieve the water and atmospheric properties and only “lightly constrain” the water-leaving spectral reflectance (Lavender and Nagur, 2002; Ibrahim et al., 2018; Vanhellemont, 2019). Most approaches retrieve all components on a pixel-by-pixel basis, but some are optimized to use features in the imagery to help constrain one or more parameter in the solution for the entire scene under investigation (Guanter et al., 2010; Vanhellemont, 2019). Hence, different techniques can be used for imagery collected close to land or within land compared to open ocean. Another problem is the influence of reflectance from adjacent land/ice pixels that can be scattered into the field of view of the sensor and “contaminate” the water signal with often brighter and spectrally disparate material. This adjacency is particularly problematic for water near bright ice surfaces and inland waters surrounded by bright land and vegetation, and can interfere with techniques to atmospherically correct for aerosols and other imagery. Various techniques have been proposed for addressing adjacency (Reinersman and Carder, 1995; Santer and Schmechtig, 2000; Sterckx et al., 2011; Bulgarelli and Zibordi, 2018), but challenges occur in making the approach applicable across all diverse landscapes. New approaches have also been proposed for dealing with pixels containing whitecaps and foam (Dierssen, 2019). Finally, as further highlighted below, inverse methods that simultaneously retrieve parameters from a coupled ocean and atmosphere system without individual atmospheric correction routines are becoming more common (Chomko et al., 2003; Stamnes, 2003).
Cooking up an Algorithm Storm
The number of unknown parameters that can be retrieved from hyperspectral data will be much less than the number of spectral bands because of the correlated information between wavebands (Dekker et al., 2018). Therefore, hyperspectral data may be most diagnostic for environmental conditions that result in independent variation of the different optically active constituents, or to collections of environments (regional or global scope) each with its own correlation structure. Those can include systems with surface blooms of different algal communities that present unique absorption and scattering properties, and water masses that are potentially influenced by land processes (e.g., erosion, organic leaching), benthic-pelagic coupling (e.g., tidal and/or wind driven resuspension of sediments), and flocculation driven by time-space dependent turbulence and chemistry (estuaries). Much of the information content studies have been done with simulated data that may not fully represent the inherent optical properties of natural systems and do not typically model inelastic processes such as Raman scattering and fluorescence (Lee et al., 2007; Wolanin et al., 2016), polarization (Harmel, 2016) and directional dependency, particularly for three-dimensional benthic structures (Hedley et al., 2017, 2018). Presently, there is a lack of hyperspectral backscattering data for different types of aquatic particles for use in simulations. Therefore, optical closure between models (Werdell et al., 2018) and measurement techniques is challenging in bloom conditions and complex water types. To aid in algorithm development, we have compiled a list of hyperspectral datasets that may be useful for evaluating different approaches for estimating aquatic parameters, including phytoplankton and benthic community composition (Table 4). With more hyperspectral field data across a wide variety of conditions (Dierssen et al., 2020), particularly for applications for inland and coastal waters, we may find potentially new information in parts of the spectrum previously overlooked. Even without this further information, hyperspectral data will help reduce the uncertainty in the retrieved parameters (Werdell et al., 2018).
Blondeau-Patissier et al. (2014) provide a detailed description of many common indices and numerical techniques used in multi-spectral ocean color remote sensing. A more recent review of ocean color methods for quantifying the inherent optical properties (IOPs) grouped algorithmic approaches into three broad categories; empirical, semi-analytical (also referred to as quasi-analytical), and spectral libraries (also referred to as look-up tables) (Werdell et al., 2018). However, we believe that a more general framework is possible to unify approaches and reduce community-specific jargon with modern terminology of computer and data science. Empirical relations are inevitably introduced in our methods because purely analytical approaches are not possible from remote sensing above the water surface due to complete internal reflection which limits the angular distribution of light crossing the air-sea interface and lack of information on the vertical distribution of particles (Zaneveld, 1995). More information is available from sensors simultaneously measuring at multiple angles from a sea surface. Still, such signals can be challenging to interpret given variable path lengths through the atmosphere and the ever-changing nature of sea surfaces with non-random distributions of waves and swells. Ocean color sensors typically have a small solid angle of detection and measure light fields that originated from near-nadir directions (upwards) within the water (McClain, 2009).
Rather than merely list algorithms, we step back and consider the fundamentals of developing an algorithm with independent steps of “Data Transformations” and “Retrieval Algorithms.” This grouping is done because many of the techniques are often identified with the name of the numerical transformation (e.g., derivatives, principal components). Still, the parameters of interest are identified through statistical techniques like multiple linear regression. Many of the so-called “Semi-analytical” algorithms fall within the broader category of “Spectra as References,” where the remote sensed spectrum is used as a reference that is matched to spectra modeled using approximations of the forward radiative transfer equations and spectral shape functions. Some algorithms are less sensitive to uncertainties in atmospheric correction such as those focused on narrowband features, and others may require highly calibrated data across the spectrum. While uncertainties can be quantified directly with some techniques, like so-called Optimal Estimation (Rodgers, 1998), techniques have been proposed for propagating uncertainties through other types of algorithms (McKinna et al., 2019).
Data Transformations
Data transformations are performed to amplify spectral signals that are related to the specific components to be retrieved. Sometimes the transformed data becomes the parameter of interest, such as the normalized fluorescence line height or normalized difference vegetation index (NDVI). Still, often the transformed data is then related to a parameter of interest via other solution methods as outlined in Retrieval Algorithms below. In the latter case, transformations are conducted prior to retrieval of the parameter of interest. The most fundamental transformation applied in remote sensing is the normalization of the measured radiance by the estimated downwelling irradiance to remove variations induced by the quantity and quality of the incident light field, increasing the correlation of the measured signal across multiple conditions with the system properties of interest. After this transformation to reflectance, several methods are applied to amplify the signal further. Listed below are some of the most common transformations, such as normalization of the signal magnitude, reflectance line heights, spectral derivatives, and coordinate system transformation.
Band Math
The simplest transformations are conducted by applying a common mathematical operation or function to the reflectance spectrum. One classical example typically used in multispectral algorithms is to conduct a ratio of two different bands to evaluate relative differences rather than the absolute magnitude (Dierssen, 2010). Similarly, the normalized difference index (NDI), a generalized version of the NDVI, is calculated as the difference between two bands normalized by the sum of the two bands (Hu and Feng, 2016). A common transformation conducted in hyperspectral analyses is to normalize all bands to a given band, approximately removing the dominance of magnitude differences over the signal, enhancing the relative spectral differences. A similar effect can be achieved by normalizing the spectra to its spectral integral (Spyrakos et al., 2018).
Hyperspectral data are particularly suited for investigating absorption and fluorescence features that appear as local minima and maxima in the reflectance spectrum. Such feature-centric algorithms are mathematically simple and work well when the feature of interest covers a narrow spectral range and dominates the reflectance spectrum. Narrow-band analyses are generally less sensitive to uncertainties in the atmospheric correction. A common method for finding such narrowband features is to produce a correlation matrix quantifying the relationships between the feature of interest and all pairs of wavebands across the spectrum. For example, such an approach was used to assess the age of floating seagrass wrack using a narrowband water absorption feature at 750 nm (Figure 2) (Dierssen et al., 2015).
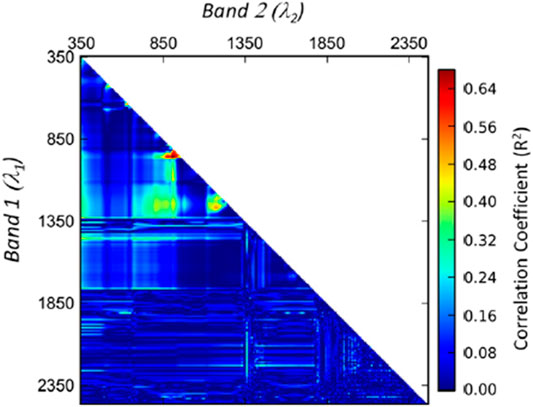
FIGURE 2. A pairwise correlation analysis can provide information on narrowband features that may be related to a parameter of interest. (Modified from Dierssen et al. (2015)).
Line heights (LH) can be calculated following from a continuum formed by the line between bands (B1 and B3) surrounding the spectral feature of interest (B2) following:
Using widely spaced multispectral data for line heights, such as in the NASA normalized fluorescence line height algorithm (nflh) (Behrenfeld et al., 2009), can be problematic because the selected bands may not be isolated around the feature of interest. The nflh algorithm, for example, uses 748 nm for Band 3, which is over 60 nm away from the fluorescence peak. Such a distant anchor point works reasonably well for average Chlorophyll waters (Figure 3A), but produces an order of magnitude higher nflh under high suspended sediment load with no fluorescence peak (Figure 3B) (e.g., Zhao and Ghedira, 2014; Amin and Shulman, 2015).
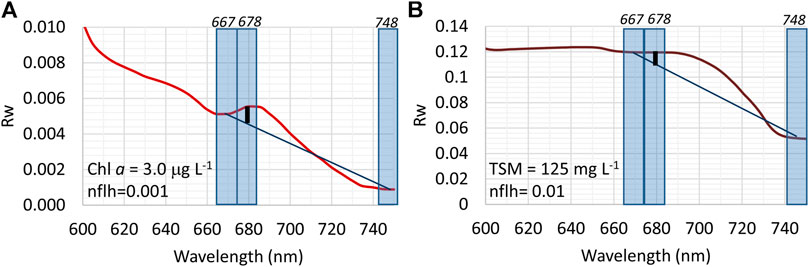
FIGURE 3. Narrowband features like chlorophyll fluorescence can be inaccurately estimated when using multi-spectral bands that are distant from the feature, such as the blue bands used in the normalized fluorescence line height (nflh). Examples of (A) water-leaving Reflectance (Rw) spectrum with a typical chlorophyll fluorescence feature and (B) a spectrum representative of high sediment water with no observable chlorophyll fluorescence leads to an erroneously high nflh.
Hyperspectral line heights have been used to detect specific accessory pigments like Chlorophyll-c3 at 467 nm (Astoreca et al., 2008). Variations on line heights and shifting peak wavelengths can also untangle the complexities of fluorescence in coastal waters where scattered light (elastic reflectance) significantly influences the fluorescence band (Gilerson et al., 2007) and for some types of bloom or seasons when fluorescence is not as prevalent as scattering enhancements observed in the far red wavelengths, also referred to as the “red edge” (Gower et al., 2008; Matthews et al., 2012; Freitas and Dierssen, 2019). Additionally, hyperspectral data can also reveal different fluorescence peaks such as the yellow fluorescence observed from phycoerythrin pigment in the ciliate Mesodinium rubrum (Dierssen et al., 2015).
Derivative Analysis
Spectral derivatives solved as finite difference derivative approximations (e.g., Torrecilla et al., 2011) can reduce relative magnitudes variation in the spectrum, enhancing the signal of the presence of local minima and maxima. Spectral derivatives have long been used to evaluate phytoplankton pigments such that a trough in reflectance is related to absorption and a peak in reflectance associated with reduced of absorption or pigment fluorescence. Since the second derivative is a measure of curvature, it amplifies high-frequency features of interest that are otherwise quite subtle within the spectrum and depresses low-frequency changes, such as the gradual decrease in absorption due to colored dissolved and detrital matter. However, this also means that very high-frequency features, such as band-to-band differences due to sensor noise and relative calibration errors, will also be amplified within the derivative spectrum. For this reason, spectral smoothing is often conducted before the application of derivative analysis, and various techniques have been recommended for how to smooth data for effective derivative analysis. Often, a statistical approach like multiple linear regression or optimization is then used to link the derivative spectrum to the parameters of interest, but decision trees based on arguments of the maxima and minima (argmax and argmin) have also been used (Lubac et al., 2008). Application of derivative analysis to aquatic remote sensing data has been useful for detecting the presence of pigments associated with phytoplankton including harmful species (Bidigare et al., 1989; Craig et al., 2006; Lubac et al., 2008; Cheng et al., 2013) and benthic organisms associated with shallow coral reef habitats (Hochberg and Atkinson, 2000; Louchard et al., 2003).
Coordinate Transformations
Transformations of the coordinate system are typically used to produce a set of orthogonal variables with uneven distribution of the total variance, as in classical Principal Components Analysis (a.k.a., Empirical Orthogonal Functions). The new variables can be used as a descriptive multivariate method of the optical data, in which case further transformations of the variables might be relevant for interpretation (e.g., varimax PCA). They can also be used for prediction in methods that condition the rotation in the optical data matrix to achieve an improved classification of (e.g., Linear Discriminant Analysis) or correlation with the dependent variable (e.g., Partial Least Squares Regression). In its simplest application for retrievals, the coordinate transformation with PCA is an isolated step after which the new variables are included in multilinear regression. Preisendorfer also provided a fundamental text on PCA (Preisendorfer, 1988). These approaches are increasingly being used to identify different groups of phytoplankton and suspended mineral composition (Catlett and Siegel, 2018; Ortiz et al., 2019; Bracher et al., 2020a; Smith and Bernard, 2020). A related concept is the transformation of the hyperspectral information to chromaticity coordinates (hue angle and saturation).
Retrieval Algorithms
A wide variety of hyperspectral methodologies rely on machine learning techniques across the broad field of environmental science, as detailed in extensive reviews over the last decade (reviewed in Paoletti et al., 2019). The basis of most algorithms is considered within the framework of machine learning, where statistics theory is used to find patterns in data or to estimate the process that generates the data. In the first case, methods fall in the class of unsupervized machine learning with cluster analysis and other methods that also take in consideration the spatial context, as in object based image analysis. In the latter case, methods are either in the context of supervised machine learning, spectral matching or linear matrix inversion. Within this group, a variety of approaches are used to related a spectrum, either measured at-sensor, atmospherically corrected (Finding the Ocean Needle in the Atmospheric Haystack), or subject to one or more transformations (Data Transformations), to a parameter or set of parameters of interest. Parameters can include classes of optical water types, optical properties including absorption and backscattering, and biogeochemical quantities of interest like pigment or sediment concentrations. Clearly, the more representative the training data available across space and time, the better the models will perform across the domain. The field of machine learning is advancing rapidly and influencing the way nearly all algorithms are developed, tested, and implemented. Some approaches are focused more on prediction rather than understanding or description of processes, and many final algorithms involve multiple steps including data transformations and statistical analyses. Here, we group the retrieval approaches by the way the spectra are used in the algorithm (Figure 4).
Spectra as Descriptors
The following section highlights some of the common methods where spectra are used to estimate indices or categories.
Optical Indices
In addition to various band math indices like normalized fluorescence line height discussed above in Data Transformations, many different other types of indices have been proposed to detect differences between floating Sargassum and seagrass wrack (Dierssen et al., 2015) or to detect different harmful algal bloom formers (Smith and Bernard, 2020). A spectral classification index was recently developed by Vandermuelen et al. (2020) to quantitatively describe the shape of a hyperspectral dataset using a weighted harmonic mean. Similar to transformations of hyperspectral data to chromaticity coordinates that relate to colors observed by the human eye (Dierssen et al., 2006; Wernand et al., 2013), the Apparent Visible Wavelength (AVW) represents a one-dimensional geophysical metric of “color” that is correlated to spectral shape. Such simple metrics correspond to conceptual changes that can easily be explained to a broad audience (e.g., the water is “greener” or “bluer”) and can be diagnostic of slight optical changes that are masked in chlorophyll retrievals.
Cluster Analysis
Many different indices have been developed to classify water types broadly from clear to turbid similar to the original water classes of Jerlov (1963) or to identify specific types of algal blooms or properties of interest. Clustering methods are frequently used to partition out different types of spectra locally or globally and track water mass movement and change over time (Lubac and Loisel, 2007; Aurin et al., 2010; Uitz et al., 2015; Ye et al., 2016). Clustering methods can include K-means, fuzzy C-means, hierarchical clustering (e.g., Euclidean distance, Ward linkage), and hybrid methods. Classification approaches in the pelagic ocean (i.e., “Seascapes”) have been used to identify water masses with particular biogeochemical features that can be linked to biomes and biophysical provinces and provide rapid information to decision-makers about the changing environmental conditions (Kavanaugh et al., 2016). Seascapes are classified using a suite of synoptic time-series observations from satellites (Montes et al., 2020).
Object Based Image Analysis
Traditional pixel-based image classification considers all pixels similarly without the context of neighboring pixels or the image as a whole. However, OBIA segments an image and groups pixels together into vector objects (Blaschke, 2010). Such approaches are not common to ocean optics given the relative homogeneity of ocean surfaces and the lack of structures like rectangular buildings and long and narrow roads. However, these techniques are gaining popularity in optically shallow water remote sensing where bottom features may have spatial patterns and features (Phinn et al., 2012; Roelfsema et al., 2018). A variety of methods are available to segment an image based on the shape and spectral information prior to classification (Table 2). This can also prevent unwanted salt and pepper retrievals where classes vary unrealistically within an aquascape.
Spectra as Predictors
The following section presents example techniques where the spectra or the transformed spectra are used as independent variables to predict parameters of the system. Prediction requires the supervised approach to machine learning and comprises methods of classification and regression analysis. The term “classification” is used when the dependent variable is categorical and the term “regression” is used when the it is continuous. Most familiar methods are those of parametric regression using linear or non-linear models, but nonparametric models such as artificial neural networks are gaining popularity. Below we present an overview of some common methods.
Parametric Regression
Parametric regression imposes a structure to the modeled relationship between spectral data and a continuous target parameter. This requires specifying the relationship structure a priori, from dedicated exploratory data analysis. For complex data relations, it demands sophistication in terms of mathematical functions and orthogonal basis expansions. A benefit of this approach is that it is data-efficient and can be applied to sparsely populated sample space. As a contrast, nonparametric regression does not impose a model structure and often requires a more densely populated sample space. As discussed above, data is often transformed first and then linked to a parameter of interest using linear or nonlinear regression techniques. This type of approach has been used for the current band ratio chlorophyll a algorithms and for other parameters like ancillary pigments, phytoplankton groups, and total suspended matter (Catlett and Siegel, 2018; Ortiz et al., 2019; Bracher et al., 2020; Smith and Bernard, 2020). For many aquatic parameters, the regression analysis is conducted on log-transformed data to cover the full range of environmental conditions.
Neural Networks
Recently, Artificial Neural Networks have gained much popularity due to their flexibility in addressing different applications and can be used with raw data or applied after data transformations. Training data sets may consist of field measurements or model simulations representing the wide range of conditions found in the natural world. Researchers “train” a neural network over time by analyzing its outputs on different problems and comparing them with the correct answers. As in any regression, model development must consider trade-offs between adding complexity to improve prediction and increasing the risk of overfitting the solution to the training data. Accumulation of global datasets covering a wide range of conditions and advances in computer processing power have enabled machine learning researchers to vastly expand the size and complexity of the models, simulate larger datasets for training, train the models faster, and overall achieve better results. Neural networks have been gaining popularity in the aquatic remote sensing and used to derive the diffuse attenuation coefficient (Jamet et al., 2012; Chen et al., 2014), uncouple constituents in optically complex waters (Doerffer and Helmut, 2000; Ioannou et al., 2013), map shallow-water benthic features (Sandidge and Holyer, 1998; Filippi, 2007; Liu et al., 2015), and to evaluate features across different scales (e.g., Hieronymi et al., 2017; Pahlevan et al., 2020). Salcedo-Sanz and colleagues, for example, implemented a neural network to map shallow benthic coral reef features and concluded that neural network approaches generally out perform traditional empirical approaches to the problem such as supervised and unsupervized classification and regression analysis (Salcedo-Sanz et al., 2016).
Decision Trees
In a decision tree, the analysis begins at the top of the tree where a single feature of the data is considered and analysis proceeds downwards based on the decisions made in previous levels of the tree. At each level of the tree, the computation splits off into two or more children nodes based on features of the data. The computation ends when you reach a terminal node at the bottom of the tree. Even in the simplest algorithms, many decisions are required to assess whether reflectance in a pixel is appropriate for subsequent analysis. Some of these decisions may be considered as part of the “Atmospheric Correction” decisions such as “Is this a water pixel?” or “Is there a cloud or cloud shadow obscuring the pixel?” or “Is this optically deep water?” Many common algorithms in ocean color are decision trees that use different approaches depending on criteria established from the spectrum, such as NASA’s current Chlorophyll a algorithm which uses two different algebraic approaches depending on whether the pixel is considered a high or low chlorophyll pixel. Such decisions often involve subjective or fitted thresholds which determine which approach will be used. Setting thresholds can be challenging, and such delineation often does not apply widely across different images collected in space and time and require tuning for each application. Decision Trees are common for differentiating water types, benthic flora and floating vegetation (Hill et al., 2014; Ye et al., 2016; Castagna et al., 2020).
The supervised Random Forest (RF) classifier belongs to the group of classifiers that use decision trees and independent random vectors, with the approach driven by the relationship between the training and the response dataset rather than starting with a data model (Breiman, 2001). It provides several benefits over other supervised classification algorithms, including the ability to calculate internal error estimates and variable importance, as well as the capacity to handle weak explanatory variables (Gislason et al., 2006). The approach has received attention within the remote sensing community due to both the classification accuracy and processing speed. Applications include the classification of submerged vegetation (Espel et al., 2020) and estimation of bathymetric depth (Yunus et al., 2019).
Spectra as References
A different approach to estimation of a continous variable occurs when the measured spectrum is taken as a reference and the methods involve calculating the mixing of endmembers or the system composition that would result in the observed spectrum. This approach is typically solved numerically with optimization, where the measured spectra is taken as a reference to be matched against by spectra in a spectral library. The parameter values of the best matching spectra are taken as estimates for the measurement. An analytical solution via linear matrix inversion is possible in linear problems, such as linear mixing of endmembers or when using a linear approximation to the radiative transfer equation. In the latter, the analysis calculates the magnitudes (eigenvalues) associated with predefined relative spectral shapes (eigenvectors) of system components that would result in the observed spectrum.
The spectral library can contain previous measurements in which the parameters of interest are known, or can be built from simulation with a physical model. In the case of simulations, the library can be generated dynamically at each iteration step or through the use of a static library or “look-up table” of measurements or pre-computed simulations. Iterative approaches can be computationally expensive when applied to each pixel in a remote sensing image. The computational time involved in searching the library for the best matching spectrum, however, may also be high given the number of properties that must be considered, the number of spectral bands, and how each parameter varies across the expected range. Library size can be decreased to an extent by limiting the dimensionality of the hyperspectral signal to regions of the spectrum containing most of the information pertaining to the properties of interest. Spectral libraries have been developed and applied to differentiating phytoplankton groups or biodiversity (Palacios et al., 2015) and in optically shallow water to retrieve bottom depth and benthic cover (Louchard et al., 2003; Mobley et al., 2005).
Many of the so-called “Semi-analytical Models” fall within the broader category of optimization using a physical model representative of the system. Such techniques involve: 1) Formulation of the problem in a forward model; 2) Iterating or inverting the forward model algebraically or numerically; and 3) Finding the best solution in terms of uniqueness, accuracy and efficiency of the model. The formulation of the forward model in aquatic models often relate inherent optical properties of the water column (typically the absorption and backscattering properties of the water constituents) through radiative transfer theory (Gege, 2017). To-date, most of the aquatic models have used an approximation of the radiative transfer equation relating the ratio of backscattering to absorption with an empirical parameter, gi, that accounts for the angular distribution of incoming and scattered light (Figure 5A) (Gordon and Brown, 1988). A more detailed formulation of the forward model was recently proposed, the Zaneveld-Twardowski-Tonizzo (ZTT) Model, that parameterizes the angular distribution of both incoming and upwelled light in the water column (Figure 5B) (Twardowski and Tonizzo, 2018). Angular considerations become more critical when retrieving optical properties in more complex coastal and inland waters.
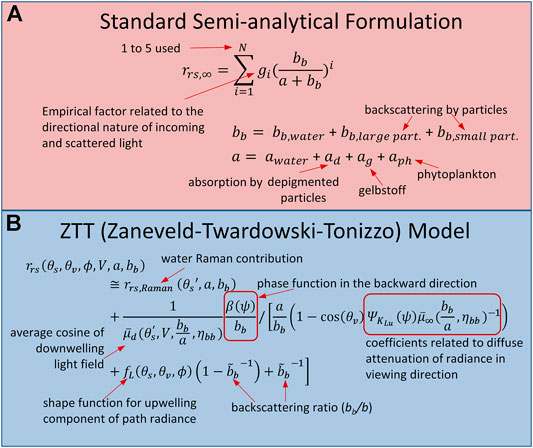
FIGURE 5. Physical models link the water column optical properties to the light field measured by a satellite. (A) Most semi-analytical algorithms use a simplification where the directional nature of light is approximated with empirical constants (Werdell et al., 2018). (B) A newly posed ZTT model parameterizes the shape of light in the backward direction (phase function), the shape of the upwelling component of path radiance and the average cosine of downwelling light Twardowski and Tonizzo (2018).
Many different numerical solutions have been used from non-linear spectral optimization to adaptive linear matrix inversion (e.g., see Table 4 of Werdell et al., 2018). Some form of empirically determined spectral shape functions are required to retrieve the inherent optical properties of different constituents, including particulate backscattering and absorption by phytoplankton, gelbstoff, and depigmented particles. The term “depigmented particles” is used rather than tripton, detritus or non-algal particles because the measurement includes all types of depigmented particles, including algal cells (Neeley et al., 2018). The solution involves varying the quantities of each parameter until the difference between the derived and the measured spectrum is sufficiently minimized. Many solution methods include techniques to avoid local minima and maxima and sample the whole parameters space (e.g., Dierssen et al., 2019).
Derived inherent optical properties can then be related to other parameters of interest such as concentrations of chlorophyll a, total suspended matter, and potentially estimates of phytoplankton community composition via supervised regression techniques discussed above. In addition to the optical properties regularly produced from ocean color imagery (Lee et al., 2002; Werdell et al., 2013), similar approaches have also been successfully used in simultaneously retrieving water column depth, inherent optical properties and benthic diversity from hyperspectral imagery (Hedley et al., 2016b; Garcia et al., 2018; Dierssen et al., 2019). A significant advantage of optimization approaches is that uncertainties due to instrumental and environmental noise can be estimated numerically via the addition of spectral noise before inversion (Garcia et al., 2014).
A class of optimization techniques well suited for hyperspectral imagery are referred to as “Optimal Estimation” using Bayes’ theorem (Rodgers, 1998). It entails specifying probability distributions for the natural variability of the hidden physical processes (“priors”), a distribution for the spectral measurement errors, and an explicit forward model. Assuming all distributional parameters are known, the retrieved state is then the maximum a posteriori (MAP) estimate of the state given the observed, noisy radiances. Specifying the sources of variability within a Bayesian framework allows for the parameterization of the sources of error and the ability to propagate them into estimates of retrieval uncertainty (Nguyen et al., 2019).
Unlocking Biodiversity: Neither Fish nor Fowl
Biodiversity, the diversity within species, between species, and of ecosystems, is a fundamental characteristic of Earth, and underpins the structure and functioning of Earth’s ecosystems, as well as human health, nutrition, and economic livelihoods (Secretariat of the Convention on Biological Diversity, 2020). Many of the benefits that we gain from aquatic ecosystems depend on the number and abundance of species, the interactions between the organisms and the environment, and the number of different habitats (Malone et al., 2014). In recognition of the global threats to biodiversity, the Convention on Biological Diversity (CBD) has listed several targets for preserving aquatic biodiversity including: sustainable management of fisheries and aquaculture, prevention of nutrient pollution, as well as minimization of anthropogenic pressures on coral reefs and other vulnerable ecosystems. Measures for biodiversity referred to as Essential Biodiversity Variables (EBVs) are commonly grouped into six classes: genetic composition, species populations species traits, community composition, ecosystem structure, and ecosystem function. Since Muller-Karger et al. (2018), more biodiversity variables for phytoplankton are routinely produced from satellites like Sentinel-2 OLCI, including pigments (chlorophyll a and phycocyanin) and certain taxonomic groups like cyanobacteria are routinely produced from aquatic remote sensing platforms) (Figure 6).
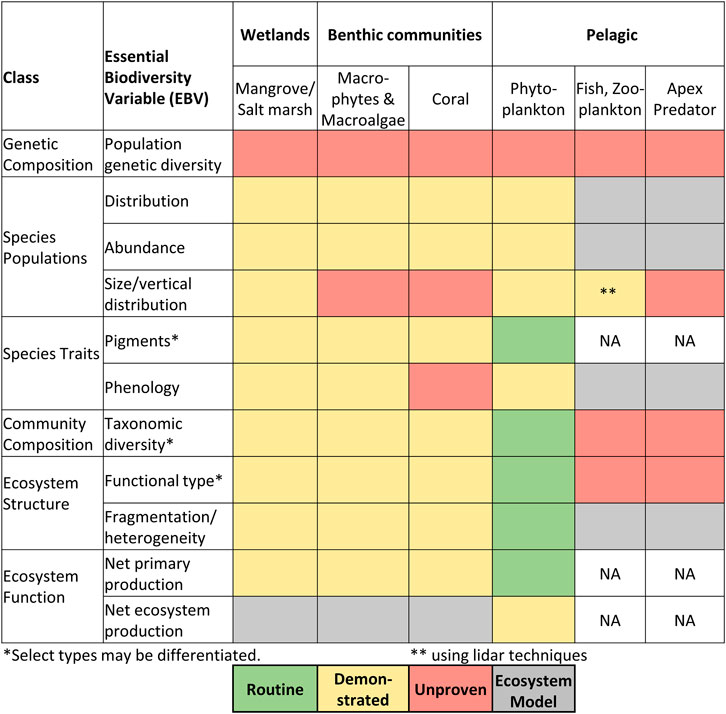
FIGURE 6. Current capabilities of remotely sensed data for measuring Essential Biodiversity Variables modified from Muller-Karger et al. (2018). “Unproven” indicates that methods have not yet been developed to collect these measurements from remote sensing imagery. “Demonstrated” are methods that have been demonstrated and could potentially be produced with hyperspectral imagery. “Routine” indicates measurements that are produced regularly. “Ecosystem model” indicates EBVs that can be predicted on the basis of ecosystem models that may incorporate remote sensing imagery. “NA” indicates that the observation is not applicable.
Measurements of distribution, abundance, and phenology of bulk phytoplankton in the open ocean (i.e., derived chlorophyll-a) are regularly produced from satellite remote sensing. Parameters that Muller-Karger et al. (2018) classified as “demonstrated limited cases” could become operational with regular, hyperspectral observations. In particular, high spatial and radiometric resolution hyperspectral data is required with a high repeat frequency would enable measurements of primary producers such as phytoplankton, wetland and submersed plant and macroalgae species and distribution (including vertical), pigments and other traits, taxonomic diversity and functional types (Santos et al., 2012; Anderson et al., 2016; Santos et al., 2016; Bracher et al., 2017a; Hedley et al., 2017). Where species cannot be measured directly, hyperspectral remote sensing can be used to monitor drivers and proxies of biodiversity. For example, hyperspectral remote sensing can be used to discriminate the water quality constituents and the spectral underwater light field of the aquatic environment, which forms the niche for many fish species. Spectral diversity of the primary producers in aquatic and wetland ecosystems can be used to make inferences about biodiversity and ecosystem function (Muller-Karger et al., 2018; Rebelo et al., 2018). Indeed, monitoring of biodiversity of benthic and pelagic organisms in aquatic ecosystems will advance remote sensing capabilities with diverse applications that serve both the research and management communities (Figure 7).
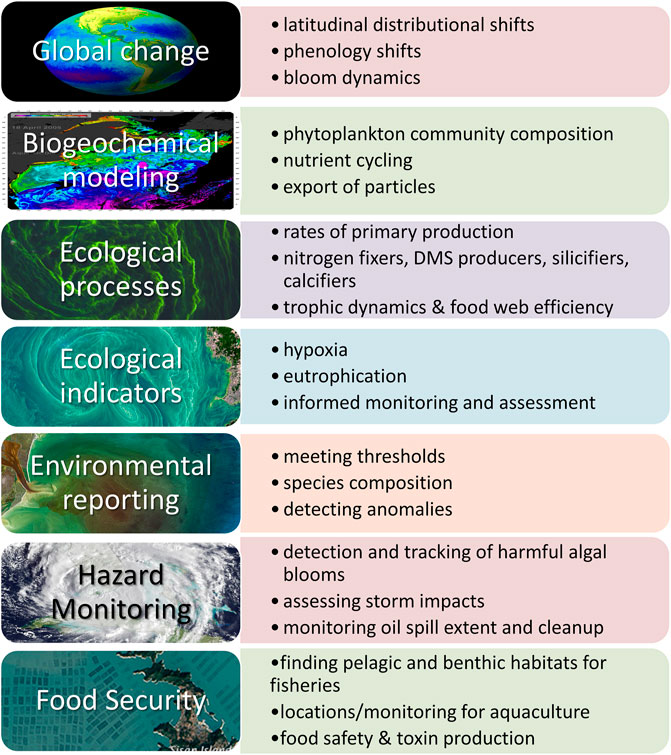
FIGURE 7. A host of new applications will be available with better discrimination of pelagic and benthic biodiversity promised by hyperspectral imagery. Modified from Dierssen et al. (2020).
Spectral “fingerprints” can be used to elucidate different light absorbing, scattering, and fluorescence properties that are related to the biodiversity of photosynthesizing flora and fauna in the water column (pelagic), on the seafloor (benthos), and floating on the sea surface. Photosynthesizing flora includes different assemblages of phytoplankton which provide the bulk of the photosynthesis in the world ocean, and photosynthesizing fauna includes tropical coral reefs and select mixotrophic plankton like ciliates. Algorithms for estimating pigment concentration are usually associated with large uncertainty when applied globally because of the variability in size and shape of phytoplankton and cellular levels of pigment related to environmental conditions (Dierssen et al., 2020). Increasingly studies show that only a limited number of phytoplankton groups (∼5) may be differentiated globally using hyperspectral absorption spectra in visible wavelengths (Kramer and Siegel, 2019). The spectral signatures of the different phytoplankton groups of interest are similar within the uncertainty of the measurement largely because of the considerable overlap in pigment composition between different groups (Cael et al., 2020). Recent analyses of the oligotrophic ocean from the Tara Oceans expedition, for example, suggests that only around five different phytoplankton groups can be reliably differentiated from their spectral signatures (Cael et al., 2020). Data from the Santa Barbara Channel, California revealed that around five phytoplankton pigment communities, which are covarying assemblages of phytoplankton groups, could be differentiated based on their spectral properties (Catlett and Siegel, 2018). Similarly, data from the upwelling waters around South Africa also was used to retrieve five different phytoplankton communities relevant to the aquaculture industry (Smith and Bernard, 2020). However, remote sensing algorithms and biogeochemical models can be derived and tuned for the regional or local phytoplankton groups down to specific taxa, if they are known to occur in an area. And, we have only begun to assess the hyperspectral scattering and fluorescence properties that may also aid in differentiating different types and stages of blooms, as well as relationships to seasonal trends and other remotely sensed parameters including polarization parameters, temperature, photosynthetically available radiation, and salinity.
Peering Into the Abyss
As the pressures of human population and climate change on coastal and inland aquatic environments rise, the need will increase for more accurate and timely environmental information to support research and resource management. With an economic value to the Asia Pacific region alone currently estimated at U.S. $372 billion, jumping to U.S. $1.35 trillion by 2030 (Commonwealth of Australia, 2019) Earth and marine observing is emerging as a significant business in its own right. Hyperspectral remote sensing, in particular, is becoming a powerful mapping and survey tool that is well suited for optically complicated coastal and inland aquatic environments, as well as a host of other applications of societal importance, such as in areas of agriculture, mineral exploration, forestry, and urban planning. This broad scope of application will ensure that hyperspectral technology and access will continue to improve with time. It is, therefore, reasonable to speculate how future technological advances may improve how we sense and understand aquatic environments and to point out key challenges to overcome in order to capitalize on future technology (Figures 7).
As noted in Suborbital Systems, sensor miniaturization is one of the most exciting recent advances in hyperspectral technology for observing highly heterogeneous benthic environments. We can now mount small and lightweight imaging spectrometers on drone platforms to fly at low altitude (Parsons et al., 2018), or within submersible housings to acquire data with an unparalleled spatial resolution (Chennu et al., 2017; Mogstad and Johnsen, 2017; Bongiorno et al., 2018). The combined hyperspectral hyperspatial data will allow us to revisit the research findings in aquatic and marine applications using point-based spectrometers of the early 2000s and apply it in a new spatially explicit manner, while including context, shape, size, and textural measures for more detailed classifications. Further, with the availability of sensors of the caliber that were previously limited to military or high-end commercial consulting, data capture is rapidly decentralizing and distributing within an expanding user community. This expansion of platforms results in potentially many more data sets available to be analyzed and exploited.
The shift in imaging system access and control from external organizations and large satellites to the individual researcher with UAS is analogous to transferring computing power from mainframes to personal computers in the 1980s. By embedding the technology within research activities, users have more flexibility in deciding when, where, and how frequently to collect observations, thus increasing data value and, therefore, project efficiency. Users can readily create custom UAS-based systems, or purchase complete systems including sensors, aircraft, and mission planning and data analysis software, tailored to applications (Adão et al., 2017). This is a careful balance though, as the adoption of UAS systems often comes at the expense of loss of coverage across large areas without great investment in personnel time and extremely large data volumes still not manageable for many individual research groups or investigators (Bolch et al., 2021).
However, more data is not always synonymous with better data. Further, neither necessarily leads to better decisions. Decentralized data capture can lead to inconsistencies in quality, making comparisons between studies difficult. By popularizing the collection of remote sensing data, standardized community best practices in data capture and quality control are needed to avoid ‘garbage in, garbage out’ scenarios (Joyce et al., 2019). Development of standardized instrument calibration procedures and data capture protocols, including thorough accompanying metadata (Janssen et al., 2012; Kalinauskaitė, 2017), should be a future priority of the user community. For example, no accepted protocols are currently available on how to best calibrate commercial off-the-shelf hyperspectral imaging spectrometers for aquatic applications. Such standardization will be particularly important when conducting multi-temporal analyses and when sharing datasets between users.
Data sharing is widely recognized for promoting innovation and growth. Recent moves toward creating open and FAIR (findable, accessible, interoperable, and reusable) data archives have largely contributed to an acceleration of new remote sensing applications. One only needs to look to the vast number of new and exciting applications of Landsat data that followed open access to the 30 + year archives to realize the value in this approach (Wulder et al., 2016; Zhu et al., 2019). This challenges the assumption that we only obtain value through a financial transaction when the data collector sells its raw data. Instead, FAIR data policies place value on the benefits of derived products. We expect that continued FAIR data practices will continue to remove barriers to remote sensing technology, including the availability of hyperspectral imagery, and result in a greater diversity of people engaging with the data to address the many environmental challenges facing society.
To allow users to truly exploit raw data, derive products, and create new knowledge, it is essential to support FAIR data with the appropriate infrastructure and systems architecture on similarly accessible platforms (Janssen et al., 2012; Kalinauskaitė, 2017). Supporting open-source software options such as QGIS (see Table 2) are critical in continuing to grow relevant applications through open-minded community collaboration. Yet, locally hosted software options are unlikely to be the future for hyperspectral data processing. Hyperspectral data fits within the category of “big geo data” (Krämer and Senner, 2015) and is, therefore, better suited to scalable and distributed cloud processing rather than local computing capabilities (Wilson et al., 2018; Haut et al., 2019). Although cloud-based high-performance computing (HPC) is not a new concept (e.g., Plaza et al., 2011), its intersection with hyperspectral data for environmental analyses–particularly in aquatic environments–is in its infancy and remains an area for considerable future growth.
Technological advances in computing technology, data storage, and processing combined with the potential for increased data availability due to decentralized data capture presents exciting challenges and opportunities for hyperspectral aquatic remote sensing in the future. The inherent high dimensionality, noise, and intra-class variability plague the deep learning models that are otherwise becoming a favored means for analyzing remotely sensed data (Paoletti et al., 2019). However, with distributed analyses, opportunities for community collaboration and crowdsourcing emerge. Examples of this dynamic abound within the rapidly developing field of machine learning and artificial intelligence (Chirayath and Li, 2019).
Similarly, global initiatives such as AquaWatch and IOCCG connect global networks of people, sensors, and platforms to provide an integrated approach to aquatic ecosystem analyses (Table 5). Such international groups can also provide a framework for broad data access spanning nations and individual satellite missions. In addition, the ocean optics community should be engaged closely with the broader scientific community involved with biogeochemical and ecosystem modeling (IOCCG, 2020). Going forward, better quantification of parameter uncertainties will provide ocean modelers with the metrics needed for assessing model performance. Finally, merging of hyperspectral imagery with other types of remote sensing, such as polarimetry (Chowdhary et al., 2019), provides exciting new avenues for growth in what can be retrieved across Earth’s diverse aquascape. There will continue to be a mutual benefit for the hyperspectral community to continue to engage more broadly with Earth and marine observations.
In conclusion, the field of hyperspectral aquatic remote sensing is on the cusp of providing novel ways to sense aquatic ecosystems and new parameters that can be better used by decision-makers and scientists alike. The lists of resources and tools provided here are only a starting point and will expand over time as more hyperspectral data from satellites, drones and other platforms becomes accessible to the larger community of scientists and practitioners. To aid in this effort, we recommend posting resource information on public forums and updating resource lists and tools regularly. While the foundations for algorithm development and data processing are becoming well established, a significant gap is the availability of field data for both algorithm training and validation across the global ocean and inland waterways including common and episodic environmental conditions. This includes not just the collection of well-calibrated hyperspectral imagery but merged datasets coupling hyperspectral reflectance with ancillary biodiversity and biogeochemical properties obtained with consistent methodology and metadata. Such efforts will require global cooperation and data sharing. Indeed, interdisciplinary, international, commercial, and citizen collaborations will drive us forward as a community to realize the hype of hyperspectral remote sensing and provide important new insights into the blue marble Earth.
Author Contributions
HD was the lead author of the manuscript. All of the coauthors contributed to conceptual discussions about paper content and writing and editing of the manuscript.
Funding
Funding was provided by the Alliance for Coastal Technologies program sponsored by the National Oceanic and Atmospheric Administration/US Integrated Observing System (US.IOOS Award number: NA16NOS012OO). Additional funding was provided to HD from NASA Ocean Biology and Biogeochemistry (80NSSC20M0206). AC was funded by the Belgian Science Policy Office (BELSPO) project HYPERMAQ (SR/00/335). Support for SA was provided through the Naval Research Laboratory base funding program.
Conflict of Interest
SL was employed by Pixalytics Ltd.
The remaining authors declare that the research was conducted in the absence of any commercial or financial relationships that could be construed as a potential conflict of interest.
Acknowledgments
We would like to acknowledge the organizers of the 2018 Alliance for Coastal Technologies (ACT) Hyperspectral Imaging of Coastal Waters Workshop, Mario Tamburri the director of ACT, and all workshop participants. We would also like to acknowledge Astrid Bracher who provided additional resource information.
References
Aasen, H., Honkavaara, E., Lucieer, A., and Zarco-Tejada, P. (2018). Quantitative Remote Sensing at Ultra-high Resolution with UAV Spectroscopy: a Review of Sensor Technology, Measurement Procedures, and Data Correction Workflows. Remote Sensing 10, 1091. doi:10.3390/rs10071091
Acker, J. G. (2015). The Color of the Atmosphere with the Ocean below: A History of NASA’s Ocean Color Missions. Scotts Valley, CA: CreateSpace Independent Publishing Platform.
Ackleson, S. G., Smith, J. P., Rodriguez, L. M., Moses, W. J., and Russell, B. J. (2017). Autonomous Coral Reef Survey in Support of Remote Sensing. Front. Mar. Sci. 4, 325. doi:10.3389/fmars.2017.00325
Adams, M. S., Scarpace, F. L., Scherz, J. P., and Woelkerling, W. J. (1977). Assessment Of Aquatic Environments By Remote Sensing. IES Report 84. Madison, WI: University of Wisconsin-Madison.
Adão, T., Hruška, J., Pádua, L., Bessa, J., Peres, E., Morais, R., et al. (2017). Hyperspectral Imaging: A Review on UAV-Based Sensors, Data Processing and Applications for Agriculture and Forestry. Remote Sensing 9, 1110. doi:10.3390/rs9111110
Amin, R., and Shulman, I. (2015). Hourly Turbidity Monitoring Using Geostationary Ocean Color Imager Fluorescence Bands. J. Appl. Remote Sens 9, 096024. doi:10.1117/1.JRS.9.096024
Anderson, F. E., Bergamaschi, B., Sturtevant, C., Knox, S., Hastings, L., Windham‐Myers, L., et al. (2016). Variation of Energy and Carbon Fluxes from a Restored Temperate Freshwater Wetland and Implications for Carbon Market Verification Protocols. J. Geophys. Res. Biogeosci. 121, 777–795. doi:10.1002/2015JG003083
Astoreca, R., Rousseau, V., Ruddick, K., Knechciak, C., Van Mol, B., Parent, J.-Y., et al. (2008). Development and Application of an Algorithm for Detecting Phaeocystis Globosa Blooms in the Case 2 Southern North Sea Waters. J. plankton Res. 31, 287–300. doi:10.1093/plankt/fbn116
Aurin, D. A., Dierssen, H. M., Twardowski, M. S., and Roesler, C. S. (2010). Optical Complexity in Long Island Sound and Implications for Coastal Ocean Color Remote Sensing. J. Geophys. Res. 115, 1–11. doi:10.1029/2009JC005837
Bagniewski, W., Fennel, K., Perry, M. J., and D'Asaro, E. A. (2011). Optimizing Models of the North Atlantic Spring Bloom Using Physical, Chemical and Bio-Optical Observations from a Lagrangian Float. Biogeosciences 8, 1291–1307. doi:10.5194/bg-8-1291-2011
Banks, A. C., Vendt, R., Alikas, K., Bialek, A., Kuusk, J., Lerebourg, C., et al. (2020). Fiducial Reference Measurements for Satellite Ocean Colour (FRM4SOC). Remote Sensing 12, 1322. doi:10.3390/rs12081322
Becker, R. H., Sayers, M., Dehm, D., Shuchman, R., Quintero, K., Bosse, K., et al. (2019). Unmanned Aerial System Based Spectroradiometer for Monitoring Harmful Algal Blooms: A New Paradigm in Water Quality Monitoring. J. Great Lakes Res. 45, 444–453. doi:10.1016/j.jglr.2019.03.006
Behrenfeld, M. J., Westberry, T. K., Boss, E. S., O'Malley, R. T., Siegel, D. A., Wiggert, J. D., et al. (2009). Satellite-detected Fluorescence Reveals Global Physiology of Ocean Phytoplankton. Biogeosciences 6, 779–794. doi:10.5194/bg-6-779-2009
Bender, H. A., Mouroulis, P., Dierssen, H. M., Painter, T. H., Thompson, D. R., Smith, C. D., et al. (2018). Snow and Water Imaging Spectrometer: Mission and Instrument Concepts for Earth-Orbiting CubeSats. 12, 044001, 12–21. doi:10.1117/1.jrs.12.044001
Bidigare, R. R., Morrow, J. H., and Kiefer, D. A. (1989). Derivative Analysis of Spectral Absorption by Photosynthetic Pigments in the Western Sargasso Sea. J. Mar. Res. 47, 323–341. doi:10.1357/002224089785076325
Blaschke, T. (2010). Object Based Image Analysis for Remote Sensing. ISPRS J. Photogrammetry Remote Sensing 65, 2–16. doi:10.1016/j.isprsjprs.2009.06.004
Blondeau-Patissier, D., Gower, J. F. R., Dekker, A. G., Phinn, S. R., and Brando, V. E. (2014). A Review of Ocean Color Remote Sensing Methods and Statistical Techniques for the Detection, Mapping and Analysis of Phytoplankton Blooms in Coastal and Open Oceans. Prog. oceanography 123, 123–144. doi:10.1016/j.pocean.2013.12.008
Bolch, E. A., Hestir, E. L., and Khanna, S. (2021). Performance and Feasibility of Drone-Mounted Imaging Spectroscopy for Invasive Aquatic Vegetation Detection. in Remote Sens. 13, 582. doi:10.3390/rs13040582
Bolch, E. A., Santos, M. J., Ade, C., Khanna, S., Basinger, N. T., Reader, M. O., et al. (2020). “Remote Detection of Invasive Alien Species,” in Remote Sensing Of Plant Biodiversity (Cham, Switzerland: Springer), 267–307. doi:10.1007/978-3-030-33157-3_12
Bongiorno, D. L., Bryson, M., Bridge, T. C. L., Dansereau, D. G., and Williams, S. B. (2018). Coregistered Hyperspectral and Stereo Image Seafloor Mapping from an Autonomous Underwater Vehicle. J. Field Robotics 35, 312–329. doi:10.1002/rob.21713
Boucher, Y., Poutier, L., Achard, V., Lenot, X., and Miesch, C. (2002). “Validation and Robustness of an Atmospheric Correction Algorithm for Hyperspectral Images,” in Algorithms and Technologies for Multispectral, Hyperspectral, and Ultraspectral Imagery VIII (Washington, DC: International Society for Optics and Photonics), 459–470.
Bracher, A., Bouman, H. A., Brewin, R. J. W., Bricaud, A., Brotas, V., Ciotti, A. M., et al. (2017a). Obtaining Phytoplankton Diversity from Ocean Color: A Scientific Roadmap for Future Development. Front. Mar. Sci. 4, 55. doi:10.3389/fmars.2017.00055
Bracher, A., Dinter, T., Wolanin, A., Rozanov, V. V., Losa, S. N., Soppa, M. A., et al. (2017b). “Global Monthly Mean Chlorophyll a Surface Concentrations from August 2002 to April 2012 for Diatoms, Coccolithophores and Cyanobacteria from PhytoDOAS Algorithm Version 3.3 Applied to SCIAMACHY Data, Link to NetCDF Files in ZIP Archive,” PANGEA. doi:10.1594/PANGAEA.870486
Bracher, A., Vountas, M., Dinter, T., Burrows, J. P., Röttgers, R., and Peeken, I. (2009). Quantitative Observation of Cyanobacteria and Diatoms from Space Using PhytoDOAS on SCIAMACHY Data. Biogeosciences 6, 751–764. doi:10.5194/bg-6-751-2009
Bracher, A., Xi, H., Dinter, T., Mangin, A., Strass, V., Von Appen, W.-J., et al. (2020a). High Resolution Water Column Phytoplankton Composition across the Atlantic Ocean from Ship-Towed Vertical Undulating Radiometry. Front. Mar. Sci. 7, 235. doi:10.3389/fmars.2020.00235
Bracher, A., Xi, H., Dinter, T., and Wiegmann, S. (2020b). Phytoplankton Pigment Concentration and Phytoplankton Groups Measured on Water Samples and from Radiometric Measurements Obtained during POLARSTERN Cruise PS113 in the Atlantic Ocean. doi:10.1594/PANGAEA.913536
Brando, V. E., Anstee, J. M., Wettle, M., Dekker, A. G., Phinn, S. R., and Roelfsema, C. (2009). A Physics Based Retrieval and Quality Assessment of Bathymetry from Suboptimal Hyperspectral Data. Remote Sensing Environ. 113, 755–770. doi:10.1016/j.rse.2008.12.003
Bulgarelli, B., and Zibordi, G. (2018). On the Detectability of Adjacency Effects in Ocean Color Remote Sensing of Mid-latitude Coastal Environments by SeaWiFS, MODIS-A, MERIS, OLCI, OLI and MSI. Remote sensing Environ. 209, 423–438. doi:10.1016/j.rse.2017.12.021
Cael, B. B., Chase, A., and Boss, E. (2020). Information Content of Absorption Spectra and Implications for Ocean Color Inversion. Appl. Opt. 59, 3971–3984. doi:10.1364/ao.389189
Carder, K. L., Reinersman, P., Chen, R. F., Muller-Karger, F., Davis, C. O., and Hamilton, M. (1993). AVIRIS Calibration and Application in Coastal Oceanic Environments. Remote Sensing Environ. 44, 205–216. doi:10.1016/0034-4257(93)90016-q
Casey, K. A., Rousseaux, C. S., Gregg, W. W., Boss, E., Chase, A. P., Craig, S. E., et al. (2020). A Global Compilation of In Situ Aquatic High Spectral Resolution Inherent and Apparent Optical Property Data for Remote Sensing Applications. Earth Syst. Sci. Data 12, 1123–1139. doi:10.5194/essd-12-1123-2020
Castagna, A., Simis, S., Dierssen, H., Vanhellemont, Q., Sabbe, K., and Vyverman, W. (2020). Extending Landsat 8: Retrieval of an Orange Contra-band for Inland Water Quality Applications. Remote Sensing 12, 637. doi:10.3390/rs12040637
Catlett, D., and Siegel, D. A. (2018). Phytoplankton Pigment Communities Can Be Modeled Using Unique Relationships with Spectral Absorption Signatures in a Dynamic Coastal Environment. J. Geophys. Res. Oceans 123, 246–264. doi:10.1002/2017jc013195
Chang, G., Mahoney, K., Briggs-Whitmire, A., Kohler, D., Mobley, C., Lewis, M., et al. (2004). The New Age of Hyperspectral Oceanography. oceanog 17, 16–23. doi:10.5670/oceanog.2004.43
Chen, J., Cui, T., Ishizaka, J., and Lin, C. (2014). A Neural Network Model for Remote Sensing of Diffuse Attenuation Coefficient in Global Oceanic and Coastal Waters: Exemplifying the Applicability of the Model to the Coastal Regions in Eastern China Seas. Remote Sensing Environ. 148, 168–177. doi:10.1016/j.rse.2014.02.019
Cheng, C., Wei, Y., Sun, X., and Zhou, Y. (2013). Estimation of Chlorophyll-A Concentration in Turbid Lake Using Spectral Smoothing and Derivative Analysis. Ijerph 10, 2979–2994. doi:10.3390/ijerph10072979
Chennu, A., Färber, P., De’ath, G., de Beer, D., and Fabricius, K. E. (2017). A Diver-Operated Hyperspectral Imaging and Topographic Surveying System for Automated Mapping of Benthic Habitats. Sci. Rep. 7, 7122. doi:10.1038/s41598-017-07337-y
Chirayath, V., and Li, A. (2019). Next-Generation Optical Sensing Technologies for Exploring Ocean Worlds-NASA FluidCam, MiDAR, and NeMO-Net. Front. Mar. Sci. 6. doi:10.3389/fmars.2019.00521
Chomko, R. M., Gordon, H. R., Maritorena, S., and Siegel, D. A. (2003). Simultaneous Retrieval of Oceanic and Atmospheric Parameters for Ocean Color Imagery by Spectral Optimization: a Validation. Remote Sensing Environ. 84, 208–220. doi:10.1016/S0034-4257(02)00108-6
Chowdhary, J., Zhai, P., Boss, E., Dierssen, H. M., Frouin, R. J., Ibrahim, A. I., et al. (2019). Modeling Atmosphere-Ocean Radiative Transfer: A PACE Mission Perspective. Front. Earth Sci. 7, 100. doi:10.3389/feart.2019.00100
Clarke, G. L., Ewing, G. C., and Lorenzen, C. J. (1970). Spectra of Backscattered Light from the Sea Obtained from Aircraft as a Measure of Chlorophyll Concentration. Science 167, 1119–1121. doi:10.1126/science.167.3921.1119
Clementson, L. A., and Wojtasiewicz, B. (2019a). Dataset on the Absorption Characteristics of Extracted Phytoplankton Pigments. Data in Brief 24, 103875. doi:10.1016/j.dib.2019.103875
Clementson, L. A., and Wojtasiewicz, B. (2019b). Dataset on the In Vivo Absorption Characteristics and Pigment Composition of Various Phytoplankton Species. Data in Brief 25, 104020. doi:10.1016/j.dib.2019.104020
Cooley, T., Anderson, G. P., Felde, G. W., Hoke, M. L., Ratkowski, A. J., Chetwynd, J. H., et al. (2002). FLAASH, a MODTRAN4-based atmospheric correction algorithm, its application and validation. IEEE Intern. Geosci. Remote Sensing Sympo. 3, 1414–1418. doi:10.1109/IGARSS.2002.1026134
Colefax, A. P., Butcher, P. A., and Kelaher, B. P. (2018). The Potential for Unmanned Aerial Vehicles (UAVs) to Conduct Marine Fauna Surveys in Place of Manned Aircraft. ICES J. Mar. Sci. 75, 1–8. doi:10.1093/icesjms/fsx100
Collins, M., and Pattiaratchi, C. (1984). Identification of Suspended Sediment in Coastal Waters Using Airborne Thematic Mapper Data. Int. J. Remote Sensing 5, 635–657. doi:10.1080/01431168408948848
Colomina, I., and Molina, P. (2014). Unmanned Aerial Systems for Photogrammetry and Remote Sensing: A Review. ISPRS J. photogrammetry remote sensing 92, 79–97. doi:10.1016/j.isprsjprs.2014.02.013
Commonwealth of Australia (2019). Current and Future Value of Earth and Marine Observing to the Asia-Pacific Region. G. A. P. E. Corporation. Available at: https://www.industry.gov.au/sites/default/files/2019-11/current-and-future-value-of-earth-and-marine-observing-to-asia-pacific-region.pdf.A.
Cornet, V. J., and Joyce, K. E.. (in review) Assessing the Potential of Remotely-Sensed Drone Spectroscopy to Determine Live Coral Cover on Heron Reef. Drones.
Crabbé, A. H., Somers, B., Roberts, D. A., Halligan, K., Dennison, P., and Dudley, K. (2020). MESMA QGIS Plugin.
Craig, S. E., Lee, Z., and Du, K. (2020). Top of Atmosphere, Hyperspectral Synthetic Dataset for PACE (Phytoplankton, Aerosol, and Ocean Ecosystem) Ocean Color Algorithm Development. Washington, DC: National Aeronautics and Space Administration. doi:10.1594/PANGAEA.915747
Craig, S. E., Lohrenz, S. E., Lee, Z., Mahoney, K. L., Kirkpatrick, G. J., Schofield, O. M., et al. (2006). Use of Hyperspectral Remote Sensing Reflectance for Detection and Assessment of the Harmful Alga, Karenia Brevis. Appl. Opt. 45, 5414–5425. doi:10.1364/AO.45.005414
Cummings, M. E., and Endler, J. A. (2018). 25 Years of Sensory Drive: the Evidence and its Watery Bias. Curr. Zool 64, 471–484. doi:10.1093/cz/zoy043
Cutter, M. A., Johns, L. S., Lobb, D. R., Williams, T. L., and Settle, J. J. (2004). “Flight Experience of the Compact High-Resolution Imaging Spectrometer (CHRIS),” in Imaging Spectrometry IX (Washington, DC: International Society for Optics and Photonics), 392–405.
Davis, C., Bowles, J., Leathers, R., Korwan, D., Downes, T. V., Snyder, W., et al. (2002). Ocean PHILLS Hyperspectral Imager: Design, Characterization, and Calibration. Opt. Express 10, 210–221. doi:10.1364/oe.10.000210
Davis, C. O. (2001). Airborne Hyperspectral Remote Sensing. Washington, DC: Naval Research Lab. Available at: https://apps.dtic.mil/sti/citations/ADA625021 (Accessed November 10, 2020) doi:10.21236/ada625021
De Keukelaere, L., Sterckx, S., Adriaensen, S., Knaeps, E., Reusen, I., Giardino, C., et al. (2018). Atmospheric Correction of Landsat-8/OLI and Sentinel-2/MSI Data Using iCOR Algorithm: Validation for Coastal and Inland Waters. Eur. J. Remote Sensing 51, 525–542. doi:10.1080/22797254.2018.1457937
Dekker, A., (2018). Feasibility Study for an Aquatic Ecosystem Earth Observing System. Available at: www.ceos.org (Committee on Earth Observation Satellites (CEOS).
Dekker, A. G., Brando, V. E., Anstee, J., Pinnel, N., and Held, A. (2001). “Preliminary Assessment of the Performance of Hyperion in Coastal Waters. Cal/Val Activities in Moreton Bay, Queensland, Australia,” in IGARSS 2001. Scanning the Present and Resolving the Future. Proceedings. IEEE 2001 International Geoscience and Remote Sensing Symposium (Cat. No. 01CH37217) (New York, NY: IEEE), 2665–2667.
Dekker, A. G., and Donze, M. (1994). “Imaging Spectrometry as a Research Tool for Inland Water Resources Analysis,” in Imaging Spectrometry—a Tool for Environmental Observations (Springer), 295–317.
Dickey, T. D., and Chang, G. C. (2002). Recent Advances and Future Visions: Temporal Variability of Optical and Bio-Optical Properties of the Ocean, 14. Washington, Dc: Oceanography Society, 15–29.
Dierssen, H., Bracher, A., Brando, V., Loisel, H., and Ruddick, K. (2020). Data Needs for Hyperspectral Detection of Algal Diversity across the Globe. Oceanog 33, 74–79. doi:10.5670/oceanog.2020.111
Dierssen, H. M., Bostrom, K. J., Chlus, A., Hammerstrom, K., Thompson, D. R., and Lee, Z. (2019). Pushing the Limits of Seagrass Remote Sensing in the Turbid Waters of Elkhorn Slough, California. Remote Sensing 11, 1664. doi:10.3390/rs11141664
Dierssen, H. M., Chlus, A., and Russell, B. (2015). Hyperspectral Discrimination of Floating Mats of Seagrass Wrack and the Macroalgae Sargassum in Coastal Waters of Greater Florida Bay Using Airborne Remote Sensing. Remote Sensing Environ. 167, 247–258. doi:10.1016/j.rse.2015.01.027
Dierssen, H., McManus, G. B., Chlus, A., Qiu, D., Gao, B.-C., and Lin, S. (2015b). Space Station Image Captures a Red Tide Ciliate Bloom at High Spectral and Spatial Resolution. Proc. Natl. Acad. Sci. USA 112, 14783–14787. doi:10.1073/pnas.1512538112
Dierssen, H. M. (2019). Hyperspectral Measurements, Parameterizations, and Atmospheric Correction of Whitecaps and Foam from Visible to Shortwave Infrared for Ocean Color Remote Sensing. Front. Earth Sci. 7, 14. doi:10.3389/feart.2019.00014
Dierssen, H. M., Kudela, R. M., Ryan, J. P., and Zimmerman, R. C. (2006). Red and Black Tides: Quantitative Analysis of Water-Leaving Radiance and Perceived Color for Phytoplankton, Colored Dissolved Organic Matter, and Suspended Sediments. Limnol. Oceanogr. 51, 2646–2659. doi:10.4319/lo.2006.51.6.2646
Dierssen, H. M. (2010). Perspectives on Empirical Approaches for Ocean Color Remote Sensing of Chlorophyll in a Changing Climate. Proc. Natl. Acad. Sci. 107, 17073–17078. doi:10.1073/pnas.0913800107
Doerffer, R., and Helmut, S. (2000). “Neural Network for Retrieval of Concentrations of Water Constituents with the Possibility of Detecting Exceptional Out of Scope Spectra,” in IGARSS 2000. IEEE 2000 International Geoscience and Remote Sensing Symposium. Taking the Pulse of the Planet: The Role of Remote Sensing in Managing the Environment. Proceedings (Cat. No.00CH37120), Berlin, Germany: Springer. 2, 714–717. doi:10.1109/IGARSS.2000.861680
Doubleday, J., Chien, S., Norton, C., Wagstaff, K., Thompson, D. R., Bellardo, J., et al. (2015). “Autonomy for Remote Sensing—Experiences from the IPEX CubeSat,” in Proceedings of the 2015 IEEE International Geoscience and Remote Sensing Symposium (IGARSS), Milan, Italy, July 26–31, 2015. 5308–5311.
Duffy, J. E., Amaral-Zettler, L. A., Fautin, D. G., Paulay, G., Rynearson, T. A., Sosik, H. M., et al. (2013). Envisioning a Marine Biodiversity Observation Network. Bioscience 63, 350–361. doi:10.1525/bio.2013.63.5.8
IOCCG (2019). “Synergy between Ocean Colour and Biogeochemical/Ecosystem Models,” in IOCCG Report 19. Editor S. Dutkiewicz, 184.
Erickson, Z. K., Frankenberg, C., Thompson, D. R., Thompson, A. F., and Gierach, M. (2019). Remote Sensing of Chlorophyll Fluorescence in the Ocean Using Imaging Spectrometry: Toward a Vertical Profile of Fluorescence. Geophys. Res. Lett. 46, 1571–1579. doi:10.1029/2018gl081273
Espel, D., Courty, S., Auda, Y., Sheeren, D., and Elger, A. (2020). Submerged Macrophyte Assessment in Rivers: An Automatic Mapping Method Using Pléiades Imagery. Water Res. 186, 116353. doi:10.1016/j.watres.2020.116353
Fichot, C. G., Downing, B. D., Bergamaschi, B. A., Windham-Myers, L., Marvin-DiPasquale, M., Thompson, D. R., et al. (2016). High-Resolution Remote Sensing of Water Quality in the San Francisco Bay-Delta Estuary. Environ. Sci. Technol. 50, 573–583. doi:10.1021/acs.est.5b03518
Filippi, A. M. (2007). Derivative-Neural Spectroscopy for Hyperspectral Bathymetric Inversion. The Prof. Geographer 59, 236–255. doi:10.1111/j.1467-9272.2007.00610.x
Freitas, F. H., and Dierssen, H. M. (2019). Evaluating the Seasonal and Decadal Performance of Red Band Difference Algorithms for Chlorophyll in an Optically Complex Estuary with Winter and Summer Blooms. Remote Sensing Environ. 231, 111228.
Frouin, R. J., Franz, B. A., Ibrahim, A., Knobelspiesse, K., Ahmad, Z., Cairns, B., et al. (2019). Atmospheric Correction of Satellite Ocean-Color Imagery during the PACE Era. Front. Earth Sci. 7, 145. doi:10.3389/feart.2019.00145
Gao, B.-C., Montes, M. J., Davis, C. O., and Goetz, A. F. H. (2009). Atmospheric Correction Algorithms for Hyperspectral Remote Sensing Data of Land and Ocean. Remote sensing Environ. 113, S17–S24. doi:10.1016/j.rse.2007.12.015
Garcia, R. A., McKinna, L. I. W., Hedley, J. D., and Fearns, P. R. C. S. (2014). Improving the Optimization Solution for a Semi-analytical Shallow Water Inversion Model in the Presence of Spectrally Correlated Noise. Limnol. Oceanogr. 12, 651–669. doi:10.4319/lom.2014.12.651
Garcia, R., Lee, Z., and Hochberg, E. (2018). Hyperspectral Shallow-Water Remote Sensing with an Enhanced Benthic Classifier. Remote Sensing 10, 147. doi:10.3390/rs10010147
Gege, P., and Dekker, A. G. (2020). Spectral and Radiometric Measurement Requirements for Inland, Coastal and Reef Waters. Remote Sensing 12, 2247. doi:10.3390/rs12142247
Gege, P. (2017). “Radiative Transfer Theory for Inland Waters,” in Bio-optical Modeling And Remote Sensing Of Inland Waters (Amsterdam, Netherlands: Elsevier), 25–67. doi:10.1016/b978-0-12-804644-9.00002-1
Gege, P. (2015). “WASI-2D: A Software for the Analysis of Remote Sensing Images from Deep and Shallow Waters,” in EOS Topical Meeting on Blue Photonics, Barcelona, Spain, May 11–13, 2015.
Gholizadeh, M., Melesse, A., and Reddi, L. (2016). A Comprehensive Review on Water Quality Parameters Estimation Using Remote Sensing Techniques. Sensors 16, 1298. doi:10.3390/s16081298
Giardino, C., Brando, V. E., Gege, P., Pinnel, N., Hochberg, E., Knaeps, E., et al. (2019). Imaging Spectrometry of Inland and Coastal Waters: State of the Art, Achievements and Perspectives. Surv. Geophys. 40, 401–429. doi:10.1007/s10712-018-9476-0
Giardino, C., Bresciani, M., Braga, F., Fabbretto, A., Ghirardi, N., Pepe, M., et al. (2020). First Evaluation of PRISMA Level 1 Data for Water Applications. Sensors 20, 4553. doi:10.3390/s20164553
Gilerson, A., Zhou, J., Hlaing, S., Ioannou, I., Schalles, J., Gross, B., et al. (2007). Fluorescence Component in the Reflectance Spectra from Coastal Waters. Dependence on Water Composition. Opt. Express 15, 15702–15721. doi:10.1364/oe.15.015702
Gislason, P. O., Benediktsson, J. A., and Sveinsson, J. R. (2006). Random Forests for Land Cover Classification. Pattern Recognition Lett. 27, 294–300. doi:10.1016/j.patrec.2005.08.011
Goldman, C. R., Richards, R. C., Paerl, H. W., Wrigley, R. C., Oberbeck, V. R., and Quaide, W. L. (1974). Limnological Studies and Remote Sensing of the Upper Truckee River Sediment Plume in Lake Tahoe, California-Nevada. Remote Sensing Environ. 3, 49–67. doi:10.1016/0034-4257(74)90038-8
Gordon, H. R., and Brown, O. B. (1988). A Semianalytic Radiance Model of Ocean Color. J. Geophys. Res. 93, 10909–10924. doi:10.1029/jd093id09p10909
Gordon, H. R., Brown, O. B., and Jacobs, M. M. (1975). Computed Relationships between the Inherent and Apparent Optical Properties of a Flat Homogeneous Ocean. Appl. Opt. 14, 417–427. doi:10.1364/AO.14.000417
Gordon, H. R., and McCluney, W. R. (1975). Estimation of the Depth of Sunlight Penetration in the Sea for Remote Sensing. Appl. Opt. 14, 413–416. doi:10.1364/AO.14.000413
Gordon, H. R., and Morel, A. Y. (1983). Remote Assessment of Ocean Color for Interpretation of Satellite Visible Imagery: A Review. New York, NY: Springer-Verlag.
Gower, J., King, S., Borstad, G., and Brown, L. (2008). The Importance of a Band at 709 Nm for Interpreting Water-Leaving Spectral Radiance. Can. J. Remote Sensing 34, 287–295.
Greb, S., Dekker, A., and Binding, C. (2018). Earth Observations in Support of Global Water Quality Monitoring. IOCCG Report Series, No. 17. Dartmouth, Canada: International Ocean Colour Coordinating Group.
Gregg, W. W., and Rousseaux, C. S. (2017). Simulating PACE Global Ocean Radiances. Front. Mar. Sci. 4. doi:10.3389/fmars.2017.00060
Guanter, L., Ruiz-Verdú, A., Odermatt, D., Giardino, C., Simis, S., Estellés, V., et al. (2010). Atmospheric Correction of ENVISAT/MERIS Data over Inland Waters: Validation for European Lakes. Remote Sensing Environ. 114, 467–480. doi:10.1016/j.rse.2009.10.004
Harmel, T. (2016). “Recent Developments in the Use of Light Polarization for Marine Environment Monitoring from Space,” in Light Scattering Reviews 10: Light Scattering And Radiative Transfer Springer Praxis Books. Editor A. A. Kokhanovsky (Berlin, Heidelberg: Springer), 41–84. doi:10.1007/978-3-662-46762-6_2
Haut, J. M., Gallardo, J. A., Paoletti, M. E., Cavallaro, G., Plaza, J., Plaza, A., et al. (2019). Cloud Deep Networks for Hyperspectral Image Analysis. IEEE Trans. Geosci. Remote Sensing 57, 9832–9848. doi:10.1109/TGRS.2019.2929731
Hedley, J. D., Russell, B. J., Randolph, K., Pérez-Castro, M. Á., Vásquez-Elizondo, R. M., Enríquez, S., et al. (2017). Remote Sensing of Seagrass Leaf Area Index and Species: The Capability of a Model Inversion Method Assessed by Sensitivity Analysis and Hyperspectral Data of Florida Bay. Front. Mar. Sci. 4, 362. doi:10.3389/fmars.2017.00362
Hedley, J., Mirhakak, M., Wentworth, A., and Dierssen, H. (2018). Influence of Three-Dimensional Coral Structures on Hyperspectral Benthic Reflectance and Water-Leaving Reflectance. Appl. Sci. 8, 2688. doi:10.3390/app8122688
Hedley, J., Roelfsema, C., Chollett, I., Harborne, A., Heron, S., Weeks, S., et al. (2016a). Remote Sensing of Coral Reefs for Monitoring and Management: A Review. Remote Sensing 8, 118. doi:10.3390/rs8020118
Hedley, J., Russell, B., Randolph, K., and Dierssen, H. (2016b). A Physics-Based Method for the Remote Sensing of Seagrasses. Remote Sensing Environ. 174, 134–147. doi:10.1016/j.rse.2015.12.001
Hestir, E. L., Brando, V. E., Bresciani, M., Giardino, C., Matta, E., Villa, P., et al. (2015). Measuring Freshwater Aquatic Ecosystems: The Need for a Hyperspectral Global Mapping Satellite Mission. Remote Sensing Environ. 167, 181–195. doi:10.1016/j.rse.2015.05.023
Heupel, E. E., Dierssen, H. M., Gao, B., Green, R. O., and Mouroulis, P. (2013). Hyperspectral Remote Sensing in Coastal Regions: Prism Field Validation in Elkhorn Slough. in Proceedings of the 2013 5th Workshop on Hyperspectral Image and Signal Processing: Evolution in Remote Sensing, Gainesville, FL, June 26–28, 2013. 1–4.
Hieronymi, M., Müller, D., and Doerffer, R. (2017). The OLCI Neural Network Swarm (ONNS): A Bio-Geo-Optical Algorithm for Open Ocean and Coastal Waters. Front. Mar. Sci. 4. doi:10.3389/fmars.2017.00140
Hill, V. J., Zimmerman, R. C., Bissett, W. P., Dierssen, H., and Kohler, D. D. (2014). Evaluating Light Availability, Seagrass Biomass, and Productivity Using Hyperspectral Airborne Remote Sensing in Saint Joseph’s Bay, Florida. Estuaries and Coasts, 1–23.
Hochberg, E. J., and Atkinson, M. J. (2000). Spectral Discrimination of Coral Reef Benthic Communities. Coral Reefs 19, 164–171. doi:10.1007/s003380000087
Hovis, W. A., Clark, D. K., Anderson, F., Austin, R. W., Wilson, W. H., Baker, E. T., et al. (1980). Nimbus-7 Coastal Zone Color Scanner: System Description and Initial Imagery. Science 210, 60–63. doi:10.1126/science.210.4465.60
Hu, C., and Feng, L. (2016). Modified MODIS Fluorescence Line Height Data Product to Improve Image Interpretation for Red Tide Monitoring in the Eastern Gulf of Mexico. J. Appl. Remote Sensing 11. doi:10.1117/1.jrs.11.012003
Ibrahim, A., Franz, B., Ahmad, Z., Healy, R., Knobelspiesse, K., Gao, B.-C., et al. (2018). Atmospheric Correction for Hyperspectral Ocean Color Retrieval with Application to the Hyperspectral Imager for the Coastal Ocean (HICO). Remote Sensing Environ. 204, 60–75. doi:10.1016/j.rse.2017.10.041
Ioannou, I., Gilerson, A., Gross, B., Moshary, F., and Ahmed, S. (2013). Deriving Ocean Color Products Using Neural Networks. Remote Sensing Environ. 134, 78–91. doi:10.1016/j.rse.2013.02.015
Jamet, C., Loisel, H., and Dessailly, D. (2012). Retrieval of the Spectral Diffuse Attenuation Coefficient Kd (λ) in Open and Coastal Ocean Waters Using a Neural Network Inversion. J. Geophys. Res. Oceans 117. doi:10.1029/2012jc008076
Jamet, C., Thiria, S., Moulin, C., and Crépon, M. (2005). Use of a Neurovariational Inversion for Retrieving Oceanic and Atmospheric Constituents from Ocean Color Imagery: A Feasibility Study. J. Atmos. Oceanic Tech. 22, 460–475. doi:10.1175/jtech1688.1
Janssen, M., Charalabidis, Y., and Zuiderwijk, A. (2012). Benefits, Adoption Barriers and Myths of Open Data and Open Government. Inf. Syst. Manage. 29, 258–268. doi:10.1080/10580530.2012.716740
Jerlov, N. G. (1951). Optical Measurement of Particle Distribution in the Sea. Tellus 3, 122–128. doi:10.3402/tellusa.v3i3.8637
Jeziorska, J. (2019). UAS for Wetland Mapping and Hydrological Modeling. Remote Sensing 11, 1997. doi:10.3390/rs11171997
Johnsen, G., Volent, Z., Dierssen, H. M., Pettersen, R., Van Aredelan, M., Søreide, F., et al. (2013). “Underwater Hyperspectral Imagery to Create Biogeochemical Maps of Seafloor Properties,” In Subsea Optics And Imaging (Cambridge, United Kingdom: Woodhead Publishing).
Joyce, K. E., Duce, S., Leahy, S. M., Leon, J., and Maier, S. W. (2019). Principles and Practice of Acquiring Drone-Based Image Data in Marine Environments. Mar. Freshw. Res. 70, 952–963. doi:10.1071/mf17380
Kalinauskaitė, D. (2017). To Be Findable, Accessible, Interoperable and Reusable: Language Data and Technology Infrastructure for Supporting the FAIR Data Approach. Available at: https://www.vdu.lt/cris/handle/20.500.12259/35989 (Accessed November 10, 2020).
Kavanaugh, M. T., Oliver, M. J., Chavez, F. P., Letelier, R. M., Muller-Karger, F. E., and Doney, S. C. (2016). Seascapes as a New Vernacular for Pelagic Ocean Monitoring, Management and Conservation. ICES J. Mar. Sci. 73, 1839–1850. doi:10.1093/icesjms/fsw086
Khan, M. J., Khan, H. S., Yousaf, A., Khurshid, K., and Abbas, A. (2018). Modern Trends in Hyperspectral Image Analysis: a Review. IEEE Access 6, 14118–14129. doi:10.1109/access.2018.2812999
Klemas, V. V. (2015). Coastal and Environmental Remote Sensing from Unmanned Aerial Vehicles: An Overview. J. coastal Res. 315, 1260–1267. doi:10.2112/jcoastres-d-15-00005.1
Knaeps, E., Doxaran, D., Dogliotti, A., Nechad, B., Ruddick, K., Raymaekers, D., et al. (2018). The SeaSWIR Dataset. Earth Syst. Sci. Data 10, 1439–1449. doi:10.5194/essd-10-1439-2018
Krämer, M., and Senner, I. (2015). A Modular Software Architecture for Processing of Big Geospatial Data in the Cloud. Comput. Graphics 49, 69–81. doi:10.1016/j.cag.2015.02.005
Kramer, S. J., and Siegel, D. A. (2019). How Can Phytoplankton Pigments Be Best Used to Characterize Surface Ocean Phytoplankton Groups for Ocean Color Remote Sensing Algorithms?. J. Geophys. Res. Oceans 124, 7557–7574. doi:10.1029/2019jc015604
Kuchinke, C. P., Gordon, H. R., and Franz, B. A. (2009). Spectral Optimization for Constituent Retrieval in Case 2 Waters I: Implementation and Performance. Remote Sensing Environ. 113, 571–587. doi:10.1016/j.rse.2008.11.001
Kutser, T., Hedley, J., Giardino, C., Roelfsema, C., and Brando, V. E. (2020). Remote Sensing of Shallow Waters - A 50 Year Retrospective and Future Directions. Remote Sensing Environ. 240, 111619. doi:10.1016/j.rse.2019.111619
Lavender, S. (2014). Multi-sensor Ocean Colour Atmospheric Correction for Time-Series Data: Application to LANDSAT ETM+ and OLI Data. EARSeL eProceedings 13, 58–66.
Lavender, S. J., and Nagur, C. R. C. (2002). Mapping Coastal Waters with High Resolution Imagery: Atmospheric Correction of Multi-Height Airborne Imagery. J. Opt. A: Pure Appl. Opt. 4, S50–S55. doi:10.1088/1464-4258/4/4/363
Lee, Z., Carder, K., Arnone, R., and He, M. (2007). Determination of Primary Spectral Bands for Remote Sensing of Aquatic Environments. Sensors 7, 3428–3441. doi:10.3390/s7123428
Lee, Z., Carder, K. L., and Arnone, R. A. (2002). Deriving Inherent Optical Properties from Water Color: a Multiband Quasi-Analytical Algorithm for Optically Deep Waters. Appl. Opt. 41, 5755–5772. doi:10.1364/ao.41.005755
Liu, S., Gao, Y., Zheng, W., and Li, X. (2015). Performance of Two Neural Network Models in Bathymetry. Remote sensing Lett. 6, 321–330. doi:10.1080/2150704x.2015.1034885
Liu, Y.-N., Zhang, J., Zhang, Y., Sun, W.-W., Jiao, L.-L., Sun, D.-X., et al. (2019). The Advanced Hyperspectral Imager: Aboard China's GaoFen-5 Satellite. IEEE Geosci. Remote Sens. Mag. 7, 23–32. doi:10.1109/mgrs.2019.2927687
Lodhi, V., Chakravarty, D., and Mitra, P. (2018). Hyperspectral Imaging for Earth Observation: Platforms and Instruments. J. Indian Inst. Sci. 98, 429–443. doi:10.1007/s41745-018-0070-8
Louchard, E. M., Reid, R. P., Stephens, F. C., Davis, C. O., Leathers, R. A., and T. Valerie, D. (2003). Optical Remote Sensing of Benthic Habitats and Bathymetry in Coastal Environments at Lee Stocking Island, Bahamas: A Comparative Spectral Classification Approach. Limnol. Oceanogr. 48, 511–521. doi:10.4319/lo.2003.48.1_part_2.0511
Lubac, B., Loisel, H., Guiselin, N., Astoreca, R., Felipe Artigas, L., and Mériaux, X. (2008). Hyperspectral and Multispectral Ocean Color Inversions to Detect Phaeocystis Globosa Blooms in Coastal Waters. J. Geophys. Res. Oceans 113Available at: http://onlinelibrary.wiley.com/doi/10.1029/2007JC004451/full (Accessed July 10, 2017). doi:10.1029/2007jc004451
Lubac, B., and Loisel, H. (2007). Variability and Classification of Remote Sensing Reflectance Spectra in the Eastern English Channel and Southern North Sea. Remote Sensing Environ. 110, 45–58. doi:10.1016/j.rse.2007.02.012
Lyzenga, D. R. (1978). Passive Remote Sensing Techniques for Mapping Water Depth and Bottom Features. Appl. Opt. 17, 379–383. doi:10.1364/ao.17.000379
Malone, T. C., DiGiacomo, P. M., Gonçalves, E., Knap, A. H., Talaue-McManus, L., and de Mora, S. (2014). A Global Ocean Observing System Framework for Sustainable Development. Mar. Pol. 43, 262–272. doi:10.1016/j.marpol.2013.06.008
Manfreda, S., McCabe, M., Miller, P., Lucas, R., Pajuelo Madrigal, V., Mallinis, G., et al. (2018). On the Use of Unmanned Aerial Systems for Environmental Monitoring. Remote sensing 10, 641. doi:10.3390/rs10040641
Matthews, M. W., Bernard, S., and Robertson, L. (2012). An Algorithm for Detecting Trophic Status (Chlorophyll-a), Cyanobacterial-Dominance, Surface Scums and Floating Vegetation in Inland and Coastal Waters. Remote Sensing Environ. 124, 637–652. doi:10.1016/j.rse.2012.05.032
Mazel, C. H. (2002). Coastal Benthic Optical Properties (CoBOP): Optical Properties of Benthic Marine Organisms and Substrates. Andover, MA: Physical Sciences Inc..
McClain, C. R. (2009). A Decade of Satellite Ocean Color Observations. Annu. Rev. Mar. Sci. 1, 19–42. doi:10.1146/annurev.marine.010908.163650
McKinna, L. I. W., Cetinić, I., Chase, A. P., and Werdell, P. J. (2019). Approach for Propagating Radiometric Data Uncertainties through NASA Ocean Color Algorithms. Front. Earth Sci. 7. doi:10.3389/feart.2019.00176
Mishra, D. R., Ogashawara, I., and Gitelson, A. A. (2017). Bio-optical Modeling and Remote Sensing of Inland Waters. Amsterdam, Netherlands: Elsevier.
Mobley, C. D. (1994). Light and Water: Radiative Transfer in Natural Waters. San Diego, CA: Academic Press.
Mobley, C. D., Sundman, L. K., Davis, C. O., Bowles, J. H., Downes, T. V., Leathers, R. A., et al. (2005). Interpretation of Hyperspectral Remote-Sensing Imagery by Spectrum Matching and Look-Up Tables. Appl. Opt. 44, 3576–3592. doi:10.1364/ao.44.003576
Mobley, C. D., Werdell, J., Franz, B., Ahmad, Z., and Bailey, S. (2016). Atmospheric Correction for Satellite Ocean Color Radiometry.
Mogstad, A. A., and Johnsen, G. (2017). Spectral Characteristics of Coralline Algae: a Multi-Instrumental Approach, with Emphasis on Underwater Hyperspectral Imaging. Appl. Opt. 56, 9957–9975. doi:10.1364/AO.56.009957
Moline, M. A., Blackwell, S. M., von Alt, C., Allen, B., Austin, T., Case, J., et al. (2005). Remote Environmental Monitoring Units: An Autonomous Vehicle for Characterizing Coastal Environments. J. Atmos. Oceanic Tech. 22, 1797–1808. doi:10.1175/JTECH1809.1
Montes, E., Djurhuus, A., Muller-Karger, F. E., Otis, D., Kelble, C. R., and Kavanaugh, M. T. (2020). Dynamic Satellite Seascapes as a Biogeographic Framework for Understanding Phytoplankton Assemblages in the Florida Keys National Marine Sanctuary, United States. Front. Mar. Sci. 7, 575. doi:10.3389/fmars.2020.00575
Moore, G. F., Aiken, J., and Lavender, S. J. (1999). The Atmospheric Correction of Water Colour and the Quantitative Retrieval of Suspended Particulate Matter in Case II Waters: Application to MERIS. Int. J. Remote Sensing 20, 1713–1733. doi:10.1080/014311699212434
Mortelmans, J., Deneudt, K., Cattrijsse, A., Beauchard, O., Daveloose, I., Vyverman, W., et al. (2019). Nutrient, Pigment, Suspended Matter and Turbidity Measurements in the Belgian Part of the North Sea. Scientific data 6, 1–8. doi:10.1038/s41597-019-0032-7
Mouw, C. B., Greb, S., Aurin, D., DiGiacomo, P. M., Lee, Z., and Twardowski, M. (2015). Aquatic color radiometry remote sensing of coastal and inland waters: Challenges and recommendations for future satellite missions. Rem. Sens. Environ. 160, 15–30. doi:10.1016/j.rse.2015.02.001
Mouroulis, P., Green, R. O., and Wilson, D. W. (2008). Optical Design of a Coastal Ocean Imaging Spectrometer. Opt. Express 16, 9087–9096. doi:10.1364/oe.16.009087
Mouroulis, P., Van Gorp, B., Green, R. O., Dierssen, H., Wilson, D. W., Eastwood, M., et al. (2014). Portable Remote Imaging Spectrometer Coastal Ocean Sensor: Design, Characteristics, and First Flight Results. Appl. Opt. 53, 1363–1380. doi:10.1364/ao.53.001363
Müller, R., Avbelj, J., Carmona, E., Eckardt, A., Gerasch, B., Graham, L., et al. (2016). The New Hyperspectral Sensor Desis on the Multi-Payload Platform Muses Installed on the Iss. Int. Arch. Photogramm. Remote Sens. Spat. Inf. Sci. XLI-B1, 461–467. doi:10.5194/isprsarchives-xli-b1-461-2016
Muller-Karger, F. E., Hestir, E., Ade, C., Turpie, K., Roberts, D. A., Siegel, D., et al. (2018). Satellite Sensor Requirements for Monitoring Essential Biodiversity Variables of Coastal Ecosystems. Ecol. Appl. 28, 749–760. doi:10.1002/eap.1682
Mumby, P., Green, E., Edwards, A., and Clark, C. (1997). Measurement of Seagrass Standing Crop Using Satellite and Digital Airborne Remote Sensing. Mar. Ecol. Prog. Ser. 159, 51–60. doi:10.3354/meps159051
Murfitt, S. L., Allan, B. M., Bellgrove, A., Rattray, A., Young, M. A., and Ierodiaconou, D. (2017). Applications of Unmanned Aerial Vehicles in Intertidal Reef Monitoring. Scientific Rep. 7, 1–11. doi:10.1038/s41598-017-10818-9
Muslim, A. M., Chong, W. S., Safuan, C. D. M., Khalil, I., and Hossain, M. S. (2019). Coral Reef Mapping of UAV: a Comparison of Sun Glint Correction Methods. Remote Sensing 11, 2422. doi:10.3390/rs11202422
Neeley, A. R., and Mannino, A.IOCCG (2018). Ocean Optics and Biogeochemistry Protocols for Satellite Ocean Colour Sensor Validation; Volume 1.0. Inherent Optical Property Measurements and Protocols: Absorption Coefficient. Int. Ocean Colour Coordinating Group (Ioccg). doi:10.25607/OBP-119
Nguyen, H., Cressie, N., and Hobbs, J. (2019). Sensitivity of Optimal Estimation Satellite Retrievals to Misspecification of the Prior Mean and Covariance, with Application to OCO-2 Retrievals. Remote Sensing 11, 2770. doi:10.3390/rs11232770
Nowak, M. M., Dziób, K., and Bogawski, P. (2018). Unmanned Aerial Vehicles (UAVs) in Environmental Biology: A Review. Eur. J. Ecol. 4, 56–74.
Omanović, D., Santinelli, C., Marcinek, S., and Gonnelli, M. (2019). ASFit - an All-Inclusive Tool for Analysis of UV–Vis Spectra of Colored Dissolved Organic Matter (CDOM). Comput. Geosciences 133, 104334. doi:10.1016/j.cageo.2019.104334
Ortiz, J. D., Avouris, D. M., Schiller, S. J., Luvall, J. C., Lekki, J. D., Tokars, R. P., et al. (2019). Evaluating Visible Derivative Spectroscopy by Varimax-Rotated, Principal Component Analysis of Aerial Hyperspectral Images from the Western Basin of Lake Erie. J. Great Lakes Res. 45, 522–535. doi:10.1016/j.jglr.2019.03.005
Pahlevan, N., Smith, B., Schalles, J., Binding, C., Cao, Z., Ma, R., et al. (2020). Seamless Retrievals of Chlorophyll-A from Sentinel-2 (MSI) and Sentinel-3 (OLCI) in Inland and Coastal Waters: A Machine-Learning Approach. Remote Sensing Environ. 240, 111604. doi:10.1016/j.rse.2019.111604
Palacios, S. L., Kudela, R. M., Guild, L. S., Negrey, K. H., Torres-Perez, J., and Broughton, J. (2015). Remote Sensing of Phytoplankton Functional Types in the Coastal Ocean from the HyspIRI Preparatory Flight Campaign. Remote Sensing Environ. 167, 269–280. doi:10.1016/j.rse.2015.05.014
Palmer, S. C., Kutser, T., and Hunter, P. D. (2015). Remote Sensing of Inland Waters: Challenges, Progress and Future Directions. Rem. Sens. Environ. 157, 1–8. doi:10.1016/j.rse.2014.09.021
Paoletti, M. E., Haut, J. M., Plaza, J., and Plaza, A. (2019). Deep Learning Classifiers for Hyperspectral Imaging: A Review. ISPRS J. Photogrammetry Remote Sensing 158, 279–317. doi:10.1016/j.isprsjprs.2019.09.006
Parsons, M., Bratanov, D., Gaston, K., and Gonzalez, F. (2018). UAVs, Hyperspectral Remote Sensing, and Machine Learning Revolutionizing Reef Monitoring. Sensors 18, 2026. doi:10.3390/s18072026
Pettit, E. (1936). On the Color of Crater Lake Water. Proc. Natl. Acad. Sci. 22, 139–146. doi:10.1073/pnas.22.2.139
Phinn, S. R., Roelfsema, C. M., and Mumby, P. J. (2012). Multi-scale, Object-Based Image Analysis for Mapping Geomorphic and Ecological Zones on Coral Reefs. Int. J. Remote Sensing 33, 3768–3797. doi:10.1080/01431161.2011.633122
Pietzsch, T., Saalfeld, S., Preibisch, S., and Tomancak, P. (2015). BigDataViewer: Visualization and Processing for Large Image Data Sets. Nat. Methods 12, 481–483. doi:10.1038/nmeth.3392
Plaza, A., Du, Q., Chang, Y.-L., and King, R. L. (2011). High Performance Computing for Hyperspectral Remote Sensing. IEEE J. Sel. Top. Appl. Earth Observations Remote Sensing 4, 528–544. doi:10.1109/JSTARS.2010.2095495
Polcyn, F. C., Brown, W. L., and Sattinger, I. J. (1970). The Measurement of Water Depth by Remote Sensing Techniques. Report 8973-26F. Ann Arbor, MI: Willow Run Laboratories, The University of Michigan.
Preisendorfer, R. W. (1976). Hydrologic Optics. Honolulu, Hawaii: U.S. Dept. of Commerce, National Oceanic and Atmospheric Administration.
Preisendorfer, R. W., and Mobley, C. D. (1988). Principal Component Analysis in Meteorology and Oceanography. Dev. Atmos. Sci. 17.
Pu, H., Liu, D., Qu, J.-H., and Sun, D.-W. (2017). Applications of Imaging Spectrometry in Inland Water Quality Monitoring—A Review of Recent Developments. Water Air Soil Pollut. 228, 131. doi:10.1007/s11270-017-3294-8
Qu, Z., Kindel, B. C., and Goetz, A. F. (2003). The High Accuracy Atmospheric Correction for Hyperspectral Data (HATCH) Model. IEEE Trans. Geosci. Remote Sensing 41, 1223–1231.
Raman, C. V. (1922). On the Molecular Scattering of Light in Water and the Colour of the Sea. Proc. R. Soc. Lond. Ser. A, Containing Pap. a Math. Phys. Character 101, 64–80. doi:10.1098/rspa.1922.0025
Rebelo, A. J., Somers, B., Esler, K. J., and Meire, P. (2018). Can Wetland Plant Functional Groups Be Spectrally Discriminated?. Remote Sensing Environ. 210, 25–34. doi:10.1016/j.rse.2018.02.031
Reinersman, P. N., and Carder, K. L. (1995). Monte Carlo Simulation of the Atmospheric Point-Spread Function with an Application to Correction for the Adjacency Effect. Appl. Opt. 34, 4453–4471. doi:10.1364/ao.34.004453
Rodgers, C. D. (1998). Information Content and Optimisation of High Spectral Resolution Remote Measurements. Adv. Space Res. 21, 361–367. doi:10.1016/s0273-1177(97)00915-0
Roelfsema, C., Kovacs, E., Roos, P., Terzano, D., Lyons, M., and Phinn, S. (2018). Use of a Semi-automated Object Based Analysis to Map Benthic Composition, Heron Reef, Southern Great Barrier Reef. Remote Sensing Lett. 9, 324–333. doi:10.1080/2150704x.2017.1420927
Rozanov, V.V., Dinter, T., Rozanov, A.V., Wolanin, A., Bracher, A., and Burrows, J. P. (2017). Radiative transfer modeling through terrestrial atmosphere and ocean accounting for inelastic processes: Software package SCIATRAN. J. Quant Spectrosc. Radiat. Transf. 194, 65–85. doi:10.1016/j.jqsrt.2017.03.009
Rozanov, V. V., Rozanov, A. V., Kokhanovsky, A. A., and Burrows, J. P. (2014). Radiative Transfer through Terrestrial Atmosphere and Ocean: Software Package SCIATRAN. J. Quantitative Spectrosc. Radiative Transfer 133, 13–71. doi:10.1016/j.jqsrt.2013.07.004
Russell, B. J., and Dierssen, H. M. (2015). Use of Hyperspectral Imagery to Assess Cryptic Color Matching in Sargassum Associated Crabs. PloS one 10. doi:10.1371/journal.pone.0136260
Ryan, J., Davis, C., Tufillaro, N., Kudela, R., and Gao, B.-C. (2014). Application of the Hyperspectral Imager for the Coastal Ocean to Phytoplankton Ecology Studies in Monterey Bay, CA, USA. Remote Sensing 6, 1007–1025. doi:10.3390/rs6021007
Ryan, J. P., Johnson, S. B., Sherman, A., Rajan, K., Py, F., Thomas, H., et al. (2010). Mobile Autonomous Process Sampling within Coastal Ocean Observing Systems. Limnol. Oceanogr. Methods 8, 394–402. doi:10.4319/lom.2010.8.394
Salcedo-Sanz, S., Cuadra, L., and Vermeij, M. J. A. (2016). A Review of Computational Intelligence Techniques in Coral Reef-Related Applications. Ecol. Inform. 32, 107–123. doi:10.1016/j.ecoinf.2016.01.008
Sandidge, J. C., and Holyer, R. J. (1998). Coastal Bathymetry from Hyperspectral Observations of Water Radiance. Remote Sensing Environ. 65, 341–352. doi:10.1016/s0034-4257(98)00043-1
Santer, R., and Schmechtig, C. (2000). Adjacency Effects on Water Surfaces: Primary Scattering Approximation and Sensitivity Study. Appl. Opt. 39, 361–375. doi:10.1364/AO.39.000361
Santos, M. J., Hestir, E. L., Khanna, S., and Ustin, S. L. (2012). Image Spectroscopy and Stable Isotopes Elucidate Functional Dissimilarity between Native and Nonnative Plant Species in the Aquatic Environment. New Phytol. 193, 683–695. doi:10.1111/j.1469-8137.2011.03955.x
Santos, M. J., Khanna, S., Hestir, E. L., Greenberg, J. A., and Ustin, S. L. (2016). Measuring Landscape-Scale Spread and Persistence of an Invaded Submerged Plant Community from Airborne Remote Sensing. Ecol. Appl. 26, 1733–1744. doi:10.1890/15-0615
Sathyendranath, S., Rao, D. V. S., Chen, Z., Stuart, V., Platt, T., Bugden, G. L., et al. (1997). Aircraft Remote Sensing of Toxic Phytoplankton Blooms: a Case Study from Cardigan River, Prince Edward Island. Can. J. remote sensing 23, 15–23. doi:10.1080/07038992.1997.10874674
Secretariat of the Convention on Biological Diversity (2020). Administrator of Global Biodiversity: SpringerLink. Available at: https://link.springer.com/article/10.1007/s10531-006-9043-8 (Accessed November 9, 2020).
Shang, S., Lee, Z., Lin, G., Hu, C., Shi, L., Zhang, Y., et al. (2017). Sensing an Intense Phytoplankton Bloom in the Western Taiwan Strait from Radiometric Measurements on a UAV. Remote Sensing Environ. 198, 85–94. doi:10.1016/j.rse.2017.05.036
Smith, M. E., and Bernard, S. (2020). Satellite Ocean Color Based Harmful Algal Bloom Indicators for Aquaculture Decision Support in the Southern Benguela. Front. Mar. Sci. 7, 61. doi:10.3389/fmars.2020.00061
Smith, V., Rogers, R., and Reed, L. (1975). Automated Mapping and Inventory of Great Barrier Reef Zonation with LANDSAT Data. OCEAN 75 Conference (New York, NY: IEEE), 775–780.
Spyrakos, E., O'Donnell, R., Hunter, P. D., Miller, C., Scott, M., Simis, S. G. H., et al. (2018). Optical Types of Inland and Coastal Waters. Limnol. Oceanogr. 63, 846–870. doi:10.1002/lno.10674
Stamnes, K., Li, W., Yan, B., Eide, H., Barnard, A., Pegau, W. S., et al. (2003). Accurate and Self-Consistent Ocean Color Algorithm: Simultaneous Retrieval of Aerosol Optical Properties and Chlorophyll Concentrations. Appl. Opt. 42, 939–951. doi:10.1364/ao.42.000939
Stamnes, S., Hostetler, C., Ferrare, R., Burton, S., Liu, X., Hair, J., et al. (2018). Simultaneous Polarimeter Retrievals of Microphysical Aerosol and Ocean Color Parameters from the "MAPP" Algorithm with Comparison to High-Spectral-Resolution Lidar Aerosol and Ocean Products. Appl. Opt. 57, 2394–2413. doi:10.1364/ao.57.002394
Steinmetz, F., Deschamps, P.-Y., and Ramon, D. (2011). Atmospheric Correction in Presence of Sun Glint: Application to MERIS. Opt. Express 19, 9783–9800. doi:10.1364/OE.19.009783
Sterckx, S., Knaeps, E., and Ruddick, K. (2011). Detection and Correction of Adjacency Effects in Hyperspectral Airborne Data of Coastal and Inland Waters: the Use of the Near Infrared Similarity Spectrum. Int. J. Remote Sensing 32, 6479–6505. doi:10.1080/01431161.2010.512930
Striebel, M., Behl, S., Diehl, S., and Stibor, H. (2009). Spectral Niche Complementarity and Carbon Dynamics in Pelagic Ecosystems. The Am. Naturalist 174, 141–147. doi:10.1086/599294
Strong, A. E. (1974). Remote Sensing of Algal Blooms by Aircraft and Satellite in Lake Erie and Utah Lake. Remote sensing Environ. 3, 99–107. doi:10.1016/0034-4257(74)90052-2
Szabó, G., Bertalan, L., Barkóczi, N., Kovács, Z., Burai, P., and Lénárt, C. (2018). “Zooming on Aerial Survey,” in Small Flying Drones (Berlin, Germany: Springer), 91–126. doi:10.1007/978-3-319-66577-1_4
Thamaga, K. H., and Dube, T. (2018). Remote Sensing of Invasive Water Hyacinth (Eichhornia crassipes): A Review on Applications and Challenges. Remote Sensing Appl. Soc. Environ. 10, 36–46. doi:10.1016/j.rsase.2018.02.005
Thompson, D. R., Cawse-Nicholson, K., Erickson, Z., Fichot, C. G., Frankenberg, C., Gao, B.-C., et al. (2019). A Unified Approach to Estimate Land and Water Reflectances with Uncertainties for Coastal Imaging Spectroscopy. Remote Sensing Environ. 231, 111198. doi:10.1016/j.rse.2019.05.017
Thompson, D. R., Gao, B.-C., Green, R. O., Roberts, D. A., Dennison, P. E., and Lundeen, S. R. (2015). Atmospheric Correction for Global Mapping Spectroscopy: ATREM Advances for the HyspIRI Preparatory Campaign. Remote Sensing Environ. 167, 64–77. doi:10.1016/j.rse.2015.02.010
Tong, Q., Zheng, L., Xue, Y., Zhang, B., Zhao, Y., and Liu, L. (2001). “Hyperspectral Remote Sensing in China,” in Multispectral and Hyperspectral Image Acquisition and Processing (Washington, DC: International Society for Optics and Photonics), 1–9.
Torrecilla, E., Stramski, D., Reynolds, R. A., Millán-Núñez, E., and Piera, J. (2011). Cluster Analysis of Hyperspectral Optical Data for Discriminating Phytoplankton Pigment Assemblages in the Open Ocean. Remote Sensing Environ. 115, 2578–2593. doi:10.1016/j.rse.2011.05.014
Twardowski, M., and Tonizzo, A. (2018). Ocean Color Analytical Model Explicitly Dependent on the Volume Scattering Function. Appl. Sci. 8, 2684. doi:10.3390/app8122684
Tyler, A. N., Hunter, P. D., Spyrakos, E., Groom, S., Constantinescu, A. M., and Kitchen, J. (2016). Developments in Earth Observation for the Assessment and Monitoring of Inland, Transitional, Coastal and Shelf-Sea Waters. Sci. Total Environ. 572, 1307–1321. doi:10.1016/j.scitotenv.2016.01.020
Tyler, J. E. (1970). Expedition Report, SCOR Working Group 15, Discoverer Expedition. San Diego, CA: Scripps Institution of Oceanography.
Uitz, J., Stramski, D., Reynolds, R. A., and Dubranna, J. (2015). Assessing Phytoplankton Community Composition from Hyperspectral Measurements of Phytoplankton Absorption Coefficient and Remote-Sensing Reflectance in Open-Ocean Environments. Remote Sensing Environ. 171, 58–74. doi:10.1016/j.rse.2015.09.027
Vandermeulen, R. A., Mannino, A., Craig, S. E., and Werdell, P. J. (2020). 150 Shades of Green: Using the Full Spectrum of Remote Sensing Reflectance to Elucidate Color Shifts in the Ocean. Remote Sensing Environ. 247, 111900. doi:10.1016/j.rse.2020.111900
Vandermeulen, R. A., Mannino, A., Neeley, A., Werdell, J., and Arnone, R. (2017). Determining the Optimal Spectral Sampling Frequency and Uncertainty Thresholds for Hyperspectral Remote Sensing of Ocean Color. Opt. Express 25, A785–A797. doi:10.1364/oe.25.00a785
Vanhellemont, Q. (2019). Adaptation of the Dark Spectrum Fitting Atmospheric Correction for Aquatic Applications of the Landsat and Sentinel-2 Archives. Remote Sensing Environ. 225, 175–192. doi:10.1016/j.rse.2019.03.010
Velez-Reyes, M., rosario-torres, S., and Jimenez-Rodriguez, L. (2015). The MATLAB Hyperspectral Image Analysis Toolbox. doi:10.13140/RG.2.1.1220.7203
Voss, K. J., Johnson, C. B., Yarbrough, M. A., Gleason, A., Flora, S. J., Feinholz, M. E., et al. (2017). An Overview of the Marine Optical Buoy (MOBY): Past, Present and Future. Proceedings of the D-240 FRM4SOC-PROC1 Proceedings of WKP-1 (PROC-1) Fiducial Reference Measurements for Satellite Ocean Colour (FRM4SOC). Tartu, Estonia, 8–13.
Wang, P. (2004). A Method to Quantify the Uncertainties Associated with Semi-analytic Algorithm for Inversion of Ocean Color. Available at: https://digitalcommons.library.umaine.edu/etd/1560 (Accessed November 10, 2020).
Werdell, P. J., Franz, B. A., Bailey, S. W., Feldman, G. C., Boss, E., Brando, V. E., et al. (2013). Generalized Ocean Color Inversion Model for Retrieving Marine Inherent Optical Properties. Appl. Opt. 52, 2019. doi:10.1364/AO.52.002019
Werdell, P. J., McKinna, L. I. W., Boss, E., Ackleson, S. G., Craig, S. E., Gregg, W. W., et al. (2018). An Overview of Approaches and Challenges for Retrieving Marine Inherent Optical Properties from Ocean Color Remote Sensing. Prog. oceanography 160, 186–212. doi:10.1016/j.pocean.2018.01.001
Wernand, M. R. (2011). Poseidon’s Paintbox: Historical Archives of Ocean Colour in Global-Change Perspective. PhDT.
Wernand, M. R., van der Woerd, H. J., and Gieskes, W. W. (2013). Trends in Ocean Colour and Chlorophyll Concentration from 1889 to 2000, Worldwide. Available at: http://dx.plos.org/10.1371/journal.pone.0063766 (Accessed December 7, 2015).
Wester-Ebbinghaus, W. (1980). Aerial Photography by Radio Controlled Model Helicopter. Photogrammetric Rec. 10, 85–92.
Wilson, A., Lindholm, D. M., Coddington, O., Pilewskie, P., and Kent, J. (2018). Hylatis, a Cloud-Based Hyperspectral Image Analysis Toolkit, 51. AGU Fall Meeting AbstractsAvailable at: http://adsabs.harvard.edu/abs/2018AGUFMIN51B0579W (Accessed December 10, 2020).
Wolanin, A., Soppa, M., and Bracher, A. (2016). Investigation of Spectral Band Requirements for Improving Retrievals of Phytoplankton Functional Types. Remote Sensing 8, 871. doi:10.3390/rs8100871
Wu, D., Li, R., Zhang, F., and Liu, J. (2019). A Review on Drone-Based Harmful Algae Blooms Monitoring. Environ. Monit. Assess. 191, 211. doi:10.1007/s10661-019-7365-8
Wulder, M. A., White, J. C., Loveland, T. R., Woodcock, C. E., Belward, A. S., Cohen, W. B., et al. (2016). The Global Landsat Archive: Status, Consolidation, and Direction. Remote Sensing Environ. 185, 271–283. doi:10.1016/j.rse.2015.11.032
Xu, F., Gao, Z., Jiang, X., Ning, J., Zheng, X., Song, D., et al. (2017). “Mapping of Green Tide Using True Color Aerial Photographs Taken from a Unmanned Aerial Vehicle,” in Remote Sensing and Modeling of Ecosystems for Sustainability XIV (Washington, DC: International Society for Optics and Photonics). doi:10.1117/12.2271732
Ye, H., Li, J., Li, T., Shen, Q., Zhu, J., Wang, X., et al. (2016). Spectral Classification of the Yellow Sea and Implications for Coastal Ocean Color Remote Sensing. Remote Sensing 8, 321. doi:10.3390/rs8040321
Yunus, A. P., Dou, J., Song, X., and Avtar, R. (2019). Improved Bathymetric Mapping of Coastal and Lake Environments Using Sentinel-2 and Landsat-8 Images. Sensors 19, 2788. doi:10.3390/s19122788
Zaneveld, J. R. V. (1995). A Theoretical Derivation of the Dependence of the Remotely Sensed Reflectance of the Ocean on the Inherent Optical Properties. J. Geophys. Res. 100 (13), 135–213. doi:10.1029/95jc00453
Zeng, C., Richardson, M., and King, D. J. (2017). The Impacts of Environmental Variables on Water Reflectance Measured Using a Lightweight Unmanned Aerial Vehicle (UAV)-based Spectrometer System. ISPRS J. Photogrammetry Remote Sensing 130, 217–230. doi:10.1016/j.isprsjprs.2017.06.004
Zhao, J., and Ghedira, H. (2014). Monitoring Red Tide with Satellite Imagery and Numerical Models: A Case Study in the Arabian Gulf. Mar. Pollut. Bull. 79, 305–313. doi:10.1016/j.marpolbul.2013.10.057
Keywords: imaging spectroscopy, aquatic optics, surface biology and geology (SBG), plankton aerosol cloud and ocean ecosystem (PACE), Unoccupied Aerial Vehicles (UAV)
Citation: Dierssen HM, Ackleson SG, Joyce KE, Hestir EL, Castagna A, Lavender S and McManus MA (2021) Living up to the Hype of Hyperspectral Aquatic Remote Sensing: Science, Resources and Outlook. Front. Environ. Sci. 9:649528. doi: 10.3389/fenvs.2021.649528
Received: 04 January 2021; Accepted: 14 April 2021;
Published: 07 June 2021.
Edited by:
Xuan Zhu, Monash University, AustraliaReviewed by:
Dimitris Poursanidis, Foundation for Research and Technology Hellas (FORTH), GreeceCédric Jamet, UMR8187 Laboratoire d'océanologie et de géosciences (LOG), France
Copyright © 2021 Dierssen, Ackleson, Joyce, Hestir, Castagna, Lavender and McManus. This is an open-access article distributed under the terms of the Creative Commons Attribution License (CC BY). The use, distribution or reproduction in other forums is permitted, provided the original author(s) and the copyright owner(s) are credited and that the original publication in this journal is cited, in accordance with accepted academic practice. No use, distribution or reproduction is permitted which does not comply with these terms.
*Correspondence: Heidi M. Dierssen, aGVpZGkuZGllcnNzZW5AdWNvbm4uZWR1